- Department of Neurology and Neuroscience Center, First Hospital of Jilin University, Changchun, China
Alzheimer’s disease (AD) is the most common neurodegenerative disease in the elderly and the leading cause of dementia in humans. Evidence shows that cellular trafficking and recycling machineries are associated with AD risk. A recent study found that the coat protein complex I (COPI)–dependent trafficking in vivo could significantly reduce amyloid plaques in the cortex and hippocampus of neurological in the AD mouse models and identified 12 single-nucleotide polymorphisms in COPI genes to be significantly associated with increased AD risk using 6,795 samples. Here, we used a large-scale GWAS dataset to investigate the potential association between the COPI genes and AD susceptibility by both SNP and gene-based tests. The results showed that only rs9898218 was associated with AD risk with P = 0.017. We further conducted an expression quantitative trait loci (eQTLs) analysis and found that rs9898218 G allele was associated with increased COPZ2 expression in cerebellar cortex with P = 0.0184. Importantly, the eQTLs analysis in whole blood further indicated that 11 of these 12 genetic variants could significantly regulate the expression of COPI genes. Hence, these findings may contribute to understand the association between COPI genes and AD susceptibility.
Introduction
Alzheimer’s disease (AD) is the most common neurodegenerative disease in the elderly and the leading cause of dementia in humans (Jiang et al., 2017; Liu et al., 2017b). It is suggested that genetic risk factors could cause AD (Van Cauwenberghe et al., 2016). In recent years, kinds of methods have been used to detect the underlying AD genetic factors. For example, candidate gene studies have identified mutations in APP, PSEN1, and PSEN2 to be associated with autosomal dominant AD (Van Cauwenberghe et al., 2016). APOE has been reported to be associated with both early- and late-onset AD (Van Cauwenberghe et al., 2016). Large-scale genome-wide association studies (GWASs) have identified several novel genetic risk loci in European population, and candidate gene studies have replicated these findings in other populations (Liu et al., 2012; Liu et al., 2013b; Liu et al., 2013c; Liu et al., 2013d; Liu et al., 2014a; Liu et al., 2014b; Liu et al., 2014c; Chen et al., 2015; Li et al., 2015; Shen et al., 2015; Zhang et al., 2015; Chang et al., 2016; Li et al., 2016; Liu and Jiang, 2016; Liu et al., 2016; Ma et al., 2016; Tan et al., 2016; Zhang et al., 2016b). Whole-genome sequencing has highlighted the role of TREM2 in AD (Jiang et al., 2016; Zhang et al., 2016a; Ulland and Colonna, 2018). However, these AD susceptibility loci could only explain 28.57% AD genetic risk (Cuyvers and Sleegers, 2016). Large proportion of AD heritability remains unclear.
It is reported that the cellular trafficking and recycling machineries are associated with AD risk (Bettayeb et al., 2016). Bettayeb et al. (2016) found that the coat protein complex I (COPI)–dependent trafficking in vivo could significantly reduce amyloid plaques in the cortex and hippocampus of neurological in the AD mouse models. Bettayeb et al. (2016) further analyzed the human genetic association study data and identified 12 single-nucleotide polymorphisms (SNPs) in COPI genes, which are significantly associated with increased AD risk including COPA (rs7531886 and rs12033011), COPB1 (rs72868007), COPD/IFT46 (rs73022058 and rs3132828), COPD/PHLDB1 (rs498872), COPZ1 (rs34280607 and rs61614746), COPZ2 (rs757352, rs9898218, rs7216504), and COPZ2/NFE2L1 (rs11650615).
In their study, Bettayeb et al. Bettayeb et al. (2016) selected six independent study cohorts including two family-based studies and four case-control association studies and further performed a meta-analysis using a total of 6,795 samples (4,018 AD cases and 2,777 controls). Until now, large-scale GWASs have been performed (Harold et al., 2009; Lambert et al., 2009; Holliday et al., 2012; Lambert et al., 2013; Jansen et al., 2019). Hence, we used a large-scale AD GWAS dataset to investigate the association of these 12 genetic variants and the COPI genes and AD risk by a single SNP test and a gene-based test (Lambert et al., 2013). Meanwhile, considering the unknown function of the significant SNP, we conducted an expression quantitative trait loci (eQTLs) analysis.
