- 1Department of Medicine, Vanderbilt University, Nashville, TN, USA
- 2Department of Biomedical Informatics, Vanderbilt University, Nashville, TN, USA
- 3Office of Research, Vanderbilt University, Nashville, TN, USA
- 4Department of Molecular Physiology and Biophysics, Center for Human Genetics Research, Vanderbilt University, Nashville, TN, USA
- 5Department of Pharmacology, Vanderbilt University, Nashville, TN, USA
- 6Vanderbilt Epidemiology Center, Vanderbilt University, Nashville, TN, USA
- 7Center for Human Genetics, Marshfield Clinic Research Foundation, Marshfield, WI, USA
- 8Biomedical Informatics Research Center, Marshfield Clinic Research Foundation, Marshfield, WI, USA
- 9Division of Genomic Medicine, National Human Genome Research Institute, Bethesda, MD, USA
- 10Department of Medicine, University of Washington, Seattle, WA, USA
- 11Department of Biochemistry and Molecular Biology, Center for Systems Genomics, The Pennsylvania State University, University Park, PA, USA
- 12Divisions of Biomedical Informatics and Statistics, Mayo Clinic, Rochester, MN, USA
- 13Division of Epidemiology, Mayo Clinic, Rochester, MN, USA
- 14Group Health Research Institute, Seattle, WA, USA
- 15Department of Genome Sciences, University of Washington, Seattle, WA, USA
- 16Genomic Medicine Institute, Geisinger Health System, Danville, PA, USA
- 17Weis Center for Research, Geisinger Health System, Danville, PA, USA
- 18Essentia Institute of Rural Health, Duluth, MN, USA
- 19Division of Cardiovascular Diseases, Mayo Clinic, Rochester, MN, USA
- 20The Charles Bronfman Institute for Personalized Medicine, Icahn School of Medicine at Mount Sinai, New York, NY, USA
- 21Department of Cell and Molecular Biology, Feinberg School of Medicine, Northwestern University, Evanston, IL, USA
Phenome-wide association studies (PheWAS) have demonstrated utility in validating genetic associations derived from traditional genetic studies as well as identifying novel genetic associations. Here we used an electronic health record (EHR)-based PheWAS to explore pleiotropy of genetic variants in the fat mass and obesity associated gene (FTO), some of which have been previously associated with obesity and type 2 diabetes (T2D). We used a population of 10,487 individuals of European ancestry with genome-wide genotyping from the Electronic Medical Records and Genomics (eMERGE) Network and another population of 13,711 individuals of European ancestry from the BioVU DNA biobank at Vanderbilt genotyped using Illumina HumanExome BeadChip. A meta-analysis of the two study populations replicated the well-described associations between FTO variants and obesity (odds ratio [OR] = 1.25, 95% Confidence Interval = 1.11–1.24, p = 2.10 × 10−9) and FTO variants and T2D (OR = 1.14, 95% CI = 1.08–1.21, p = 2.34 × 10−6). The meta-analysis also demonstrated that FTO variant rs8050136 was significantly associated with sleep apnea (OR = 1.14, 95% CI = 1.07–1.22, p = 3.33 × 10−5); however, the association was attenuated after adjustment for body mass index (BMI). Novel phenotype associations with obesity-associated FTO variants included fibrocystic breast disease (rs9941349, OR = 0.81, 95% CI = 0.74–0.91, p = 5.41 × 10−5) and trends toward associations with non-alcoholic liver disease and gram-positive bacterial infections. FTO variants not associated with obesity demonstrated other potential disease associations including non-inflammatory disorders of the cervix and chronic periodontitis. These results suggest that genetic variants in FTO may have pleiotropic associations, some of which are not mediated by obesity.
Introduction
Pleiotropy, the phenomenon in which a single gene or genetic variant is associated with multiple phenotypes, is essential to the functionality of the human genome (Crespi, 2010; Wagner and Zhang, 2011; Pavlicev and Wagner, 2012). Through comparing multiple genome-wide association studies (GWAS) and candidate gene studies, pleiotropy has been noted in many single nucleotide polymorphisms (SNPs) and genes, potentially providing greater insight into putative biological mechanisms (Sivakumaran et al., 2011; Stranger et al., 2011; Solovieff et al., 2013). The increasing prevalence of DNA biobanks linked to rich phenotype resources and large epidemiological databases have enabled the development of phenome-wide association study (PheWAS) method as an additional tool to investigate pleiotropy (Denny et al., 2010a; Pendergrass et al., 2011). As a complement to GWAS, PheWAS enables both the validation of genotype-phenotype associations identified through traditional GWAS and the generation of new hypotheses, identifying potentially novel associations as well as putative instances of genetic pleiotropy (Denny et al., 2011; Pendergrass et al., 2013). A recent application of PheWAS to 3144 GWAS-identified variants, replicated 210 known associations and noted 63 new, pleiotropic associations (Denny et al., 2013).
The Electronic Medical Records and Genomics (eMERGE) Network was formed in 2007 to use phenotypes derived from electronic health record (EHR) data to perform GWAS and other genomic investigations (Kullo et al., 2011; Pathak et al., 2011; Crosslin et al., 2013; Ding et al., 2013). eMERGE investigators have also used EHR-based PheWAS methods to evaluate multiple phenotypes associated with specific genetic variants (Denny et al., 2010a; Pathak et al., 2012; Hebbring et al., 2013). PheWAS has been used to enhance our understanding of the genetic determinants of complex traits discovered through GWAS. For example, a PheWAS of variants associated with longer cardiac conduction (Ritchie et al., 2013) demonstrated an association with atrial fibrillation, and a PheWAS of variants affecting platelet count and size identified associations with autoimmune diseases (Shameer et al., 2013).
Variants in the fat mass and obesity associated gene (FTO) have been studied since 2007, when it was discovered that some were associated with body mass index (BMI) and obesity (Frayling et al., 2007). Multiple GWAS have demonstrated further associations between variants in FTO and obesity (Jacobsson et al., 2012). Some of these variants have also been noted to be associated with both obesity and type 2 diabetes (T2D) (Hertel et al., 2011; Rees et al., 2011; Li et al., 2012) including SNPs rs9939609 and rs8050136, which are in high linkage disequilibrium (LD) with each other in people of European ancestry (r2 = 1.00; using 1000 Genomes Pilot 1 reference in the CEU population). The SNP rs8050136 is located in an intronic region where the transcription factor cut-like homeobox (CUTL1) protein (Li et al., 2000) is predicted to bind (Stratigopoulos et al., 2008). This variant has been associated with T2D and obesity in Han Chinese and European populations (Hubacek et al., 2008; Liu et al., 2010; Hotta et al., 2011) but other studies found no association between this variant and T2D or obesity in the Chinese Han population (Li et al., 2008; Xi and Mi, 2009). These differences in associations of SNPs with phenotypes have been further analyzed through fine mapping of BMI loci (Gong et al., 2013). This study reported that GWAS studies primarily performed in European populations of numerous loci associated with BMI are not generalizable to other ethnic groups, for example African Americans. Another study demonstrated that rs8050136 was associated with increased energy intake from fat with similar total energy intake (Park et al., 2013). A more recent study noted that the mechanism of action for common variants in FTO may be through regulation of IRX3 expression, which is highly expressed in the brain (Smemo et al., 2014).
There is also evidence of other putative disease associations with FTO variants that have not achieved genome-wide significance, such as pancreatic cancer, Alzheimer's disease, attention deficit hyperactivity disorder, alcoholism, and osteoarthritis (Keller et al., 2011; Lurie et al., 2011; Sobczyk-Kopciol et al., 2011; arcOGEN Consortium et al., 2012; Corella et al., 2012; Reitz et al., 2012; Velders et al., 2012). These varied disease-SNP associations suggest that SNPs in FTO may have pleiotropic effects. Utilizing the population and diagnostic diversity contained within the real-world clinical environment for variants within FTO, our goal was to determine whether an EHR-based PheWAS could identify genetic pleiotropy that might otherwise remain undetected in traditional cohort study designs. In the present study, we utilized PheWAS method and data sets from the eMERGE network (McCarty et al., 2011; Gottesman et al., 2013) to evaluate pleiotropy of variants in FTO.
Materials and Methods
Participation of eMERGE Sites
The eMERGE Network data used in this study consists of seven institutions (Group Health Cooperative and University of Washington, Marshfield Clinic, Mayo Clinic, Northwestern University, Mount Sinai, Geisinger Health System, and Vanderbilt University Medical Center), each with DNA biorepositories linked to their EHRs. Each site pulled demographic, vital sign, and billing data from their EHR research data repositories for this study. All projects were either approved by local IRBs or classified as IRB exempt as non-human subjects research.
