- Department of Pharmacology, Masonic Cancer Center, University of Minnesota, Minneapolis, MN, USA
A biochemical activity, that is, enzymatic reaction or molecular interaction, frequently involves a molecule, for example, an enzyme, capable of interacting with numerous substrates or partners. Specificity is a fundamental property of biochemical activities, and relative specificity refers to the situation whereby a molecule interacts with multiple substrates or partners but with different affinities. Here, a hypothesis is proposed that any molecule, such as an enzyme, would have a range of preferences or relative specificity for its many native substrates, which differentially impacts the phenotypes of these substrates and hence shapes the relevant biological processes in vivo. While the mechanisms underlying the specific recognition between enzymes and individual substrates have been studied extensively, whether any enzyme exhibits intrinsic selectivity toward its ensemble of substrates is often overlooked, and whether this selectivity has any functional consequences is much less appreciated. There are, however, several lines of evidence in the literature that are consistent with the hypothesis and reviewed here. Furthermore, this hypothesis is supported by our analyses of a number of diverse biochemical systems at a large scale. Thus, the human microRNA processing machinery possesses relative specificity toward its hundreds of substrates, which might contribute to differential microRNA biogenesis; the promoter binding affinity of the transcription factor Ndt80 might regulate Ndt80 target mRNA expression in the budding yeast; Cdk1 kinase specificity might lead to variable substrate phosphorylation in vivo; and the density of HuR deposition to its thousands of RNA targets might partly explain differential RNA expression in human cells. It is proposed, therefore, that relative specificity is a universal property of complex biochemical systems and that the hypothesis could denote a general principle in biology.
Introduction
Specificity in biochemical activities has two components, absolute specificity and relative specificity. For absolute specificity, a molecule, symbolized as E here, recognizes a group of substrates or interacting partners (symbolized as {S}) but not any others. For relative specificity, an E interacts with more than one cognate {S} differentially. The functional implication of absolute specificity is self-evident, so the focus here is on relative specificity, although absolute specificity can be viewed as an extreme case of relative specificity. This paper addresses a hypothesis that an E has a range of affinities or preferences for its many {S}, and that such selectivity differentially impacts the {S} to influence the underlying biological processes at a large scale in vivo (Figure 1A). Below, I will first explain the hypothesis in more detail. While the proposition appears intuitively plausible, it is actually understudied and not grounded in real data in most biochemical systems. Next, potential evidence in the literature will be summarized. Our own work will then be presented to further support the hypothesis and to demonstrate strategies to test the hypothesis directly. Lastly, the implications of the hypothesis will be discussed.
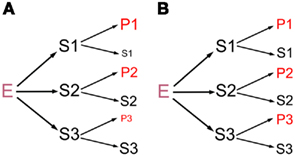
Figure 1. Schematics of how an E interacts with {S}, represented by S1, S2, and S3. The interactions yield the respective products P1, P2, and P3, with the remaining S1, S2, and S3. The letter size symbolizes the abundance of S and P, with the starting S1–S3 being equal for simplicity. (A) E discriminates amongst S to yield different amounts of P1, P2, and P3, as the central hypothesis predicts. (B) E interacts with S1–S3 identically, a commonly assumed situation. Because S and P likely have different activities in vivo, (A) and (B) will lead to different functional outcomes downstream.
Relative Substrate Preferences are Not Well Understood at the Systems Level
The number of {S} varies greatly with E. For example, hemoglobin binds a handful of {S}, e.g., O2, CO2, CO, with CO having a higher affinity than O2 for hemoglobin, leading to a textbook consequence. On the other hand, the ribosome and RNA polymerase II have tens of thousands of {S} in multicellular organisms. In the middle of the spectrum, an enzyme may catalyze the conversion of dozens or hundreds of substrates, and a molecule may interact with a large number of partners; some examples are protein kinases, transcription factors, and microRNAs (miRNAs). The hypothesis of interest is broadly applicable but was conceived with complex systems in mind wherein an E has at least dozens or hundreds of cognate {S}. The significance of specific recognition sequences in individual or model targets has been extensively investigated for E such as kinases and transcription factors, but whether an E possesses selectivity toward its ensemble of {S} is often unknown, and whether this selectivity has any in vivo relevance is seldom reported in the literature. For example, protein kinases and transcription factors typically recognize degenerate sequences in hundreds of native {S}, so it is plausible yet hardly reported that, based on their sequences, some {S} would be better phosphorylated or transcribed than others in vivo. Additionally, do the core transcription machinery and the core translation machinery possess any innate preferences toward their tens of thousands of targets genes and mRNAs, respectively, and do the preferences contribute to differential gene expression in vivo? Components of these machineries might have isoforms and be differentially expressed, which is regulatory in nature (Goodrich and Tjian, 2010; Kondrashov et al., 2011). The hypothesis formulated here, however, differs from other theories by projecting that regulation can originate at the most basic level from the biased interactions between a single E and its vast number of {S}.
