- 1Agricultural Research Council-Animal Production Institute, Pretoria, South Africa
- 2Department of Animal and Wildlife Sciences, University of Pretoria, Pretoria, South Africa
- 3Division of Animal Sciences, University of Missouri, Columbia, MO, USA
- 4Agricultural Research Council-Biotechnology Platform, Pretoria, South Africa
- 5Department of Animal, Wildlife and Grassland Sciences, University of Free State, Bloemfontein, South Africa
- 6Delta G, Miles City, MT, USA
Knowledge on the extent of linkage disequilibrium (LD) in livestock populations is essential to determine the minimum distance between markers required for effective coverage when conducting genome-wide association studies (GWAS). This study evaluated the extent of LD, persistence of allelic phase and effective population size (Ne) for four Sanga cattle breeds in South Africa including the Afrikaner (n = 44), Nguni (n = 54), Drakensberger (n = 47), and Bonsmara breeds (n = 46), using Angus (n = 31) and Holstein (n = 29) as reference populations. We found that moderate LD extends up to inter-marker distances of 40–60 kb in Angus (0.21) and Holstein (0.21) and up to 100 kb in Afrikaner (0.20). This suggests that genomic selection and association studies performed within these breeds using an average inter-marker r2≥ 0.20 would require about 30,000–50,000 SNPs. However, r2≥ 0.20 extended only up to 10–20 kb in the Nguni and Drakensberger and 20–40 kb in the Bonsmara indicating that 75,000 to 150,000 SNPs would be necessary for GWAS in these breeds. Correlation between alleles at contiguous loci indicated that phase was not strongly preserved between breeds. This suggests the need for breed-specific reference populations in which a much greater density of markers should be scored to identify breed specific haplotypes which may then be imputed into multi-breed commercial populations. Analysis of effective population size based on the extent of LD, revealed Ne = 95 (Nguni), Ne = 87 (Drakensberger), Ne = 77 (Bonsmara), and Ne = 41 (Afrikaner). Results of this study form the basis for implementation of genomic selection programs in the Sanga breeds of South Africa.
Background
Conventional selection programs based on quantitative principles have worked remarkably well and have allowed for significant genetic progress in South African beef cattle breeds over many decades (Scholtz, 2010). However, more effective selection and breeding strategies may result from the incorporation of molecular information (Goddard and Hayes, 2007). This may be achieved through marker assisted selection (MAS) or genomic selection (Meuwissen et al., 2001). Marker-assisted selection in livestock breeding programs relies on linkage between quantitative trait loci (QTL) and genetic markers, whereas genomic selection relies on the estimation of identity by descent using markers to establish identity by state. Markers can be directly causal for genetic variation, or may be in linkage equilibrium (LE) or linkage disequilibrium (LD) with causal variants (Dekkers, 2004). Causal markers have historically been very difficult to discover and it has been even more challenging to prove functional causality (Dekkers, 2010). Markers found to be in linkage with causal variants within families, but in LE in the population as a whole are not effective for use in MAS (Goddard and Hayes, 2007; Dekkers, 2010). In contrast, markers in LD with causal variants provide an alternative approach to the implementation of MAS in livestock populations and may be discovered in genome-wide association studies (GWAS) by searching for genomic regions that are associated with traits of economic importance (Goddard and Hayes, 2007). Discovery of these regions has been enabled by advances in genomic technologies, including sequencing of whole genomes of livestock species that include cattle, chickens, sheep, and goats (Hayes et al., 2009).
Successful application of LD information in different populations requires significant population-wide disequilibrium between markers and a large-effect QTL in order that marker alleles can predict the QTL alleles in individuals sampled from across the entire population (Hayes et al., 2009). McKay et al. (2007) assessed the extent of LD in cattle using 2670 single nucleotide polymorphism (SNP) markers genotyped in eight breeds from Bos taurus and Bos indicus cattle. Results from their study revealed that moderate LD (r2 = 0.20) extended to 40–60 kb in cattle, which indicated that about 50,000 SNPs would capture most of the LD information necessary for GWAS in European B. taurus breeds. In another study, Thévenon et al. (2007) evaluated the extent of LD in n = 363 B. indicus × B. taurus cattle from western Africa using 42 microsatellite markers on chromosomes 1, 4, and 7. Their results indicated that LD extended for shorter distances than had previously been observed in cattle from developed countries. This indicated that GWAS performed in these hybrids would require from75,000 to 300,000 SNP markers. Edea et al. (2014) evaluated the extent of LD in the indigenous Zebu and Sanga breeds of Ethiopia using the Illumina BovineSNP50 BeadChip (Matukumalli et al., 2009) and found that the extent of LD was lower within Zebu and Sanga breeds compared to European B. taurus. They attributed the reduced extent of LD detected in these breeds to the SNP ascertainment bias that was due to the discovery of SNPs used in the design of this assay in European B. taurus breeds.
Success of LD applications across populations relies on the preservation of allele phase relationships between markers and QTL across populations (Hayes et al., 2009). Using SNP data, de Roos et al. (2008) showed that as two populations became genetically more diverged, the allele phase relationships were less likely to be conserved. Therefore, it is essential that the extent of LD and persistence of allelic phase relationships be estimated for populations in which GWAS, MAS and genomic selection are to be implemented. This information will be essential for identification of the optimal array to apply with regard to cost (number of genotyped individuals) and marker density (to achieve a satisfactory inter-marker LD) (Goddard and Hayes, 2007).
Extent of genome-wide LD has not yet been assessed in South African cattle breeds. The South African Sanga cattle breeds (Afrikaner, Nguni, Drakensberger, and Bonsmara) were previously described by Makina et al. (2014) and were shown to be genetically distinct from Angus and Holstein (European B. taurus). Sanga cattle are thought to be a hybrid of B. taurus and B. indicus origin which likely occurred as B. taurus Egyptian Longhorn cattle migrated south from Egypt and the Sudan, and B. indicus Lateral Horned Zebu cattle migrated from Arabia and India (Scholtz et al., 2011). Afrikaner, Nguni, and Drakensberger are indigenous breeds while the Bonsmara is a composite breed developed during the 1960s through the cross breeding of Afrikaner, Hereford, and Shorthorn (Bonsma, 1980). The four breeds included in this study were shown by Makina et al. (2014) to share some level of coancestry, but the breeds were clearly distinguishable from each other. Previous studies have shown that LD is population specific and can be heterogeneous between populations depending on the demographic histories of the populations (McKay et al., 2007; Thévenon et al., 2007; Edea et al., 2014). Thus, the aim of this study was to quantify the extent of genome-wide LD, determine the persistence of allele phase relationships and estimate effective population size for the South African Afrikaner, Nguni, Drakensberger, and Bonsmara Sanga cattle breeds using Angus and Holstein as reference groups since these breeds have previously been extensively characterized in other countries.
