- 1Facultad de Medicina, Universidad Nacional de Colombia, Bogotá, Colombia
- 2Departamento de Estadística, Grupo de Investigación en Bioinformática y Biología de sistemas – GiBBS, Facultad de Ciencias, Universidad Nacional de Colombia, Bogotá, Colombia
- 3Departamento de Medicina Interna, Hospital Universitario San Ignacio, Bogotá, Colombia
- 4Instituto de Genética Humana, Facultad de Medicina, Pontificia Universidad Javeriana, Bogotá, Colombia
Background: Epidemiological and clinical evidence points cancer comorbidity with pulmonary chronic disease. The acquisition of some hallmarks of cancer by cells affected with lung pathologies as a cell adaptive mechanism to a shear stress, suggests that could be associated with the establishment of tumoral processes.
Objective: To propose a bioinformatic pipeline for the identification of all deregulated genes and the transcriptional regulators (TFs) that are coexpressed during lung cancer establishment, and therefore could be important for the acquisition of the hallmarks of cancer.
Methods: Ten microarray datasets (six of lung cancer, four of lung diseases) comparing normal and diseases-related lung tissue were selected to identify hub differentiated expressed genes (DEGs) in common between lung pathologies and lung cancer, along with transcriptional regulators through the utilization of specialized libraries from R language. DAVID bioinformatics tool for gene enrichment analyses was used to identify genes with experimental evidence associated to tumoral processes and signaling pathways. Coexpression networks of DEGs and TFs in lung cancer establishment were created with Coexnet library, and a survival analysis of the main hub genes was made.
Results: Two hundred ten DEGs were identified in common between lung cancer and other lung diseases related to the acquisition of tumoral characteristics, which are coexpressed in a lung cancer network with TFs, suggesting that could be related to the establishment of the tumoral pathology in lung. The comparison of the coexpression networks of lung cancer and other lung diseases allowed the identification of common connectivity patterns (CCPs) with DEGs and TFs correlated to important tumoral processes and signaling pathways, that haven´t been studied to experimentally validate their role in the early stages of lung cancer. Some of the TFs identified showed a correlation between its expression levels and the survival of lung cancer patients.
Conclusion: Our findings indicate that lung diseases share genes with lung cancer which are coexpressed in lung cancer, and might be able to explain the epidemiological observations that point to direct and inverse comorbid associations between some chronic lung diseases and lung cancer and represent a complex transcriptomic scenario.
Introduction
Lung cancer is the leading cause of cancer death in men and women in the world (Siegel et al., 2015). Lung cancer is a malignant tumor of uncontrolled cell growth with the ability to metastasize in distant tissues (Dela Cruz et al., 2011). Lung cancer can be divided into two main histological types: non-small cell cancer (NSCLC), representing 85% of all lung cancers, and small cell cancer (SCLC) (Gridelli et al., 2015). The global cancer burden reported by GLOBOCAN 2018 and the International Agency for Research on Cancer estimates that there will be 18.1 million new cancer cases (Bray et al., 2018). Lung cancer is the most commonly diagnosed cancer (11.6% of total cases) and the leading cause of cancer death (18.4% of total deaths due to cancer), followed by breast cancer (11.6%), prostate cancer (7.1%) and colorectal cancer (6.1%) (Bray et al., 2018). Lung cancer is the most frequent cancer and the leading cause of cancer death in men. In women, breast cancer is the most frequently diagnosed cancer and the leading cause of cancer death, followed by colorectal and pulmonary cancer (IARC and Boyle, 2018). The increase in mortality rates during the last decades have been associated with a late diagnosis, which limits their potential treatment, and is evidence of the important lack of biomarkers for the development of specific treatments against the disease (Dela Cruz et al., 2011).
The interstitial lung diseases (ILDs) are a heterogenic group of conditions with clinic, radiologic, and functional manifestations, among which the most important are anatomopathological alterations that affect alveolointerstitial structures, like the epithelium, alveolar walls, capillary endothelium and connective tissue (perilymphatic and perivascular) between the septa and the peribronchial and peribronchiolar tissue (Raghu et al., 2018). Environmental factors (silica dust, asbestos fibers, grain dust, bird droppings, and animals), infections, medications (anti-inflammatory, antibiotics and cardiovascular), radiation exposure, and autoimmune, granulomatous, metabolic, systemic (connective tissue) and childhood-specific diseases are between the known associated causes (Raghu et al., 2004; Nathan et al., 2012; Griese et al., 2015). The ILDs can affect men and women of any age who are exposed to specific concentrations of any of the environmental factors, and/or are carriers of genetic and epigenetic factors that predispose them for the development of the disease (Coultas et al., 1994; Griese et al., 2015). The ILDs include a wide range of diffuse pulmonary disorders that often end in pulmonary fibrosis and may occur in isolation or associated with systemic diseases (Spagnolo et al., 2014; Kropski et al., 2015).
In recent decades, studies have shown a possible relationship between pathological processes in lung tissue and the development of cancer. Specifically, ILDs and lung cancer share pathogenic mechanisms such as inflammation, increased resistance to apoptosis, focal hypoxia, an increase in proliferation, viability and accumulation of cells such as fibroblasts, in regions with repeated epithelial lesions (Archontogeorgis et al., 2012). The epithelial mesenchymal transition (EMT) process characteristic of tumor cells and essential to carrying out metastasis processes, is a phenomenon that also occurs in epithelial type II alveolar cells that are transformed into mesenchymal cells to produce fibroblasts and myofibroblasts that directly contribute to the fibrotic event in ILDs (Willis and Borok, 2009). In lung diseases, epithelial cells exposure to matrix metalloproteinases can lead to an increase in reactive oxygen species levels that promote myofibroblasts differentiation (Sokai et al., 2015). The deregulation of metalloproteinases expression associated with a defective matrix and increase levels of reactive oxygen species are also characteristic of malignancy, suggesting a relationship between the two pathologies (Radisky et al., 2007). Several studies suggest a possible association between other lungs diseases and lung cancer, through different physiopathogenic mechanisms. The presence of common tumorigenic processes between lung cancer and other lung diseases suggests a possible causal association in the establishment of this tumoral pathology, and that the joint transcriptomic analysis can ultimately result in identifying potential novel biomarkers/drug targets for lung cancer early diagnosis, if it is associated with the risk.
Cancer Biomarkers are used in clinical practice for diagnosis, prognosis, the identification of sensitive patients, and the prediction of cancer patients response to treatment (Kamel and Al-Amodi, 2017). There are several types of biomarkers: Prognostic biomarkers are used to identify the patients that need treatment in the future, Predictive biomarkers direct patient’s treatment selection, and Diagnostic biomarkers contribute to the diagnosis and classification of the disease, and can be useful to monitor the therapeutic response in patients (Fenton et al., 2011). Cancer research has identified a significant number of predictive biomarkers for some types of cancer according to their clinical usefulness (Kamel and Al-Amodi, 2017). In lung cancer NSCLC have been identified some predictive biomarkers whose expression can predict the response to a specific treatment (BRCA1, TP53, and KRAS) (Kamel and Al-Amodi, 2017).
The identification of genetic risk factors, as mutations in somatic and germ cells (EGFR, TP53, KRAS, BRAF, ERBB2, MET, STK11, PIK3CA), gene amplifications (EGFR, ERBB2, MET, PIK3CA, and NKX2), deletions (DOK2) (Berger et al., 2010), and presence of fusion genes (ALK/EML4) (Soda et al., 2007), have allowed to develop specific treatments, but although they increase the susceptibility to develop lung cancer (Braun et al., 1994), they have not shown a concordance with the mortality rates because their limited ability to treat patients only with the associated risk factor (Blanchon et al., 2006). In the postgenomic era, the biomarker identification studies have begun to take into account two important components for clarifying the etiology of the tumoral diseases: First, the great inter and intratumoral variability at the molecular and cellular level in each individual and/or population of individuals; and second, the complexity of lung cancer, in terms of the huge number of deregulated genes that have been identified with genomic studies, which highlights the complexity of cancer and generates a problem in the development of therapeutic treatments for each possible variation, that must be solved.
Genomic studies such as microarrays and RNA-Seq have the ability to provide information for the identification of all groups of transcriptionally dysregulated genes involved in the modulation of biological functions and signaling pathways, describing expression patterns associated with tumor grade, differentiation state, metastatic potential, and patients’ survival, when comparing tumoral and healthy tissue (Wang and Liotta, 2011; Barretina et al., 2012; Eswaran et al., 2013; Li et al., 2017; Ma et al., 2019). Our research group believes that the combined bioinformatics analysis of a selected group of these studies can take advantage of all the transcriptomic knowledge generated with these technologies, finding potentially valuable information that could be applied to increase the understanding of cellular processes related to complex diseases such as cancer. This kind of analysis can help us to understand the complexity of the disease from a global perspective, through the identification of all deregulated genes that participate in the establishment of lung cancer. Additionally, the comparison of different pathologies when performing a global analysis of several databases, allow us to find specificities and common processes, which, when analyzing each study separately, cannot be detected.
The study of complex systems can be based on communication between signaling pathways in gene networks, and on the identification of prognosis modules in co-expression networks associated with a biological process, which can become good targets for pharmaceutical treatment. (Zhou et al., 2019). Currently, published studies look for cancer biomarkers associated with the hallmarks of cancer (Hanahan and Weinberg, 2011), based on the generation of co-expression networks using transcriptomic studies in different types of cancer (Yu et al., 2019b), through the weighted gene coexpression networks (WGCNA) method, which identified more than 3,000 coexpression modules associated with tumoral processes such as cell proliferation, extracellular matrix remodeling, hypoxia, inflammation, angiogenesis, and cell differentiation (Ivliev et al., 2016); with Gaussian mixing models (GMM) an important number of modules in a pairwise gene comparison to reduce extrinsic background noise in the co-expression network (Ficklin et al., 2017); and using a permutations test, the communities present in all networks have been identified, followed by an enrichment analysis to identify the associated biological processes, and a survival analysis to differentiate communities capable of differentiating cancer patients from healthy individuals (Yu et al., 2019a).
We propose a new methodology for the creation of co-expression networks, which was developed by Dr. López-Kleine research group, based on the use of the library “Coexnet” (Henao et al., 2018). This method allows the creation of co-expression networks from microarray data sets, the identification of multiple nodes connected in an intersection network in common when comparing two or several biological networks, which have been called common connectivity patterns (CCPs) (Henao et al., 2018). Then, Coexnet allows to create coexpresión networks of lung cancer and other lung diseases, to compare them and identify CCPs in common between pathologies, and TFs deregulated and coexpressed during the establishment of tumoral pathology in the lung. Therefore, we were able to visualize the complexity of lung cancer, as the previous methods, however, we also generate within the same bioinformatic pipeline a methodology to address the huge complexity of the disease, identifying the transcriptional regulators of all deregulated and coexpressed genes in lung cancer associated with the acquisition of the hallmarks of cancers, in order to take a reasonable number of genes as candidate biomarkers into the experimental validation phase, for the future the development of specific and personalized treatments of lung cancer.
Methods
Selection of Gene Expression Data
Ten sets of expression data (microarrays) were selected from the public repositories Gene Expression Omnibus (GEO) from NCBI and ArrayExpress from EBI. Six sets compare tumor tissue from patients with lung cancer and normal lung tissue, and the other four sets compare lung tissue affected with different lung diseases with normal lung tissue (Table 1). The analysis follows 3 stages: 1) Data Pre-processing (quality verification, filter, normalization, etc.) based on the methodology proposed by Leal et al. (2014) 2) Detection of differentially expressed genes (DEGs) (Oshlack et al., 2010), 3) Identification of transcription factors among DEGs detected. In the first stage, the quality of the data was evaluated in order to work with those that have acceptable experimental validity. In the second and third stage, an important number of DEGs associated with biological functions relevant to the oncogenic process were obtained from other lung diseases and lung cancer.
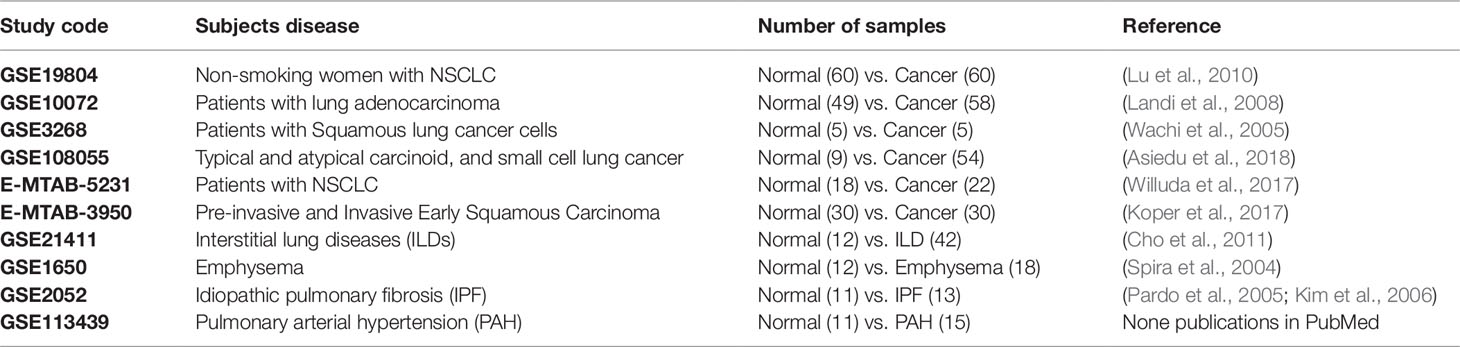
Table 1 Analyzed data sets, each study code, subjects’ related disease, number of samples per condition and reference.
Differentially Expressed Genes (DEGs) Analysis
Differentially expressed genes (DEGs) identification is based on finding if there is statistical evidence to declare that a gene is more or less active in a condition (“lung pathology”) with respect to a control (“normal lung”). R language (R Core Team, 2019) and specialized libraries were used to identify DEGs in every selected data set. First, pre-processing and quality control of each data set was performed through exploratory analysis of the data. This analysis consisted of evaluating the similar gene expression distribution per sample and examining that there were no genes with atypical expressions or samples that had a different behavior with respect to the others, through boxplots, density plots and summary statistics. Second, normalization was conducted in each data set in order to reduce data dispersion and make samples comparable each other using Variance Stabilizing Normalization (VSN) method using VSN library (Huber et al., 2002; Gautier et al., 2004), evaluating the similar gene expression distribution per sample in boxplots and density plots to use only statistically comparable data sets. When each data set passed pre-processing and normalization steps, it proceeded with the detection of DEGs.
The DEGs were selected based on the method of Significance analysis of microarray data (SAM) employing SAM function of siggenes package (Schwender, 2012), with the lowest error rate (q-value < 0.01), and under 15% FDR (Tibshirani et al., 2018). The q-value (Storey’s q-value) indicates the probability that a DEG identified is a false positive, corrected by multiple tests significance. This analysis was carried out for both lung cancer (LC) and other lung diseases (LD) data sets and the DEGs list in each data set was divided into positive and negative deregulated genes according to its fold change. Finally, all common genes between LC and other LD, varying in the same sense (over-expressed or repressed in the pathology with respect to healthy tissue) in the majority, 7 of the 10 sets analyzed, (in at least five of the six lung cancer sets, and one or two of other lung disease) were established as our “winner DEGs”. These were highlighted in the functional analysis, classifying those involved in tumorigenic processes.
Functional Annotation and Enrichment Analysis
The analysis of enrichment and functional annotation allows the identification of significant and specific biologic functional categories within our list of genes, considering the current scientific knowledge and the organism. This analysis was carried out with the online DAVID tool (Bioinformatics Resources 6.8, NIAID/NIH” (https://david.ncifcrf.gov/summary.jsp) (Dennis et al., 2003), which associates the winner DEGs found with specific biological functions. The analysis of associated diseases and functional categories for winning genes positively or negatively deregulated in the majority of sets of lung cancer and in other lung diseases, allow us to identify the DEGs with greater scientific evidence associated with oncogenic processes. The P value was corrected (because of multiple tests analyses) with Benjamini’s method (Benjamini and Hochberg, 1995), and it was considered a significant enrichment for functional categories P values ≤0.05. An extensive literature search in the common overregulated winner DEGs related to cancer was preformed to validated its association with lung cancer and other lung diseases.
