- 1 Institute for Advanced Biosciences, Keio University, Tsuruoka, Japan
- 2 Systems Biology Program, Graduate School of Media and Governance, Keio University, Fujisawa, Japan
- 3 Department of Environment and Information, Keio University, Fujisawa, Japan
MicroRNAs (miRNAs) are members of the small non-coding RNAs, which are principally known for their functions as post-transcriptional regulators of target genes. Regulation by miRNAs is triggered by the translational repression or degradation of their complementary target messenger RNAs (mRNAs). The growing number of reported miRNAs and the estimate that hundreds or thousands of genes are regulated by them suggest a magnificent gene regulatory network in which these molecules are embedded. Indeed, recent reports have suggested critical roles for miRNAs in various biological functions, such as cell differentiation, development, oncogenesis, and the immune responses, which are mediated by systems-wide changes in gene expression profiles. Therefore, it is essential to analyze this complex regulatory network at the transcriptome and proteome levels, which should be possible with approaches that include both high-throughput experiments and computational methodologies. Here, we introduce several systems-level approaches that have been applied to miRNA research, and discuss their potential to reveal miRNA-guided gene regulatory systems and their impacts on biological functions.
Introduction
The control of translation and messenger RNA (mRNA) degradation are important factors in appropriate gene expression, with key roles in various aspects of biology. A class of small non-coding RNAs, called microRNAs (miRNAs), with the ability to alter the expression of large populations of mRNAs has been identified (Ambros et al., 2003; Bartel, 2004; He and Hannon, 2004). miRNAs are transcribed as primary miRNAs (pri-miRNAs), processed to precursor miRNAs (pre-miRNAs), and then to mature miRNAs, which regulate their target genes. Within the Bilateria, mature miRNAs are incorporated to microRNA-induced silencing complexes (miRISCs), which interact mainly with the 3′ untranslated region (UTR) sequences of their target genes. Currently, ∼15,000 miRNAs are registered in miRBase (Release 16), a comprehensive database of miRNAs derived from 142 species, including 1,048 from humans, 672 from the mouse, and even 235 from viral species (Griffiths-Jones et al., 2008). Various studies of a wide variety of species have shown the involvement of miRNA-guided gene regulation in a broad range of biological functions, such as development, cell division, cell differentiation, oncogenesis, immune responses, and cell death, possibly by regulating tens of thousands of mRNAs (Lewis et al., 2003; Bartel and Chen, 2004; Xu et al., 2004; Giraldez et al., 2005; Lim et al., 2005; Miska, 2005; Xie et al., 2005; Zamore and Haley, 2005; Kloosterman and Plasterk, 2006). Moreover, estimates based on computational and experimental analyses suggest that a large number of protein-coding genes (>60% according to Friedman et al., 2009) are regulated by these small RNAs (Lewis et al., 2005; Lim et al., 2005; Friedman et al., 2009), confirming their extensive roles in biological functions through complex gene regulatory networks. Because these biological functions are frequently regulated co-operatively by large numbers of genes, it is important to take a comprehensive view of the massive miRNA–mRNA interactions as a “system”. For this purpose, high-throughput experimental methods combined with computational approaches that allow us to observe gene expression profiles at the transcriptome or proteome level are essential.
The computational prediction of miRNA target genes has contributed greatly to the identification of potential target genes and the prediction of their biological roles (Watanabe et al., 2007; Alexiou et al., 2009). Most of the computational prediction algorithms are based on the binding patterns between miRNAs and their target sites within the 3′ UTR regions of mRNAs, their evolutionary conservation, energetic stability, and some features of the mRNAs outside the miRNA-binding sites (Bartel, 2009; Thomas et al., 2010). Although computational predictions can provide a list of potential target genes relatively easily without experiments, they are not perfect. The major problem is that hundreds or even thousands of potential target genes are predicted, regardless of the specificity of their spatial and temporal expression. For example, the possible combinations of miRNA–mRNA defined only by computational prediction may contain false-positive results in terms of “functional” pairs, because the two molecules may not be expressed together. Therefore, it is essential to integrate experimental information with computational analysis, possibly collected using high-throughput approaches, to identify miRNA–mRNA interactions with higher specificity. This integrated approach will be critical to understanding the biological impact of miRNA-mediated gene regulation.
Here, we focus especially on methodologies that identify transcriptome and proteome profiles applied to miRNA research, and also transcriptome analysis combined with immunoprecipitation (IP). We also summarize some recent findings when these systems-level approaches have been used, which have begun to reveal the overall picture of the complex gene regulation mediated by miRNAs.
Systems-Level Approaches to Analyzing miRNA Expression
Systems-level analysis of miRNA functions mainly consists of two components: miRNA expression profiles and target mRNA expression profiles. High-throughput experimentally based research has expanded from the detection of transcriptomes, in which the expression levels of both mature miRNAs, which are processed and functional form of miRNAs, and their target mRNAs are analyzed (Table 1). Among the several methods used in transcriptome analysis to detect the expression of mature miRNAs, the microarray is the most commonly used, most well developed, and most effective in inexpensively monitoring the expression of miRNAs and/or mRNAs. Recently, another method of transcriptome analysis has been developed that allows us to amplify a variety of mature miRNAs simultaneously with real-time PCR (Schmittgen et al., 2004; Jiang et al., 2005). This method has also been used in a variety of research fields (Bravo et al., 2007; Chen and Stallings, 2007; Tavazoie et al., 2008), because it has the advantages of requiring only small amounts of RNA and better sensitivity and specificity than the microarray (Chen et al., 2009). The major disadvantage of this technique is that it requires specifically designed primers, which could limit detection, as is also the case with the microarray. Another powerful method for transcriptome analysis is RNA-seq (deep sequencing). RNA-seq has an advantage over the microarray or the real-time PCR array in that it does not depend on genome annotation for prior probe or primer selection, which circumvents the problem of bias. Therefore, some genes with specific expression patterns or low abundance that cannot be detected with microarray analysis can be identified with this technique. RNA-seq is also useful for analyzing a variety of species for which no microarray platform is available. However, because the processing and analysis pipelines are relatively easier for the microarray, this method is still the preferred option, especially when large numbers of samples from model organisms with annotated genomes are analyzed.
Application of High-throughput Transcriptome Analysis to miRNA Expression Profiling
When considering miRNA-mediated gene regulation, how miRNA expression is itself regulated is one of the first concerns. Initially, the microarray contributed greatly to establishing an overview of the types of miRNAs that are expressed under different conditions, such as in cells or tissues, at different stages of development, and in physiological or pathological states (Davison et al., 2006; Yin et al., 2008; Table 2). For examples, studies of miRNA expression patterns in diverse cells and tissues have shown that miR-142, miR-155, miR-181, and miR-223 were expressed specifically in 17 malignant hematopoietic cell lines (Ramkissoon et al., 2006), and miR-1, miR-133a, and miR-206 are thought to be highly expressed in the heart and skeletal muscle (Sood et al., 2006). Through these studies, several specific miRNAs have been inferred to be important in maintaining tissue and cell identities or functions, and the expression of miRNAs may have important functions in preventing disease. Microarray data have contributed greatly to clarifying the effects of miRNA expression on disease, including the roles miRNAs play in tumors such as lung cancer, colorectal neoplasia, glioblastoma, and breast cancer (Lu et al., 2005b; Cummins et al., 2006; Esquela-Kerscher and Slack, 2006). These studies have not only demonstrated distinct miRNA expression profiles in cancer samples and normal samples, but also within various tumor types. This indicates that some miRNAs can be used as biomarkers or as useful indices for the precise diagnosis of cancers. The recently developed real-time PCR array method (Keys et al., 2010) allows a more quantitative analysis of miRNA expression than do microarrays. Because the assay does not require a large amount of RNA, which is a major benefit, it has been successfully applied to the study of samples that are difficult to obtain in large populations. In one reported example, the expression profiles of 157 miRNAs were analyzed within 35 types of primary neuroblastoma tumors (Chen and Stallings, 2007). By comparing the miRNA expression profiles obtained, the authors observed that the miRNA expression levels were substantially altered in cells in which the MYCN gene was amplified, a gene involved in the aggressive pathogenicity of neuroblastoma. This finding suggests that this gene exerts a tumorigenic effect, possibly through regulation of miRNA gene expression.
