- 1Department of Entomology and Plant Pathology, North Carolina State University, Raleigh, NC, United States
- 2Department of Biology, University of North Carolina at Greensboro, Greensboro, NC, United States
- 3High Performance Cluster, Office of Information Technology, North Carolina State University, Raleigh, NC, United States
- 4High Performance Computing in Biology, University of Illinois at Urbana-Champaign, Urbana, IL, United States
- 5Army Research Office, Army Research Laboratory, Research Triangle Park, NC, United States
- 6W.M. Keck Center for Behavioral Biology, North Carolina State University, Raleigh, NC, United States
Honey bees (Apis mellifera L.) suffer from many brood pathogens, including viruses. Despite considerable research, the molecular responses and dynamics of honey bee pupae to viral pathogens remain poorly understood. Israeli Acute Paralysis Virus (IAPV) is emerging as a model virus since its association with severe colony losses. Using worker pupae, we studied the transcriptomic and methylomic consequences of IAPV infection over three distinct time points after inoculation. Contrasts of gene expression and 5 mC DNA methylation profiles between IAPV-infected and control individuals at these time points – corresponding to the pre-replicative (5 h), replicative (20 h), and terminal (48 h) phase of infection – indicate that profound immune responses and distinct manipulation of host molecular processes accompany the lethal progression of this virus. We identify the temporal dynamics of the transcriptomic response to with more genes differentially expressed in the replicative and terminal phases than in the pre-replicative phase. However, the number of differentially methylated regions decreased dramatically from the pre-replicative to the replicative and terminal phase. Several cellular pathways experienced hyper- and hypo-methylation in the pre-replicative phase and later dramatically increased in gene expression at the terminal phase, including the MAPK, Jak-STAT, Hippo, mTOR, TGF-beta signaling pathways, ubiquitin mediated proteolysis, and spliceosome. These affected biological functions suggest that adaptive host responses to combat the virus are mixed with viral manipulations of the host to increase its own reproduction, all of which are involved in anti-viral immune response, cell growth, and proliferation. Comparative genomic analyses with other studies of viral infections of honey bees and fruit flies indicated that similar immune pathways are shared. Our results further suggest that dynamic DNA methylation responds to viral infections quickly, regulating subsequent gene activities. Our study provides new insights of molecular mechanisms involved in epigenetic that can serve as foundation for the long-term goal to develop anti-viral strategies for honey bees, the most important commercial pollinator.
Author Summary
Honey bees, the most important managed pollinators, are experiencing unsustainable mortality. Israeli Acute Paralysis Virus (IAPV) causes economically important disease in honey bees, and it is emerging as a model system to study viral pathogen-host interactions in pollinators. The pupation stage is important for bee development but individuals are particularly vulnerable for parasitic mite infestations and viral infections. Currently, it is unclear how honey bee pupae respond to this virus. However, these responses, including gene expression and DNA methylomic changes, are critical to understand so that anti-viral genes can be identified and new anti-viral strategies be developed. Here, we use next-generation sequencing tools to reveal the dynamic changes of gene expression and DNA methylation as pupae succumb to IAPV infections after 5, 20, and 48 h. We found that IAPV causes changes in regions of DNA methylation more at the beginning of infection than later. The activity of several common insect immune pathways are affected by the IAPV infections, as are some other fundamental biological processes. Expression of critical enzymes in DNA methylation are also induced by IAPV in a temporal manner. By comparing our results to other virus studies of honey bees and fruit flies, we identified common anti-viral immune responses. Thus, our study provides new insight on the genome responses of honey bees over the course of a fatal virus infection with theoretical and practical implications.
Introduction
Infectious disease results from the breakdown of an organism’s normal physiological state because of the presence or proliferation of a pathogen. This disruption can be molecularly characterized by transcriptome profiling to permit a systemic understanding of host-pathogen interactions, particularly in combination with other system-level approaches (Arvey et al., 2012; Tanca et al., 2013; Gardy and Loman, 2018). Transcriptomic and epigenomic changes in response to pathogen infections are important to understand because they are interdependent but relate to different temporal dynamics.
DNA methylation of Cytosine (5 mC) is a central epigenetic regulator of gene expression and alternative splicing (Zemach et al., 2010; Li-Byarlay et al., 2013), affecting diverse aspects of organismal function and disease (Edwards and Myers, 2007). Epigenetic programming may also be the target of host manipulations by pathogens (De Monerri and Kim, 2014) and affect host defenses and susceptibility to infection (Merkling et al., 2015; Kuss-Duerkop et al., 2018). The virus induced changes in host immune response and gene expression via DNA methylation is still a new study field. It is understudied whether changing host DNA methylation such as gene expression of certain antiviral immune genes is a main mechanism utilized by DNA or RNA viruses to manipulate immune responses. Genome-wide analyses of methylome and transcriptome indicated that the infection of DNA tumor virus induced hypermethylation of host genes during viral persistence (Kuss-Duerkop et al., 2018). In plants, DNA hypomethylation was reported after 24 h of viral infection (Pavet et al., 2006).
Honey bees (Apis mellifera L.) were the first insects in which a fully functional 5 mC DNA methylation system was discovered (Wang et al., 2006; Kucharski et al., 2008). Social hymenopterans, such as bees, wasps, and ants, have low levels of DNA methylation (Hunt et al., 2010; Glastad et al., 2015; Bewick et al., 2017). The methylated 5 mC sites can be one of three different kinds (CpG dinucleotides, CHG, and CHH, H represents A, T or C) (Lyko et al., 2010). The CpG is the most common kind in honey bees. CpG DNA methylation in insects occurs predominantly in gene bodies, associated with histone modifications and alternative splicing (Lyko et al., 2010; Zemach et al., 2010; Hunt et al., 2013; Li-Byarlay et al., 2013; Glastad et al., 2014; Yan et al., 2014; Glastad et al., 2016). Its phenotypic consequences range from behavioral plasticity (Herb et al., 2012; Opachaloemphan et al., 2018) to alternative development trajectories (Kucharski et al., 2008; Glastad et al., 2019) and disease responses (Galbraith et al., 2015). Especially the study from Herb et al. (2012) showed gene expression differences related to differentially methylated regions (DMRs) and also suggests wider effect because many DMRs are related to transcription factors, in which the alternative splicing may affect their function. Despite this breath of effects, few studies have investigated the role of CpG DNA methylation in insect development, immune system, and disease (Mukherjee et al., 2015; Burggren, 2017; Vilcinskas, 2017; Grozinger and Flenniken, 2019).
