- 1Department of Animal Sciences, University of Illinois at Urbana-Champaign, Urbana, IL, United States
- 2Department of Biosciences, COMSATS Institute of Information Technology, Islamabad, Pakistan
- 3Evolutionary Bioinformatics Laboratory, Department of Crop Sciences, University of Illinois at Urbana-Champaign, Urbana, IL, United States
Genome-wide global detection of genes involved in horizontal gene transfer (HGT) remains an active area of research in medical microbiology and evolutionary genomics. Utilizing the explicit evolutionary method of comparing topologies of a total of 154,805 orthologous gene trees against corresponding 16S rRNA “reference” trees, we previously detected a total of 660,894 candidate HGT events in 2,472 completely-sequenced prokaryotic genomes. Here, we report an HGT-index for each individual gene-reference tree pair reconciliation, representing the total number of detected HGT events on the gene tree divided by the total number of genomes (taxa) member of that tree. HGT-index is thus a simple measure indicating the sensitivity of prokaryotic genes to participate (or not participate) in HGT. Our preliminary list provides HGT-indices for a total of 69,365 genes (detected in >10 and <50% available prokaryotic genomes) that are involved in a wide range of biological processes such as metabolism, information, and bacterial response to environment. Identification of horizontally-derived genes is important to combat antibiotic resistance and is a step forward toward reconstructions of improved phylogenies describing the history of life. Our effort is thus expected to benefit ongoing research in the fields of clinical microbiology and evolutionary biology.
Introduction
Prokaryotic organisms often exchange genetic material directly with other organisms not necessarily related by vertical descent (Soucy et al., 2015). This phenomenon, commonly referred to as horizontal gene transfer (HGT), plays important roles in bacterial evolution by mediating, for example, transfer of antibiotic resistance and virulence, and genes involved in metabolism and stress response among related and non-related prokaryotic species (Ochman et al., 2000; Soucy et al., 2015). HGT therefore remains a major obstacle in slowing down or stopping the emergence of multidrug resistant bacterial pathogens and its global impact on prokaryote evolution and human health and economy continues to be investigated (von Wintersdorff et al., 2016).
Accurate detection of HGT through bioinformatics approaches however remains a technical and conceptual challenge (Ravenhall et al., 2015). To overcome the challenge of genome-wide global detection of HGT events in prokaryotes, we recently developed a sequence analysis pipeline and database to detect and store pre-calculated HGT events for a total of 2,472 completely sequenced prokaryotic genomes (156 Archaea and 2,316 Bacteria; Jeong et al., 2016). Unlike the existing HGT detection methods that rely on evaluating either genomic content bias across species (e.g., GC or codon usage; Becq et al., 2010) or methods that detect phylogenetically atypical signatures (Podell et al., 2008), we performed an explicit evolutionary study by reconstructing “approximate” maximum likelihood (ML) (Price et al., 2010) gene trees for a total of 154,805 putatively orthologous gene sets (putative since we test their HGT sensitivity in downstream analysis) detected in 2,472 genomes and reconciling the gene trees against corresponding reference species trees built from 16S ribosomal RNA (rRNA) gene alignments. Incongruence between gene and species tree pairs was evaluated for three likely scenarios, (i) gene duplication, (ii) HGT, and (iii) gene loss (known as the DTL reconciliation, Bansal et al., 2012), out of which the most parsimonious reconciliations supported by HGT were kept in the online HGTree database available from http://hgtree.snu.ac.kr.
HGTree is a rich resource of pre-calculated HGT events in prokaryotic genomes and offers a fast and reliably utility for users to explore HGT quality and quantity in their own genomic datasets. While, we already studied the fraction of genes in prokaryotic genomes that participate in HGT previously (see Figure 3 in Jeong et al., 2016), we did not study the HGT sensitivity of each individual prokaryotic gene. In this study, we therefore retrieved the pre-calculated HGT events along with corresponding gene and reference (i.e., 16S rRNA) tree pairs from HGTree and studied the sensitivity of each gene to participate or not participate in HGT. HGT sensitivity was given by an HGT-index simply indicating the total number of detected HGT events on a gene tree when reconciled with the corresponding 16S rRNA tree and divided by the size of the gene set in terms of number of member genomes. Following this simple statistic, and keeping in mind the associated limitations (see Section Discussion), we produced lists of frequently and non-frequently transferred genes in prokaryotic genomes (Datasets S1, S2). Further, we also mapped the identified genes to protein family (Punta et al., 2012) and functional annotations (Gene Ontology Consortium, 2015) to yield deeper insights into the nature of horizontal genetic exchange being mediated by microorganisms.