There is a dearth of studies that explicitly address the above or analogous questions, for two reasons. The first is that the potential, regulatory role of E:{S} selectivity is often overlooked. By default, an E is frequently portrayed to operate passively or constitutively, doing an assembly line-like task on its entire set of {S}, regulated only from outside of the system (Figure 1B). The second reason is that it is difficult to study the phenomena in complex systems. Firstly, we usually do not know all or most of the genuine {S} for any E. Secondly, {S} or the products in vivo must be quantified at a large scale, but the essential genomics or proteomics tools were not available until the late 1990s. Thirdly, a representative assay might not exist to characterize biochemical interactions in vitro. For example, only a few artificial or authentic {S} have been tested using the in vitro transcription or translation systems, and the products usually do not phenocopy full-length pre-mRNAs or proteins typical in vivo. As a result, there are few reports of E discriminating amongst its large set of {S}. Lastly, one has to isolate the effect of E on {S}, as {S} are invariably controlled by multiple factors besides E in vivo. Thus, even if an E’s relative specificity toward a small number of {S} in vitro correlates with the phenotypes of {S} in vivo, it remains possible that the correlation results from the fortuitous action of factors other than the E.
Potential Supporting Evidence in the Literature
Despite the challenges, there is evidence in the literature that might support the hypothesis in complex biochemical systems. An example is eIF4E, which binds the 5′ m7GpppN cap of mRNAs to initiate mRNA scanning and translation (Sonenberg, 2008). All capped mRNAs are eIF4E substrates, but eIF4E overexpression preferentially stimulates the translation of a subset of mRNAs that promote tumorigenesis in mammalian systems. These mRNAs often have a long and stable 5′ untranslated region that presumably requires elevated eIF4E activity, although the direct mRNA selectivity of eIF4E has not been extensively examined in vitro. A transcript-specific role has also been ascribed to ribosomal proteins (Kondrashov et al., 2011; Topisirovic and Sonenberg, 2011). Deficiency in ribosomal protein L28 reduces the translation of a subset of Hox mRNAs in mouse embryos, which is not observed in mutants of several other ribosomal proteins (Kondrashov et al., 2011). Likewise, mutations of general transcription factors differentially impact the expression of distinct sets of genes (Holstege et al., 1998). The origin of the selectivity of L28 or general transcription factors, however, is unknown.
Another example is ligand–receptor interaction. Distinct agonists ({S}) can differentially impact downstream signaling pathways even when bound to the same receptor (E), e.g., the μ-opioid receptor, β2-adrenergic receptor, vasopressin, serotonin, and dopamine receptors (reviewed in Urban et al., 2007). The concepts of intrinsic efficacy, functional selectivity, or agonist-selectivity signaling have been proposed to explain this phenomenon (Urban et al., 2007). How E discriminates amongst {S} is not well characterized, but it likely necessitates E to adopt multiple active conformations upon binding by different {S}. Even less understood is how these conformations could differentially activate downstream signaling molecules, quantitatively or qualitatively. An analogous mechanism may partially explain the multifunctionality or promiscuity of other proteins, e.g., the cytochrome P450 enzymes (Khersonsky and Tawfik, 2010; Atkins and Qian, 2011). The general explanation is that a protein (E) exists in a multiplicity of conformations due to inherent structural plasticity. {S} may bind it with different affinities at overlapping but non-identical sites to form complexes with different conformations, and the {S} reactive groups may position in the complexes differently, thereby affecting the subsequent catalysis or product release. Nevertheless, in most cases we probably do not even realize the full spectrum of substrates for such E, and a single molecule can be the substrates of several enzymes in a cell or tissue specific manner, which hampers the global analysis of relative specificity in vitro and in vivo.