Honey bees are the most important managed pollinator in agriculture and crop production (Calderone, 2012). However, recent declines in honey bee health have led to unsustainably high annual colony losses in the apicultural industry (Calderone, 2012; Kulhanek et al., 2017) for which multiple, potentially interacting factors are likely the cause (Cornman et al., 2012; Goulson et al., 2015). One major contributor to widespread colony mortality is pathogenic viral infection. Many of the most important viruses are either associated with or can be directly vectored by parasitic Varroa mites (Bailey et al., 1963; Chen and Siede, 2007; Di Prisco et al., 2011; Gisder and Genersch, 2017). Pathogenic viral stressors affect the morphology, physiology, behavior, and growth of honey bees at different developmental stages (Chen and Siede, 2007; Runckel et al., 2011; Cornman et al., 2012; McMenamin and Genersch, 2015), ultimately leading to reduced colony productivity and mortality. The developmental pupal stage is critical for many viral diseases because the Varroa mite feeds on the pupae for a prolonged time (Di Prisco et al., 2011).
Honey bees employ a combination of individual- and group-level defenses against pathogens (Wilson-Rich et al., 2009). The main innate immune pathways of insects are present in honey bees and respond to virus infections (Evans et al., 2006; Brutscher et al., 2015), including the Toll, Janus kinase and Signal Transducer and Activator of Transcription (JAK-STAT), Immune Deficiency (Imd), c-Jun NH2-Terminal Kinase (JNK) pathways, antimicrobial peptides (AMPs), endocytosis, and phagocytosis mechanisms (Brutscher et al., 2015). Activation of some immune pathways, such as the RNAi mechanism, can lead to increased virus resistance (Brutscher et al., 2017). Thus, we understand that some honey bee genes respond to virus infection but a systematic characterization of the temporal dynamics of the immune responses and the general transcriptome and methylome have not been investigated, although such studies can yield important information (Lemaitre and Hoffmann, 2007).
Israeli Acute Paralysis Virus (IAPV) has been associated with colony losses (Cox-Foster et al., 2007; Maori et al., 2009; Tantillo et al., 2015), particularly during the winter (Chen et al., 2014). IAPV belongs to the picornavirus-like family Dicistroviridae, that includes single-strand, positive sense RNA viruses that are pathogenic to a range of insects (Bonning and Miller, 2010). IAPV is relatively common in honey bees and affects all life history stages and castes (Chen et al., 2014). When cells are infected, the positive strand of RNA is released into the cytosol and translated by the host ribosomes. Then the assembled complexes for viral replication synthesize negative-sense RNA from which more descendant positive strands of the RNA are produced. These are assembled with viral proteins into virions that are then released to infect other cells (Nagy and Pogany, 2012; Chen et al., 2014). Covert infections persist through vertical and horizontal transmission, but IAPV can also readily cause paralysis and death of infected individuals (Maori et al., 2007). Acute infections of IAPV profoundly affect cellular transcription at the pupal stage (Boncristiani et al., 2013), and microarray analyses of larvae and adults indicate significant and stage-specific responses to IAPV infection (Chen et al., 2014). Parallel transcriptomic and epigenomic profiling of adult IAPV infection identified some additional molecular responses, including expression changes in immune genes and epigenetic pathways (Galbraith et al., 2015). However, little overlap of identified responses within and among studies leave the molecular characterization of IAPV infection in honey bees unresolved, and more detailed studies of dynamic responses in the time-course of IAPV infection are needed.
Here, we experimentally test the hypothesis that viral infections can affect not only transcriptomic profiles but also induce DNA methylomic changes in honey bee pupae. Specifically, we compared the complete transcriptome and methylome profiles across three distinct phases of a lethal IAPV infection. Furthermore, we tested if our results indicate significant overlap of immune genes with fruit flies as well as other stages of honey bees under IAPV viral infections.
Results
Temporal Viral Infections Cause Transcriptomic Changes in Gene Expressions
The transcriptomes of whole honey bee pupae injected with PBS or infected with IAPV were compared at three time points: 5, 20, and 48 h post-injection (Figure 1). On average, IAPV-infected pupae yielded slightly more RNA-seq reads than the corresponding PBS controls (5 h: IAPV = 46,001,777 vs. PBS = 41,666,177; 20 h: IAPV = 47,915,086 vs. PBS = 42,429,737; and 48 h: IAPV = 42,649,987 vs. PBS = 39,248,297). Coverage thus varied among individual samples from 41x to 65x (Supplementary Table 1). Differentially expressed genes (DEGs) increased with disease progression over time from 432 after 5 h to 5,913 after 20 h and 5,984 after 48 h (Supplementary Table 2).
