- 1Department of Genetics, Shahid Chamran University of Ahvaz, Ahvaz, Iran
- 2Department of Genetics, School of Biological Sciences, Tarbiat Modares University, Tehran, Iran
- 3Molecular and Integrative Physiological Sciences Program, Department of Environmental Health, Harvard School of Public Health, Boston, MA, USA
Limited understanding of the Rb1 locus hinders genetic and epigenetic analyses of Retinoblastoma, a childhood cancer of the nervous systems. In this study, we used in silico tools to investigate and review putative genetic and epigenetic elements of the Rb1 gene. We report transcription start sites, CpG islands, and regulatory moieties that are likely to influence transcriptional states of this gene. These might contribute genetic and epigenetic information modulating tissue-specific transcripts and expression levels of Rb1. The elements we identified include tandem repeats that reside within or next to CpG islands near Rb1's transcriptional start site, and that are likely to be polymorphic among individuals. Our analyses highlight the complexity of this gene and suggest opportunities and limitations for future studies of retinoblastoma, genetic counseling, and the accurate identification of patients at greater risk of developing the malignancy.
Introduction
The retinoblastoma gene (Rb1) is one of the most widely studied tumor suppressors (Vogelstein and Kinzler, 2004). Retinoblastoma (RB) is a prototype cancer driven in large part by lesions in Rb1, a well-defined genetic element and clinical target. Point mutations, deletions, and epigenetic alterations in Rb1 are also associated with a number of other malignancies (De La Rosa-Velázquez et al., 2007). Recent advances in genomics and epigenomics have made it possible to study RB in novel ways, with approaches combining multiple complementary techniques revealing key genetic and epigenetic steps at the origin of this malignancy (Reis et al., 2012).
Cryptic genetic and epigenetic variation in Rb1 might contribute variation in the progression and drug response of RB tumors. It is plausible that differential penetrance and variation in the age of onset, which have been observed in patients with hereditary and non-hereditary RB, are attributed to epigenetic regulation of Rb1 (Kanber et al., 2009). Three CpG islands (CpG106, 42, and 85) potentially involved in regulation of Rb1 expression have been identified and investigated in detail (Greger et al., 1989). However, uncovering the genetic and epigenetic complexity of the Rb1 locus remains challenging. This is in part due to a lack of complete understanding of the cis-regulatory elements controlling the expression of the gene. Furthermore, evidence of imprinted expression of Rb1 suggests that epigenetic mechanisms might play a central role in the regulation of Rb1 (reviewed in Reis et al., 2012). We expect that comprehensive analyses of the genetic and epigenetic properties of the human Rb1 gene might reveal new aspects underlying its regulation. In this study, we have characterized a number of features of Rb1 and presented some potential mechanisms that might be involved in regulation of this gene. Combining the results of several approaches and databanks will promote a better biological understanding of Rb1, and contribute toward improved clinical management and counseling of RB patients.
Materials and Methods
We combined a set of methods to identify putative functional elements in the Rb1 locus. Our inferences are based on publicly available databases and re-analyses of experimental data. Table 1 lists the softwares used in this study. We defined the Genomic Region under Analysis (GRA) as a sequence that spans from 2 kb upstream of annotated Transcription start site (TSS) of Rb1 to the end of the gene. This was based on previous studies which defined human putative promoter regions as sequences that correspond to −2000 to +1000 bp relative to the TSS (Marino-Ramirez et al., 2004).
Results
Expression of Rb1 and mRNA Isoforms
According to AceView, Rb1 is expressed at 3.1 times the average gene. The database provides a comprehensive and non-redundant sequence representation of public mRNA sequences, and identified 33 potentially distinct GT-AG introns in Rb1 (Thierry-Mieg and Thierry-Mieg, 2006). These result in 17 different mRNAs, 10 of which are produced through alternative splicing. There are 3 probable alternative promoters, 3 non-overlapping alternative last exons, and 3 validated alternative polyadenylation sites (http://www.ncbi.nlm.nih.gov/IEB/Research/Acembly/). One variant has a supporting clone (NM_000321.2) in Refseq database. According to the UCSC browser, there are three different transcripts, one of which is represented by Refseq (Figure 1). Finally, the GNF Atlas indicates that Rb1 is expressed at variable levels across tissues (supplementary Figure 1).