Coexpression Network Analysis
The web-based system oPOSSUM (http://opossum.cisreg.ca/cgi-bin/oPOSSUM3/opossum_human_ssa) was used to detect over-represented conserved transcription factor binding sites and binding site combinations in the 45 overexpressed genes related to cancer according to DAVIDs analysis and verified with literature searching. The common 45 DEGs and the 45 TF (90 genes in total) were used to construct a gene co-expression network, a heatmap and a corrplot. The normalized expression profiles of the 59 of the 77 genes of interest were extracted from the gene expression data set GSE19804 and a similarity matrix was constructed using the absolute value of Pearson correlation. On this matrix, the threshold was identified to establish a final edge on the co-expression network using the methodology of Leal et al. (2014), based on the comparison of the obtained clustering coefficient with the clustering coefficient of a random graph (Leal et al., 2014).
The R library “Coexnet” was used to construct coexpression networks of one lung cancer data set (GSE19804) and the four sets of other lung diseases (GSE21411, GSE1650, GSE2052, GSE113439). Coexnet compared each lung disease network with the lung cancer network in order to identify common connectivity patterns (CCPs) between them, allowing the identification of molecular components linked together and common in the biological networks (Henao et al., 2018). CCPs networks analysis with Cytoscape (Shannon et al., 2003) and iRegulon application allowed the identification of transcription factors or master regulators of every CCP identified (Janky et al., 2014).
Survival Analysis of Transcription Factors
The effect of the expression of the hub genes or TFs identified with the transcriptomic, enrichment and coexpression analyses, in the survival of patients with lung cancer was assessed with the Kaplan Meier-plotter tool (KM plotter: http://kmplot.com/analysis/index.php?p=service&cancer=lung) (Gyorffy et al., 2013). KM plotter information about overall surviving (OS) is calculated and plotted with datasets from GEO, EGA and TCGA, based on hazard ratio (HR) of 95% confidence intervals and with a log rank P-value (LogrankP-value) associated.
Results
Differentially Expressed Genes (DEGs) in Common Between Lung Cancer and Other Lung Diseases
In general, there is a greater number of DEGs in lung cancer than in other lung diseases, and a greater number of overregulated genes than downregulated genes in both groups (Figure 1). However, there is a high number of deregulated genes in Pulmonary arterial hypertension (PAH) dataset equivalent to lung cancer datasets. The identification of 395 genes that were differentially expressed in lung cancer and other lung diseases in at least seven sets of the 10 data sets analyzed, indicated that the joint microarray data sets analysis allows us to find a characteristic metafirm of lung diseases establishment processes at molecular level. 210 of the DEGs were equally deregulated in both, lung cancer and other lung diseases, among which 116 were overregulated and 94 downregulated (Supplementary Table 1).
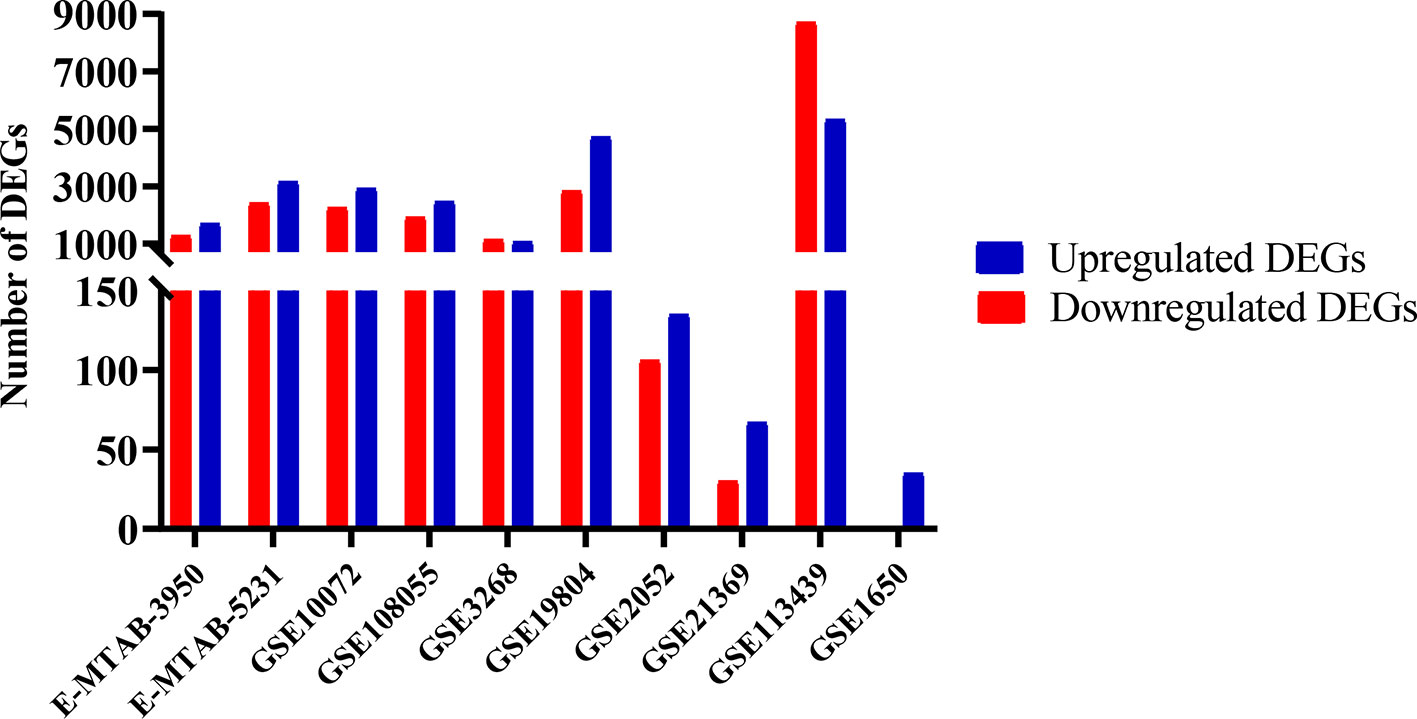
Figure 1 Differentially expressed genes (DEGs) in lung cancer and other lung diseases studies. The image was made using GraphPad Prism version 8.00 for Windows, GraphPad Software, La Jolla California USA, www.graphpad.com.
Functional Annotation and Enrichment Analysis
The downregulated “winner” DEGs are associated with angiogenesis, cell adhesion and the negative regulation of transcription from RNA polymerase II promoter (Figure 2). The overregulated “winner” DEGs are associated with several cell cycle processes (Figure 3), DNA replication, mismatch repair, and p53 signaling pathways (Figure 4). The functional annotation analysis of the 116 overregulated common genes identified 45 genes associated with cancer with a significant corrected P value.
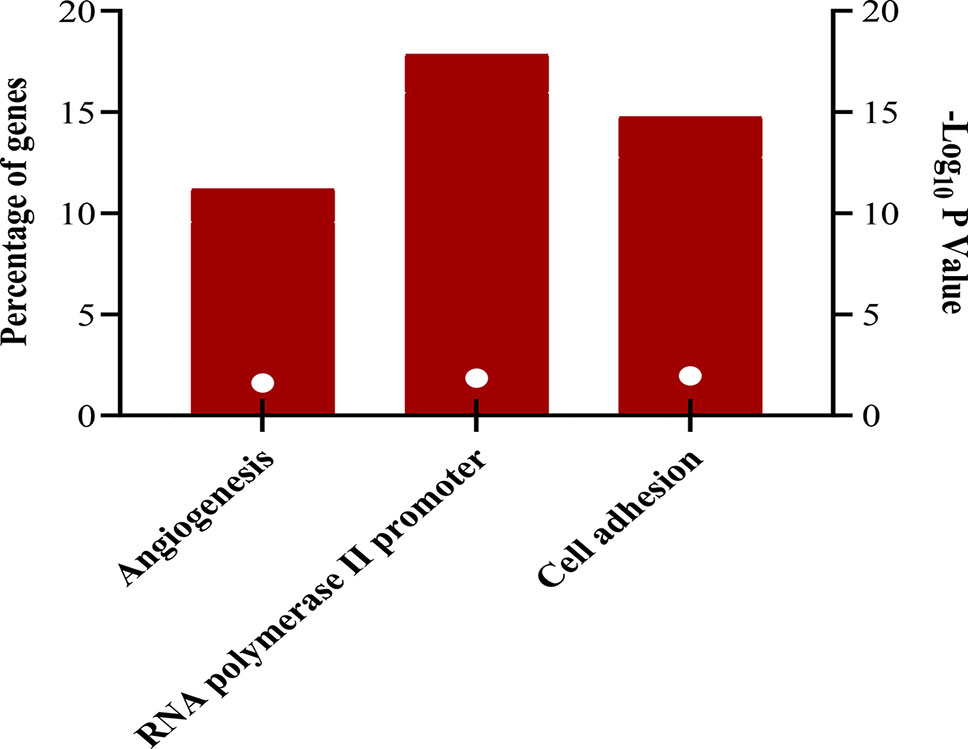
Figure 2 Biological processes associated to downregulated genes common in lung cancer and other lung diseases. The image was made using GraphPad Prism version 8.00 for Windows, GraphPad Software, La Jolla California USA, www.graphpad.com.
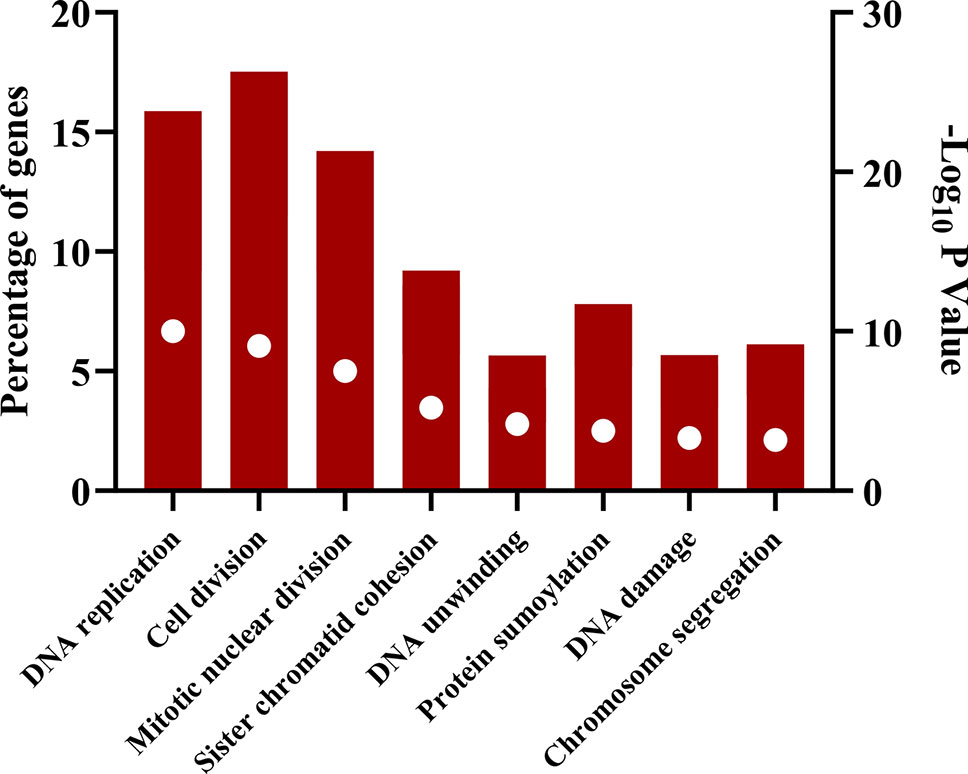
Figure 3 Biological processes associated with common overregulated genes in lung cancer and other lung diseases. The image was made using GraphPad Prism version 8.00 for Windows, GraphPad Software, La Jolla California USA, www.graphpad.com.
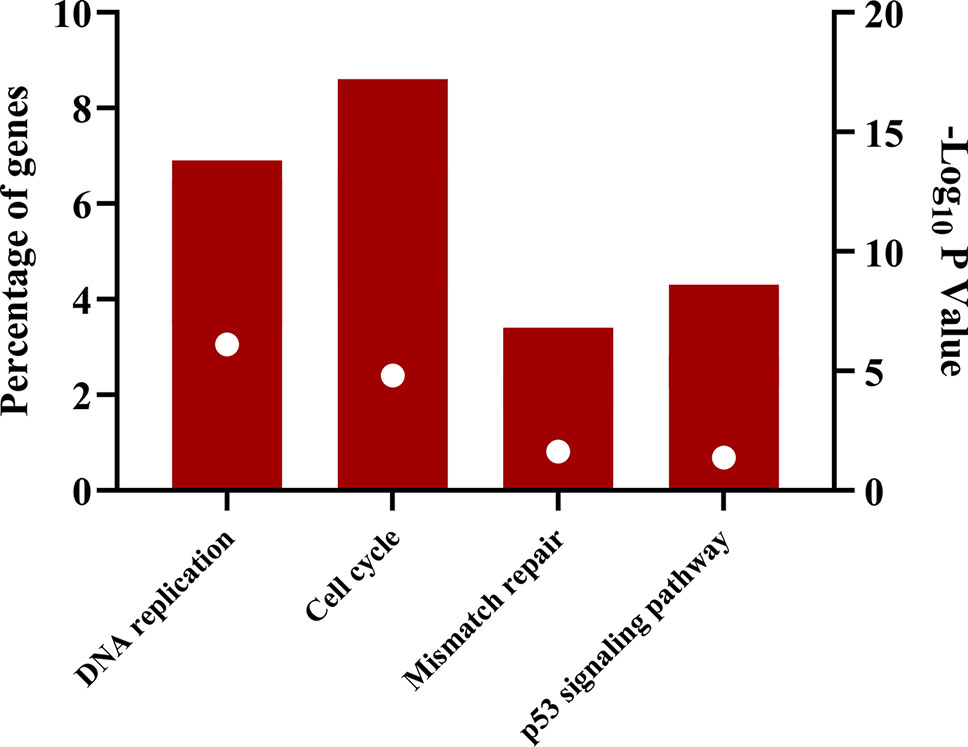
Figure 4 Signaling pathways associated with common overregulated genes in lung cancer and other lung diseases. The image was made using GraphPad Prism version 8.00 for Windows, GraphPad Software, La Jolla California USA, www.graphpad.com.
Coexpression Network Analysis
The heatmap and corrplot show The coexpression network created with the 45 DEGs associated with cancer in DAVIDs enrichment analysis and the 45 TFs identified with Opossum as their possible regulators, has 35 DEGs coexpressed with four TFs (YY1, ZEB1, E2F1, and NR4A2) (Figure 5) (Shannon et al., 2003). The heatmap and corrplot show the correlation between all the genes used to create the network (Figures 6 and 7). YY1 is overregulated in three lung cancer datasets and downregulated in PAH dataset. NR4A2 is downregulated in five lung cancer datasets and overregulated in PAH dataset. ZEB1 is downregulated in four lung cancer and ILDs datasets, and overregulated in PAH. E2F1 is only overexpressed in five lung cancer datasets. The extensive literature search associates most of the coexpression network genes with lung cancer, and most of them with other lung diseases (Supplementary Table 2). 36 genes of the coexpression network were deregulated in PAH, 12 genes in IPF, 1 in ILDs, and 1 in emphysema dataset, but none was reported or analyzed in their specific lung disease study.