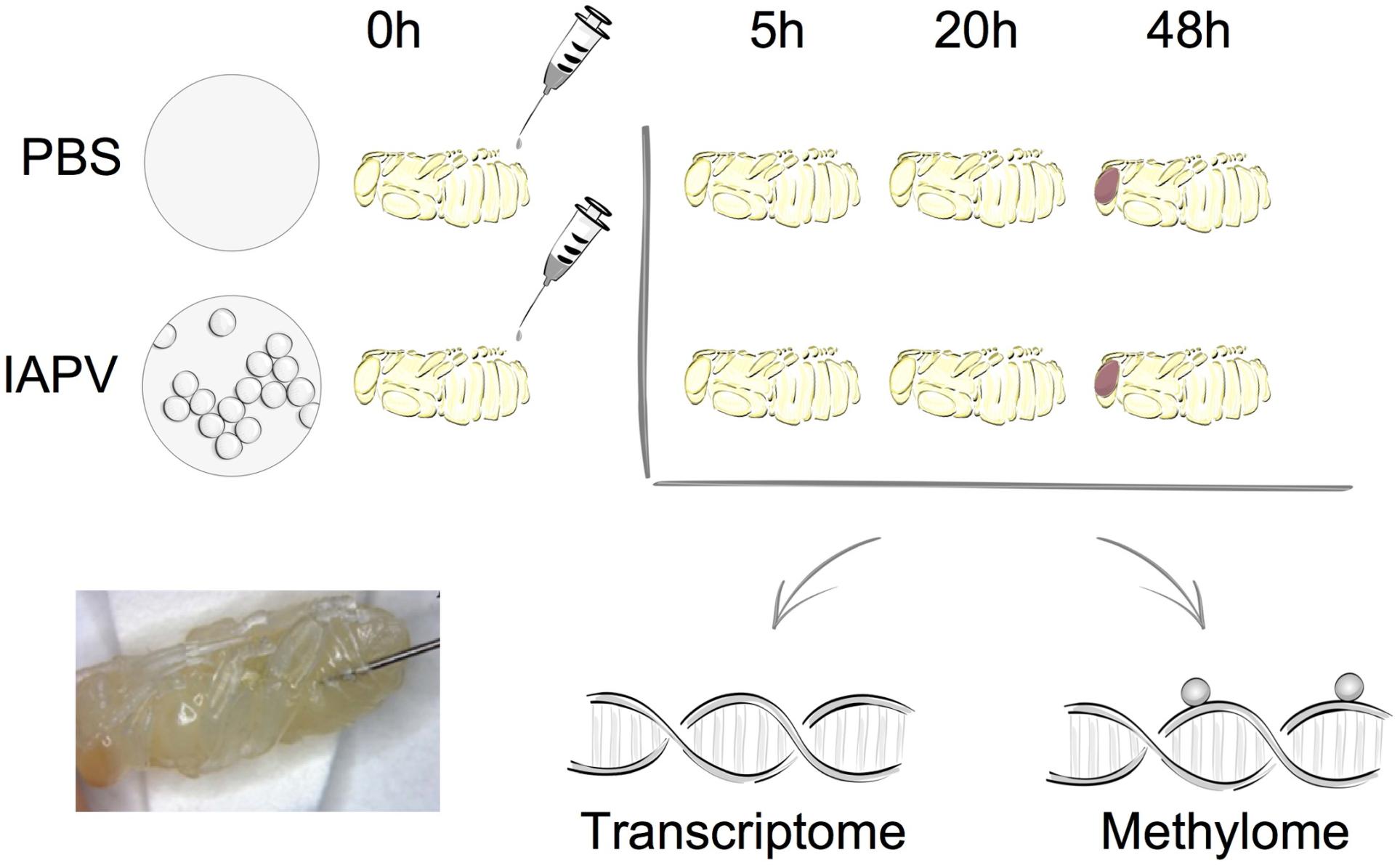
Figure 1. Overall experimental design. The two experimental groups included either sham treatment (PBS injection) or Israeli Acute Paralysis Virus (IAPV) inoculations via injection. At each investigated time point (5, 20, or 48 h post-injection) three biological replicates were collected, each consisting of one whole pupa that was individually processed for further transcriptomic and methylomic analyses.
Relative to the PBS control, IAPV-infected pupae had 198 genes significantly up-regulated and 234 genes significantly down-regulated after 5h; 2,738 genes were significantly up-regulated and 3,175 genes significantly down-regulated after 20 h; and 3,021 genes significantly up-regulated and 2,963 genes significantly down-regulated after 48 h (Supplementary Figures 1–3). DEG overlap between all significantly up- or down-regulated genes was significant in all pairwise directional comparisons (up- and down-regulated genes separately) among the three time points (Figure 2; up-regulated 5 h vs. 20 h: p < 0.05; all other tests: p < 0.001). For the > 8-fold DEGs, significant overlap was found for all pairwise comparisons among the IAPV up-regulated gene sets (p < 0.001) and also for the down-regulated gene sets at 20 and 48 h (p < 0.001) (Supplementary Table 2).
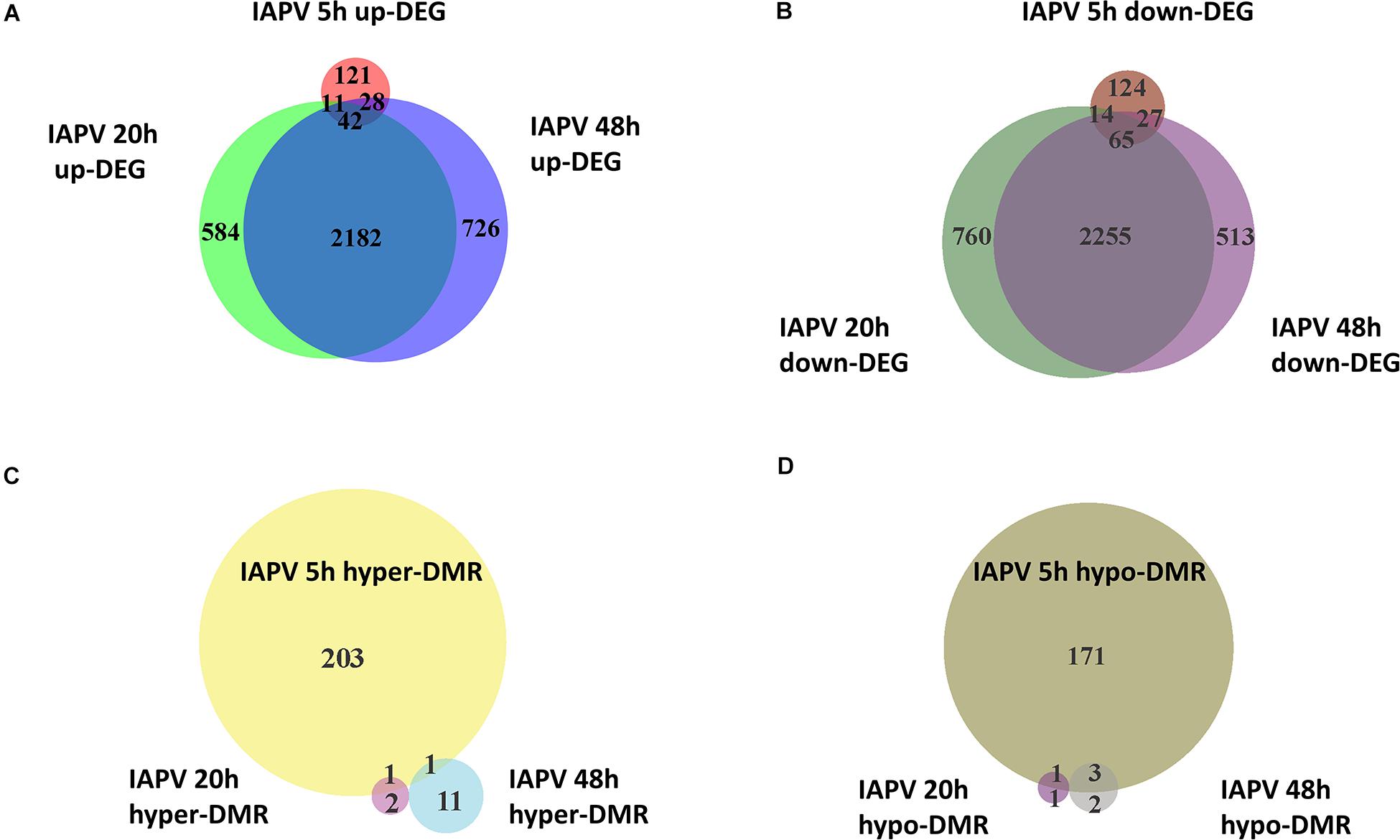
Figure 2. Directional overlap of differentially regulated genes among time points. (A) Venn diagram showing genes that were differentially expressed as up-regulated (FDR < 0.05). Number of DEGs only in post IAPV 5, 20, and 48 h are 121, 584, and 726. Number of genes between IAPV 5 and 20 h only overlap is 11. Number of DEGs between IAPV 5 and 48 h only overlap is 28. Number of DEGs between IAPV 20 and 48 h only overlap is 2182. Number of DEGs from IAPV 5–20–48 h overlap is 42. (B) Venn diagram showing genes that are differentially expressed as down-regulated (FDR < 0.05). Number of DEGs only in post IAPV 5, 20, and 48 h are 124, 760, and 513. Number of DEGs between IAPV 5 and 20 h only overlap is 14. Number of DEGs between IAPV 5 and 48 h only overlap is 27. Number of DEGs between IAPV 20 and 48 h only overlap is 2255. Number of DEGs from IAPV 5–20–48 h overlap is 65. (C) Venn diagram showing genes that are differentially methylated (percent methylation difference larger than 10%, q < 0.01) as hyper-methylation among three time points (5, 20, and 48 h after IAPV infection). Number of DMRs only in post IAPV 5, 20, and 48 h are 203, 2, and 11. Number of DMRs between IAPV 5 and 20 h only overlap is 1. Number of DMRs between IAPV 5 and 48 h only overlap is 1. (D) Venn diagram showing genes that are differentially methylated (percent methylation difference larger than 10%, q < 0.01) as hypo-methylation among three time points (5, 20, and 48 h after IAPV infection). Number of DMRs only in post IAPV 5, 20, and 48 h are 171, 1, and 2. Number of DMRs between IAPV 5 and 20 h only overlap is 1. Number of DMRs between IAPV 5 and 48 h only overlap is 3.