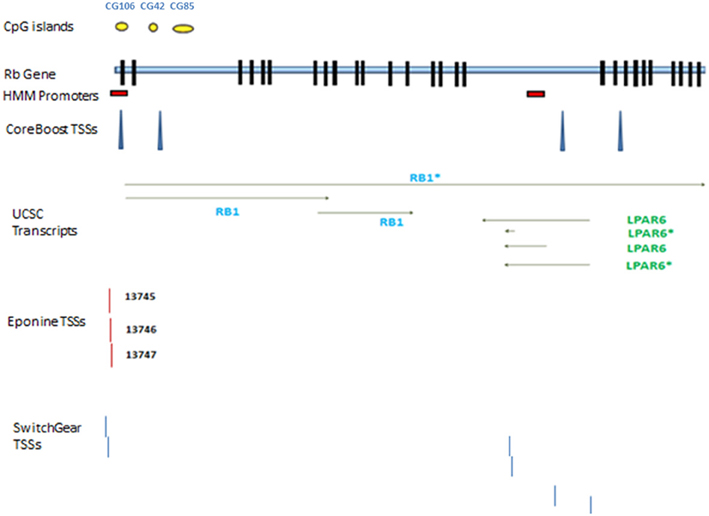
Figure 1. Rb1 gene structure. Transcribed RNAs from this locus identified by UCSC genome browser. The exons are represented by black blocks. Different promoters and transcription start sites of Rb1 locus are shown. The diagram shows a schematic representation of results from different databases and programs which are described in the text. The yellow circles show CpG islands identified by UCSC genome browser. There are two red boxes which show the promoters identified by HMM-Promoter prediction algorithm. TSSs (Transcription start sites) are recognized by different algorithms such as CoreBoost, Eponine, and SwitchGear. LPAR is a gene within Rb1.
Promoters and TSSs
Chromatin state segmentation using Hidden Markov Model (HMM) (Pedersen et al., 1996) indicates that at least two promoters might be found in the Rb1 region. One promoter is near the canonical TSS and another is within one of its introns. According to current annotation, there is a gene named LPAR (P2RY5) within this intron. Alternative splicing of LPAR results in multiple transcript variants. The second active promoter overlaps with TSS of Rb1 (Figure 1). Promoter prediction with CoreBoost_HM identifies 4 hits in the GRA (Figure 1). CoreBoost_HM integrates DNA sequence features with epigenetic information to identify RNA polymerase II core-promoters (Wang et al., 2009). In addition, multiple TSSs were found using Eponine and SwitchGear (Figure 1). “Eponine” provides a probabilistic method for detecting TSS, with good specificity and positional accuracy (Down and Hubbard, 2002). “SwithGear” describes the location of TSSs throughout the genome along with a confidence measure for each TSS based on experimental evidence (http://genome.ucsc.edu/). Finally, the DBTSS database, which is based on the TSS sequencing method (TSS-Seq), suggests that distinct TSSs might be active in different cell lines (Table 2) (Yamashita et al., 2012). Altogether, the results point to alternative promoters and TSSs in the Rb1 gene.
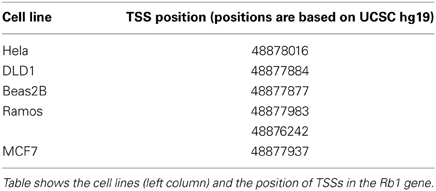
Table 2. Transcription start sites (TSSs) identified in the DBTSS database for different cell lines.
