- 1 Department of Epidemiology, School of Public Health, University of Alabama at Birmingham, Birmingham, AL, USA
- 2 MRC Social, Genetic and Developmental Psychiatry Centre, Institute of Psychiatry, King’s College London, London, UK
Previous research suggests an association between hyperactivity–impulsivity – one of the two behavioral dimensions that form attention deficit hyperactivity disorder – and the temperament characteristic of novelty seeking. We aimed to examine etiological links underlying the co-occurrence between these behaviors using a general population sample of 668 twin pairs, ages 7–10, for whom we obtained parent ratings in middle childhood; and pilot longitudinal data on 76 children. Structural equation modeling confirmed a shared genetic etiology (genetic correlation, rD = 0.81; 95% confidence intervals = 0.34–1.00) and showed that much (64%) of the covariation can be accounted for by shared genetic effects. In addition, causal paths were modeled between the two behaviors; 12% of the variance in novelty seeking at age 7 was accounted for by hyperactive–impulsive behaviors at the same age. The causal effects model fits with the current characterization of hyperactive–impulsive behaviors reflecting a heightened need for stimulation. This has important implications for the management of hyperactive–impulsive behaviors in clinical settings.
Introduction
A key issue for epidemiological studies into the causes of trait co-occurrence is the issue of causation. It remains a challenge for epidemiologists to disentangle whether traits co-occur due to shared risk factors or effects where having the liability for trait A causes a change in the individual, or their environment, such that trait B may arise. This has important implications for where interventions aimed at preventing secondary trait development should be targeted. Longitudinal data can be a strong way to address etiological issues of comorbidity, where causal effects are suspected. However, longitudinal studies are subject to stringent methodological requirements (Neale et al., 1994), in addition to a high time and cost (Gillespie et al., 2003). An alternative, and little understood, approach is to harness the power of family data where there are known degrees of genetic and environmental sharing between family pairs. Structural equation models, where cross-sectional data are collected on pairs of relatives, can be used to model causal paths between behaviors. When collected on groups of relatives with different genetic similarities, models that specify causal influences between phenotypes will generate different expected variance/covariance matrices, to those which model only shared etiological factors. By comparing the relative the fit of alternative models it is possible to establish which model provides a better explanation of the data. Here we apply this application to the co-occurrence between hyperactivity–impulsivity and novelty seeking traits.
Attention deficit hyperactivity disorder (ADHD) is characterized by developmentally inappropriate levels of overactivity–impulsivity and/or inattentiveness. The optimal stimulation (Zentall and Zentall, 1983) theory predicts that hyperactive behavior will occur as self-stimulation to regulate suboptimal arousal. Novelty seeking, defined by Cloninger (1987) as a heritable tendency to exhibit exploratory activity in pursuit of reward and the avoidance of monotony, is also linked to arousal regulation, although not all novel situations have the same effects (Mayes, 2000). In ADHD, where arousal regulation may be dysfunctional (van der Meere, 2002; O’Connell et al., 2008), this may result in a complex interplay between novelty seeking and hyperactive behaviors in attempting to regulate or optimize arousal levels. However, apart from evidence that both phenotypes contribute to a highly heritable latent trait (Young et al., 2000; Schlaepfer et al., 2007), to our knowledge, studies have yet to address the etiological links between childhood hyperactive–impulsive symptoms and novelty seeking scores in quantitative genetic studies.
This study aimed to examine the etiological links underlying the overlap between parent ratings of novelty seeking and hyperactivity–impulsivity in a sample of 668 twin pairs between 7 and 10 years of age. The fit of two alternative models is compared, to test whether co-occurrence between the two behaviors is best explained by shared environmental and/or genetic etiological factors, or by phenotypic causal effects where one behavior influences the other. In addition to using cross-sectional twin data, we aimed to strengthen our design by including pilot, longitudinal data on 38 twin pairs with parent ratings of hyperactivity–impulsivity obtained in middle childhood and novelty seeking scores obtained in middle adolescence, to examine if links uphold longitudinally, and to increase our power to establish the direction of causation (i.e., to be able to detect whether novelty seeking more strongly influences hyperactivity–impulsivity or vice versa).
Materials and Methods
Sample
The analyses include both cross-sectional and longitudinal data conducted on two different samples, but combined into one model.
Cross-sectional analyses
Participants are members of the study of activity and impulsivity levels (SAIL) in children, a study of a general population sample of twins at age 7–10 years. The sample was recruited from a birth cohort study, the twins’ early development study (TEDS; Trouton et al., 2002), which had invited parents of all twins born in England and Wales during 1994–1996 to enroll. Despite attrition, the TEDS families continue to be reasonably representative of the UK population with respect to parental occupation, education, and ethnicity (Spinath and O’Connor, 2003). Zygosity has been determined using a standard zygosity questionnaire, which has been shown to have 95% accuracy when compared to zygosity status determined by genotype data (Price et al., 2000). Families on the TEDS register were invited to take part if they fulfilled the following SAIL project inclusion criteria: twins’ birthdates between 1st September 1995 and 31st December 1996; lived within feasible traveling distance of the Research Center (return day trip); ethnic origin white European (to reduce population heterogeneity for molecular genetic studies); recent participation in TEDS, as indicated by return of questionnaires at either 4- or 7-year data collection point; no extreme pregnancy or perinatal difficulties (15 pairs excluded), specific medical syndromes, chromosomal anomalies (two pairs excluded) or epilepsy (one pair excluded); not participating in other current TEDS sub-studies (45 pairs excluded); and not on stimulant or other neuropsychiatric medications (two pairs excluded).