Materials and Methods
Animal Samples and Quality Control
The genotypic data for this study originated from the work by Makina et al. (2014) that included Afrikaner (n = 44); Nguni (n = 54), Drakensberger (n = 47), Bonsmara (n = 44), Angus (n = 31), and Holstein (n = 29). Two hundred and forty nine samples were selected based on pedigree data to select against full- and half-sib animals in order to maximize the genetic diversity within each sampled population. Blood and semen were used as sources of genomic DNA with approval of the Animal Ethics Committee of the University of Pretoria (E097-12). Genotypic data were generated using the Illumina BovineSNP50 BeadChip v2 (Matukumalli et al., 2009).
Quality control was performed within breed and included removing any SNPs with less than a 95% call rate, SNPs with minor allele frequency (MAF) less than 5% and SNPs which deviated significantly from Hardy-Weinberg Equilibrium (P < 0.001; Purcell et al., 2007). Only SNPs that were uniquely mapped to autosomes were included in the analyses. Samples with more than 10% missing genotypes and one sample of any pair with an identity by descent score of more than 0.25 were also excluded (Purcell et al., 2007). SNPs remaining after quality control are reported for each breed in Supplementary Material 1 and these were used for analyses. SNP Variation Suite (SVS) version 8.1 (SVS 8.1; Golden Helix Inc., Bozeman, Montana; Golden Helix Inc., 2012) was used to calculate the chromosome length (Mb) spanned by the retained markers, number of SNPs per chromosome and the average gap between SNPs in this study (Supplementary Material 2). Details of the physical positions of the markers used in this study were obtained from Illumina map files: (http://support.illumina.com/array/array_kits/bovinesnp50_v2_dna_analysis_kit/downloads.html).
Minor Allele Frequency
The MAF for each breed was calculated using PLINK v1.07 under default settings (Purcell et al., 2007) for all autosomal SNPs. The distribution of allele frequencies was analyzed using R software (R Development Core Team, 2013) and proportions of SNPs in different frequency categories were plotted.
Pattern of Haplotype Blocks
Haplotype blocks are defined as the particular combinations of alleles observed in a particular population for a genomic region in which less than 5% of comparisons among informative SNP pairs show strong evidence of historical recombination (Gabriel et al., 2002). Haplotype block based methods provide greater information content than single SNP methods in GWAS (Qanbari et al., 2010). In this study, the inference of haplotype was carried out directly from unphased genotypic data for each chromosome within each breed using the E-M algorithm approach implemented in PLINK v1.07 (Purcell et al., 2007). The direct phasing of genotypes across breeds was avoided, as this would likely harmonize the haplotypes into a set that would actually be less diverse than is correct due to the assumption that they all belong to a single panmictic population. PLINK v1.07 (Purcell et al., 2007) was used to estimate haplotype block structure, using default setting in haploview (http://www.broad.mit.edu/mpg/haploview/).
Linkage Disequilibrium Analysis
To predict the extent of LD the r-squared statistic was chosen over the D' estimator. This choice allows for comparisons of results from this study with previous studies in cattle and other domestic animals. The D' estimate also tends to be inflated with small sample sizes or at low haplotype frequencies (McRae et al., 2002). The r-squared estimator is accepted as a measure of LD in the context of QTL mapping, due to applicability for estimation of the number of loci required for association studies (Pritchard and Przeworski, 2001). The r2 between syntenic pairwise SNPs separated by up to a distance of 1 Mb was used to estimate the extent of LD in PLINK v1.07 (Purcell et al., 2007) based on haplotype frequencies estimated via the E-M algorithm using the following parameters: –ld-snp-list mysnplist –ld-window-kb 10000 –ld-window 99999 –ld-window r2 0. LD was estimated genome-wide separately for each breed. The decay of LD was then analyzed for the following different genetic distance classes between SNP pairs [0, < 10 (10); 10, < 20 (20); 20, < 40 (40); 40, < 60 (60); 60, < 100 (100); 100, < 200 (200); 200, < 500 (500); 500, < 1000 (1000) kb]. To test the effects of MAF on the estimates of LD, the LD was calculated as previously described with different minimum MAF thresholds (MAF ≥ 0.05, MAF ≥ 0.1, MAF ≥ 0.2). R software (R Development Core Team, 2013) was used to visualize the effects of MAF on the genome-wide LD levels by plotting LD levels for the different breeds.
Persistence of Allele Phase and Time Since Breed Divergence
Persistence of allele phase between the breeds can be used to determine the history and the relationships among breeds within a species. It can also be used to determine the reliability of MAS when performed across populations (Goddard et al., 2006). In this study, the persistence of phase between alleles on the same chromosome was estimated using r, (LD estimator is r2) for marker pairs that were in common (n = 21.869) amongst the six breeds using PLINK software version 1.07 (Purcell et al., 2007), with the following parameters: –r –ld-window-kb 1000 –ld-window 9999. The correlations among r-values between breeds were estimated for 10 kb intervals (from 0 to 1000 kb) using the PROC CORR procedure in SAS (SAS Institute Inc., Cary, USA).
The phase correlations were used to estimate the number of generations since the breeds diverged from a common ancestral population (Sved et al., 2008). When two populations have originated from a common ancestral population, their phase correlation can be expressed as , where is a measure of LD in the common ancestral population, c is the recombination distance between markers, and T is time since breed divergence in generations (de Roos et al., 2008). The expected correlation of r between two breeds can be expressed as e−2cT (de Roos et al., 2008). The recombination distance (c) is almost zero for markers separated by only 10 kb, thus the correlation of phase at these short distances (10 kb) serves as an approximation for the extent of LD in the common ancestral population (Badke et al., 2012). To estimate divergence time for South African cattle breeds, SNPs with pairwise distances between 0 and 500 kb in 10 kb intervals were used. A linear regression of the natural logarithm of the estimated correlation of r on average pairwise distance c was calculated. The slope of this regression gives −2T, which is divided by −2 to estimate T (de Roos et al., 2008).
