- Chair of Traffic Engineering and Control, Technical University (TU) Munich, Munich, Germany
Economic instruments are considered promising to achieve the urgently needed reduction of greenhouse gas emissions in the transportation sector. In this context, tradable credit schemes have received more and more attention in recent years. These cap-and-trade systems have the primary goal of limiting congestion, but they can also incorporate emission reduction goals. In this paper, we present the conceptual extension of a tradable credit scheme from a congestion and emission-oriented to a holistic, full-trip, multi-modal mobility traffic management system. In addition to the demand it also includes the management of the supply side. The integration of all existing modes into one holistic scheme ensures that the overall system goals are reached as all behavioral responses remain within the system boundaries. The system comprises two key innovations. First, a central agency has the possibility to provide full-trip incentives across modes to support the overall system’s goal. Second, users of the system can spend parts of their allocated mobility budget for transport infrastructure upgrades as an addition to paying for mobility or monetizing it on the market. Those innovations are a distinct extension to the idea of tradable credits. Commonly used smartphones would serve as the enabling technology of the proposed system. They offer all technical requirements and almost every citizen has access to one. Smartphones are affordable compared to dedicated traffic management infrastructure and they are flexible to accommodate system changes, e.g., new modes, through software updates. Besides the potential technical implementation, overall design questions, social aspects as well as general implications of the concept are covered.
1 Introduction
Problems of limited space and greenhouse gas (GHG) emission levels in the transportation sector are well perceptible in urban areas worldwide, impacting the environment and human well-being. Extended travel times due to congestion further raise emission levels and increase travelers’ dissatisfaction. So far, providing more infrastructure may only temporarily solve the congestion problem due to induced demand and population growth, while it could be infeasible without available space. The industry is addressing some of these issues through innovation, e.g., shifting from internal combustion engines to less carbon-intensive engines. This may reduce GHG emissions—the space consumption of the vehicles and congestion problems remain unchanged. Further urbanization and ongoing demographic change intensify the circumstances for the limited urban space. Economists meanwhile have had only limited success in promoting effective economic transportation and environmental externality measures (Lindsey and Santos, 2020). In addition, the shift away from fossil fuels leads to a lack of energy tax revenue, which reduces the public budget commonly used for funding the transportation infrastructure. Therefore, there is a need for a policy instrument, based on the costs-by-cause principle, that is simultaneously capable of managing traffic and internalizing the resulting externalities while closing the funding gap for transport infrastructure (Pigou and Aslanbeigui, 2017). Unlike various incremental measures that have been implemented and could not lead to the desired results so far, the herein described cap-and-trade policy instrument is tailored to the mobility sector. It is an all-encompassing approach, affecting mobility supply and demand side across all available modes.
Based on the idea of a tradable credit scheme, first introduced by Verhoef et al. (1997), we propose an extended generic full-trip policy instrument called MobilityCoins. Every user receives a credit budget at the beginning of each period which can be used in three major ways: Mobility (demand), market (trading) and crowdfunding (supply). First, for mobility, credits can be used across the entire trip, starting with the planning of the route and mode via the choice of on-trip amenities until completing the journey with post-trip options for parking. Second, they can also be traded among users of the system. The limited supply of credits creates a market price which acts as an economic incentive encouraging the use of sustainable, lower charged transportation modes. Once users run out of credits, they have the choice to buy additional credits on the market, while users with excess credits can monetize them. Third, credits can also be invested in supply-side crowdfunding measures. Those measures are offered by the agency to improve the travelers’ generalized cost of travel as an alternative to generate monetary income, e.g., free flow speed improvements or reallocation of space. The latter further enables users to actively take part in the design of the supply-side, which may increase the public’s support for such a policy instrument (Bao et al., 2019; Krabbenborg et al., 2020). The idea of crowdfunding public infrastructure is not new and already present in the sustainable energy sector (Lam and Law, 2016; Vasileiadou et al., 2016). It has also been reported a few times in transport, e.g., public transport (crowdsourced transport, 2022) or for bicycle infrastructure (NEXTCITY, 2014), but as yet it remains a niche. Public acceptability of carbon pricing can be improved through a tangible application and proper utilization of the revenues raised, e.g., for the crowdfunding of infrastructure (Klenert et al., 2018). Those extended features differentiate this concept from the idea of tradable credits and brings it closer to an all-encompassing currency in the mobility system. It is capable of reallocating funds within the system boundaries without loopholes. We define it as the MobilityCoin System.
Technological developments like smartphones and seamless cellular network coverage facilitate the implementation of such a system. Ultimately, smartphone applications evolve transportation from the simple purpose of moving a traveler from origin to destination into an user-centric, overarching system that connects modes, services and technologies in order to offer the best service for users (Kocher, 2015).
Following a summary of relevant, preliminary works, we introduce the policy instrument’s main features in this paper and illustrate its fundamental mechanisms.
2 Literature review
This section provides essential scientific groundwork underlying the MobilityCoin System. Beginning with the fundamental idea of tradable credit schemes, followed by an assessment on transport policies and a comprehensive examination of smartphone applications in mobility.
2.1 Tradable credit schemes
The proposed MobilityCoin policy instrument is a tradable credit schemes (TCS): A cap-and-trade system for mobility. TCS are adaptable to a certain degree and can be designed to achieve different effects. After a brief introduction, we explain several scopes of TCS for the most common applications.
2.1.1 The general idea of TCS
First considerations for TCS go back to the seminal work by Coase (1960) and Dales (1968) on managing public resources. Verhoef et al. (1997) were the first to propose the idea of TCS in road traffic management. Generally, a distinction can be made between TCS and mobility permit schemes. The former means that eligible users obtain an initial credit budget out of which they pay the charges for any of their trips (e.g., Grant-Muller and Xu, 2014). The latter means that travelers have to bid for or buy the required permits for a specific link (e.g., a bottleneck) within a certain time period (e.g., Wada and Akamatsu, 2013). Goddard (1997) was one of the first using tradable permits to control vehicle emissions, congestion and urban decentralization and Yang and Wang (2011) were the first to algebraically express TCS in small transportation networks. In recent years, different approaches came up with various characteristics in terms of credit allocation, validity or heterogeneity of users, and application to different kind of networks. Most of the existing schemes consider a small period of expiration when some allow banking: the transfer of remaining credits to the subsequent period. The initial allocation, the period of validity, and the possibility of banking are crucial factors for a successful system design as it influences market dynamics and usage of credits (Ellerman and Joskow, 2008; Miralinaghi and Peeta, 2016). In theory, TCS proved successful in achieving a congestion reduction goal (Yang and Wang, 2011; de Palma et al., 2018), and could support in meeting climate change targets (Musso and Rothengatter, 2013). Nevertheless, Krabbenborg et al. (2021) note that a TCS for mobility is still far from applying to our current mobility system, while at the same time describing it as a promising (theoretical) instrument (Lindsey and Santos, 2020). Incorporating the transportation supply side, Wang et al. (2014) applies a TCS with improvement of road capacity and Wang et al. (2020) combined a TCS and link capacity improvement measures in a bi-objective bi-level model to balance economic growth and environmental management.
2.1.2 TCS for congestion
Demand management is widely accepted as an essential component to resolve traffic congestion and, when correctly applied, it can be effective and efficient (Vickrey, 1955). Based on the work of Pigou (1920), congestion pricing became one of the most applied measure in demand management. Economists claim that congestion occurs because a road user solely considers the own marginal costs but does not take the effect on others into account. As a result, the marginal social costs outweigh the marginal private costs. A congestion toll can increase private marginal costs and accordingly social welfare by reducing the gap to social marginal costs (de Palma and Lindsey, 2011). Several demand management schemes were developed in attempt to enhance equity and effectiveness. In this paper, we focus on cap-and-trade systems. Yang and Wang (2011) were the first to describe the user and market equilibria mathematically by formulating a variational inequality problem with homogeneous and heterogeneous users (Wang et al., 2012). Xiao et al. (2013) reviewed the case of commuting with heterogeneous users and Grant-Muller and Xu (2014) made a comparison to see if a TCS could have advantages over other instruments. de Palma et al. (2018) examined whether congestion pricing schemes (dollars) or quantity based mobility schemes (tokens) are more preferable in terms of social welfare and equity. For a dynamic case, Bao et al. (2019) showed that the equilibrium may not be unique for particular models of congestion, e.g., the first-best solution for the conventional Vickrey’s bottleneck model. Liu et al. (2020) used a the trip-based Macroscopic Fundamental Diagram (MFD) model to show the efficiency and effectiveness of TCS (for the morning commute). Policy applications of tradable permits and credits for managing congestion are evaluated by Raux (2004). Verhoef et al. (1997) identified applications of permits usage to manage transport externalities. Kockelman and Kalmanje (2005) states that acceptance of the system is strongly related to the knowledge about the system. The social, political, economic and technical feasibility of TCS for congestion management is analyzed by Krabbenborg et al. (2021). Seilabi et al. (2020) assessed travel demand management for an autonomous vehicle enabled TCS and lane management strategies to minimize total travel time under user equity constraints.