The current analyses focus on data obtained following contact with the first 1230 suitable families on the register. Of these, 672 families agreed to participate, reflecting a participation rate of 55%. Thirty individual children were subsequently excluded (16 children with IQs below 70, three children due to epilepsy, three children due to mild autism, two with obsessive–compulsive disorder, and one child due to each of the following: neurofibromatosis, hyperthyroidism, dyspraxia, severe autism, sickness on day of testing and on stimulant medication for ADHD).
This leaves a final sample of 1314 children. All participants were invited to a research center for cognitive assessment see Kuntsi et al. (2006), where Conners’ rating scale data by the parents were collected for the sample. The final sample consisted of 513 identical (monozygotic, MZ) twins (data for 255 complete twin pairs), 374 same-sex non-identical (dizygotic, DZ) twins (data for 184 complete twin pairs) and 427 opposite-sex DZ twins (207 complete twin pairs). The data for the remaining 22 “singleton” twins were also used for model fitting in the structural equation modeling (see Neale and Cardon, 1992). The mean age was 8.83 years (SD = 0.67). Parents of all participants have given informed consent and the Institute of Psychiatry Ethical Committee approved the study.
Longitudinal analyses
Initial recruitment. Twin pairs were initially recruited from a general population sample of same-sex twins aged between 7 and 11 years, by contacting all primary schools in 16 Local Education Authorities in southern England. Teacher ratings on ADHD symptoms were obtained for 1316 twin pairs and parent ratings for a selected sub-sample of 268 twin pairs [selected based on teacher ratings on the impulsive–hyperactive subscale of Conners’ teacher rating scales (CTRS-28; Goyette et al., 1978), to obtain potential “hyperactive” and control groups; for further details see Kuntsi et al., 2001]. Twin pairs were excluded (n = 11 pairs) if one or both of them had serious disabilities or medical conditions or if they were on stimulant medication. Zygosity was determined by the Twin Similarity Questionnaire (TSQ) and (for those whom were on the borderline of the TSQ) photographs rated by up to five independent raters (see Kuntsi and Stevenson, 2001). Those of this sample who participated in the follow-up study had a mean age of 7.50 (SD = 1.15) at the time of the initial study.
Follow-up sample. All twin pairs who had been invited for cognitive assessments during the initial study (n = 149 pairs) were sent a letter inviting them to take part in the follow-up study. The letters were sent to their home addresses that were current 7–8 years previously, during the initial study. This was followed by a telephone call or a reminder letter, where appropriate. Where we received information that the family had moved out, we attempted to trace the families through the primary school the twins had attended. Although aware of the likely difficulties in tracing families for whom we had no previous home address, we aimed to trace a further 500 twin pairs through the primary schools the twins had attended, and subsequently the secondary schools the twins were attending, asking the schools to forward the invitation letters to the families. Whereas only 14 families refused to participate in the follow-up study, the numbers of participants also remained modest at 76 children (38 families); we received no response from the reminder of the families we had attempted to trace. The final sample had a mean age of 15.25 (SD = 1.25) and consisted of 15 MZ pairs and 23 DZ pairs.
Measures
Cross-sectional analyses
Ratings of hyperactivity–impulsivity. Parents completed the Long Version of the Conners’ parent rating scale (CPRS-R:L; Conners et al., 1998). Data was used from the 9-item DSM-IV Hyperactive–Impulsive subscale. In a few cases, missing data in Conners’ scales were pro-rated if there was more than 75% completion for each subscale.
Ratings of novelty seeking. Parents completed the 18-item novelty seeking subscale of the junior temperament and character inventory (JTCI v2-R; Cloninger et al., 1994).
Longitudinal analyses
Early ratings of hyperactivity–impulsivity. Parents completed the Conners’ parent rating scale (CPRS-48; Goyette et al., 1978) and the 8-item impulsive–hyperactive subscale was used.
Analyses
The structural equation modeling program Mx (Neale et al., 2006) was used to conduct the analyses. Structural equation models work on the basis that for the specific parameters of a model, an expected variance/covariance structure for the data is generated. The fit of these expectations are compared to the observed variance/covariance structure in the data points, and the discrepancy between the two gives a measure of the model fit (please see Bollen, 2005 and Sánchez et al., 2005 for further details on structural equation methodology). Models were fitted to standardized age- and sex-regressed residual scores, which had been normalized using the optimal minimal skew command lnskew0 in STATA v.9.2 (STATAcorps, TX, USA) using raw data analysis. Participants with incomplete data were included in the analyses as Mx provides a method for handling incomplete data by using raw maximum likelihood estimation, in which a likelihood statistic (−2LL) of the data for each observation is calculated. A χ2-difference test can be performed to compare the fit of nested models. For non-nested models, where likelihood ratio tests cannot be applied, the Akaike’s information criteria (AIC) index (X2 − 2 df) can be used to see which model has more support. Low (ideally negative) AIC values indicate less difference between the observed and predicted variances/covariances and, therefore, better fit (Williams and Holahan, 1994).