Materials and Methods
AD GWAS Dataset
We selected the AD GWAS dataset from the International Genomics of Alzheimer’s Project (IGAP) (Lambert et al., 2013). International Genomics of Alzheimer’s Project is a large two-stage study based on GWAS on individuals of European ancestry. In stage 1, IGAP used genotyped and imputed data on 7,055,881 SNPs for meta-analysis of four previously published GWAS datasets consisting of 17,008 Alzheimer’s disease cases and 37,154 controls (European Alzheimer’s disease Initiative, Alzheimer Disease Genetics Consortium, Cohorts for Heart and Aging Research in Genomic Epidemiology consortium, Genetic and Environmental Risk in AD consortium) (Lambert et al., 2013). All patients with AD satisfied the National Institute of Neurological and Communicative Disorders and Stroke and the Alzheimer’s Disease and Related Disorders Association criteria or the Diagnostic and Statistical Manual of Mental Disorders, Fourth Edition guidelines (Lambert et al., 2013). Previous studies have provided more detailed information about IGAP dataset (Jiang et al., 2017; Liu et al., 2017a; Liu et al., 2018d).
SNP-Based Test
Here, we investigated the association between these 12 variants and AD susceptibility using the summary association results from the above study (Lambert et al., 2013). If one of these 12 variants is not available in the AD GWAS dataset, we used HaploReg (version 4) to identify the proxy SNPs based on the linkage disequilibrium (LD) information in 1000 Genomes Project (Ward and Kellis, 2012).
Gene-Based Test
We performed a gene-based test of this large-scale AD GWAS dataset using a common method PLINK (SET SCREEN TEST) (Moskvina et al., 2011). PLINK is a meta-analysis using all the SNPs in the corresponding genes (Moskvina et al., 2011). The method uses an approximate Fisher’s test to combine P values across all the SNPs in genes and adjusts for LD (Moskvina et al., 2011). Meanwhile, we performed a gene-based test of this large-scale AD GWAS dataset using VEGAS (Liu et al., 2010). VEGAS software incorporates information from all SNPs within a gene and adjusts the gene sizes, SNP density, and the LD between SNPs (Liu et al., 2010). VEGAS assigns SNPs to 17,787 autosomal genes according to the positions of SNPs and genes (± 50 kb from the 5′ and 3′ UTR) (Liu et al., 2010). Previous studies have provided more detailed information about the PLINK and VEGAS methods (Liu et al., 2013a; Liu et al., 2017d; Li et al., 2018; Lang et al., 2019).
eQTLs Analysis
We selected the eQTLs dataset from the Brain eQTL Almanac (Braineac), which is a web-based resource to access the UK Brain Expression Consortium dataset (Ramasamy et al., 2014). This resource included 134 neuropathologically normal individuals of European descent in 10 brain tissues (Ramasamy et al., 2014). For each sample, we got his/her COPI gene expression data and the genotype data for 12 SNPs (Ramasamy et al., 2014). We then evaluated their association with nearby gene expression using a linear regression analysis under an additive model. In addition to normal human brain tissues, we further evaluated whether these genetic variants could regulate the expression of nearby genes in neurodegenerative disease tissues. We selected two eQTLs datasets from 197 AD cerebellar samples and 202 AD temporal cortex samples (Zou et al., 2012). The significance level is P < 0.05. Recent studies have provided more detailed information about the eQTLs using Braineac (Hu et al., 2017; Liu et al., 2017a; Liu et al., 2017c; Liu et al., 2018a; Liu et al., 2018b; Liu et al., 2018c; Zhang et al., 2018). In addition, we conducted an eQTLs analysis in whole blood using the large-scale dataset from the eQTLGen Consortium (Võsa et al., 2018). The consortium incorporates 37 datasets, with a total of 31,684 individuals (Võsa et al., 2018).
Results
SNP-Based Test
Using SNP-based test, we found that 10 of the 12 SNPs were included in this GWAS dataset except rs7531886 and rs34280607 variants. We further applied HaploReg (version 4) to identify their proxy SNPs based on the LD information from the 1000 Genomes Project (EUR) (Ward and Kellis, 2012). We selected two best tagged SNPs including rs2298104 LD with rs7531886 (r2 = 0.84 and D′ = 0.99), as well as rs34192202 LD with rs34280607 (r2 = 0.69 and D′ = 0.91). The results indicated that among these 12 SNPs only rs9898218 showed significant association with AD risk with P = 0.017, as described in Table 1.