2.1.3 TCS for emissions
Every TCS system is designed around one or many objectives from which the overall allocation and the mobility pricing are determined; this is not limited to congestion. The system can also be arranged to effect emission externalities in order to achieve greenhouse gas (GHG) reduction. Nagurney (2000) introduced market-based implementations for emissions standard attainment proposing origin-destination based pollution permits. Abdul Aziz and Ukkusuri (2013) worked on a TCS system that redistributes link flow patterns to obtain minimum emissions for the whole network, and extend it to bi-objectives (low emissions and low travel times). Miralinaghi et al. (2019) considered a vehicle type specific and OD-based credit allocation in a multi-period TCS framework. Moreover, they proposed a charging system that is based on the vehicle type (zero-emission vehicle versus internal combustion engine vehicles) and the links travelers are using according to their vehicle type. The latter work incentivizes zero-emission vehicle usage, while the former redistributes flows to reach a bi-level objective of minimum emissions and minimum travel time.
2.1.4 TCS for parking
Zhang et al. (2011) studied the travel efficiency by parking permits distribution and trading, comparing three scheme variants in respect of uniform, Pareto improving, and optimum distribution of parking permits to commuters. Recently, Bao and Ng (2022) suggested the idea of tradable parking permits for demand management in cities with freedom of mode choice. Here, a tradable parking permit scheme works in a similar fashion as for traffic management; the tradable parking permit scheme functions by setting a constraint on the total number of car park places in a specified area and time period. Parking permits are also initially endowed to eligible participants and redeemed when parking a car. Through trading on a market, the permits are allocated to the user with the highest value of car use. Ultimately, on the one hand, the scheme economically attracts the reduction of aggregated costs of all users, on the other hand sets incentives to reduce car travel or one step further car ownership. Bao and Ng (2022) state that tradable parking permit schemes are feasible and efficient with regard to reference dependence and endowment effect. Environmental consciousness can further improve the effectiveness by shifting to public transport.
2.2 Review on transport policies
Policies in transport can be grouped according to pre-trip, on-trip or post-trip measures, depending on which element of a trip they are mainly affecting. In this section, existing and relevant policy instruments that generally support the idea of or can be linked to a tradable credit scheme are summarized. Those instruments include technology and construction specifications, financial incentives and (technical) control procedures.
2.2.1 Pre-trip: Mode-choice, route-choice and travel-time-choice
The pre-trip decision is about mode-choice, route-choice and departure-time-choice, depends on a variety of factors and is quite complex in terms of understanding the decision-making process behind. In literature, individual characteristics of socioeconomic status are described that influence mode-choice, like gender, age, household size, presence of school children, flexible work hours, level of education, occupation or household income level. Other characteristics are more related to travel conditions and trip characteristics: home location, the influence of weather and habits of using a specific route, the usual duration of daily congestion, the frequency of traffic congestion during the week, travel time and the frequency of late arrival at work due to congestion (De Palma and Rochat, 2000).
The matter of Travel Demand Management (TDM) can be located in this phase of the decision-making process. TDM covers both, the implementation of strategies to either reduce travel demand or redistribute this demand temporally or spatially (Nelson, 2000). TDM covers the plain provision of travel information or options to rather strict measures to force behavior change. Generally, strategies can be categorized as “soft” and “hard” measures (Hu et al., 2015). Measures that use charges to compel travel behavior changes are called hard policy measures and usually include toll roads, congestion and parking pricing which are explained later in this section (Friman et al., 2013). “Soft” strategies influence travelers’ decision by offering traffic information like incidents, weather or road conditions, optimal routes, recommended speeds, and lane restrictions through television, radio, computer or smartphone. Those systems are consolidated advanced traveller information systems (ATIS). They intend to reduce unnecessary trips or use transit instead, encouraging working from home and online conferencing, or encouraging carpooling respectively ridesharing (Möser and Bamberg, 2008). The MobilityCoin System incorporates elements of both areas, providing pre-trip traffic information supported by an incentive mechanism.
In order to spread traffic concentration at peak travel times and avoid consequences like congestion as well as high fuel consumption, the understanding of commuter travel time decisions including underlying factors have been studied and modelled in the past. First studies go back to Small (1982) and Abkowitz (1981). The research of departure time choice is quite complicated due to the high number of influencing factors. Broadly speaking, the model is coming from the simplified assumption that travelers have a preferred arrival time and that they set their departure time under consideration of travel time and the consequences of being early or rather late. Numerous conclusions were derived based on departure time model results and related analyses, stating that “work schedule flexibility, mode, occupation, income, age, and transportation level of service” influence departure time choice (Abkowitz, 1981). Results were carried into field studies like the Metropia study in Los Angeles in an area with occurrence of major traffic congestion. An incentive-based active demand management system was implemented, targeting car use of commuters. Contributing to the prediction of future traffic conditions, Metropia suggests time-dependent shortest paths with a routing algorithm for different departure times. These results are aligned with user requests and result in the relevant departure times and route choices for the user. Each of these choices are linked with a rating scheme, so that travelling during off-peak times and choosing less congested routes is rewarded with more points, and vice versa (Hu et al., 2015). The goal of the MobilityCoin’s incentive framework corresponds to said active demand management system: improving traffic flow, decreasing commuter travel times, and reducing congested areas without additional infrastructure by changing travel behavior due to incentives.
2.2.1.1 Regulation, subsidy, rebates and taxes
Regulation of vehicle technology accelerates changes in mobility tool ownership towards greener technologies. CO2 emission performance standards for passenger cars and commercial vehicles are constantly aligned with increased climate ambitions, for instance due to the European Union CO2 emissions regulation. Policies to promote electric vehicle deployment came into force. Broad fiscal incentives accelerated the development of electric vehicles (EV) and substantiated the scale up in EV manufacturing. In order to reduce price gaps with conventional cars, purchase subsidies and vehicle purchase or registration tax rebates were designed (Zhang et al., 2020). Such measures were firstly implemented in Norway in the 1990s (Lévay et al., 2017). Additionally, fuel taxes can actuate the shift to sustainable modes. Policy attention is brought to other transport modes as well, including commercial vehicles and buses since their share in emissions is not negligible. In early 2020, Germany, France and Italy increased purchase incentives. Governments announced more radical measures like prohibitions of internal combustion engines and economy wide net-zero ambitions.
2.2.1.2 Road tolling
Since 1990, Singapore started to control the growth-rate of licensed motor vehicles with a purchase-based vehicle quota system (VQS). As the first of its kind, the number of new allowed motor vehicles were fixed for each year. Buyers could bet on allowances via a sealed bid uniform price auction. In 2009, a usage-based road toll collection system was implemented complementary. The Singapore example shows that traffic response to changing charges is significant and higher for cars than for other vehicles and higher for expressways than for the city centre (Olszewski and Xie, 2005). In London, a per day cordon toll/congestion charge is collected since 2003 to reduce congestion and environmental pollution, but also to raise revenue for investment in London’s transportation infrastructure (Prud’Homme and Bocarejo, 2005; Leape, 2006). The Stockholm congestion tax is a congestion pricing system for vehicles entering and exiting central Stockholm which was introduced in 2007. The purpose of the congestion charge is both, the mitigation of traffic congestion and environmental improvements. The collected funds are used for infrastructure constructions and maintenance in the Stockholm area.
2.2.1.3 Environmental/low emission zones
Many metropolitan areas exceed air quality limit values for e.g., nitrogen dioxide (NO2) and/or particulate matter. In order to reduce those emissions, Low Emission Zones (LEZ) have been established in various cities or metropolitan areas globally, approximately 200 in the European Union alone. LEZs are delimited areas from which vehicles are banned that produce to many pollutants and do not meet specific emissions standards, typically older vehicles. The restriction varies considerably in legislation and scope. For instance, some cover all vehicles including passenger cars while others are narrowed to heavy-duty and light-duty vehicles. And yet others distinguish between inner and outer LEZ (Greece). While getting the advantage to fit the LEZ to local air quality needs, it has the potential to make a journey through more cities complicated without obtaining information prior to the trip. Furthermore, the number of urban deliveries are supposed to be reduced due to LEZ as well (Dablanc and Montenon, 2015). The results regarding efficacy of LEZ vary largely. There is some evidence from ambient measurements in Germany, that restricting passenger cars and heavy-duty vehicles lead to long term reductions in particulate matter and nitrogen dioxide concentrations. In other areas, where restrictions are bounded to heavy-duty vehicle, the results are less conclusive. Given the many underlying factors, e.g., meteorological impacts or other at the same time introduced policies, identifying the contribution of LEZ is very challenging (Holman et al., 2015).