Effective Population Size
Effective population size (Ne) of a real population X can be defined as the size of a hypothetical ideal population that will result in the same amount of genetic drift as in the (actual) population (Wright, 1931). It is an important population parameter that helps to explain how populations have evolved (Falconer and Mackay, 1996) and it can be used to improve understanding and modeling of the genetic architecture underlying complex traits (Hayes et al., 2003). In this study, the relationship between variance in LD and Ne was used to infer ancestral and recent effective population sizes and this was regarded as NeLD (Hayes et al., 2003; Tenesa et al., 2007). NeLD of a real population X with an observed LD for a given interval length was defined as the size of hypothetic ideal population that in an equilibrium state would display the same pattern of LD for the same interval length as observed in real finite population (Simianer, 2012). The relationship between LD and effective population size in the presence of mutation was estimated using the SNeP tool (Barbato et al., 2015) using the result of Corbin et al. (2012):
Where NT is the effective population size T generations ago calculated as T = (Hayes et al., 2003), ct is the recombination rate for specific physical distance between markers calculated by SNeP tool (Barbato et al., 2015) using default values (1 Mb~1 cM), r2adj = r2− (βn)−1 where r2adj is the LD value adjusted for sample size (n is the sample size, β = 2 when gametic phase is known and β = 1 if unknown) and α is a correction for the occurrence of mutation.
Results
Marker Statistics
Afrikaner had the smallest percentage of polymorphic SNPs remaining after data filtering (n = 30.484), while the Drakensberger and Holstein had the largest (n = 40.789 and 40,734, respectively; Supplementary Material 1). A summary of the SNP distribution by breed and chromosome is presented in Supplementary Material 2. The SNPs spanned approximately 2.49 Gb of the bovine autosomal genome. The distributions of SNPs varied amongst chromosomes with BTA1, the largest bovine autosome, containing the largest number of variable SNPs (2040–2674) after filtering. The lowest numbers of variable SNPs was observed on Chromosomes 25, 27, and 28; and BTA 27 and 28 in Afrikaner cattle, and Nguni respectively. In Drakensberger, Bonsmara, Angus, and Holstein the fewest variable SNPs was on BTA27. Chromosomes 25, 27, and 28 are among the smaller chromosomes and therefore expected that these would have fewer variable SNPs. The greatest average physical distance between SNPs was observed for Afrikaner (81.65 kb) whilst the smallest average inter-marker interval was observed in Drakensberger (61.09 kb) and Holstein (61.08 kb).
Minor Allele Frequency
The average MAF over all chromosomes was 0.25 ± 0.13 (Afrikaner), 0.26 ± 0.13 (Nguni), 0.27 ± 0.13 (Drakensberger), 0.26 ± 0.13 (Bonsmara), 0.28 ± 0.12 (Angus), and 0.28 ± 0.13 (Holstein). The distribution of MAF for these breeds is presented in Figure 1. Afrikaner and Nguni cattle had the highest percentage of SNPs with MAF in the range 0.05–0.1, while Holstein and Angus had the lowest percentage in this range.
Haplotype Block Structure
The genome-wide haplotype block distribution across the studied breeds is presented in Table 1. A total of 320, 223, 285, 314, 446, and 452 haplotype blocks spanning between 0.65 and 1.67 Mb of the genome were detected in Afrikaner, Nguni, Drakensberger, Bonsmara, Angus, and Holstein, respectively. The mean number of SNPs contained within the haplotype blocks ranged from 3.30 (Nguni) to 3.73 (Afrikaner). The distribution of haplotype block size per breed is shown in Figure 2. Afrikaner cattle had the longest haplotype blocks and Drakensberger had the shortest.
Linkage Disequilibrium Decay
Genome-wide average LD (r2) decreased with increasing genomic distance for all breeds (Figure 3). Large differences between the Afrikaner cattle and other local breeds (Nguni, Drakensberger, and Bonsmara) were observed in this study. Nguni, Drakensberger, and Bonsmara had lower LD, which rapidly decayed with increasing distance between markers compared to the Afrikaner. As expected the Angus and Holstein cattle had higher LD compared to local breeds with the exception of the Afrikaner.
MAF and LD
Three different minimum MAF thresholds (0.05, 0.1, and 0.2) were used to study the effect of MAF on the extent of LD. Figure 4 shows that MAF had an effect on the average value of r2, especially over short inter-marker distances (< 100 kb). The average r2 increased with MAF, for example, for markers with MAF ≥ 0.05 and separated by 0–10 kb, the average r2 was 0.47, 0.37, 0.37, 0.37, 0.46, and 0.45. For markers with MAF ≥ 0.1, the r2 estimates increased to 0.55, 0.42, 0.46, 0.42, 0.50, and 0.50; these estimates further increased to 0.61, 0.45, 0.49, 0.52, 0.60, and 0.57 with MAF ≥ 0.2, for Afrikaner, Nguni, Drakensberger, Bonsmara, Angus, and Holstein, respectively.
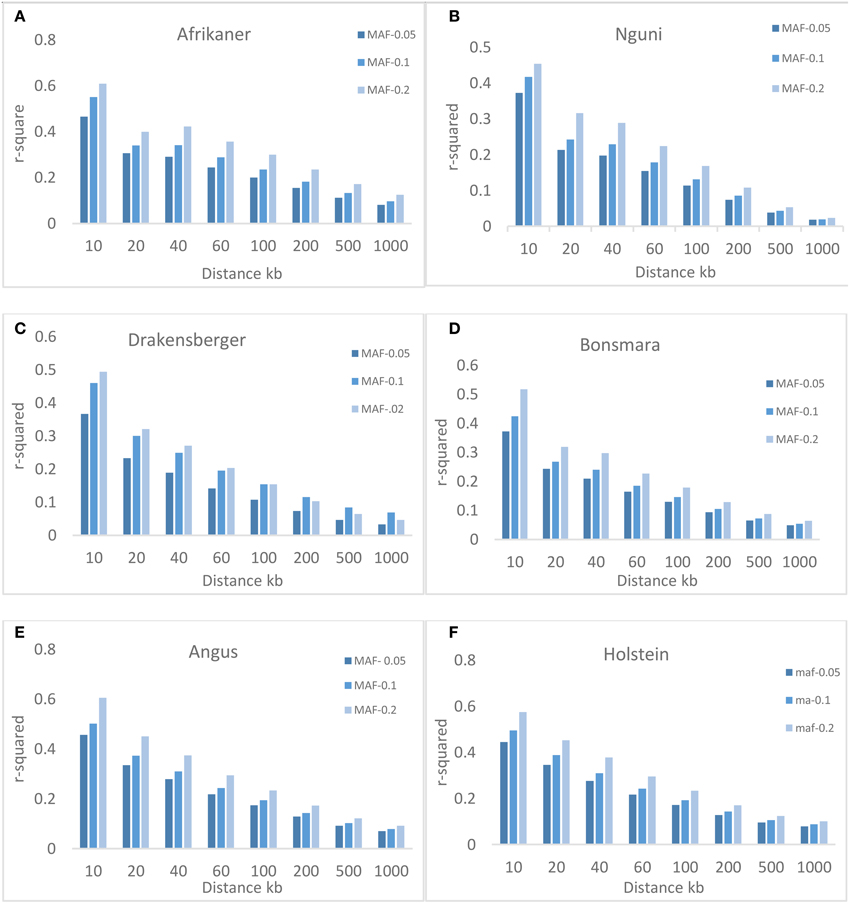
Figure 4. Average r2 estimates at different genetic distances for three different minor allele frequency (MAF) thresholds. Average LD estimates are pooled over all autosomal chromosomes: (A) Afrikaner, (B) Nguni, (C) Drakensberger, (D) Bonsmara, (E) Angus and, (F) Holstein.