The most revealing case is perhaps the HuR protein, which stabilizes RNAs by binding preferentially to short uridine stretches. Lebedeva et al. (2011) and Mukherjee et al. (2011) identified HuR substrates at the global scale and showed that HuR has thousands of RNA targets with variable, potential HuR binding sites and HuR association in cells, and that the degree of HuR binding correlates with HuR-dependent stability of the target RNAs.
While these studies reported that E:{S} interactions can lead to distinct effects depending on {S}, the phenomena have not been generalized, and the detailed mechanism is unavailable with the exception of HuR (but see below). This is largely due to the lack of suitable in vitro biochemical assays or detailed, structural data, leaving open alternative explanations.
Regulation of miRNA Expression by the General miRNA Processing Machinery
The rationale behind our hypothesis is that relative specificity is likely a universal, direct, and natural consequence when an E has to interact with many native {S} with different sequences or structures in any complex system, and that in vivo phenotypes can partly be explained by the relative specificity of the underlying biochemical activities. Our own work has aimed to directly test this hypothesis. First we studied the biogenesis of miRNAs. miRNAs are a class of ~22-nucleotide-long RNAs, in mammals encoded by hundreds of known miRNA genes (Ambros, 2004; Newman and Hammond, 2010). A canonical miRNA is initially transcribed as part of a long primary transcript. An RNase called Drosha cleaves this transcript to liberate a hairpin precursor of ~60 nucleotides. Dicer, another RNase, cleaves the precursor to produce an ~22 basepair RNA duplex intermediate. Subsequently, an Argonaute (Ago) protein binds to the duplex and selects the mature, single-stranded miRNA. The Ago:miRNA complex then functions by repressing target gene expression downstream.
We investigated the cleavage of hundreds of human primary miRNA substrates by Drosha in vitro (Feng et al., 2011). Drosha (E) cleaves these RNAs ({S}) with different efficiencies, which positively correlates with the relative expression levels of the corresponding mature miRNAs in vivo, and the specificity could be partially explained by different structural properties of the substrates. Considering the well-known biochemical function of Drosha, we suggested that Drosha selectivity determines, to a significant extent, whether a transcript encodes a miRNA or not, and how efficiently a miRNA is produced in vivo (Feng et al., 2011).
In a related study, we showed that stable Ago2 overexpression increases or decreases the maturation of distinct miRNAs in human cells (Zhang et al., 2009). This result is analogous to that of eIF4E. Because Ago2:miRNA complexes inhibit the expression of target mRNAs, the differential effects of Ago2 might be accounted for by the interdependence of the expression of specific mRNAs and miRNAs (Zhang et al., 2009).
Selectivity in Ndt80 and Cdk1 Functions
Our Drosha study combined in vitro assays and in vivo data analysis to explicitly test the hypothesis. No other biological systems, however, have been examined in a similar and deliberate manner. The best functional genomics studies have been performed in S. cerevisiae, so I carried out literature search, data mining, and analysis for this model organism.