Genotyping of eMERGE Subjects
Variants for eMERGE subjects were selected from extant genome-wide genotypes with either the Human660W-Quadv1_A or Illumina OmniExpress chips. The Human660W-Quadv1_A BeadChip was completed at the Center for Genotyping and Analysis at the Broad Institute, and the Center for Inherited Disease Research at Johns Hopkins University. Genotyping for Illumina OmniExpress BeadChips was performed at the University of Pittsburgh Genomics and Proteomics Core Laboratories. These genotyping data comprised 10,487 individuals of European ancestry, as designated in the EHRs.
Quality-control (QC) of the genotype data was performed using a pipeline developed by the eMERGE Genomics Working Group (Turner et al., 2011). This process included call rate restrictions listed below, identification of sex mismatch and anomalies, checking duplicate and HapMap concordance, as well as identifying batch effects, sample relatedness, and minor allele frequency (MAF). Population stratification was evaluated using STRUCTURE (Pritchard et al., 2000) and EIGENSTRAT (Price et al., 2006). Only SNPs with call rates >99% and MAF >0.01 in unrelated samples were included for further study. Relatedness was determined on the basis of identity by descent (IBD) estimates generated from the genome-wide genotype data in PLINK (Purcell et al., 2007). All study sites had pairs of individuals with an IBD estimate greater than 0.25; only one of the individuals in each related pair was randomly selected and used in the analysis. Additional genotypes were imputed using IMPUTE2 (Marchini et al., 2007) and 1000 Genomes Project as the reference (1000 Genomes Project Consortium et al., 2010). We used imputed SNPs with a minimum info score of 0.7 and called genotypes based on the maximum posterior probability.
We selected 54 SNPs, of which 51 were imputed in at least one site, located in FTO that met the QC criteria above and were previously associated with obesity (Jacobsson et al., 2012). QC and subsequent association tests were performed using PLINK (Purcell et al., 2007) and the R statistical package (R Core Team, 2013).
Genotyping of Vanderbilt Subjects Using HumanExome BeadChips
We selected 13,711 individuals of European ancestry from the BioVU DNA databank with BMI data who were genotyped using the Illumina Infinium HumanExome BeadChip, which includes >240,000 markers, mostly within exonic regions, as well as SNPs from the GWAS catalog (Welter et al., 2014) including rs8050136 in FTO. Genotyping was performed at the Vanderbilt Technologies for Advanced Genomics (VANTAGE) Core, and genomic data were processed by the Vanderbilt Technologies for Advanced Genomics Analysis and Research Design (VANGARD) Core. Clustering was performed using GenomeStudio's GenTrain clustering algorithm followed by manual review and reclustering; genotype calling was performed using GenomeStudio's GenCall algorithm. Genotyping quality was evaluated using SNP call rates and concordance rates with HapMap controls; SNPs with <99.8% call rate or <98% concordance were excluded. In the first analysis, we focused on rs8050136, which had a call rate of >99.9%. In the subsequent analyses, we further analyzed eight FTO SNPs on the Exome chip, which had call rates greater than 99.8% and MAFs >0.01. Similar to the eMERGE set, for individuals with an IBD estimate greater than 0.25; only one of the individuals in each related group was selected randomly and used in our analyses.
PheWAS Analyses
We first tested the 54 eMERGE SNPs for association with BMI using linear regression. We calculated LD with our reference SNP rs8050136, chosen as the reference because of its GWAS associations with BMI and T2D in the literature and since it was directly genotyped on all of the platforms. To evaluate phenotype associations and potential pleiotropy among different FTO SNPs, we grouped SNPs into three groups for convenience based on their LD with rs805136: high LD (r2 > 0.80), moderate LD (0.80 ≥ r2 > 0.60) and low LD (r2 ≤ 0.60) with rs8050136. Our hypothesis was that SNPs in high LD would show similar patterns of phenotype associations with rs8050136, and that different patterns may be observed in SNPs with lower LD.
Analyses for the eMERGE and the BioVU datasets were conducted separately and then meta-analyzed. The eMERGE population had 54 SNPs and the BioVU population had nine SNPs for analysis, which were also present in the eMERGE dataset. We used logistic regression adjusted for age, sex, eMERGE site, and the first three principal components as calculated for each dataset by EIGENSTRAT, using an additive genetic model. We performed PheWAS using each SNP using methods and code groupings described previously (Denny et al., 2013) using the R PheWAS package (Carroll et al., 2014), briefly, calculating comprehensive associations between SNPs and a total of 1645 clinical phenotypes derived from the International Classification of Disease, 9th CM (ICD-9) edition codes from each site's EHR. The ICD-9 codes that are associated with each phenotype can be found at the PheWAS catalog located online at http://phewas.mc.vanderbilt.edu/. Cases for a given disease were defined as having at least two relevant ICD-9 codes on different days. The PheWAS method also defines control groups for each disease, which ensures that related diseases do not serve as controls for the current disease being analyzed. We performed association testing for all PheWAS phenotypes occurring in at least 20 individuals (effectively 20 “cases”).
We then compared our results to performing PheWAS for each FTO SNP adjusting for BMI. The BMI, obtained from each site's EHR, was estimated using the average BMI from individuals within our dataset. To minimize erroneous data, we only used BMI measurements between 15 and 70, a range that we have used in prior studies and has good precision (Denny et al., 2010b). Plotting was performed in R using the PheWAS and ggplot2 packages.
Meta-analysis was performed using the inverse-variance method (Hunter et al., 1982) for the nine shared SNPs. There were 1010 phenotypes that were in common across both datasets and met our minimum case criteria of at least 20 cases. This yields a Bonferroni corrected p-value of 4.95 × 10−5, (p = 0.05/1010 = 4.95 × 10−5), for a single SNP. We chose a single SNP, phenome-wide correction threshold since most of the SNPs in this analysis were in high LD with each other and thus do not represent truly independent tests. A false discovery rate (FDR) of q = 0.05, calculated with the Benjamin and Hochberg method using the R p.adjust method, yields a p-value of 2.48 × 10−4 (Benjamini and Hochberg, 1995). For our latter analyses, we considered a total of 54 SNPs. Since many phenotypes are correlated with each other and many of the SNPs are in LD, we also used simpleM (Gao et al., 2010) to estimate the number of unique tests performed, leading to an adjustment of p = 2.36 × 10−6. All analyses assumed a two-tailed distribution.
Results
Overview
A total of 24,198 individuals were used in our analyses (Table 1). Both the eMERGE and BioVU datasets were similar in median age, sex, and BMI. Our analysis of the association of the FTO SNPs with BMI (Table 2) showed that most SNPs in high linkage disequilibrium with rs8050136 (r2 > 0.80) have highly significant p-values (<3 × 10−9) and betas for BMI (Table 2). SNPs with lower correlations with rs8050136 have highly variable associations with BMI.
PheWAS of FTO rs8050136 Unadjusted for BMI
In the BioVU population, we observed that obesity (OR = 1.22, p = 1.4 × 10−6, 95% CI = 1.13–1.33) was significantly associated with rs8050136. Three obesity-related diseases also trended toward significance; T2D (OR = 1.14, p = 5.3 × 10−5, 95% CI = 1.07–1.21), obstructive sleep apnea (OSA; OR = 1.15, p = 4.6 × 10−3, 95% CI = 1.04–1.26) and chronic non-alcoholic liver disease (NAFLD; OR = 1.20, p = 6.06 × 10−3, 95% CI = 1.05–1.38) (Supplementary Table 1). We observed similar odds ratios for obesity and T2D in eMERGE (obesity: OR = 1.37, p = 1.88 × 10−4, 95% CI = 1.16–1.61; T2D: OR = 1.16, p = 0.014, 95% CI = 1.03–1.32). eMERGE results also demonstrated similar trends toward significant associations with OSA (OR = 1.14, p = 2.4 × 10−3, 95% CI = 1.05–1.24) (Supplementary Table 1). After meta-analysis, obesity (OR = 1.25, p = 2.1 × 10−9, 95% CI = 1.16–1.35), morbid obesity (OR = 1.34, p = 1.07 × 10−7, 95% CI = 1.20–1.48), and two obesity-related diseases, T2D (OR = 1.14, p = 2.3 × 10−6, 95% CI = 1.08–1.21) and OSA (OR = 1.15, p = 3.3 × 10−5, 95% CI = 1.07–1.22), were associated with rs8050136 (Table 3). Additionally, the associations with NAFLD and fibrocystic breast disease were also q < 0.05.