2.2.2 On-trip: Route-adjustments, travel behavior adjustments and level of urgency
Once the mode, route and time of the trip is decided, the users switch into the on-trip feature section. The on-trip category covers the decision of route-adjustments and level of urgency. There is a vast variety of existing instruments influencing this sector by now. Managed lanes are separated from the general lanes setting definite rules by implementing operational strategies in reaction to changing conditions (Obenberger, 2004). Managed lanes can be classified into pricing (value priced lanes and toll lanes), vehicle eligibility (high-occupancy vehicle (HOV) lanes, bus or truck lanes), or access control/dynamic (express lanes or reversible lanes) that provide more inbound or outbound lines according to traffic. Intelligent traffic systems are advanced traffic control systems like intelligent traffic lights that use traffic data collected at intersections and future traffic information provided by agencies in order to dynamically adjust, maximizing traffic flow through an intersection. Variable speed limits is another kind of control system. Those systems are used to reduce traffic density in congested areas by adapting speed limits according to weather or road conditions. All of these systems can be incorporated into the MobilityCoin framework in some way, e.g., by getting access to express lanes for a surcharge or receiving a rebate for using HOV lanes.
2.2.3 Post-trip: Conclusion-decision and/or follow-on decision
The last step in the travellers decision process includes the conclusion of the trip and/or hypothetical follow-on decisions. In case the user did not arrive at the desired location, the decision making process loops back to the pre-trip decision to continue the journey until the trip eventually ends. The conclusion of the trip can be simple for some modes by arriving at the desired location without the need to park the means of transport. However, others need to be parked or garaged in some way, consuming space even when not in use. Private cars, commercial vehicles, but also shared vehicles like e-scooters, bike-sharing or car-sharing consume a considerable amount of space (Drut, 2018). Therefore, parking pricing is used to let motorists directly pay for occupying parking facilities. Parking pricing can be advanced to parking management strategy in order to reduce parking problems, to finance parking facility costs, or to generate revenue for different purposes. Nevertheless, most pricing is not streamlined since fees are flat and thus not optimized regarding parking facility use. Performance-based pricing addresses this topic (Mackowski et al., 2015). Furthermore, for shared vehicles, reallocation of shared vehicles can be taken into account in the pricing mechanism, suggesting the termination of trips in areas with a lack of vehicle capacities for discount rates. By now, smart parking apps make information about parking availability, costs, and payment types available. Following Shaheen et al. (2016) those apps can be categorized in e-Parking and e-Valet apps. E-Parking includes the involvement technologies to improve the parking process while e-Valet incorporates for-hire parking services.
2.3 Smartphone based mobility
When smartphones became more affordable throughout the late 2000s, their impact on travel behavior has been studied (Work and Bayen, 2008). First use cases were derived with the use of contextual information, e.g., real-time information for traffic and public transport journey information, which now is considered to make a substantial difference in route and mode choice. Furthermore, users can compare travel times, travel costs, health benefits and carbon footprint of travel alternatives. Underlying behavior change support systems (BCSS)—originally developed for health applications and then adapted for travel behavior—can enhance mode-shift. Studies in Boston and San Francisco showed that people are more likely to use public transport when they have access to devices to manage and plan their trips (Barry, 2011). However, persuasive features such as tunneling, tailoring, rehearsal and social facilitation are not sufficiently represented in travel behavior change yet (Sunio and Schmöcker, 2017). Andersson et al. (2018) revealed further essential factors for creating persuasive tools to stimulate the use of more environmentally friendly modes. They name customization to the user, commitment, relevant and contextualized information and appealing design as important aspects to drive behavior change through smartphone applications. A prominent development in recent years is GoEco!, a smartphone application that includes mobility tracking, eco-feedback, social comparison and gamification elements to encourage travelers’ mode-shift (Bucher et al., 2016). Cellina et al. (2019) noticed varying effectiveness for different areas: A decrease in CO2 emissions and energy consumption per kilometer for systematic home-to-home loops in highly car-dependent urban areas, but no statistically significant results in areas with high quality public transport. Generally, existing apps can be grouped into at least three categories that we discuss in the following: Journey Information Apps (Section 2.3.1), Mobility Services Apps (Section 2.3.2), Payment and Ticketing Apps (Section 2.3.3).
2.3.1 Journey information apps
There is evidence from several studies that Journey Information Apps and Routing Apps in particular can influence travel and mode-choice behavior. Guin et al. (2021) showed that navigation apps have a considerable effect on routing. Within their sample the travelers followed a suggested route for 80–99% of trips while 25% of the users followed the suggested route for 100% of trips. For the multi-modal case, Shaheen et al. (2017) reported that 39% of their sample drove less or much less due to the apps. They conclude that app users change their travel behavior across modes due to provided information and that apps can contribute to reduce the number of cars on the streets and therefore mitigating congestion. Some routing apps focus on different externalities like physical injuries by offering routing for safe walking based on smartphone sensors integration and fuzzy logic strategy Lozano Domínguez and Mateo Sanguino (2021).
2.3.2 Mobility services apps
Mobility Services Apps assist travelers in planning and understanding their mobility choices by providing information and/or improving access to alternative modes. Mobility Services Apps can be subdivided in the following categories according to the incorporated user groups, covered modes and major purposes: 1) Business-to-Consumer (B2C) Sharing Apps (e.g., SHARE NOW), 2) Peer-to-Peer (P2P) Sharing Apps (e.g., Drivy), 3) Public Transit Apps (e.g., MVV Munich), 4) Ridesourcing Apps (e.g., BlaBlaCar), 5) Taxi e-Hail Apps (e.g., MyTaxi), 6) Trip Aggregator Apps (e.g., Transit App), 7) Real-Time Information Apps (e.g., Öffi), and 8) Mobility Trackers (e.g., GPS Tracker Pro) FHWA (2016).
A special and very important category of apps are Smart Parking Apps. They are not for mobility per se, but are relevant to conclude a trip by car. Information about parking fee, payment options, and availability are all available through smart parking apps, called eParking services (Shaheen and Kemmerer, 2008). A similar service is eValet that offers typical valet services via apps, e.g., pick-up, park and return or further amenities like fueling or cleaning (FHWA, 2016).
2.3.3 Smart payment and ticketing apps
A third category of smartphone apps are Smart Payment and Ticketing Apps. They can be included in the previous two types as well. Around the world, various apps have emerged that offer such services, consequently underlining the digital transformation of the mobility system. For example, apps allow cities to establish dynamic road pricing schemes without traditional roadside infrastructure (e.g., Parker, 2019; ClearRoad, 2022; Yunex, 2022), which have already been used in experimental setups (Molloy, 2021).
For public transport, Mesoraca and Brakewood (2018) summarized mobile ticketing in 14 cities in the United States regarding the ticket validation process, ticket types offered in the mobile app, additional features in the app and the process for transferring to other modes using the app. Wirtz and Klähr (2019) described a smartphone based ticketing system using a check-in/check-out technology that enables travelers to use public transport and get charged the lowest fare based on their journey trajectory. A data-driven solution where user-generated data are collected to extract information on the share of each used transport service for revenue-sharing between service provider is presented by Tuveri et al. (2022). However, there is a large variety of apps and each serve their own purpose. Single point of contact apps including trip planning, trip information and multi-modal payment mechanisms are still lacking. As a response, the Swiss government put a regulation into place that should enforce cooperation between such Mobility Pricing apps (UVEK, 2018).
To recap, in Section 2 we showed the theoretical foundation and past research structured in three segments. With TCS, a cap-and-trade system for mobility, we described the underlying scheme of the MobilityCoin System. Policy instruments that resemble the mechanisms of the system are dealt with subsequently. We conclude this section with a broad overview about smartphone based mobility solutions, since smartphones could serve as the enabling technology and the functionalities of those solutions partially are alike some of the system’s features. All relevant fields have now been identified in order to introduce the MobilityCoin System.
3 The MobilityCoin System
The MobilityCoin is a new comprehensive system for the management of multi-modal urban transportation. This concept is a full-trip tradable-credit-scheme that aims to optimize the mobility supply and demand side for metropolitan areas (Bogenberger et al., 2021). It can integrate all transport policies discussed in Section 2.2, making it a holistic instrument for the transportation system. The full-trip feature of the MobilityCoin System includes pre-trip, on-trip and post-trip elements. Figure 1 illustrates how MobilityCoins are used along the full trip chain. The two key innovations of the MobilityCoin System are the possibility of the central agency to provide full-trip incentives across modes to support the overall system’s objective as well as the possibility to use parts of the allocated mobility budget for transport infrastructure upgrades instead of using them for mobility or by monetizing it. Precisely those innovations differentiate the MobilityCoin System from the original idea of tradable credits, which is shown in Figure 1.
In this section, we introduce the three major building blocks of the MobilityCoin System in Section 3.1. Then, we discuss in Section 3.2 options on designing and allocating the initial budget in the MobilityCoin System, borrowing insights from other tradable credit schemes. In Section 3.3, we further discuss possibilities to discover the core parameters of a successful MobilityCoin System in practice.
3.1 Three building blocks of the MobilityCoin System
We consider the MobilityCoin System as a user-centric system. From user’s perspective, the MobilityCoin System consists of three major building blocks, shown in Figure 2. 1) The mobility side including the pricing mechanism which is one of the major levers to influence the user’s mode-choice, route-choice and departure-time-choice. 2) The MobilityCoin market in which coins can be traded and revenue generated with transaction fees and taxes, and lastly 3) the investment can directly impact the mobility supply side with the implementation of spatial or infrastructure measures proposed by the agency and financed through system-generated revenue.