Gene Enrichment and Functional Analyses
To identify IAPV-induced expression patterns across all genes regardless of significance threshold, GO Mann-Whitney U-tests and a Weighted Gene Co-expression Network Analysis were performed on the expression differences between IAPV infected and control samples at three time points. Post 5h of IAPV infection, multiple biological process GO terms were observed with upregulation of RNA processing (ncRNA, tRNA, and rRNA) as the most significant GO terms (p < 0.001). Only one term was significantly downregulated at this time point: “small GTPase mediated signal transduction.” 20 h post IAPV infection, no GO terms passed the strictest significance threshold (p < 0.001) for upregulation, and GO term “translation” was the most significantly downregulated (p < 0.001). Finally, in post 48h IAPV infected samples, there was still no term passing the strictest significance in upregulation, and GO terms of “translation,” “homophilic cell adhesion,” and “aminoglycan metabolic process” were the most significantly downregulated (Figure 3). As to molecular function, significant GO terms associated with downregulation in post 20 and 48 h IAPV infected samples were “structural molecules,” “structural constituent of ribosome,” “structural constituent of cuticle,” and “chitin binding” (Supplementary Figure 4). As to cellular component, significant GO terms associated with downregulation in post 20 and 48 h IAPV infected samples were “ribosomal subunit,” “ribonucleoprotein complex,” and “intracellular non-membrane organelle” (Supplementary Figure 4). Although numerous other terms were less significantly enriched in up- or down-regulated genes, no other GO terms surpassed the most stringent significance criterion.
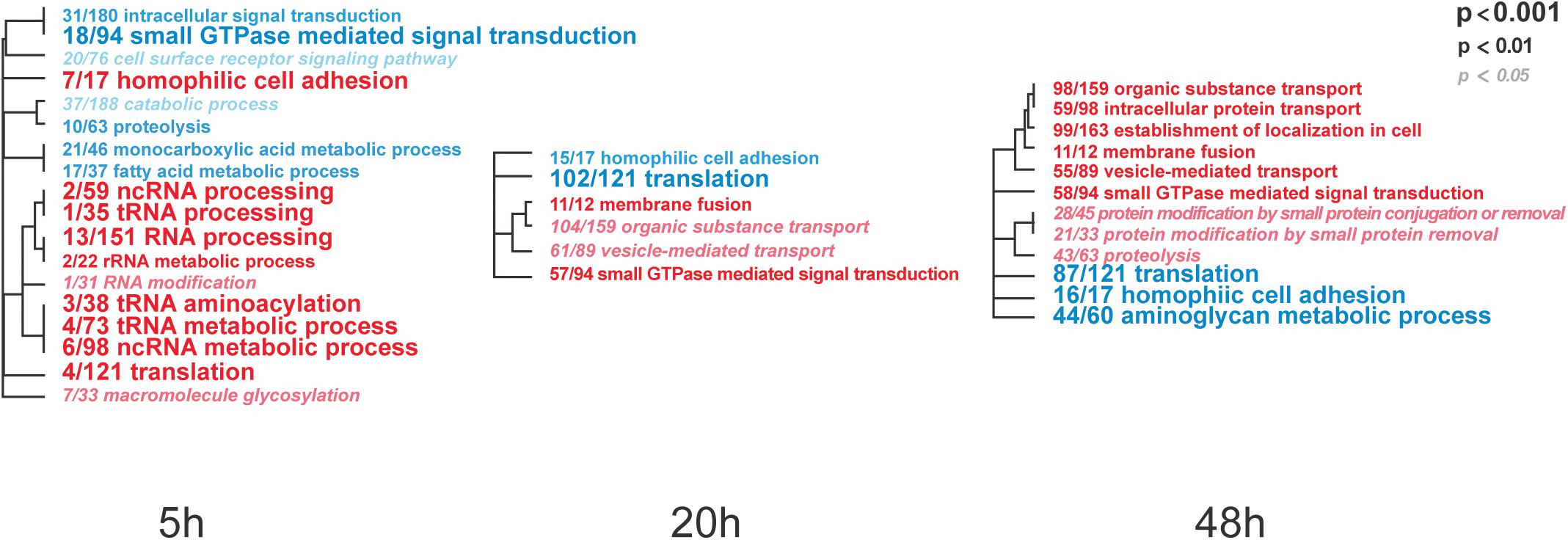
Figure 3. Overall trends in biological processes affected by progressing IAPV infection. Gene Ontology Mann-Whitney U tests of the expression differences between IAPV and control samples at the three time points included all data points regardless of their individual level of statistical significance. Red and blue fonts indicate GO terms that are enriched in up- and down-regulated DEGs in IAPV relative to control samples, respectively. Font size indicates the level of significance for the particular GO term and clustering is based on empirical GO-term similarity in our dataset.