PheWAS of FTO rs8050136 Adjusted for BMI
After adjusting for average BMI, some of the associations were greatly attenuated, while others remained relatively unchanged (Table 3, Figure 1). The associations with obesity and OSA were largely attenuated by adjustment for BMI (obesity: OR = 1.11, p = 0.017, 95% CI = 1.02–1.22; morbid obesity: OR = 1.17, p = 0.016, 95% CI = 1.03–1.33; OSA: OR = 1.07, p = 0.040, 95% CI = 1.00–1.15). Chronic non-alcoholic liver disease demonstrated a possible association with rs8050136, which was only slightly attenuated between unadjusted and BMI-adjusted analyses (OR: 1.23 vs. 1.19; p: 2.2 × 10−4 vs. 1.9 × 10−3, 95% CI = 1.10–1.37 vs. 1.07–1.33). Additional phenotypes trended toward association with rs8050136, including fibrocystic breast disease (OR = 0.84, p = 4.8 × 10−4, 95% CI = 0.75–0.92), staphylococcal infections (OR = 1.16, p = 5.8 × 10−3, 95% CI = 1.04–1.29), streptococcal infections (OR = 1.21, p = 6.6 × 10−3, 95% CI = 1.05–1.39), osteomyelitis (OR = 1.21, p = 0.011, 95% CI = 1.04–1.41), and joint effusions (OR = 1.22, p = 6.9 × 10−3, 95% CI = 1.06–1.41). These were not notably changed by BMI adjustment. Due to the number of gram-positive bacterial infections, we tested post hoc for the association between the SNP and a composite phenotype of all gram-positive infections, which were defined as staphylococcal infections, streptococcal infections, pneumococcal pneumonia, and gram positive septicemia. When combining all gram-positive phenotypes, the result was similar to the individual phenotypes (n = 1095, OR = 1.15 95% confidence interval [95% CI] = 1.06–1.26).
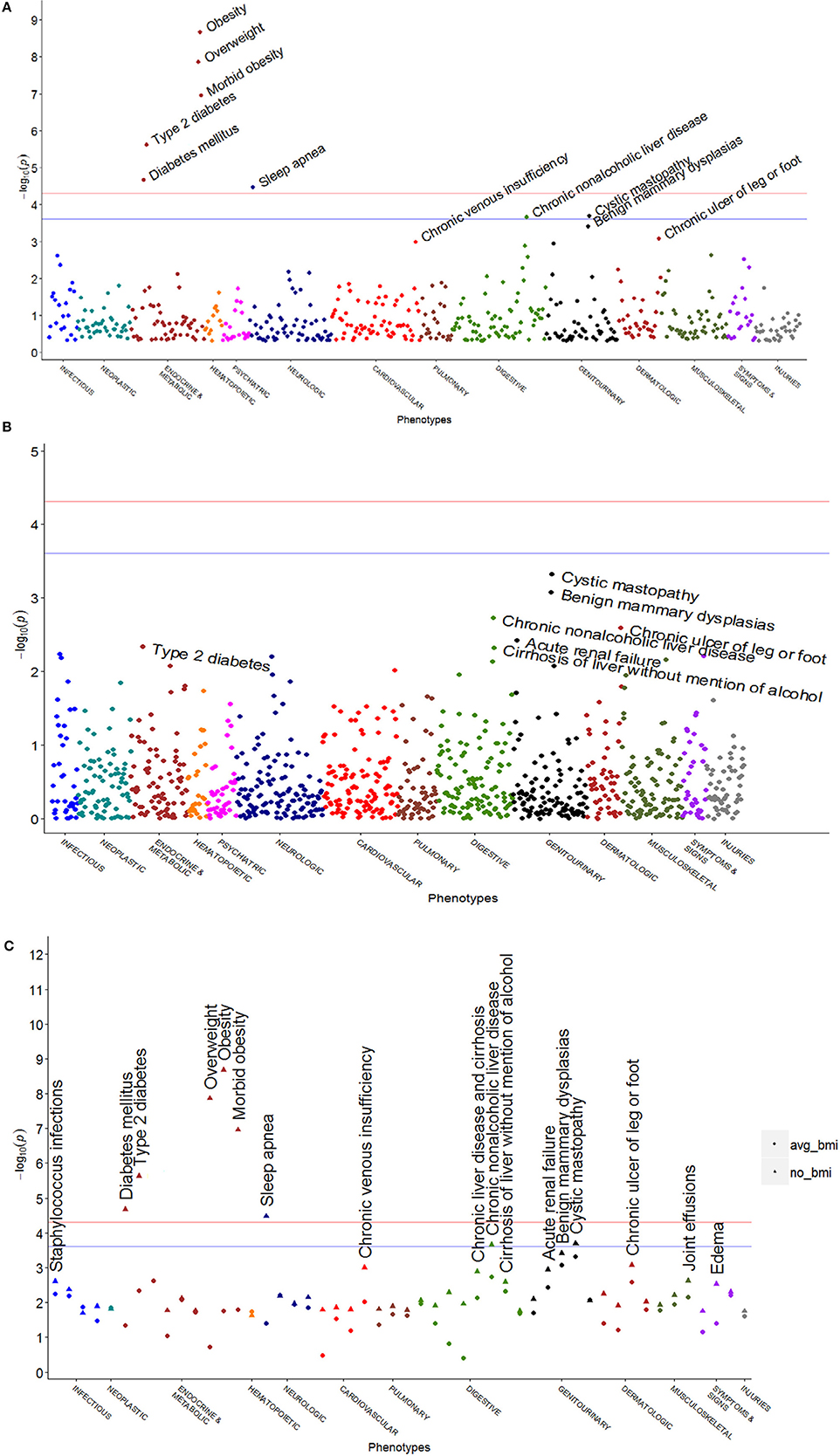
Figure 1. PheWAS plots for FTO rs8050136 with and without BMI adjustment. The pink horizontal line represents p = 4.95 × 10−5, which is the Bonferroni correction, and the blue horizontal line represents an FDR q = 0.05 (p = 2.48 × 10−4). (A) without BMI adjustment, (B) with BMI adjustment, and (C) most significant phenotypic associations before and after BMI adjustment (BMI-unadjusted values are shown as triangles and average BMI values are shown as dots) plotted on the same axis. The colors of points indicate the membership according to the phenotype classes identified on the X axis.
PheWAS of Other FTO SNPs Associated with Obesity
The results of SNPs in high LD with rs8050136 (r2 > 0.8) showed a similar pattern of phenotypes to rs8050136 (Figures 2A,B). Rs9941349, which is in LD with rs8050136 (r2 = 0.92) trended toward association with cystic mastopathy prior to BMI adjustment (p = 5.4 × 10−5, OR = 0.81, 95% CI = 0.73–0.90). SNPs with moderate to low correlation with rs8050136 had much different patterns of associations. Some of these SNPs demonstrated associations with obesity (e.g., rs9939609, rs9941349), and some did not (e.g., rs6499640, rs7199182; see Table 2). Of these SNPs, we only had eMERGE and BioVU data for rs6499640 (Figure 3A). All other SNPs were only available in the eMERGE data. “Non-inflammatory disorders of the cervix” was associated with some FTO SNPs (rs16952520: n = 21, p = 1.92 × 10−6, OR = 6.76, 95% CI = 3.08–14.84), and was unaffected by adjustment for BMI (OR = 6.66, 95% CI = 3.03–14.64, p = 2.36 × 10−6) (Figure 3B, MAF = 0.087). One less common genetic variant rs7199182 (Figure 3C, MAF = 0.064) was associated with chronic periodontitis (202 cases, OR = 14.58, 95% CI = 3.97–53.57, p = 5.40 × 10−5), and was not changed with adjustment for BMI with the signal being slightly stronger (OR = 14.66, 95% CI = 3.99–53.84, p = 5.20 × 10−5). Neither rs16952520 nor rs7199182 were associated with obesity or T2D. Detailed results for selected SNPs are shown in Supplementary Tables 3, 4.