The twin model. Using the fact that MZ twins share 100% of their inherited parental chromosomes and DZ twins on average 50%, greater MZ than DZ phenotypic similarity suggests additive genetic effects on the variance of the phenotype, modeled as the A parameter. However, if MZ twins are significantly more than twice as similar as DZ twins, non-additive genetic effects, such as dominance (D) are indicated. On the other hand, in pairs that are reared together, if MZ twins are less than twice as similar as DZ twins, the variance component C (common or shared environment) is suggested, and if, despite sharing all their genes, MZ twins are less than 100% concordant for a phenotype, it is indicated that experiences unique to each twin have reduced the twins’ behavioral similarity; in model fitting this yields the E parameter (child-specific environmental variance, which, since it also includes measurement error, cannot be omitted from any model).
The univariate model fitting informs parameter selection in the multivariate model fitting (e.g., the choice to fit D or C parameters), but due to the increased power of multivariate models (Schmitz et al., 1998), only parameter estimates from the latter are presented here.
Multivariate genetic analyses
Cholesky (triangular) decomposition with correlated factors solution. Multivariate genetic analyses allow us to investigate whether the same genetic and environmental factors influence activity level across the three measures (Figure 1). The power to do this is given by the information implied by the MZ:DZ ratio of the cross-twin cross-trait correlations: that is the correlation of one twin’s score on a trait with the co-twin’s score on another trait. If cross-trait twin correlations are greater for MZ than for DZ twins, this implies that genetic factors contribute to the covariation across traits. A genetic correlation (rA) indicates the extent to which genetic influences on one trait overlap with those on another trait (regardless of their individual heritabilities). Correlations can similarly be estimated for shared environment influences (rC) and for child-specific environmental influences (rE). Based on the heritability of each trait, and the estimated genetic correlations, the proportion of the phenotypic correlations that are due to genetic influences can also be calculated. The correlated factor solution (Figure 1) of the Cholesky model is interpreted. This is mathematically equivalent to the Cholesky, but has the advantage of retaining the arbitrary ordering of variables (Loehlin, 1996).
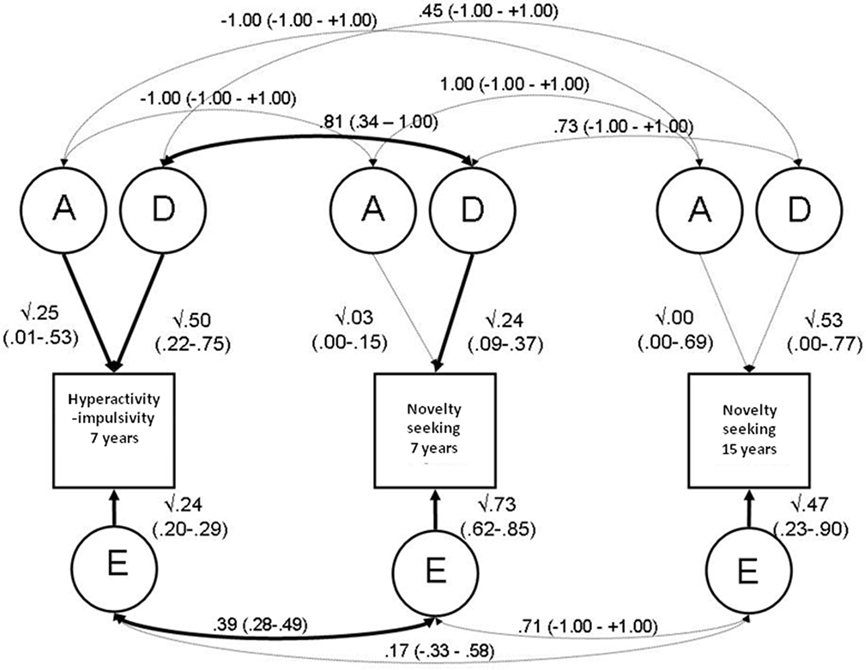
Figure 1. Correlated factors solution of the Cholesky model (+95% confidence intervals; significant paths in bold). Note: Measured variables are represented by squares and described in the Section “Materials and Methods.” Latent (unmeasured) variables are represented by circles according to tradition (McArdle, 1980).
Direction of causation models. The reciprocal causation model (Figure 2) divides the variation in each measure into a latent trait and residual variance (RV). To ensure that the model is identified, in the absence of multiple rating data, the loadings of the latent trait on the measured traits are constrained to one, and the RVs equated. Excluding the RVs can lead to errors in causality inference and direction of causation parameters may be overestimated (Heath et al., 1994).