Persistence of Phase and Time Since Breed Divergence
The statistic r was used to examine the extent of persistence of marker allele phase in the studied breeds. For example, if r was positive in one population and negative in another population, then different haplotypes are prevalent in the two populations and phase is not preserved between them. However, if r is positive in both populations, then similar haplotypes are common in both populations and phase tends to be preserved. A correlation of 1 between r values indicates that the marker phase persists across populations while a correlation of zero indicates that the marker phase in population one cannot predict phase in the other population (de Roos et al., 2008). In this study, the correlation between alleles at contiguous loci indicated that phase was not strongly preserved between breeds and decreased with increasing genetic distance between the breeds (Figure 5). The largest correlation was observed between the Nguni and Bonsmara pair (0.50 at 10 kb) whilst the lowest correlation was observed between Afrikaner and Drakensberger (0.29 at 10 kb). As expected, the correlations between r statistics for all B. taurus-African breed pairs were lower than those found among the South African breeds (Figure 5) with the lowest correlation observed between Afrikaner and Angus for marker pairs separated by less than 10 kb. This was supported by the estimated time of divergence of these breeds that suggested that South African cattle breeds diverged from each other approximately 131 (Nguni vs. Afrikaner) to 192 (Afrikaner vs. Drakensberger) generations ago (Supplementary Material 3). On the other hand B. taurus breeds diverged from the South African cattle breeds approximately 245 (Bonsmara vs. Angus) to 884 generations ago (Nguni and Holstein). Figure 6 shows the neighbor-joining tree based on time since breed divergence and also a neighbor-joining tree based on the Fst-values reported by Makina et al. (2014).
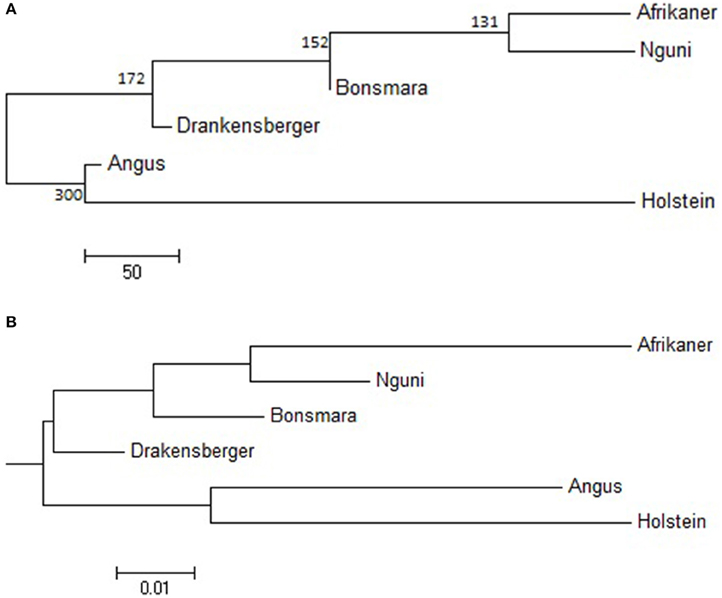
Figure 6. (A) Neighbor-joining tree showing time since breed divergence in generations, (B) Neighbor-joining tree showing the genetic relationship among breeds based on FST.
Effective Population Size
Past and recent effective population sizes were estimated from the average r2 for markers separated by various genomic distances. The extent of LD over greater recombinational distances indicated more recent Ne while that over shorter distances provided ancestral Ne (Hayes et al., 2003). Figure 7 presents historical Ne, i.e., from 1000 to 100 generations ago while Figure 8 shows more recent Ne. In general, Ne declined over time from larger to smaller Ne across the breeds. Nguni, Drakensberger, and Bonsmara had greater estimates of effective population size than did the Afrikaner, Angus, and Holstein at all generations.
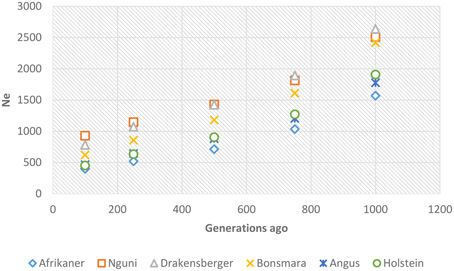
Figure 7. Genome-wide estimates of historical effective population size (Ne) over the previous 1000–100 generations based on estimates of linkage disequilibrium.
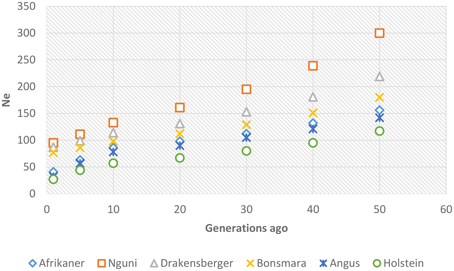
Figure 8. Genome-wide estimates of recent effective population size (Ne) from 1–50 generations in the past based on estimates of linkage disequilibrium.
Discussion
This study utilized the BovineSNP50 genotyping assay to estimate the extent of LD, Ne, and the persistence of phase among cattle breeds in South Africa. As previously reported by Qwabe et al. (2013), South African cattle breeds with the exception of Drakensberger cattle had fewer polymorphic loci and a higher percentage of SNPs with low MAF (MAF < 0.05) compared to the European taurine breeds (Angus and Holstein). The lower percentage of polymorphic loci among South African cattle breeds has previously been attributed to the ascertainment bias associated with the design of the BovineSNP50 BeadChip (Qwabe et al., 2013), as the SNP used in the design of this assay were detected in European B. taurus breeds, resulting in the MAF being lower in B. indicus breeds. SNPs with low allele frequencies tend to underestimate r2 estimates of LD between markers (Khatkar et al., 2008; Bohmanova et al., 2010; Qanbari et al., 2010; Espigolan et al., 2013). Three different MAF thresholds (0.05, 0.1, and 0.2) were used to study the effect of MAF on the extent of LD (Figure 4) and LD was found to increase as MAF increased across all breeds, especially for SNP pairs separated by short distances (< 100 kb). This phenomenon was previously observed by Khatkar et al. (2008) in Australian Holstein-Friesian cattle. They found that the mean r2 was 0.59 for SNP with MAF ≥ 0.05 but was higher (0.70) for SNP with MAF ≥ 0.2 separated by 1–10 kb. They attributed this to the fact that as the MAF threshold increases, there is an increase in the number of SNP pairs with similar allele frequencies and therefore an increase in r2 (Khatkar et al., 2008). On the other hand, Bohmanova et al. (2010) showed that the D' parameter was the highest for SNP with low MAF and thus overestimated LD among these loci while LD was underestimated for SNP with higher MAF. A study by Qanbari et al. (2010) reported that by minimizing the allele frequency difference between SNPs a more sensitive and useful metric for characterizing LD was achieved. In another study, using the BovineHD Chip on European taurine and Zebu cattle, O'Brien et al. (2014) observed no evidence of an influence of MAF on r2 estimates. They concluded that unbiased estimates of LD were obtained provided that MAF > 0.05 unless low density SNP coverage assays were used.