Viral and Ribosomal RNA Content
Four transcripts (IAPV, Deformed Wing Virus (DWV), Large Sub-Unit rRNA (LSU rRNA), and Small Sub-Unit rRNA (SSU-rRNA) accounted for 63.5% of all sequenced reads (Supplementary Figure 5). As expected, a large number of IAPV reads was observed in the IAPV-infected pupae, with the proportion being similarly high at 20 h (86% ave) and 48 h (90% ave). Additionally, five of nine control samples (all time points included) had a large proportion of reads mapping to DWV.
Comparative Transcriptomic Analyses of Immune Genes With Other Pathogen Infection in Honey Bees and Drosophila
A comparison with a previously published list of 186 immune genes from Evans et al. (2006) showed that 1 and 89 of them were represented in our 5 h, 20 h/48 h DEG lists respectively (Figure 4A and Supplementary Tables 3, 4). The overlap between immune genes and our DEGs was significant for up-regulated DEGs at 5 h and down-regulated DEGs at 20 h (Supplementary Tables 3, 4). Comparison of our data with IAPV-induced gene expression changes in adult honey bees in Galbraith et al. (2015) revealed 6 and 358 genes overlapped, indicating a significant directional overlap of our up- and down-regulated DEGs at 5 h and 20 h/48 h with DEGs in adults (Figure 4C, for specific gene names, see Supplementary Tables 3, 4). When compared to immune genes associated with anti-viral responses in Drosophila (Xu et al., 2012), 11, 212, and 219 genes overlapped in our 5h, 20h, and 48h DEG lists (Supplementary Table 5), representing significant directional overlap of our up- and down-regulated DEGs at 20 h and 48 h (for specific gene names see Supplementary Table 5).
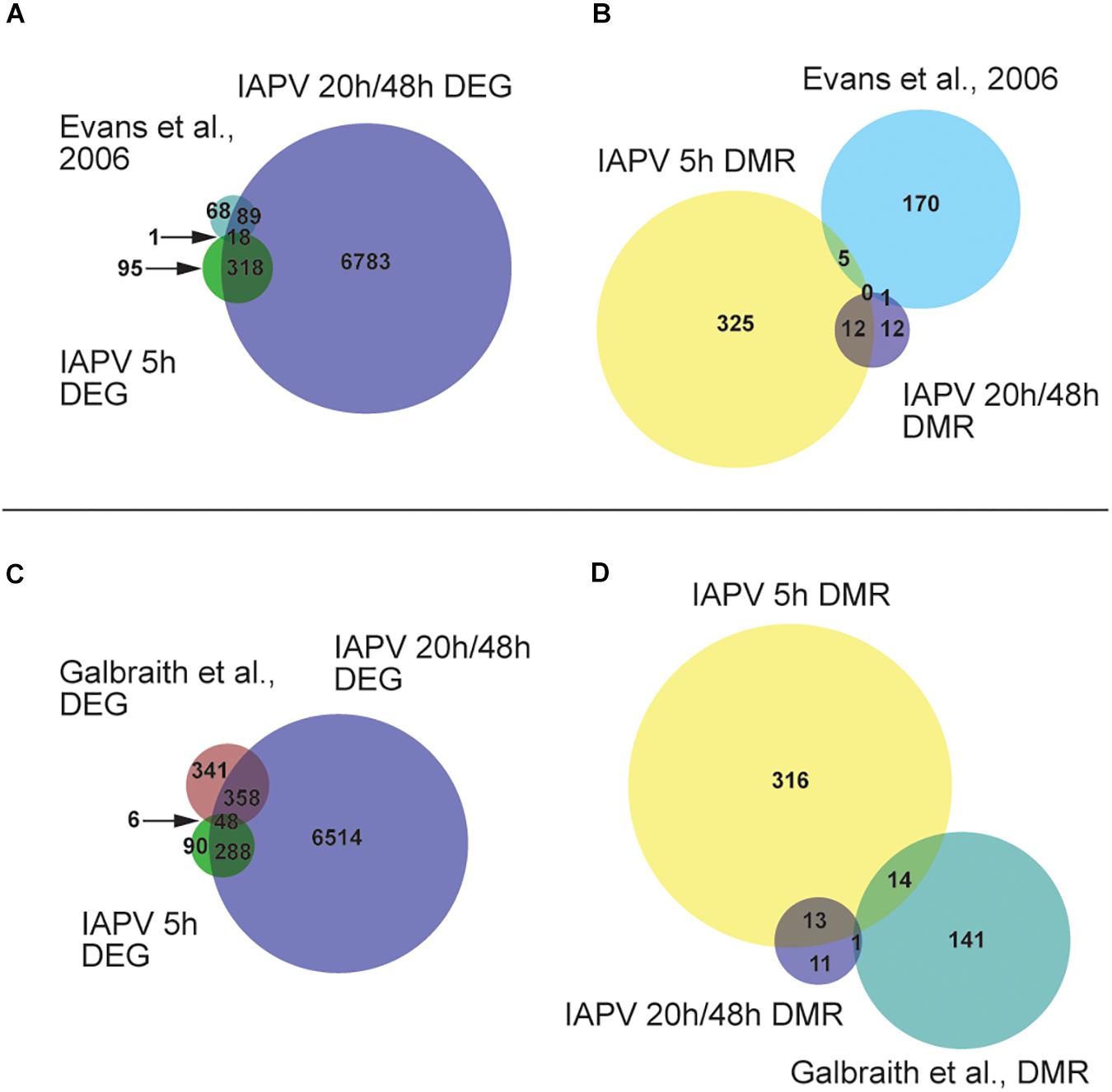
Figure 4. Comparisons or our results to other studies revealed significant overlap. (A) Venn diagram showing overlap of genes that are differentially expressed (FDR < 0.05; p < 0.05). Number of DEGs (differentially expressed genes) only in IAPV 5, 20/48 h, or Evans et al. (2006) immune gene list is 95, 6783, or 68. Number of DEGs overlap between IAPV 20 h/48 h and Evans et al. is 89. Number of DEGs overlap between IAPV 5 and 20 h/48 h is 318. Number of DEGs overlap between IAPV 5 h and Evans et al. is 1. (B) differentially methylated (>10%, p < 0.05) among three time points (5, 20, and 48 h after viral infection) and immune gene list from Gisder and