- Department of Epidemiology and Biostatistics, Michigan State University, East Lansing, MI, USA
Cocaine-associated biomedical and psychosocial problems are substantial twenty-first century global burdens of disease. This burden is largely driven by a cocaine dependence process that becomes engaged with increasing occasions of cocaine product use. For this reason, the development of a risk-prediction model for cocaine dependence may be of special value. Ultimately, success in building such a risk-prediction model may help promote personalized cocaine dependence prediction, prevention, and treatment approaches not presently available. As an initial step toward this goal, we conducted a genome-environmental risk-prediction study for cocaine dependence, simultaneously considering 948,658 single nucleotide polymorphisms (SNPs), six potentially cocaine-related facets of environment, and three personal characteristics. In this study, a novel statistical approach was applied to 1045 case-control samples from the Family Study of Cocaine Dependence. The results identify 330 low- to medium-effect size SNPs (i.e., those with a single-locus p-value of less than 10−4) that made a substantial contribution to cocaine dependence risk prediction (AUC = 0.718). Inclusion of six facets of environment and three personal characteristics yielded greater accuracy (AUC = 0.809). Of special importance was the joint effect of childhood abuse (CA) among trauma experiences and the GBE1 gene in cocaine dependence risk prediction. Genome-environmental risk-prediction models may become more promising in future risk-prediction research, once a more substantial array of environmental facets are taken into account, sometimes with model improvement when gene-by-environment product terms are included as part of these risk predication models.
Introduction
Cocaine use and dependence continue to represent major biomedical and psychosocial problems affecting individual drug users, their families, and society as a whole. Within the United States (US) population, cocaine ranks second among the most commonly used internationally regulated drugs (IRD). It is estimated that roughly one in six who start using cocaine will develop a cocaine dependence syndrome. By comparison, for cannabis, which is the IRD used most often, the corresponding estimate is 1 in 11 (Anthony, 2010). Development of cocaine dependence involves multiple steps after the first chance to try this drug, and the dependence process can be traced from the initiation of cocaine use toward subsequent dependence processes; each step is plausibly influenced by both genes and environmental conditions (Anthony, 2010). Against a background of evidence showing that facets of environment are largely important in relation to the occurrence of chances to try cocaine, it seems clear that the genetic variants play a more substantial role in the initial responses to first cocaine use, and in the transitions from transient occasions of use in the direction of the cocaine dependence process (Wagner and Anthony, 2002; Uhl, 2004; Compton et al., 2005; Anthony, 2010; Ho et al., 2010; Bierut, 2011).
Evidence from twin and family studies is supportive with respect to genetic influences on cocaine dependence. For example, estimated heritability has ranged from 0.4 upward toward 0.8 (Kendler and Prescott, 1998; Van Den Bree et al., 1998; Kendler et al., 2000). With heritability estimates of this size, the hope is that genetic variants alone, or coupled with facets of environment, might be used to improve prediction of risk for becoming cocaine dependent. If successful, a cocaine dependence risk-prediction model might be used to guide early prevention and intervention initiatives in population-wide interventions, to identify high risk subgroups of the population deserving of special attention, and to shine new light on research, both pre-clinical and clinical, as might help us come to a better understanding of the processes at play when dependence syndromes and serious drug addiction follows initial drug experiences.
On one hand, the task of developing a risk-prediction model for cocaine dependence is hampered by the present state of evidence, in which few individual SNP markers have been identified as substantial influences on the heritability values just mentioned. On the other hand, from a risk-prediction perspective, it is not necessary to require all such predictors in the risk-prediction model to be confirmed disease-susceptibility loci. High-dimensional risk-prediction studies have recently been conducted in exploration of a large ensemble of genetic predictors and their interactions, with resulting improvements in risk prediction for type I diabetes and other complex diseases (Evans et al., 2009; Wei et al., 2009; Kooperberg et al., 2010). In these improvements, the risk-prediction models have been improved substantially via extension of the models to encompass the possibility of gene–gene interactions, in addition to the potential influence of individual genes.