Small sample size (n ≤ 25) can also lead to biased estimates of LD (Khatkar et al., 2008). However, the results of Bohmanova et al. (2010) illustrated that sample size did not have a large effect on r2-values when at least 55 samples were used for the calculation. In this study, the results for Holstein and Angus cattle were comparable to previous studies employing larger sample sizes (>100; Khatkar et al., 2008; Bohmanova et al., 2010; Lu et al., 2012). However, the interpretation of these results should be performed with caution, as the establishment of the true LD values will require large sample sizes of unrelated individuals. Thus, future assessments of LD for South African Sanga cattle using the BovineHD Chip or whole genome sequence data and larger samples may address any biases that might have been introduced in this study due to sample size or ascertainment bias.
In general, the Angus and Holstein had more haplotype blocks than did the South African cattle breeds. Gautier et al. (2007) observed a similar trend for West African cattle breeds. This is likely due to the ascertainment bias that is associated with the design of the SNP assays. The number of haplotype blocks reported for Holstein (452) in this study was fewer than that reported by Qanbari et al. (2010) in German Holstein (712) and by Khatkar et al. (2008) in Australian Holstein-Friesian cattle (727). However, this was higher than the number reported by Gautier et al. (2007) in Holstein (94). The observed differences may be ascribed to the different marker densities used between these studies again due to ascertainment bias in assay design. The mean block size varied from 74 (Drakensberger) to 104 kb (Afrikaner) and an average of 3.30–3.73 markers per block was observed across the breeds. These results are comparable to those reported by Villa-Angulo et al. (2009) in cattle, who reported haplotype block ranges of between 30 and 75 kb and average markers of 3.8 in blocks. Haplotypic methods have been suggested to be more powerful for detecting genetic variation responsible for phenotypes (Villa-Angulo et al., 2009), because they provide more information to estimate whether two alleles are identical by descent; they reduce the number of association tests performed and hence the type I error rate, allow informed testing between clades of haplotype alleles by capturing evolutionary information and provide more power than single SNPs when multiple alleles exist at a causal locus (Gautier et al., 2007; Villa-Angulo et al., 2009; Qanbari et al., 2010).
The estimate of LD for SNP pairs separated by 40–60 kb was lower in the Nguni (0.15), Drakensberger (0.14), and Bonsmara (0.16) compared to the Afrikaner (0.24), Angus (0.21), and Holstein (0.21) which were similar to estimates reported for Angus and Holstein cattle located in other regions of the world (McKay et al., 2007; Khatkar et al., 2008; Lu et al., 2012). Low levels of LD have previously been reported by Edea et al. (2014) for Ethiopian cattle (0.14) and by Espigolan et al. (2013) in Nellore cattle (0.17) at a similar genetic distance. Low levels of LD among indicus, Zebu, and Sanga breeds have previously been attributed to the ascertainment bias of the SNPs located on the BovineSNP50 assay (Edea et al., 2014). In addition, the removal of many SNPs with very low MAF was previously found to be associated with biased estimates of LD (Khatkar et al., 2008; Espigolan et al., 2013). However, in this study, the Afrikaner cattle which had about 20% less variable SNPs and a higher proportion of low MAF SNPs compared to Drakensberger, Angus, and Holstein cattle, had higher levels of LD at all genetic distances. Consequently, the differences in the estimates of LD generated in this study can be ascribed to different evolutionary and molecular forces that have acted differently on these cattle breeds (Lee et al., 2011). Decker et al. (2012) showed that at least 80% of the US Angus genome was under strong selection, thus selection could be considered to be one of the causes of LD in livestock. Small effective population size is also usually implicated as the key source of extensive LD in livestock populations (Hayes et al., 2003). This is likely to be the situation for the Afrikaner cattle as in the recent past, this breed has significantly declined in popularity and in numbers due to high levels of inbreeding and reduced fertility (Coetzer and van Marle, 1972; Pienaar et al., 2014). The Nguni, Drakensberger, and Bonsmara reveal similar declines in LD, probably because these breeds share some ancestry (Makina et al., 2014) and are similar in effective population sizes and history.
Persistence of allele phase was used to infer the history and the genetic relationships among breeds based on their extent of divergence (de Roos et al., 2008). The correlation between alleles at contiguous loci indicated that phase was not strongly preserved between breeds. This suggests the need for breed-specific reference populations in which a much greater density of markers should be scored to identify breed specific haplotypes which may then be imputed into multi-breed commercial populations. The observed correlation of allele phases between the breeds in this study was much lower than previously reported by de Roos et al. (2008) for B. taurus breeds and by Gautier et al. (2007) between European cattle breeds at a short range (<10 kb).
Persistency of phase was used to estimate the time since breed divergence using the method proposed by de Roos et al. (2008). Results for Angus and Holstein (T = 300 generations ago) were in agreement with those reported by de Roos et al. (2008) for these breeds. In addition, our results suggested that the South African cattle breeds diverged approximately 131–192 generations ago, with Afrikaner and Nguni (131) having the lowest divergence time. This is in agreement with the recorded history of these breeds; Afrikaner and Nguni cattle are both Sanga breeds and are believed to have been derived from a common ancestor, a cross-bred between B. indicus and B. taurus cattle, which was assumed to originate from cattle that migrated south from Egypt and Sudan, and cattle that migrated from Arabia and India (Scholtz et al., 2011). Angus and Holstein are diverged from South African cattle breeds by approximately 245 and 900 generations, respectively, with the Angus having a considerably smaller divergence time than Holstein. This was in agreement with the previous estimates of divergence times between European B. taurus and African cattle (McKay et al., 2008).