Detection of CpGIs
According to the UCSC browser searching criteria for CpGIs (traditional method), there were 3 CpG islands (Figure 2) in the Rb1 (CGIs106, 42, and 85). UCSC identifies CpGIs of human genes using three criteria: (1) GC content greater than 50%, (2) length greater than 200 bp, and (3) large ratio between observed and expected number of CG dinucleotides (Gardiner-Garden and Frommer, 1987). Further analysis indicates additional putative segments containing CpGs. The “bona fide” strategy integrates genomic and epigenomic information to screen functional CGIs (Bock et al., 2007). We found eight bona fide CpGIs residing within the Rb1 region (CGI 775-83). Three of them demonstrated positional overlap or neighborhood with three CpGIs predicted by traditional methods and previous studies. Only one of the CpGIs (106 in traditional finding and 775 in Bona fide CGIs) was near the canonical TSS of Rb1. The remaining CGIs were in intron 2 (Figure 2). Analysis of the targeted genomic region with the “CpGProD” program points to different CGIs over the length of Rb1 (Figure 2 and Table 3). The program investigates prediction of promoter-overlapping CGIs with a longer length and greater CpGo/e ratio compared with non-overlapping start site CGIs (Ponger and Mouchiroud, 2002). Further, the “CpG cluster” program detects CpGIs based on the distance between neighboring CpGs. Because a minimum threshold length is not required, CpG cluster can find short but fully functional CGIs usually missed by other algorithms. In our study, most of the CpGs identified by this program overlap with the bona fide CGI regions (Table 3). Finally, the “Weizmann Evolutionary CpGIs” identified two different CpGIs (CpG2 and 2.6) (Figure 2). This custom track of UCSC predicts genome's regulatory elements with highly conserved sequences. Table 3 shows a comparison of the CpGIs positions identified by different programs.
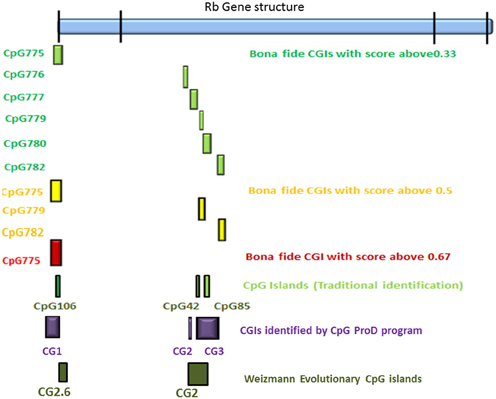
Figure 2. The positions of CpG islands in the Rb1 locus. The first and last blocks in the schematic gene represent the first and fourth exons of Rb1, respectively. “Bona fide” strategy accounts for a number of functional CGIs and estimates their strengths (see scores in the figure). Also, CpGProD program predicts promoter- overlapping CGIs. “Weizmann CpG islands” predicts highly conserved CGIs. Although different methods were used, the results are largely concordant.
Estimation of the CGI's Methylation Status
Several programs can be used to predict CGIs methylation status (Carson et al., 2008). The scores reflect the ability of each CGI to maintain its unmethylated state. All genomic CGIs are grouped into four sets: B1(0–0.33), B2(0.33–0.50), B3(0.50–0.67), and B4(0.67–1), whereby CGIs with combined scores >0.5 represent CGIs that are strongly associated with epigenetic regulatory function (http://epigraph.mpi-inf.mpg.de/download/CpG_islands_revisited/). Also, we evaluated two other indicators of methylation status in CGIs: the over-representation of CCGC motif within sequences of CpG islands (Bock et al., 2007) and the presence of H3K4me3 marks in CGIs (Su et al., 2010). We found three CpG islands (CpG775, 779, and 782) within groups B3 and B4. All these CpG islands had CCGC motif in their sequences. Also, we observed other regions which were methylated in different cell lines of ENCODE project (http://genome.ucsc.edu/cgi-bin/hgTracks?position=chr13:48875883-49056026&hgsid=347686961&wgEncodeHaibMethyl450=dense).