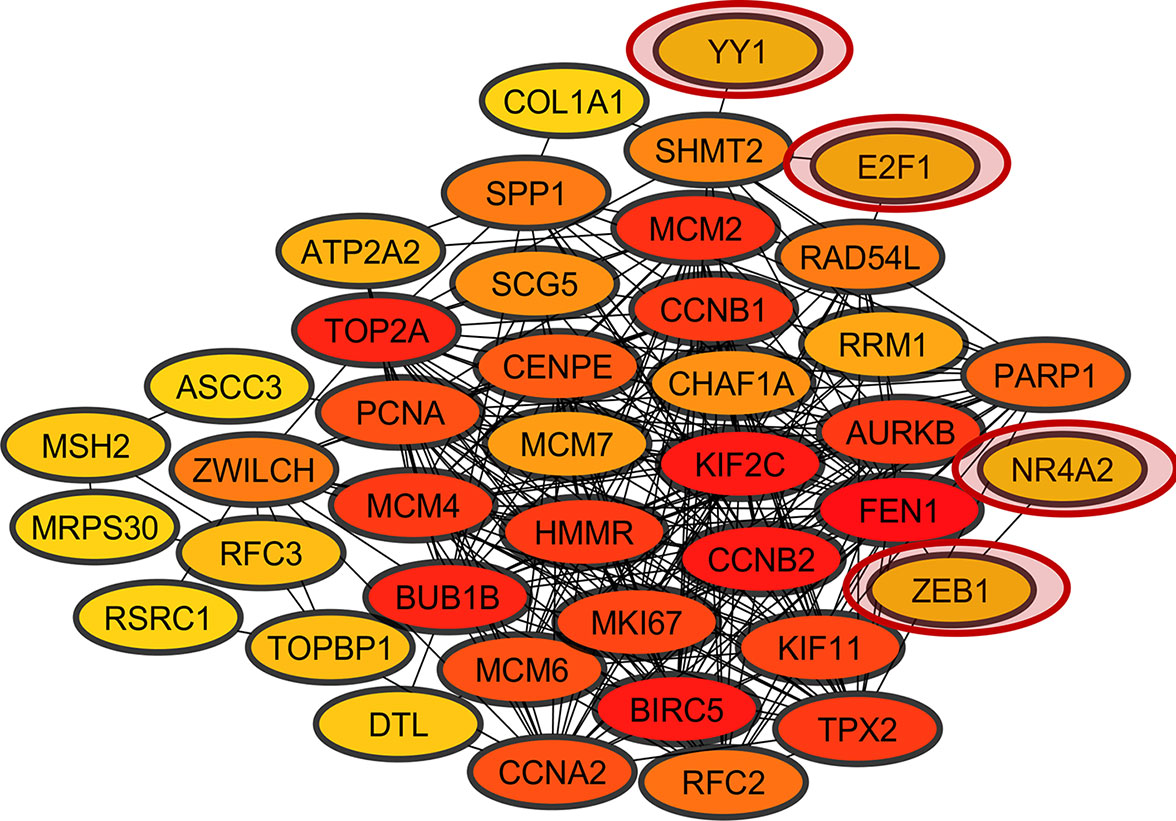
Figure 5 Coexpression network of overregulated genes common in lung cancer and other lung diseases (LC&LD coexpression network). The red circles show the TFs coexpressed in the network.
Furthermore, the coexpression network genes were related with the seven of the 10 Hallmarks of Cancer proposed by Hanahan and Weinberg (2011) (Figure 8). NR4A2 and E2F1 function have been related with resisting to cell death and sustaining a proliferative signal processes in lung cancer. Meanwhile, YY1 and ZEB1 function have been related with the activation of invasion and metastasis processes (Figure 8). Most of the coexpression network genes carry out their function in the nucleus, some in the endoplasmic reticulum and the cytoskeleton. Additionally, the functional annotation of the coexpressed genes of the network showed an important association with cancer and the same signaling pathways of the overregulated genes in common between lung cancer and other lung diseases, with significant P values.
The library Coexnet was able to find a CCP in every comparison between the coexpression networks of the lung cancer study and the other lung diseases studies. The CCP of lung cancer and ILDs has 15 nodes and 99 edges, the CCP of lung cancer with emphysema has 185 nodes and 15,954 edges, the CCP of lung cancer and IPF has 353 nodes and 60,696 edges, and the CCP of lung cancer and PAH has 3,389 nodes and 5,537,212 edges. DAVIDs analysis of the genes in every CCP showed important associations of LC-IPF CCP with biological processes related with the acquisition of tumoral characteristics, and the LC-PAH CCP with deregulation in metabolism and signaling pathways related to inflammatory processes in cancer. The Cytoscape network analysis showed that the CCPs are highly interconnected (Supplementary Table 3), and highly associated with important signaling pathways deregulated in cancer, and regulated by specific TFs found in our joint transcriptomic analysis.
Survival Analysis of Transcription Factors
The KM plotter analysis for the transcription factors identified during the present study revealed a statistically significant association between the expression levels of YY1 and NR4A2 with the survival of lung cancer patients (Figure 9) (Gyorffy et al., 2013). The multivariate analysis showed association of some of the transcription factors expression with the stage, and smoking history of lung cancer patients (Table 2).
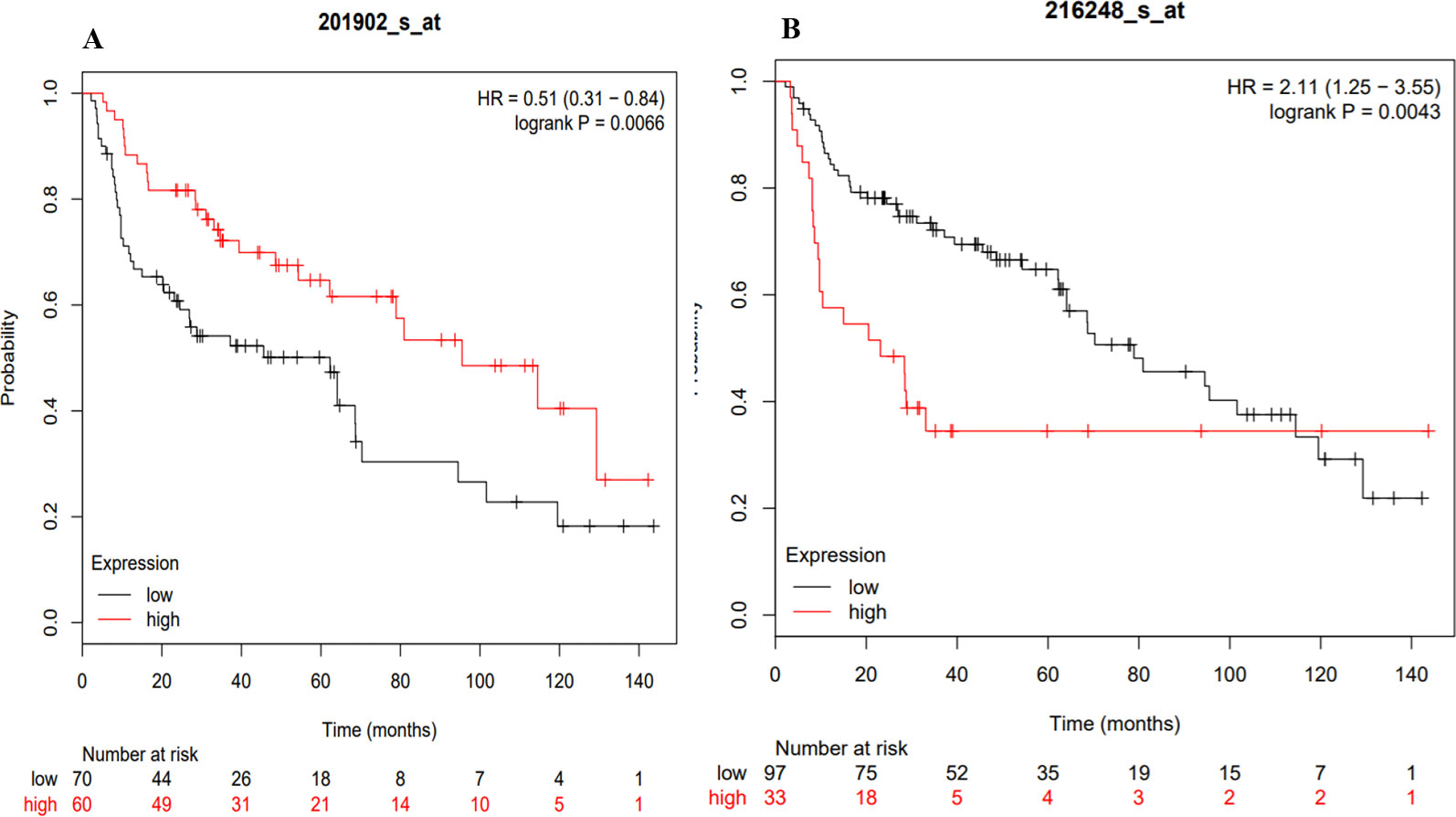
Figure 9 KM plots of the TFs associated with the survival of lung cancer patients. (A) YY1, (B) NR4A2.
Discussion
Recent cancer studies have shown that it manifests as abnormal expression patterns of a significant number of genes, allowing the identification of more than 450 genetic variations through extensive GWAS association analysis (Stadler et al., 2010). The main genetic biomarkers identified have been associated with the increased risk of developing cancer (Sud et al., 2017). However, pharmaceutical gene expression regulation has not contributed significantly to stop and/or reverse tumor expansion in all patients affected with the tumoral pathology in the lung (Das et al., 2015). The great complexity of cancer etiology represented in the significant number of dysregulated genes identified, generates an intricate problem for therapeutic treatments development since it cannot be directed to each and every one of them (Gridelli et al., 2015). Moreover, until now, no biomarker has shown the ability to characterize the pathology, always reversing the tumor process in all patients affected.
Currently, neoplasms research has highlighted the ability of transcription factors (TFs) to regulate the expression of a large number of genes, which participate as important terminal regulators, being points of convergence in oncogenic signaling pathways; therefore, becoming promising biomarkers for cancer therapies (Yeh et al., 2013). TFs are important for cell differentiation during embryogenesis, but when they are deregulated in adult tissue abnormally activate signaling pathways related to tumor phenotype acquisition (Cameron and Neil, 2004). The LC&LD coexpression network analysis created with the DEGs identified with DAVIDs-Opossum analysis allowed the identification of 4 TFs capable to regulate and important number of the 35 DEGs found in common between lung cancer and other lung diseases (Figure 5). LC&LD network represent the genes with strongest experimental evidence about its association with tumorigenic processes, that could be associated with early stages of lung cancer, as they are also deregulated in other lung diseases. On the other hand, the CCPs found in common between lung cancer and other lung diseases, comparing lung cancer (LC) and other lung diseases (LD) coexpression networks represent another approximation that allows to find new hub genes that could be even more important for lung cancer establishment than those previously studied, because these are highly and equally connected and deregulated genes coexpressed in lung cancer and other lung diseases. For every CCP a transcription factor that regulates and important number of genes in the subnetwork was identified.
The four TFs (YY1, ZEB1, NR4A2 and E2F1) found coexpressed and identified with the DAVID-Opossum analysis have evidence of its association with tumorigenic processes, that could relate them to establishment of lung cancer. YY1 is a highly multifunctional transcription factor that promotes cell proliferation and invasion in lung cancer cells (Huang et al., 2017), and EMT in H1155 cells (Gao et al., 2018). YY1 has been associated with a regulatory loop with cancer stem cell transcription factors (SOX2, OCT4, BMI1) during the cross-talk between the NF-kB/PI3K/AKT pathways (Kaufhold et al., 2016). Therefore, YY1 overexpression in lung cancer might suggest an association of these transcription factor in driving tumorigenic processes and the acquisition of stem-like characteristics in early stages of lung cancer. YY1 is directly connected with SHMT2, a gene that support tumorigenesis (Mattaini et al., 2016), stimulates proliferation of c-Myc deficient cells (Cooper et al., 2003) and increases lung tumors growth (Zhang et al., 2012; Lee et al., 2014; DeNicola et al., 2015; Zhang et al., 2017). SHMT2 is overexpressed in all LC datasets, IPF and PAH datasets.
ZEB1 represses E-cadherin promoter, induces EMT by recruiting SMARCA4/BRG1 (Sánchez-Tilló et al., 2010), and promotes tumorigenicity by repressing stemness-inhibiting microRNAs (Wellner et al., 2009). ZEB1 expression has been related to early stage IB of NSCLC, tumor-node-metastasis stage, and EMT (Larsen et al., 2016). ZEB1 also has a tumor suppressive role, independent of its ability to induce EMT, given that ZEB1 is able to induce EMT in both KRAS- and EGFR mutant cell lines (Yochum et al., 2009). Therefore, ZEB1 downregulation in lung cancer could be related to its ability to inhibit tumor growth, which should be important to maintain in early stages of lung cancer. ZEB1 interacts with FEN1 and NR4A2, the third transcription factor coexpressed in LC&LD coexpression network. FEN1 is an enzyme that removes the 5 ‘ends during DNA repair, processes the 5’ ends of the Okazaki fragments during DNA replication (Tishkoff et al., 1997). FEN1 is an endonuclease XPG/RAD2 essential for DNA replication and the protection of the genome against mutations (Gordenin et al., 1997). FEN1 promotes tumor progression (He et al., 2017a), proliferation and poor prognosis of NSCLC (Zhang et al., 2018a). FEN1 is overexpressed in all LC datasets and PAH dataset. YY1 and ZEB1 also appeared as the regulators of the CCP formed between LC and Emphysema, giving another layer of evidence of their importance in the establishment of LC in patients with an emphysema antecedent.
NR4A2 transcriptionally regulates cell proliferation, apoptosis, inflammation, neuronal development, and carcinogenesis (Safe et al., 2013; Safe et al., 2016). NR4A2 overexpression blocks p53 target genes activation, like mir-34a, which rescues cells from p53-induced inhibition of proliferation (Beard et al., 2016). NR4A2 is downregulated in lung cancer and overexpressed in PAH dataset, suggesting an association with tumoral processes during early stages of lung cancer related to this lung disease. NR4A2 only interacts with ZEB1, the second transcription coexpressed in the network, which might suggest a possible coregulatory relationship between these two transcription factors and the association with the same tumorigenic processes related with ZEB1.
E2F1 is a transcription activator overexpressed in lung adenocarcinoma and squamous cell lung carcinoma tissues, associated with cellular proliferation by counteracting the negative effects of cyclin-cdk inhibitors (Eymin et al., 2001). ANKRD22 promotes progression of NSCLC through transcriptional up-regulation of E2F1 (Yin et al., 2017). E2F1 was one the transcription factor that Opossum selected as possible regulator of the 45 common genes overregulated and related to cancer, and appeared in the coexpression network, even when it is not deregulated in the selected sets of other lung diseases, probably suggesting its importance at the end of early stages of lung cancer. Moreover, E2F1 was the TF identified as the most important regulator with iRegulon for the LC&LD coexpression network, in terms of enrichment if its TFBS, and the number of network targets that can regulate. E2F1 interacts with SHMT2 like YY1, and RAD54L. RAD54L is a recombinational DNA repair protein, and a double-stranded DNA-dependent ATPase that stimulates homologous recombination, inducing a DNA topological changes and induces DNA repair of DNA double-strand breaks (Swagemakers et al., 1998; Ristic et al., 2002; Sigurdsson et al., 2002). RAD54L is upregulated in NSCLC and associated with dsDNA break repair (Välk et al., 2011). RAD54L is overexpressed in all lung cancer datasets and PAH dataset.
The CCP formed between LC and ILD is regulated by IRF1, a transcriptional regulator of cellular responses, during hematopoiesis, inflammation, immune responses, cell proliferation and differentiation (Romeo et al., 2002). IRF1 regulation is associated with cell cycle, induction of growth arrest and programmed cell death following DNA damage (Bowie et al., 2008). IRF1 is downregulated in lung cancer, IPF and PAH datasets, probably because it represses the expression of genes involved in anti-proliferative response, such as BIRC5/survivin, CCNB1, CCNE1, CDK1, CDK2 and CDK4 (Armstrong et al., 2012) and in immune response, such as FOXP3, IL4, ANXA2 and TLR4 (Fragale et al., 2014). IRF1 stimulates p53/TP53-dependent transcription, improving EP300 recruitment which leads to an increase of p53/TP53 acetylation (Dornan et al., 2004). IRF1 is also known as tumor suppressor, capable to avoid tumor cell growth (Bouker et al., 2005), and stimulate an immune response against tumor cells (Tanaka et al., 1996).