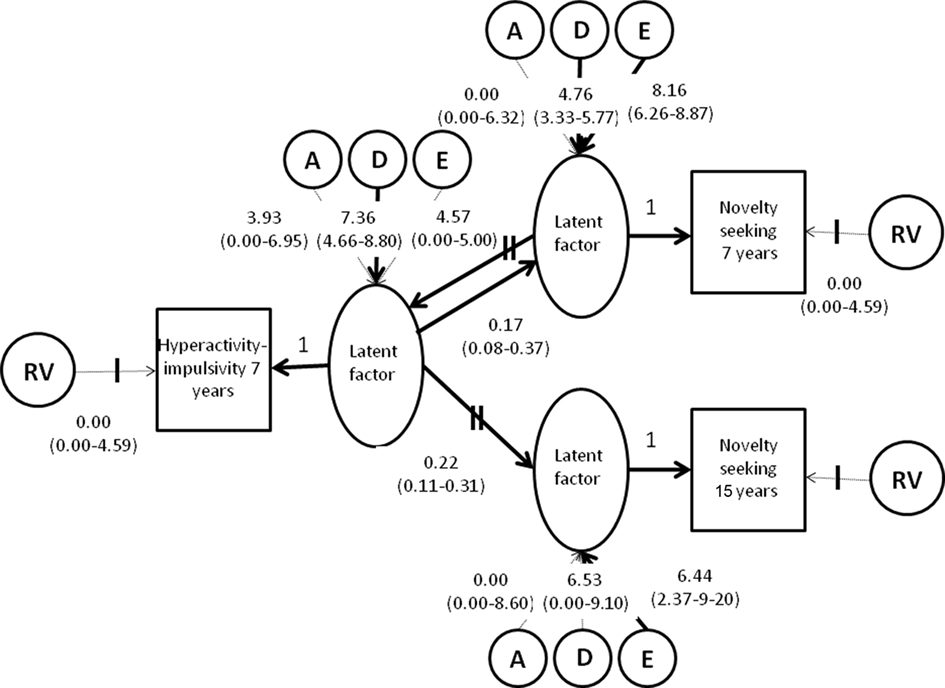
Figure 2. Unstandardized solution of the best fitting direction of causation model (+95% confidence intervals; significant paths in bold). Note: Measured variables are represented by squares and described in the “Materials and Methods.” Latent (unmeasured) variables are represented by circles according to tradition (McArdle, 1980).
Direction of causation (DOC) paths are specified between the latent factors. These can be: unidirectional according to prior hypotheses or the nature of the data, e.g., in the case of the longitudinal data here hyperactivity at 7 years can influence novelty seeking at 15 (H7 → NS15; Figure 2), but the reverse is non-sensical, or reciprocal as in the case of the cross-sectional data where hyperactivity and novelty seeking at 7 can influence each other (H7 → NS7, H7 ← NS7, Figure 2). In this latter case, the significance of each path can be tested. The variance of each latent trait is explained by unique A, C/D, and E influences, RVs, and causal influences from other measured traits. The covariation between measured traits is explained by causal pathways between the traits and the total variance of the causing trait, but not the RV.
This fit of the full model is compared to that of the Cholesky decomposition, to see if there is a significant drop in fit by imposing a reduced model. The fit of reduced models where DOC parameters are dropped are compared to that of the full DOC model, to test the significance of any causation paths.
Results
As assumed by the genetic model, the phenotypic and twin correlations are presented from a constrained model, where phenotypic correlations across twin 1 and 2 and across zygosity are equated. Hyperactivity–impulsivity and novelty seeking were significantly correlated in both samples, with a cross-sectional correlation of r = 0.36 (0.31–0.41) and a longitudinal correlation of 0.26 (0.09–0.41).
For all traits MZ correlations were more than twice DZ correlations suggesting dominant genetic contributions the behaviors (Table 1). This was confirmed in the univariate modeling, where an ACE model provided a significant drop in fit, compared to the ADE model. Further, for all traits, compared to the saturated phenotypic model, imposing an ADE model on the data did not represent a significant drop in fit (further details available for first author).
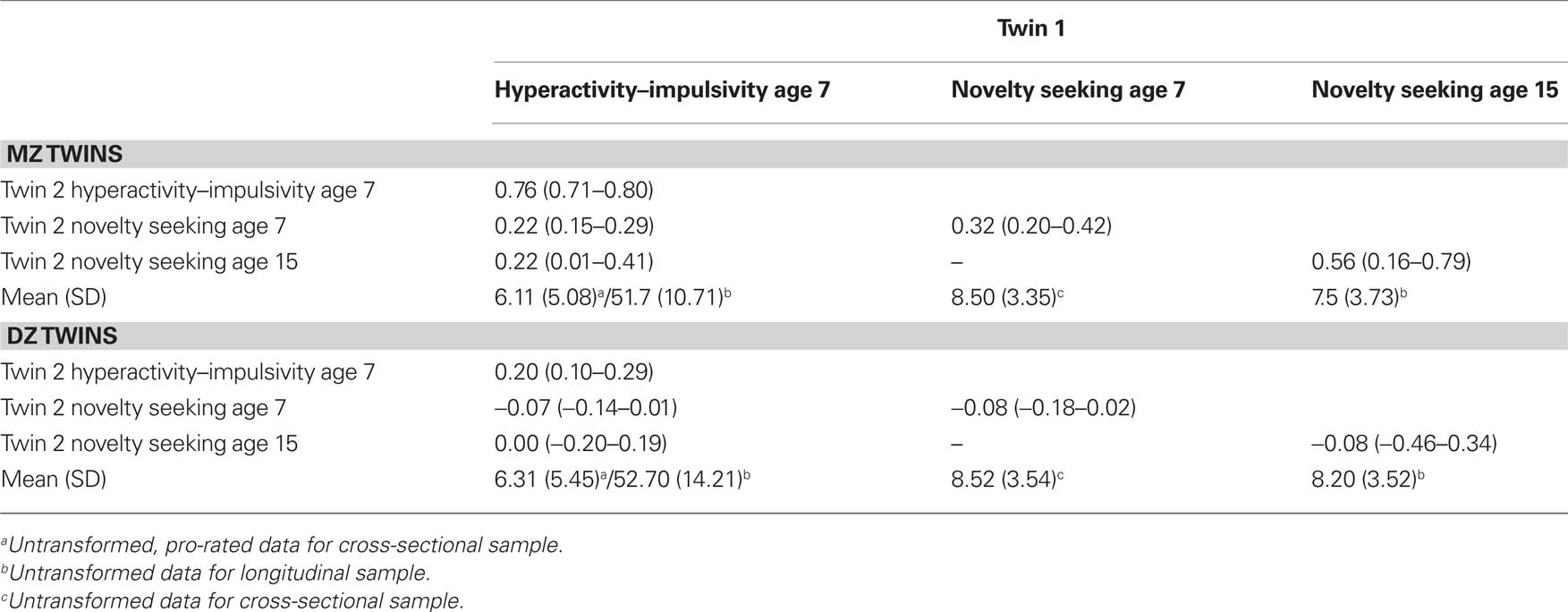
Table 1. Maximum likelihood twin correlations (95% confidence intervals in brackets) and means (SD in brackets) for and across hyperactivity–impulsivity age 7, novelty seeking age 7, and novelty seeking age 15.