Gene-Based Test
Using the gene-based test, our results further showed no significant association between these COPI genes and AD susceptibility (significance level 0.05), including COPA (P = 0.3186 and 0.342), COPB1 (P = 0.6095 and 0.212), COPD/PHLDB1 (P = 0.2942 and 0.325), COPZ1 (P = 0.3803 and 0.454), and COPZ2 (P = 0.08175 and 0.177) using PLINK and VEGAS, respectively.
eQTLs Analysis
As provided in Table 1, rs9898218 is the only SNP associated with AD risk with P = 0.017. We first evaluated the association between rs9898218 and COPZ2 expression using the Braineac dataset. The results showed that rs9898218 G allele was associated with increased COPZ2 expression in cerebellar cortex (P = 1.84E−02), but not in other nine brain tissues (Table 2).
In cerebellar cortex, rs2298104 and rs7531886 were associated with the expression of COPA, and the rs11650615 was associated with the expression of COPZ2. In occipital cortex, both rs12033011 and rs7531886 were associated with the expression of COPA. In putamen, rs12033011, rs2298104, and rs7531886 were associated with the expression of COPA (Table 3).
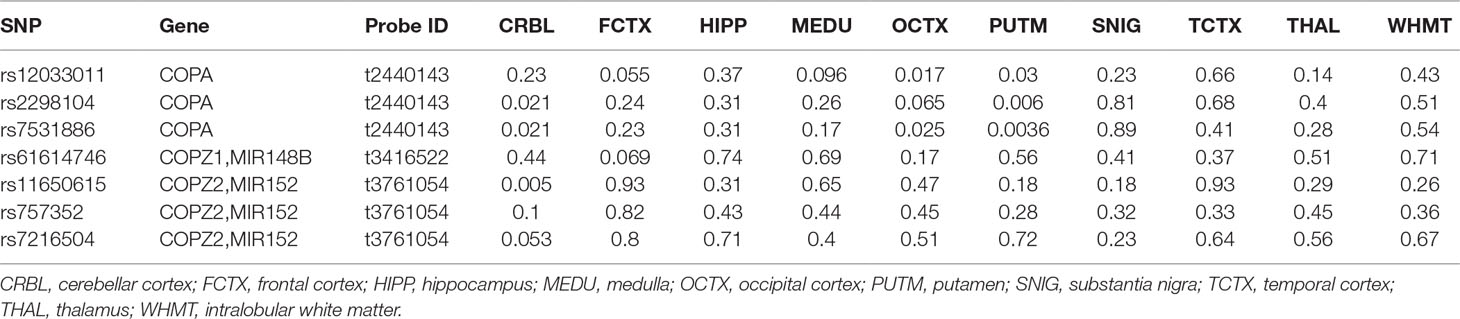
Table 3 P values for the association between other variants excluding rs9898218 and COPI gene expression in the Braineac dataset.
In two AD eQTLs datasets, 3 (rs11650615, rs9898218, and rs498872) of these 12 genetic variants are available. However, none of these three genetic variants was associated with the COPI gene expression in AD cerebellar and temporal cortex tissues (Table 4).
In whole blood, the eQTLs analysis showed that 11 of these 12 genetic variants could significantly regulate the expression of COPI genes including COPA, COPB1, COPZ1, COPZ2, IFT46, NFE2L1, and PHLDB1 (Table 5).
Discussion
In recent years, COPI genes have been reported to be potentially involved in AD (Liu et al., 2015). For example, a cluster analysis of microarray data indicated the association between COPA and AD (Guttula et al., 2012). Dynamic regulatory network reconstruction analysis showed gradually depressed activity of COPA (Kong et al., 2014). Bettayeb et al. (2016) highlighted 12 SNPs including rs7531886, rs12033011, rs72868007, rs73022058, rs3132828, rs498872, rs34280607, rs61614746, rs757352, rs9898218, rs7216504, and rs11650615 in COPI genes COPA, COPB1, COPD/IFT46, COPD/PHLDB1, COPZ1, COPZ2, and COPZ2/NFE2L1 to be significantly associated with increased AD risk.