The CCP formed between LC and IPF is regulated by NF1, a Ras‐GTPase activating protein, which function as negative regulators for Ras proteins (Ballester et al., 1990), preventing the downstream activation of Ras effector pathways, MAPK and PI3K/Akt/mTOR pathways that drive the pro-proliferation, survival, differentiation (Xu et al., 1990; Bollag and McCormick, 1991), cell adhesion, and migration (Kweh et al., 2009). NF1 is a mutated tumor suppressor in both of the NSCLC subtypes, adenocarcinoma (Collisson et al., 2014) and squamous cell (Hammerman et al., 2012). Lung cancers with NF1 mutations have also been characterized by downstream activation of Ras signaling (Redig et al., 2016). Therefore, there is evidence that suggest that NF1 mutations in lung cancer con also activate Ras signaling pathway, playing a key role in the incorrect signal transduction, proliferation and malignant transformation (Reuter et al., 2000). The CCP formed between LC and PAH is regulated by BRCA1, a E3 ubiquitin-protein ligase that mediates ‘Lys-6’-linked polyubiquitin chains formation, enabling cellular responses to DNA damage during DNA repair (Lee et al., 2000; Wang et al., 2007). BRCA1 has a tumor suppressor function related to the transcriptional regulation to maintain genomic stability, which is directly related to hereditary breast cancer (Li and Greenberg, 2012; Sedic et al., 2015). However, BRCA1 is overregulated in LC and PAH, and it expression has been related to the occurrence and development processes of NSCLC (Sun et al., 2018b).
The probability of surviving was associated with the expression of two transcription factors (YY1 and NR4A2) (Figure 9) identified with the coexpression analysis. Moreover, the Kaplan–Meier Multivariate analysis also associate their expression with stage, gender and smoking history. Therefore, the clinical history of patients could help in the future to prevent or apply most accurate treatments to lung cancer patients. At the moment, the clinical data highlights that risk of developing lung cancer is 1.5 times higher in patients with ILD compared with COPD and with the general population (Jung et al., 2011), while lung cancer prevalence in IPF patients can reach 48% (Le Jeune et al., 2007).
Most of the genes in the LC&LD coexpression network are deregulated in PAH and some in IPF, and only one in ILDs and Emphysema (Supplementary Table 2). The pathogenesis of PAH has been associated with genetic factors that confer a predisposition for development or progression, such as mutations of the bone morphogenic receptor type II gene (BMPR2), a member of the transforming growth factor superfamily TGF-β (Newman et al., 2001), mutations in the activin A receptor type II 1 (ACVRL1) (Girerd et al., 2010), endoglin (ENG) (Chaouat et al., 2004), SMAD9, caveolin-1 (CAV1) and KCNK3 (Ma et al., 2013). The majority of BMPR2 mutations result in a loss of function, but show low penetrance (∼20%) in more than 70% of patients with familial PAH and in 11–40% of patients with sporadic PAH, affecting only 20% of carriers during their lifetime (Lane et al., 2000; Machado et al., 2001). However, BMPR2 is one of the “guardians” of pulmonary vessels homeostasis during repair processes, controlling both apoptosis and cell proliferation of pulmonary vascular smooth muscle and endothelial cells (Morrell, 2006). Therefore, epigenetic studies have begun to study the regulatory mechanisms associated with BMP signaling in abnormal BMPR2 function, without necessarily implying a mutation in the BMPR2 gene, such as, for example, the importance of BMP/TGF-β signaling in maintaining normal pulmonary arteriolar structure (Trembath et al., 2001; Nasim et al., 2011), STAT3/miRNA-17-92 axis activation in normal human lung endothelial cells after interleukin (IL) -6 exposure (Brock et al., 2009), leads to the development of PAH (Steiner et al., 2009), and angiobliterant vascular remodeling and robust hypertrophy of the right ventricle (Gomez-Arroyo et al., 2012). Overexpression of miR-17 increases the proliferation of smooth muscle cells of human pulmonary arteries, and its inhibition improves pulmonary and cardiac function (Pullamsetti et al., 2012). The miR-204 is negatively regulated in the smooth muscle cells of the pulmonary artery isolated from patients with PAH, and an apoptosis-resistant phenotype in smooth muscle cells is associated with induction (Courboulin et al., 2011).
Patients with pulmonary arterial hypertension (PAH) have a sustained elevation of resistance and pulmonary arterial pressure, which makes them increasingly narrow until they are completely blocked (Montani et al., 2013), leading to the pumping of blood by the heart weakens the muscles until they reach heart failure through the right ventricle (Bogaard et al., 2009). Likewise, PAH is characterized by vascular remodeling in the three layers (intimate, middle and adventitia) of the wall of small and medium pulmonary arterioles (<500 µm), in response to continuous vascular lesions that lead to abnormal muscle muscularization of distal and medial capillary arteries, loss of precapillary arteries, and thickening of arterial walls (Guignabert and Dorfmuller, 2013). PAH begins to develop under constant stress conditions such as inflammation and pseudo-hypoxia, initially inducing the death of endothelial cells (Guignabert and Dorfmuller, 2013). However, cells eventually acquire hyperproliferative abilities (Lee et al., 1998), associated with instability of short sequences of microsatellite DNA within plexiform lesions (Yeager et al., 2001), and the presence of somatic chromosomal abnormalities in the lung patients with PAH (Aldred et al., 2010); a deregulated cell metabolism (Xu et al., 2007; Aldred et al., 2010; Sutendra et al., 2010; Tuder et al., 2012), with reduced oxygen consumption, reduced mitochondrial respiration and increased glycolytic metabolism (Xu et al., 2007); and the ability to evade apoptosis (Masri et al., 2007), with an increase in the expression of important anti-apoptotic genes such as Bcl-xL, Bcl-2 and survivin (McMurtry et al., 2005; Tu et al., 2012); in clones of fibroblasts, smooth muscle cells (PASMC) and endothelial cells of the pulmonary arteries (PAEC), which allows vascular remodeling during PAH (Guignabert et al., 2013).
The hypothesis of establishment or “quasi-malignancy” is also associated with the concept of an angiogenic/stem cell tumor (CSC) niche (Rai et al., 2008). Precursor cells can divide and migrate to pulmonary vascular adventitia through the vasa vasorum (Ergün et al., 2011; Yeager et al., 2011), and stem cells of small microvascular pulmonary endothelial cells of small arteries can proliferate to the point of obliteration (Alvarez et al., 2008). The pathobiological model of intraluminal angioproliferation is based on the growth of endothelial cells in PAH, where the “endothelial cell monolayer law” has been broken in favor of a tumor-like intraluminal growth (Tuder et al., 2001; Sakao et al., 2005). The endothelial cell such as the cell that initiates a complex lesion can come from three possible sources of proliferating endothelial cells: (1) An endothelial cell similar to a stem cell that is resistant to apoptosis and grows after neighboring monolayer cells intimate have been injured and have died (Yoder et al., 2007), (2) A precursor cell derived from the bone marrow (Sahara et al., 2007), and (3) An endothelial cell derived from the vascular endothelial growth factor (VEGF) action in dendritic cell-directed transdifferentiation (Steinman and Banchereau, 2007). The complex vascular lesions of PAH are governed by some, but not all, cancer characteristics. Therefore, although the lesions are not completely tumor, they are certainly neoplasms in the sense that there is a process of abnormal and uncontrolled cell growth.
Pulmonary arterial hypertension (PAH) showed great complexity as did cancer, something that could be evidenced by the large number of DEGs, comparable to those observed in lung cancer, which may be partly explained by PAH is so difficult to treat. Vasodilator-based treatments have not demonstrated the ability to reverse vascular remodeling and do not affect survival (Lajoie et al., 2016), but the acquisition of cancer characteristics in PAH-associated cells suggests the potential use of anticancer drugs in patients with PAH, such as imatinib, a tyrosine kinase inhibitor, which could potentially be beneficial in patients with PAH after evaluation of potential side effects for safe use in clinical practice (Hoeper et al., 2013).
Conclusion
In the present work, we show how the re-analysis of gene expression data available in the databases within an organized and focused bioinformatic pipeline can be used to obtain new knowledge and give more evidence that supports a biological hypothesis, as well as find potential biomarkers associated with molecular functions in common for different diseases. The methodology we propose for the identification of the transcriptional regulators of all deregulated genes related to the acquisition of the hallmarks of cancer during lung tumorogénesis, allowed us to truly visualize the massive complexity of lung cancer. The transcriptomic analyzes of multiple gene expression data and the creation and comparison of coexpression networks allowed to identify all the genes involved and the molecular events associated with the lung tumoral process, and the transcription factors or regulators of all these genes that can participate in the establishment of the disease, as biomarkers, which will lead in the future to establish in each patient the specific molecular event that causes the pathology, and to implement an effective personalized therapy against lung cancer.
The existence of common DEGs among different lung pathologies strengthens the experimental evidence that supports the hypothesis about a possible causal relationship between different lung diseases and lung cancer, because the deregulated biological functions in other lung diseases are related with oncogenic processes, and may be the first functions affected and those necessary for the establishment of a tumor pathology. Therefore, this group of genes can be more specific for the early stages of lung cancer, and might be more useful for early detection, and eventually could be integrated into the clinical practice to increase early detection probability of lung cancer, and used in the development of personalized therapies. The TFs coexpressed in the LC&LD network and the CCPs LC–LD have experimental evidence of their association with the acquisition of hallmarks of cancer during its establishment that must be validated experimentally. Common DEGs and TFs among lung pathologies might have the potential to be used as possible biomarkers for diagnosis and treatment that should be evaluated experimentally in patients, to demonstrate that its deregulation is crucial for the initiation and establishment of the tumor process in the lung, and therefore, the regulation of its expression is sufficient to prevent, control and revert the tumor process in a more efficient and specific way in every patient.
The levels of expression of the TFs associated with the establishment of lung according to our joint transcriptomic and coexpression analysis must be evaluated experimentally, along with the expression of the winner DEGs coexpressed in lung cancer. Functional analysis must be performed for every TF and winner DEGs to establish the association with the acquisition of which hallmark of cancer are related. Moreover, all functional and direct targets of every TF identified in lung cancer must be identified to fully understand their regulatory function during early stages of the disease. Also, the TFs identified as the key regulators for its ability to control and reverse the tumor process must be studied in depth, to find the regulatory mechanisms associated with their expression in lung cancer, like histone modifications, DNA methylation and non-coding RNAs, as the basis to develop specific and effective techniques of early detection and treatments against the tumoral pathology of the lung.
The joint transcriptomic laid the foundation to guide the research field of our lab providing potential candidate biomarkers that must be experimentally validated within the all regulatory transcriptional network of lung cancer, to become prognostic, diagnostic, and predictor biomarkers of lung cancer, which in the future will also guide the clinician process to establish the specific molecular event that causes a tumoral pathology in each patient, and to implement an effective personalized therapy against the disease.
Data Availability Statement
All microarray data sets are available for the scientific community, in the Gene Expression Omnibus (GEO) and ArrayExpress.
Author Contributions
LL-K proposed the combined analysis to identify common genes between pathologies, the step-by-step methodology applied and led the process of data analysis. MF did the data pre-processing and the detection of differentially expressed genes (DEGs) using specific libraries in R. BO-O did the functional/enrichment analysis, the literature searching, the coexpression network and survival analysis, and contribute with the manuscript writing. DU contributed with the literature searching of other lung diseases. AR and AA contributed to the study conception and design, leading the results interpretation and making important contributions to the Discussion section. All authors critically reviewed and approved final manuscript and agree to be accountable for all aspects of the work.
Funding
The current article was funded by the grant number 41492 from Universidad Nacional de Colombia, and PUJ ID7687 from Pontificia Universidad Javeriana.
Conflict of Interest
The authors declare that the research was conducted in the absence of any commercial or financial relationships that could be construed as a potential conflict of interest.
Supplementary Material
The Supplementary Material for this article can be found online at: https://www.frontiersin.org/articles/10.3389/fgene.2019.01260/full#supplementary-material
References
Aldred, M. A., Comhair, S. A., Varella-Garcia, M., Asosingh, K., Xu, W., Noon, G. P., et al. (2010). Somatic chromosome abnormalities in the lungs of patients with pulmonary arterial hypertension. Am. J. Respir. Crit. Care Med. 182, 1153–1160. doi: 10.1164/rccm.201003-0491OC
Alvarez, D. F., Huang, L., King, J. A., Elzarrad, M. K., Yoder, M. C., Stevens, T. (2008). Lung microvascular endothelium is enriched with progenitor cells that exhibit vasculogenic capacity. Am. J. Physiol.-Lung Cell. Mol. Physiol. 294, L419–L430. doi: 10.1152/ajplung.00314.2007
Archontogeorgis, K., Steiropoulos, P., Tzouvelekis, A., Nena, E., Bouros, D. (2012). Lung cancer and interstitial lung diseases: a systematic review. Pulm. Med. 2012, 1–11. doi: 10.1155/2012/315918
Armstrong, M. J., Stang, M. T., Liu, Y., Gao, J., Ren, B., Zuckerbraun, B. S., et al. (2012). Interferon Regulatory Factor 1 (IRF-1) induces p21 WAF1/CIP1 dependent cell cycle arrest and p21 WAF1/CIP1 independent modulation of survivin in cancer cells. Cancer Lett. 319, 56–65. doi: 10.1016/j.canlet.2011.12.027
Asiedu, M. K., Thomas, C. F., Dong, J., Schulte, S. C., Khadka, P., Sun, Z., et al. (2018). Pathways impacted by genomic alterations in pulmonary carcinoid tumors. Clin. Cancer Res. 24, 1691–1704. doi: 10.1158/1078-0432.CCR-17-0252
Bai, Y., Xiong, L., Zhu, M., Yang, Z., Zhao, J., Tang, H. (2019). Co-expression network analysis identified KIF2C in association with progression and prognosis in lung adenocarcinoma. Cancer Biomarkers 24, 371–382. doi: 10.3233/CBM-181512
Ballester, R., Marchuk, D., Boguski, M., Saulino, A., Letcher, R., Wigler, M., et al. (1990). The NF1 locus encodes a protein functionally related to mammalian GAP and yeast IRA proteins. Cell 63, 851–859. doi: 10.1016/0092-8674(90)90151-4
Barretina, J., Caponigro, G., Stransky, N., Venkatesan, K., Margolin, A. A., Kim, S., et al. (2012). The cancer cell line Encyclopedia enables predictive modelling of anticancer drug sensitivity. Nature 483, 603–607. doi: 10.1038/nature11003
Beard, J. A., Tenga, A., Hills, J., Hoyer, J. D., Cherian, M. T., Wang, Y. D., et al. (2016). The orphan nuclear receptor NR4A2 is part of a p53-microRNA-34 network. Sci. Rep. 6, 25108. doi: 10.1038/srep25108
Benjamini, Y., Hochberg, Y. (1995). Controlling the false discovery rate: a practical and powerful approach to multiple testing. J. R. Stat. Soc. Ser. B 57, 289–300. doi: 10.2307/2346101
Bepler, G., Kusmartseva, I., Sharma, S., Gautam, A., Cantor, A., Sharma, A., et al. (2006). RRM1 modulated in vitro and in vivo efficacy of gemcitabine and platinum in non–small-cell lung cancer. J. Clin. Oncol. 24, 4731–4737. doi: 10.1200/JCO.2006.06.1101
Berger, A. H., Niki, M., Morotti, A., Taylor, B. S., Socci, N. D., Viale, A., et al. (2010). Identification of DOK genes as lung tumor suppressors. Nat. Genet. 42, 216–223. doi: 10.1038/ng.527
Bertran-Alamillo, J., Cattan, V., Schoumacher, M., Codony-Servat, J., Giménez-Capitán, A., Cantero, F., et al. (2019). AURKB as a target in non-small cell lung cancer with acquired resistance to anti-EGFR therapy. Nat. Commun. 10, 1812. doi: 10.1038/s41467-019-09734-5
Blanchon, F., Grivaux, M., Asselain, B., Lebas, F. X., Orlando, J. P., Piquet, J., et al. (2006). 4-year mortality in patients with non-small-cell lung cancer: development and validation of a prognostic index. Lancet Oncol. 7, 829–836. doi: 10.1016/S1470-2045(06)70868-3
Bogaard, H. J., Abe, K., Noordegmaf, A. V., Voelkel, N. F. (2009). The right ventricle under pressure. Chest 135, 794–804. doi: 10.1378/chest.08-0492
Bollag, G., McCormick, F. (1991). Differential regulation of rasGAP and neurofibromatosis gene product activities. Nature 351, 576–579. doi: 10.1038/351576a0
Bouker, K. B., Skaar, T. C., Riggins, R. B., Harburger, D. S., Fernandez, D. R., Zwart, A., et al. (2005). Interferon regulatory factor-1 (IRF-1) exhibits tumor suppressor activities in breast cancer associated with caspase activation and induction of apoptosis. Carcinogenesis 26, 1527–1535. doi: 10.1093/carcin/bgi113
Bowie, M. L., Ibarra, C., Seewalt, V. L. (2008). IRF-1 promotes apoptosis in p53-damaged basal-type human mammary epithelial cells: a model for early basal-type mammary carcinogenesis. Adv. In Exp. Med. Biol., 617, 367–374. doi: 10.1007/978-0-387-69080-3_35
Braun, M. M., Caporaso, N. E., Page, W. F., Hoover, R. N. (1994). Genetic component of lung cancer: cohort study of twins. Lancet (London England) 344, 440–443. doi: 10.1016/S0140-6736(94)91770-1
Bray, F., Ferlay, J., Soerjomataram, I., Siegel, R. L., Torre, L. A., Jemal, A. (2018). Global cancer statistics 2018: GLOBOCAN estimates of incidence and mortality worldwide for 36 cancers in 185 countries. CA. Cancer J. Clin. 68, 394–424. doi: 10.3322/caac.21492
Brock, M., Trenkmann, M., Gay, R. E., Michel, B. A., Gay, S., Fischler, M., et al. (2009). Interleukin-6 modulates the expression of the bone morphogenic protein receptor type ii through a novel STAT3-microRNA cluster 17/92 pathway. Circ. Res. 104, 1184–1191. doi: 10.1161/CIRCRESAHA.109.197491
Cai, Y., Dong, Z.-Y., Wang, J.-Y. (2018). MiR-520b inhibited metastasis and proliferation of non-small cell lung cancer by targeting CHAF1A. Eur. Rev. Med. Pharmacol. Sci. 22, 7742–7749. doi: 10.26355/eurrev_201811_16396.