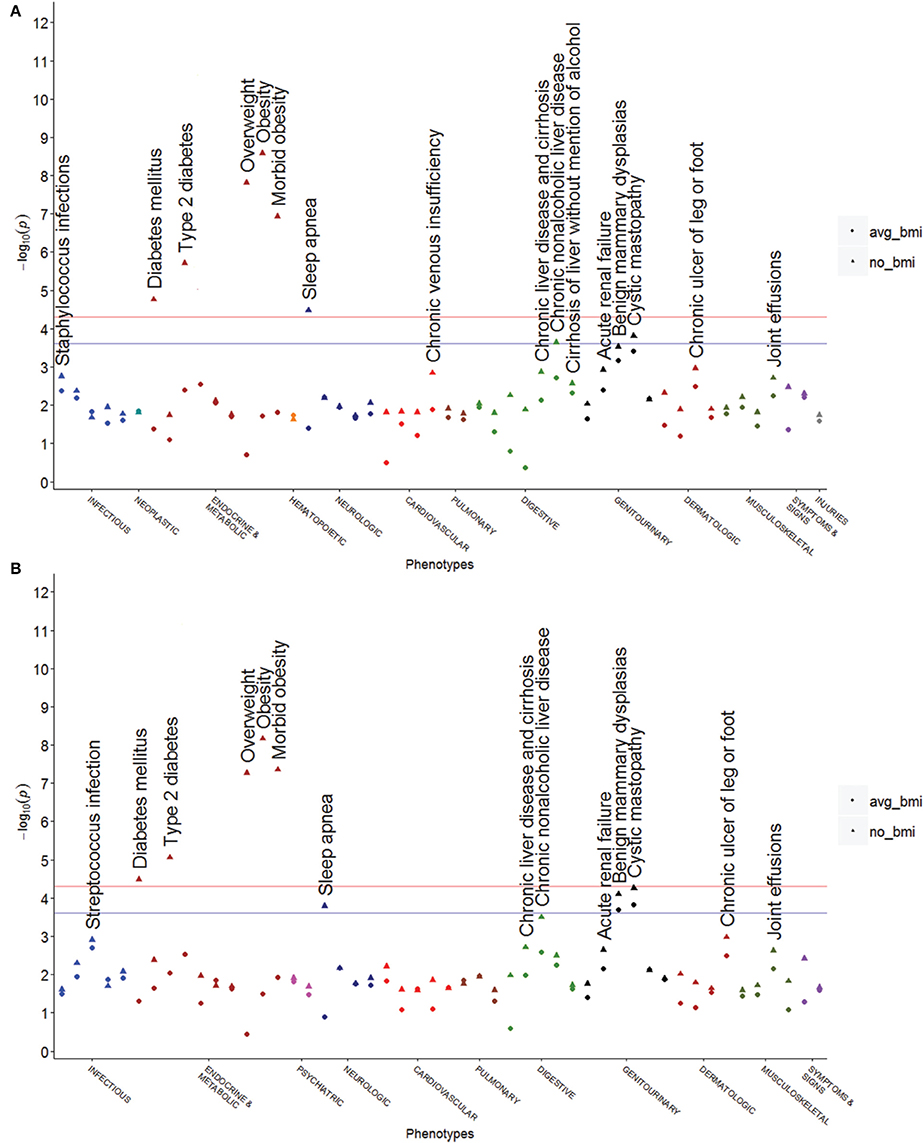
Figure 2. PheWAS plots for other obesity associated SNPs in high LD with rs8050136. These plots show unadjusted values and the average BMI adjusted values on the same axis. These SNPs are associated with BMI and have different correlations with rs8050136. These SNPs are present in both datasets and are presented as meta-analyses below. The pink horizontal line represents p = 4.95 × 10−5, which is the Bonferroni correction, and the blue horizontal line represents an FDR q = 0.05 (p = 2.48 × 10−4). (A) rs9939609 is reported widely in the literature and has a nearly identical pattern of associations to rs8050136 (r2 = 0.96). (B) rs9941349 also has a similar pattern to rs8050136 but cystic mastopathy is marginally more associated (p = 5.41 × 10−5, OR = 0.81 before BMI adjustment) than in rs8050136 (r2 = 0.88).
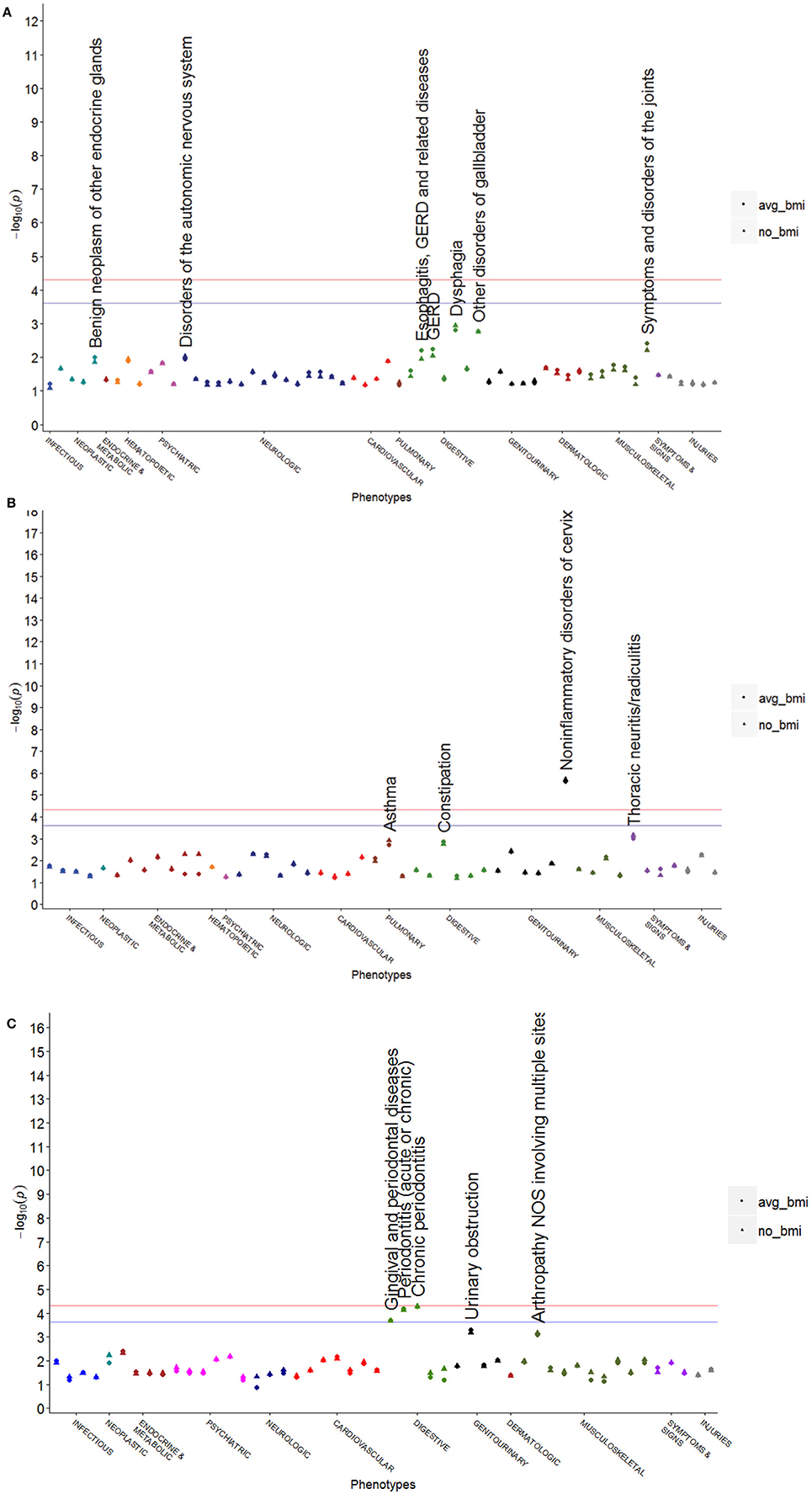
Figure 3. PheWAS plots for other obesity associated SNPs in low LD with rs8050136. These plots show values without adjustment for BMI (shown as triangles) and with adjustment for average BMI (shown as dots) plotted on the same axis. (A) rs6499640 is in both datasets with a lower LD with rs8050136 (r2 = 0.06) and has a different phenotype pattern than rs8050136 (B) rs16952520 is only present in the eMERGE population and has low LD with rs8050136 (r2 = 0.03) and while not strongly associated with obesity or diabetes does show significant association with non-inflammatory disorders of the cervix (OR = 6.76, p = 1.92 × 10−6), unaffected by adjustment for BMI (OR = 6.66, p = 2.36 × 10−6) (C) rs7199182 is only present in the eMERGE population and has a low LD with rs8050136 (r2 = 0.04) and is associated with chronic periodontitis before and after BMI adjustment (no adjustment: p = 5.40 × 10−5; BMI adjustment: p = 5.20 × 10−5).