Ndt80 is a transcription factor involved in middle gene induction (~5 h) during sporulation (Chu and Herskowitz, 1998). Jolly et al. (2005) showed that Ndt80 binds DNA with nucleotide preferences in an in vitro binding assay, generated a corresponding, position weight matrix (PWM), and then curated a list of 145 known and predicted Ndt80 target genes. For this analysis, the potential Ndt80 binding sites in these 145 genes were retrieved using the YEASTRACT database (Teixeira et al., 2006), and their PWM scores calculated (Table A1 in Appendix). If a gene has more than one potential Ndt80 site, the highest PWM score was used as the representative. Not surprisingly, the target genes have variable PMW scores (Table A1 in Appendix), so Ndt80 could associate with the promoters with different affinities or probabilities, suggestive of relative specificity in vivo. Next, mRNA expression data were extracted from Chu et al. (1998) where yeast gene expression from 0 to 11.5 h after the initiation of sporulation was determined by microarray analyses (Table A1 in Appendix). The PWM scores were then correlated with target mRNA expression at the different time points (SPSS, IBM). At 0 h, the Spearman rank correlation coefficient ρ = 0.008, p value = 0.93; at 0.5 h, ρ = −0.013, p = 0.88; at 2 h, ρ = 0.077, p = 0.36; at 5 h, ρ = 0.35, p = 0.00002; at 7 h, ρ = 0.30, p = 0.0003; at 9 h ρ = 0.31, p = 0.0002; at 11.5 h, ρ = 0.29, p = 0.0004. The positive correlation peaks at 5 h, consistent with the known function of Ndt80 (Chu and Herskowitz, 1998). This result suggested that Ndt80 binding specificity, determined in vitro, could translate to differential target gene expression in vivo.
The other example is protein phosphorylation by S. cerevisiae Cdc28, or Cdk1, which controls cell cycle progression. Ubersax et al. (2003) showed that the mitotic Cdk1 phosphorylates hundreds of proteins in vitro. The extent of substrate phosphorylation, or P-scores, ranged over seven orders of magnitude, which cannot be explained by the differences in possible phosphorylation sites. Cdk1, therefore, discriminates amongst {S} in vitro, just like Drosha.
Does this specificity impact the phosphorylation levels of Cdk1 substrates in vivo? Using mass spectrometry, Holt et al. (2009) identified phosphorylation sites in hundreds of Cdk1 substrates in especially cells arrested by the overexpression of a stable mitotic cyclin. For this analysis the Signal/Noise (S/N) ratio was used as a proxy for phosphorylation in vivo, although data from Holt et al. (2009) did not allow for normalization against total protein expression. For a protein with more than one phosphopeptide, the sum of all the S/N ratios was used to represent its phosphorylation (Table A2 in Appendix). P-scores correlated positively and significantly with S/N ratios: n = 144, ρ = 0.29, p = 0.0005, so Cdk1 specificity in vitro (Ubersax et al., 2003) might partially explain differential substrate phosphorylation in vivo (Holt et al., 2009).
The analyses of Ndt80 and Cdk1 functions demonstrated for the first time a global correlation between the in vitro specificity and in vivo functions of a transcription factor and a protein kinase, respectively. Does the relative specificity have a physiological impact? Differential miRNA and mRNA expression is presumably regulatory and functionally relevant in vivo. And because a phosphoprotein and its non-phosphorylated counterpart often differ in their activity, subcellular localization, and/or stability, Cdk1 might phosphorylate its many substrates to various degrees to regulate cell cycle progression. This is a novel angle to look at Cdk1 functions that merits further investigations.
HuR Binding and Target RNA Expression
Lebedeva et al. (2011) and Mukherjee et al. (2011) convincingly demonstrated that the degree of HuR association predicts HuR-dependent RNA stabilization. The authors measured HuR association by RNA sequencing or arrays following HuR immunoprecipitation from cell cultures, and HuR-dependent RNA stabilization by the HuR knockdown strategy. A prediction from our hypothesis, not explicitly tested in these studies, is that HuR specificity positively contributes to the absolute expression levels of target RNAs.
HuR consensus RNA binding sequences are very degenerate and not precisely defined (Levine et al., 1993; de Silanes et al., 2004; Meisner et al., 2004; Ray et al., 2009), so a PWM score might not adequately depict a potential HuR:target interaction. Furthermore, both Lebedeva et al. (2011) and Mukherjee et al. (2011) showed that RNAs ({S}) can vary substantially in their numbers of HuR binding sites, a sign of HuR relative specificity. Consequently, the number of HuR binding sites or its variations were used here to represent HuR:RNA association, and the data of Lebedeva et al. (2011) and Mukherjee et al. (2011) re-analyzed. The strongest correlation was detected between the fragments/read pairs per kilobase of exon per million read pairs, reflecting relative mRNA expression, and the fraction of length covered by binding clusters, reflecting HuR association normalized against transcript size (Table S2 in Lebedeva et al., 2011). For the 4,845 consensus HuR targets, the Spearman rank correlation coefficient is 0.39, p = 2.6 × 10−171; for the 1,216 conservative targets, the coefficient is 0.36, p = 2.8 × 10−38 (Table S2 in Lebedeva et al., 2011). Using the predicted or verified HuR binding site numbers without transcript size normalization yielded positive but less significant correlations to the RNA sequencing or array data (Lebedeva et al., 2011; Mukherjee et al., 2011; data not shown). This new result suggested that the density of HuR deposition best explains the differential expression of HuR target RNAs.