In line with these successful high-dimensional risk-prediction modeling approaches for other complex diseases, we have completed a genome-environmental risk-prediction study for cocaine dependence. In this work, there is simultaneous consideration of 948,658 single nucleotide polymorphisms (SNPs), six cocaine-related facets of environment, three personal characteristics, and their potential interactions. To pursue our risk-prediction model development aim, we applied a novel tree-assembling ROC (TA-ROC) approach, with data from de-identified case–control samples supplied from the Family Study of Cocaine Dependence (FSCD). Post-prediction analyses then were used to evaluate potential contributions of each individual predictor within an overall cocaine dependence risk-prediction model.
Materials and Methods
FSCD Genome-Wide Association Study Dataset
De-identified data from the FSCD originate with 440 unrelated cases and 605 unrelated controls. FSCD cases, diagnosed in relation to DSM-IV cocaine dependence criteria, were identified and recruited in chemical dependency treatment units of the greater St. Louis metropolitan area. FSCD controls were identified and recruited through driver’s license records with matching for race, age, sex, and residential zip code. FSCD assessment plans for personal characteristics (e.g., age), and for facets of environment (e.g., trauma experiences) were guided by standardized interview protocols, as described in prior FSCD publications (Bierut et al., 2008, 2010) and on the study’s web site. Blood samples were collected for all 1045 unrelated subjects. Genotyping used Illumina Human 1Mv1_C BeadChips and the Illumina Infinium II assay protocol (Bierut et al., 2010). In total, 948,658 SNPs passed the FSCD quality control filter (QC). These were the QC-filtered SNPs used in development of our cocaine dependence risk-prediction model.
Genome-Environmental Risk-Prediction Analysis
We are aware that this genome-environmental risk-prediction study simultaneously considers nearly one million predictors and their possible interactions. To handle such a large amount of data, we make use of a newly developed receiver operating characteristic approach: TA-ROC. TA-ROC represents an extension of our previously developed approach, Forward ROC (F-ROC), possessing the same advantage of being computationally efficient and capable of capturing higher order interactions (Ye et al., 2011). Moreover, within TA-ROC, there is a built-in trees-assembling process that is capable of integrating hundreds of low- to medium-effect potential risk predictors and their interactions into the model with an intent to improve accuracy of prediction.
The entire process of the TA-ROC approach is described in Figure 1. To build a risk-prediction model, the TA-ROC first draws T bootstrap samples from the original case–control data and uses the remaining samples (i.e., those not selected as the bootstrap sample) as the out-of-bag samples (OOB samples). For each bootstrap sample, a small set of predictors is selected. Thereafter, a forward selection algorithm (i.e., our F-ROC algorithm) is used to form an optimal risk-prediction model. The F-ROC algorithm starts with a null model containing no predictors. In each step, it adds a new predictor into the model and splits the samples into different risk groups, so that the model’s accuracy is optimized. The splitting process continues until a full-size prediction model is reached (i.e., risk groups cannot be split further). Applying the F-ROC algorithm to all T bootstrap samples yields an ensemble of prediction models. Via assembly of a large number of prediction models, each containing different sets of predictors, TA-ROC can simultaneously consider a large number of genetic/environmental predictors and their interactions. TA-ROC also has the advantage of taking low-marginal-effect predictors and their interactions into account via random selection of a subset of the predictors, some of which are low-marginal-effect predictors. The final model built by TA-ROC is then applied to the OOB samples to obtain an unbiased estimate of the model’s accuracy.