With the wide application of GWAS method in AD, it is possible and rapid to validate a finding using large-scale AD GWAS dataset. Here, we selected a large-scale AD GWAS dataset and performed both SNP and gene-based tests. We think that this large-scale dataset may be more powerful than the original dataset (Bettayeb et al., 2016). Using SNP-based test, the results showed that rs9898218 T allele could increase AD risk with β = 0.040 and P = 0.017. Two gene-based test methods indicated no significant association between these COPI genes and AD susceptibility. Interestingly, eQTLs analysis further showed that rs9898218 T allele could reduce COPZ2 expression in cerebellar cortex with β = −0.069 and P = 1.84E−02, but not in other nine brain tissues. Meanwhile, we identified other four genetic variants (rs12033011, rs2298104, rs7531886, and rs11650615) regulating the COPI gene expression in other human brain tissues. Importantly, the eQTLs analysis in whole blood further indicated that 11 of these 12 genetic variants could significantly regulate the expression of COPI genes.
COPZ2 encodes a member of the adaptor complexes small subunit family (Shtutman et al., 2011). Evidence showed down-regulated COPZ2 expression in most tumor cell lines and in individuals with kinds of cancer types (Shtutman et al., 2011). Interestingly, recent studies have highlighted the role of COPZ2 in AD (Ciryam et al., 2016; Wan Nasri et al., 2018). Wan Nasri et al. (2018) evaluated the effect of 6 months of tocotrienol rich fraction supplementation on gene expression in the hippocampus of wild-type group (n = 4) and APPswe/PS1dE9 double transgenic AD mice (n = 4). They found that Copz2 was significantly down-regulated in AD group compared with the WT group (P = 6.44E−05 and fold change = −4.5788) (Wan Nasri et al., 2018). Ciryam et al. (2016) conducted a meta-analysis of gene expression data from about 1,600 human central nervous system tissues to investigate the transcriptional changes upon aging and as a result of AD. They found that COPZ2 was up-regulated in AD (P = 3.90E−05 and fold change = 1.13).
In summary, these findings may provide important information about the association between COPI genes and AD susceptibility. Meanwhile, future studies are required to replicate these findings using large-scale GWAS and eQTLs datasets.
Data Availability
Publicly available datasets were analyzed in this study. These data can be found here: http://web.pasteur-lille.fr/en/recherche/u744/igap/igap_download.php.
Author Contributions
LS and HZ proposed the project. YY collected and analyzed the data. All authors wrote the manuscript and approved the final version of the manuscript.
Conflict of Interest Statement
The authors declare that the research was conducted in the absence of any commercial or financial relationships that could be construed as a potential conflict of interest.
Acknowledgments
This work was supported by the National Natural Science Foundation of China (NSFC 81571231 and 81500911), Fund of Health and Family Planning Commission of Jilin Province (20152043), Key National Research Projects on Prevention and Control of Major Chronic Non-communicable Disease (2018YFC1312300) and the Fund of Science and Technology Development Project of Jilin Province (20180414041GH). The funders had no role in study design, data collection and analysis, decision to publish, or preparation of the manuscript.