Interpretations of Our Results
Our studies serve as examples to illustrate how one can test the hypothesis directly. As the first step, one needs to realize that relative specificity is a general and functioning characteristic in any complex systems. In step 2, a biochemical assay, usually with purified components in vitro, will examine the interactions between an E and {S} at a large scale. In step 3, a global approach will characterize {S} or the products in cells or in vivo. As the last step, a significant statistics correlation is expected between the results from steps 2 and 3. In our work, because the biochemical activities of Drosha, Ndt80, Cdk1, and HuR are firmly established, the observed correlations present strong evidence that the relative biochemical specificity is causal and functional in vivo.
Somewhat remarkably, our conclusions were reached despite the complexities in the underlying biological problems and data acquisition. For example, Drosha cleavage efficiencies can only be approximated (Feng et al., 2011), S/N ratio depends on phosphorylation efficiency, peptide extraction or ionization, and protein expression, and PWM gives mere estimates. Concentrations of {S} in vivo might deviate greatly from Km, and {S} will compete for the access to E, which might skew or complicate the effects of E selectivity. Multiple steps and factors influence miRNA maturation, mRNA expression, or protein phosphorylation. Our analyses assumed that the specificity of E was sufficiently independent of all the other contributing factors, such that examining a large number of {S} would enable us to assess statistical significance in the complex processes. No strategy, however, can completely filter out the effects by every interfering factor in vivo.
With these considerations, it is not surprising that relatively small correlation coefficients were obtained. There are two angles to look at the numbers. One is that they reflect spurious correlations. Nevertheless, the coefficients are similar to many reported in the literature (e.g., Lackner et al., 2007; Tuller et al., 2010), the correlations are significant in diverse systems, and they can all be explained biochemically. Alternatively, they suggest that the relative E:{S} specificity is only one of myriad factors that contribute to the final phenotype in vivo, but it still plays an integral and important role. For example, protein degradation does not contribute to global protein expression as significantly as other factors, but it is arguably still an important regulatory mechanism (Schwanhäusser et al., 2011). Furthermore, for the reasons given above, these coefficients likely underestimate the relationships in vivo, and more inclusive and precise measurements and better analytic tools in the future may improve the values and elucidate the true significance of E:{S} specificity.
Implications and Concluding Remarks
How biological processes are regulated is a subject of intense investigations yet remains incompletely understood, especially at the systems level. This hypothesis posits that relative specificity is the rule rather than the exception at a global scale such that we must pay more attention to the functional role of substrate preferences in individual biochemical activities. Take a familiar example, to comprehend how genes A, B, C, etc are differentially transcribed, a standard work plan is to identify the relevant chromatin modifiers, specific transcription factors, and RNA-processing complexes. The knowledge is incomplete, however, until we understand their relative affinities or efficacies for the specific genes, elucidate the non-identical impact from RNA polymerase II and the general transcription factors, and quantify their individual contributions.
That biochemical activities regulate biological processes is a truism, and as stated by Mukherjee et al. (2011), “All targets were not quantitatively equivalent.” This hypothesis, however, explicates how a certain level of regulation is achieved and brings attention to the regulatory properties of many entities that have been traditionally ignored or greatly underappreciated. Testing the hypothesis for the functions of Drosha, Ndt80, Cdk1, and HuR sheds light on how diverse systems are regulated in vivo. In addition, studying global relative specificity has provided new insights into its biological significance. For example, eIF4E selectivity manifests itself in promoting tumorigenesis, agonist-selective signaling may explain, in part, how, e.g., different opioid drugs elicit different physiological responses, and Drosha processing may control the specificity and efficiency of miRNA biogenesis.