The major difference between TA-ROC and F-ROC is that TA-ROC ensembles a large number of tree models built on a random subset of the original data. Each of these models is built by F-ROC on a small set of predictors. Therefore, if the underlying disease model involves a larger number of predictors, TA-ROC has the advantage of considering a large ensemble of predictors for improved accuracy, while F-ROC only utilizes a small set of predictors with limited accuracy. A simple simulation study was conducted to demonstrate the improvement of TA-ROC over F-ROC. In the simulation, we varied the number of risk predictors from 2 to 10, and included additional 20 noise predictors. The underlying disease models were assumed to be a multiplicative-effect model, a multiplicative-interaction model, a threshold-effect model (Marchini et al., 2005), and a pure interaction model with no marginal effect (Ritchie et al., 2003). Thousand replicates were generated for each disease model. In each replicate, we applied TA-ROC and F-ROC to build risk-prediction models and calculate the AUCs. In this limited simulation, we demonstrated that the proposed TA-ROC attained higher accuracy than the existing F-ROC when an increased number of risk predictors were involved (Figure 2).
Results
Descriptive Analysis
Table 1 describes the FSCD sample of 440 cocaine dependence and 605 controls in relation to the distribution of personal characteristics (e.g., age at interview) and facets of environment (e.g., trauma experience). Noteworthy differences between cases and controls are observed for physical trauma, childhood sex abuse, and childhood physical abuse. For example, almost 80% of cases has experienced physical trauma versus fewer than 40% of controls. In these case–control contrasts, more or less balanced distributions were found for the matching variables (e.g., age and race).
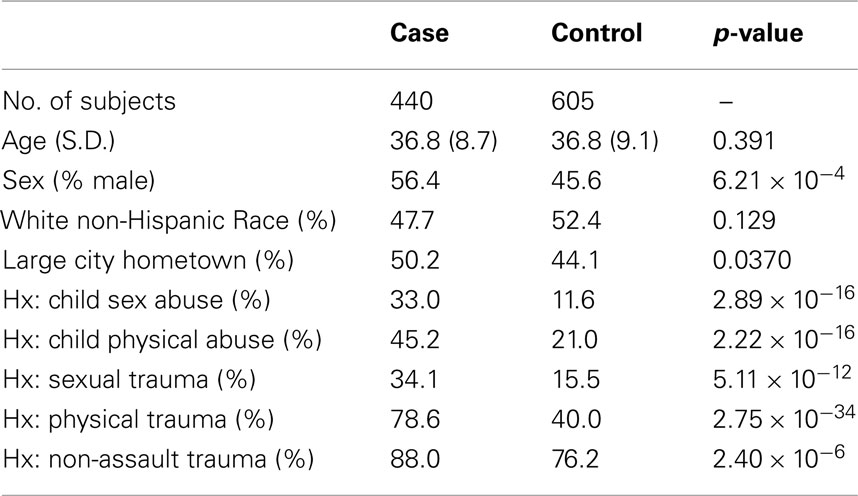
Table 1. Distribution of FSCD-assessed personal characteristics and facets of environment, and the estimated p-value for cocaine dependence associations in this study.
Genome-Environmental Risk Prediction of Cocaine Dependence
When prior risk-prediction studies have focused attention of the modeling process on known risk predictors (e.g., confirmed disease-susceptibility loci), there is a disadvantage. Namely, for many complex traits such as cocaine dependence, the existing disease-susceptibility loci account for no more than a small fraction of the total genetic variation. As such, they are likely to hold limited value in the task of disease prediction. Addressing this limitation of established approaches, we conducted a genome-wide risk-prediction analysis by applying the TA-ROC approach to all available QC-filtered SNPs (i.e., n = 948,658 SNPs, as described above). In this context, risk-prediction model built on such a large ensemble of predictors may be subject to over-fitting due to the presence of noise predictors, which can be quite common when almost one million predictors are being considered. To reduce the number of noise predictors, we used a simple filtering strategy described elsewhere (Wei et al., 2009). As such, it was possible to filter out SNPs based on their single-locus p-value. For each p-value threshold (i.e., 10−2, 10−3, …, 10−6), we selected the corresponding set of SNPs (i.e., filtering out those with a p-value larger than the defined p-value threshold), and applied the TA-ROC method to the selected SNPs to form a risk-prediction model. Adopting a more stringent p-value threshold could filter out some important predictors, while using a less stringent threshold may introduce too many noise loci. To determine the most parsimonious risk-prediction model built on appropriate number of loci, we evaluated risk-prediction models built on each p-value threshold on the out-of-bag (OOB) samples (i.e., the validation samples). Through this analysis, we found a risk-prediction model built on the top three SNPs, all of which passed the most stringent p-value threshold of 10−6. This model achieved limited accuracy in the OOB samples (AUC = 0.580, SD = 0.018). It was possible to increase model accuracy by increasing the range of additional potential risk predictors within the model (Figure 3). Thereafter, the model was amended to include 330 SNPs (i.e., those with p-value less than 10−4), which obtained more optimal performance (AUC = 0.718, SD = 0.016). Addition of more SNPs did not improve the model’s accuracy. In fact, when p-value thresholds of 10−3 and 10−2 were used, the AUC values decreased to 0.712 and 0.705, respectively. Therefore, the model based on 330 SNPs was chosen as the best risk-prediction model from these data, based entirely upon information from the SNPs.