References
Bettayeb, K., Hooli, B. V., Parrado, A. R., Randolph, L., Varotsis, D., Aryal, S., et al. (2016). Relevance of the COPI complex for Alzheimer’s disease progression in vivo. Proc. Natl. Acad. Sci. U.S.A. 113, 5418–5423. doi: 10.1073/pnas.1604176113
Chang, X. L., Tan, L., Tan, M. S., Wang, H. F., Tan, C. C., Zhang, W., et al. (2016). Association of HMGCR polymorphism with late-onset Alzheimer’s disease in Han Chinese. Oncotarget 7, 22746–22751. doi: 10.18632/oncotarget.8176
Chen, H., Wu, G., Jiang, Y., Feng, R., Liao, M., Zhang, L., et al. (2015). Analyzing 54,936 samples supports the association between CD2AP rs9349407 polymorphism and Alzheimer’s disease susceptibility. Mol. Neurobiol. 52, 1–7. doi: 10.1007/s12035-014-8834-2
Ciryam, P., Kundra, R., Freer, R., Morimoto, R. I., Dobson, C. M., Vendruscolo, M. (2016). A transcriptional signature of Alzheimer’s disease is associated with a metastable subproteome at risk for aggregation. Proc. Natl. Acad. Sci. U.S.A. 113, 4753–4758. doi: 10.1073/pnas.1516604113
Cuyvers, E., Sleegers, K. (2016). Genetic variations underlying Alzheimer’s disease: evidence from genome-wide association studies and beyond. Lancet Neurol. 15, 857–868. doi: 10.1016/S1474-4422(16)00127-7
Guttula, S. V., Allam, A., Gumpeny, R. S. (2012). Analyzing microarray data of Alzheimer’s using cluster analysis to identify the biomarker genes. Int. J. Alzheimers Dis. 2012, 649456. doi: 10.1155/2012/649456
Harold, D., Abraham, R., Hollingworth, P., Sims, R., Gerrish, A., Hamshere, M. L., et al. (2009). Genome-wide association study identifies variants at CLU and PICALM associated with Alzheimer’s disease. Nat. Genet. 41, 1088–1093. doi: 10.1038/ng.440
Holliday, E. G., Maguire, J. M., Evans, T. J., Koblar, S. A., Jannes, J., Sturm, J. W., et al. (2012). Common variants at 6p21.1 are associated with large artery atherosclerotic stroke. Nat. Genet. 44, 1147–1151. doi: 10.1038/ng.2397
Hu, Y., Cheng, L., Zhang, Y., Bai, W., Zhou, W., Wang, T., et al. (2017). Rs4878104 contributes to Alzheimer’s disease risk and regulates DAPK1 gene expression. Neurol. Sci. 38, 1255–1262. doi: 10.1007/s10072-017-2959-9
Jansen, I. E., Savage, J. E., Watanabe, K., Bryois, J., Williams, D. M., Steinberg, S., et al. (2019). Genome-wide meta-analysis identifies new loci and functional pathways influencing Alzheimer’s disease risk. Nat. Genet. 51, 404–413. doi: 10.1038/s41588-018-0311-9
Jiang, Q., Jin, S., Jiang, Y., Liao, M., Feng, R., Zhang, L., et al. (2017). Alzheimer’s disease variants with the genome-wide significance are significantly enriched in immune pathways and active in immune cells. Mol. Neurobiol. 54, 594–600. doi: 10.1007/s12035-015-9670-8
Jiang, T., Hou, J. K., Gao, Q., Yu, J. T., Zhou, J. S., Zhao, H. D., et al. (2016). TREM2 p.H157Y variant and the risk of Alzheimer’s disease: a meta-analysis involving 14,510 subjects. Curr. Neurovasc. Res. 13, 318–320. doi: 10.2174/1567202613666160808095530
Kong, W., Mou, X., Zhi, X., Zhang, X., Yang, Y. (2014). Dynamic regulatory network reconstruction for Alzheimer’s disease based on matrix decomposition techniques. Comput. Math. Methods Med. 2014, 891761. doi: 10.1155/2014/891761
Lambert, J. C., Heath, S., Even, G., Campion, D., Sleegers, K., Hiltunen, M., et al. (2009). Genome-wide association study identifies variants at CLU and CR1 associated with Alzheimer’s disease. Nat. Genet. 41, 1094–1099. doi: 10.1038/ng.439