LD structure can be used to provide insights into the evolutionary history of a population (Hill, 1981) and in this study the strength of LD at different genetic distances between loci was used to estimate ancestral effective population sizes. We found a decline in Ne throughout time in all breeds; between about 1000 generations until 100 generations ago, Ne declined across the breeds. This decrease in Ne may be associated with the post-domestication events of human migration with cattle that ultimately led to breed formation (Gautier et al., 2007). The most rapid decline in Ne occurred over the last 100 generations (approximately 500 years ago until present—assuming a generation interval of 5 years) in all breeds. This may suggest that a significant bottleneck occurred at breed formation and that population subdivision resulted in significantly reduced Ne (Daetwyler et al., 2010). Nguni, Drakensberger and Bonsmara had greater effective population sizes at all generations compared to Afrikaner probably due to presence of admixture within these breeds (Makina et al., 2014). de Roos et al. (2008) estimated effective population size in Australian Holstein-Friesian, Jersey and Angus cattle and found that the Ne for these breeds has decreased over the last 50 generations to approximately 100. Similarly, Villa-Angulo et al. (2009) estimated Ne for US Angus and found a rapid decline in Ne in Angus over the last 100 generations. In addition, Decker et al. (2012) estimated Ne in North American Angus using molecular inbreeding coefficients and found current Ne to be 94. On the other hand, effective population sizes obtained for Afrikaner, Nguni, and Bonsmara cattle in this study were in agreement with those reported based on pedigree data for the Afrikaner (Pienaar et al., 2014), Nguni (Matjuda, 2012), and Bonsmara (Santana et al., 2015). The low effective population sizes for the Angus and Holstein breeds compared to those for the Nguni, Bonsmara, and Drakensberger at more recent generations, could be due to intense selection and probably widespread use of artificial insemination and the use of relatively few elite sires after 1970 (Hayes et al., 2009). On the other hand, the low effective population size observed in the Afrikaner cattle could be associated with the bottleneck history of this breed (Coetzer and van Marle, 1972; Pienaar et al., 2014).
Implications for QTL Mapping and Genomic Selection
The extent and patterning of LD within the breeds represented in this study was used to assess the number of markers that would be required for GWAS of the six cattle breeds in South Africa. LD can be defined as the r2 between a marker and a QTL and this estimator is the proportion of QTL variance that can be observed at the marker (Hayes et al., 2009). Thus, the threshold for useful LD was assumed to be r2≥ 0.20 in this study as proposed by Hayes et al. (2003) for the application of genomic selection and GWAS. We found that for the Holstein and Angus cattle breeds, SNP spacing should be approximately 40–60 kb for genome-wide association scans. Assuming that any QTL will be at most in the middle of the interval, and therefore no more than 30 kb away from any marker, a minimum of 50,000 evenly spaced and informative markers would be sufficient to enable genome-wide scans in these breeds. This agrees with McKay et al. (2007) who suggested that 50,000 SNPs would capture most of the LD information necessary for GWAS in European B. taurus cattle populations. On the other hand, useful LD extended up to 100 kb in the Afrikaner cattle suggesting that approximately 30,000 uniformly distributed SNPs would be necessary in this breed in agreement with the findings of Villa-Angulo et al. (2009). However, for the Nguni and Drakensberger, the average r2 of 0.23 and 0.21 was achieved for inter-marker distances of 10–20 kb. This suggests that there should be an informative SNP every 20 kb to achieve the same GWAS power as in Afrikaner, Holstein and Angus, indicating that about 150,000 SNPs would be required in these breeds for genome-wide association and genomic selection studies, while 75,000 SNPs should be sufficient in Bonsmara. These results agree with Gautier et al. (2007) and Khatkar et al. (2008) who suggested that 75,000–300,000 informative SNPs would capture most of the LD information within the world's cattle breeds.
Persistence of allele phase between breeds in this study was used to determine the marker density to conduct multi-breed genomic selection. Correlation between alleles at contiguous loci indicated that phase was not strongly preserved between breeds. For example if a QTL was found in a chromosomal region in Nguni, SNPs linked to the QTL will only have a 50% chance of predicting the same QTL alleles in the Bonsmara breed given that region has an LD of at least 0.2 (Hayes et al., 2009). This suggests that there is considerable diversity amongst South African cattle breeds, and to find markers that consistently predict QTL alleles, i.e., with an r correlation >0.8 (de Roos et al., 2008), across all South African breeds of cattle, the marker to QTL interval should be less than 5 kb, which corresponds to the need to assay approximately 560,000 markers evenly distributed throughout the genome. This compares favorably with the studies of Goddard et al. (2006), de Roos et al. (2008), and Villa-Angulo et al. (2009) who indicated the value of having a large number of SNPs to cover the genome for genomic selection when analyzing data from multi-breed populations. In general, these results suggest that genomic based improvement programs for South African cattle breeds should consider using a much higher density SNP panel such as the Illumina BovineHD assay with 770 K SNPs and breed-specific reference populations to establish haplotypes or include purebred representatives from each breed in the reference population if a multi-breed GWAS is performed.
Conclusions
This study revealed significant differences in the extent of LD between South African breeds. Afrikaner cattle had the highest levels of LD compared to the other indigenous breeds. The higher LD suggests that Afrikaner cattle have experienced considerable bottlenecks restricting their effective population size in contrast to the other indigenous breeds. This result also indicates that this breed would require lower marker density panels relative to those required for the Nguni, Drakensberger, and Bonsmara cattle to associate genetic variation with economically important traits. Furthermore, our results suggest the necessity of much higher SNP density (e.g., 770 K) panels and breed-specific reference populations or adequate representation of each breed in the training population if a multi-breed training population is to be used for genomic improvement programs.
Author Contributions
SM collected the genetic materials, carried out the laboratory analyses, statistical analyses, and interpretation of the data and drafted the manuscript. SM, FM, and AM assisted with the acquisition of funding. All authors participated in the design and coordination of the study. JT and MLM assisted with the statistical analyses. JT, EV, FM, MLM, MDM, and AM revised the manuscript critically for important scientific content. All authors read and approved the final manuscript.
Conflict of Interest Statement
The authors declare that the research was conducted in the absence of any commercial or financial relationships that could be construed as a potential conflict of interest.
Acknowledgments
The authors would like to thank the breeders and research institutions for provision of animal blood samples. Provision of semen on Holstein bulls by Taurus Co-operative is also acknowledged. Ms Pienaar for sharing Afrikaner cattle hair samples. ARC-Biotechnology Platform is acknowledged for genotyping the samples. Financial support from the ARC and Red Meat Research and development Trust of South Africa is greatly appreciated. The authors would also like to thank the reviewers for constructive comments.