Results
We retrieved a total of 660,894 candidate HGT events detected on a total of 154,805 orthologous gene-reference tree pair reconciliations from the HGTree database (Jeong et al., 2016). Based on the total number of detected HGT events on each gene-reference pair reconciliation and the size of the gene set (i.e., total number of genomes or taxa), we calculated an HGT-index to indicate the sensitivity of each gene to participate or not participate in HGT (Datasets S1, S2). Mathematically, the HGT-index ranges from 0 (i.e., no HGT) to higher values, although <1 in all observed cases (Datasets S1, S2). In other words, no gene was “universally” exchanged horizontally among all genomes.
Out of the total 154,805 gene sets, we excluded 37,460 (24%) gene sets where either all pair-wise neighbor-joining (NJ) distances were close to zero, genomes include only one species, or harbored <10 genomes, from the downstream analysis. Of the remaining, 69,365/117,345 (59%) gene sets were sufficiently large (i.e., >10 genomes and <50% of available genomes, see Section Discussion) and yielded detectable gene-species tree conflict indicative of horizontal evolution (hereafter “HGT-genes,” Dataset S1). Notably, a total of 47,980 genes (41%) reported no detectable conflict during gene and species trees reconciliations and thus tentatively could be termed “HGT-free” genes (Dataset S2). Unsurprisingly, most of the HGT-free gene sets included very few genomes (<82 genomes) belonging to only a specific division of bacteria indicating they either evolved recently via vertical inheritance or were more likely to be exchanged among closely related members of the same species that do not result in topological tree conflict and were thus missed by our phylogeny-based method of HGT detection (Jeong et al., 2016).
Among the HGT-genes, the top-twenty genes with the highest HGT-indices included interesting proteins (Table 1). For example, the 30S ribosomal subunit protein S21 (Pfam Id: PF01165) had an HGT-index of 0.80 (153 HGT events in 191 taxa) indicating it was widely transferred. Interestingly, the 30S smaller ribosomal subunit of prokaryotic ribosomes houses the 16S rRNA and 22 ribonucleoproteins. The smaller subunit is important for maintaining the accuracy and continuity of protein translation and is an active inhibition site for routinely used antibiotics such as tetracyclines and aminoglycosides (Mehta and Champney, 2002). The antibiotics typically bind to the 30S subunit and block protein synthesis ultimately leading to bacterial death. Similarly, another smaller subunit protein (S18) (PF01084) had an HGT-index of above 0.6 for a total of 193 HGT events in 319 taxa (Dataset S1). Within the top-twenty list was also the protein family aminoglycoside/hydroxyurea antibiotic resistance kinase (PF04665) that blocks aminoglycoside antibiotics (e.g., streptomycin) by phosphorylation. Streptomycin also acts by blocking the 30S ribosomal subunit and is in fact one of the most essential medicines recognized by the World Health Organization (WHO Model List of Essential Medicines 18th list, 2013). In addition, we also identified phage integrases (PF00589) and transposases (PF02371 PF01548) protein families that had very high HGT-indices (>70%) highlighting the roles of mobile elements in spreading genes among prokaryotic species. However, in these two cases, the orthologous gene sets were very small in size and contained only 28 taxa each from Proteobacteria (20 detected HGTs) (Dataset S1).
Because the likelihood of HGT events increases for larger gene trees, next we studied the HGT-indices of genes harboring the maximum number of taxa (note that we excluded gene sets harboring >50% of genomes as their processing was computationally intensive and HGT event assignments were conceptually challenging; Table 2). Remarkably, the second-highest scoring hit belonged to the S13/S18 proteins of the 30S ribosomal subunit (PF00416) for which a total of 245 HGT events were detected in 915 taxa (HGT-index = 0.27) belonging to 11 major bacterial divisions indicating widespread transfer. The top-20 list also included fructose-1,6-bisphosphatase, an enzyme involved in cold adaptation and hibernation in mammals (Storey, 1997), other metabolic enzymes, and the S18 and (L13 at #23) proteins structural constituents of ribosomes. Interestingly, the majority of these transfers included many bacterial phyla (Table 2). Performing a keyword count on the annotated biological process gene ontology (GO) terms (Ashburner et al., 2000) revealed that most of the HGT-genes were involved in metabolic (n = 3,582 words), transport (3,565), transcription (2,766), transduction (1,791), and translation (875), along with antibiotic and toxin (27 each), stress (285), homeostasis (221), rRNA (211), and transposition (174) processes indicating the wide range of functions mediated by HGT in prokaryotes.