RNA-seq, a deep sequencing method, has contributed especially markedly to the identification of novel miRNAs (Creighton et al., 2009; Kong et al., 2009). The first examples of miRNA discovery using deep sequencing were reported in Arabidopsis thaliana and Caenorhabditis elegans (Lu et al., 2005a; Ruby et al., 2006). Both studies sequenced millions of small RNA reads, and in the case of C. elegans, these findings extended the number of identified miRNA sequences from 18 to 112. Moreover, software for analyzing the data obtained with deep sequencing, called “miRDeep,” was introduced and identified approximately 230 previously unreported miRNAs from C. elegans, human, and dog (Friedlander et al., 2008). Another study identified the expression patterns of 340, 303, and 205 distinct mature miRNAs from human, mouse, and rat, respectively, including 33 novel miRNAs. Expression data were obtained by deep sequencing 250 small RNA libraries from 26 different organs and cells of humans and rodents. A web-accessible database was constructed to contain these data (Landgraf et al., 2007). Deep sequencing has also been a powerful tool for the identification of miRNA expression profiles, as is the case for microarray and real-time PCR array analyses. It has been applied to a wide variety of research areas, determining specific miRNA expression patterns under a variety of conditions (Tarasov et al., 2007; Chiang et al., 2010; Shao et al., 2010; Umbach and Cullen, 2010), including during tumorigenesis (Lui et al., 2007; Kuchenbauer et al., 2008; Nygaard et al., 2009). One of these studies detected over 200 miRNAs, including 55 known miRNAs, in acute myeloid leukemia (AML)-progressing cells (Kuchenbauer et al., 2008). The large number of miRNAs expressed in AML cells and the nature of their differential expression during the progression of AML suggest that miRNA expression dictates leukemic progression.
MicroRNA expression profiles retrieved from high-throughput transcriptome analyses have been applied to various analyses performed from different perspectives. Among these, evolutionary perspectives have provided insight into the emergence and functional roles of miRNAs through their conservation in a variety of species. In an interesting example in which small RNAs, including miRNAs and piwi-interacting RNAs (piRNAs), were analyzed with a deep sequencing method, using four organisms diverged before the emergence of the Bilateria (Grimson et al., 2008). The expression of small RNAs and their machinery proteins, such as Argonaute (AGO), Piwi, and Dicer, within these species suggest the emergence of small RNA functions before the bilaterian species evolved. Mature miRNAs and miRNA precursors that differ greatly from those of the Bilateria were also detected, suggesting the dynamic evolution of small RNAs as they shaped gene expression during the evolution of the animal phyla. Christodoulou et al. (2010) focused on the tissue specificities of the ancient miRNAs, and analyzed the expression patterns of miRNAs in Platynereis dumerilii, Strongylocentrotus, and Nematostella using deep sequencing and whole-mount in situ hybridization methods. They showed that not only the expression of some of the ancient miRNAs was conserved, but also their tissue and cell specificity. This suggests that miRNA evolution, the establishment of tissue identities, and possibly the relationships between their target genes (Takane et al., 2010) were closely coupled during bilaterian evolution.
The tissue- or developmental-stage-specific expression of miRNAs also allows us to infer their epigenetic regulation (Fabbri, 2008). Several studies have examined the impact of methylation on miRNA expression. In that research, miRNA expression profiles were measured before and after treatment with 5-aza-2′-deoxycytidine and 4-phenylbutyric acid, which inhibit DNA methylation and histone deacetylase, respectively, to identify the miRNAs whose expression is affected by these treatments (Saito et al., 2006; Bandres et al., 2009). The authors profiled 313 human miRNAs in human bladder carcinoma T24 cells with and without treatment with 5-aza-2′-deoxycytidine and 4-phenylbutyric acid, and extracted 17 miRNAs with significant expression differences using microarray analysis. Among those miRNAs was miR-127, which regulates the proto-oncogene BCL6. The authors hypothesized that the epigenetic silencing of miRNAs is related to tumorigenesis because miR-127, which is expressed in normal cells, is not expressed in cancer cells. The correlation between epigenetic silencing and miRNA regulation is one of the key issues that arises when miRNA expression is discussed, and further analysis, with the high-throughput detection of methylation sites, may reveal the precise relationship between these phenomena at the systems level. A detailed understanding of the condition-specific expression of miRNAs, as described in this section, will be a vital step toward understanding the miRNA-guided gene regulatory network.
Systems-Level Approaches to Identify the mRNA Targets of miRNAs
Identifying mRNAs that are regulated by miRNAs is a critical step toward understanding the biological roles of miRNAs, because the functions of a broad range of mRNAs are regulated by miRNAs. Therefore, expression profiling of the target mRNAs of miRNAs is an important part of the systems-level analysis of miRNA functions, together with the profiling of miRNA expression. In addition to transcriptome analysis, which was described in the previous section, proteome analysis and IP-based transcriptome analysis have been used to determine the expression profiles of mRNAs targeted by miRNAs (Table 1). Transcriptome analysis was developed before the other methods and served as the foundation for high-throughput approaches to understanding miRNA functions. Because some miRNA regulation is triggered by the translational regulation of their target genes, proteome analysis has also been used to demonstrate the effects of miRNAs at the protein level (Baek et al., 2008; Selbach et al., 2008). However, a major concern common to both transcriptomic and proteomic methods is that they cannot distinguish direct and indirect targets. To resolve this problem, IP has been combined with transcriptome analysis (Beitzinger et al., 2007; Karginov et al., 2007; Hendrickson et al., 2008). By analyzing a whole population of RNA sequences that has been co-immunoprecipitated with the miRISC, mRNAs that interact directly with miRNAs can be extracted. However, with this method, the interacting miRNA sequence remains unknown because only the target mRNA can be rescued by IP. Therefore, advanced IP-based methods, which basically require an additional UV cross-linking step, have been developed (Chi et al., 2009; Hafner et al., 2010; Zisoulis et al., 2010). With these methods, it is possible to identify both the miRNA and target mRNA sequences after immunoprecipitation with the miRISC protein by adding a cross-linking step to the protocol. Because this provides us with information about both the miRNAs and target mRNAs, the interactions involving each of the expressed endogenous miRNAs can be analyzed together.
To conduct high-throughput experiments effectively, a computational approach must play a core role in every step of the analysis (Figure 1). First, even before the experimental analysis is conducted, a pre-analysis can be performed using published data. The raw experimental data should be normalized to produce a list of significant genes with any variations in their expression. These lists of genes are analyzed with different approaches, such as miRNA target prediction and functional analysis, which is performed mainly by data mining against known information. These resources could also be distributed to the researchers by submitting them to databases. Modifying and applying these steps according to the purpose of the research should provide useful data sets.
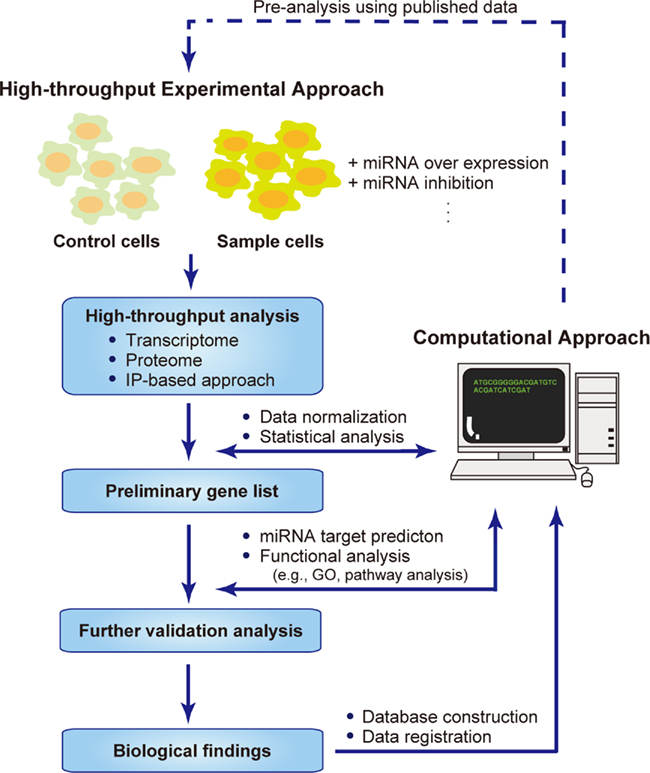
Figure 1. Systems biology approaches to identifying miRNA targets. Flow chart describing the combined high-throughput experimental approach and computational approach for the systems-level analysis of miRNA targets. Pre-analysis using published data can be performed computationally, followed by high-throughput experimental analyses, in which the samples are prepared by overexpressing or inhibiting miRNAs in a partial population, and using the untreated population as the control. The data obtained are normalized and analyzed statistically to produce a preliminary list of genes with significantly up- or down-regulated expression. Further validation analysis is conducted to extract the biological information hidden behind the mass of data. The raw and analyzed data are distributed within databases or web services, allowing other researchers to make use of this information.
Computational Approaches for miRNA Target Gene Prediction
The computational analyses of genome-wide data provides a good starting point for a systems-level understanding of the complex regulatory networks formed by miRNAs (Figure 1). Among these computational approaches, the prediction of miRNA target genes has played a critical role in identifying the biological effects of miRNAs by focusing on the genes they target. A large number of software programs have been developed for the effective prediction of miRNA target sites (Watanabe et al., 2007; Alexiou et al., 2009; Bartel, 2009).