Tandem Repeats
By using “Estimation of Repeat Variability” toolkit, we found multiple tandem repeats in the GRA (Table 4). Three characteristics of the repeats (number of repeated units, unit length, and purity) were considered to produce a numeric “VARscore,” which correlates with repeat variability (Legendre et al., 2007). In our result, CGI-775, which includes the TSS of Rb1 locus, is over a 3 bp unit VNTR. The sequence of this VNTR is: GCCGCCGCCACCGCCGCCGCTGCCGCCGCGGACCCCCGGCACCGCCGCCGCCGCC. Hence, longer alleles can add CpGs to the number of methylatable sites. Another tandem repeat identified by this software is downstream of CpGI number 6 recognized by CpG cluster. CpGI 6 was not found by bona fide as a functional island, but we observed that the CCGC motif is represented 4 times in the segment that includes CpGI 6 and the VNTR. Also, inspection for transcription factor binding sites in this segment by “TFSearch” software, indicates that there is CREB binding site motif in this region. Enrichment of representation of binding site of this transcription factor characterizes methylation free CpG islands (Tate and Bird, 1993; Sunahori et al., 2009).
Inspecting Histone Marks
We observed H3K4me1 and H3K4me3 through the annotated core Rb1 promoter (supplementary Figure 2). The observation was made with data from the ENCODE project. H3K4me1 and H3K4me3 positive marks were mostly mirroring the acetylated histones. It is of note that the regions of histone marks mostly overlapped with CGI-775 and promoters identified by different programs.
DNase I Hypersensitive Sites (DNase I HS)
We used DNase Clusters track in UCSC genome browser. In the Rb1 promoter, positions of the DNase I HS sites vary depending on cell line assayed. Notably, DNase I HS sites are mostly mapped to CGI_775, which overlaps with CG106. Also, we found that some of these hypersensitive sites are overlapped with or adjacent to other predicted CpGIs.
Transcription Factor Binding Sites
“CisRed” and “PReMode” databases were used to detect the boundaries of regulatory regions and TFBs motifs distribution. CisRed summarizes conserved sequence motifs identified by genome scale motif discovery, similarity, clustering, co-occurrence, and coexpression calculations (Robertson et al., 2006). The algorithm used in PReMode predicts transcriptional regulatory modules (Ferretti et al., 2007) in which a number of transcription factors can bind and regulate expression of nearby genes (Ben-Tabou De-Leon and Davidson, 2007; Teif, 2010). There were three modules concentrated within or next to CpGs around TSS. Two modules were near the canonical TSS. Finally, the ENCODE results in UCSC point to regions with abundant binding of transcription factors.
Insulator Sites
A comprehensive collection of experimentally determined and computationally predicted CTCF binding sites have been curated in the “CTCFBSDB” database (Bao et al., 2008). We observed 6 putative sites for CTCF binding in GRA, two of which are located in CpGI-775 (Table 5).
Discussion
Neural progenitor cells dynamically interact with their environment (Jones and Laird, 1999). The expanded two hit hypothesis proposes that both genetic and epigenetic aberrations are involved in silencing of tumor suppressor genes in cancers such as RB (Jones and Laird, 1999). Studies have shown the role of epigenetic mechanisms in Rb1 regulation (Reviewed in Reis et al., 2012), but the exact elements and their relation with cis regulatory elements already identified as important for Rb1 expression has remained elusive. Here we used in silico analyses and databases to identify and summarize putative regulatory elements that might contribute to Rb1 regulation. Identification of these elements suggests new venues for understanding Rb1 expression and its contribution to disease states. The analyses reinforce the notion that a variety of distinct epigenetic and genetic elements are involved in the control of the activity of the human Rb1 gene.