Lambert, J. C., Ibrahim-Verbaas, C. A., Harold, D., Naj, A. C., Sims, R., Bellenguez, C., et al. (2013). Meta-analysis of 74,046 individuals identifies 11 new susceptibility loci for Alzheimer’s disease. Nat. Genet. 45, 1452–1458. doi: 10.1038/ng.2802
Lang, W., Wang, J., Ma, X., Zhang, N., Li, H., Cui, P., et al. (2019). Identification of shared genes between ischemic stroke and Parkinson’s disease using genome-wide association studies. Front. Neurol. 10, 297. doi: 10.3389/fneur.2019.00297
Li, H., Chen, L., Ma, X., Cui, P., Lang, W., Hao, J. (2018). Shared gene expression between multiple sclerosis and ischemic stroke. Front. Genet. 9, 598. doi: 10.3389/fgene.2018.00598
Li, X., Shen, N., Zhang, S., Liu, J., Jiang, Q., Liao, M., et al. (2015). CD33 rs3865444 polymorphism contributes to Alzheimer’s disease susceptibility in Chinese, European, and North American populations. Mol. Neurobiol. 52, 414–421. doi: 10.1007/s12035-014-8880-9
Li, Y., Song, D., Jiang, Y., Wang, J., Feng, R., Zhang, L., et al. (2016). CR1 rs3818361 polymorphism contributes to Alzheimer’s disease susceptibility in Chinese population. Mol. Neurobiol. 53, 4054–4059. doi: 10.1007/s12035-015-9343-7
Liu, G., Jiang, Q. (2016). Alzheimer’s disease CD33 rs3865444 variant does not contribute to cognitive performance. Proc. Natl. Acad. Sci. U.S.A. 113, E1589–E1590. doi: 10.1073/pnas.1600852113
Liu, G., Jiang, Y., Chen, X., Zhang, R., Ma, G., Feng, R., et al. (2013a). Measles contributes to rheumatoid arthritis: evidence from pathway and network analyses of genome-wide association studies. PLoS One 8, e75951. doi: 10.1371/journal.pone.0075951
Liu, G., Jiang, Y., Wang, P., Feng, R., Jiang, N., Chen, X., et al. (2012). Cell adhesion molecules contribute to Alzheimer’s disease: multiple pathway analyses of two genome-wide association studies. J. Neurochem. 120, 190–198. doi: 10.1111/j.1471-4159.2011.07547.x
Liu, G., Jin, S., Hu, Y., Jiang, Q. (2018a). Disease status affects the association between rs4813620 and the expression of Alzheimer’s disease susceptibility gene TRIB3. Proc. Natl. Acad. Sci. U.S.A. 115, E10519–E10520. doi: 10.1073/pnas.1812975115
Liu, G., Li, F., Zhang, S., Jiang, Y., Ma, G., Shang, H., et al. (2014a). Analyzing large-scale samples confirms the association between the ABCA7 rs3764650 polymorphism and Alzheimer’s disease susceptibility. Mol. Neurobiol. 50, 757–764. doi: 10.1007/s12035-014-8670-4
Liu, J. Z., Mcrae, A. F., Nyholt, D. R., Medland, S. E., Wray, N. R., Brown, K. M., et al. (2010). A versatile gene-based test for genome-wide association studies. Am. J. Hum. Genet. 87, 139–145. doi: 10.1016/j.ajhg.2010.06.009
Liu, G., Sun, J. Y., Xu, M., Yang, X. Y., Sun, B. L. (2017a). SORL1 variants show different association with early-onset and late-onset Alzheimer’s disease risk. J.Alzheimers Dis. 58, 1121–1128. doi: 10.3233/JAD-170005
Liu, G., Wang, H., Liu, J., Li, J., Li, H., Ma, G., et al. (2014b). The CLU gene rs11136000 variant is significantly associated with Alzheimer’s disease in Caucasian and Asian populations. Neuromolecular Med. 16, 52–60. doi: 10.1007/s12017-013-8250-1
Liu, S. L., Wang, X. C., Tan, M. S., Wang, H. F., Zhang, W., Wang, Z. X., et al. (2016). NME8 rs2718058 polymorphism with Alzheimer’s disease risk: a replication and meta-analysis. Oncotarget 7, 36014–36020. doi: 10.18632/oncotarget.9086
Liu, G., Wang, T., Tian, R., Hu, Y., Han, Z., Wang, P., et al. (2018b). Alzheimer’s disease risk variant rs2373115 regulates GAB2 and NARS2 expression in human brain tissues. J. Mol. Neurosci. 66, 37–43. doi: 10.1007/s12031-018-1144-9