Supplementary Material
The Supplementary Material for this article can be found online at: http://journal.frontiersin.org/article/10.3389/fgene.2015.00337
References
Badke, Y. M., Bates, R. O., Ernst, C. W., Schwab, C., and Steibel, J. P. (2012). Estimation of linkage disequilibrium in four US pig breeds. BMC Genomics 13:24. doi: 10.1186/1471-2164-13-24
Barbato, M., Orozco-terWengel, P., Tapio, M., and Bruford, M. W. (2015). SNeP: a tool to estimate trends in recent effective population size trajectories using genome-wide SNP data. Front. Genet. 6:109. doi: 10.3389/fgene.2015.00109
Bohmanova, J., Sargolzaei, M., and Schenkel, F. S. (2010). Characteristics of linkage disequilibrium in North American Holsteins. BMC Genomics 11:421. doi: 10.1186/1471-2164-11-421
Bonsma, J. C. (1980). “Cross-breeding, breed creation and the genesis of the Bonsmara,” in Livestock Production - A Global Approach, ed J. C. Bonsma (Cape Town: Tafelberg Publishers Ltd), 126–136.
Coetzer, W. A., and van Marle, J. (1972). Die voorkoms van puberteit en daaropvolgende estrusperiode by vleisrasverse. S. Afr. J. Anim. Sci. 2, 17–18.
Corbin, L. J., Liu, A. Y. H., Bishop, S. C., and Woolliams, J. A. (2012). Estimation of historical effective population size using linkage disequilibria with marker data. J. Anim. Breed. Genet. 129, 257–270. doi: 10.1111/j.1439-0388.2012.01003.x
Daetwyler, H. D., Pong-Wong, R., Villanueva, B., and Woolliams, J. A. (2010). The impact of genetic architecture on genome-wide evaluation methods. Genetics 185, 1021–1031. doi: 10.1534/genetics.110.116855
Decker, J. E., Vasco, D. A., McKay, S. D., McClure, M. C., Rolf, M. M., Kim, J., et al. (2012). A novel analytical method, Birth Date Selection Mapping, detects response of the Angus (Bos taurus) genome to selection on complex traits. BMC Genomics 13:606. doi: 10.1186/1471-2164-13-606
Dekkers, J. C. (2004). Commercial application of marker- and gene-assisted selection in livestock: Strategies and lessons. J. Anim. Sci. 82(E. Suppl.), E313–E328.
Dekkers, J. C. (2010). Use of high-density marker genotyping for genetic improvement of livestock by genomic selection: perspectives in agriculture, veterinary science, nutrition and natural resources. CAB Rev. 5, 1–13. doi: 10.1079/PAVSNNR20105037
de Roos, A. P., Hayes, B. J., Spelman, R. J., and Goddard, M. E. (2008). Linkage disequilibrium and persistence of phase in Holstein-Friesian, Jersey and Angus cattle. Genetics 179, 1503–1512. doi: 10.1534/genetics.107.084301
Edea, Z., Dadi, H., Kim, S. W., Park, J. H., Shin, G. H., Dessie, T., et al. (2014). Linkage disequilibrium and genomic scan to detect selective loci in cattle populations adapted to different ecological conditions in Ethiopia. J. Anim. Breed. Genet. 13, 358–366. doi: 10.1111/jbg.12083
Espigolan, R., Baldi, F., Boligon, A. A., Souza, F. R. P., Gordo, D. G. M., Tonussi, R. L., et al. (2013). Study of whole genome linkage disequilibrium in Nellore cattle. BMC Genomics 14:305. doi: 10.1186/1471-2164-14-305
Falconer, D. S., and Mackay, T. F. C. (1996). Introduction to Quantitative Genetics, 4th Edn. Harlow, UK: Pearson Education Limited.
Gabriel, S. B., Schaffner, S. F., Nguyen, H., Moore, J. M., Roy, J., Blumenstiel, B., et al. (2002). The structure of haplotype blocks in the human genome. Science 296, 2225–2229. doi: 10.1126/science.1069424
Gautier, M., Faraut, T., Moazami-Goudarzi, K., Navratil, V., Foglio, M., Grohs, C., et al. (2007). Genetic and haplotypic structure in 14 European and African cattle breeds. Genetics 177, 1059–1070. doi: 10.1534/genetics.107.075804
Goddard, M. E., and Hayes, B. J. (2007). Genomic selection. J. Anim. Breed. Genet. 124, 323–330. doi: 10.1111/j.1439-0388.2007.00702.x
Goddard, M. E., Hayes, B., McPartlan, H., and Chamberlain, A. J. (2006). “Can the same genetic markers be used in multiple breeds?,” in 8th World Congress on Genetics Applied to Livestock Production, Vol. 8 (Belo Horizonte), 22–14.
Golden Helix Inc. (2012). SNP and Variation Suite Manual, Version 8.1. Available online at: http://www.goldenhelix.com
Hayes, B. J., Bowman, P. J., Chamberlain, A. J., and Goddard, M. E. (2009). Invited review: genomic selection in dairy cattle: progress and challenges. J. Dairy Sci. 92, 433–443. doi: 10.3168/jds.2008-1646
Hayes, B. J., Visscher, P. M., McPartlan, H. C., and Goddard, M. E. (2003). Novel multilocus measure of linkage disequilibrium to estimate past effective population size. Genome Res. 13, 635–643. doi: 10.1101/gr.387103
Hill, W. G. (1981). Estimation of effective population size from data on linkage disequilibrium. Genet. Res. 38, 209–216. doi: 10.1017/S0016672300020553
Khatkar, M. S., Nicholas, F. W., Collins, A. R., Zenger, K. R., Cavanagh, J. A., Barris, W., et al. (2008). Extent of genome-wide linkage disequilibrium in Australian Holstein-Friesian cattle based on a high- density SNP panel. BMC Genomics 9:187. doi: 10.1186/1471-2164-9-187
Lee, S. H., Cho, Y. M., Lim, D., Kim, H. C., Choi, B. H., Park, H. S., et al. (2011). Linkage disequilibrium and effective population size in Hanwoo Korean cattle. Asian Aust. J. Anim. Sci. 24, 1660–1665. doi: 10.5713/ajas.2011.11165
Lu, D., Sargolzaei, M., Kelly, M., Li, C., Vander Voort, G., Wang, Z., et al. (2012). Linkage disequilibrium in Angus, Charolais, and Crossbred beef cattle. Front. Genet. 3:152. doi: 10.3389/fgene.2012.00152
Makina, S. O., Muchadeyi, F. C., van Marle-Köster, E., MacNeil, M. D., and Maiwashe, A. (2014). Genetic diversity and population structure among six cattle breeds in South Africa using a whole genome SNP panel. Front. Genet. 5:333. doi: 10.3389/fgene.2014.00333
Matjuda, L. E. (2012). Development of Breeding Objectives for the Nguni Cattle Breed in South Africa. Ph.D. thesis, University of Limpopo.