Software for the prediction of miRNA targets mainly utilizes the features of the duplexes formed between the miRNAs and mRNAs and their evolutionary conservation. To predict miRNA targets, some software programs evaluate the accessibility of the target sites by focusing on the secondary structure of the mRNA sequence. TargetScan was the earliest software developed for miRNA target prediction and was applied to a variety of research areas (Lewis et al., 2003, 2005; Grimson et al., 2007; Friedman et al., 2009). The first version of TargetScan mainly predicted miRNA target sequences by focusing on the region complementary to bases 2–8 from the 5′ end of the miRNA (the so-called “seed region”; Lewis et al., 2003). The algorithm has been improved by considering the conservation of the seed sequence across a large variety of species and some mRNA sequence features outside the target site (Lewis et al., 2005; Grimson et al., 2007). The most recent version of TargetScan takes into account mutational biases, dinucleotide conservation rates, and the conservation rates of individual UTRs to make target prediction more effective (Friedman et al., 2009). The PicTar software also predicts miRNA target sites like TargetScan, by focusing on the evolutionary conservation of the target sequences (Krek et al., 2005). The miRanda and RNA22 softwares both focus on the binding patterns between the miRNAs and their target mRNAs (John et al., 2004; Miranda et al., 2006). However, RNA22 is quite different from the other algorithms in that it does not rely upon cross-species conservation, making it possible for the user to predict the target sites for species-specific miRNAs. Another example of a highly effective miRNA target prediction algorithm is the PITA algorithm, which tries to predict the miRNA target sequence from a different perspective (Kertesz et al., 2007). This algorithm considers target site accessibility, which is defined by the “ΔΔG score” calculated by the free energy gained in the transition from the state in which the miRNA and the target are unbound to the state in which the miRNA binds its target. Several reviews are available for more comprehensive information on miRNA prediction software (Watanabe et al., 2007; Alexiou et al., 2009; Bartel, 2009).
Which algorithm is most effective in predicting miRNA target genes? This is still an open question. One of several good approaches that try to answer this question is an analysis that determines proteomic profiles using the overexpression or knockout of miRNA target genes (Baek et al., 2008; Selbach et al., 2008). The researchers used a series of software to predict the miRNA target sites, and showed a relationship between the transcriptome and proteome data. From this analysis, the authors concluded that TargetScan and PicTar predictions correlated most strongly with their proteome data. However, the correlation between experimental and computational analyses can vary under each set of experimental conditions, and it is important to choose the most efficient software for the purpose of the research, possibly by comparing the signal-to-noise ratios of several software programs. For example, if you are analyzing the targets of miRNAs that are specific to a species, you may not want to take evolutionary conservation in account. In that case, software such as RNA22 or the more permissive version of PITA may be effective, because these algorithms do not require information about miRNA target site conservation across species. Moreover, some researchers have proposed that a combination of multiple methods is valid. For example, one study used a combination of the PITA and TargetScan algorithms to identify potential targets of miR-132, and extracted p300 as a strong candidate target gene, which they validated by further experimental analysis (Lagos et al., 2010).
Bioinformatics analyses using published “omics” data should be extremely useful for the analysis of miRNA-guided gene regulation, because of the large amount of experimental data available on various databases (van Dongen et al., 2008; Le Brigand et al., 2010; Table 3). As an example, the GenMir++ algorithm incorporates published expression profile information on miRNAs and mRNAs to effectively identify functional miRNA targets (Huang et al., 2007). It uses RNA expression data across 88 tissues and cell types and applies a Bayesian data analysis algorithm to identify a network of target predictions against 104 human miRNAs. Information can be obtained by examining the overall trends in various data sets, rather than by focusing on limited observations. This is exemplified by a study in which 151 published transfection experiments in seven different human cell types were examined to determine the effects of the transfection of small RNAs on the transcriptome, and in which the competition and saturation effects of small RNA transfections on endogenous miRNAs were discussed (Khan et al., 2009). Meanwhile, some problems can occur when handling or integrating experimental data from databases, mainly because of variations among the experimental platforms or conditions used. Therefore, it is important to construct a consensus platform for high-throughput experiments to ensure the best use of the data sets available from databases.
The computational predictions discussed here are useful tools for identifying large numbers of miRNA–mRNA interactions, although there is still substantial concern about their reliability. Different algorithms provide different predictions, and the degree of overlap is sometimes very low (Sethupathy et al., 2006). Therefore, integrating these computational approaches with high-throughput experiments, as described below, should be a very powerful approach to identifying miRNA-mediated gene regulation.
miRNA Target Detection Using Transcriptome and Proteome Analyses
Because transcriptome analyses can detect the expression patterns of mRNAs under different conditions, comparison of these expression profiles when a specific miRNA is overexpressed or inhibited has been used for the functional analysis of that miRNA. The most common methodology used to modify miRNA expression is its overexpression with synthetic miRNA mimics or expression vectors (Lim et al., 2005; Grimson et al., 2007; Linsley et al., 2007; Table 3). In one study of miRNA overexpression, the introduction of miR-1 (expressed in muscle) and miR-124a (expressed in brain), to HeLa cells induced a shift in the expression patterns of mRNAs patterns similar to those observed in the tissues that endogenously express miR-1 and miR-124a (Lim et al., 2005). In another study, different types of miRNAs were transfected individually or in combinations and the mRNA expression profiles were analyzed, demonstrating that miRNAs sharing the same seed sequence with miR-16 targeted the same transcripts, and negatively regulated cellular growth and cell-cycle progression (Linsley et al., 2007). To inhibit miRNA expression, chemically engineered anti-miRNA oligonucleotides, such as antagomirs, lock nucleic acids, or small molecule inhibitors (Krutzfeldt et al., 2005; Orom et al., 2006; Zhang et al., 2010b), have been used in high-throughput studies to analyze the effects of miRNAs on the transcriptome (Krutzfeldt et al., 2005; Nakamoto et al., 2005). Some applications in animal models have also indicated that these inhibitors are promising candidates for the development of miRNA-based therapies (Lanford et al., 2010; Ma et al., 2010). One study showed that the inhibition of miR-122, which is essential for hepatitis C virus (HCV) RNA replication (Lanford et al., 2010), led to the long-lasting suppression of HCV viremia in chimpanzees. The authors analyzed the impact of this phenomenon on the transcriptome using microarray and deep sequencing expression profiling analyses, which suggested the de-repression of target mRNAs containing miR-122 seed sites and the downregulation of interferon-regulated gene expression by miR-122 inhibition. The other option available for the analysis of miRNA-inhibited conditions is the use of animal models that are deficient in specific miRNAs or the genes involved in miRNA biogenesis (Giraldez et al., 2006; Rodriguez et al., 2007; Baek et al., 2008). For example, a transcriptome analysis was performed in bic/miR-155-deficient CD4+ T cells and identified a wide spectrum of miR-155-affected genes. This provided an overview of miR-155 functions during homeostasis and in the functioning of the immune system (Rodriguez et al., 2007). Other studies have examined both the overexpression and inhibition of miRNAs (Nicolas et al., 2008; Ziegelbauer et al., 2009). The researchers used these experimental data to estimate the numbers of direct miRNA targets by focusing on the genes commonly regulated under these two conditions. Their results showed that only 49 genes were commonly regulated under both conditions, whereas 1,236 and 466 genes were regulated within miR-140-overexpressing and miR-140-inhibited cells, respectively. This suggests the existence of large numbers of indirect or false-positive targets detected within this series of experiments.
A number of miRNA-based regulatory events are known to occur at the translation level (He and Hannon, 2004), and in this context, proteomic analysis has been used to identify miRNA targets (Vinther et al., 2006; Baek et al., 2008; Selbach et al., 2008; Tian et al., 2008; Table 3). Stable isotope labeling with amino acids in cell culture (SILAC) of miRNA expression-modified cells, followed by mass spectrometric analysis, can be used to assess the effects of the loss or overexpression of miRNAs on global protein expression (Figure 2). Changes in mRNA expression correlated well with those in protein expression and suggested their utility in the detection of miRNA target genes (Baek et al., 2008; Selbach et al., 2008). However, the large variability in protein expression levels makes it difficult to resolve the whole proteome simultaneously with current methods, with a proportion of the protein expression data undetected. A recent report showed that over 84% of the reduced protein production mediated by miRNAs is attributable to reduced mRNA levels (Guo et al., 2010). This suggests that changes in mRNA levels closely reflect the impact of miRNAs on gene expression, and that the destabilization of target mRNAs explains the reduced protein output. This finding has inspired active discussions of the gene regulatory mechanisms of miRNAs, specifically whether the expression of target mRNAs is reduced translationally or transcriptionally. The common problem shared with transcriptome analysis is that this methodology cannot distinguish the direct and indirect regulation of miRNAs.