Cameron, E. R., Neil, J. C. (2004). The Runx genes : lineage-specific oncogenes and tumor suppressors. Oncogene 23, 4308–4314. doi: 10.1038/sj.onc.1207130
Cao, Y., Zhu, W., Chen, W., Wu, J., Hou, G., Li, Y. (2019). Prognostic value of BIRC5 in lung adenocarcinoma lacking EGFR, KRAS, and ALK mutations by integrated bioinformatics analysis. Dis. Markers 2019, 1–12. doi: 10.1155/2019/5451290
Carvalho, L., Silva, A., Andrade, C., Barroso, C., Farinha, C., Fernandes, J. C., et al. (2009). [ERCC1 and RRM1 genes in lung cancer]. Rev. Port. Pneumol. 15, 683–696. doi: 10.1016/s0873-2159(15)30164-1
Chaouat, A., Coulet, F., Favre, C., Simonneau, G., Weitzenblum, E., Soubrier, F., et al. (2004). Endoglin germline mutation in a patient with hereditary haemorrhagic telangiectasia and dexfenfluramine associated pulmonary arterial hypertension. Thorax 59, 446–448. doi: 10.1136/thx.2003.11890
Chen, H., Lee, J., Kljavin, N. M., Haley, B., Daemen, A., Johnson, L., et al. (2015). Requirement for BUB1B/BUBR1 in tumor progression of lung adenocarcinoma. Genes Cancer 6, 106. doi: 10.18632/genesandcancer.53
Cheung, C. H. Y., Hsu, C. L., Chen, K. P., Chong, S. T., Wu, C. H., Huang, H. C., et al. (2017). MCM2-regulated functional networks in lung cancer by multi-dimensional proteomic approach. Sci. Rep. 7, 13302. doi: 10.1038/s41598-017-13440-x
Chhina, M. K., Nathan, S. D., Emblom-Callahan, M. C., Ahmad, S., Shlobin, O. A., Lemma, M., et al. (2010). Immunohistochemistry analysis for proliferation marker in IPF lung tissue. Chest 138, 540A. doi: 10.1378/chest.9899
Cho, J. H., Gelinas, R., Wang, K., Etheridge, A., Piper, M. G., Batte, K., et al. (2011). Systems biology of interstitial lung diseases: integration of mRNA and microRNA expression changes. BMC Med. Genomics 4, 8. doi: 10.1186/1755-8794-4-8
Choi, E.-B., Yang, A.-Y., Kim, S. C., Lee, J., Choi, J. K., Choi, C., et al. (2016). PARP1 enhances lung adenocarcinoma metastasis by novel mechanisms independent of DNA repair. Oncogene 35, 4569–4579. doi: 10.1038/onc.2016.3
Choy, B., LaLonde, A., Que, J., Wu, T., Zhou, Z. (2016). MCM4 and MCM7, potential novel proliferation markers, significantly correlated with Ki-67, Bmi1, and cyclin E expression in esophageal adenocarcinoma, squamous cell carcinoma, and precancerous lesions. Hum. Pathol. 57, 126–135. doi: 10.1016/j.humpath.2016.07.013
Christmann, R. B., Wooten, A., Sampaio-Barros, P., Borges, C. L., Carlos, C. R., Kairalla, R. A., et al. (2016). miR-155 in the progression of lung fibrosis in systemic sclerosis. Arthritis Res. Ther. 18, 155. doi: 10.1186/S13075-016-1054-6
Collisson, E. A., Campbell, J. D., Brooks, A. N., Berger, A. H., Lee, W., Chmielecki, J., et al. (2014). Comprehensive molecular profiling of lung adenocarcinoma: The cancer genome atlas research network. Nature 511, 543–550. doi: 10.1038/nature13385
Cooper, R., Bruce, M., Wootton, A., Hands, D., Daly, L. (2003). Managing design in the extended enterprise. Build. Res. Inf. 22, 367–378. doi: 10.1080/0961321032000107555
Cooper, W. A., Kohonen-Corish, M. R. J., Chan, C., Kwun, S. Y., McCaughan, B., Kennedy, C., et al. (2008). Prognostic significance of DNA repair proteins MLH1, MSH2 and MGMT expression in non-small-cell lung cancer and precursor lesions. Histopathology 52, 613–622. doi: 10.1111/j.1365-2559.2008.02999.x
Coultas, D. B., Zumwalt, R. E., Black, W. C., Sobonya, R. E. (1994). The epidemiology of interstitial lung diseases. Am. J. Respir. Crit. Care Med. 150, 967–972. doi: 10.1164/ajrccm.150.4.7921471
Courboulin, A., Paulin, R., Giguère, N. J., Saksouk, N., Perreault, T., Meloche, J., et al. (2011). Role for miR-204 in human pulmonary arterial hypertension. J. Exp. Med. 208, 535–548. doi: 10.1084/jem.20101812
Das, S. K., Menezes, M. E., Bhatia, S., Wang, X.-Y., Emdad, L., Sarkar, D., et al. (2015). Gene therapies for cancer: strategies, challenges and successes. J. Cell. Physiol. 230, 259–271. doi: 10.1002/jcp.24791
Dela Cruz, C. S., Tanoue, L. T., Matthay, R. A. (2011). Lung cancer: epidemiology, etiology, and prevention. Clin. Chest Med. 32, 605–644. doi: 10.1016/j.ccm.2011.09.001
DeNicola, G. M., Chen, P. H., Mullarky, E., Sudderth, J. A., Hu, Z., Wu, D., et al. (2015). NRF2 regulates serine biosynthesis in non-small cell lung cancer. Nat. Genet. 47, 1475–1481. doi: 10.1038/ng.3421
Dennis, G., Sherman, B. T., Hosack, D. A., Yang, J., Gao, W., Lane, H. C., et al. (2003). DAVID: Database for annotation, visualization, and integrated discovery. Genome Biol. 4. P3. doi: 10.1186/gb-2003-4-9-r60
Dingemans, A. C., van Ark-Otte, J., Span, S., Scagliotti, G. V., van der Valk, P., Postmus, P. E., et al. (2001). Topoisomerase IIalpha and other drug resistance markers in advanced non-small cell lung cancer. Lung Cancer 32, 117–128. doi: 10.1016/s0169-5002(00)00224-5
Dornan, D., Eckert, M., Wallace, M., Shimizu, H., Ramsay, E., Hupp, T. R., et al. (2004). Interferon Regulatory Factor 1 Binding to p300 Stimulates DNA-Dependent Acetylation of p53 . Mol. Cell. Biol. 24, 10083–10098. doi: 10.1128/mcb.24.22.10083-10098.2004
Dos Santos, E. O., Carneiro-Lobo, T. C., Aoki, M. N., Levantini, E., Bassères, D. S. (2016). Aurora kinase targeting in lung cancer reduces KRAS-induced transformation. Mol. Cancer 15, 12. doi: 10.1186/s12943-016-0494-6
El-Chemaly, S., Cheung, F., Kotliarov, Y., O’Brien, K. J., Gahl, W. A., Chen, J., et al. (2018a). The immunome in two inherited forms of pulmonary fibrosis. Front. Immunol. 9, 76. doi: 10.3389/fimmu.2018.00076
El-Chemaly, S., Cheung, F., Kotliarov, Y., O’Brien, K. J., Gahl, W. A., Chen, J., et al. (2018b). The immunome in two inherited forms of pulmonary fibrosis. Front. Immunol. 9, 76. doi: 10.3389/fimmu.2018.00076
Ergün, S., Tilki, D., Klein, D. (2011). Vascular wall as a reservoir for different types of stem and progenitor cells. Antioxidants Redox Signal. 15, 981–995. doi: 10.1089/ars.2010.3507
Eswaran, J., Horvath, A., Godbole, S., Reddy, S. D., Mudvari, P., Ohshiro, K., et al. (2013). RNA sequencing of cancer reveals novel splicing alterations. Sci. Rep. 3, 1689. doi: 10.1038/srep01689
Eymin, B., Gazzeri, S., Brambilla, C., Brambilla, E. (2001). Distinct pattern of E2F1 expression in human lung tumours: E2F1 is upregulated in small cell lung carcinoma. Oncogene 20, 1678–1687. doi: 10.1038/sj.onc.1204242
Falleni, M., Pellegrini, C., Marchetti, A., Oprandi, B., Buttitta, F., Barassi, F., et al. (2003). Survivin gene expression in early-stage non-small cell lung cancer. J. Pathol. 200, 620–626. doi: 10.1002/path.1388
Fei, L., Ma, Y., Zhang, M., Liu, X., Luo, Y., Wang, C., et al. (2017). RACK1 promotes lung cancer cell growth via an MCM7/RACK1/Akt signaling complex. Oncotarget 8, 40501–40513. doi: 10.18632/oncotarget.17120
Fenton, M. D., Weyrich, M. S., Durbin, S., Liu, Y., Bang, H., Melnikow, J. (2011). Prostate-Specific Antigen-Based Screening for Prostate Cancer ((US): Agency for Healthcare Research and Quality). Available at: http://www.ncbi.nlm.nih.gov/pubmed/22171385 [Accessed October 25, 2018].