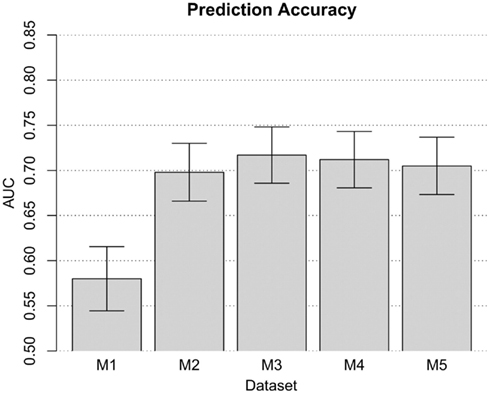
Figure 3. The performance of the risk-prediction model at each p-value threshold. Risk-prediction models M1, M2, M3, M4, and M5, comprised of 3, 38, 330, 2761, and 25460 SNPs with a p-value threshold of 10−6, 10−5, 10−4, 10−3, and 10−2, respectively.
Consistent with contemporary views of various facets of environment that might influence occurrence of cocaine dependence, we extended the SNP-based risk-prediction model development to include variables reflecting facets of environment and experience, as well as personal variables mentioned above. The risk-prediction model built on nine variables on facets of environment and personal characteristics reached medium classification accuracy (AUC = 0.754; SD = 0.015; Figure 4). Combining of 9 non-genetic variables and the 330 genetic variables improved the accuracy of the resulting risk-prediction model (AUC = 0.809; SD = 0.013; Figure 4). We note that the AUC from risk-prediction models based solely in genetic influences was in a range from 0.5 to roughly 0.7. With the addition of the nine additional variables on facets of the environment and personal characteristics, the resulting genome-environmental risk-prediction model reached tangibly high classification accuracy.
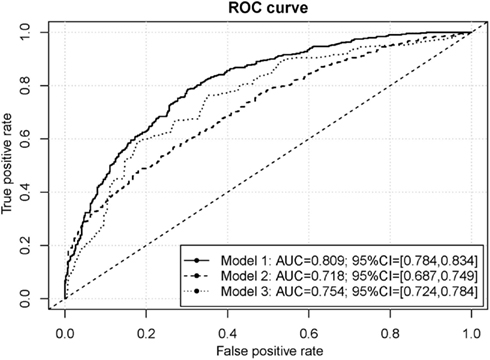
Figure 4. ROC curves for the cocaine dependence risk prediction models. The genome-environmental risk-prediction model (Model 1) is comprised of 330 SNPs and 9 facets of environment, while the genome-wide risk-prediction model (Model 2) is comprised of only 330 SNPs and the environmental risk-prediction model (Model 3) is comprised of the 9 facets of environment.