Genersch (2017). Number of DMRs (differentially methylated regions) only in IAPV 5, 20/48 h, or Evans et al. (2006) immune gene list is 325, 12, or 170. Number of DMRs overlap between IAPV 20 h/48 h and Evans et al. is 1. Number of DMRs overlap between IAPV 5 and 20/48 h is 12. Number of DMRs overlap between IAPV 5 h and Evans et al. is 5. (C) Venn diagram showing overlap of DEGs (FDR < 0.05; p < 0.05) compared to Galbraith et al. Number of DEGs only in IAPV 5, 20/48 h, or Galbraith et al. is 90, 6514, or 341. Number of DEGs overlap between IAPV 20 h/48 h and Galbraith et al. is 358. Number of DEGs overlap between IAPV 5 and 20 h/48 h is 288. Number of DEGs overlap between IAPV 5 h and Galbraith et al. is 6. (D) differentially methylated (>10%, p < 0.05) among three time points (5, 20, and 48 h after IAPV infection) and immune gene list from Galbraith et al. DMR list. Number of DMRs (differentially methylated regions) only in IAPV 5, 20 h/48 h, or Galbraith et al. DMR list is 316, 11, or 141. Number of DMRs overlap between IAPV 20 h/48 h and Galbraith et al. is 1. Number of DMRs overlap between IAPV 5 and 20 h/48 h is 14. Number of DMRs overlap between IAPV 5 h and Galbraith et al. is 13.
Temporal Dynamics of Genes and Molecular Pathways Based on Transcriptomic Data
Analyses of the three infection time points revealed dramatic changes of gene expression levels from several key metabolic and immune pathways, including lipid metabolism, the Jak-STAT pathway, and AMPs. With regards to lipid metabolism, the expression of the gene Cyp6as5 was significantly increased in the infected samples from 5 to 20 h, and from 20 to 48 h, similarly to 9 more genes that displayed similar expression profiles (Supplementary Figure 7).
In the Jak-STAT immune pathway, the expression of gene SoS was significantly increased in the infected samples from 20 to 48 h (Supplementary Figure 6). Nine more genes displaying similar expression profiles as SoS after viral infection were identified (Supplementary Figure 7). Among the AMP genes, the expression of gene defensin-1 was significantly increased in the infected samples from 5 to 20 h, and from 20 to 48 h (Supplementary Figure 6). The gene expression of EGFR and a group of other genes were transiently downregulated from 5 to 20 h post-infection and from 20 to 48 h post-infection by IAPV (Supplementary Figure 7). Gene GB55029 (uncharacterized) showed an increase from 5 to 20 h infection, but then a significant decrease of expression from 20 to 48 h infection (Supplementary Figure 7).