Discussion
We studied the pleiotropic patterns for FTO variants with and without adjustment for BMI using phenome-wide associations in two large EHR cohorts. Consistent with other studies, we identified statistically significant associations with obesity, morbid obesity, and T2D among SNPs known to be associated with BMI; these associations were attenuated by adjustment for BMI. We also identified an association with OSA and trends toward association with NAFLD, fibrocystic breast disease, and infections, primarily gram-positive, with obesity-related SNPs. Some of these potential associations seem independent of BMI adjustment. Fibrocystic breast changes are a common benign breast disease and traditionally not thought related to obesity, including several epidemiological studies (Friedenreich et al., 2000; Baer et al., 2005; Li et al., 2005). Gram-positive infections could be explained in part by higher incidence of T2D in genetic variants of FTO. By analyzing other SNPs not significantly associated with BMI in our analysis, we also identified a few other potential associations with less common traits not associated with obesity (periodontitis, non-inflammatory diseases of the cervix); neither of these SNPs is in high LD with obesity-related SNPs. The most common ICD-9 code for “non-inflammatory disorders of the cervix” is cervical stenosis or stricture not related to congenital abnormalities or labor, which can result from surgical procedures, radiation, trauma, repeated vaginal infections, or menopause-related atrophy. These results, along with the recent association of FTO variants with IRX3 regulation (Smemo et al., 2014), suggest a broader role for FTO beyond that of regulating fat mass.
The question of whether the association of FTO variants and T2D is influenced by obesity or both obesity and FTO has been studied previously. A UK study of 9103 individuals demonstrated the loss of association after adjustment for BMI, as the T2D-FTO association prior to adjustment for BMI showed an OR = 1.15, p = 9 × 10−6 and after adjustment showed an OR = 1.03, p = 0.44 (Frayling et al., 2007). However, other studies suggest that T2D's association with FTO remains after adjustment for BMI (Hertel et al., 2011; Li et al., 2012). Li et al. studied 96,551 East and South Asians and demonstrated an association with T2D (OR = 1.15, p = 5.5 × 10−8) that was only partially attenuated after adjustment for BMI (OR = 1.10, p = 6.6 × 10−5) (Li et al., 2012). Similarly, Hertel et al. observed a significant T2D-FTO association even after adjustment for BMI in 41,504 Scandinavians, with the OR prior to adjustment of 1.13, p = 4.5 × 10−8 and after adjustment, OR = 1.09, p = 1.2 × 10−4 (Hertel et al., 2011). Finally a meta-analysis of 24,198 individuals demonstrated FTO rs9939609 (in high LD with rs8050136 with r2 > 0.8) was highly significantly associated with T2D before and after adjustment for BMI (before adjustment OR = 1.14, 95% CI = 1.12–1.16, p = 1.00 × 10−41; after adjustment OR = 1.07, 95% CI = 1.05–1.09, p = 6.42 × 10−41) (Xi et al., 2014). However, among individuals of European ancestry, the association was markedly attenuated after adjustment for BMI (before adjustment OR = 1.14, 95% CI = 1.11–1.16, p = 1.36 × 10−36; after adjustment OR = 1.06, 95% CI = 1.04–1.09, p = 3.51 × 10−8). In our study, the association between FTO and T2D did not decrease after adjustment for BMI as markedly as phenotypes such as obesity or sleep apnea. The effect sizes of these associations with T2D in our study closely parallels these larger studies (before BMI adjustment: OR = 1.14, 95% CI = 1.08–1.21, p = 2.11 × 10−6; after adjustment: OR = 1.09, 95% CI = 1.03–1.15, p = 2.62 × 10−3). Although these results show an association of FTO with T2D, a mediation analysis first demonstrating the associations of FTO SNPs with BMI and pre-diagnostic BMI with T2D, and subsequently modeling both FTO SNPs and pre-diagnostic BMI on T2D would help determine the direct and indirect effects of FTO on T2D.
Many of our findings, while having strong signals, were not significant after Bonferroni correction. The significant associations using Bonferroni correction included obesity, T2D, and OSA prior to BMI adjustment. After adjustment for average BMI, no associations retained statistical significance, but multiple phenotypes approached significance including T2D, NAFLD, and the protective effect on fibrocystic breast disease.
There is still much debate and uncertainty about both phenotypic association and protein functionality of FTO. Human FTO protein expression studies fail to replicate FTO's association with obesity observed in mouse models (Klöting et al., 2008; Wåhlén et al., 2008; Grunnet et al., 2009). Recent studies have shown that the SNPs in FTO that are associated with obesity regulate IRX3 expression, which is highly expressed in the brain (Smemo et al., 2014). Studies have described the association between FTO and obesity, while the association between T2D and FTO is debated (Hubacek et al., 2008; Li et al., 2008; Xi and Mi, 2009; Liu et al., 2010; Hotta et al., 2011). More studies with larger populations are required to assess the validity of many of these associations. The results of these associations show the power of the PheWAS method to efficiently detect known and novel pleiotropic associations of genetic variants.
BMI is an inexact surrogate for adiposity. Indeed, individuals with a high BMI do not necessarily have a high body fat percentage, thus BMI may not be the optimal definition of the phenotype (Müller et al., 2010). However, BMI has been shown to be as good a surrogate for obesity and diabetes as other central obesity indicators in multiple studies and meta-analyses (Vazquez et al., 2007; Nyamdorj et al., 2008, 2009).
Prior studies have suggested several other phenotypes that may be associated with FTO variants, including pancreatic cancer, Alzheimer's disease, attention deficit hyperactivity disorder, and alcoholism (Keller et al., 2011; Lurie et al., 2011; Sobczyk-Kopciol et al., 2011; arcOGEN Consortium et al., 2012; Corella et al., 2012; Reitz et al., 2012; Velders et al., 2012). We did not find evidence for these associations in our data set (p > 0.05) (Table 4), but in these cases we may be underpowered to find an association, with case sizes of 76 (attention deficit hyperactivity disorder), 183 (pancreatic cancer), 192 (Alzheimer's disease), and 267 (alcoholism) in our population. A trend toward association between FTO rs8044769 and osteoarthritis was observed in a previous GWAS study (rs8044769, r2 = 0.647 with rs8050136, p = 4 × 10−6) (arcOGEN Consortium et al., 2012). Our observation of a trend toward associations with joint effusions, which may be caused by osteoarthritis, lends some support to this inflammatory association.
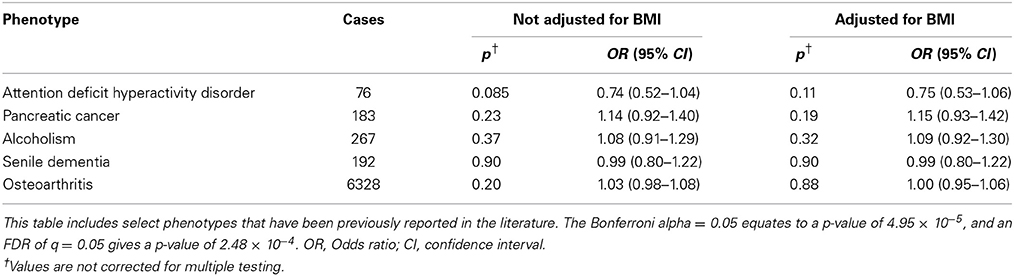
Table 4. Meta-analysis PheWAS results of rs8050136 for previously reported phenotypes associated with genetic variants.