To conclude, this paper emphasizes the pervasive, functional role of relative specificity in biochemical activities and outlines a general strategy to analyze its significance in complex systems. Future studies to test the hypothesis will advance our knowledge of how biological processes are regulated and how relative specificity has evolved.
Conflict of Interest Statement
The author declares that the research was conducted in the absence of any commercial or financial relationships that could be construed as a potential conflict of interest.
Acknowledgments
I thank Drs Xiaoxiao Zhang and Yong Feng for work in the laboratory and Drs Paul Graves and Clifford Steer for comments on the manuscript.
References
Atkins, W. M., and Qian, H. (2011). Stochastic ensembles, conformationally adaptive teamwork, and enzymatic detoxification. Biochemistry 50, 3866–3872.
Chu, S., DeRisi, J., Eisen, M., Mulholland, J., Botstein, D., Brown, P. O., and Herskowitz, I. (1998). The transcriptional program of sporulation in budding yeast. Science 282, 699–705.
Chu, S., and Herskowitz, I. (1998). Gametogenesis in yeast is regulated by a transcriptional cascade dependent on Ndt80. Mol. Cell 1, 685–696.
de Silanes, I. L., Zhan, M., Lal, A., Yang, X., and Gorospe, M. (2004). Identification of a target RNA motif for RNA-binding protein HuR. Proc. Natl. Acad. Sci. U.S.A. 101, 2987–2992.
Feng, Y., Zhang, X., Song, Q., Li, T., and Zeng, Y. (2011). Drosha processing controls the specificity and efficiency of global microRNA expression. Biochim. Biophys. Acta doi: 10.1016/j.bbagrm.2011.05.015. [Epub ahead of print].
Goodrich, J. A., and Tjian, R. (2010). Unexpected roles for core promoter recognition factors in cell-type-specific transcription and gene regulation. Nat. Rev. Genet. 11, 549–558.
Holstege, F. C., Jennings, E. G., Wyrick, J. J., Lee, T. I., Hengartner, C. J., Green, M. R., Golub, T. R., Lander, E. S., and Young, R. A. (1998). Dissecting the regulatory circuitry of a eukaryotic genome. Cell 95, 717–728.
Holt, L. J., Tuch, B. B., Villén, J., Johnson, A. D., Gygi, S. P., and Morgan, D. O. (2009). Global analysis of Cdk1 substrate phosphorylation sites provides insights into evolution. Science 325, 1682–1686.
Jolly, E. R., Chin, C. S., Herskowitz, I., and Li, H. (2005). Genome-wide identification of the regulatory targets of a transcription factor using biochemical characterization and computational genomic analysis. BMC Bioinformatics 6, 275. doi: 10.1186/1471-2105-6-275
Khersonsky, O., and Tawfik, D. S. (2010). Enzyme promiscuity: a mechanistic and evolutionary perspective. Annu. Rev. Biochem. 79, 471–505.
Kondrashov, N., Pusic, A., Stumpf, C., Shimizu, K., Hsieh, A. C., Xue, S., Ishijima, J., Shiroishi, T., and Barna, M. (2011). Ribosome-mediated specificity in Hox mRNA translation and vertebrate tissue patterning. Cell 145, 383–397.
Lackner, D. H., Beilharz, T. H., Marguerat, S., Mata, J., Watt, S., Schubert, F., Preiss, T., and Bähler, J. (2007). A network of multiple regulatory layers shapes gene expression in fission yeast. Mol. Cell 26, 145–155.
Lebedeva, S., Jens, M., Theil, K., Schwanhäusser, B., Selbach, M., Landthaler, M., and Rajewsky, N. (2011). Transcriptome-wide analysis of regulatory interactions of the RNA-binding protein HuR. Mol. Cell 43, 340–352.
Levine, T. D., Gao, F., King, P. H., Andrews, L. G., and Keene, J. D. (1993). Hel-N1: an autoimmune RNA-binding protein with specificity for 30 uridylate rich untranslated regions of growth factor mRNAs. Mol. Cell Biol. 13, 3494–3504.