Contribution of Each Predictor to Cocaine Dependence Risk Prediction
In post-estimation exploratory data analyses (i.e., after AUC estimation), we probed into which characteristics might be important individual predictions of cocaine dependence risk in these data. The contribution of each predictor is measured by dAUC, defined as the average AUC increase as might be attributable to the individual predictor. Table 2 shows the contributions of the top predictors from across the array of SNPs, facets of environment, and personal characteristics. Physical trauma was listed as the most important predictor of cocaine dependence, with a dAUC value of 0.124. Among the remaining environmental conditions, childhood physical abuse, childhood sex abuse, and sexual trauma also contributed significantly to cocaine dependence risk prediction (i.e., ranked 2nd, 3rd, and 4th). The top genetic predictors were 5th to 11th in rank. The top genetic predictors were 5th to 11th in rank. Among the 7 top-ranked SNPs, the first 5 SNPs were all belong to gene GBE1 and were highly correlated (i.e., all pairwise correlation coefficients were great than 0.9). The other two SNPs were located within the gene desert of chromosome 4 and were also highly correlated (i.e., the pairwise correlation coefficient was great than 0.9).
Discussion
Translation of genetic discoveries into useful models for disease prediction has been recognized as an essential step toward enhanced personalized healthcare and disease prevention. In research to date, the risk-prediction models focused on recently discovered genetic loci have lacked sufficient accuracy for clinical use. Since the majority of genetic variants on the genome have not yet been studied for their predictive or functional importance with respect to risk, it may be helpful for improved accuracy and performance if future disease prediction discoveries were to shift toward genome-wide risk-prediction studies of broad range – considering all available genetic variants and minimally plausible facets of environment, experience, and general or specific host characteristics, as well as their possible interactions. In this present study of genome-environmental risk prediction for cocaine dependence, we searched the entire QC-filtered genomic profile of SNPs, as well as nine facets of environment and personal characteristics, and product terms, to devise a genome-environmental risk-prediction model for cocaine dependence. The genome-environmental risk-prediction model reached a high classification accuracy (AUC = 0.809). Even the model with genetic variants alone had a medium level of accuracy (AUC = 0.718). To the best of our knowledge, no previous high-dimensional cocaine dependence risk-prediction model of this type has been developed by others. The results should serve as the first step toward establishing improved prediction models for cocaine dependence. With more validation and investigation, we might be able to refine this cocaine dependence risk-prediction model toward applications as follows: (1) improvements in population-based prevention, (2) improvements in indicated or targeted interventions for high risk population subgroups, and (3) improvements in research, both pre-clinical and clinical, as might help us come to a better understanding of the processes at play when dependence syndromes and serious drug addiction follows initial drug experiences.
There is a potentially important finding in that the cocaine dependence risk-prediction model achieved a noteworthy improvement when the model was extended to include facets of environment and experience such as physical trauma, childhood sex abuse, and sexual trauma. With these variables in the model, in addition to terms for SNP markers, and their interactions, the estimated AUC of the resulting risk-prediction model was as large as 0.8. Among the environmental risk predictors, we found that physical trauma, childhood sex abuse, and childhood physical abuse made noteworthy contributions to cocaine dependence risk prediction. Indeed, in related prior studies, these types of trauma have been found to be strong predictors of drug use disorders (Cottler et al., 1992; Dube et al., 2003; Fergusson et al., 2008; Douglas et al., 2010; Enoch et al., 2010). To illustrate, in one study, Dube et al. (2003) found adverse childhood experiences to be strongly associated with illegal drug taking, and this is not the first study to link childhood trauma with adversities occurring in the context of cocaine problems and other neurobehavioral outcomes in adulthood (Wegman and Stetler, 2009; Narvaez et al., 2012). More specifically, in a case–control study of 832 African-American men, Enoch et al. (2010) found that childhood trauma, as well as its interaction with a specific genetic variant, were associated with increased risk of cocaine dependence. A second finding of potential note is the implication of GBE1, which is a chromosome 3p12 gene that encodes the 1,4-alpha-glucan-branching enzyme, previously noted in relation to the metabolic syndrome and in autosomal recessive glycogen storage syndromes and polyglucosan body disease, with associated disturbances of executive function, often in accompaniment with disturbances of gait, bladder function, and sensation in the distal lower extremities of the body (Ubogu et al., 2005; D’Angelo and Bresolin, 2006). Perhaps of greater importance in the context of cocaine dependence is that GBE1 is linked or predicted to be linked to glycogen synthase kinase 3 beta (GSK3B) in relation to co-expression and co-occurrence, and GSK3B has been described as a mediator for both cocaine reward memory and cocaine-induced behavioral sensitization (Xu et al., 2009, 2011).