Liu, W., Wu, A., Pellegrini, M., Wang, X. (2015). Integrative analysis of human protein, function and disease networks. Sci. Rep. 5, 14344. doi: 10.1038/srep14344
Liu, G., Xu, Y., Jiang, Y., Zhang, L., Feng, R., Jiang, Q. (2017b). PICALM rs3851179 variant confers susceptibility to Alzheimer’s disease in Chinese population. Mol. Neurobiol. 54, 3131–3136. doi: 10.1007/s12035-016-9886-2
Liu, G., Yao, L., Liu, J., Jiang, Y., Ma, G., Chen, Z., et al. (2014c). Cardiovascular disease contributes to Alzheimer’s disease: evidence from large-scale genome-wide association studies. Neurobiol. Aging 35, 786–792. doi: 10.1016/j.neurobiolaging.2013.10.084
Liu, G., Zhang, S., Cai, Z., Li, Y., Cui, L., Ma, G., et al. (2013c). BIN1 gene rs744373 polymorphism contributes to Alzheimer’s disease in East Asian population. Neurosci. Lett. 544, 47–51. doi: 10.1016/j.neulet.2013.02.075
Liu, G., Zhang, S., Cai, Z., Ma, G., Zhang, L., Jiang, Y., et al. (2013d). PICALM gene rs3851179 polymorphism contributes to Alzheimer’s disease in an Asian population. Neuromolecular Med. 15, 384–388. doi: 10.1007/s12017-013-8225-2
Liu, G., Zhang, L., Feng, R., Liao, M., Jiang, Y., Chen, Z., et al. (2013b). Lack of association between PICALM rs3851179 polymorphism and Alzheimer’s disease in Chinese population and APOEepsilon4-negative subgroup. Neurobiol. Aging 341310, e1319–e1310. doi: 10.1016/j.neurobiolaging.2012.08.015
Liu, G., Zhang, F., Jiang, Y., Hu, Y., Gong, Z., Liu, S., et al. (2017d). Integrating genome-wide association studies and gene expression data highlights dysregulated multiple sclerosis risk pathways. Mult. Scler. 23, 205–212. doi: 10.1177/1352458516649038
Liu, G., Zhang, F., Hu, Y., Jiang, Y., Gong, Z., Liu, S., et al. (2017c). Genetic variants and multiple sclerosis risk gene SLC9A9 expression in distinct human brain regions. Mol. Neurobiol. 54, 6820–6826. doi: 10.1007/s12035-016-0208-5
Liu, G., Zhang, Y., Wang, L., Xu, J., Chen, X., Bao, Y., et al. (2018c). Alzheimer’s disease rs11767557 variant regulates EPHA1 gene expression specifically in human whole blood. J. Alzheimers Dis. 61, 1077–1088. doi: 10.3233/JAD-170468
Liu, G., Zhao, Y., Jin, S., Hu, Y., Wang, T., Tian, R., et al. (2018d). Circulating vitamin E levels and Alzheimer’s disease: a Mendelian randomization study. Neurobiol. Aging 72, 189 e181–189 e189. doi: 10.1016/j.neurobiolaging.2018.08.008
Ma, J., Zhang, W., Tan, L., Wang, H. F., Wan, Y., Sun, F. R., et al. (2016). MS4A6A genotypes are associated with the atrophy rates of Alzheimer’s disease related brain structures. Oncotarget 7, 58779–58788. doi: 10.18632/oncotarget.9563
Moskvina, V., O’dushlaine, C., Purcell, S., Craddock, N., Holmans, P., O’donovan, M. C. (2011). Evaluation of an approximation method for assessment of overall significance of multiple-dependent tests in a genomewide association study. Genet. Epidemiol. 35, 861–866. doi: 10.1002/gepi.20636
Ramasamy, A., Trabzuni, D., Guelfi, S., Varghese, V., Smith, C., Walker, R., et al. (2014). Genetic variability in the regulation of gene expression in ten regions of the human brain. Nat. Neurosci. 17, 1418–1428. doi: 10.1038/nn.3801
Shen, N., Chen, B., Jiang, Y., Feng, R., Liao, M., Zhang, L., et al. (2015). An updated analysis with 85,939 samples confirms the association between CR1 rs6656401 polymorphism and Alzheimer’s disease. Mol. Neurobiol. 51, 1017–1023. doi: 10.1007/s12035-014-8761-2
Shtutman, M., Baig, M., Levina, E., Hurteau, G., Lim, C. U., Broude, E., et al. (2011). Tumor-specific silencing of COPZ2 gene encoding coatomer protein complex subunit zeta 2 renders tumor cells dependent on its paralogous gene COPZ1. Proc. Natl. Acad. Sci. U.S.A. 108, 12449–12454. doi: 10.1073/pnas.1103842108