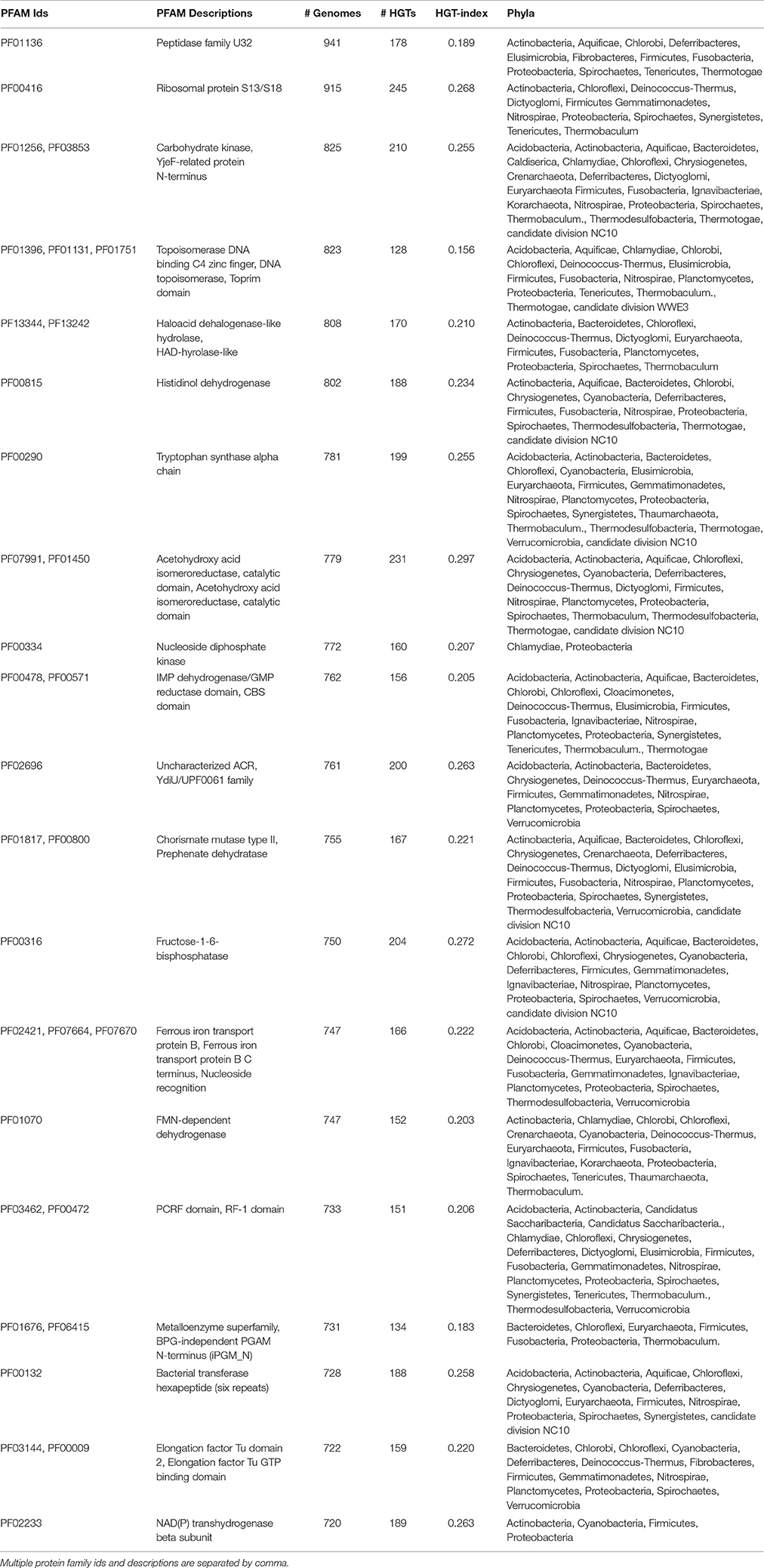
Table 2. Pfam Ids and descriptions are given for top-twenty HGT-genes with the highest number of genomes.