3.1.1 Mobility (demand/pricing)
The MobilityCoin System is a full-trip scheme. All parts of the trip, beginning with the pre-trip mode and route decision, followed by on-trip capabilities and post-trip options to conclude the trip, are based on one currency. In the following section we describe each of those three trip phases in more depths.
As described in Section 3.2, all users of the system receive a MobilityCoin budget. The users’ decision-making process starts with a pre-trip decision about the mode that is being chosen for the upcoming trip, the route that the user will take and the start-time window, with trip costs being positive or negative dependent on the externalities caused, such as GHG emissions and occupied space. A negative price refers to a payback to incentivise greener modes. This should encourage users to think about their mode of transportation, the switch to more ecological routes or reconsideration of departure time, some of the major purposes of the MobilityCoin System. Therefore the system tends to stimulate optimization of existing offers rather than fundamental transformation, what could make the system effective in the short term.
The mobility price function determines the costs pMMoC for a trip which will be charged in MobilityCoins. The price for a trip is usually known as a priori. The function is defined by the agency and depends in its simplest form on several variables and parameters following Eq. 1. The distance of the trip d, the state of the transportation system when the trip takes place as well as the mode-specific parameters of space and emission m(s, e) impact the charge to the user. The latter are displayed for a variety of modes in Figure 3. The state of the system is represented via the crowding coefficient c. It increases with the overall demand or vehicle flows, but is mode specific.
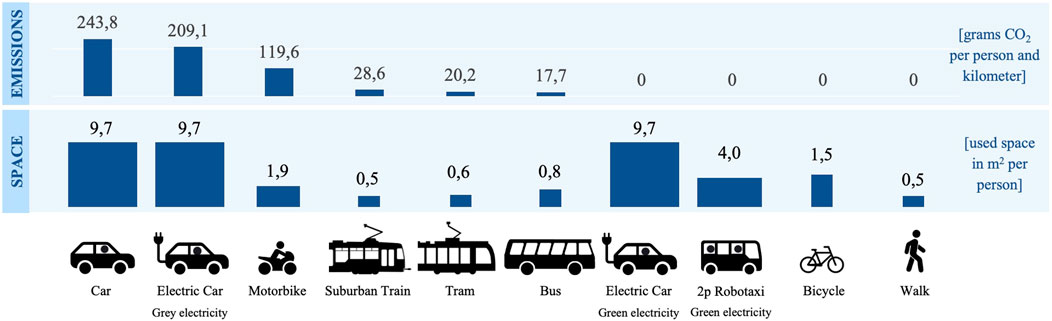
Figure 3. Input parameters for pricing function—specific space consumption and emissions of different modes (For Sensible Transport, 2021)
Once the decision about the desired mode, route and departure-time is made, the base charge is fixed. Now, on-trip options for the chosen mode become available. The selection within the on-trip section can be categorized by route-adjustments, travel-behavior-adjustments and level of urgency. Following the long-term goal of reducing GHG emissions, further incentives can lead users to take an ecological route, by reducing cruising speed or by carpooling. Park and Ride systems can be suggested by the system to promote the change to public transport. A stop can be initiated to recharge electric vehicle batteries at a service station with subsidized electricity rates, to continue driving at an off-peak time. The on-trip features enable the agency to generate further revenue and constitute supplementary incentives even for presumed unsustainable modes. Additionally, mobility providers can use a new market place to offer amenities and conveniences for their customers. With either digital services like smartphone charging options and WiFi plans or comfort features with space and seating options, the utility of credits can be extended beyond pure mobility usage. This facet of the MobilityCoin System serves as additional revenue generation capability for shared transportation modes and makes these modes more attractive. However, it deviates from the core purpose of the MobilityCoin System, which is why there is no further elaboration on this part in the paper.
Eventually, the trip is concluded with a hypothetical post-trip element. When a journey is terminated, one has basically two options. For the first option, the journey is over and completed. In this case a parking option might be needed, depending on the previously chosen mode, see Figure 1. Parking is charged according to Eq. 2, that slightly distinguishes from mobility pricing. The price pPMoC consists of parking duration d, position of parking space p and space consumption parameter s of active mode m. The parking position framework influencing parameter m(s) is based on the selective dimensions 1) parking space ownership: Public or private, 2) flow interference: on-street or off-street, and 3) location: central or surrounding areas. Moreover, space parameters are equal to the space parameters in the mobility case shown in Figure 3. On the originator-side, parking is being charged as described, however, fees can be raised by private or corporate users for providing parking space. For the second option, a connection trip has to be planned, which loops the user back to the pre-trip decision. This represents the logical, sequential decision-making process. With regard to real life application, multi-mode and -stop trips may be planned at once.
As one can see in Figure 1, a user could select many options like taking the car or a bus and is charged a certain amount. And then, after choosing the car, if the user would like to travel faster he gets charged additionally. At the end of the trip the user is charged based on the parking scheme, in this case parking on the street in a central location. Pricing follows the externalities caused across all three segments.
3.1.2 Investment (supply)
Additionally, the system envisions transferring changes in mobility demand directly to transportation supply side. This means that after a specific period of time, e.g., one year, users can choose what to do with their remaining budget—either, they can monetize it by selling the coins to the agency or they can spend it on investment options. This process of investing money from the remaining budget (that is no longer required for personal mobility needs) into infrastructure or spatial improvements that fit the individual mobility preferences is voluntary. Some people might profit from monetizing their remaining budget (e.g., because of a lower socioeconomic status), some people might give priority to transportation improvements. For the latter, the city proposes different city-wide measures that can be invested in, e.g., a bike highway, new speed limit zones or capacity improvements in the street network. Generally, all proposed measures serve the MobilityCoin Systems’ superior objective of reducing GHG emissions and the measures with the highest overall user investment will then be realized. This shifts the public’s role in the planning process from passive to active and could further improve the acceptance of the system. Moreover, a self-reinforcing feedback-loop is created: Through the limited budget and the pricing of trips, mobility behaviour is shifted to more sustainable transport modes. Therefore, people might want to invest in better infrastructure for sustainable transportation modes. A better infrastructure for active mobility and public transport will then make the mode shift easier, which again reduces the amount of coins needed to pay for trips.
3.1.3 Market
A carbon tax sets the price of carbon dioxide emissions and the market determines the quantity reduction of emissions. For cap-and-trade it is the other way round. The quantity of emission reductions is set and the market determines the price (He et al., 2012). That may offer the possibility to design strategic road-maps for long-term GHG emissions mitigation due to gradually reducing the cap. Additionally, the pricing mechanism should be designed to cause mode shifts and distribution of travel time concentrations to reduce congestion. Because of the high amount of actors on the market, user behavior and price developments are difficult to predict. To reduce the degree of freedom within the market, the MobilityCoin market is set-up as a regulated market. A regulated market is a market over which an entity practices a level of oversight and monitoring, including user’s rights and freedom of trading. Usually, market regulation is controlled by the government and involves several conditions, e.g., determining who can enter the market and the prices they may charge (Schmalensee and Stavins, 2017). For the MobilityCoin System, the MobilityCoin agency (following mentioned as agency) acts as the respective government’s body. The agency’s function in a market economy is to regulate and monitor the financial and economic system. For the MobilityCoin System, the scope of action is extended by the monitoring of GHG emissions caused by the transportation sector within the system boundaries. Furthermore, the agency has to intervene with short-term and long-term measures in order to achieve overall climate targets. Prices can be adjusted to spread demand at peak travel times while the overall amount of allocated credits can be reduced in the long-term. Since MobilityCoins are the only “goods” traded on the market, initially allocated in number by the agency and charged for a stipulated fare by the agency, the frame-conditions of the market are quite manageable. Nevertheless, one potential disadvantage of cap-and-trade is that without a limit on the market price, the cost of abatement can exceed the benefit of the same. However, this problem can be solved on the part of the agency by setting an upper restraint on the market price. On the opposite side, price support programs setting a minimum credit price can be used to balance concentration on the buyer side and coin generation for beneficial modes can also be limited. These measures turn the system into a hybrid system, a “cap-and-trade system with a price ceiling and/or price floor” (Goulder and Schein, 2013). Above all, the trading price of a credit can be set by the agency as well which completes the tool set of the agency.
Yang and Wang (2011) point out that the market price of a credit is non-zero if and only if all the credits are consumed (Yang and Wang, 2011). However, as mentioned before, the MobilityCoin System covers additional on-trip and post-trip credit flows as well as the possibility for investment in the side of transportation supply which makes a considerable difference to the rating of each credit on the market. That means, the market-clearing condition specifying market dynamics and credit price embrace the aforementioned aspects as well as shown in eqn. 3. The sum of spent coins in pre-trip κpre, on-trip κon, post-trip offers κpre, and investments S cannot exceed the initially allocated number of coins I plus additionally bought number of coins R out of the reserve.