A study by Greger et al. (1989) was among the first to provide evidence that changes in the methylation of Rb1 might play a role in the emergence and progression of RB tumors. They found that CpG106, which overlaps the Rb1 promoter and exon E1, is methylated in some RB cases. Two other CpGs (CpG 42 and 85) were investigated in other studies. Kanber et al. (2009) observed that an alternative transcript of Rb1 is preferentially expressed from the maternal allele. It seems that imprinted expression of Rb1 is linked to a differentially methylated CpG island in intron 2 of this gene (CpG-85) (Kanber et al., 2009). Also, it has been reported that CpG 42 is biallelically methylated, whereas CpG-106 is biallelically unmethylated (Buiting et al., 2010).
We identified additional CpG islands in the Rb1 locus and sought to assess their epigenetic state by evaluating other data such as co-occurrence of histone modifications, DNAse 1 sensitivity, transcription factor binding sites, and presence of genomic insulators. One possibility is that these genetic and epigenetic features cooperate to fine tune Rb1 regulation. Our observations highlight two points. First, the Rb1 locus includes multiple genomic elements exhibiting potential sensitivity to differential DNA methylation and histone modification. Independent tools identified multiple CpG islands in the locus. In spite of differences between softwares, all of them pointed to multiple CpGs, some of which were corroborated by multiple lines of evidence. These are promising targets for downstream functional analysis. Second, repeats occur within or next to some CpG islands. Hence we expect that the methylation status of the Rb1 regulatory regions in genomes of different individuals might be affected by repeat number variations in nearby sequences. The potential contribution of these regions to the epigenetic regulation of Rb1 alleles might be worthy of further study. Individual methylation profile might lead to variable expressivity and penetrance in different patients.
Several mammalian genes contain more than a single TSS (Valen et al., 2009) and Rb1 does not appear to be an exception. Genes with alternative promoters, often display only one promoter with a CGI (Cheong et al., 2006). On the other hand, most of the putative alternative promoters of Rb1 are distributed in or next to putative CpG islands. Since methylation sensitive regions carry distinctly different information about gene expression and exhibit different sensitivity to regulatory signals, this type of positioning should not be neglected. Besides, DNA methylation appears to play a significant role in differential usage of alternative promoters and be related to functional diversification between CpGI-containing promoters and CpGI-less promoters. Furthermore, chromatin marks and transcription elements such as enhancers or insulators could cause differential expression levels in Rb1 or even differential usage of the gene's TSSs. The presence of multiple regulatory elements within the locus confers combinatorial control of regulation through which the number of unique expression states can increase (Maston et al., 2006).
The distribution and amount of histone marks like H3K4me1-3 provide a basis for nucleosome positioning in the Rb1 locus. H3K4me1 is associated with enhancers and DNA regions downstream of TSSs. The H3K4me3 histone mark is associated with promoters that are active or poised to be activated (Karliæ et al., 2010). This histone mark seems to be an indicator of functional CpG islands (Su et al., 2010). We observed an overlap between the regions including this mark and predicted CpGIs (supplementary Figure 2).
It has been reported that DNA methylation correlates with DNase 1 hypersensitivity (Crawford et al., 2006). We found that DNase 1 hypersensitive regions mapped to CGI_775. This CpG island overlaps with the canonical promoter of Rb1 and this observation is in agreement with studies indicating that regulatory regions in the promoters tend to be DNase sensitive (Crawford et al., 2006). Noteworthy, we observed several CTCF binding sites in the Rb1 locus. In vertebrates, the transcription regulator CCCTC-binding factor (CTCF) is the only trans-acting factor that is a primary part of insulator sequences that block the interaction between enhancers and promoters (Ohlsson et al., 2001). Hence, CTCF is at the core of the machinery that exerts epigenetic control of diverse imprinted loci and participates in promoter activation and repression. Evidence points toward a role for the 11-zinc finger CCCTC-binding factor (CTCF) in the establishment of DNA methylation free zones and the regulation of cell cycle–related genes (Tang et al., 2002; Filippova et al., 2005). CTCF-bound insulators separate transcriptionally active and silent chromatin domains, with their function depending strongly on the local status of DNA methylation and chromatin modifications. It has been suggested that active genes have a DNA fragment with insulator properties and CTCF binding sites in their 5' ends (Filippova et al., 2005).