Multivariate Genetic Models
Table 2 provides the model fitting results for the multivariate models. The reciprocal causation model was a good fit to the data (X2 = 35.44, df = 29, p = 0.19) and not a worse fit than the full Cholesky (X2 = 3.12, df = 3, p = 0.37). Although the reciprocal causation model would be adopted through the principle of parsimony, and the lower AIC indicates substantial evidence for causal influences being a better explanation for the relationship between hyperactivity–impulsivity and novelty seeking (ΔAIC = 6.88), the Cholesky presents variance component correlations so parameter estimates from both models are presented.
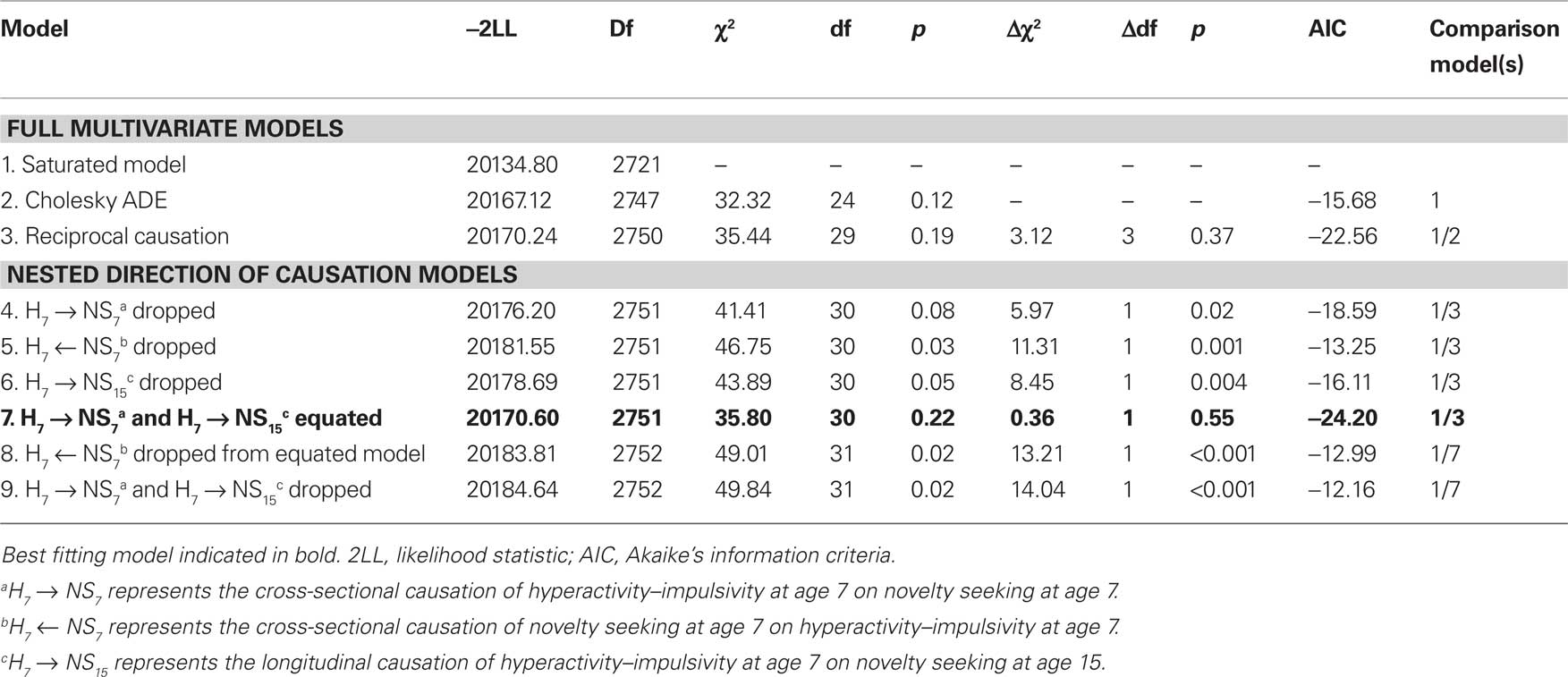
Table 2. Fit of structural equation models examining the etiology between and within hyperactivity–impulsivity age 7, novelty seeking age 7, and novelty seeking age 15.