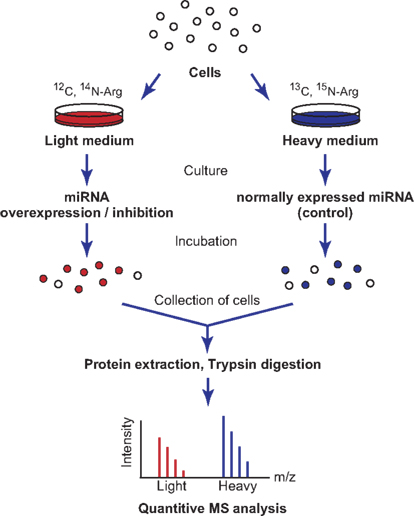
Figure 2. Schematic representation of SILAC labeling and proteome analysis. Cells are split and cultured in heavy or light medium containing different amino acid isotopes. The miRNAs are then overexpressed or inhibited within these cells, and the cells are incubated for several more hours. The cells are collected and their proteins are purified for further mass spectrometric analysis. The protein levels in the two samples are compared by quantifying the heavy and light peptides, because isotopic labeling will affect their migration times.
IP- and Cross-Linking and Immunoprecipitation-Based Analyses Identify miRNA–mRNA Interactions
To understand the miRNA–mRNA interactome with higher resolution, it is important to distinguish direct and indirect regulation by miRNAs. To do this, some approaches have combined IP with transcriptome analysis (Easow et al., 2007; Zhang et al., 2007; Hausser et al., 2009; Hendrickson et al., 2009; Table 3). IP is performed with antibodies that target components of miRISC, such as AGO proteins and TNRC6A-C, to pull down the RNA sequences combined within this complex (Hafner et al., 2010). Those RNA sequences are identified using a microarray or deep sequencing.
As an example, a research group analyzed the functions of miR-124a by overexpressing and inhibiting this miRNA in HEK293 cells and immunoprecipitated epitope-tagged AGO2. The results showed the significant enrichment of 294 mRNAs, including several known targets of miR-124a. They also performed a luciferase assay using the 3′ UTR sequences of extracted candidates, and successfully validated 21 of the 30 tested targets (Karginov et al., 2007). The modes of action of miRNAs were analyzed as another example of the capacity of the IP-based approach. For instance, variations in an AGO1- or AGO2-bound target mRNA population was shown in humans. The authors showed that only a limited number of sequences are common to the AGO1 and AGO2 co-immunoprecipitated mRNAs, suggesting that many miRNA targets are specific for one or other AGO protein (Beitzinger et al., 2007). Further analysis showed redundant overlaps between the miRNA targets incorporated within different AGOs, suggesting partial overlaps between the AGO functions (Landthaler et al., 2008), but discussion of this issue is ongoing. IP-based methods are more effective in miRNA target identification compared to previously developed approaches (Karginov et al., 2007; Hendrickson et al., 2008). Although, the remaining difficulty in these approaches is that they cannot identify which miRNAs target the mRNAs enriched by co-IP with the miRISC protein. Using computational approach, miRNA target prediction has been applied to IP-based analyses, as in case of mirWIP algorithm. It predicts miRNA target genes using both the characteristics of the miRNA–mRNA duplex and information about the IP-enriched RNA sequences, and successfully detected miRNA–mRNA target relationships in C. elegans with high sensitivity and specificity compared to the other miRNA target prediction algorithms (Hammell et al., 2008). Moreover, to overcome this problem using experimental methods, improved IP-based methods have been introduced.
The key improvement on the IP-based methodology for miRNA target detection has been achieved by adding a cross-linking step to the protocol, in the processes of high-throughput sequencing by cross-linking and immunoprecipitation (HITS–CLIP; Chi et al., 2009), cross-linking and immunoprecipitation coupled to high-throughput sequencing (CLIP-seq; Zisoulis et al., 2010), and photoactivatable-ribonucleoside-enhanced cross-linking and immunoprecipitation (PAR-CLIP; Hafner et al., 2010). These methods directly identify miRNA–mRNA duplexes by IP. Unbound RNAs are digested, leaving the miRISC-protected RNA fragments, which are analyzed by high-throughput RNA sequencing to identify both the AGO-associated miRNAs and their targets. In PAR-CLIP, RNA recovery improved 100- to 1,000-fold when 4-thiouridine nucleosides were used. The reverse transcription of 4-thiouridine nucleotides leads to T → C transitions, which improved the accurate identification of the miRISC-interaction sites within the detected RNA sequences (Figure 3). From all the AGO IP experiments, 17,319 clusters of sequences were successfully identified (Hafner et al., 2010). Another interesting finding of this research was that about 50% of the detected miRNA-binding sites were located in the coding sequence (CDS). This suggests that gene expression is potentially regulated within the CDS region, although it has also been shown that the regulation induced by binding sites in the CDS is not as significant as that induced in the 3′ UTR. This method was a breakthrough in detecting the direct interactions between miRNAs and their target genes in a high-throughput manner.
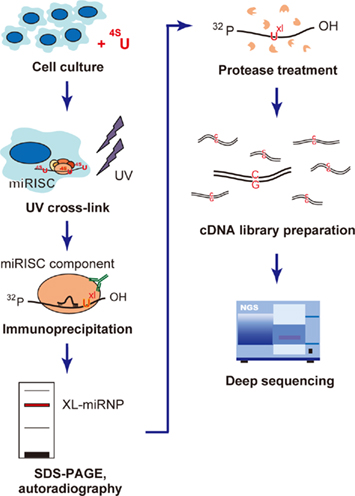
Figure 3. Flow chart of the photoactivatable-ribonucleoside- enhanced cross-linking and immunoprecipitation (PAR-CLIP) methodology. PAR-CLIP analysis of miRISC component-binding RNAs. The cells are first cultured with photoreactive 4-thiouridine (4SU), which causes uridine to be incorporated during culture, and UV cross-linked to miRNP (UXL). The cross-linked miRNP–RNA complexes are immunoprecipitated using an antibody directed against miRNP, and then size fractionized by SDS-PAGE. The miRNP–RNA complexes are extracted from the gel and digested with protease. The recovered RNA molecules are converted into cDNA, where the incorporated 4-thiouridine causes T → C transitions. This transition plays a key role in the accurate mapping of the miRNP-binding sites. The cDNA library is analyzed with the deep sequencing method to determine the RNA sequences capable of interacting with miRNP.
Systems-Level Analyses Reveal miRNA Functions in Gene Regulatory Networks
Integrating computational approaches with high-throughput experiments should provide a long list of potential miRNA target genes, and the next critical step will be the identification of their biological functions using that list. Because miRNAs have numerous target genes, with an average of 300 conserved targets per miRNA family (Bartel, 2009), it is conceivable that their interactive network, including their target genes, is enormous. It is also highly likely that miRNAs interact with a variety of genes that are functionally related to each other, playing essential roles as the “hubs” of gene regulatory networks and/or biological pathways (Ke et al., 2003; Herranz and Cohen, 2010). These functional analyses have predominantly been performed with data mining tools and databases (Figure 1), and have proven effective in extracting lists of the potential mRNA targets involved in a specific signal transduction pathway or biological function. Furthermore, the roles of miRNAs demonstrated within these pathways and functions have suggested that miRNAs can regulate positive or negative feedback systems, and this research has provided insight into how miRNAs play very specific roles in biological regulation (Herranz and Cohen, 2010).
One direct approach to the analysis of miRNA functions within specific instances of biological regulation should be to map miRNA interactions to known protein–protein interactions (PPIs). Several databases of known PPIs, such as the database of interacting proteins (DIP; Xenarios et al., 2000) and the mammalian protein–protein interaction database (MPPI; Mewes et al., 2004), are good resources for these analyses, and PPI networks can be visualized together with miRNA interaction information on a platform such as Cytoscape (Cline et al., 2007). Another approach will be to map miRNA interactions to known biological pathways. The Kyoto encyclopedia of genes and genomes (KEGG) pathway (Ogata et al., 1999) is a good reference for groups of miRNA target genes that may share molecular interactions and reaction networks. This database provides biological pathway information, allowing us to determine whether groups of miRNA target genes are enriched in specific biological pathways. To determine the common functions of miRNA target genes and to connect miRNA gene regulatory pathways to PPI networks or biological pathways, functional annotation by gene ontology (GO) is commonly used. GO provides a controlled vocabulary or systematic language for the description of the attributes of genes and gene products, which can be used across genomic databases (Ashburner et al., 2000). This systematic ontology acts as a key tool to annotate common functions within large clusters of genes, as in the case of predicting miRNA targets (Enright et al., 2003; Shalgi et al., 2007), and provides clues to the interactions or pathways in which miRNAs are involved.