Discussion
We identified genes that are frequently or non-frequently exchanged among prokaryotic species (Datasets S1, S2). Identification of frequently-transferred proteins among prokaryotes is invaluable for ongoing evolutionary biology and microbiology studies to combat, for example, the widespread antibiotic resistance (Huddleston, 2014) and to better understand bacterial evolution in natural habitats (Smillie et al., 2011). In turn, identification of genes not massively influenced by HGT carries implications for our efforts to reconstruct the deep evolutionary history of cellular organisms (and viruses) by accurate depictions of the tree of life (ToL) using molecular markers that are relatively robust to non-vertical evolution. Our effort thus could become important starting points from both public health and basic science perspectives, as we provide a preliminary list of HGT-related genes in prokaryotes through exhaustive implementations of tree reconstructions and reconciliations in available completely-sequenced prokaryotic genomes.
We refer to the list of HGT genes as “preliminary” since we only studied HGT sensitivity of prokaryotic genes that were distributed in at least 10 but <50% of available genomes. The former criterion was implemented to ensure HGT-indices across some genes were relatively comparable. For example, a gene detected in only four genomes could have two transfers resulting in a higher HGT-index of 0.5. In turn, the latter criterion of excluding very large gene sets comprising of >50% of available genomes in Jeong et al. (2016) could be a major limitation of our study. While, widespread genes are more likely to be inherited vertically (Nasir and Caetano-Anollés, 2013), it is possible that spread of some of these genes was increased laterally over evolutionary timespans leading to their presence in nearly all known prokaryotic species. Exploring the HGT sensitivity of widespread genes, for example, ribosomal proteins, is especially important since it is common practice to concatenate ribosomal proteins in attempts to reconstruct trees of life describing the evolutionary history of cellular organisms (e.g., in Hug et al., 2016). However, dealing with large gene sets followed by large-scale reconstructions and reconciliations is computationally and conceptually challenging (prompting gene concatenation) and that is why, gene sets carrying >50% genomes were excluded during the initial reconstruction of HGTree (Jeong et al., 2016). This is however a challenge we are undertaking in an independent study (Jeong et al., unpublished data).
Our approach of HGT detection relies on an explicit evolutionary method of tree reconstruction and reconciliation, generally known to be reliable in HGT detection, especially of ancestral events (Lawrence and Ochman, 2002). The method however differs starkly from some of the composition-based methods, such as evaluating GC and codon biases across genomes to infer HGT (Lawrence and Ochman, 1997, 1998; Ochman et al., 2000), that generally lose effectiveness in detecting HGTs involving longer evolutionary timespans (Lawrence and Ochman, 2002; Ravenhall et al., 2015). Moreover, inferring HGT using different genomic signatures can sometimes lead to different results (Becq et al., 2010). Hence, the composition-based method is perhaps not well suited for large-scale genome-wide global detection of HGT events across thousands of sequenced genomes, and especially, for detection of ancestral gene transfers (Lawrence and Ochman, 2002).
On balance, the phylogenetic method of HGT detection is expected to not perform very well for HGTs occurring between very closely related strains or species that likely do not produce detectable tree incongruence (see Adato et al., 2015 for a possible solution based on the synteny index). In addition, the method, by default, is restricted to genes and protein coding sequences of prokaryotic genomes and precludes detection of transfer of non-coding DNA. Similarly, addition of viral and eukaryotic genomes to the phylogeny-based HGT detection pipeline remains an open challenge since both sets of genomes pose unique challenges. For example, integration of eukaryotic genomes will raise questions regarding the choice of correct “outgroup” to root the reference ToL during tree reconciliation step, a topic that remains controversial (Gouy et al., 2015). Eukaryotic genomes also house large chunks of non-coding sequences that are horizontally acquired such as the acquisition of endogenous viral-like elements (Katzourakis and Gifford, 2010). In turn, viruses are frequent mediators of genetic exchange and there are numerous instances of gene transfer in either direction from cells to viruses and from viruses to cells, albeit the latter seems to be quantitatively dominant as demonstrated by recent large-scale bioinformatics studies (Daubin et al., 2003; Cortez et al., 2009; Nasir and Caetano-Anollés, 2015; Forterre, 2016; Nasir et al., 2017). However, the place of viruses in the ToL is hotly debated (Claverie and Ogata, 2009; Moreira and Lopez-Garcia, 2009), again a concept that is now increasingly been revisited (Nasir et al., 2012; Nasir and Caetano-Anollés, 2015; Claverie and Abergel, 2016; Forterre, 2016). Importantly, viruses do not encode a universal gene marker like 16S or 18S rRNA in cells to be used for the reconstruction of reference species trees. Therefore, virus-mediated gene transfer can perhaps be better studied by utilizing comparative genomics approaches, as in Nasir and Caetano-Anollés (2015), or using protein-folds (Nasir and Caetano-Anollés, 2017) that are relatively more conserved than molecular sequences (Illergård et al., 2009; Abrescia et al., 2012).