Ficklin, S. P., Dunwoodie, L. J., Poehlman, W. L., Watson, C., Roche, K. E., Feltus, F. A. (2017). Discovering condition-specific gene co-expression patterns using gaussian mixture models: a cancer case study. Sci. Rep. 7, 1–11. doi: 10.1038/s41598-017-09094-4
Fragale, A., Gabriele, L., Stellacci, E., Borghi, P., Perrotti, E., Ilari, R., et al. (2014). IFN Regulatory Factor-1 Negatively Regulates CD4+CD25+ Regulatory T cell differentiation by repressing Foxp3 expression. J. Immunol. 181, 1673–1682. doi: 10.4049/jimmunol.181.3.1673
Gahring, L. C., Myers, E. J., Dunn, D. M., Weiss, R. B., Rogers, S. W. (2017). Lung epithelial response to cigarette smoke and modulation by the nicotinic alpha 7 receptor. PloS ONE 12, e0187773. doi: 10.1371/journal.pone.0187773
Gao, D., Wang, L., Zhang, H., Yan, X., Yang, J., Zhou, R., et al. (2018). Spleen tyrosine kinase SYK(L) interacts with YY1 and coordinately suppresses SNAI2 transcription in lung cancer cells. FEBS J. 285, 4229–4245. doi: 10.1111/febs.14665
Gautier, L., Cope, L., Bolstad, B. M., Irizarry, R. A. (2004). Affy - Analysis of affymetrix genechip data at the probe level. Bioinformatics 20, 307–315. doi: 10.1093/bioinformatics/btg405
Giopanou, I., Papaleonidopoulos, V., Lilis, I., Kanellakis, N., Agalioti, T., Stathopoulos, G. (2016). Tumor cell-derived osteopontin promotes lung metastasis via both cell-autonomous and paracrine pathways. in 11.1 . Lung Cancer European Resp. Soc. 48, OA1525. doi: 10.1183/13993003.congress-2016.oa1525
Girerd, B., Montani, D., Coulet, F., Sztrymf, B., Yaici, A., Jaïs, X., et al. (2010). Clinical outcomes of pulmonary arterial hypertension in patients carrying an ACVRL1 (ALK1) mutation. Am. J. Respir. Crit. Care Med. 181, 851–861. doi: 10.1164/rccm.200908-1284OC
Gomez-Arroyo, J., Saleem, S. J., Mizuno, S., Syed, A. A., Bogaard, H. J., Abbate, A., et al. (2012). A brief overview of mouse models of pulmonary arterial hypertension: problems and prospects. Am. J. Physiol. - Lung Cell. Mol. Physiol. 10, 302. doi: 10.1152/ajplung.00362.2011
Gordenin, D. A., Kunkel, T. A., Resnick, M. A. (1997). Repeat expansion - All in a flap? Nat. Genet. 16, 116–118. doi: 10.1038/ng0697-116
Gridelli, C., Rossi, A., Carbone, D. P., Guarize, J., Karachaliou, N., Mok, T., et al. (2015). Non-small-cell lung cancer. Nat. Rev. Dis. Prim. 1, 15009. doi: 10.1038/nrdp.2015.9
Griese, M., Irnstetter, A., Hengst, M., Burmester, H., Nagel, F., Ripper, J., et al. (2015). Categorizing diffuse parenchymal lung disease in children rare pulmonary diseases. Orphanet J. Rare Dis. 10, 122. doi: 10.1186/s13023-015-0339-1
Guignabert, C., Dorfmuller, P. (2013). Pathology and pathobiology of pulmonary hypertension. Semin. Respir. Crit. Care Med. 34, 551–559. doi: 10.1055/s-0033-1356496
Guignabert, C., Tu, L., Le Hiress, M., Ricard, N., Sattler, C., Seferian, A., et al. (2013). Pathogenesis of pulmonary arterial hypertension: lessons from cancer. Eur. Respir. Rev. 22, 543–551. doi: 10.1183/09059180.00007513
Gyorffy, B., Surowiak, P., Budczies, J., Lánczky, A. (2013). Online survival analysis software to assess the prognostic value of biomarkers using transcriptomic data in non-small-cell lung cancer. PloS ONE 8, e82241. doi: 10.1371/journal.pone.0082241
Hammerman, P. S., Voet, D., Lawrence, M. S., Voet, D., Jing, R., Cibulskis, K., et al. (2012). Comprehensive genomic characterization of squamous cell lung cancers. Nature 489, 519–525. doi: 10.1038/nature11404
Hanahan, D., Weinberg, R. (2011). Hallmarks of cancer: the next generation. Cell 144, 646–674. doi: 10.1016/j.cell.2011.02.013
He, L., Luo, L., Zhu, H., Yang, H., Zhang, Y., Wu, H., et al. (2017a). FEN1 promotes tumor progression and confers cisplatin resistance in non-small-cell lung cancer. Mol. Oncol. 11, 640–654. doi: 10.1002/1878-0261.12058
He, Z. Y., Wu, S. G., Peng, F., Zhang, Q., Luo, Y., Chen, M., et al. (2017b). Up-regulation of RFC3 promotes triple negative breast cancer metastasis and is associated with poor prognosis via EMT. Transl. Oncol. 10, 1–9. doi: 10.1016/j.tranon.2016.10.004
Henao, J., Lopez-Kleine, L., Pinzon-Velasco, A. (2018). coexnet: coexnet: An R package to build CO-EXpression NETworks from Microarray Data. R package version 1.4.0
Hirano, H., Maeda, H., Yamaguchi, T., Yokota, S., Mori, M., Sakoda, S. (2015). Survivin expression in lung cancer: association with smoking, histological types and pathological stages. Oncol. Lett. 10, 1456–1462. doi: 10.3892/ol.2015.3374
Hoeper, M. M., Barst, R. J., Bourge, R. C., Feldman, J., Frost, A. E., Galié, N., et al. (2013). Imatinib mesylate as add-on therapy for pulmonary arterial hypertension: Results of the randomized IMPRES study. Circulation 127, 1128–1138. doi: 10.1161/CIRCULATIONAHA.112.000765
Hou, G. X., Liu, P., Yang, J., Wen, S. (2017). Mining expression and prognosis of topoisomerase isoforms in non-small-cell lung cancer by using Oncomine and Kaplan-Meier plotter. PloS One 12, e0174515. doi: 10.1371/journal.pone.0174515
Huang, R., Gao, L. (2018). Identification of potential diagnostic and prognostic biomarkers in non-small cell lung cancer based on microarray data. Oncol. Lett. 15, 6436–6442. doi: 10.3892/ol.2018.8153
Huang, T., Wang, G., Yang, L., Peng, B., Wen, Y., Ding, G., et al. (2017). Transcription factor YY1 modulates lung cancer progression by activating lncRNA-PVT1 . DNA Cell Biol. 36, 947–958. doi: 10.1089/dna.2017.3857
Huber, W., Von Heydebreck, A., Sültmann, H., Poustka, A., Vingron, M. (2002). Variance stabilization applied to microarray data calibration and to the quantification of differential expression. Bioinformatics 18, S96–S104. doi: 10.1093/bioinformatics/18.suppl_1.S96
IARC, Boyle, P. (2018). World cancer report 2018 . Cancer Control 199, 512. doi: 10.1016/j.cma.2010.02.010
Iguchi, H., Hara, N., Hayashi, I., Ohta, M., Bloom, S. R., Chrétien, M. (1989). Elevation of a novel pituitary protein (7B2) in the plasma in small cell carcinoma of the lung. Eur. J. Cancer Clin. Oncol. 25, 1225–1232. doi: 10.1016/0277-5379(89)90419-7
Ivliev, A. E., Hoen, P. A., Borisevich, D., Nikolsky, Y., Sergeeva, M. G. (2016). Drug repositioning through systematic mining of gene coexpression networks in cancer. PloS One 11, e0165059. doi: 10.1371/journal.pone.0165059
Janky, R., Verfaillie, A., Imrichová, H., de Sande, B., Standaert, L., Christiaens, V., et al. (2014). iRegulon: from a gene list to a gene regulatory network using large motif and track collections. PloS Comput. Biol. 10, e1003731. doi: 10.1371/journal.pcbi.1003731
Jiang, L. H., Zhang, H., Tang, J. H. (2018). MiR-30a: a novel biomarker and potential therapeutic target for cancer. J. Oncol. 2018, 1–9. doi: 10.1155/2018/5167829
Jung, H. I., Park, J. S., Lee, M.-Y., Park, B., Kim, H. J., Park, S. H., et al. (2011). Prevalence of lung cancer in patients with interstitial lung disease is higher than in those with chronic obstructive pulmonary disease. Med. (Baltimore). 97, 1–5. doi: 10.1097/MD.0000000000010071
Kamel, H. F. M., Al-Amodi, H. S. A. B. (2017). Exploitation of Gene Expression and cancer biomarkers in paving the path to era of personalized medicine. Genomics Proteomics Bioinforma. 15, 220–235. doi: 10.1016/j.gpb.2016.11.005
Kato, T., Lee, D., Huang, H., Cruz, W., Ujiie, H., Fujino, K., et al. (2018). Personalized siRNA-nanoparticle systemic therapy using metastatic lymph node specimens obtained with EBUS-TBNA in lung cancer. Mol. Cancer Res. 16, 47–57. doi: 10.1158/1541-7786.MCR-16-0341
Kaufhold, S., Garbán, H., Bonavida, B. (2016). Yin Yang 1 is associated with cancer stem cell transcription factors (SOX2, OCT4, BMI1) and clinical implication. J. Exp. Clin. Cancer Res. 35, 1–14. doi: 10.1186/s13046-016-0359-2
Kim, H. P., Kaminski, N., Feghali-Bostwick, C. A., Xu, X., Oury, T. D., Choi, A. M. K., et al. (2006). Caveolin-1: a critical regulator of lung fibrosis in idiopathic pulmonary fibrosis. J. Exp. Med. 203, 2895–2906. doi: 10.1084/jem.20061536
Kogan, E. A., Tyong, F. V., Demura, S. A. (2010). The mechanism of lung tissue remodeling in the progression of idiopathic pulmonary fibrosis. Arkh. Patol. 72, 30–36. Available at: http://www.ncbi.nlm.nih.gov/pubmed/21086635 [Accessed December 9, 2018].
Konishi, K., Gibson, K. F., Lindell, K. O., Richards, T. J., Zhang, Y., Dhir, R., et al. (2009). Gene expression profiles of acute exacerbations of idiopathic pulmonary fibrosis. Am. J. Respir. Crit. Care Med. 180, 167–175. doi: 10.1164/rccm.200810-1596OC
Koper, A., Zeef, L. A. H., Joseph, L., Kerr, K., Gosney, J., Lindsay, M. A., et al. (2017). Whole transcriptome analysis of pre-invasive and invasive early squamous lung carcinoma in archival laser microdissected samples. Respir. Res. 18, 12. doi: 10.1186/s12931-016-0496-3
Korošec, B., Glavač, D., Rott, T., Ravnik-Glavač, M. (2006). Alterations in the ATP2A2 gene in correlation with colon and lung cancer. Cancer Genet. Cytogenet. 171, 105–111. doi: 10.1016/j.cancergencyto.2006.06.016
Kropski, J. A., Blackwell, T. S., Loyd, J. E. (2015). The genetic basis of idiopathic pulmonary fibrosis. Eur. Respir. J. 45, 1717–1727. doi: 10.1183/09031936.00163814
Kweh, F., Min, Z., Kurenova, E., Wallace, M., Golubovskaya, V., Cance, W. G. (2009). Neurofibromin physically interacts with the N-terminal domain of focal adhesion kinase. Mol. Carcinog. 48, 1005–1017. doi: 10.1002/mc.20552
Lajoie, A. C., Lauzière, G., Lega, J. C., Lacasse, Y., Martin, S., Simard, S., et al. (2016). Combination therapy versus monotherapy for pulmonary arterial hypertension: a meta-analysis. Lancet Respir. Med. 4, 291–305. doi: 10.1016/S2213-2600(16)00027-8
Landi, M. T., Dracheva, T., Rotunno, M., Figueroa, J. D., Liu, H., Dasgupta, A., et al. (2008). Gene expression signature of cigarette smoking and its role in lung adenocarcinoma development and survival. PloS ONE 3, e1651. doi: 10.1371/journal.pone.0001651
Lane, K. B., Machado, R. D., Pauciulo, M. W., Thomson, J. R., Phillips, J. A., Loyd, J. E., et al. (2000). Heterozygous germline mutations in BMPR2, encoding a TGF-β receptor, cause familial primary pulmonary hypertension. Nat. Genet. 26, 81–84. doi: 10.1038/79226
Larsen, J. E., Nathan, V., Osborne, J. K., Farrow, R. K., Deb, D., Sullivan, J. P., et al. (2016). ZEB1 drives epithelial-to-mesenchymal transition in lung cancer. J. Clin. Invest. 126, 3219–3235. doi: 10.1172/JCI76725
Le Jeune, I., Gribbin, J., West, J., Smith, C., Cullinan, P., Hubbard, R. (2007). The incidence of cancer in patients with idiopathic pulmonary fibrosis and sarcoidosis in the UK. Respir. Med. 101, 2534–2540. doi: 10.1016/j.rmed.2007.07.012
Leal, L. G., López, C., López-Kleine, L. (2014). Construction and comparison of gene co-expression networks shows complex plant immune responses. PeerJ 2, e610. doi: 10.7717/peerj.610
Lee, S., Shroyer, K. R., Markham, N. E., Cool, C. D., Voelkel, N. F., Tuder, R. M. (1998). Monoclonal endothelial cell proliferation is present in primary but not secondary pulmonary hypertension. J. Clin. Invest. 101, 927–934. doi: 10.1172/JCI1910
Lee, J. S., Collins, K. M., Brown, A. L., Lee, C. H., Chung, J. H. (2000). hCds1-mediated phosphorylation of BRCA1 regulates the DNA damage response. Nature 404, 201–204. doi: 10.1038/35004614
Lee, G. Y., Haverty, P. M., Li, L., Kljavin, N. M., Bourgon, R., Lee, J., et al. (2014). Comparative oncogenomics identifies psmb4 and shmt2 as potential cancer driver genes. Cancer Res. 74, 3114–3126. doi: 10.1158/0008-5472.CAN-13-2683
Li, M. L., Greenberg, R. A. (2012). Links between genome integrity and BRCA1 tumor suppression. Trends Biochem. Sci. 37, 418–424. doi: 10.1016/j.tibs.2012.06.007
Li, Z., Zhao, K., Tian, H. (2017). Integrated analysis of differential expression and alternative splicing of non-small cell lung cancer based on RNA sequencing. Oncol. Lett. 14, 1519–1525. doi: 10.3892/ol.2017.6300
Li, Y., Gu, J., Xu, F., Zhu, Q., Ge, D., Lu, C. (2018). Transcriptomic and functional network features of lung squamous cell carcinoma through integrative analysis of GEO and TCGA data. Sci. Rep. 8, 15834. doi: 10.1038/s41598-018-34160-w
Liu, K., Luo, Y., Lin, F. T., Lin, W. C. (2004). TopBP1 recruits Brg1/Brm to repress E2F1-induced apoptosis, a novel pRb-independent and E2F1-specific control for cell survival. Genes Dev. 18, 673–686. doi: 10.1101/gad.1180204
Liu, K., Bellam, N., Lin, H.-Y., Wang, B., Stockard, C. R., Grizzle, W. E., et al. (2009). Regulation of p53 by TopBP1: a potential mechanism for p53 inactivation in Cancer. Mol. Cell. Biol. 29, 2673–2693. doi: 10.1128/mcb.01140-08
Liu, Y. Z., Jiang, Y. Y., Hao, J. J., Lu, S. S., Zhang, T. T., Shang, L., et al. (2012). Prognostic significance of MCM7 expression in the bronchial brushings of patients with non-small cell lung cancer (NSCLC). Lung Cancer 77, 176–182. doi: 10.1016/j.lungcan.2012.03.001
Liu, Y. Z., Jiang, Y. Y., Wang, B. S., Hao, J. J., Shang, L., Zhang, T. T., et al. (2014). A panel of protein markers for the early detection of lung cancer with bronchial brushing specimens. Cancer Cytopathol. 122, 833–841. doi: 10.1002/cncy.21461
Liu, T., Wei, J., Jiang, C., Wang, C., Zhang, X., Du, Y., et al. (2017a). CHAF1A, the largest subunit of the chromatin assembly factor 1 complex, regulates the growth of H1299 human non-small cell lung cancer cells by inducing G0/G1 cell cycle arrest. Exp. Ther. Med. 14, 4681–4686. doi: 10.3892/etm.2017.5201
Liu, Y. Z., Wang, B. S., Jiang, Y. Y., Cao, J., Hao, J. J., Zhang, Y., et al. (2017b). MCMs expression in lung cancer: implication of prognostic significance. J. Cancer 8, 3641–3647. doi: 10.7150/jca.20777
Lu, T. P., Tsai, M. H., Lee, J. M., Hsu, C. P., Chen, P. C., Lin, C. W., et al. (2010). Identification of a novel biomarker, SEMA5A, for non-small cell lung carcinoma in nonsmoking women. Cancer Epidemiol. Biomarkers Prev. 19, 2590–2597. doi: 10.1158/1055-9965.EPI-10-0332
Ma, L., Roman-Campos, D., Austin, E. D., Eyries, M., Sampson, K. S., Soubrier, F., et al. (2013). A novel channelopathy in pulmonary arterial hypertension. N. Engl. J. Med. 369, 351–361. doi: 10.1056/NEJMoa1211097
Ma, K.-Y., Schonnesen, A. A., Brock, A., Van Den Berg, C., Eckhardt, S. G., Liu, Z., et al. (2019). Single-cell RNA sequencing of lung adenocarcinoma reveals heterogeneity of immune response–related genes. JCI Insight 4, 1–10. doi: 10.1172/jci.insight.121387
Machado, R. D., Pauciulo, M. W., Thomson, J. R., Lane, K. B., Morgan, N. V., Wheeler, L., et al. (2001). BMPR2 haploinsufficiency as the inherited molecular mechanism for primary pulmonary hypertension. Am. J. Hum. Genet. 68, 92–102. doi: 10.1086/316947
Mason, J. M., Dusad, K., Wright, W. D., Grubb, J., Budke, B., Heyer, W. D., et al. (2015). RAD54 family translocases counter genotoxic effects of RAD51 in human tumor cells. Nucleic Acids Res. 43, 3180–3196. doi: 10.1093/nar/gkv175
Masri, F. A., Xu, W., Comhair, S. A. A., Asosingh, K., Koo, M., Vasanji, A., et al. (2007). Hyperproliferative apoptosis-resistant endothelial cells in idiopathic pulmonary arterial hypertension. Am. J. Physiol. - Lung Cell. Mol. Physiol. 293, 293. doi: 10.1152/ajplung.00428.2006
Mattaini, K. R., Sullivan, M. R., Vander Heiden, M. G. (2016). The importance of serine metabolism in cancer. J. Cell Biol. 214, 249–257. doi: 10.1083/jcb.201604085
McDaniel, L. D., Conkrite, K. L., Chang, X., Capasso, M., Vaksman, Z., Oldridge, D. A., et al. (2017). Common variants upstream of MLF1 at 3q25 and within CPZ at 4p16 associated with neuroblastoma. PloS Genet. 13, e1006787. doi: 10.1371/journal.pgen.1006787
McMurtry, M. S., Archer, S. L., Altieri, D. C., Bonnet, S., Haromy, A., Harry, G., et al. (2005). Gene therapy targeting survivin selectively induces pulmonary vascular apoptosis and reverses pulmonary arterial hypertension. J. Clin. Invest. 115, 1479–1491. doi: 10.1172/JCI23203
Montani, D., Günther, S., Dorfmüller, P., Perros, F., Girerd, B., Garcia, G., et al. (2013). Pulmonary arterial hypertension. Orphanet J. Rare Dis. 8, 97. doi: 10.1186/1750-1172-8-97
Morrell, N. W. (2006). Pulmonary hypertension due to BMPR2 mutation: A new paradigm for tissue remodeling. Proc. Am. Thorac. Soc. 680–686. doi: 10.1513/pats.200605-118SF
Mura, M., Cecchini, M. J., Joseph, M., Granton, J. T. (2019). Osteopontin lung gene expression is a marker of disease severity in pulmonary arterial hypertension. Resp. Resp. 32, 13557. doi: 10.1111/resp.13557
Nasim, M. T., Ogo, T., Ahmed, M., Randall, R., Chowdhury, H. M., Snape, K. M., et al. (2011). Molecular genetic characterization of SMAD signaling molecules in pulmonary arterial hypertension. Hum. Mutat. 32, 1385–1389. doi: 10.1002/humu.21605
Nathan, N., Taam, R. A., Epaud, R., Delacourt, C., Deschildre, A., Reix, P., et al. (2012). A national internet-linked based database for pediatric interstitial lung diseases: The French network. Orphanet J. Rare Dis. 7, 1–11. doi: 10.1186/1750-1172-7-40
Neubauer, E., Wirtz, R. M., Kaemmerer, D., Athelogou, M., Schmidt, L., Sänger, J., et al. (2016). Comparative evaluation of three proliferation markers, Ki-67, TOP2A, and RacGAP1, in bronchopulmonary neuroendocrine neoplasms: Issues and prospects. Oncotarget 7, 41959–41973. doi: 10.18632/oncotarget.9747
Newman, J. H., Wheeler, L., Lane, K. B., Loyd, E., Gaddipati, R., Phillips, J. A., et al. (2001). Mutation in the gene for bone morphogenetic protein receptor II as a cause of primary pulmonary hypertension in a large kindred. N. Engl. J. Med. 345, 319–324. doi: 10.1056/NEJM200108023450502
Oleksiewicz, U., Liloglou, T., Tasopoulou, K. M., Daskoulidou, N., Gosney, J. R., Field, J. K., et al. (2017). COL1A1, PRPF40A, and UCP2 correlate with hypoxia markers in non-small cell lung cancer. J. Cancer Res. Clin. Oncol. 143, 1133–1141. doi: 10.1007/s00432-017-2381-y
Oshlack, A., Robinson, M. D., Young, M. D. (2010). From RNA-seq reads to differential expression results. Genome Biol. 11, 220. doi: 10.1186/gb-2010-11-12-220
Pardo, A., Gibson, K., Cisneros, J., Richards, T. J., Yang, Y., Becerril, C., et al. (2005). Up-regulation and profibrotic role of osteopontin in human idiopathic pulmonary fibrosis. PloS Med. 2, 0891–0903. doi: 10.1371/journal.pmed.0020251
Patel, N. M., Kawut, S. M., Jelic, S., Arcasoy, S. M., Lederer, D. J., Borczuk, A. C. (2013). Pulmonary arteriole gene expression signature in idiopathic pulmonary fibrosis. Eur. Respir. J. 41, 1324–1330. doi: 10.1183/09031936.0084112
Pierce, J. A., Hocott, J. B., Ebert, R. V. (1961). The collagen and elastin content of the lung in emphysema. Ann. Intern. Med. 55, 210–222. doi: 10.7326/0003-4819-55-2-210
Pullamsetti, S. S., Doebele, C., Fischer, A., Savai, R., Kojonazarov, B., Dahal, B. K., et al. (2012). Inhibition of microRNA-17 improves lung and heart function in experimental pulmonary hypertension. Am. J. Respir. Crit. Care Med. 185, 409–419. doi: 10.1164/rccm.201106-1093OC
Putkey, F. R., Cramer, T., Morphew, M. K., Silk, A. D., Johnson, R. S., McIntosh, J. R., et al. (2002). Unstable kinetochore-microtubule capture and chromosomal instability following deletion of CENP-E. Dev. Cell 3, 351–365. doi: 10.1016/S1534-5807(02)00255-1
R. Core Team. (2019). R: A language and environment for statistical computing (R Foundation for Statistical Computing). Available at: http://www.r-project.org/.