The genome-environmental risk-prediction analysis was conducted by utilizing a computationally efficient and powerful approach, TA-ROC. It took only 248 s to form the final genome-environmental risk-prediction model. Compared to existing high-dimensional risk-prediction tools, it has the advantage of considering low-marginal-effect predictors and high-order interactions. As demonstrated by our risk-prediction analysis, TA-ROC could not only assess the model’s overall classification accuracy, but also yields dAUC to evaluate the role of each predictor in risk prediction, which could facilitate our understanding of the functional utility of individual covariates within the risk-prediction model. It must be acknowledged that risk-prediction models built on a large number of predictors may be subject to over-fitting. However, in Wei et al. (2009) and in our previous genome-wide risk-prediction study (Ye et al., 2011), there is evidence of robust performances of the genome-wide risk prediction. In addition, TA-ROC makes use of an assembling process to provide reliable performance, and implements a built-in cross-validation procedure (i.e., the OOB validation), yielding an unbiased accuracy assessment of a risk-prediction model.
The limitations of the study largely are related to the fact that the development of any risk-prediction model must proceed through multiple stages that start with data from retrospective and exploratory investigations. These early studies must show promising results before it is possible to secure resources required to probe the boundary conditions of the risk-prediction models in the form of more definitive prospective studies, experimental trials, and disease impact determinations (Pepe et al., 2001). Nonetheless, our use of a readily available cocaine dependence case–control data archive might be criticized by readers who give greater priority to prospective and longitudinal investigations when the goal is development of early risk-prediction models. With these readers, we share a concern that there should be replication of the study with data from a well-designed, large-scale prospective study or experimental trial (e.g., possibly a current trial underway to prevent cocaine dependence using novel intervention methods).
In conclusion, the risk-prediction model based on the genome profile and an additional six facets of environment, plus three personal characteristics, has achieved a noteworthy level of accuracy in discrimination of cocaine dependence cases from controls. Cocaine risk prediction by genetic information alone did not yield the superior AUC estimates. The risk-prediction model reached high accuracy when genetic information was combined with information about facets of the environment and adverse experiences. To be sure, replication and follow-up studies are needed in order to validate these findings and provide a more comprehensive view of the cocaine dependence risk-prediction model. Ultimately, the application of greatest public health significance may be in the development of new cocaine dependence risk-prediction models for clinical research and application or for use in more population-based prevention and intervention programs, in addition to application for high risk subgroups of the population.
Conflict of Interest Statement
The authors declare that the research was conducted in the absence of any commercial or financial relationships that could be construed as a potential conflict of interest.
Acknowledgments
This work was supported by a start-up fund from Michigan State University and a NIDA K05 Senior Scientist Award (K05DA015799).
References
Anthony, J. C. (2010). Novel phenotype issues raised in cross-national epidemiological research on drug dependence. Ann. N. Y. Acad. Sci. 1187, 353–369.
Bierut, L. J. (2011). Genetic vulnerability and susceptibility to substance dependence. Neuron 69, 618–627.
Bierut, L. J., Agrawal, A., Bucholz, K. K., Doheny, K. F., Laurie, C., Pugh, E., Fisher, S., Fox, L., Howells, W., Bertelsen, S., Hinrichs, A. L., Almasy, L., Breslau, N., Culverhouse, R. C., Dick, D. M., Edenberg, H. J., Foroud, T., Grucza, R. A., Hatsukami, D., Hesselbrock, V., Johnson, E. O., Kramer, J., Krueger, R. F., Kuperman, S., Lynskey, M., Mann, K., Neuman, R. J., Nöthen, M. M., Nurnberger, J. I., Porjesz, B., Ridinger, M., Saccone, N. L., Saccone, S. F., Schuckit, M. A., Tischfield, J. A., Wang, J. C., Rietschel, M., Goate, A. M., Rice, J. P., and As Part of the Gene E. A. S. C. (2010). A genome-wide association study of alcohol dependence. Proc. Natl. Acad. Sci. U.S.A. 107, 5082–5087.