Considering the above-mentioned limitations, the number of HGTs detected in our studies are likely to be underestimated. However, no (computational) method of HGT detection is expected to be 100% accurate owing to the inherent complexity underlying the myriad of ways microorganisms interact with each other in natural environments and considering the billions of years of evolutionary timespan for the age of some of the microbial genomes. Therefore, the reported lists of genes that are frequently (Dataset S1) or non-frequently transferred (Dataset S2) among prokaryotic genomes only represent “candidate” HGT genes and should be interpreted that way. We also caution that “orthologous” gene sets vary greatly in species composition and thus HGT-indices across genes may not always be comparable. For example, a gene could be present in 40% available genomes belonging to several microbial phyla and genera indicating high phylogenetic diversity. In comparison, some genes could be narrowly distributed among prokaryotes indicating low phylogenetic diversity. It is logical to think that the number of HGTs would correlate with the phylogenetic diversity of the gene set (as conflicts would become more visible) and thus HGT-index could perhaps further be normalized by the tree length of the reference species tree (or by addition of some other parameters). As counter argument, however, it can be argued that HGTs would increase among organisms that are either closely-related in evolution (expected to harbor a similar mobilome) or reside in close physical proximity (allows greater cell-to-cell contact to facilitate more HGTs) than among microorganisms that are distantly-related either phylogenetically or ecologically (Smillie et al., 2011). In other words, a lot remains to be understood about HGT, especially since the emergence of metagenomic and microbiome datasets that enable a systems biology exploration of microbial life. Considering these complications, we have, for now, restricted the calculation of HGT-index of each gene by simply dividing the total number of detected HGT events on a gene tree by the total number of genomes member of that gene tree. Since, in our opinion, true HGT potential of a gene should be calculated considering both genomes that do and do not participate in its horizontal transfer. In a separate study, we are investigating the phylogenetic vs. ecological effect on the human microbiome dataset using the tree-based HGT-detection method to better understand the “rules” behind increasing/decreasing HGT (Jeong et al., unpublished data). Therefore, our study should be taken as a first step toward meeting the genomics and microbiology needs posed in the post-genomic era. Our lists are preliminary, identify interesting proteins that participate or interfere in antibiotic usage pathways that could have broad utility for evolutionary biologists and microbiologists, and are expected to inspire improved future studies on this topic to better tackle HGT in prokaryotic genomes.
Materials and Methods
Orthologous genes and HGT event mappings were retrieved directly from Jeong et al. (2016). In brief, protein sequences from 2,472 completely-sequenced prokaryotic organisms (156 Archaea and 2,316 Bacteria) were processed using the Ensembl homology prediction pipeline (Vilella et al., 2009) to produce homologous gene sets. Mestortho (ver. 2.0) (Kim et al., 2008) was used to extract orthologous gene sets from homologous clusters. 16S rRNA genes were detected by RNammer (ver. 1.2) (Lagesen et al., 2007). Multiple sequence alignments of orthologous genes and 16S rRNA genes were performed using CLUSTAL-omega (ver. 1.2.1) (Sievers et al., 2014). ML gene and reference trees were reconstructed using FastTree (ver. 2.0) (Price et al., 2010). For 16S rRNA reference trees, 18S rRNA sequence from Saccharomyces cerevisiae was added and treated as “outgroup” to root the reference tree. Gene and reference trees were reconciled using RANGER-DTL-U algorithm (ver. 1.0) (Bansal et al., 2012). Protein families (Finn et al., 2014) were assigned to orthologous gene sets based on the 80% “majority” rule [i.e., when 80% of proteins in a given gene set yielded the same Pfam ID when scanned via the PfamScan (ver. 1.3) algorithm]. Molecular functions, biological processes, and cellular component descriptions, as defined by the GO database (Ashburner et al., 2000). HGT events on gene trees were compared using in-house Python scripts. The impact of multiple equally parsimonious reconciliations between gene and reference trees and their influence on resulting duplication, transfer, or loss event assignments was not explored. Similarly, the choice of root among equally parsimonious rootings of gene tree when reconciling against rooted species tree was also not evaluated. Both due to computational infeasibility considering the size of our dataset and available computational resources.
Author Contributions
Both authors contributed to the design and analysis of the study and wrote, edited, and approved the final manuscript.