A number of studies have used these data mining tools to analyze the specific biological functions regulated by miRNAs (Liang and Li, 2007; Neilson et al., 2007; Bonci et al., 2008; Sarachana et al., 2010; Zhang et al., 2010a). For example, in one approach, GO analysis was used together with KEGG pathway analysis to determine the roles of 21 miRNAs shown to be expressed in hepatic stellate cells (HSCs) in an miRNA microarray analysis (Guo et al., 2009). These results suggested that apoptosis was the most enriched transduction pathway when the top 25% of the computationally predicted miRNA targets were analyzed. Another example involved the analysis of the functions of the target genes of miR-24 (Lal et al., 2009a, b). Potential miRNA target genes were identified experimentally using microarray analysis, by detecting significantly downregulated genes among miR-24 transfection with potential miR-24 binding site. The functions of the potential miR-24 target genes were analyzed using GO analysis and the Ingenuity Pathways software. This approach suggested that miR-24 regulates cell-cycle progression and DNA repair. As shown in these cases, computational prediction is a valuable tool for identifying miRNA gene networks under physiological and pathological conditions. Other studies have suggested the miRNA-guided regulation of transcription factors, which underlines the key functions of miRNAs within whole gene regulatory networks (Marson et al., 2008; Ragusa et al., 2010; Osella et al., 2011; Ponomarev et al., 2011; Schlesinger et al., 2011; Starnes and Sorrentino, 2011). These analyses suggest that the overrepresentation of miRNAs within gene regulatory networks may reflect their essential roles in the mediation of feedback and feedforward regulation in cellular systems and the maintenance of cellular stability during environmental perturbation (Herranz and Cohen, 2010).
Conclusion
Using high-throughput experiments, we have retrieved large amounts of data containing a variety of information, including the expression profiles of miRNAs and/or mRNAs. This will allow us to identify and compare whole gene expression profiles in a systematic way under different biological conditions: in cells or tissues, at different stages of development, and in physiological or pathological states. Although the primary data obtained are a treasure trove, including much interesting information, it is also true that a large proportion of these are false-positive or insignificant information. The computational approach has played a key role in extracting lists of genes that warrant further analysis. These lists can be compared with one another, combined together to identify tendencies, incorporated with other information obtained from public databases, and so on. Using computational approaches, and in this way, a variety of biological knowledge can be extracted from an overview of these phenomena. The need to look at whole gene expression patterns is especially true for complex biological functions, such as those of miRNAs, where the target mRNAs are regulated in a one-to-many and a many-to-one manner and the degree of regulation varies case by case. When accumulated miRNA–mRNA interactions identify biological functions, it will be necessary to look at those interactions comprehensively and recognize them as part of a gene regulatory network. Therefore, we suggest that further weight should be given to high-throughput analyses combined with computational approaches, as an effective methodology to achieve a systems-level understanding of complex biological functions.
Conflict of Interest Statement
The authors declare that the research was conducted in the absence of any commercial or financial relationships that could be construed as a potential conflict of interest.
Acknowledgments
The authors would like to thank Kahori Takane (Keio University, Japan) for critical reading of this paper, and the members of the RNA group at the Institute for Advanced Biosciences, Keio University, for their helpful discussions. Y. W. was supported by a Grant-in-Aid from the Japan Society for the Promotion of Science. This work was also supported by research funds from the Yamagata Government and Tsuruoka City, Japan.
References
Alexiou, P., Maragkakis, M., Papadopoulos, G. L., Reczko, M., and Hatzigeorgiou, A. G. (2009). Lost in translation: an assessment and perspective for computational microRNA target identification. Bioinformatics 25, 3049–3055.
Ambros, V., Bartel, B., Bartel, D. P., Burge, C. B., Carrington, J. C., Chen, X., Dreyfuss, G., Eddy, S. R., Griffiths-Jones, S., Marshall, M., Matzke, M., Ruvkun, G., and Tuschl, T. (2003). A uniform system for microRNA annotation. RNA 9, 277–279.
Ashburner, M., Ball, C. A., Blake, J. A., Botstein, D., Butler, H., Cherry, J. M., Davis, A. P., Dolinski, K., Dwight, S. S., Eppig, J. T., Harris, M. A., Hill, D. P., Issel-Tarver, L., Kasarskis, A., Lewis, S., Matese, J. C., Richardson, J. E., Ringwald, M., Rubin, G. M., and Sherlock, G. (2000). Gene ontology: tool for the unification of biology. The gene ontology consortium. Nat. Genet. 25, 25–29.
Baek, D., Villen, J., Shin, C., Camargo, F. D., Gygi, S. P., and Bartel, D. P. (2008). The impact of microRNAs on protein output. Nature 455, 64–71.
Bandres, E., Agirre, X., Bitarte, N., Ramirez, N., Zarate, R., Roman-Gomez, J., Prosper, F., and Garcia-Foncillas, J. (2009). Epigenetic regulation of microRNA expression in colorectal cancer. Int. J. Cancer 125, 2737–2743.
Bartel, D. P., and Chen, C. Z. (2004). Micromanagers of gene expression: the potentially widespread influence of metazoan microRNAs. Nat. Rev. Genet. 5, 396–400.
Beitzinger, M., Peters, L., Zhu, J. Y., Kremmer, E., and Meister, G. (2007). Identification of human microRNA targets from isolated argonaute protein complexes. RNA Biol. 4, 76–84.
Bonci, D., Coppola, V., Musumeci, M., Addario, A., Giuffrida, R., Memeo, L., D’urso, L., Pagliuca, A., Biffoni, M., Labbaye, C., Bartucci, M., Muto, G., Peschle, C., and De Maria, R. (2008). The miR-15a-miR-16-1 cluster controls prostate cancer by targeting multiple oncogenic activities. Nat. Med. 14, 1271–1277.
Bravo, V., Rosero, S., Ricordi, C., and Pastori, R. L. (2007). Instability of miRNA and cDNAs derivatives in RNA preparations. Biochem. Biophys. Res. Commun. 353, 1052–1055.
Chen, Y., Gelfond, J. A., Mcmanus, L. M., and Shireman, P. K. (2009). Reproducibility of quantitative RT-PCR array in miRNA expression profiling and comparison with microarray analysis. BMC Genomics 10, 407. doi: 10.1186/1471-2164-10-407
Chen, Y., and Stallings, R. L. (2007). Differential patterns of microRNA expression in neuroblastoma are correlated with prognosis, differentiation, and apoptosis. Cancer Res. 67, 976–983.
Chi, S. W., Zang, J. B., Mele, A., and Darnell, R. B. (2009). Argonaute HITS-CLIP decodes microRNA-mRNA interaction maps. Nature 460, 479–486.
Chiang, H. R., Schoenfeld, L. W., Ruby, J. G., Auyeung, V. C., Spies, N., Baek, D., Johnston, W. K., Russ, C., Luo, S., Babiarz, J. E., Blelloch, R., Schroth, G. P., Nusbaum, C., and Bartel, D. P. (2010). Mammalian microRNAs: experimental evaluation of novel and previously annotated genes. Genes Dev. 24, 992–1009.
Christodoulou, F., Raible, F., Tomer, R., Simakov, O., Trachana, K., Klaus, S., Snyman, H., Hannon, G. J., Bork, P., and Arendt, D. (2010). Ancient animal microRNAs and the evolution of tissue identity. Nature 463, 1084–1088.
Cline, M. S., Smoot, M., Cerami, E., Kuchinsky, A., Landys, N., Workman, C., Christmas, R., Avila-Campilo, I., Creech, M., Gross, B., Hanspers, K., Isserlin, R., Kelley, R., Killcoyne, S., Lotia, S., Maere, S., Morris, J., Ono, K., Pavlovic, V., Pico, A. R., Vailaya, A., Wang, P. L., Adler, A., Conklin, B. R., Hood, L., Kuiper, M., Sander, C., Schmulevich, I., Schwikowski, B., Warner, G. J., Ideker, T., and Bader, G. D. (2007). Integration of biological networks and gene expression data using Cytoscape. Nat. Protoc. 2, 2366–2382.
Creighton, C. J., Reid, J. G., and Gunaratne, P. H. (2009). Expression profiling of microRNAs by deep sequencing. Brief. Bioinformatics 10, 490–497.
Cummins, J. M., He, Y., Leary, R. J., Pagliarini, R., Diaz, L. A. Jr., Sjoblom, T., Barad, O., Bentwich, Z., Szafranska, A. E., Labourier, E., Raymond, C. K., Roberts, B. S., Juhl, H., Kinzler, K. W., Vogelstein, B., and Velculescu, V. E. (2006). The colorectal microRNAome. Proc. Natl. Acad. Sci. U.S.A. 103, 3687–3692.