Therefore, the amount of coins allocated to the users (supply) needs to be somewhere between full mobility (pre-/on-/post-trip) capabilities and a set goal in which to push the market, while taking rising market prices and reductions in GHG emissions into consideration. Nevertheless, there are risks of inefficient market operation and market failures. This can be caused by seller/buyer concentration or because a market does not form, respectively cannot sustain operation due to active free riders who benefit from market exchanges while not bearing the costs. For this reason endowments and the pricing mechanism have to be set up properly. Market failure can also be caused by poor user decisions. Since a large, heterogeneous group is affected by an introduction of a MobilityCoin System, processing of information and understanding about the system varies largely, which underpins that fact. Since the agency decides about the initial endowment, prices and rules of credit exchange, the risk for monopolies or narrow oligopolies are neglectable. The decision about a transaction tax is substantial when it comes to revenue generation. Others encourage taxes to reduce non-beneficial behavior of users on the market. Nie (2012a) state that transaction costs put importance on the market arrangement, especially on the initial endowment regarding the results of the system. They showed that in a negotiated market, the initial allocation can depart the equilibrium from the desired spot. For tradable permits, transaction cost can reduce trade volume (Stavins, 1995; Nagurney and Dhanda, 2000). This can be the case for credits as well, by either raising the supply curve upward once the seller pays the tax, or moving the demand curve downward when the tax incurs on the buyer side. Stigler (1971) capture theory says that government’s regulation benefits conditions for the parties being regulated contrary to the public’s interest in reducing market failure. The MobilityCoin market takes a special role concerning this theory, since regulated parties and public derive from the same group. Eventually, the agency itself can make errors if it takes in a special role within the MobilityCoin System. In general, fraud and theft have to be prohibited.
The MobilityCoin agency plays an important role and can actively intervene in the system at all times, e.g., regarding the initial endowment, the market supply regulation or charging mechanism to influence transportation behavior. She can adjust the market volume of credits over time, the credit prices per trip (short-term) as well as the selection and pricing of investment measures to mitigate GHG emissions following a long-term objective, e.g., of minimizing emissions in order to meet the Paris Climate Agreement. One main task of the agency is balancing the credit flow within the system. It starts with a proper set-up of budgets and execution of the initial endowment. Followed by a correct pricing during the redistribution phase with the short-term objective of spreading congestion away from peak hours and reducing GHG emission in the long-term. Finally, to sustain the systems’ operation and to finance investments, revenue must be generated out of the system from transaction taxes and selling of additional credits. However, the costs of operating a tradable credit scheme and market requires further analysis and research (Nie, 2012a).
3.2 Initial endowment
In the tradable credit system literature, various forms of initial allocation procedures have been proposed. There are disparities in the way how credits can be obtained, e.g., free allocation (Xu et al., 2018; Chen et al., 2021), grandfathering (Wadud et al., 2008; Wadud, 2011), auctioning (Wada and Akamatsu, 2013), negotiating (Nie, 2012b), and booking. The validity period of credits differ from validity at link-level in a given time interval (Lessan et al., 2020) to monthly validity (Jose, 2001). Some acknowledge only vehicle owners as eligible (Wadud et al., 2008) while others legitimate only people in a certain area. The amount of issued credits is, for instance, derived based on existing previous emissions (Verhoef et al., 1997) or OD-patterns (Brands et al., 2020).
The MobilityCoin System proposes initial coin allocation based on the following assumptions: The agency issues the coins based on free allocation and the total quantity is constrained by an emission reduction target. Free allocation is chosen, as this can positively impact the social acceptability of the system and reduces the complexity of the system (Fan and Jiang, 2013). The individual allocation for each user depends on personal attributes. Important attributes considered are, for example, health (e.g., an allocation bonus for mobility impaired people), public transport accessibility and the jobs-housing balance. The frequency or necessity of work-related trips are not considered here, as companies might receive specific coin budgets for their employees. Eligible budget receivers are people living inside of the predefined system borders (e.g., a metropolitan area) and must be over 18 years old. The validity of the budget is 1 year, in order to align the system with other societal systems (insurance costs, tax declaration, etc.). It is not possible to accumulate coins over consecutive years. The budget expires after 1 year and the allocation process starts anew. The coin budget must be used to pay for the external costs of trips with all eligible modes in the system (car, public transport, bike, sharing services).
3.3 Parametrizing the MobilityCoin System
It can be expected that most governing parameters of the MobilityCoin System are context or city specific. Thus they must be identified for every context beginning with a throughout assessment of the status quo. The variety of objective functions, involved number of (nonlinear) dependencies between instruments and actors as well as possible behavioral responses make it impossible to formulate one solvable optimization problem to identify the optimal parameters.
A starting point for the identification of relevant parameters are expert interviews (Krabbenborg et al., 2021; Hamm and Bogenberger, 2022). These allow to derive reasonable temporal and spatial system boundaries as well as important variables in the initial credit allocation function. In interviews summarized by Hamm and Bogenberger (2022), experts suggested that it would be important to integrate commuter flows and both urban and rural areas into a TCS system. Regarding the validity period, some experts favored monthly, others yearly validity periods. A reported key argument for a monthly validity period: 1 month gives users the ability to better manage their short-term expenses and revenues and has the same rhythm as salary payments and monthly subscriptions, but it cannot balance out seasonal fluctuations in credit demand. Regarding the initial credit allocation, the experts considered grade of disability, accessibility, distance to public transport and the workplace location as most important variables. Interestingly, most experts agreed that an individual budget function would be suitable which is opposed to the assumptions of uniform or OD-based budgets reported in literature (e.g., Fan and Jiang, 2013; Grant-Muller and Xu, 2014; Brands et al., 2020).
First assessment of the behavioral responses to such a system can be made using focus group (Krabbenborg et al., 2020), stated-preference experiments on the implementation of such as system (Krabbenborg et al., 2021), and stated-preference experiments on mode choice when such a system is in place (Hamm et al., 2022). Especially the latter approach allows to identify the willingess-to-trade in the TCS market, the willingness-to-pay and price elasticities. The mentioned approaches consequently help to identify what level of intervention is required to move the system towards its set goals. Once suitable parameters have been identified, they can be tested in various scenarios in an agent-based and activity-orientated mobility simulator, e.g., MATSim (Horni et al., 2016). These scenarios can also test different pricing schemes and initial budget allocation scenarios. This can be iterated until politically feasible, economically reasonable and public opinion convincing parameters have been identified.
4 Technology—App based system implementation
New technologies play a key role in the realization of the MobilityCoin System. Because of its multi-modal nature, it is a simple way to shift the necessary infrastructure to the user, carrying the device across all modes instead of upgrading all means of transport infrastructure for enabling the system. Smartphones with high computing power and broadband cellular networks like 5G offer great potential for the systems’ implementation and all of its features. From technical perspective, regarding net coverage and general technical achievements, the ground is set. However, broad experiments and implementations are still lacking.
A simple visualization of the realization of the MobilityCoin’s core building blocks are shown in Figure 4. A shows the mobility feature including routing of available modes, estimated mode-specific externalities, e.g., emissions and space, and the charge in MobilityCoins. The calculation of routes, external costs and the MobilityCoin charge is implemented on a central server that processes the route requests from all smartphones and returns the relevant trip information that will be displayed as shown. The market environment covering trading abilities and market price development is displayed in B and a conceivable start for the investment in infrastructure measures can be seen in C. Measures offered by the agency are located on a map. Following the investment funnel, user’s get further information regarding the measures and crowdfunding status like implementation period or potential funding threshold that needs to be reached or exceeded to start implementation.
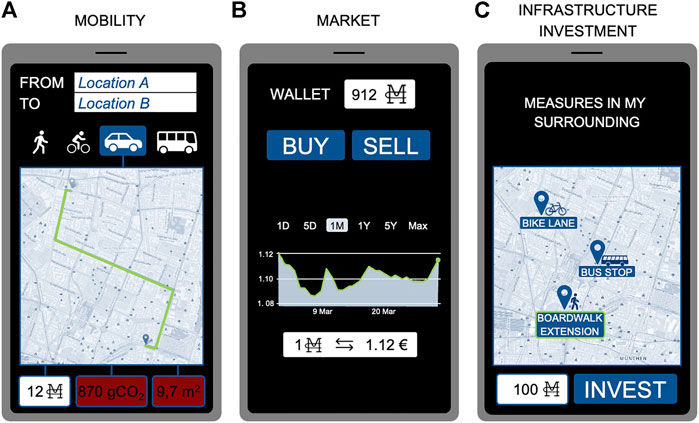
Figure 4. MobilityCoin app interface, (A) is mobility incl. routing and pricing, (B) is the MobilityCoin market and, (C) is the main page for the infrastructure investment funnel.
Since the MobilityCoin System is all about setting incentives to reach the desired outcome of reducing emissions, users can be nudged by seeing their individual environmental performance compared to other users or groups, therefore “gameifying” the road towards the Paris Agreement goals, illustrated in Figure 5A. Paris Climate Goals for the transportation sector can be derived and reflected to the user’s mobility behavior, enabling the user to directly track the caused emissions in short term which can substantially help in changing mobility habits. In Figure 5B users get insight about their activity levels, picking up the ongoing trend of fitness tracking via wearables (Dinh-Le et al., 2019). Further on, the linkage of such wearables as well as the integration of fitness data is feasible, extending the scope of incentives beyond basic mobility needs to leisure sport activities in order to promote a healthy lifestyle. GHG savings compared to other modes can also be visualized. The third building block is shown in Figure 5C with a personal summary of the coins invested via the crowdfunding feature.