Funding
AN is supported by the Higher Education Commission, Start-up Research Grant Program (Project no. 21-519/SRGP/R&D/HEC/2014).
Conflict of Interest Statement
The authors declare that the research was conducted in the absence of any commercial or financial relationships that could be construed as a potential conflict of interest.
Acknowledgments
We thank Kyung Mo Kim and Heebal Kim for valuable discussions related to HGT that led to the present study.
Supplementary Material
The Supplementary Material for this article can be found online at: http://journal.frontiersin.org/article/10.3389/fgene.2017.00112/full#supplementary-material
Deposited Data and Information to the User
Datasets S1, S2 have been deposited to figshare and can be retrieved at https://figshare.com/s/ab90c3e792d651a61d39. Users can download and reuse the data for research purpose only with an acknowledgment to us and citing this paper and the original HGTree study (Jeong et al., 2016).
Dataset S1. A list of 69,365 candidate HGT-genes. Each gene set is given a numeric Id. The second column gives accession numbers for all proteins member of the gene set. Protein family assignments and GO annotations for biological processes, cellular component, and molecular function GO terms are listed next to each gene set. Multiple Pfam and GO hits are separated by comma. Pfam assignments rely on at least 80% of genes in any orthologous gene set matching the same Pfam Id. HGT-index is the fraction of total detected HGT events in each gene tree divided by the total number of taxa (genomes) in that gene tree. Data sorted by HGT-index in a descending manner.
Dataset S2. A list of 47,980 candidate HGT-free genes. Each gene set is given a numeric Id. The second column gives accession numbers for all proteins member of the gene set. Protein family assignments and GO annotations for biological processes, cellular component, and molecular function GO terms are listed next to each gene set. Multiple Pfam and GO hits are separated by comma. Pfam assignments rely on at least 80% of genes in any orthologous gene set matching the same Pfam Id.
References
Abrescia, N. G., Bamford, D. H., Grimes, J. M., and Stuart, D. I. (2012). Structure unifies the viral universe. Annu. Rev. Biochem. 81, 795–822. doi: 10.1146/annurev-biochem-060910-095130
Adato, O., Ninyo, N., Gophna, U., Snir, S., Koonin, E. V., Galperin, M. Y., et al. (2015). Detecting horizontal gene transfer between closely related taxa. PLOS Comput. Biol. 11:e1004408. doi: 10.1371/journal.pcbi.1004408
Ashburner, M., Ball, C. A., Blake, J. A., Botstein, D., Butler, H., Cherry, J. M., et al. (2000). Gene ontology: tool for the unification of biology. Gene Ontol. Consort. Nat. Genet. 25, 25–29. doi: 10.1038/75556
Bansal, M. S., Alm, E. J., and Kellis, M. (2012). Efficient algorithms for the reconciliation problem with gene duplication, horizontal transfer and loss. Bioinformatics 28, i283–i291. doi: 10.1093/bioinformatics/bts225
Becq, J., Churlaud, C., and Deschavanne, P. (2010). A benchmark of parametric methods for horizontal transfers detection. PLoS ONE 5:e9989. doi: 10.1371/journal.pone.0009989
Claverie, J. M., and Abergel, C. (2016). Giant viruses: the difficult breaking of multiple epistemological barriers. Stud. Hist. Philos. Biol. Biomed. Sci. 59, 89–99. doi: 10.1016/j.shpsc.2016.02.015
Claverie, J. M., and Ogata, H. (2009). Ten good reasons not to exclude giruses from the evolutionary picture. Nat. Rev. 7:615; author reply: 615. doi: 10.1038/nrmicro2108-c3
Cortez, D., Forterre, P., and Gribaldo, S. (2009). A hidden reservoir of integrative elements is the major source of recently acquired foreign genes and ORFans in archaeal and bacterial genomes. Genome Biol. 10:R65. doi: 10.1186/gb-2009-10-6-r65
Daubin, V., Lerat, E., Perrière, G., Sueoka, N., Grantham, R., Gautier, C., et al. (2003). The source of laterally transferred genes in bacterial genomes. Genome Biol. 4:R57. doi: 10.1186/gb-2003-4-9-r57