Davison, T. S., Johnson, C. D., and Andruss, B. F. (2006). Analyzing micro-RNA expression using microarrays. Meth. Enzymol. 411, 14–34.
Easow, G., Teleman, A. A., and Cohen, S. M. (2007). Isolation of microRNA targets by miRNP immunopurification. RNA 13, 1198–1204.
Enright, A. J., John, B., Gaul, U., Tuschl, T., Sander, C., and Marks, D. S. (2003). MicroRNA targets in Drosophila. Genome Biol. 5, R1.
Esquela-Kerscher, A., and Slack, F. J. (2006). Oncomirs – microRNAs with a role in cancer. Nat. Rev. Cancer 6, 259–269.
Friedlander, M. R., Chen, W., Adamidi, C., Maaskola, J., Einspanier, R., Knespel, S., and Rajewsky, N. (2008). Discovering microRNAs from deep sequencing data using miRDeep. Nat. Biotechnol. 26, 407–415.
Friedman, R. C., Farh, K. K., Burge, C. B., and Bartel, D. P. (2009). Most mammalian mRNAs are conserved targets of microRNAs. Genome Res. 19, 92–105.
Giraldez, A. J., Cinalli, R. M., Glasner, M. E., Enright, A. J., Thomson, J. M., Baskerville, S., Hammond, S. M., Bartel, D. P., and Schier, A. F. (2005). MicroRNAs regulate brain morphogenesis in zebrafish. Science 308, 833–838.
Giraldez, A. J., Mishima, Y., Rihel, J., Grocock, R. J., van Dongen, S., Inoue, K., Enright, A. J., and Schier, A. F. (2006). Zebrafish MiR-430 promotes deadenylation and clearance of maternal mRNAs. Science 312, 75–79.
Griffiths-Jones, S., Saini, H. K., van Dongen, S., and Enright, A. J. (2008). miRBase: tools for microRNA genomics. Nucleic Acids Res. 36, D154–D158.
Grimson, A., Farh, K. K., Johnston, W. K., Garrett-Engele, P., Lim, L. P., and Bartel, D. P. (2007). MicroRNA targeting specificity in mammals: determinants beyond seed pairing. Mol. Cell 27, 91–105.
Grimson, A., Srivastava, M., Fahey, B., Woodcroft, B. J., Chiang, H. R., King, N., Degnan, B. M., Rokhsar, D. S., and Bartel, D. P. (2008). Early origins and evolution of microRNAs and Piwi-interacting RNAs in animals. Nature 455, 1193–1197.
Guo, C. J., Pan, Q., Cheng, T., Jiang, B., Chen, G. Y., and Li, D. G. (2009). Changes in microRNAs associated with hepatic stellate cell activation status identify signaling pathways. FEBS J. 276, 5163–5176.
Guo, H., Ingolia, N. T., Weissman, J. S., and Bartel, D. P. (2010). Mammalian microRNAs predominantly act to decrease target mRNA levels. Nature 466, 835–840.
Hafner, M., Landthaler, M., Burger, L., Khorshid, M., Hausser, J., Berninger, P., Rothballer, A., Ascano, M. Jr., Jungkamp, A. C., Munschauer, M., Ulrich, A., Wardle, G. S., Dewell, S., Zavolan, M., and Tuschl, T. (2010). Transcriptome-wide identification of RNA-binding protein and microRNA target sites by PAR-CLIP. Cell 141, 129–141.
Hammell, M., Long, D., Zhang, L., Lee, A., Carmack, C. S., Han, M., Ding, Y., and Ambros, V. (2008). mirWIP: microRNA target prediction based on microRNA-containing ribonucleoprotein-enriched transcripts. Nat. Methods 5, 813–819.
Hausser, J., Landthaler, M., Jaskiewicz, L., Gaidatzis, D., and Zavolan, M. (2009). Relative contribution of sequence and structure features to the mRNA binding of argonaute/EIF2C-miRNA complexes and the degradation of miRNA targets. Genome Res. 19, 2009–2020.
He, L., and Hannon, G. J. (2004). MicroRNAs: small RNAs with a big role in gene regulation. Nat. Rev. Genet. 5, 522–531.
Hendrickson, D. G., Hogan, D. J., Herschlag, D., Ferrell, J. E., and Brown, P. O. (2008). Systematic identification of mRNAs recruited to argonaute 2 by specific microRNAs and corresponding changes in transcript abundance. PLoS ONE 3, e2126. doi:10.1371/journal.pone.0002126
Hendrickson, D. G., Hogan, D. J., Mccullough, H. L., Myers, J. W., Herschlag, D., Ferrell, J. E., and Brown, P. O. (2009). Concordant regulation of translation and mRNA abundance for hundreds of targets of a human microRNA. PLoS Biol. 7, e1000238. doi: 10.1371/journal.pbio.1000238
Herranz, H., and Cohen, S. M. (2010). MicroRNAs and gene regulatory networks: managing the impact of noise in biological systems. Genes Dev. 24, 1339–1344.
Huang, J. C., Babak, T., Corson, T. W., Chua, G., Khan, S., Gallie, B. L., Hughes, T. R., Blencowe, B. J., Frey, B. J., and Morris, D. (2007). Using expression profiling data to identify human microRNA targets. Nat. Methods 4, 1045–1049.
Jiang, J., Lee, E. J., Gusev, Y., and Schmittgen, T. D. (2005). Real-time expression profiling of microRNA precursors in human cancer cell lines. Nucleic Acids Res. 33, 5394–5403.
John, B., Enright, A. J., Aravin, A., Tuschl, T., Sander, C., and Marks, D. S. (2004). Human MicroRNA targets. PLoS Biol. 2, e363. doi: 10.1371/journal.pbio.0020363
Karginov, F. V., Conaco, C., Xuan, Z., Schmidt, B. H., Parker, J. S., Mandel, G., and Hannon, G. J. (2007a). A biochemical approach to identifying microRNA targets. Proc. Natl. Acad. Sci. U.S.A. 104, 19291–19296.
Ke, X. S., Liu, C. M., Liu, D. P., and Liang, C. C. (2003). MicroRNAs: key participants in gene regulatory networks. Curr. Opin. Chem. Biol. 7, 516–523.
Kertesz, M., Iovino, N., Unnerstall, U., Gaul, U., and Segal, E. (2007). The role of site accessibility in microRNA target recognition. Nat. Genet. 39, 1278–1284.
Keys, D. N., Au-Young, J. K., and Fekete, R. A. (2010). TaqMan array cards in pharmaceutical research. Methods Mol. Biol. 632, 87–97.
Khan, A. A., Betel, D., Miller, M. L., Sander, C., Leslie, C. S., and Marks, D. S. (2009). Transfection of small RNAs globally perturbs gene regulation by endogenous microRNAs. Nat. Biotechnol. 27, 549–555.
Kloosterman, W. P., and Plasterk, R. H. (2006). The diverse functions of microRNAs in animal development and disease. Dev. Cell 11, 441–450.
Kong, W., Zhao, J. J., He, L., and Cheng, J. Q. (2009). Strategies for profiling microRNA expression. J. Cell. Physiol. 218, 22–25.
Krek, A., Grun, D., Poy, M. N., Wolf, R., Rosenberg, L., Epstein, E. J., Macmenamin, P., Da Piedade, I., Gunsalus, K. C., Stoffel, M., and Rajewsky, N. (2005). Combinatorial microRNA target predictions. Nat. Genet. 37, 495–500.
Krutzfeldt, J., Rajewsky, N., Braich, R., Rajeev, K. G., Tuschl, T., Manoharan, M., and Stoffel, M. (2005). Silencing of microRNAs in vivo with “antagomirs”. Nature 438, 685–689.
Kuchenbauer, F., Morin, R. D., Argiropoulos, B., Petriv, O. I., Griffith, M., Heuser, M., Yung, E., Piper, J., Delaney, A., Prabhu, A. L., Zhao, Y., Mcdonald, H., Zeng, T., Hirst, M., Hansen, C. L., Marra, M. A., and Humphries, R. K. (2008). In-depth characterization of the microRNA transcriptome in a leukemia progression model. Genome Res. 18, 1787–1797.
Lagos, D., Pollara, G., Henderson, S., Gratrix, F., Fabani, M., Milne, R. S., Gotch, F., and Boshoff, C. (2010). miR-132 regulates antiviral innate immunity through suppression of the p300 transcriptional co-activator. Nat. Cell Biol. 12, 513–519.
Lal, A., Navarro, F., Maher, C. A., Maliszewski, L. E., Yan, N., O’day, E., Chowdhury, D., Dykxhoorn, D. M., Tsai, P., Hofmann, O., Becker, K. G., Gorospe, M., Hide, W., and Lieberman, J. (2009a). miR-24 Inhibits cell proliferation by targeting E2F2, MYC, and other cell-cycle genes via binding to “seedless” 3′UTR microRNA recognition elements. Mol. Cell 35, 610–625.