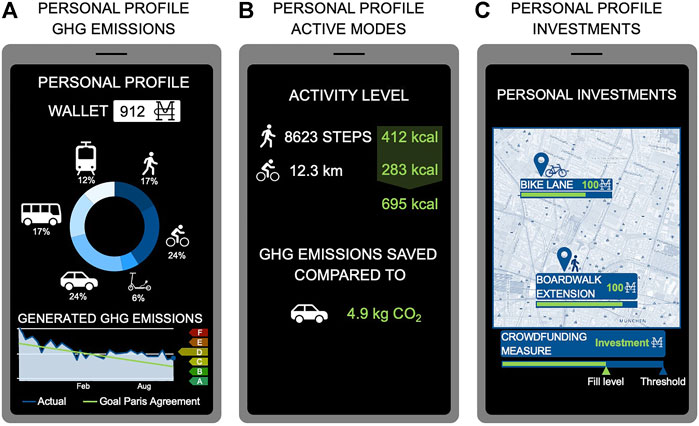
Figure 5. MobilityCoin user personal profile consisting of (A) mobility overview, (B) active mode overview and, (C) personal infrastructure investment summary.
From user’s perspective, basically all features of the MobilityCoin System can be comfortably mapped in one app, which can be convenient for the initial adaptation and continuous use. Nevertheless, owning a smartphone cannot be a basic prerequisite for the use of the MobilityCoin System, since not all users own a smartphone or not all users have their smartphones always on hand. Thus, the accessibility to the system needs to be guaranteed without high barriers. The major part of generated revenue is supposed to go into infrastructure measures. In consideration of the costs for the implementation and operation of the system, alternatives and complementary technologies are not supposed to be investment intensive. One arguable alternative could cover these (basic) functions are smart cards in combination with terminals (Verhoef et al., 1997). There are different kind of smart cards, from simple chip cards solely storing data to microprocessor cards (McDonald, 2000). Latter and hybrid cards can include many configurations of chips that can be helpful for running the MobilityCoin System, e.g., radio frequency (RFID) for contactless communication or secure identification of users. Read and write contactless cards are well known in transportation applications for charging and reloading of fare values. Lower security was a minor issue for those types of application, in contrast to the extended features and potentially higher amounts of funds circulating (Basics, 2010). However, the app based operation realizes the full potential of the MobilityCoin System.
5 Challenges
Putting aside vehicle quota or licensing plate rationing schemes, there are currently no tradable credit schemes for congestion management. Before an implementation, either of a conventional tradable credit scheme or MobilityCoin System, several challenges have to be addressed. Among which is the identification of suitable and acceptable policy parameters that lead to successful outcomes, e.g., reaching of climate change goals. To identify these parameters, economic equilibrium and agent-based modeling is necessary along behavioral experiments on acceptance, trading and mobility behavior in such a scheme. Further, technical and regulatory aspects of the implementation as well as the policy process must be discussed with stakeholders and decision-makers. The aspect of equity is crucial as well and must be carefully considered during the design of the system. An assessment on how to account for equity in the MobilityCoin System starts with the initial endowment of credits. Most of the literature assumes a uniform or OD-based initial credit allocation, however, individual attributes such as disabilities, the distance and accessibility to public transport services, and the location of work could be important parameters for an individual budget allocation function. While some employees are flexible in place and time, several work places require personnel attendance causing increased mobility demand. Pricing schemes are another system element in which equity issues arise. Assuming that one can generate revenue by using an active mode, it should be taken into account that some users are limited in their mode choice due to, e.g., health issues or age. We also face equity issues in the trading of coins in terms of accessibility to the market, technical or trading know-how or plainly time constraints. The forecasting of market prices as well as the planning of budget can be complex and time-consuming. Also the crowdfunding element of the system entails equity issues. Some users rely on more expensive modes, leaving them with a lower remaining budget for preferred crowdfunding measures. This may lead to inequalities in the investment or crowdfunding power of the users.
6 Conclusion
In this paper, we have presented an extension to a car- and congestion-oriented tradable credit scheme: the MobilityCoin System. Its holistic and multi-modal approach to include all relevant trip elements with charges or subsidies as well as its idea of allowing travelers to invest parts of their mobility credit budget in supply-side measures, set it apart from existing proposed system designs.
The MobilityCoin System can create benefits for various stakeholders like industries, governments and users of the system. First, users of the system get an all-in-one mobility solution with the aforementioned benefits. With the initial MobilityCoin endowment, they receive a budget for sustainable basic mobility and are integrated into the infrastructure planning process. The MobilityCoin market offers market based allocation and the ability to monetize coins. To raise equity, agreements could lead to subsidized mobility for certain groups of people such as specific age and/or vulnerable groups. Second, industries can apply the system in different beneficial ways. Employers can raise attractiveness for employees with offers of MobilityCoin budgets, e.g., as part of the salary (job-ticket) at the same time supporting change in transportation behavior. Various business models are conceivable by offering services of third-parties in the MobilityCoin on-trip section, enabling the same to get access to (new) customers. Transport service provider may be able to raise profits due to offered amenities. Third, the MobilityCoin System can support governments in different respects. It can be an efficient and effective tool to reach climate goals. Transaction tax revenues can be used to close the energy tax gap that results from the shift to greener mode as well as finance the crowdfunding feature. The same can help governments to understand the needs in transportation planning as they get access to the knowledge and experience of residents or commuters.
In closing, only the integration of almost every transport policy instrument into a single scheme ensures that in the end the transportation sector will meet its obligation towards reaching emission targets. As the market volume of credits will be larger compared to a congestion-oriented scheme only, it could be expected that the trading behavior leads to a better market price discovery, followed by more efficient market outcomes.
Data availability statement
The original contributions presented in the study are included in the article/Supplementary Material, further inquiries can be directed to the corresponding author.
Author contributions
All authors contributed to conception and design of the system. LH contributed to the “initial endowment” and “infrastructure invest” part, the remaining parts were written by PS and AL. All authors contributed to manuscript revision, read, and approved the submitted version.
Funding
This work is supported by the Free State of Bavaria and by the Federal Ministry of Education and Research (BMBF) funded project MCube-SASIM (Grant-no. 03ZU1105GA).
Conflict of interest
The authors declare that the research was conducted in the absence of any commercial or financial relationships that could be construed as a potential conflict of interest.
Publisher’s note
All claims expressed in this article are solely those of the authors and do not necessarily represent those of their affiliated organizations, or those of the publisher, the editors and the reviewers. Any product that may be evaluated in this article, or claim that may be made by its manufacturer, is not guaranteed or endorsed by the publisher.
References
Abdul Aziz, H. M., and Ukkusuri, S. V. (2013). Tradable emissions credits for personal travel: a market-based approach to achieve air quality standards. Int. J. Adv. Eng. Sci. Appl. Math. 5, 145–157. doi:10.1007/s12572-013-0092-4
Abkowitz, M. D. (1981). An analysis of the commuter departure time decision. Transportation 10, 283–297. doi:10.1007/bf00148464
Andersson, A., Hiselius, L. W., and Adell, E. (2018). Promoting sustainable travel behaviour through the use of smartphone applications: a review and development of a conceptual model. Travel Behav. Soc. 11, 52–61. doi:10.1016/j.tbs.2017.12.008
Bao, H. X., and Ng, J. (2022). Tradable parking permits as a transportation demand management strategy: a behavioural investigation. Cities 120. doi:10.1016/j.cities.2021.103463
Bao, Y., Verhoef, E. T., and Koster, P. (2019). Regulating dynamic congestion externalities with tradable credit schemes: does a unique equilibrium exist? Transp. Res. Part B Methodol. 127, 225–236. doi:10.1016/j.trb.2019.07.012
Barry, (2011). How smartphones can improve public transit. Available at: https://www.wired.com/2011/04/how-smartphones-can-improve-public-transit/(Accessed 05, 12 2022).
Bogenberger, K., Blum, P., Dandl, F., Hamm, L.-S., Loder, A., Malcolm, P., et al. (2021). Mobilitycoins – a new currency for the multimodal urban transportation system. arXiv, 13441.
Brands, D. K., Verhoef, E. T., Knockaert, J., and Koster, P. R. (2020). Tradable permits to manage urban mobility: Market design and experimental implementation. Transp. Res. Part A Policy Pract. 137, 34–46. doi:10.1016/j.tra.2020.04.008
Bucher, D., Cellina, F., Mangili, F., Raubal, M., Rudel, R., Rizzoli, A. E., et al. (2016). “Exploiting fitness apps for sustainable mobility-challenges deploying the goeco app,” in ICT for sustainability 2016 (Atlantis Press), 89–98.
Cellina, F., Bucher, D., Mangili, F., Veiga Simão, J., Rudel, R., Raubal, M., et al. (2019). A large scale, app-based behaviour change experiment persuading sustainable mobility patterns: methods, results and lessons learnt. Sustainability 11, 2674. doi:10.3390/su11092674
Chen, S., Seshadri, R., Azevedo, C. L., Akkinepally, A. P., Liu, R., Araldo, A., et al. (2021). Analysis and design of markets for tradable mobilitycredit schemes. arXiv.