Cholesky model
All parameter estimates from the correlated factors solution of the Cholesky model (with 95% confidence interval, CIs) are presented in Figure 1. To avoid artificially inflating parameters, parameter estimates are presented from the full ADE Cholesky model. Hyperactivity–impulsivity and novelty seeking at age 7 both showed a significant genetic influence with the remaining variance accounted for by child-specific environmental factors, which include possible measurement error. There was a significant epistatic genetic correlation (rD) of 0.81 (0.34–1.00) between hyperactivity–impulsivity at age 7 and novelty seeking at age 7, suggesting that over three quarters of the dominant genetic influences influencing hyperactivity–impulsivity also influence novelty seeking. The child-specific environment correlation (rE) between the two measures was 0.39 (0.28–0.49).
Direction of causation models
All the direction of causation parameters were significant (Table 2; Figure 2), implying that we do not have the power to show direction of causation at age 7. However, the much lower AIC when dropping the causal path from hyperactivity-impulsivity to novelty seeking than vice versa (ΔAIC = 5.34) indicates “substantial evidence” for hyperactivity–impulsivity behaviors having a stronger causal influence on novelty seeking (Williams and Holahan, 1994). This line of evidence was not supported by parameter point estimates, which were similar for both paths.
To try to resolve this ourselves, and strengthen our power to test for causality in one direction, the causal path from hyperactivity–impulsivity to novelty seeking at age 7 was equated to the causal path of hyperactivity at age 7 to novelty seeking at age 15. This did not result in a significant drop in fit (Table 2) suggesting that the influence of hyperactivity–impulsivity at age 7 on novelty seeking is stable across childhood and adolescence. From this reduced model, we dropped in turn the causal parameters between novelty seeking and hyperactivity–impulsivity (H7 ← NS7, Figure 2) and vice versa (H7 → NS7, and H7 → NS15, Figure 2). Both parameters where significant [Δχ2 (1 df) = 13.21, p 0.001 and 14.04, p 0.001, respectively, Table 2] and, thus, this exercise did not ultimately resolve the direction of causation question.
All parameter estimates from the full direction of causation model are presented in Figure 2. Unlike the correlated factors solution, the reciprocal causation model is not a standardized solution, so standardized parameter estimates are also presented in Table 3. The overall heritability of the phenotypes was as expected from the correlated factors solution of the Cholesky model (Figure 1) ±5%, and so not presented here. In addition, for hyperactivity–impulsivity at 7, 10% of the overall variance (5–39%) was due to the effects of novelty seeking at 7. In turn, hyperactivity–impulsivity at 7 accounted for 12% (7–36%) of the variance in novelty seeking at 7. The pilot data suggested that hyperactivity–impulsivity at age 7 accounts for a similar amount of variance in novelty seeking longitudinally with 6% (2–34%) of the variance in novelty seeking at age 15 being accounted for by causal influences from hyperactivity–impulsivity.

Table 3. Parameter estimates (+95% confidence intervals) from the best fitting direction of causation model.
Discussion
We used a twin design, on a large general population sample obtained in middle childhood, to show that the best fitting model to explain the overlap between hyperactivity–impulsivity and novelty seeking was not one of shared etiological factors, but a model with phenotypic causal influences between the behaviors. We could not determine a unidirectional effect. AIC values indicated a stronger causal impact from hyperactive–impulsive behaviors on novelty seeking than from novelty seeking to hyperactive–impulsive behaviors. However, the model fitting results indicated similar point estimates for both causal paths: 12% (7–36%) of the variance in novelty seeking at age 7 was accounted for by hyperactivity at age 7, and 10% (5–39%) of the variance in hyperactivity–impulsivity at age 7 was accounted for by novelty seeking at age 7.
A small number of studies have examined the link between ADHD and novelty seeking. Yoo et al. (2006) reported an association between ADHD symptoms scores (assessed by the DuPaul’s ADHD Rating Scale standardized Korean version; So et al., 2002); and both parent- and self-rated novelty seeking scores among 9- to 14-year-old children. In adults, parents of children with ADHD showed elevated self-rated novelty seeking scores when they themselves also had a retrospectively diagnosed childhood DSM-IV ADHD diagnosis, compared to parents of children with ADHD who did not have such a diagnosis (Lynn et al., 2005). Yet a further study did not find a significant difference in mother-rated novelty seeking scores between 6 and 18 year old ADHD probands and sibling controls (Rettew et al., 2004), although the lack of a genetically unrelated control group makes interpretation of the findings more difficult. Our data support the association between overactivity and novelty seeking behaviors at both the phenotypic and genetic level.
The causal relationship between a domain of ADHD symptoms and novelty seeking fits with the proposal that ADHD is linked to a heightened need for stimulation and “excitement” (van der Meere, 2002), and the need to regulate arousal results in an interplay between seeking novel situations and hyperactive behaviors. The stronger evidence that novelty seeking influences hyperactive–impulsive behaviors would fit with the theory that arousal dysfunction requires increased stimulation, which when not obtained through environmental stimulation leads to increased activity levels (Antrop et al., 2000).