Differentially Spliced Genes
Number of genes with significant isoform switches in samples 5, 20, and 48 h post IAPV infection were 193, 620, and 990 (Supplementary Figure 8 and Supplementary Table 6). Number of significant differential transcripts usage (DTU) or alternative splicing is much higher post 20 and 48 h of IAPV infection (Supplementary Figure 9). All the splicing events are categorized in Table 1. Among all the categories, there are significant trends of DTU to be in shorter 3′ UTRs, longer 5′ UTRs, Exon gain, Transcription Start Site (TSS) more downstream in 20 and 48 h IAPV post infection treatments (Figure 5).
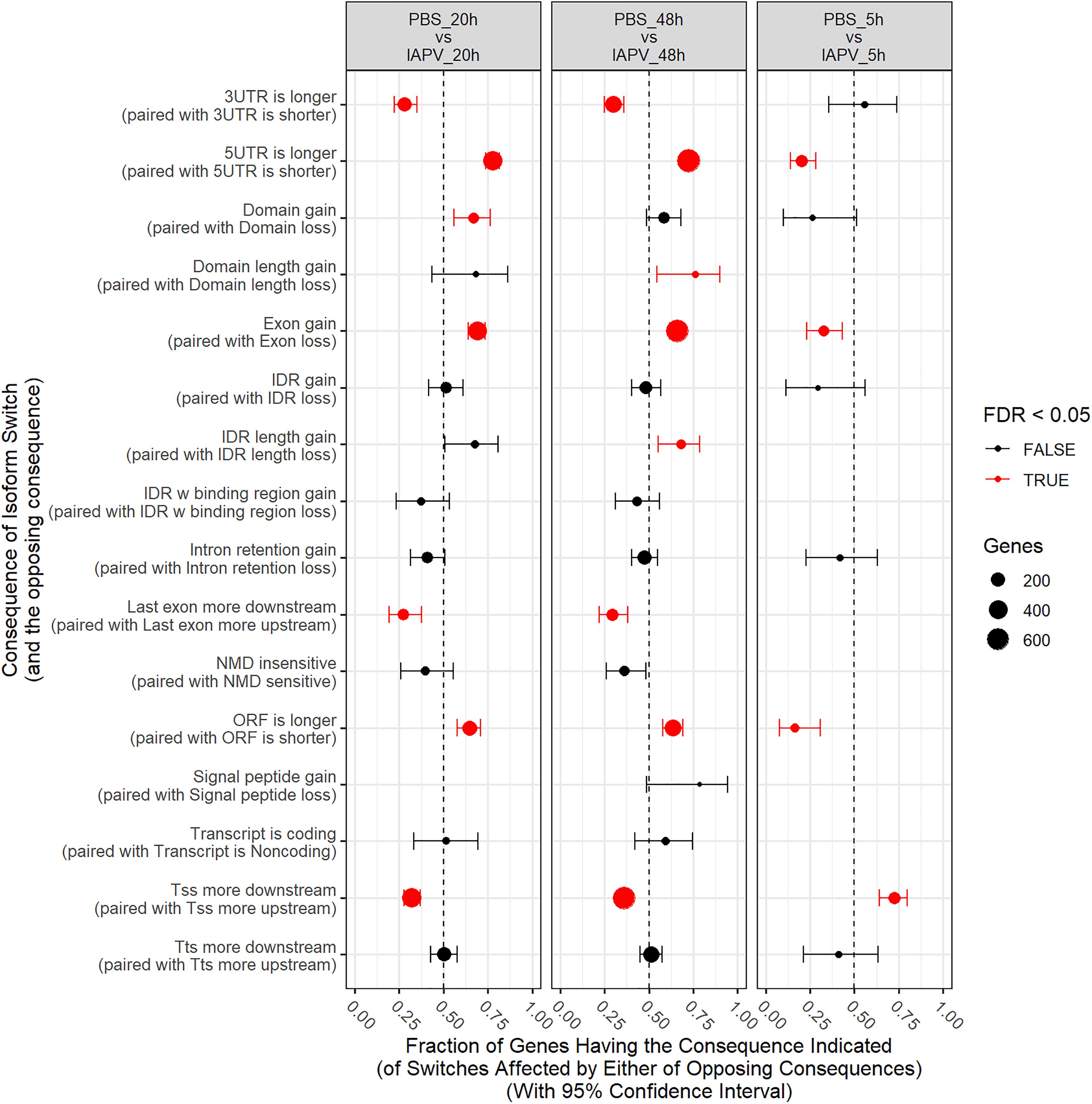
Figure 5. Significant trends in consequences of isoform switches or alternative splicing were observed, and were generally similar at 20 and 48 h, often with opposite consequences at 5 h. Fraction of genes having the consequence indicated with 95% Confidence Interval. UTR-untranslated region, ITR-Inverted Terminal Repeat, ORF-open reading frame, NMD- nonsense-mediated decay, TSS-transcription start site, TTS-transcription terminal site.
Enzymes Involved in DNA Methylation
Gene expression differences were also identified in the DNA methylation system, specifically the two important enzymes, DNA methyl-transferase 1 (DNMT1) and 3 (DNMT3). When compared between IAPV and control samples, DNMT1 (GB47348) was significantly down-regulated 1.5-fold (p < 0.001) at 20 h post-infection, and 1.7-fold (p < 0.001) at 48 h post-infection (Figure 6). Similarly, DNMT3 (GB55485) was significantly down-regulated 1.7-fold (p < 0.001) in 20 h post-infection samples, and 2.7-fold (p < 0.001) in 48 h post-infection samples (Figure 6). There was no significant difference of gene expression between IAPV and controls post 5 h post-viral infection (Figure 6).
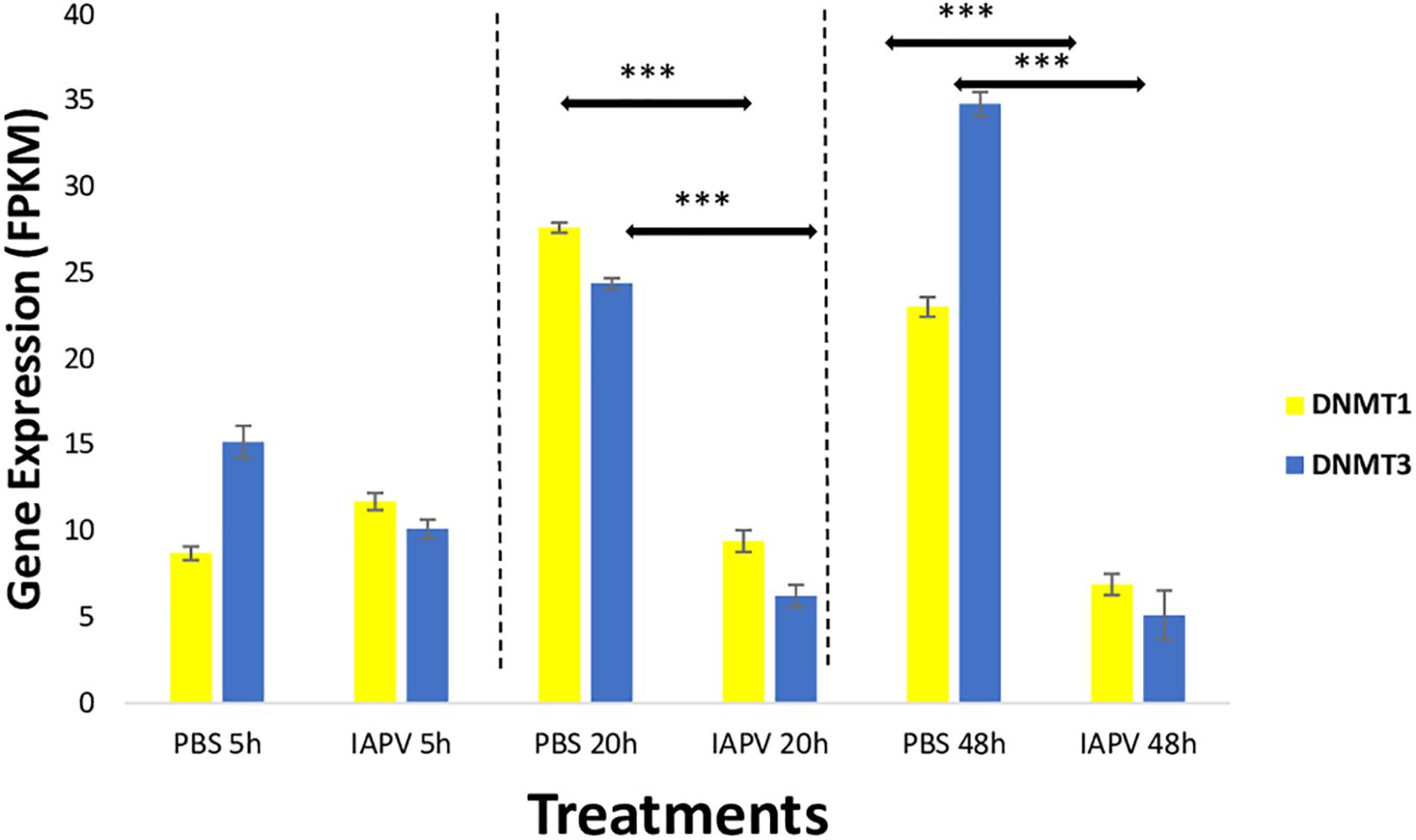
Figure 6. Gene expression patterns (mean ± SE) of the major DNA Methyl-Transferases (DNMTs). Both DNMT1 (GB47348) and DNMT3 (GB55485) showed significant down-regulation when comparing sham control (N = 3) and IAPV samples (N = 3) after 20 and 48 h after IAPV infection respectively. ∗∗∗p < 0.001. False Discovery Rate (FDR) < 0.05.