Further analysis of multiple SNPs associated with obesity in FTO yielded some interesting results. First, the SNPs that are in high correlation with rs8050136 (r2 > 0.8) have very similar results to rs8050136, which is what we would expect. There are also SNPs that were associated with fibrocystic breast disease prior to adjustment for BMI. rs7199182, is in low LD with rs8050136 (r2 < 0.01), showed significant associations with chronic periodontitis before and after adjustment for BMI. Further analysis of this SNP and its association with chronic periodontitis will need to be investigated to validate this finding. One important consideration of this analysis is the small overlap of genotyped SNPs between the BioVU and eMERGE population. There are multiple SNPs that are present in both datasets and are highly correlated with rs8050136, but only rs6499640, which is in weak LD with rs8050136 (r2 = 0.06), was genotyped in both datasets. We are unable to impute the BioVU. The lack of overlapping SNPs limits our sample size to evaluate more of the potentially novel findings. Limitations caution interpretation of this study. Some of the case sizes were small and will require larger populations to validate. PheWAS analyses require robust EHR systems that can query patient cohorts efficiently. We used ICD-9 codes for the determination of phenotypes, codes which can be unreliable, inaccurate, and incomplete (Kern et al., 2006; Campbell et al., 2011); however, this could tend to result in missed, rather than false, associations. In addition to the caveats of ICD-9 codes, there are limitations of multiple hypothesis testing that come with comparisons of over 1000 phenotypes. Significance corrections like Bonferroni may be too strict; some of the near-significant pleiotropic associations may, in fact, represent genuine associations. Further testing with larger populations and more carefully defined phenotypes are needed to determine whether these associations are real.
Here we demonstrate the use of the PheWAS method to illustrate pleiotropic effects of variation in the gene FTO. When examining this gene with known pleiotropy, we were able to reproduce previously-discovered associations and identify potential new associations, some of which appear independent of obesity.
Conflict of Interest Statement
The authors declare that the research was conducted in the absence of any commercial or financial relationships that could be construed as a potential conflict of interest.
Acknowledgments
This work was supported by the eMERGE Network. The eMERGE Network was initiated and funded by NHGRI through the following grants: U01HG006389 (Essentia Institute of Rural Health); U01HG006382 (Geisinger Clinic); U01HG006375, UO1AG06781 (Group Health Cooperative and University of Washington); U01HG06379 (Mayo Clinic).); U01HG006380 (Mount Sinai School of Medicine); U01HG006388 (Northwestern University); U01HG006378 (Vanderbilt University); and U01HG006385 (Vanderbilt University serving as the Coordinating Center); and by National Center for Advancing Translational Sciences (NCATS) grant UL1TR000427 (Marshfield Clinic). Development of the PheWAS method is also supported by R01-LM010685 from the National Library of Medicine. BioVU received and continues to receive support through the NCATS grant 2 UL1 TR000445.
Supplementary Material
The Supplementary Material for this article can be found online at: http://www.frontiersin.org/journal/10.3389/fgene.2014.00250/abstract
References
1000 Genomes Project Consortium, Abecasis, G. R., Altshuler, D., Auton, A., Brooks, L. D., Durbin, R. M., et al. (2010). A map of human genome variation from population-scale sequencing. Nature 467, 1061–1073. doi: 10.1038/nature09534
arcOGEN Consortium, arcOGEN Collaborators, Zeggini, E., Panoutsopoulou, K., Southam, L., Rayner, N. W., et al. (2012). Identification of new susceptibility loci for osteoarthritis (arcOGEN): a genome-wide association study. Lancet 380, 815–823. doi: 10.1016/S0140-6736(12)60681-3
Baer, H. J., Schnitt, S. J., Connolly, J. L., Byrne, C., Willett, W. C., Rosner, B., et al. (2005). Early life factors and incidence of proliferative benign breast disease. Cancer Epidemiol. Biomark. Prev. 14, 2889–2897. doi: 10.1158/1055-9965.EPI-05-0525
Benjamini, Y., and Hochberg, Y. (1995). Controlling the false discovery rate: a practical and powerful approach to multiple testing. J. R. Statist. Soc. B Methodol. 57, 289–300.
Campbell, P. G., Malone, J., Yadla, S., Chitale, R., Nasser, R., Maltenfort, M. G., et al. (2011). Comparison of ICD-9-based, retrospective, and prospective assessments of perioperative complications: assessment of accuracy in reporting. J. Neurosurg. Spine 14, 16–22. doi: 10.3171/2010.9.SPINE10151
Carroll, R. J., Bastarache, L., and Denny, J. C. (2014). R PheWAS: data analysis and plotting tools for phenome wide association studies in the R environment. Bioinformatics. doi: 10.1093/bioinformatics/btu197. [Epub ahead of print].
Corella, D., Ortega-Azorín, C., Sorlí, J. V., Covas, M. I., Carrasco, P., Salas-Salvadó, J., et al. (2012). Statistical and biological gene-lifestyle interactions of MC4R and FTO with diet and physical activity on obesity: new effects on alcohol consumption. PLoS ONE 7:e52344. doi: 10.1371/journal.pone.0052344
Crespi, B. J. (2010). The origins and evolution of genetic disease risk in modern humans. Ann. N.Y. Acad. Sci. 1206, 80–109. doi: 10.1111/j.1749-6632.2010.05707.x
Crosslin, D. R., McDavid, A., Weston, N., Zheng, X., Hart, E., de Andrade, M., et al. (2013). Genetic variation associated with circulating monocyte count in the eMERGE Network. Hum. Mol. Genet. 22, 2119–2127. doi: 10.1093/hmg/ddt010
Denny, J. C., Bastarache, L., Ritchie, M. D., Carroll, R. J., Zink, R., Mosley, J. D., et al. (2013). Systematic comparison of phenome-wide association study of electronic medical record data and genome-wide association study data. Nat. Biotechnol. 31, 1102–1110. doi: 10.1038/nbt.2749
Denny, J. C., Crawford, D. C., Ritchie, M. D., Bielinski, S. J., Basford, M. A., Bradford, Y., et al. (2011). Variants near FOXE1 are associated with hypothyroidism and other thyroid conditions: using electronic medical records for genome- and phenome-wide studies. Am. J. Hum. Genet. 89, 529–542. doi: 10.1016/j.ajhg.2011.09.008
Denny, J. C., Ritchie, M. D., Basford, M. A., Pulley, J. M., Bastarache, L., Brown-Gentry, K., et al. (2010a). PheWAS: demonstrating the feasibility of a phenome-wide scan to discover gene-disease associations. Bioinformatics 26, 1205–1210. doi: 10.1093/bioinformatics/btq126
Denny, J. C., Ritchie, M. D., Crawford, D. C., Schildcrout, J. S., Ramirez, A. H., Pulley, J. M., et al. (2010b). Identification of genomic predictors of atrioventricular conduction: using electronic medical records as a tool for genome science. Circulation 122, 2016–2021. doi: 10.1161/CIRCULATIONAHA.110.948828
Ding, K., de Andrade, M., Manolio, T. A., Crawford, D. C., Rasmussen-Torvik, L. J., Ritchie, M. D., et al. (2013). Genetic variants that confer resistance to malaria are associated with red blood cell traits in African-Americans: an electronic medical record-based genome-wide association study. G3 (Bethesda) 3, 1061–1068. doi: 10.1534/g3.113.006452
Frayling, T. M., Timpson, N. J., Weedon, M. N., Zeggini, E., Freathy, R. M., Lindgren, C. M., et al. (2007). A common variant in the FTO gene is associated with body mass index and predisposes to childhood and adult obesity. Science 316, 889–894. doi: 10.1126/science.1141634
Friedenreich, C., Bryant, H., Alexander, F., Hugh, J., Danyluk, J., and Page, D. (2000). Risk factors for benign proliferative breast disease. Int. J. Epidemiol. 29, 637–644. doi: 10.1093/ije/29.4.637
Gao, X., Becker, L. C., Becker, D. M., Starmer, J. D., and Province, M. A. (2010). Avoiding the high Bonferroni penalty in genome-wide association studies. Genet. Epidemiol. 34, 100–105. doi: 10.1002/gepi.20430
Gong, J., Schumacher, F., Lim, U., Hindorff, L. A., Haessler, J., Buyske, S., et al. (2013). Fine Mapping and Identification of BMI Loci in African Americans. Am. J. Hum. Genet. 93, 661–671. doi: 10.1016/j.ajhg.2013.08.012
Gottesman, O., Kuivaniemi, H., Tromp, G., Faucett, W. A., Li, R., Manolio, T. A., et al. (2013). The Electronic Medical Records and Genomics (eMERGE) Network: past, present, and future. Genet. Med. 15, 761–771. doi: 10.1038/gim.2013.72
Grunnet, L. G., Nilsson, E., Ling, C., Hansen, T., Pedersen, O., Groop, L., et al. (2009). Regulation and function of FTO mRNA expression in human skeletal muscle and subcutaneous adipose tissue. Diabetes 58, 2402–2408. doi: 10.2337/db09-0205
Hebbring, S. J., Schrodi, S. J., Ye, Z., Zhou, Z., Page, D., and Brilliant, M. H. (2013). A PheWAS approach in studying HLA-DRB1*1501. Genes Immun. 14, 187–191. doi: 10.1038/gene.2013.2
Hertel, J. K., Johansson, S., Sonestedt, E., Jonsson, A., Lie, R. T., Platou, C. G. P., et al. (2011). FTO, type 2 diabetes, and weight gain throughout adult life: a meta-analysis of 41,504 subjects from the Scandinavian HUNT, MDC, and MPP studies. Diabetes 60, 1637–1644. doi: 10.2337/db10-1340
Hotta, K., Kitamoto, T., Kitamoto, A., Mizusawa, S., Matsuo, T., Nakata, Y., et al. (2011). Association of variations in the FTO, SCG3 and MTMR9 genes with metabolic syndrome in a Japanese population. J. Hum. Genet. 56, 647–651. doi: 10.1038/jhg.2011.74
Hubacek, J. A., Bohuslavova, R., Kuthanova, L., Kubinova, R., Peasey, A., Pikhart, H., et al. (2008). The FTO gene and obesity in a large Eastern European population sample: the HAPIEE study. Obesity (Silver Spring) 16, 2764–2766. doi: 10.1038/oby.2008.421
Hunter, J. E., Schmidt, F. L., and Jackson, G. B. (1982). Meta-Analysis. Beverly Hills, CA: Sage Publ.