Numerous experimental and clinical studies investigate the role of DNA methylation and other epigenetic marks in human diseases (Kanwal and Gupta, 2012). However, in spite of genome-wide patterns, the association between genomic polymorphisms and altered epigenetic status of specific genes is elusive. One interesting possibility is that genetic variations in the Rb1 gene (including VNTRs) might contribute to the methylation status of the region. Hence, experimental methylation analysis would benefit most if coupled with the sequencing of primary genomic samples. Furthermore, genetic variations in repetitive segments not usually targeted in mutation screens might enable a better understanding of unexpected confounders due to personal genome variation. The proposed set of Rb1 regulatory elements offers venues to understand the developmental dynamics and individual variation in the expression of the Rb1 gene. Altogether, we expect that interactions between genetic and epigenetic elements of Rb1 might cause tissue-specific alternative transcripts, different expression level, and possibly variable penetrance and disease severity in patients with RB.
Conflict of Interest Statement
The authors declare that the research was conducted in the absence of any commercial or financial relationships that could be construed as a potential conflict of interest.
Supplementary Material
The Supplementary Material for this article can be found online at: http://www.frontiersin.org/journal/10.3389/fgene.2014.00002/abstract
References
Bao, L., Zhou, M., and Cui, Y. (2008). CTCFBSDB: a CTCF-binding site database for characterization of vertebrate genomic insulators. Nucleic Acids Res. 36, D83–D87. doi: 10.1093/nar/gkm875
Ben-Tabou De-Leon, S., and Davidson, E. H. (2007). Gene regulation: gene control network in development. Annu. Rev. Biophys. Biomol. Struct. 36, 191. doi: 10.1146/annurev.biophys.35.040405.102002
Bock, C., Walter, J., Paulsen, M., and Lengauer, T. (2007). CpG island mapping by epigenome prediction. PLoS Comput. Biol. 3:e110. doi: 10.1371/journal.pcbi.0030110
Buiting, K., Kanber, D., Horsthemke, B., and Lohmann, D. (2010). Imprinting of RB1(the new kid on the block). Brief. Funct. Genomics 9, 347–353. doi: 10.1093/bfgp/elq014
Carson, M. B., Langlois, R., and Lu, H. (2008). Mining knowledge for the methylation status of CpG islands using alternating decision trees. Conf. Proc. IEEE Eng. Med. Biol. Soc. 2008, 3787–3790. doi: 10.1109/IEMBS.2008.4650033
Cheong, J., Yamada, Y., Yamashita, R., Irie, T., Kanai, A., Wakaguri, H., et al. (2006). Diverse DNA methylation statuses at alternative promoters of human genes in various tissues. DNA Res. 13, 155–167. doi: 10.1093/dnares/dsl008
Crawford, G. E., Davis, S., Scacheri, P. C., Renaud, G., Halawi, M. J., Erdos, M. R., et al. (2006). DNase-chip: a high-resolution method to identify DNase I hypersensitive sites using tiled microarrays. Nat. Meth. 3, 503–509. doi: 10.1038/nmeth888