Lal, A., Pan, Y., Navarro, F., Dykxhoorn, D. M., Moreau, L., Meire, E., Bentwich, Z., Lieberman, J., and Chowdhury, D. (2009b). miR-24-mediated downregulation of H2AX suppresses DNA repair in terminally differentiated blood cells. Nat. Struct. Mol. Biol. 16, 492–498.
Landgraf, P., Rusu, M., Sheridan, R., Sewer, A., Iovino, N., Aravin, A., Pfeffer, S., Rice, A., Kamphorst, A. O., Landthaler, M., Lin, C., Socci, N. D., Hermida, L., Fulci, V., Chiaretti, S., Foa, R., Schliwka, J., Fuchs, U., Novosel, A., Muller, R. U., Schermer, B., Bissels, U., Inman, J., Phan, Q., Chien, M., Weir, D. B., Choksi, R., De Vita, G., Frezzetti, D., Trompeter, H. I., Hornung, V., Teng, G., Hartmann, G., Palkovits, M., Di Lauro, R., Wernet, P., Macino, G., Rogler, C. E., Nagle, J. W., Ju, J., Papavasiliou, F. N., Benzing, T., Lichter, P., Tam, W., Brownstein, M. J., Bosio, A., Borkhardt, A., Russo, J. J., Sander, C., Zavolan, M., and Tuschl, T. (2007). A mammalian microRNA expression atlas based on small RNA library sequencing. Cell 129, 1401–1414.
Landthaler, M., Gaidatzis, D., Rothballer, A., Chen, P. Y., Soll, S. J., Dinic, L., Ojo, T., Hafner, M., Zavolan, M., and Tuschl, T. (2008). Molecular characterization of human argonaute-containing ribonucleoprotein complexes and their bound target mRNAs. RNA 14, 2580–2596.
Lanford, R. E., Hildebrandt-Eriksen, E. S., Petri, A., Persson, R., Lindow, M., Munk, M. E., Kauppinen, S., and Orum, H. (2010). Therapeutic silencing of microRNA-122 in primates with chronic hepatitis C virus infection. Science 327, 198–201.
Le Brigand, K., Robbe-Sermesant, K., Mari, B., and Barbry, P. (2010). MiRonTop: mining microRNAs targets across large scale gene expression studies. Bioinformatics 26, 3131–3132.
Lewis, B. P., Burge, C. B., and Bartel, D. P. (2005). Conserved seed pairing, often flanked by adenosines, indicates that thousands of human genes are microRNA targets. Cell 120, 15–20.
Lewis, B. P., Shih, I. H., Jones-Rhoades, M. W., Bartel, D. P., and Burge, C. B. (2003). Prediction of mammalian microRNA targets. Cell 115, 787–798.
Liang, H., and Li, W. H. (2007). MicroRNA regulation of human protein protein interaction network. RNA 13, 1402–1408.
Lim, L. P., Lau, N. C., Garrett-Engele, P., Grimson, A., Schelter, J. M., Castle, J., Bartel, D. P., Linsley, P. S., and Johnson, J. M. (2005). Microarray analysis shows that some microRNAs downregulate large numbers of target mRNAs. Nature 433, 769–773.
Linsley, P. S., Schelter, J., Burchard, J., Kibukawa, M., Martin, M. M., Bartz, S. R., Johnson, J. M., Cummins, J. M., Raymond, C. K., Dai, H., Chau, N., Cleary, M., Jackson, A. L., Carleton, M., and Lim, L. (2007). Transcripts targeted by the microRNA-16 family cooperatively regulate cell cycle progression. Mol. Cell. Biol. 27, 2240–2252.
Lu, C., Tej, S. S., Luo, S., Haudenschild, C. D., Meyers, B. C., and Green, P. J. (2005a). Elucidation of the small RNA component of the transcriptome. Science 309, 1567–1569.
Lu, J., Getz, G., Miska, E. A., Alvarez-Saavedra, E., Lamb, J., Peck, D., Sweet-Cordero, A., Ebert, B. L., Mak, R. H., Ferrando, A. A., Downing, J. R., Jacks, T., Horvitz, H. R., and Golub, T. R. (2005b). MicroRNA expression profiles classify human cancers. Nature 435, 834–838.
Lui, W. O., Pourmand, N., Patterson, B. K., and Fire, A. (2007). Patterns of known and novel small RNAs in human cervical cancer. Cancer Res. 67, 6031–6043.
Ma, L., Reinhardt, F., Pan, E., Soutschek, J., Bhat, B., Marcusson, E. G., Teruya-Feldstein, J., Bell, G. W., and Weinberg, R. A. (2010). Therapeutic silencing of miR-10b inhibits metastasis in a mouse mammary tumor model. Nat. Biotechnol. 28, 341–347.
Marson, A., Levine, S. S., Cole, M. F., Frampton, G. M., Brambrink, T., Johnstone, S., Guenther, M. G., Johnston, W. K., Wernig, M., Newman, J., Calabrese, J. M., Dennis, L. M., Volkert, T. L., Gupta, S., Love, J., Hannett, N., Sharp, P. A., Bartel, D. P., Jaenisch, R., and Young, R. A. (2008). Connecting microRNA genes to the core transcriptional regulatory circuitry of embryonic stem cells. Cell 134, 521–533.
Mewes, H. W., Amid, C., Arnold, R., Frishman, D., Guldener, U., Mannhaupt, G., Munsterkotter, M., Pagel, P., Strack, N., Stumpflen, V., Warfsmann, J., and Ruepp, A. (2004). MIPS: analysis and annotation of proteins from whole genomes. Nucleic Acids Res. 32, D41–44.
Miranda, K. C., Huynh, T., Tay, Y., Ang, Y. S., Tam, W. L., Thomson, A. M., Lim, B., and Rigoutsos, I. (2006). A pattern-based method for the identification of microRNA binding sites and their corresponding heteroduplexes. Cell 126, 1203–1217.
Miska, E. A. (2005). How microRNAs control cell division, differentiation and death. Curr. Opin. Genet. Dev. 15, 563–568.
Nakamoto, M., Jin, P., O’donnell, W. T., and Warren, S. T. (2005). Physiological identification of human transcripts translationally regulated by a specific microRNA. Hum. Mol. Genet. 14, 3813–3821.
Neilson, J. R., Zheng, G. X., Burge, C. B., and Sharp, P. A. (2007). Dynamic regulation of miRNA expression in ordered stages of cellular development. Genes Dev. 21, 578–589.
Nicolas, F. E., Pais, H., Schwach, F., Lindow, M., Kauppinen, S., Moulton, V., and Dalmay, T. (2008). Experimental identification of microRNA-140 targets by silencing and overexpressing miR-140. RNA 14, 2513–2520.
Nygaard, S., Jacobsen, A., Lindow, M., Eriksen, J., Balslev, E., Flyger, H., Tolstrup, N., Moller, S., Krogh, A., and Litman, T. (2009). Identification and analysis of miRNAs in human breast cancer and teratoma samples using deep sequencing. BMC Med. Genomics 2, 35. doi:10.1186/1755-8794-2-35
Ogata, H., Goto, S., Sato, K., Fujibuchi, W., Bono, H., and Kanehisa, M. (1999). KEGG: Kyoto encyclopedia of genes and genomes. Nucleic Acids Res. 27, 29–34.
Orom, U. A., Kauppinen, S., and Lund, A. H. (2006). LNA-modified oligonucleotides mediate specific inhibition of microRNA function. Gene 372, 137–141.
Osella, M., Bosia, C., Cora, D., and Caselle, M. (2011). The role of incoherent microRNA-mediated feedforward loops in noise buffering. PLoS Comput. Biol. 7, e1001101. doi:10.1186/1755-8794-2-35
Ponomarev, E. D., Veremeyko, T., Barteneva, N., Krichevsky, A. M., and Weiner, H. L. (2011). MicroRNA-124 promotes microglia quiescence and suppresses EAE by deactivating macrophages via the C/EBP-alpha-PU.1 pathway. Nat. Med. 17, 64–70.
Ragusa, M., Majorana, A., Statello, L., Maugeri, M., Salito, L., Barbagallo, D., Guglielmino, M. R., Duro, L. R., Angelica, R., Caltabiano, R., Biondi, A., Di Vita, M., Privitera, G., Scalia, M., Cappellani, A., Vasquez, E., Lanzafame, S., Basile, F., Di Pietro, C., and Purrello, M. (2010). Specific alterations of microRNA transcriptome and global network structure in colorectal carcinoma after cetuximab treatment. Mol. Cancer Ther. 9, 3396–3409.
Ramkissoon, S. H., Mainwaring, L. A., Ogasawara, Y., Keyvanfar, K., Mccoy, J. P. Jr., Sloand, E. M., Kajigaya, S., and Young, N. S. (2006). Hematopoietic-specific microRNA expression in human cells. Leuk. Res. 30, 643–647.