Finn, R. D., Bateman, A., Clements, J., Coggill, P., Eberhardt, R. Y., Eddy, S. R., et al. (2014). Pfam: the protein families database. Nucleic Acids Res. 42, D222–D230. doi: 10.1093/nar/gkt1223
Forterre, P. (2016). To be or not to be alive: how recent discoveries challenge the traditional definitions of viruses and life. Stud. Hist. Philos. Biol. Biomed. Sci. 59, 100–108. doi: 10.1016/j.shpsc.2016.02.013
Gene Ontology Consortium (2015). Gene Ontology Consortium: going forward. Nucleic Acids Res. 43, D1049–D1056. doi: 10.1093/nar/gku1179
Gouy, R., Baurain, D., and Philippe, H. (2015). Rooting the tree of life: the phylogenetic jury is still out. Philos. Trans. R. Soc. Lond. B Biol. Sci. 370:20140329. doi: 10.1098/rstb.2014.0329
Huddleston, J. R. (2014). Horizontal gene transfer in the human gastrointestinal tract: potential spread of antibiotic resistance genes. Infect. Drug Resist. 7, 167–176. doi: 10.2147/IDR.S48820
Hug, L. A., Baker, B. J., Anantharaman, K., Brown, C. T., Probst, A. J., Castelle, C. J., et al. (2016). A new view of the tree of life. Nat. Microbiol. 1:16048. doi: 10.1038/nmicrobiol.2016.48
Illergård, K., Ardell, D. H., and Elofsson, A. (2009). Structure is three to ten times more conserved than sequence–a study of structural response in protein cores. Proteins 77, 499–508. doi: 10.1002/prot.22458
Jeong, H., Sung, S., Kwon, T., Seo, M., Caetano-Anollés, K., Choi, S. H., et al. (2016). HGTree: database of horizontally transferred genes determined by tree reconciliation. Nucleic Acids Res. 44, D610–619. doi: 10.1093/nar/gkv1245
Katzourakis, A., and Gifford, R. J. (2010). Endogenous viral elements in animal genomes. PLoS Genet. 6:e1001191. doi: 10.1371/journal.pgen.1001191
Kim, K. M., Sung, S., Caetano-Anolles, G., Han, J. Y., Kim, H., Caetano-Anollés, G., et al. (2008). An approach of orthology detection from homologous sequences under minimum evolution. Nucleic Acids Res. 36:e110. doi: 10.1093/nar/gkn485
Lagesen, K., Hallin, P., Rodland, E. A., Staerfeldt, H. H., Rognes, T., and Ussery, D. W. (2007). RNAmmer: consistent and rapid annotation of ribosomal RNA genes. Nucleic Acids Res. 35, 3100–3108. doi: 10.1093/nar/gkm160
Lawrence, J. G., and Ochman, H. (1997). Amelioration of bacterial genomes: rates of change and exchange. J. Mol. Evol. 44, 383–397. doi: 10.1007/PL00006158
Lawrence, J. G., and Ochman, H. (1998). Molecular archaeology of the Escherichia coli genome. Proc. Natl. Acad. Sci. U.S.A. 95, 9413–9417. doi: 10.1073/pnas.95.16.9413
Lawrence, J. G., and Ochman, H. (2002). Reconciling the many faces of lateral gene transfer. Trends Microbiol. 10, 1–4. doi: 10.1016/S0966-842X(01)02282-X
Mehta, R., and Champney, W. S. (2002). 30S ribosomal subunit assembly is a target for inhibition by aminoglycosides in Escherichia coli. Antimicrob. Agents Chemother. 46, 1546–1549. doi: 10.1128/AAC.46.5.1546-1549.2002
Moreira, D., and Lopez-Garcia, P. (2009). Ten reasons to exclude viruses from the tree of life. Nat. Rev. 7, 306–311. doi: 10.1038/nrmicro2108
Nasir, A., and Caetano-Anollés, G. (2013). Comparative analysis of proteomes and functionomes provides insights into origins of cellular diversification. Archaea 2013:648746. doi: 10.1155/2013/648746
Nasir, A., and Caetano-Anollés, G. (2015). A phylogenomic data-driven exploration of viral origins and evolution. Sci. Adv. 1:e1500527. doi: 10.1126/sciadv.1500527
Nasir, A., and Caetano-Anollés, G. (2017). Identification of capsid/coat related protein folds and their utility for virus classification. Front. Microbiol. 8:380. doi: 10.3389/fmicb.2017.00380
Nasir, A., Kim, K. M., and Caetano-Anolles, G. (2012). Giant viruses coexisted with the cellular ancestors and represent a distinct supergroup along with superkingdoms Archaea, Bacteria and Eukarya. BMC Evol. Biol. 12:156. doi: 10.1186/1471-2148-12-156