De La Rosa-Velázquez, I. A., Rincón-Arano, H., Benítez-Bribiesca, L., and Recillas-Targa, F. (2007). Epigenetic regulation of the human retinoblastoma tumor suppressor gene promoter by CTCF. Cancer Res. 67, 2577–2585. doi: 10.1158/0008-5472.CAN-06-2024
Down, T. A., and Hubbard, T. J. (2002). Computational detection and location of transcription start sites in mammalian genomic DNA. Genome Res. 12, 458–461. doi: 10.1101/gr.216102
Ferretti, V., Poitras, C., Bergeron, D., Coulombe, B., Robert, F., and Blanchette, M. (2007). PReMod: a database of genome-wide mammalian cis-regulatory module predictions. Nucleic Acids Res. 35, D122–D126. doi: 10.1093/nar/gkl879
Filippova, G. N., Cheng, M. K., Moore, J. M., Truong, J. P., Hu, Y. J., Nguyen, D. K. et al. (2005). Boundaries between chromosomal domains of X inactivation and escape bind CTCF and lack CpG methylation during early development. Dev. cell 8, 31–42. doi: 10.1016/j.devcel.2004.10.018
Gardiner-Garden, M., and Frommer, M. (1987). CpG islands in vertebrate genomes. J. Mol. Biol. 196, 261–282. doi: 10.1016/0022-2836(87)90689-9
Greger, V., Passarge, E., Hǒpping, W., Messmer, E., and Horsthemke, B. (1989). Epigenetic changes may contribute to the formation and spontaneous regression of retinoblastoma. Hum. Genet. 83, 155–158. doi: 10.1007/BF00286709
Jones, P. A., and Laird, P. W. (1999). Cancer epigenetics comes of age. Nat. Genet. 21, 163–167. doi: 10.1038/5947
Kanber, D., Berulava, T., Ammerpohl, O., Mitter, D., Richter, J., Siebert, R., et al. (2009). The human retinoblastoma gene is imprinted. PLoS Genet. 5:e1000790. doi: 10.1371/journal.pgen.1000790
Kanwal, R., and Gupta, S. (2012). Epigenetic modifications in cancer. Clin. Genet. 81, 303–311. doi: 10.1111/j.1399-0004.2011.01809.x
Karliæ, R., Chung, H. R., Lasserre, J., Vlahovicek, K., and Vingron, M. (2010). Histone modification levels are predictive for gene expression. Proc. Natl. Acad. Sci. U.S.A. 107, 2926–2931. doi: 10.1073/pnas.0909344107
Legendre, M., Pochet, N., Pak, T., and Verstrepen, K. J. (2007). Sequence-based estimation of minisatellite and microsatellite repeat variability. Genome Res. 17, 1787–1796. doi: 10.1101/gr.6554007
Marino-Ramirez, L., Spouge, J. L., Kanga, G. C., and Landsman, D. (2004). Statistical analysis of over-represented words in human promoter sequences. Nucleic Acids Res. 32, 949–958. doi: 10.1093/nar/gkh246
Maston, G. A., Evans, S. K., and Green, M. R. (2006). Transcriptional Regulatory Elements in the Human Genome. Annu. Rev. Genomics Hum. Genet. 7, 29–59. doi: 10.1146/annurev.genom.7.080505.115623
Ohlsson, R., Renkawitz, R., and Lobanenkov, V. (2001). CTCF is a uniquely versatile transcriptional regulator linked to epigenetics and disease. Trends Genet. 17, 520–527. doi: 10.1016/S0168-9525(01)02366-6
Pedersen, A. G., Baldi, P., Brunak, S., and Chauvin, Y. (1996). Characterization of prokaryotic and eukaryotic promoters using hidden Markov models. Proc. Int. Conf. Intell. Syst. Mol. Biol. 4, 182–191.