Radisky, D. C., Kenny, P. A., Bissell, M. J. (2007). Fibrosis and cancer: Do myofibroblasts come also from epithelial cells via EMT? J. Cell. Biochem. 101, 830–839. doi: 10.1002/jcb.21186
Raghu, G., Nyberg, F., Morgan, G. (2004). The epidemiology of interstitial lung disease and its association with lung cancer. Br. J. Cancer 91, S3–S10. doi: 10.1038/sj.bjc.6602061
Raghu, G., Remy-Jardin, M., Myers, J. L., Richeldi, L., Ryerson, C. J., Lederer, D. J., et al. (2018). Diagnosis of idiopathic pulmonary fibrosis An Official ATS/ERS/JRS/ALAT Clinical practice guideline. Am. J. Respir. Crit. Care Med. 198, e44–e68. doi: 10.1164/rccm.201807-1255ST
Rai, P. R., Cool, C. D., King, J. A. C., Stevens, T., Burns, N., Winn, R. A., et al. (2008). The cancer paradigm of severe pulmonary arterial hypertension. Am. J. Respir. Crit. Care Med. 178, 558–564. doi: 10.1164/rccm.200709-1369PP
Redig, A. J., Capelletti, M., Dahlberg, S. E., Sholl, L. M., Mach, S., Fontes, C., et al. (2016). Clinical and molecular characteristics of NF1-mutant lung cancer. Clin. Cancer Res. 22, 3148–3156. doi: 10.1158/1078-0432.CCR-15-2377
Relli, V., Trerotola, M., Guerra, E., Alberti, S. (2018). Distinct lung cancer subtypes associate to distinct drivers of tumor progression. Oncotarget 9, 35528–35540. doi: 10.18632/oncotarget.26217
Reuter, C. W., Morgan, M. A., Bergmann, L. (2000). Targeting the Ras signaling pathway: a rational, mechanism-based treatment for hematologic malignancies? Blood 96, 1655–1669. Available at: http://www.ncbi.nlm.nih.gov/pubmed/10961860 [Accessed July 9, 2019].
Ristic, D., Wyman, C., Paulusma, C., Kanaar, R. (2002). The architecture of the human Rad54-DNA complex provides evidence for protein translocation along DNA. Proc. Natl. Acad. Sci. 98, 8454–8460. doi: 10.1073/pnas.151056798
Roebroek, A. J. M., Martens, G. J. M., Duits, A. J., Schalken, J. A., Bokhoven, A., Wagenaar, S. S., et al. (1989). Differential expression of the gene encoding the novel pituitary polypeptide 7B2 in human lung cancer cells. Cancer Res. 49, 4154–4158. Available at: http://www.ncbi.nlm.nih.gov/pubmed/2545336 [Accessed December 12, 2018].
Romeo, G., Fiorucci, G., Chiantore, M. V., Percario, Z. A., Vannucchi, S., Affabris, E. (2002). Review: IRF-1 as a negative regulator of cell proliferation. J. Interf. Cytokine Res. 22, 39–47. doi: 10.1089/107999002753452647
Sánchez-Tilló, E., Lázaro, A., Torrent, R., Cuatrecasas, M., Vaquero, E. C., Castells, A., et al. (2010). ZEB1 represses E-cadherin and induces an EMT by recruiting the SWI/SNF chromatin-remodeling protein BRG1 . Oncogene 29, 3490–3500. doi: 10.1038/onc.2010.102
Safe, S., Jin, U.-H., Hedrick, E., Reeder, A., Lee, S.-O. (2013). Minireview: role of orphan nuclear receptors in cancer and potential as drug targets. Mol. Endocrinol. 28, 157–172. doi: 10.1210/me.2013-1291
Safe, S., Jin, U. H., Morpurgo, B., Abudayyeh, A., Singh, M., Tjalkens, R. B. (2016). Nuclear receptor 4A (NR4A) family - orphans no more. J. Steroid Biochem. Mol. Biol. 157, 48–60. doi: 10.1016/j.jsbmb.2015.04.016
Sahara, M., Sata, M., Morita, T., Nakamura, K., Hirata, Y., Nagai, R. (2007). Diverse contribution of bone marrow-derived cells to vascular remodeling associated with pulmonary arterial hypertension and arterial neointimal formation. Circulation 115, 509–517. doi: 10.1161/CIRCULATIONAHA.106.655837
Sakao, S., Taraseviciene-Stewart, L., Lee, J. D., Wood, K., Cool, C. D., Voelkel, N. F. (2005). Initial apoptosis is followed by increased proliferation of apoptosis-resistant endothelial cells. FASEB J. 19, 1178–1180. doi: 10.1096/fj.04-3261fje
Sauler, M., Lamontagne, M., Finnemore, E., Herazo-Maya, J. D., Tedrow, J., Zhang, X., et al. (2018). The DNA repair transcriptome in severe COPD. Eur. Respir. J. 52, 1701994. doi: 10.1183/13993003.01994-2017
Schneider, M. A., Christopoulos, P., Muley, T., Warth, A., Klingmueller, U., Thomas, M., et al. (2017a). AURKA, DLGAP5, TPX2, KIF11 and CKAP5: Five specific mitosis-associated genes correlate with poor prognosis for non-small cell lung cancer patients. Int. J. Oncol. 50, 365–372. doi: 10.3892/ijo.2017.3834
Schneider, M. A., Christopoulos, P., Muley, T., Warth, A., Klingmueller, U., Thomas, M., et al. (2017b). AURKA, DLGAP5, TPX2, KIF11 and CKAP5: Five specific mitosis-associated genes correlate with poor prognosis for non-small cell lung cancer patients. Int. J. Oncol. 50, 365–372. doi: 10.3892/ijo.2017.3834
Schwender, H. (2012). siggenes: Multiple testing using SAM and Efron’s empirical Bayes approaches. R package version 1.60.0. 1–70. Available at: https://git.bioconductor.org/packages/siggenes.
Sedic, M., Skibinski, A., Brown, N., Gallardo, M., Mulligan, P., Martinez, P., et al. (2015). Haploinsufficiency for BRCA1 leads to cell-type-specific genomic instability and premature senescence. Nat. Commun. 6, 7505. doi: 10.1038/ncomms8505
Shan, L., Zhao, M., Lu, Y., Ning, H., Yang, S., Song, Y., et al. (2019). CENPE promotes lung adenocarcinoma proliferation and is directly regulated by FOXM1 . Int. J. Oncol. 55, 257–266. doi: 10.3892/ijo.2019.4805
Shan Ruan, J., Zhou, H., Yang, L., Wang, L., Sheng Jiang, Z., Ming Wang, S. (2017). Original Article CCNA2 facilitates epithelial-to-mesenchymal transition via the integrin αvβ3 signaling in NSCLC. Int. J. Clin. Exp. Pathol. 10 (8), 8324–8333. Available at: www.ijcep.com/[Accessed April 3, 2019].
Shannon, P., Markiel, A., Ozier, O., Baliga, N. S., Wang, J. T., Ramage, D., et al. (2003). Cytoscape: A software Environment for integrated models of biomolecular interaction networks. Genome Res. 13, 2498–2504. doi: 10.1101/gr.1239303
Siegel, R., Miller, K., Jemal, A. (2015). Cancer statistics, 2015 . CA Cancer J. Clin. 65, 29. doi: 10.3322/caac.21254
Sigurdsson, S., Van Komen, S., Petukhova, G., Sung, P. (2002). Homologous DNA pairing by human recombination factors Rad51 and Rad54 . J. Biol. Chem. 277, 42790–42794. doi: 10.1074/jbc.M208004200
Soda, M., Choi, Y. L., Enomoto, M., Takada, S., Yamashita, Y., Ishikawa, S., et al. (2007). Identification of the transforming EML4-ALK fusion gene in non-small-cell lung cancer. Nature 448, 561–566. doi: 10.1038/nature05945
Sokai, A., Handa, T., Tanizawa, K., Oga, T., Uno, K., Tsuruyama, T., et al. (2015). Matrix metalloproteinase-10: a novel biomarker for idiopathic pulmonary fibrosis. Respir. Res. 16, 1–8. doi: 10.1186/s12931-015-0280-9
Song, Y.-J., Tan, J., Gao, X.-H., Wang, L.-X. (2018). Integrated analysis reveals key genes with prognostic value in lung adenocarcinoma. Cancer Manage. Res. 10, 6097–6108. doi: 10.2147/CMAR.S168636
Soria, J. C., Jang, S. J., Khuri, F. R., Hassan, K., Liu, D., Hong, W. K., et al. (2000). Overexpression of cyclin B1 in early-stage non-small cell lung cancer and its clinical implication. Cancer Res. 60, 4000–4004. Available at: http://www.ncbi.nlm.nih.gov/pubmed/10945597 [Accessed June 20, 2019].
Sotgia, F., Lisanti, M. P. (2017). Mitochondrial markers predict survival and progression in non-small cell lung cancer (NSCLC) patients: use as companion diagnostics. Oncotarget 8, 68095–68107. doi: 10.18632/oncotarget.19677
Spagnolo, P., Grunewald, J., Du Bois, R. M. (2014). Genetic determinants of pulmonary fibrosis: evolving concepts. Lancet Respir. Med. 2, 416–428. doi: 10.1016/S2213-2600(14)70047-5
Spira, A., Beane, J., Pinto-Plata, V., Kadar, A., Liu, G., Shah, V., et al. (2004). Gene expression profiling of human lung tissue from smokers with severe emphysema. Am. J. Respir. Cell Mol. Biol. 31, 601–610. doi: 10.1165/rcmb.2004-0273OC
Stadler, Z. K., Thom, P., Robson, M. E., Weitzel, J. N., Kauff, N. D., Hurley, K. E., et al. (2010). Genome-wide association studies of cancer. J. Clin. Oncol. 28, 4255–4267. doi: 10.1200/JCO.2009.25.7816.
Steiner, M. K., Syrkina, O. L., Kolliputi, N., Mark, E. J., Hales, C. A., Waxman, A. B. (2009). Interleukin-6 overexpression induces pulmonary hypertension. Circ. Res. 104, 236–244. doi: 10.1161/CIRCRESAHA.108.182014
Steinman, R. M., Banchereau, J. (2007). Taking dendritic cells into medicine. Nature 449, 419–426. doi: 10.1038/nature06175
Stevens, L. E., Zhao, M., Liu, Z., Nguyen, D. (2015). “A novel molecular subset of metastatic lung adenocarcinoma is defined by the function of the proteoglycan receptor HMMR,” in Tumor Biology (American Association for Cancer Research), 2269–2269. doi: 10.1158/1538-7445.am2015-2269
Stevens, L. E., Cheung, W. K. C., Adua, S. J., Arnal-Estapé, A., Zhao, M., Liu, Z., et al. (2017). Extracellular matrix receptor expression in subtypes of lung adenocarcinoma potentiates outgrowth of micrometastases. Cancer Res. 77, 1905–1917. doi: 10.1158/0008-5472.CAN-16-1978
Stoimenov, I., Helleday, T. (2009). PCNA on the crossroad of cancer. Biochem. Soc Trans. 37, 605–613. doi: 10.1042/BST0370605
Sud, A., Kinnersley, B., Houlston, R. S. (2017). Genome-wide association studies of cancer: current insights and future perspectives. Nat. Rev. Cancer 17, 692–704. doi: 10.1038/nrc.2017.82
Sun, C. C., Zhou, Q., Hu, W.-D., Li, S. J., Zhang, F., Chen, Z. L., et al. (2018a). Transcriptional E2F1/2/5/8 as potential targets and transcriptional E2F3/6/7 as new biomarkers for the prognosis of human lung carcinoma. Aging (Albany. NY). 10, 973–987. doi: 10.18632/aging.101441
Sun, X., Cui, F., Yin, H., Wu, D., Wang, N., Yuan, M., et al. (2018b). Association between EGFR mutation and expression of BRCA1 and RAP80 in non-small cell lung cancer. Oncol. Lett. 16, 2201–2206. doi: 10.3892/ol.2018.8938
Sutendra, G., Bonnet, S., Rochefort, G., Haromy, A., Folmes, K. D., Lopaschuk, G. D., et al. (2010). Fatty acid oxidation and malonyl-CoA decarboxylase in the vascular remodeling of pulmonary hypertension. Sci. Transl. Med. 2, 44ra58. doi: 10.1126/scitranslmed.3001327
Swagemakers, S. M., Essers, J., de Wit, J., Hoeijmakers, J. H., Kanaar, R. (1998). The human RAD54 recombinational DNA repair protein is a double-stranded DNA-dependent ATPase. J. Biol. Chem. 273, 28292–28297. doi: 10.1074/jbc.273.43.28292
Tan, D. F., Huberman, J. A., Hyland, A., Loewen, G. M., Brooks, J. S., Beck, A. F., et al. (2001). MCM2 - A promising marker for premalignant lesions of the lung: A cohort study. BMC Cancer 1, 6. doi: 10.1186/1471-2407-1-6
Tanaka, N., Ishihara, M., Lamphier, M. S., Nozawa, H., Matsuyama, T., Mak, T. W., et al. (1996). Cooperation of the tumour suppressors IRF-1 and p53 in response to DNA damage. Nature 382, 816–818. doi: 10.1038/382816a0
Tibshirani, R., Michael, J., Seo, G., Balasubramanian, Chu, Narasimhan, J. L. (2018). samr: SAM: significance analysis of microarrays. Available at: https://cran.r-project.org/package=samr.