Rodriguez, A., Vigorito, E., Clare, S., Warren, M. V., Couttet, P., Soond, D. R., Van Dongen, S., Grocock, R. J., Das, P. P., Miska, E. A., Vetrie, D., Okkenhaug, K., Enright, A. J., Dougan, G., Turner, M., and Bradley, A. (2007). Requirement of bic/microRNA-155 for normal immune function. Science 316, 608–611.
Ruby, J. G., Jan, C., Player, C., Axtell, M. J., Lee, W., Nusbaum, C., Ge, H., and Bartel, D. P. (2006). Large-scale sequencing reveals 21U-RNAs and additional microRNAs and endogenous siRNAs in C. elegans. Cell 127, 1193–1207.
Saito, Y., Liang, G., Egger, G., Friedman, J. M., Chuang, J. C., Coetzee, G. A., and Jones, P. A. (2006). Specific activation of microRNA-127 with downregulation of the proto-oncogene BCL6 by chromatin-modifying drugs in human cancer cells. Cancer Cell 9, 435–443.
Sarachana, T., Zhou, R., Chen, G., Manji, H. K., and Hu, V. W. (2010). Investigation of post-transcriptional gene regulatory networks associated with autism spectrum disorders by microRNA expression profiling of lymphoblastoid cell lines. Genome Med. 2, 23.
Schlesinger, J., Schueler, M., Grunert, M., Fischer, J. J., Zhang, Q., Krueger, T., Lange, M., Tonjes, M., Dunkel, I., and Sperling, S. R. (2011). The cardiac transcription network modulated by Gata4, Mef2a, Nkx2.5, Srf, histone modifications, and microRNAs. PLoS Genet. 7, e1001313. doi: 10.1371/journal.pgen.1001313
Schmittgen, T. D., Jiang, J., Liu, Q., and Yang, L. (2004). A high-throughput method to monitor the expression of microRNA precursors. Nucleic Acids Res. 32, e43.
Selbach, M., Schwanhausser, B., Thierfelder, N., Fang, Z., Khanin, R., and Rajewsky, N. (2008). Widespread changes in protein synthesis induced by microRNAs. Nature 455, 58–63.
Sethupathy, P., Megraw, M., and Hatzigeorgiou, A. G. (2006). A guide through present computational approaches for the identification of mammalian microRNA targets. Nat. Methods 3, 881–886.
Shalgi, R., Lieber, D., Oren, M., and Pilpel, Y. (2007). Global and local architecture of the mammalian microRNA-transcription factor regulatory network. PLoS Comput. Biol. 3, e131. doi:10.1371/journal.pcbi.0030131
Shao, N. Y., Hu, H. Y., Yan, Z., Xu, Y., Hu, H., Menzel, C., Li, N., Chen, W., and Khaitovich, P. (2010). Comprehensive survey of human brain microRNA by deep sequencing. BMC Genomics 11, 409. doi: 10.1186/1471-2164-11-409
Sood, P., Krek, A., Zavolan, M., Macino, G., and Rajewsky, N. (2006). Cell-type-specific signatures of microRNAs on target mRNA expression. Proc. Natl. Acad. Sci. U.S.A. 103, 2746–2751.
Starnes, L. M., and Sorrentino, A. (2011). Regulatory circuitries coordinated by transcription factors and microRNAs at the cornerstone of hematopoietic stem cell self-renewal and differentiation. Curr. Stem Cell Res. Ther. 6, 142–161.
Takane, K., Fujishima, K., Watanabe, Y., Sato, A., Saito, N., Tomita, M., and Kanai, A. (2010). Computational prediction and experimental validation of evolutionarily conserved microRNA target genes in bilaterian animals. BMC Genomics 11, 101. doi: 10.1186/1471-2164-11-101
Tarasov, V., Jung, P., Verdoodt, B., Lodygin, D., Epanchintsev, A., Menssen, A., Meister, G., and Hermeking, H. (2007). Differential regulation of microRNAs by p53 revealed by massively parallel sequencing: miR-34a is a p53 target that induces apoptosis and G1-arrest. Cell Cycle 6, 1586–1593.
Tavazoie, S. F., Alarcon, C., Oskarsson, T., Padua, D., Wang, Q., Bos, P. D., Gerald, W. L., and Massague, J. (2008). Endogenous human microRNAs that suppress breast cancer metastasis. Nature 451, 147–152.
Thomas, M., Lieberman, J., and Lal, A. (2010). Desperately seeking microRNA targets. Nat. Struct. Mol. Biol. 17, 1169–1174.
Tian, Z., Greene, A. S., Pietrusz, J. L., Matus, I. R., and Liang, M. (2008). MicroRNA-target pairs in the rat kidney identified by microRNA microarray, proteomic, and bioinformatic analysis. Genome Res. 18, 404–411.
Umbach, J. L., and Cullen, B. R. (2010). In-depth analysis of Kaposi’s sarcoma-associated herpesvirus microRNA expression provides insights into the mammalian microRNA-processing machinery. J. Virol. 84, 695–703.
van Dongen, S., Abreu-Goodger, C., and Enright, A. J. (2008). Detecting microRNA binding and siRNA off-target effects from expression data. Nat. Methods 5, 1023–1025.
Vinther, J., Hedegaard, M. M., Gardner, P. P., Andersen, J. S., and Arctander, P. (2006). Identification of miRNA targets with stable isotope labeling by amino acids in cell culture. Nucleic Acids Res. 34, e107.
Watanabe, Y., Tomita, M., and Kanai, A. (2007). Computational methods for microRNA target prediction. Meth. Enzymol. 427, 65–86.
Xenarios, I., Rice, D. W., Salwinski, L., Baron, M. K., Marcotte, E. M., and Eisenberg, D. (2000). DIP: the database of interacting proteins. Nucleic Acids Res. 28, 289–291.
Xie, X., Lu, J., Kulbokas, E. J., Golub, T. R., Mootha, V., Lindblad-Toh, K., Lander, E. S., and Kellis, M. (2005). Systematic discovery of regulatory motifs in human promoters and 3′ UTRs by comparison of several mammals. Nature 434, 338–345.
Xu, G., Fewel, C., Taylor, C., Deng, N., Hedges, D., Wang, X., Zhang, K., Lacey, M., Zhang, H., Yin, Q., Cameron, J., Lin, Z., Zhu, D., and Flemington, E. K. (2010). Transcriptome and targetome analysis in MIR155 expressing cells using RNA-seq. RNA 16, 1610–1622.
Xu, P., Guo, M., and Hay, B. A. (2004). MicroRNAs and the regulation of cell death. Trends Genet. 20, 617–624.
Yin, J. Q., Zhao, R. C., and Morris, K. V. (2008). Profiling microRNA expression with microarrays. Trends Biotechnol. 26, 70–76.
Zamore, P. D., and Haley, B. (2005). Ribo-gnome: the big world of small RNAs. Science 309, 1519–1524.
Zhang, B., Pan, X., Cobb, G. P., and Anderson, T. A. (2007). MicroRNAs as oncogenes and tumor suppressors. Dev. Biol. 302, 1–12.
Zhang, H., Li, Y., and Lai, M. (2010a). The microRNA network and tumor metastasis. Oncogene 29, 937–948.
Zhang, S., Chen, L., Jung, E. J., and Calin, G. A. (2010b). Targeting microRNAs with small molecules: from dream to reality. Clin. Pharmacol. Ther. 87, 754–758.
Ziegelbauer, J. M., Sullivan, C. S., and Ganem, D. (2009). Tandem array-based expression screens identify host mRNA targets of virus-encoded microRNAs. Nat. Genet. 41, 130–134.
Keywords: microRNA, systems biology, gene regulatory network, transcriptome, proteome, immunoprecipitation
Citation: Watanabe Y and Kanai A (2011) Systems biology reveals microRNA-mediated gene regulation. Front. Gene. 2:29. doi: 10.3389/fgene.2011.00029
Received: 08 April 2011;
Accepted: 30 May 2011;
Published online: 23 June 2011.
Edited by:
Stephan Vagner, Institut National de la Santé et de la Recherche Médicale, FranceReviewed by:
Bernard Mari, Centre National de La Recherche Scientifique, FranceScott Tenenbaum, University at Albany-SUNY, USA
Copyright: © 2011 Watanabe and Kanai. This is an open-access article subject to a non-exclusive license between the authors and Frontiers Media SA, which permits use, distribution and reproduction in other forums, provided the original authors and source are credited and other Frontiers conditions are complied with.
*Correspondence: Akio Kanai, Institute for Advanced Biosciences, Keio University, Tsuruoka, Yamagata 997-0017, Japan e-mail:YWtpb0BzZmMua2Vpby5hYy5qcA==