Meisner, N. C., Hackermüller, J., Uhl, V., Aszódi, A., Jaritz, M., and Auer, M. (2004). mRNA openers and closers: modulating AU-rich element-controlled mRNA stability by a molecular switch in mRNA secondary structure. Chembiochem 5, 1432–1447.
Mukherjee, N., Corcoran, D. L., Nusbaum, J. D., Reid, D. W., Georgiev, S., Hafner, M., Ascano, M., Tuschl, T., Ohler, U., and Keene, J. D. (2011). Integrative regulatory mapping indicates that the RNA-binding protein HuR couples pre-mRNA processing and mRNA stability. Mol. Cell 43, 327–339.
Newman, M. A., and Hammond, S. M. (2010). Emerging paradigms of regulated microRNA processing. Genes Dev. 24, 1086–1092.
Ray, D., Kazan, H., Chan, E. T., Peña Castillo, L., Chaudhry, S., Talukder, S., Blencowe, B. J., Morris, Q., and Hughes, T. R. (2009). Rapid and systematic analysis of the RNA recognition specificities of RNA-binding proteins. Nat. Biotechnol. 27, 667–670.
Schwanhäusser, B., Busse, D., Li, N., Dittmar, G., Schuchhardt, J., Wolf, J., Chen, W., and Selbach, M. (2011). Global quantification of mammalian gene expression control. Nature 473, 337–342.
Sonenberg, N. (2008). eIF4E, the mRNA cap-binding protein, from basic discovery to translational research. Biochem. Cell Biol. 86, 178–183.
Teixeira, M. C., Monteiro, P., Jain, P., Tenreiro, S., Fernandes, A. R., Mira, N. P., Alenquer, M., Freitas, A. T., Oliveira, A. L., and Sá-Correia, I. (2006). The YEASTRACT database, a tool for the analysis of transcription regulatory associations in Saccharomyces cerevisiae. Nucleic Acids Res. 34, D446–D451.
Topisirovic, I., and Sonenberg, N. (2011). Translational control by the eukaryotic ribosome. Cell 145, 333–334.
Tuller, T., Waldman, Y. Y., Kupiec, M., and Ruppin, E. (2010). Translation efficiency is determined by both codon bias and folding energy. Proc. Natl. Acad. Sci. U.S.A. 107, 3645–3650.
Ubersax, J. A., Woodbury, E. L., Quang, P. N., Paraz, M., Blethrow, J. D., Shah, K., Shokat, K. M., and Morgan, D. O. (2003). Targets of the cyclin-dependent kinase Cdk1. Nature 425, 859–864.
Urban, J. D., Clarke, W. P., von Zastrow, M., Nichols, D. E., Kobilka, B., Weinstein, H., Javitch, J. A., Roth, B. L., Christopoulos, A., Sexton, P. M., Miller, K. J., Spedding, M., and Mailman, R. B. (2007). Functional selectivity and classical concepts of quantitative pharmacology. J. Pharmacol. Exp. Ther. 320, 1–13.
Zhang, X., Graves, P. R., and Zeng, Y. (2009). Stable Argonaute2 overexpression differentially regulates microRNA production. Biochim. Biophys. Acta 1789, 153–159.
Appendix
Keywords: biochemical activity, relative specificity, substrate selectivity, regulatory function
Citation: Zeng Y (2011) The functional consequences of relative substrate specificity in complex biochemical systems. Front. Gene. 2:65. doi: 10.3389/fgene.2011.00065
Received: 24 July 2011;
Accepted: 30 August 2011;
Published online: 20 September 2011.
Edited by:
Josselin Noirel, University of Sheffield, UKReviewed by:
Necmettin Yildirim, New College of Florida, USAAnne-Gaëlle Planson, Institute of Systems and Synthetic Biology, France
Paul Dobson, University of Sheffield, UK
Copyright: © 2011 Zeng. This is an open-access article subject to a non-exclusive license between the authors and Frontiers Media SA, which permits use, distribution and reproduction in other forums, provided the original authors and source are credited and other Frontiers conditions are complied with.
*Correspondence: Yan Zeng, Department of Pharmacology, University of Minnesota, 6-120 Jackson Hall, 321 Church Street SE, Minneapolis, MN 55455, USA. e-mail:emVuZ3gwMzNAdW1uLmVkdQ==