Jacobsson, J. A., Schiöth, H. B., and Fredriksson, R. (2012). The impact of intronic single nucleotide polymorphisms and ethnic diversity for studies on the obesity gene FTO. Obes. Rev. 13, 1096–1109. doi: 10.1111/j.1467-789X.2012.01025.x
Keller, L., Xu, W., Wang, H.-X., Winblad, B., Fratiglioni, L., and Graff, C. (2011). The obesity related gene, FTO, interacts with APOE, and is associated with Alzheimer's disease risk: a prospective cohort study. J. Alzheimers Dis. 23, 461–469. doi: 10.3233/JAD-2010-101068
Kern, E. F. O., Maney, M., Miller, D. R., Tseng, C.-L., Tiwari, A., Rajan, M., et al. (2006). Failure of ICD-9-CM codes to identify patients with comorbid chronic kidney disease in diabetes. Health Serv. Res. 41, 564–580. doi: 10.1111/j.1475-6773.2005.00482.x
Klöting, N., Schleinitz, D., Ruschke, K., Berndt, J., Fasshauer, M., Tönjes, A., et al. (2008). Inverse relationship between obesity and FTO gene expression in visceral adipose tissue in humans. Diabetologia 51, 641–647. doi: 10.1007/s00125-008-0928-9
Kullo, I. J., Ding, K., Shameer, K., McCarty, C. A., Jarvik, G. P., Denny, J. C., et al. (2011). Complement receptor 1 gene variants are associated with erythrocyte sedimentation rate. Am. J. Hum. Genet. 89, 131–138. doi: 10.1016/j.ajhg.2011.05.019
Li, H., Kilpeläinen, T. O., Liu, C., Zhu, J., Liu, Y., Hu, C., et al. (2012). Association of genetic variation in FTO with risk of obesity and type 2 diabetes with data from 96,551 East and South Asians. Diabetologia 55, 981–995. doi: 10.1007/s00125-011-2370-7
Li, H., Wu, Y., Loos, R. J. F., Hu, F. B., Liu, Y., Wang, J., et al. (2008). Variants in the fat mass- and obesity-associated (FTO) gene are not associated with obesity in a Chinese Han population. Diabetes 57, 264–268. doi: 10.2337/db07-1130
Li, S., Aufiero, B., Schiltz, R. L., and Walsh, M. J. (2000). Regulation of the homeodomain CCAAT displacement/cut protein function by histone acetyltransferases p300/CREB-binding protein (CBP)-associated factor and CBP. Proc. Natl. Acad. Sci. U.S.A. 97, 7166–7171. doi: 10.1073/pnas.130028697
Li, W., Ray, R. M., Lampe, J. W., Lin, M.-G., Gao, D. L., Wu, C., et al. (2005). Dietary and other risk factors in women having fibrocystic breast conditions with and without concurrent breast cancer: a nested case-control study in Shanghai, China. Int. J. Cancer 115, 981–993. doi: 10.1002/ijc.20964
Liu, Y., Liu, Z., Song, Y., Zhou, D., Zhang, D., Zhao, T., et al. (2010). Meta-analysis added power to identify variants in FTO associated with type 2 diabetes and obesity in the Asian population. Obesity (Silver Spring) 18, 1619–1624. doi: 10.1038/oby.2009.469
Lurie, G., Gaudet, M. M., Spurdle, A. B., Carney, M. E., Wilkens, L. R., Yang, H. P., et al. (2011). The obesity-associated polymorphisms FTO rs9939609 and MC4R rs17782313 and endometrial cancer risk in non-Hispanic white women. PLoS ONE 6:e16756. doi: 10.1371/journal.pone.0016756
Marchini, J., Howie, B., Myers, S., McVean, G., and Donnelly, P. (2007). A new multipoint method for genome-wide association studies by imputation of genotypes. Nat. Genet. 39, 906–913. doi: 10.1038/ng2088
McCarty, C. A., Chisholm, R. L., Chute, C. G., Kullo, I. J., Jarvik, G. P., Larson, E. B., et al. (2011). The eMERGE Network: a consortium of biorepositories linked to electronic medical records data for conducting genomic studies. BMC Med. Genomics 4:13. doi: 10.1186/1755-8794-4-13
Müller, M. J., Bosy-Westphal, A., and Krawczak, M. (2010). Genetic studies of common types of obesity: a critique of the current use of phenotypes. Obes. Rev. 11, 612–618. doi: 10.1111/j.1467-789X.2010.00734.x
Nyamdorj, R., Qiao, Q., Söderberg, S., Pitkäniemi, J., Zimmet, P., Shaw, J., et al. (2008). Comparison of body mass index with waist circumference, waist-to-hip ratio, and waist-to-stature ratio as a predictor of hypertension incidence in Mauritius. J. Hypertens. 26, 866–870. doi: 10.1097/HJH.0b013e3282f624b7
Nyamdorj, R., Qiao, Q., Söderberg, S., Pitkäniemi, J. M., Zimmet, P. Z., Shaw, J. E., et al. (2009). BMI compared with central obesity indicators as a predictor of diabetes incidence in Mauritius. Obesity (Silver Spring) 17, 342–348. doi: 10.1038/oby.2008.503
Park, S. L., Cheng, I., Pendergrass, S. A., Kucharska-Newton, A. M., Lim, U., Ambite, J. L., et al. (2013). Association of the FTO obesity risk variant rs8050136 with percentage of energy intake from fat in multiple racial/ethnic populations: the PAGE study. Am. J. Epidemiol. 178, 780–790. doi: 10.1093/aje/kwt028
Pathak, J., Kiefer, R. C., Bielinski, S. J., and Chute, C. G. (2012). Mining the human phenome using semantic web technologies: a case study for Type 2 Diabetes. AMIA Annu. Symp. Proc. 2012, 699–708.
Pathak, J., Wang, J., Kashyap, S., Basford, M., Li, R., Masys, D. R., et al. (2011). Mapping clinical phenotype data elements to standardized metadata repositories and controlled terminologies: the eMERGE Network experience. J. Am. Med. Inform. Assoc. 18, 376–386. doi: 10.1136/amiajnl-2010-000061
Pavlicev, M., and Wagner, G. P. (2012). A model of developmental evolution: selection, pleiotropy and compensation. Trends Ecol. Evol. 27, 316–322. doi: 10.1016/j.tree.2012.01.016
Pendergrass, S. A., Brown-Gentry, K., Dudek, S., Frase, A., Torstenson, E. S., Goodloe, R., et al. (2013). Phenome-wide association study (PheWAS) for detection of pleiotropy within the Population Architecture using Genomics and Epidemiology (PAGE) Network. PLoS Genet. 9:e1003087. doi: 10.1371/journal.pgen.1003087
Pendergrass, S. A., Brown-Gentry, K., Dudek, S. M., Torstenson, E. S., Ambite, J. L., Avery, C. L., et al. (2011). The use of phenome-wide association studies (PheWAS) for exploration of novel genotype-phenotype relationships and pleiotropy discovery. Genet. Epidemiol. 35, 410–422. doi: 10.1002/gepi.20589
Price, A. L., Patterson, N. J., Plenge, R. M., Weinblatt, M. E., Shadick, N. A., and Reich, D. (2006). Principal components analysis corrects for stratification in genome-wide association studies. Nat. Genet. 38, 904–909. doi: 10.1038/ng1847
Pritchard, J. K., Stephens, M., and Donnelly, P. (2000). Inference of population structure using multilocus genotype data. Genetics 155, 945–959.