If there are significant causal relationships between hyperactivity–impulsivity and novelty seeking, one would expect there to be shared etiological influences. If trait A causes trait B, then that which causes A will also, to some extent, underlie B. This was reflected by the good fit of a shared etiological factor model which suggested that over three quarters of the dominant genetic influences underlying hyperactivity at age 7 are shared with novelty seeking at age 7 (rD = 0.81;0.34–1.00), and over a third of the child-specific environmental influences were similarly shared (rE = 0.39;0.28–0.49).
The results of these analyses should be interpreted in the context of several potential limitations. First, there are limitations inherent in the twin design and the genetic models (see Rijsdijk and Sham, 2002 for a fuller discussion), including a batch test for 95% CI which does not control for the multiple testing issues inherent in structural equation modeling where fully saturated models necessarily specify more than one parameter. Second, the data uses only parent sources of information; further research should examine whether “shared method variance” may artificially inflate the overlap between the two behaviors. Third, our follow-up response rate for the longitudinal sample was modest. The longitudinal sample was included in an attempt to increase power for the direction of causation analyses and discriminate between the models using p-values as well as AIC model fit. It was included in the Cholesky model only for comparability to the causal models, and parameter estimates are not interpreted from this sample. A larger response rate in future studies would both increase power, when allied with cross-sectional data, and make it possible to interpret findings from the longitudinal data alone.
This study shows that a significant amount of variance in hyperactive–impulsive behaviors and novelty seeking behaviors can be considered to have been attributed by causal effects of the other behavior, plus shared etiological influences underlying the two behaviors. These findings emphasize the potential usefulness of considering temperament characteristics in developmental investigations of ADHD.
Conflict of Interest Statement
The authors declare that the research was conducted in the absence of any commercial or financial relationships that could be construed as a potential conflict of interest.
Acknowledgments
The study of activity and impulsivity levels (SAIL) in children is funded by a project grant from the Wellcome Trust (GR070345MF). Thank you to all who make this research possible: the TEDS–SAIL families, who give their time and support so unstintingly; Eda Salih, Hannah Rogers, Rebecca Gibbs, Greer Swinard, Kate Lievesley, Kayley O’Flynn, Suzi Marquis, and Rebecca Whittemore, and everyone on the TEDS team. The follow-up of adolescent behaviors (FAB) study is funded by a grant from the British Academy (SG-36684). We thank the FAB families, who gave their time and support across such a long time span; Lisa Cheung, Jenny Van Heerden, Daniel Symons, and Professor Jim Stevenson.
References
Antrop, I., Roeyers, H., Oost, P. V., and Buysse, A. (2000). Stimulation seeking and hyperactivity in children with ADHD. J. Child Psychol. Psychiatry 41, 225–231.
Cloninger, C. R. (1987). The Tridimensional Personality Questionaire: Version 4. St Louis: Department of Psychiatry, Washington University School of Medicine.
Cloninger, C. R., Przybeck, T. R., Svrakic, D. M., and Wetzel, R. D. (1994). The Temperament and Character Inventory (TCI): A Guide to Its Development and Use. St Louis: Center for Psychobiology and Personality.
Conners, C. K., Sitarenios, G., Parker, J. D., and Epstein, J. N. (1998). The revised Conners’ parent rating scale (CPRS-R): factor structure, reliability, and criterion validity. J. Abnorm. Child. Psychol. 26, 257–268.
Gillespie, N. A., Zhu, G., Neale, M. C., Heath, A. C., and Martin, N. G. (2003). Direction of causation modeling between cross-sectional measures of parenting and psychological distress in female twins. Behav. Genet. 33, 383–396.
Goyette, C. H., Conners, C. K., and Ulrich, R. F. (1978). Normative data on revised Conners parent and teacher rating scales. J. Abnorm. Child. Psychol. 6, 221–236.
Heath, A. C., Cloninger, C. R., and Martin, N. G. (1994). Testing a model for the genetic structure of personality: a comparison of the personality systems of Cloninger and Eysenck. J. Pers. Soc. Psychol. 66, 762–775.
Kuntsi, J., Oosterlaan, J., and Stevenson, J. (2001). Psychological mechanisms in hyperactivity: I. Response inhibition deficit, working memory impairment, delay aversion, or something else? J. Child Psychol. Psychiatry 42, 199–210.
Kuntsi, J., Rogers, H., Swinard, G., Borger, N., Meere, J., Rijsdijk, F., and Asheron, P. (2006). Reaction time, inhibition, working memory and “delay aversion” performance: genetic influences and their interpretation. Psychol. Med. 36, 1613–1624.
Kuntsi, J., and Stevenson, J. (2001). Psychological mechanisms in hyperactivity: II. The role of genetic factors. J. Child Psychol. Psychiatry 42, 211–219.
Lynn, D. E., Lubke, G., Yang, M., McCracken, J. T., McGough, J. J., Ishii, J., Loo, S. K., Nelson, S. F., and Smalley, S. L. (2005). Temperament and character profiles and the dopamine D4 receptor gene in ADHD. Am. J. Psychiatry 162, 906–913.