Ponger, L., and Mouchiroud, D. (2002). CpGProD: identifying CpG islands associated with transcription start sites in large genomic mammalian sequences. Bioinformatics 18, 631–633. doi: 10.1093/bioinformatics/18.4.631
Reis, A. H., Vargas, F. R., and Lemos, B. (2012). More epigenetic hits than meets the eye: microRNAs and genes associated with the tumorigenesis of retinoblastoma. Front. Genet. 3, 284. doi: 10.3389/fgene.2012.00284
Robertson, G., Bilenky, M., Lin, K., He, A., Yuen, W., Dagpinar, M. et al. (2006). cisRED: a database system for genome-scale computational discovery of regulatory elements. Nucleic Acids Res. 34, D68–D73. doi: 10.1093/nar/gkj075
Su, J., Zhang, Y., Lv, J., Liu, H., Tang, X., and Wang, F. (2010). CpG_MI: a novel approach for identifying functional CpG islands in mammalian genomes. Nucleic Acids Res. 38, e6. doi: 10.1093/nar/gkp882
Sunahori, K., Juang, Y. T., and Tsokos, G. C. (2009). Methylation status of CpG islands flanking a cAMP response element motif on the protein phosphatase 2Ac alpha promoter determines CREB binding and activity. J. Immunol. 182, 1500–1508.
Tang, M. H., Klenova, E. M., Morse, H.C. 3rd., Ohlsson, R., and Lobanenkov, V. V. (2002).The novel BORIS + CTCF gene family is uniquely involved in the epigenetics of normal biology and cancer. Semin. Cancer Biol. 12, 399–414. doi: 10.1016/S1044-579X(02)00060-3
Tate, P. H., and Bird, A. P. (1993). Effects of DNA methylation on DNA-binding proteins and gene expression. Curr. Opin. Genet. Dev. 3, 226–231. doi: 10.1016/0959-437X(93)90027-M
Teif, V. B. (2010). Predicting Gene-Regulation Functions: Lessons from Temperate Bacteriophages. Biophys. J. 98, 1247–1256. doi: 10.1016/j.bpj.2009.11.046
Thierry-Mieg, D., and Thierry-Mieg, J. (2006). AceView: a comprehensive cDNA-supported gene and transcripts annotation. Genome Biol. 7(Suppl 1 S12), 11–14. doi: 10.1186/gb-2006-7-s1-s12
Valen, E., Pascarella, G., Chalk, A., Maeda, N., Kojima, M., Kawazu, C., et al. (2009). Genome-wide detection and analysis of hippocampus core promoters using DeepCAGE. Genome Res. 19, 255–265. doi: 10.1101/gr.084541.108
Vogelstein, B., and Kinzler, K. W. (2004). Cancer genes and the pathways they control. Nat. Med. 10, 789–799. doi: 10.1038/nm1087
Wang, X., Xuan, Z., Zhao, X., Li, Y., and Zhang, M. Q. (2009). High-resolution human core-promoter prediction with CoreBoost_HM. Genome Res. 19, 266–275. doi: 10.1101/gr.081638.108
Keywords: retinoblastoma, epigenetics, CpG islands, in silico analysis
Citation: Hajjari M, Khoshnevisan A and Lemos B (2014) Characterizing the Retinoblastoma 1 locus: putative elements for Rb1 regulation by in silico analysis. Front. Genet. 5:2. doi: 10.3389/fgene.2014.00002
Received: 29 September 2013; Accepted: 03 January 2014;
Published online: 28 January 2014.
Edited by:
Yoshimasa Saito, Keio University, JapanCopyright © 2014 Hajjari, Khoshnevisan and Lemos. This is an open-access article distributed under the terms of the Creative Commons Attribution License (CC BY). The use, distribution or reproduction in other forums is permitted, provided the original author(s) or licensor are credited and that the original publication in this journal is cited, in accordance with accepted academic practice. No use, distribution or reproduction is permitted which does not comply with these terms.
*Correspondence: Mohammadreza Hajjari, Faculty of Science, Department of Genetics, Shahid Chamran University of Ahvaz, Golestan Ave., Ahvaz, Iran e-mail: mohamad.hajari@gmail.com;
Bernardo Lemos, Molecular and Integrative Physiological Sciences Program, Department of Environmental Health, Harvard School of Public Health, Bldg 2, Rm 219, Boston, MA 02115, USA e-mail: blemos@hsph.harvard.edu