Nasir, A., Kim, K. M., and Caetano-Anollés, G. (2017). Long-term evolution of viruses: a Janus-faced balance. Bioessays 39:e201700026. doi: 10.1002/bies.201700026
Ochman, H., Lawrence, J. G., and Groisman, E. A. (2000). Lateral gene transfer and the nature of bacterial innovation. Nature 405, 299–304. doi: 10.1038/35012500
Podell, S., Gaasterland, T., and Allen, E. E. (2008). A database of phylogenetically atypical genes in archaeal and bacterial genomes, identified using the DarkHorse algorithm. BMC Bioinformatics 9:419. doi: 10.1186/1471-2105-9-419
Price, M. N., Dehal, P. S., and Arkin, A. P. (2010). FastTree 2 – approximately maximum-likelihood trees for large alignments. PLoS ONE 5:e9490. doi: 10.1371/journal.pone.0009490
Punta, M., Coggill, P. C., Eberhardt, R. Y., Mistry, J., Tate, J., Boursnell, C., et al. (2012). The Pfam protein families database. Nucleic Acids Res. 40, D290–D301. doi: 10.1093/nar/gkr1065
Ravenhall, M., Škunca, N., Lassalle, F., and Dessimoz, C. (2015). Inferring horizontal gene transfer. PLoS Comput. Biol. 11:e1004095. doi: 10.1371/journal.pcbi.1004095
Sievers, F., Wilm, A., Dineen, D., Gibson, T. J., Karplus, K., Li, W., et al. (2014). Fast, scalable generation of high-quality protein multiple sequence alignments using Clustal Omega. Mol. Syst. Biol. 7, 539–539. doi: 10.1038/msb.2011.75
Smillie, C. S., Smith, M. B., Friedman, J., Cordero, O. X., David, L. A., and Alm, E. J. (2011). Ecology drives a global network of gene exchange connecting the human microbiome. Nature 480, 241–244. doi: 10.1038/nature10571
Soucy, S. M., Huang, J., and Gogarten, J. P. (2015). Horizontal gene transfer: building the web of life. Nat. Rev. Genet. 16, 472–482. doi: 10.1038/nrg3962
Storey, K. B. (1997). Metabolic regulation in mammalian hibernation: enzyme and protein adaptations. Comp. Biochem. Physiol. A Physiol. 118, 1115–1124. doi: 10.1016/S0300-9629(97)00238-7
Vilella, A. J., Severin, J., Ureta-Vidal, A., Heng, L., Durbin, R., and Birney, E. (2009). EnsemblCompara GeneTrees: complete, duplication-aware phylogenetic trees in vertebrates. Genome Res. 19, 327–335. doi: 10.1101/gr.073585.107
von Wintersdorff, C. J. H., Penders, J., van Niekerk, J. M., Mills, N. D., Majumder, S., van Alphen, L. B., et al. (2016). Dissemination of antimicrobial resistance in microbial ecosystems through horizontal gene transfer. Front. Microbiol. 7:173. doi: 10.3389/fmicb.2016.00173
WHO Model List of Essential Medicines 18th list (2013). Available online at: http://www.who.int/medicines/publications/essentialmedicines/en/index.html (Accessed December 28, 2016).
Keywords: phylogenetics, horizontal gene transfer, bacterial evolution, tree reconstruction, tree reconciliation
Citation: Jeong H and Nasir A (2017) A Preliminary List of Horizontally Transferred Genes in Prokaryotes Determined by Tree Reconstruction and Reconciliation. Front. Genet. 8:112. doi: 10.3389/fgene.2017.00112
Received: 16 June 2017; Accepted: 14 August 2017;
Published: 28 August 2017.
Edited by:
Peng Luo, South China Sea Institute of Oceanology (CAS), ChinaReviewed by:
Camilo E. Khatchikian, University of Texas at El Paso, United StatesVinicius Abreu, University of São Paulo, Brazil
Copyright © 2017 Jeong and Nasir. This is an open-access article distributed under the terms of the Creative Commons Attribution License (CC BY). The use, distribution or reproduction in other forums is permitted, provided the original author(s) or licensor are credited and that the original publication in this journal is cited, in accordance with accepted academic practice. No use, distribution or reproduction is permitted which does not comply with these terms.
*Correspondence: Arshan Nasir, YXJzaGFuLm5hc2lyQGdtYWlsLmNvbQ==
†Present Address: Hyeonsoo Jeong, School of Biological Sciences, Georgia Institute of Technology, Atlanta, GA, United States