ClearRoad, (2022). Digital tools for 21st century roads. Available at: https://www.clearroad.io (Accessed 05, 30 2022).
crowdsourced transport (2022). Public transport crowdsourcing. Available at: https://crowdsourced-transport.com/crowdsourced-public-transport/(Accessed 0605 2022).
Dablanc, L., and Montenon, A. (2015). Impacts of environmental access restrictions on freight delivery activities: Example of low emissions zones in Europe. Transp. Res. Rec. 2478, 12–18. doi:10.3141/2478-02
de Palma, A., and Lindsey, R. (2011). Traffic congestion pricing methodologies and technologies. Transp. Res. Part C Emerg. Technol. 19, 1377–1399. doi:10.1016/j.trc.2011.02.010
de Palma, A., Proost, S., Seshadri, R., and Ben-Akiva, M. (2018). Congestion tolling - dollars versus tokens: a comparative analysis. Transp. Res. Part B Methodol. 108, 261–280. doi:10.1016/j.trb.2017.12.005
De Palma, A., and Rochat, D. (2000). Mode choices for trips to work in geneva: an empirical analysis. J. Transp. Geogr. 8, 43–51. doi:10.1016/s0966-6923(99)00026-5
Dinh-Le, C., Chuang, R., Chokshi, S., Mann, D., et al. (2019). Wearable health technology and electronic health record integration: scoping review and future directions. JMIR Mhealth Uhealth 7, e12861. doi:10.2196/12861
Drut, M. (2018). Spatial issues revisited: The role of shared transportation modes. Transp. policy 66, 85–95. doi:10.1016/j.tranpol.2018.02.003
Ellerman, A. D., and Joskow, P. L. (2008). The European Union’s emissions trading system in perspective. Arlington, VA: Pew Center on Global Climate Change.
Fan, W., and Jiang, X. (2013). Tradable mobility permits in roadway capacity allocation: review and appraisal. Transp. Policy 30, 132–142. doi:10.1016/j.tranpol.2013.09.002
Fhwa, (2016). Smartphone applications to influence travel choices practices and policies. Technical Reports
Friman, M., Larhult, L., and Gärling, T. (2013). An analysis of soft transport policy measures implemented in Sweden to reduce private car use. Transportation 40, 109–129. doi:10.1007/s11116-012-9412-y
Goddard, H. C. (1997). Using tradeable permits to achieve sustainability in the world’s large cities policy design issues and efficiency conditions for controlling vehicle emissions, congestion and urban decentralization with an application to Mexico city. Environ. Resour. Econ. 10, 63–99. doi:10.1023/a:1026444113237
Goulder, L. H., and Schein, A. R. (2013). Carbon taxes versus cap and trade: a critical review. Clim. Chang. Econ. (Singap). 4, 1350010. doi:10.1142/s2010007813500103
Grant-Muller, S., and Xu, M. (2014). The role of tradable credit schemes in road traffic congestion management. Transp. Rev. 34, 128–149. doi:10.1080/01441647.2014.880754
Guin, A., et al. (2021). Impact of smartphone applications on trip routingTech. Rep. Southeastern Transportation Research, Innovation, Development and Education.
Hamm, L. S., and Bogenberger, K. (2022). Mobilitycoins – first results on social acceptance, system boundaries and individual budgets in a tradeable credit system in Germany.
Hamm, L. S., Loder, A., Weikl, S., Bogenberger, K., Schatzmann, T., and Axhausen, K. W. (2022). User behavior and preferences in tradeable credit schemes - an empirical study for munich, Germany (currently under review).
He, Y., Wang, L., and Wang, J. (2012). Cap-and-trade vs. carbon taxes: a quantitative comparison from a generation expansion planning perspective. Comput. Industrial Eng. 63, 708–716. doi:10.1016/j.cie.2011.10.005
Holman, C., Harrison, R., and Querol, X. (2015). Review of the efficacy of low emission zones to improve urban air quality in european cities. Atmos. Environ. 111, 161–169. doi:10.1016/j.atmosenv.2015.04.009
Horni, A., Nagel, K., and Axhausen, K. W. (2016). The multi-agent transport simulation MATSim. Ubiquity Press. Available at: Http://www.oapen.org/download?type=documentamp;docid=613715.
Hu, X., Chiu, Y.-C., and Zhu, L. (2015). Behavior insights for an incentive-based active demand management platform. Int. J. Transp. Sci. Technol. 4, 119–133. doi:10.1260/2046-0430.4.2.119
Jose, V. (2001). Making urban road pricing acceptable and effective: searching for quality and equity in urban mobility. Transp. Policy 8, 289–294. doi:10.1016/s0967-070x(01)00024-5
Klenert, D., Mattauch, L., Combet, E., Edenhofer, O., Hepburn, C., Rafaty, R., et al. (2018). Making carbon pricing work for citizens. Nat. Clim. Chang. 8, 669–677. doi:10.1038/s41558-018-0201-2
Kocher, (2015). The mobile revolution in public transport. Available at: https://www.intelligenttransport.com/transport-articles/17365/the-mobile-revolution-in-public-transport/(Accessed 05, 06 2022).
Kockelman, K. M., and Kalmanje, S. (2005). Credit-based congestion pricing: a policy proposal and the public’s response. Transp. Res. Part A Policy Pract. 39, 671–690. doi:10.1016/j.tra.2005.02.014
Krabbenborg, L., Molin, E., Annema, J. A., and van Wee, B. (2021). Exploring the feasibility of tradable credits for congestion management. Transp. Plan. Technol. 44, 246–261. doi:10.1080/03081060.2021.1883226
Krabbenborg, L., Mouter, N., Molin, E., Annema, J. A., and van Wee, B. (2020). Exploring public perceptions of tradable credits for congestion management in urban areas. Cities 107, 102877. doi:10.1016/j.cities.2020.102877
Lam, P. T., and Law, A. O. (2016). Crowdfunding for renewable and sustainable energy projects: an exploratory case study approach. Renew. Sustain. Energy Rev. 60, 11–20. doi:10.1016/J.RSER.2016.01.046
Leape, J. (2006). The London congestion charge. J. Econ. Perspect. 20, 157–176. doi:10.1257/jep.20.4.157
Lessan, J., Fu, L., and Bachmann, C. (2020). Towards user-centric, market-driven mobility management of road traffic using permit-based schemes. Transp. Res. Part E Logist. Transp. Rev. 141, 102023. doi:10.1016/j.tre.2020.102023
Lévay, P. Z., Drossinos, Y., and Thiel, C. (2017). The effect of fiscal incentives on market penetration of electric vehicles: a pairwise comparison of total cost of ownership. Energy Policy 105, 524–533. doi:10.1016/j.enpol.2017.02.054
Lindsey, R., and Santos, G. (2020). Addressing transportation and environmental externalities with economics: are policy makers listening? Res. Transp. Econ. 82, 100872. doi:10.1016/j.retrec.2020.100872
Liu, R., Chen, S., Jiang, Y., Seshadri, R., Ben-Akiva, M. E., and Azevedo, C. L. (2020). Managing network congestion with a tradable credit scheme: a trip-based mfd approach.
Lozano Domínguez, J. M., and Mateo Sanguino, T. d. J. (2021). Walking secure: safe routing planning algorithm and pedestrian’s crossing intention detector based on fuzzy logic app. Sensors 21, 529. doi:10.3390/s21020529
Mackowski, D., Bai, Y., and Ouyang, Y. (2015). Parking space management via dynamic performance-based pricing. Transp. Res. Procedia 7, 170–191. doi:10.1016/j.trpro.2015.06.010
McDonald, N. (2000). Multipurpose smart cards in transportation: benefits and barriers to use. webpage.
Mesoraca, J., and Brakewood, C.University of Tennessee in Knoxville (2018). A synthesis of mobile ticketing applications used by commuter railroads in the United States. J. Public Trans. 21, 86–100. doi:10.5038/2375-0901.21.2.6
Miralinaghi, M., Peeta, S., He, X., and Ukkusuri, S. V. (2019). Managing morning commute congestion with a tradable credit scheme under commuter heterogeneity and market loss aversion behavior. Transp. B Transp. Dyn. 7, 1780–1808. doi:10.1080/21680566.2019.1698379
Miralinaghi, M., and Peeta, S. (2016). Multi-period equilibrium modeling planning framework for tradable credit schemes. Transp. Res. Part E Logist. Transp. Rev. 93, 177–198. doi:10.1016/j.tre.2016.05.013
Molloy, J. (2021). Undertaking mobility field experiments using GPS tracking. Ph.D. thesis, ETH Zurich.