Matukumalli, L. K., Lawley, C. T., Schnabel, R. D., Taylor, J. F., Allan, M. F., Heaton, M. P., et al. (2009). Development and characterization of a high density SNP genotyping assay for cattle. PLoS ONE 4:e5350. doi: 10.1371/journal.pone.0005350
McKay, S. D., Schnabel, R. D., Murdoch, B. M., Matukumalli, L. K., Aerts, J., Coppieters, W., et al. (2007). Whole genome linkage disequilibrium maps in cattle. BMC Genet. 8:74. doi: 10.1186/1471-2156-8-74
McKay, S. D., Schnabel, R. D., Murdoch, B. M., Matukumalli, L. K., Aerts, J., Coppieters, W., et al. (2008). An assessment of population structure in eight breeds of cattle using a whole genome SNP panel. BMC Genet. 9:37. doi: 10.1186/1471-2156-9-37
McRae, A. F., McEwan, J. C., Dodds, K. G., Wilson, T., Crawford, A. M., and Slate, J. (2002). Linkage disequilibrium in domestic sheep. Genetics 160, 1113–1122.
Meuwissen, T. H. E., Hayes, B. J., and Goddard, M. E. (2001). Prediction of total genetic value using genome-wide dense marker maps. Genetics 157, 1819–1829.
O'Brien, A. M. P., Mészáros, G., Utsunomiya, Y. T., Sonstegard, T. S., Garcia, J. F., Van Tassell, C. P., et al. (2014). Linkage disequilibrium levels in Bos indicus and Bos taurus cattle using medium and high density SNP chip data and different minor allele frequency distributions. Livest. Sci. 166, 121–132. doi: 10.1016/j.livsci.2014.05.007
Pienaar, L., Grobler, J. P., Neser, F. W. C., Scholtz, M. M., Swart, H., Ehlers, K., et al. (2014). Genetic diversity in selected stud and commercial herds of Afrikaner cattle breed. S. Afr. J. Anim. Sci. 44(Suppl. 5), S80–S84.
Pritchard, J. K., and Przeworski, M. (2001). Linkage disequilibrium in humans: models and data. Am. J. Hum. Genet. 69, 1–14. doi: 10.1086/321275
Purcell, S., Neale, B., Todd-Brown, K., Thomas, L., Ferreira, M. A., Bender, D., Maller, J., et al. (2007). PLINK: a tool set for whole-genome association and population-based linkage analyses. Am. J. Hum. Genet. 81, 559–575. doi: 10.1086/519795
Qanbari, S., Pimentel, E. C. G., Tetens, J., Thaller, G., Lichtner, P., Sharifi, A. R., et al. (2010). The pattern of linkage disequilibrium in German Holstein cattle. Anim. Genet. 41, 346–356. doi: 10.1111/j.1365-2052.2009.02011.x
Qwabe, S. O., van Marle-Köster, E., Maiwashe, E., and Muchadeyi, F. C. (2013). Evaluation of the BovineSNP50 genotyping array in four South African cattle populations. S. Afr. J. Anim. Sci. 43, 64–67.
R Development Core Team (2013). R Development Core Team: R: A Language and Environment for Statistical Computing. Vienna: R Foundation for Statistical Computing.
Santana, M. L. Jr, Oliveira, P. S., Eler, J. P., Gutiérrez, J. P., and Ferraz, J. B. (2015). Pedigree analysis and inbreeding depression on growth traits in Brazilian Marchigiana and Bonsmara breeds. J. Anim. Sci. 90, 99–108. doi: 10.2527/jas.2011-4079
Scholtz, M. M., Gertenbach, W., and Hallowell, G. (2011). The Nguni Breed of Cattle: Past, Present and Future. Bloemfontein: Thee Nguni Cattle Breeders' Society.
Simianer, H. (2012). “Genetic metrics, linkage disequilibrium and effective population size,” in Livestock Conservation Genomics: Data, Tools and Trends, October 1-7, 2012 (Pag Island: ESF Genomic Resources Summer School), 42–46.
Sved, J. A., McRae, A. F., and Visscher, P. M. (2008). Divergence between human populations estimated from linkage disequilibrium. Am. J. Hum. Genet. 83, 737–743. doi: 10.1016/j.ajhg.2008.10.019
Tenesa, A., Navarro, P., Hayes, B. J., Duffy, D. L., Clarke, G. M., Goddard, M. E., et al. (2007). Recent human effective population size estimated from linkage disequilibrium. Genome Res. 17, 520–526. doi: 10.1101/gr.6023607
Thévenon, S., Dayo, G. K., Sylla, S., Sidibe, I., Berthier, D., Legros, H., et al. (2007). The extent of linkage disequilibrium in a large cattle population of western Africa and its consequences for association studies. Anim. Genet. 38, 277–286. doi: 10.1111/j.1365-2052.2007.01601.x
Villa-Angulo, R., Matukumalli, L. K., Gill, C. A., Choi, J., Van Tassell, C. P., and Grefenstette, J. J. (2009). High-resolution haplotype block structure in the cattle genome. BMC Genet. 10:19. doi: 10.1186/1471-2156-10-19
Keywords: linkage disequilibrium, effective population size, persistence of phase, cattle breeds
Citation: Makina SO, Taylor JF, van Marle-Köster E, Muchadeyi FC, Makgahlela ML, MacNeil MD and Maiwashe A (2015) Extent of Linkage Disequilibrium and Effective Population Size in Four South African Sanga Cattle Breeds. Front. Genet. 6:337. doi: 10.3389/fgene.2015.00337
Received: 06 August 2015; Accepted: 09 November 2015;
Published: 01 December 2015.
Edited by:
Yuri Tani Utsunomiya, Universidade Estadual Paulista, BrazilReviewed by:
Ino Curik, University of Zagreb, CroatiaMarco Milanesi, São Paulo State University, Brazil
Copyright © 2015 Makina, Taylor, van Marle-Köster, Muchadeyi, Makgahlela, MacNeil and Maiwashe. This is an open-access article distributed under the terms of the Creative Commons Attribution License (CC BY). The use, distribution or reproduction in other forums is permitted, provided the original author(s) or licensor are credited and that the original publication in this journal is cited, in accordance with accepted academic practice. No use, distribution or reproduction is permitted which does not comply with these terms.
*Correspondence: Sithembile O. Makina, cXdhYmVzQGFyYy5hZ3JpYy56YQ==