Bierut, L. J., Strickland, J. R., Thompson, J. R., Afful, S. E., and Cottler, L. B. (2008). Drug use and dependence in cocaine dependent subjects, community-based individuals, and their siblings. Drug Alcohol Depend. 95, 14–22.
Compton, W. M., Thomas, Y. F., Conway, K. P., and Colliver, J. D. (2005). Developments in the epidemiology of drug use and drug use disorders. Am. J. Psychiatry 162, 1494–1502.
Cottler, L. B., Compton, W. M., Mager, D., Spitznagel, E. L., and Janca, A. (1992). Posttraumatic-stress-disorder among substance users from the general-population. Am. J. Psychiatry 149, 664–670.
D’Angelo, M. G., and Bresolin, N. (2006). Cognitive impairment in neuromuscular disorders. Muscle Nerve 34, 16–33.
Douglas, K. R., Chan, G., Gelernter, J., Arias, A. J., Anton, R. F., Weiss, R. D., Brady, K., Poling, J., Farrer, L., and Kranzler, H. R. (2010). Adverse childhood events as risk factors for substance dependence: partial mediation by mood and anxiety disorders. Addict. Behav. 35, 7–13.
Dube, S. R., Felitti, V. J., Dong, M., Chapman, D. P., Giles, W. H., and Anda, R. F. (2003). Childhood abuse, neglect, and household dysfunction and the risk of illicit drug use: the adverse childhood experiences study. Pediatrics 111, 564–572.
Enoch, M. A., Hodgkinson, C. A., Yuan, Q. P., Shen, P. H., Goldman, D., and Roy, A. (2010). The influence of GABRA2, childhood trauma, and their interaction on alcohol, heroin, and cocaine dependence. Biol. Psychiatry 67, 20–27.
Evans, D. M., Visscher, P. M., and Wray, N. R. (2009). Harnessing the information contained within genome-wide association studies to improve individual prediction of complex disease risk. Hum. Mol. Genet. 18, 3525–3531.
Fergusson, D. M., Boden, J. M., and Horwood, L. J. (2008). The developmental antecedents of illicit drug use: evidence from a 25-year longitudinal study. Drug Alcohol Depend. 96, 165–177.
Ho, M. K., Goldman, D., Heinz, A., Kaprio, J., Kreek, M. J., Li, M. D., Munafo, M. R., and Tyndale, R. F. (2010). Breaking barriers in the genomics and pharmacogenetics of drug addiction. Clin. Pharmacol. Ther. 88, 779–791.
Kendler, K. S., Karkowski, L. M., Neale, M. C., and Prescott, C. A. (2000). Illicit psychoactive substance use, heavy use, abuse, and dependence in a US population-based sample of male twins. Arch. Gen. Psychiatry 57, 261–269.
Kendler, K. S., and Prescott, C. A. (1998). Cocaine use, abuse and dependence in a population-based sample of female twins. Br. J. Psychiatry 173, 345–350.
Kooperberg, C., Leblanc, M., and Obenchain, V. (2010). Risk prediction using genome-wide association studies. Genet. Epidemiol. 34, 643–652.
Marchini, J., Donnelly, P., and Cardon, L. R. (2005). Genome-wide strategies for detecting multiple loci that influence complex diseases. Nat. Genet. 37, 413–417.
Narvaez, J. C. M., Magalhães, P. V. S., Trindade, E. K., Vieira, D. C., Kauer-Sant’anna, M., Gama, C. S., Von Diemen, L., Kapczinski, N., and Kapczinski, F. (2012). Childhood trauma, impulsivity, and executive functioning in crack cocaine users. Compr. Psychiatry 53, 238–244.