Purcell, S., Neale, B., Todd-Brown, K., Thomas, L., Ferreira, M. A. R., Bender, D., et al. (2007). PLINK: a tool set for whole-genome association and population-based linkage analyses. Am. J. Hum. Genet. 81, 559–575. doi: 10.1086/519795
R Core Team. (2013). R: A Language and Environment for Statistical Computing. Vienna: R Foundation for Statistical Computing. Available online at: http://www.R-project.org/.
Rees, S. D., Islam, M., Hydrie, M. Z. I., Chaudhary, B., Bellary, S., Hashmi, S., et al. (2011). An FTO variant is associated with Type 2 diabetes in South Asian populations after accounting for body mass index and waist circumference. Diabet. Med. 28, 673–680. doi: 10.1111/j.1464-5491.2011.03257.x
Reitz, C., Tosto, G., Mayeux, R., Luchsinger, J. A., NIA-LOAD/NCRA Family Study Group, and Alzheimer's Disease Neuroimaging Initiative. (2012). Genetic variants in the Fat and Obesity Associated (FTO) gene and risk of Alzheimer's disease. PLoS ONE 7:e50354. doi: 10.1371/journal.pone.0050354
Ritchie, M. D., Denny, J. C., Zuvich, R. L., Crawford, D. C., Schildcrout, J. S., Bastarache, L., et al. (2013). Genome- and phenome-wide analyses of cardiac conduction identifies markers of arrhythmia risk. Circulation 127, 1377–1385. doi: 10.1161/CIRCULATIONAHA.112.000604
Shameer, K., Denny, J. C., Ding, K., Jouni, H., Crosslin, D. R., de Andrade, M., et al. (2013). A genome- and phenome-wide association study to identify genetic variants influencing platelet count and volume and their pleiotropic effects. Hum. Genet. 133, 95–109. doi: 10.1007/s00439-013-1355-7
Sivakumaran, S., Agakov, F., Theodoratou, E., Prendergast, J. G., Zgaga, L., Manolio, T., et al. (2011). Abundant pleiotropy in human complex diseases and traits. Am. J. Hum. Genet. 89, 607–618. doi: 10.1016/j.ajhg.2011.10.004
Smemo, S., Tena, J. J., Kim, K.-H., Gamazon, E. R., Sakabe, N. J., Gómez-Marín, C., et al. (2014). Obesity-associated variants within FTO form long-range functional connections with IRX3. Nature 507, 371–375. doi: 10.1038/nature13138
Sobczyk-Kopciol, A., Broda, G., Wojnar, M., Kurjata, P., Jakubczyk, A., Klimkiewicz, A., et al. (2011). Inverse association of the obesity predisposing FTO rs9939609 genotype with alcohol consumption and risk for alcohol dependence. Addiction 106, 739–748. doi: 10.1111/j.1360-0443.2010.03248.x
Solovieff, N., Cotsapas, C., Lee, P. H., Purcell, S. M., and Smoller, J. W. (2013). Pleiotropy in complex traits: challenges and strategies. Nat. Rev. Genet. 14, 483–495. doi: 10.1038/nrg3461
Stranger, B. E., Stahl, E. A., and Raj, T. (2011). Progress and promise of genome-wide association studies for human complex trait genetics. Genetics 187, 367–383. doi: 10.1534/genetics.110.120907
Stratigopoulos, G., Padilla, S. L., LeDuc, C. A., Watson, E., Hattersley, A. T., McCarthy, M. I., et al. (2008). Regulation of Fto/Ftm gene expression in mice and humans. Am. J. Physiol. Regul. Integr. Comp. Physiol. 294, R1185–R1196. doi: 10.1152/ajpregu.00839.2007
Turner, S., Armstrong, L. L., Bradford, Y., Carlson, C. S., Crawford, D. C., Crenshaw, A. T., et al. (2011). Quality control procedures for genome-wide association studies. Curr. Protoc. Hum. Genet. Chapter 1, Unit 1.19. doi: 10.1002/0471142905.hg0119s68
Vazquez, G., Duval, S., Jacobs, D. R. Jr., and Silventoinen, K. (2007). Comparison of body mass index, waist circumference, and waist/hip ratio in predicting incident diabetes: a meta-analysis. Epidemiol. Rev. 29, 115–128. doi: 10.1093/epirev/mxm008
Velders, F. P., De Wit, J. E., Jansen, P. W., Jaddoe, V. W. V., Hofman, A., Verhulst, F. C., et al. (2012). FTO at rs9939609, food responsiveness, emotional control and symptoms of ADHD in preschool children. PLoS ONE 7:e49131. doi: 10.1371/journal.pone.0049131
Wagner, G. P., and Zhang, J. (2011). The pleiotropic structure of the genotype-phenotype map: the evolvability of complex organisms. Nat. Rev. Genet. 12, 204–213. doi: 10.1038/nrg2949
Wåhlén, K., Sjölin, E., and Hoffstedt, J. (2008). The common rs9939609 gene variant of the fat mass- and obesity-associated gene FTO is related to fat cell lipolysis. J. Lipid Res. 49, 607–611. doi: 10.1194/jlr.M700448-JLR200
Welter, D., MacArthur, J., Morales, J., Burdett, T., Hall, P., Junkins, H., et al. (2014). The NHGRI GWAS Catalog, a curated resource of SNP-trait associations. Nucleic Acids Res. 42, D1001–D1006. doi: 10.1093/nar/gkt1229
Xi, B., and Mi, J. (2009). FTO polymorphisms are associated with obesity but not with diabetes in East Asian populations: a meta-analysis. Biomed. Environ. Sci. 22, 449–457. doi: 10.1016/S0895-3988(10)60001-3
Keywords: PheWAS, genetic association, pleiotropy, Exome chip, FTO, BMI
Citation: Cronin RM, Field JR, Bradford Y, Shaffer CM, Carroll RJ, Mosley JD, Bastarache L, Edwards TL, Hebbring SJ, Lin S, Hindorff LA, Crane PK, Pendergrass SA, Ritchie MD, Crawford DC, Pathak J, Bielinski SJ, Carrell DS, Crosslin DR, Ledbetter DH, Carey DJ, Tromp G, Williams MS, Larson EB, Jarvik GP, Peissig PL, Brilliant MH, McCarty CA, Chute CG, Kullo IJ, Bottinger E, Chisholm R, Smith ME, Roden DM and Denny JC (2014) Phenome-wide association studies demonstrating pleiotropy of genetic variants within FTO with and without adjustment for body mass index. Front. Genet. 5:250. doi: 10.3389/fgene.2014.00250
Received: 15 May 2014; Accepted: 10 July 2014;
Published online: 05 August 2014.
Edited by:
Helena Kuivaniemi, Geisinger Health System, USACopyright © 2014 Cronin, Field, Bradford, Shaffer, Carroll, Mosley, Bastarache, Edwards, Hebbring, Lin, Hindorff, Crane, Pendergrass, Ritchie, Crawford, Pathak, Bielinski, Carrell, Crosslin, Ledbetter, Carey, Tromp, Williams, Larson, Jarvik, Peissig, Brilliant, McCarty, Chute, Kullo, Bottinger, Chisholm, Smith, Roden and Denny. This is an open-access article distributed under the terms of the Creative Commons Attribution License (CC BY). The use, distribution or reproduction in other forums is permitted, provided the original author(s) or licensor are credited and that the original publication in this journal is cited, in accordance with accepted academic practice. No use, distribution or reproduction is permitted which does not comply with these terms.
*Correspondence: Robert M. Cronin, Department of Biomedical Informatics, Vanderbilt University Medical Center, 220 Garland 440 EBL, Nashville, TN 37232, USA e-mail:cm9iZXJ0LmNyb25pbkB2YW5kZXJiaWx0LmVkdQ==;
Joshua C. Denny, Department of Biomedical Informatics and Department of Medicine, Vanderbilt University Medical Center, 2525 West End Avenue, Suite 600, Nashville, TN 37203-8820, USA e-mail:am9zaC5kZW5ueUB2YW5kZXJiaWx0LmVkdQ==