Tishkoff, D. X., Filosi, N., Gaida, G. M., Kolodner, R. D. (1997). A novel mutation avoidance mechanism dependent on S. cerevisiae RAD27 is distinct from DNA mismatch repair. Cell 88, 253–263. doi: 10.1016/S0092-8674(00)81846-2
Trembath, R. C., Thomson, J. R., Machado, R. D., Morgan, N. V., Atkinson, C., Winship, I., et al. (2001). Clinical and molecular genetic features of pulmonary hypertension in patients with hereditary hemorrhagic telangiectasia. N. Engl. J. Med. 345, 325–334. doi: 10.1056/NEJM200108023450503
Tu, L., De Man, F. S., Girerd, B., Huertas, A., Chaumais, M. C., Lecerf, F., et al. (2012). A critical role for p130 Cas in the progression of pulmonary hypertension in humans and rodents. Am. J. Respir. Crit. Care Med. 186, 666–676. doi: 10.1164/rccm.201202-0309OC
Tuder, R. M., Cool, C. D., Yeager, M., Taraseviciene-Stewart, L., Bull, T. M., Voelkel, N. F. (2001). The pathobiology of pulmonary hypertension: Endothelium. Clin. Chest Med. 22, 405–418. doi: 10.1016/S0272-5231(05)70280-X
Tuder, R. M., Davis, L. A., Graham, B. B. (2012). Targeting energetic metabolism: A new frontier in the pathogenesis and treatment of pulmonary hypertension. Am. J. Respir. Crit. Care Med. 185, 260–266. doi: 10.1164/rccm.201108-1536PP
Välk, K., Vooder, T., Kolde, R., Reintam, M. A., Petzold, C., Vilo, J., et al. (2011). Gene expression profiles of non-small cell lung cancer: survival prediction and new biomarkers. Oncology 79, 283–292. doi: 10.1159/000322116
Van Duijnhoven, H. L. P., Vissers, P. M. A. M., Timmer, E. D. J., Groeneveld, A., Van De Ven, W. J. M. (1991). The 7B2 protein as marker for neuroendocrine differentiation in human lung cancer. Br. J. Cancer 63, 4154. Available at: https://europepmc.org/backend/ptpmcrender.fcgi?accid=PMC2204091&blobtype=pdf [Accessed December 12, 2018].
Vigouroux, C., Casse, J. M., Battaglia-Hsu, S. F., Brochin, L., Luc, A., Paris, C., et al. (2015). Methyl(R217)HuR and MCM6 are inversely correlated and are prognostic markers in non small cell lung carcinoma. Lung Cancer 89, 189–196. doi: 10.1016/j.lungcan.2015.05.008
Vukmirovic, M., Kaminski, N. (2018). Impact of transcriptomics on our understanding of pulmonary fibrosis. Front. Med. 5, 87. doi: 10.3389/fmed.2018.00087
Wachi, S., Yoneda, K., Wu, R. (2005). Interactome-transcriptome analysis reveals the high centrality of genes differentially expressed in lung cancer tissues. Bioinformatics 21, 4205–4208. doi: 10.1093/bioinformatics/bti688
Wang, X., Liotta, L. (2011). Clinical bioinformatics: a new emerging science. J. Clin. Bioinforma. 1, 1. doi: 10.1186/2043-9113-1-1
Wang, B., Matsuoka, S., Ballif, B. A., Zhang, D., Smogorzewska, A., Gygi, S. P., et al. (2007). Abraxas and RAP80 form a BRCA1 protein complex required for the DNA damage response. Science (80-.), 1194–1198. doi: 10.1126/science.1139476
Wang, I. M., Stepaniants, S., Boie, Y., Mortimer, J. R., Kennedy, B., Elliott, M., et al. (2008). Gene expression profiling in patients with chronic obstructive pulmonary disease and lung cancer. Am. J. Respir. Crit. Care Med. 177, 402–411. doi: 10.1164/rccm.200703-390OC
Wang, M., Yan, J., He, X., Zhong, Q., Zhan, C., Li, S. (2016). Candidate genes and pathogenesis investigation for sepsis-related acute respiratory distress syndrome based on gene expression profile. Biol. Res. 49, 25. doi: 10.1186/s40659-016-0085-4
Warth, A., Cortis, J., Soltermann, A., Meister, M., Budczies, J., Stenzinger, A., et al. (2014). Tumour cell proliferation (Ki-67) in non-small cell lung cancer: a critical reappraisal of its prognostic role. Br. J. Cancer 111, 1222–1229. doi: 10.1038/bjc.2014.402
Wellner, U., Schubert, J., Burk, U. C., Schmalhofer, O., Zhu, F., Sonntag, A., et al. (2009). The EMT-activator ZEB1 promotes tumorigenicity by repressing stemness-inhibiting microRNAs. Nat. Cell Biol. 11, 1487–1495. doi: 10.1038/ncb1998
Willis, B. C., Borok, Z. (2009). Epithelial-mesenchymal transition: Potential role in obliterative bronchiolitis? Thorax 64, 742–743. doi: 10.1136/thx.2009.114413
Willuda, J., Linden, L., Lerchen, H.-G., Kopitz, C., Stelte-Ludwig, B., Pena, C., et al. (2017). Preclinical Antitumor Efficacy of BAY 1129980—a Novel Auristatin-Based Anti-C4.4A (LYPD3) Antibody–Drug Conjugate for the Treatment of Non–Small Cell Lung Cancer. Mol. Cancer Ther. 16, 893–904. doi: 10.1158/1535-7163.MCT-16-0474
Xu, G., O’Connell, P., Viskochil, D., Cawthon, R., Robertson, M., Culver, M., et al. (1990). The neurofibromatosis type 1 gene encodes a protein related to GAP. Cell 62, 599–608. doi: 10.1016/0092-8674(90)90024-9
Xu, W., Koeck, T., Lara, A. R., Neumann, D., DiFilippo, F. P., Koo, M., et al. (2007). Alterations of cellular bioenergetics in pulmonary artery endothelial cells. Proc. Natl. Acad. Sci. U. S. A. 104, 1342–1347. doi: 10.1073/pnas.0605080104
Yan, H., Bi, L., Wang, Y., Zhang, X., Hou, Z., Wang, Q., et al. (2017). Integrative analysis of multi-omics data reveals distinct impacts of DDB1-CUL4 associated factors in human lung adenocarcinomas. Sci. Rep. 7, 333. doi: 10.1038/s41598-017-00512-1
Yang, J., Ramnath, N., Moysich, K. B., Asch, H. L., Swede, H., Alrawi, S. J., et al. (2006). Prognostic significance of MCM2, Ki-67 and gelsolin in non-small cell lung cancer. BMC Cancer 6, 203. doi: 10.1186/1471-2407-6-203
Yang, J., Wheeler, S. E., Velikoff, M., Kleaveland, K. R., Lafemina, M. J., Frank, J. A., et al. (2013). Activated alveolar epithelial cells initiate fibrosis through secretion of mesenchymal proteins. Am. J. Pathol. 183, 1559–1570. doi: 10.1016/j.ajpath.2013.07.016
Yeager, M. E., Halley, G. R., Golpon, H. A., Voelkel, N. F., Tuder, R. M. (2001). Microsatellite instability of endothelial cell growth and apoptosis genes within plexiform lesions in primary pulmonary hypertension. Circ. Res. 88, E2–E11. doi: 10.1161/01.res.88.1.e2
Yeager, M. E., Frid, M. G., Stenmark, K. R. (2011). Progenitor cells in pulmonary vascular remodeling. Pulm. Circ. 1, 3–16. doi: 10.4103/2045-8932.78095
Yeh, J. E., Toniolo, P. A., Frank, D. A. (2013). Targeting transcription factors: promising new strategies for cancer therapy. Curr. Opin. Oncol. 25, 652–658. doi: 10.1097/01.cco.0000432528.88101.1a
Yin, J., Fu, W., Dai, L., Jiang, Z., Liao, H., Chen, W., et al. (2017). ANKRD22 promotes progression of non-small cell lung cancer through transcriptional up-regulation of E2F1 . Sci. Rep. 7, 4430. doi: 10.1038/s41598-017-04818-y
Yochum, Z., Socinski, M., Burns, T. (2009). Paradoxical functions of ZEB1 in EGFR-mutant lung cancer: tumor suppressor and driver of therapeutic resistance. J. Thorac. Dis. 6, S152–S161. doi: 10.21037/jtd.2016.11.59
Yoder, M. C., Mead, L. E., Prater, D., Krier, T. R., Mroueh, K. N., Li, F., et al. (2007). Redefining endothelial progenitor cells via clonal analysis and hematopoietic stem/progenitor cell principals. Blood 109 (5), 1801–1809. doi: 10.1182/blood-2006-08-043471
Yoshida, K., Inoue, I. (2004). Expression of MCM10 and TopBP1 is regulated by cell proliferation and UV irradiation via the E2F transcription factor. Oncogene 23, 6250–6260. doi: 10.1038/sj.onc.1207829
Yu, J., Wilson, J., Taylor, L., Polgar, P. (2015). DNA microarray and signal transduction analysis in pulmonary artery smooth muscle cells from heritable and idiopathic pulmonary arterial hypertension subjects. J. Cell. Biochem. 116, 386–397. doi: 10.1002/jcb.24987
Yu, L., Fang, F., Lu, S., Li, X., Yang, Y., Wang, Z. (2017). LncRNA-HIT promotes cell proliferation of non-small cell lung cancer by association with E2F1 . Cancer Gene Ther. 24, 221–226. doi: 10.1038/cgt.2017.10
Yu, L.-H., Huang, Q.-W., Zhou, X.-H. (2019a). Identification of Cancer Hallmarks Based on the Gene Co-expression Networks of Seven Cancers. Front. Genet. 10, 1–10. doi: 10.3389/fgene.2019.00099
Yu, L. H., Huang, Q. W., Zhou, X. H. (2019b). Identification of cancer hallmarks based on the gene co-expression networks of seven cancers. Front. Genet. 10, 1–10. doi: 10.3389/fgene.2019.00099
Zhang, W. C., Ng, S. C., Yang, H., Rai, A., Umashankar, S., Ma, S., et al. (2012). Glycine decarboxylase activity drives non-small cell lung cancer tumor-initiating cells and tumorigenesis. Cell 148, 259–272. doi: 10.1016/j.cell.2011.11.050
Zhang, T., Guo, L., Creighton, C. J., Lu, Q., Gibbons, D. L., Yi, E. S., et al. (2016a). A genetic cell context-dependent role for ZEB1 in lung cancer. Nat. Commun. 7, 12231. doi: 10.1038/ncomms12231
Zhang, W., Mao, J. H., Zhu, W., Jain, A. K., Liu, K., Brown, J. B., et al. (2016b). Centromere and kinetochore gene misexpression predicts cancer patient survival and response to radiotherapy and chemotherapy. Nat. Commun. 7, 12619. doi: 10.1038/ncomms12619
Zhang, B., Zheng, A., Hydbring, P., Ambroise, G., Ouchida, A. T., Goiny, M., et al. (2017). PHGDH defines a metabolic subtype in lung adenocarcinomas with poor prognosis. Cell Rep. 19, 2289–2303. doi: 10.1016/j.celrep.2017.05.067
Zhang, K., Keymeulen, S., Nelson, R., Tong, T. R., Yuan, Y. C., Yun, X., et al. (2018a). Overexpression of flap endonuclease 1 correlates with enhanced proliferation and poor prognosis of non–small-cell lung cancer. Am. J. Pathol. 188, 242–251. doi: 10.1016/j.ajpath.2017.09.011
Zhang, M. Y., Liu, X. X., Li, H., Li, R., Liu, X., Qu, Y. Q. (2018b). Elevated mRNA levels of AURKA, CDC20 and TPX2 are associated with poor prognosis of smoking related lung adenocarcinoma using bioinformatics analysis. Int. J. Med. Sci. 15, 1676–1685. doi: 10.7150/ijms.28728
Zhang, W., Fan, J., Chen, Q., Lei, C., Qiao, B., Liu, Q. (2018c). SPP1 and AGER as potential prognostic biomarkers for lung adenocarcinoma. Oncol. Lett. 15, 7028–7036. doi: 10.3892/ol.2018.8235
Zheng, L., Dai, H., Zhou, M., Li, M., Singh, P., Qiu, J., et al. (2007a). Fen1 mutations result in autoimmunity, chronic inflammation and cancers. Nat. Med. 13, 812–819. doi: 10.1038/nm1599
Zheng, Z., Chen, T., Li, X., Haura, E., Sharma, A., Bepler, G. (2007b). DNA synthesis and repair genes RRM1 and ERCC1 in lung cancer. N. Engl. J. Med. 356, 800–808. doi: 10.1056/nejmoa065411
Zhou, X. H., Chu, X. Y., Xue, G., Xiong, J. H., Zhang, H. Y. (2019). Identifying cancer prognostic modules by module network analysis. BMC Bioinf. 20, 85. doi: 10.1186/s12859-019-2674-z
Zhu, Y. F., Zheng, S. Y., Jiang, D., Zhao, J. (2010). Clinicopathological significance of E-cadherin and PCNA expression in hunman non-small cell lung cancer. in 2010 4th International Conference on Bioinformatics and Biomedical Engineering, iCBBE 2010 . Chin. Anti-Cancer Assoc. 2010, 87–92. doi: 10.1109/ICBBE.2010.5516957
Keywords: lung cancer, interstitial lung diseases (ILDs), pulmonary arterial hypertension (PAH), differentially expressed genes (DEGs), coexpression networks, early detection and prognosis biomarkers, survival
Citation: Otálora-Otálora BA, Florez M, López-Kleine L, Canas Arboleda A, Grajales Urrego DM and Rojas A (2019) Joint Transcriptomic Analysis of Lung Cancer and Other Lung Diseases. Front. Genet. 10:1260. doi: 10.3389/fgene.2019.01260
Received: 13 August 2019; Accepted: 14 November 2019;
Published: 06 December 2019.
Edited by:
Seungchan Kim, Prairie View A&M University, United StatesReviewed by:
Fuhai Li, The Ohio State University, United StatesStefano Forte, Mediterranean Institute of Oncology (IOM), Italy
Copyright © 2019 Otálora-Otálora, Florez, López-Kleine, Canas Arboleda, Grajales Urrego and Rojas. This is an open-access article distributed under the terms of the Creative Commons Attribution License (CC BY). The use, distribution or reproduction in other forums is permitted, provided the original author(s) and the copyright owner(s) are credited and that the original publication in this journal is cited, in accordance with accepted academic practice. No use, distribution or reproduction is permitted which does not comply with these terms.
*Correspondence: Liliana López-Kleine, bGxvcGV6a0B1bmFsLmVkdS5jbw==; Adriana Rojas, cm9qYXMtYWRyaWFuYUBqYXZlcmlhbmEuZWR1LmNv