Tan, C. C., Wan, Y., Tan, M. S., Zhang, W., Wang, Z. X., Sun, F. R., et al. (2016). Association of frontotemporal dementia GWAS loci with late-onset Alzheimer’s disease in a Northern Han Chinese population. J. Alzheimers Dis. 52, 43–50. doi: 10.3233/JAD-151073
Ulland, T. K., Colonna, M. (2018). TREM2—a key player in microglial biology and Alzheimer disease. Nat. Rev. Neurol. 14, 667–675. doi: 10.1038/s41582-018-0072-1
Võsa, U., Claringbould, A., Westra, H.-J., Bonder, M. J., Deelen, P., Zeng, B., et al. (2018). Unraveling the polygenic architecture of complex traits using blood eQTL meta analysis. bioRxiv. doi: 10.1101/447367
Van Cauwenberghe, C., Van Broeckhoven, C., Sleegers, K. (2016). The genetic landscape of Alzheimer disease: clinical implications and perspectives. Genet. Med. 18, 421–430. doi: 10.1038/gim.2015.117
Wan Nasri, W. N., Makpol, S., Mazlan, M., Tooyama, I., Wan Zurinah Wan Ngah, W. Z., Damanhuri, H. A. (2018). Tocotrienol rich fraction supplementation modulate brain hippocampal gene expression in APPswe/PS1dE9 Alzheimer’s disease mouse model. J. Alzheimers Dis. 70, s1, S239–S254 doi: 10.3233/JAD-180496
Ward, L. D., Kellis, M. (2012). HaploReg: a resource for exploring chromatin states, conservation, and regulatory motif alterations within sets of genetically linked variants. Nucleic Acids Res. 40, D930–D934. doi: 10.1093/nar/gkr917
Zhang, S., Li, X., Ma, G., Jiang, Y., Liao, M., Feng, R., et al. (2016b). CLU rs9331888 polymorphism contributes to Alzheimer’s disease susceptibility in Caucasian but not East Asian populations. Mol. Neurobiol. 53, 1446–1451. doi: 10.1007/s12035-015-9098-1
Zhang, Y., Wang, L., Jia, H., Liao, M., Chen, X., Xu, J., et al. (2018). Genetic variants regulate NR1H3 expression and contribute to multiple sclerosis risk. J. Neurol. Sci. 390, 162–165. doi: 10.1016/j.jns.2018.04.037
Zhang, Q. Y., Wang, H. F., Zheng, Z. J., Kong, L. L., Tan, M. S., Tan, C. C., et al. (2016a). FERMT2 rs17125944 polymorphism with Alzheimer’s disease risk: a replication and meta-analysis. Oncotarget 7, 39044–39050. doi: 10.18632/oncotarget.9679
Zhang, S., Zhang, D., Jiang, Y., Wu, L., Shang, H., Liu, J., et al. (2015). CLU rs2279590 polymorphism contributes to Alzheimer’s disease susceptibility in Caucasian and Asian populations. J. Neural Transm. (Vienna) 122, 433–439. doi: 10.1007/s00702-014-1260-9
Keywords: Alzheimer’s disease, COPI, genome-wide association studies, expression quantitative trait loci, gene-based test
Citation: Yang Y, Wang X, Ju W, Sun L and Zhang H (2019) Genetic and Expression Analysis of COPI Genes and Alzheimer’s Disease Susceptibility. Front. Genet. 10:866. doi: 10.3389/fgene.2019.00866
Received: 18 July 2019; Accepted: 19 August 2019;
Published: 19 September 2019.
Edited by:
Guiyou Liu, Tianjin Institute of Industrial Biotechnology (CAS), ChinaCopyright © 2019 Yang, Wang, Ju, Sun and Zhang. This is an open-access article distributed under the terms of the Creative Commons Attribution License (CC BY). The use, distribution or reproduction in other forums is permitted, provided the original author(s) and the copyright owner(s) are credited and that the original publication in this journal is cited, in accordance with accepted academic practice. No use, distribution or reproduction is permitted which does not comply with these terms.
*Correspondence: Li Sun, c2pua3N1bmxpQDEyNi5jb20=; Haining Zhang, aGFpbmluZzEzOUB5ZWFoLm5ldA==