Möser, G., and Bamberg, S. (2008). The effectiveness of soft transport policy measures: a critical assessment and meta-analysis of empirical evidence. J. Environ. Psychol. 28, 10–26. doi:10.1016/j.jenvp.2007.09.001
Musso, A., and Rothengatter, W. (2013). Internalisation of external costs of transport–a target driven approach with a focus on climate change. Transp. Policy 29, 303–314. doi:10.1016/j.tranpol.2012.07.001
Nagurney, A. (2000). Alternative pollution permit systems for transportation networks based on origin/destination pairs and paths. Transp. Res. Part D Transp. Environ. 5, 37–58. doi:10.1016/s1361-9209(99)00023-1
Nagurney, A., and Dhanda, K. K. (2000). Noncompliant oligopolistic firms and marketable pollution permits: statics and dynamics. Ann. Operations Res. 95, 285–312. doi:10.1023/a:1018906225220
Nelson, D. C. (2000). Intelligent transportation primer. Washington, DC: Institute of Transportation Engineers.
NEXTCITY (2014). Downtown denver could soon have a crowdfunded bike lane. Available at: https://nextcity.org/urbanist-news/denver-bike-lanes-crowdfunding/ (Accessed 06, 06 2022).
Nie, Y. M. (2012a). Transaction costs and tradable mobility credits. Transp. Res. Part B Methodol. 46, 189–203. doi:10.1016/j.trb.2011.10.002
Nie, Y. M. (2012b). Transaction costs and tradable mobility credits. Transp. Res. Part B Methodol. 46, 189–203. doi:10.1016/j.trb.2011.10.002
Olszewski, P., and Xie, L. (2005). Modelling the effects of road pricing on traffic in Singapore. Transp. Res. Part A Policy Pract. 39, 755–772. doi:10.1016/j.tra.2005.02.015
Prud’Homme, R., and Bocarejo, J. P. (2005). The London congestion charge: a tentative economic appraisal. Transp. Policy 12, 279–287. doi:10.1016/j.tranpol.2005.03.001
Raux, C. (2004). The use of transferable permits in transport policy. Transp. Res. Part D Transp. Environ. 9, 185–197. doi:10.1016/j.trd.2004.01.001
Schmalensee, R., and Stavins, R. N. (2017). The design of environmental markets: what have we learned from experience with cap and trade? Oxf. Rev. Econ. Policy 33, 572–588. doi:10.1093/oxrep/grx040
Seilabi, S. E., Tabesh, M. T., Davatgari, A., Miralinaghi, M., and Labi, S. (2020). Promoting autonomous vehicles using travel demand and lane management strategies. Front. Built Environ. 6. doi:10.3389/fbuil.2020.560116
Shaheen, S. A., and Kemmerer, C. (2008). Smart parking linked to transit: lessons learned from field test in san francisco bay area of california. Transp. Res. Rec. 2063, 73–80. doi:10.3141/2063-09
Shaheen, S., Cohen, A., and Martin, E. (2017). “Smartphone app evolution and early understanding from a multimodal app user survey,” in Disrupting mobility (Springer), 149–164.
Shaheen, S., Cohen, A., Zohdy, I., and Kock, B. (2016). Smartphone applications to influence travel choices: practices and policies.” in Recent work, eScholarship. Institute of Transportation Studies at UC Berkeley.
Stavins, R. N. (1995). Transaction costs and tradeable permits. J. Environ. Econ. Manag. 29, 133–148. doi:10.1006/jeem.1995.1036
Stigler, G. J. (1971). The theory of economic regulation. Bell J. Econ. Manag. Sci. 2, 3. doi:10.2307/3003160
Sunio, V., and Schmöcker, J. D. (2017). Can we promote sustainable travel behavior through mobile apps? evaluation and review of evidence. Int. J. Sustain. Transp. 11, 553–566. doi:10.1080/15568318.2017.1300716
Tuveri, G., Garau, M., Sottile, E., Pintor, L., Atzori, L., Meloni, I., et al. (2022). Beep4me: automatic ticket validation to support fare clearing and service planning. Sensors 22, 1543. doi:10.3390/s22041543
UVEK (2018). Multimodale mobilitätsdienstleistungen massnahmenpläne: mobilitätsdaten und offnung vertrieb weiterer mobilitätsanbieter ausserhalb des öV. Available at: https://www.newsd.admin.ch/newsd/message/attachments/55201.pdf (Accessed 05, 06 2022).
Vasileiadou, E., Huijben, J. C., and Raven, R. P. (2016). Three is a crowd? Exploring the potential of crowdfunding for renewable energy in The Netherlands. J. Clean. Prod. 128, 142–155. doi:10.1016/J.JCLEPRO.2015.06.028
Verhoef, E., Nijkamp, P., and Rietveld, P. (1997). Tradeable permits: Their potential in the regulation of road transport externalities. Environ. Plann. B 24, 527–548. doi:10.1068/b240527
Vickrey, W. (1955). Some implications of marginal cost pricing for public utilities. Am. Econ. Rev. 45, 605
Wada, K., and Akamatsu, T. (2013). A hybrid implementation mechanism of tradable network permits system which obviates path enumeration: an auction mechanism with day-to-day capacity control. Procedia - Soc. Behav. Sci. Symposium Transp. Traffic Theory (ISTTT 2013) 80, 304–326. doi:10.1016/j.sbspro.2013.05.018
Wadud, Z., Noland, R. B., and Graham, D. J. (2008). Equity analysis of personal tradable carbon permits for the road transport sector. Environ. Sci. Policy 11, 533–544. doi:10.1016/j.envsci.2008.04.002
Wadud, Z. (2011). Personal tradable carbon permits for road transport: why, why not and who wins? Transp. Res. Part A Policy Pract. 45, 1052–1065. doi:10.1016/j.tra.2010.03.002
Wang, G., Gao, Z., Xu, M., and Sun, H. (2014). Joint link-based credit charging and road capacity improvement in continuous network design problem. Transp. Res. Part A Policy Pract. 67, 1–14. doi:10.1016/j.tra.2014.05.012
Wang, G., Xu, M., Grant-Muller, S., and Gao, Z. (2020). Combination of tradable credit scheme and link capacity improvement to balance economic growth and environmental management in sustainable-oriented transport development: a bi-objective bi-level programming approach. Transp. Res. Part A Policy Pract. 137, 459–471. doi:10.1016/j.tra.2018.10.031
Wang, X., Yang, H., Zhu, D., and Li, C. (2012). Tradable travel credits for congestion management with heterogeneous users. Transp. Res. Part E Logist. Transp. Rev. 48, 426–437. doi:10.1016/j.tre.2011.10.007
Wirtz, M. H., and Klähr, J. (2019). Smartphone based in/out ticketing systems: A new generation of ticketing in public transport and its performance testing. WIT Trans. Built Environ. 182, 351. doi:10.2495/UT180321
Work, D. B., and Bayen, A. M. (2008). Impacts of the mobile internet on transportation cyberphysical systems: Traffic monitoring using smartphones.” in Natl. Workshop Res. High-Confidence Transp. Cyber-Physical Syst. Automot. Aviat.& Rail, 18–20.
Xiao, F., Qian, Z., and Zhang, H. M. (2013). Managing bottleneck congestion with tradable credits. Transp. Res. Part B Methodol. 56, 1–14. doi:10.1016/j.trb.2013.06.016
Xu, M., Mussone, L., and Grant-Muller, S. (2018). Effects of a tradable credits scheme on mobility management: A household utility based approach incorporating travel money and travel time budgets. Case Stud. Transp. Policy 6, 432–438. doi:10.1016/j.cstp.2017.05.004
Yang, H., and Wang, X. (2011). Managing network mobility with tradable credits. Transp. Res. Part B Methodol. 45, 580–594. doi:10.1016/j.trb.2010.10.002
Yunex, (2022). Our advanced road pricing technology for intelligent demand management: the basis for future mobility. Available at: https://www.yunextraffic.com/global/en/portfolio/traffic-management/tolling-solutions (Accessed 06, 02 2022)
Zhang, S., Yu, Y., Zhu, Q., Qiu, C. M., and Tian, A. (2020). Green innovation mode under carbon tax and innovation subsidy: An evolutionary game analysis for portfolio policies. Sustain. Switz. 12, 1385. doi:10.3390/su12041385
Keywords: tradable credit scheme, full-trip mobility management, multi-modal demand management, infrastructure investment, travel demand management, active travel and demand management, smartphone-based mobility
Citation: Servatius P, Hamm L, Loder A and Bogenberger K (2022) Conceptualizing an individual full-trip tradable credit scheme for multi-modal demand and supply management: The MobilityCoin System. Front. Future Transp. 3:914496. doi: 10.3389/ffutr.2022.914496
Received: 06 April 2022; Accepted: 07 July 2022;
Published: 09 August 2022.
Edited by:
Patricia Lavieri, The University of Melbourne, AustraliaReviewed by:
Mohammad Miralinaghi, Purdue University, United StatesPrem Chhetri, RMIT University, Australia
Copyright © 2022 Servatius, Hamm, Loder and Bogenberger. This is an open-access article distributed under the terms of the Creative Commons Attribution License (CC BY). The use, distribution or reproduction in other forums is permitted, provided the original author(s) and the copyright owner(s) are credited and that the original publication in this journal is cited, in accordance with accepted academic practice. No use, distribution or reproduction is permitted which does not comply with these terms.
*Correspondence: Philipp Servatius, cGhpbGlwcC5ibHVtQHR1bS5kZQ==