Pepe, M. S., Etzioni, R., Feng, Z. D., Potter, J. D., Thompson, M. L., Thornquist, M., Winget, M., and Yasui, Y. (2001). Phases of biomarker development for early detection of cancer. J. Natl. Cancer Inst. 93, 1054–1061.
Ritchie, M. D., Hahn, L. W., and Moore, J. H. (2003). Power of multifactor dimensionality reduction for detecting gene-gene interactions in the presence of genotyping error, missing data, phenocopy, and genetic heterogeneity. Genet. Epidemiol. 24, 150–157.
Ubogu, E. E., Hong, S. T. K., Akman, H. O., Dimauro, S., Katirji, B., Preston, D. C., and Shapiro, B. E. (2005). Adult polyglucosan body disease: a case report of a manifesting heterozygote. Muscle Nerve 32, 675–681.
Uhl, G. R. (2004). Molecular genetics of substance abuse vulnerability: remarkable recent convergence of genome scan results. Ann. N. Y. Acad. Sci. 1025, 1–13.
Van Den Bree, M. B. M., Johnson, E. O., Neale, M. C., and Pickens, R. W. (1998). Genetic and environmental influences on drug use and abuse/dependence in male and female twins. Drug Alcohol Depend. 52, 231–241.
Wagner, F. A., and Anthony, J. C. (2002). From first drug use to drug dependence: developmental periods of risk for dependence upon marijuana, cocaine, and alcohol. Neuropsychopharmacology 26, 479–488.
Wegman, H. L., and Stetler, C. (2009). A meta-analytic review of the effects of childhood abuse on medical outcomes in adulthood. Psychosom. Med. 71, 805–812.
Wei, Z., Wang, K., Qu, H. Q., Zhang, H. T., Bradfield, J., Kim, C., Frackleton, E., Hou, C. P., Glessner, J. T., Chiavacci, R., Stanley, C., Monos, D., Grant, S. F. A., Polychronakos, C., and Hakonarson, H. (2009). From disease association to risk assessment: an optimistic view from genome-wide association studies on type 1 diabetes. PLoS Genet. 5. e1000678. doi:10.1371/journal.pgen.1000678
Xu, C. M., Wang, J., Wu, P., Xue, Y. X., Zhu, W. L., Li, Q. Q., Zhai, H. F., Shi, J., and Lu, L. (2011). Glycogen synthase kinase 3 beta in the nucleus accumbens core is critical for methamphetamine-induced behavioral sensitization. J. Neurochem. 118, 126–139.
Xu, C. M., Wang, J., Wu, P., Zhu, W. L., Li, Q. Q., Xue, Y. X., Zhai, H. F., Shi, J., and Lu, L. (2009). Glycogen synthase kinase 3 beta in the nucleus accumbens core mediates cocaine-induced behavioral sensitization. J. Neurochem. 111, 1357–1368.
Keywords: cocaine dependence, genome-environmental risk prediction, childhood abuse, GBE1 gene, tree-assembling ROC
Citation: Wei C, Anthony JC and Lu Q (2012) Genome-environmental risk assessment of cocaine dependence. Front. Gene. 3:83. doi: 10.3389/fgene.2012.00083
Received: 17 February 2012; Paper pending published: 05 March 2012;
Accepted: 26 April 2012; Published online: 18 May 2012.
Edited by:
Jielin Sun, Wake Forest University School of Medicine, USAReviewed by:
Xiangqing Sun, Case Western Reserve University, USAXuefeng Wang, Harvard University, USA
Chao Xing, University of Texas Southwestern Medical Center, USA
Sha Tao, Van Andel Institute, USA
Copyright: © 2012 Wei, Anthony and Lu. This is an open-access article distributed under the terms of the Creative Commons Attribution Non Commercial License, which permits non-commercial use, distribution, and reproduction in other forums, provided the original authors and source are credited.
*Correspondence: Qing Lu, Department of Epidemiology and Biostatistics, Michigan State University, B601 West Fee Hall, East Lansing, MI 48824, USA. e-mail:cWx1QGVwaS5tc3UuZWR1