Mayes, L. C. (2000). A developmental perspective on the regulation of arousal states. Semin. Perinatol. 24, 267–279.
McArdle, J. J. (1980). Causal modelling applied to psychonomic systems simulation. Behav. Res. Methods Instrum. Comput. 12, 193–209.
Neale, M. C., Boker, S. M., Xie, G., and Maes, H. (2006). Mx: Statistical Modeling, 7th Edn. Richmond: VCU Department of Psychiatry.
Neale, M. C., and Cardon, L. R. (1992). Methodology for Genetic Studies of Twins and Families. Dordrecht: Kluwer Academic Publishers.
Neale, M. C., Walters, E., Health, A. C., Kessler, R. C., Pérusse, D., Eaves, L. J., and Kendler, K. S. (1994). Depression and parental bonding: cause, consequence, or genetic covariance? Genet. Epidemiol. 11, 503–522.
O’Connell, R. G., Bellgrove, M. A., Dockree, P. M., Lau, A., Fitzgerald, M., and Robertson, I. H. (2008). Self-Alert training: volitional modulation of autonomic arousal improves sustained attention. Neuropsychologia 46, 1379–1390.
Price, T. S., Freeman, B., Craig, I., Petrill, S. A., Ebersole, L., and Plomin, R. (2000). Infant zygosity can be assigned by parental report questionnaire data. Twin Res. 3, 129–133.
Rettew, D. C., Copeland, W., Stanger, C., and Hudziak, J. J. (2004). Associations between temperament and DSM-IV externalizing disorders in children and adolescents. J. Dev. Behav. Pediatr. 25, 383–391.
Rijsdijk, F. V., and Sham, P. C. (2002). Analytic approaches to twin data using structural equation models. Brief. Bioinform. 3, 119–133.
Sánchez, B. N., Budtz-Jørgensen, E., Ryan, L. M., and Hu, H. (2005). Structural equation models. J. Am. Stat. Assoc. 100, 1443–1455.
Schlaepfer, I. R., Clegg, H. V., Corley, R. P., Crowley, T. J., Hewitt, J. K., Hopfer, C. J., Krauter, K., Lessem, J., Rhee, S. H., Stallings, M. C., Wehner, J. M., Young, S. E., and Ehringer, M. A. (2007). The human protein kinase C gamma gene (PRKCG) as a susceptibility locus for behavioral disinhibition. Addict. Biol. 12, 200–209.
Schmitz, S., Cherny, S. S., and Fulker, D. W. (1998). Increase in power through multivariate analyses. Behav. Genet. 28, 357–363.
So, Y. K., Noh, J. S., Kim, Y. S., Ko, S. G., and Koh, Y. J. (2002). The reliability and validity of Korean parent and teacher ADHD rating scale (in Korean). J. Korean Neuropsychiatr. Assoc. 41, 283–289.
Spinath, F. M., and O’Connor, T. G. (2003). A behavioral genetic study of the overlap between personality and parenting. J. Pers. 71, 785–808.
Trouton, A., Spinath, F. M., and Plomin, R. (2002). Twins early development study (TEDS): a multivariate, longitudinal genetic investigation of language, cognition and behavior problems in childhood. Twin Res. 5, 444–448.
van der Meere, J. J. (2002). “The role of attention,” in Hyperactivity Disorders of Childhood, 2nd Edn, ed. S. Sandberg (Cambridge: Cambridge University Press), 162–212.
Williams, L. J., and Holahan, P. J. (1994). Parsimony based fit indicies for multiple fit indicator models: do they work? Struct. Equ. Modeling 1, 161–198.
Yoo, H. J., Kim, M., Ha, J. H., Chung, A., Sim, M. E., Kim, S. J., and Lyoo, I. K. (2006). Biogenetic temperament and character and attention deficit hyperactivity disorder in Korean children. Psychopathology 39, 25–31.
Young, S. E., Stallings, M. C., Corley, R. P., Krauter, K. S., and Hewitt, J. K. (2000). Genetic and environmental influences on behavioral disinhibition. Am. J. Med. Genet. 96, 684–695.
Keywords: ADHD, direction of causation, heritability, hyperactive–impulsive, novelty seeking
Citation: Wood AC, Rijsdijk F, Asherson P and Kuntsi J (2011) Inferring causation from cross-sectional data: examination of the causal relationship between hyperactivity–impulsivity and novelty seeking. Front. Gene. 2:6. doi: 10.3389/fgene.2011.00006
Received: 28 December 2010;
Accepted: 10 February 2011;
Published online: 07 March 2011.
Edited by:
Dongxiao Zhu, University of New Orleans, USAReviewed by:
Qingzhao Yu, Louisiana State University Health Sciences Center, USAFeng Li, Food and Drug Administration, USA
Copyright: © 2011 Wood, Rijsdijk, Asherson and Kuntsi. This is an open-access article subject to an exclusive license agreement between the authors and Frontiers Media SA, which permits unrestricted use, distribution, and reproduction in any medium, provided the original authors and source are credited.
*Correspondence: Alexis C. Wood, School of Public Health, Department of Epidemiology, University of Alabama at Birmingham, RPHB, 230P, 1530 3rd Avenue South, Birmingham, AL 35294, USA.e-mail: lekkiwood@gmail.com