Changes of DNA Methylation After Viral Infections and Comparative Methylomic Analysis
Total methylated 5 mC sites in CpG, CHG, and CHH settings were 1,955,471; 878,838; and 1,433,905 respectively (Figure 7). The ratio of methylated CpG sites versus total CpG sites was highest in 5 h PBS control (10%), followed by 5 h IAPV post-infection samples, 20 h PBS control, 20 h IAPV post-infection samples, 48 h control, and lowest in 48 h IAPV post-infection samples (about 4%) (Figure 7). The whole genome-wide Pearson correlation matrix for CpG base profiles across all samples at each time point (5, 20, and 48 h post-infection) are listed in Supplementary Figure 10.
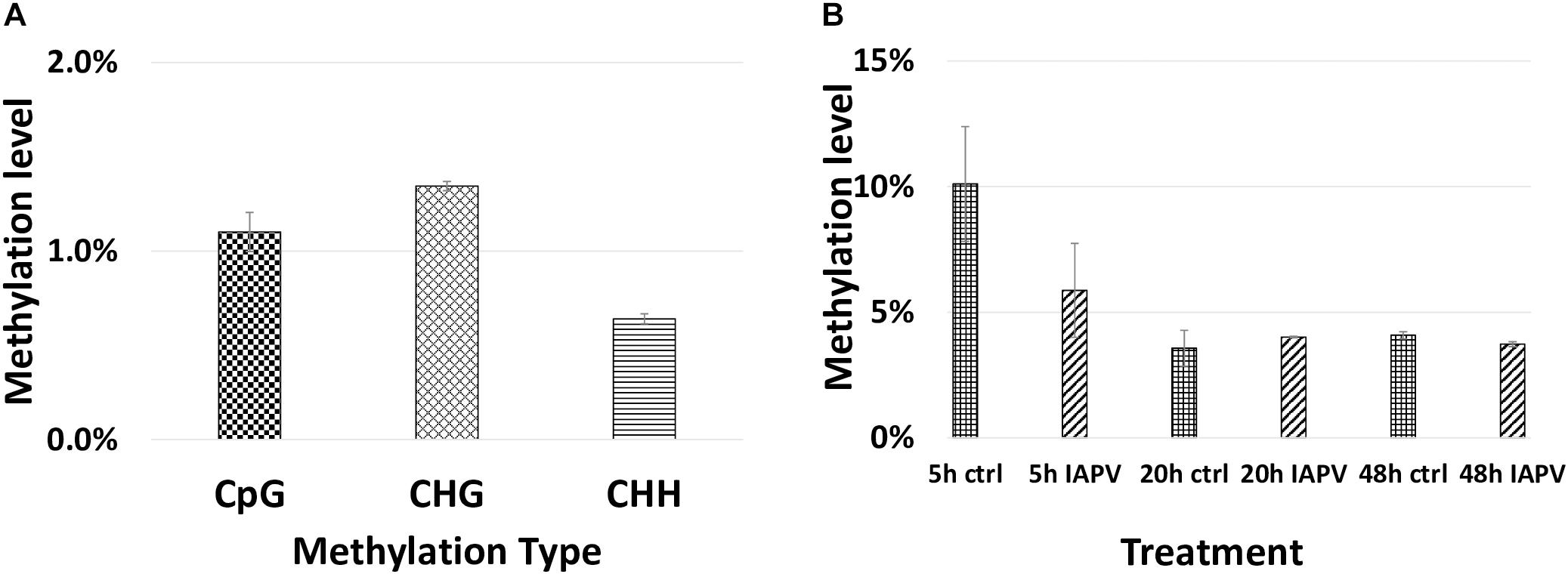
Figure 7. Genome wide methylation patterns. (A) Total methylated 5 mC sites in each group (CpG, CHG, and CHH) are shown. (B) Methylated CpG sites/Total CpG sites are shown for each treatment and time point.
We identified 523, 5, and 18 differentially methylated regions (DMRs) between IAPV and control groups in the 5, 20, and 48 h post-infection samples, respectively (Supplementary Table 7 for a detailed gene list). Significant overlap of DMRs existed between 5 and 20 h (p < 0.05) and 5 and 48 h (p < 0.0001) comparisons (Supplementary Table 4).
Our comparison of our DMR lists with the previously published immune genes of Evans et al. (2006) revealed 5 and 1 gene were overlapping with the DMRs at 5 h and 20 h/48 h post-infection, respectively (Figure 4B and Supplementary Tables 3, 4). Comparison of our data with IAPV-induced methylation changes in adult honey bees of Galbraith et al. (2015) revealed 14 and 1 genes were overlapped with DMRs at 5 h and 20 h/48 h (Figure 4D, for specific gene names see Supplementary Tables 3, 4).
We examined the genome-wide Pearson correlation matrix for CpG base profiles across all samples at each time point. Overall samples after 5 h infection have the highest correlation (0.72–0.87), and samples after 48 h infection have the lowest correlation (0.69–0.73); specific coefficients are listed in Supplementary Figure 10).
Differential DNA Methylation Leads to Differential Gene Expression Later in Several Immune Pathways
To look into the potential association between gene expression and CpG methylation, we studied whether genes that showed early changes of CpG methylation also exhibited significant changes of gene expression later. A list of 38 immune genes that were hypo- or hyper-methylated at the first time point (5 h post-IAPV infection), were found differentially expressed later (48 h post IAPV infection) (Supplementary Table 8). These cellular pathways included the MAPK (mitogen-activated protein kinase) pathway, JAK/STAT (Janus kinase/signal transducer and activator of transcription) pathway, Hippo signaling pathway, mTOR (mammalian target of rapamycin) signaling pathway, TGF-beta (transforming growth factor-beta) signaling pathway, ubiquitin mediated proteolysis, and spliceosome. Interestingly, we detected 12 genes from this group with differentially spliced patterns with a significant statistical q value (Table 2).
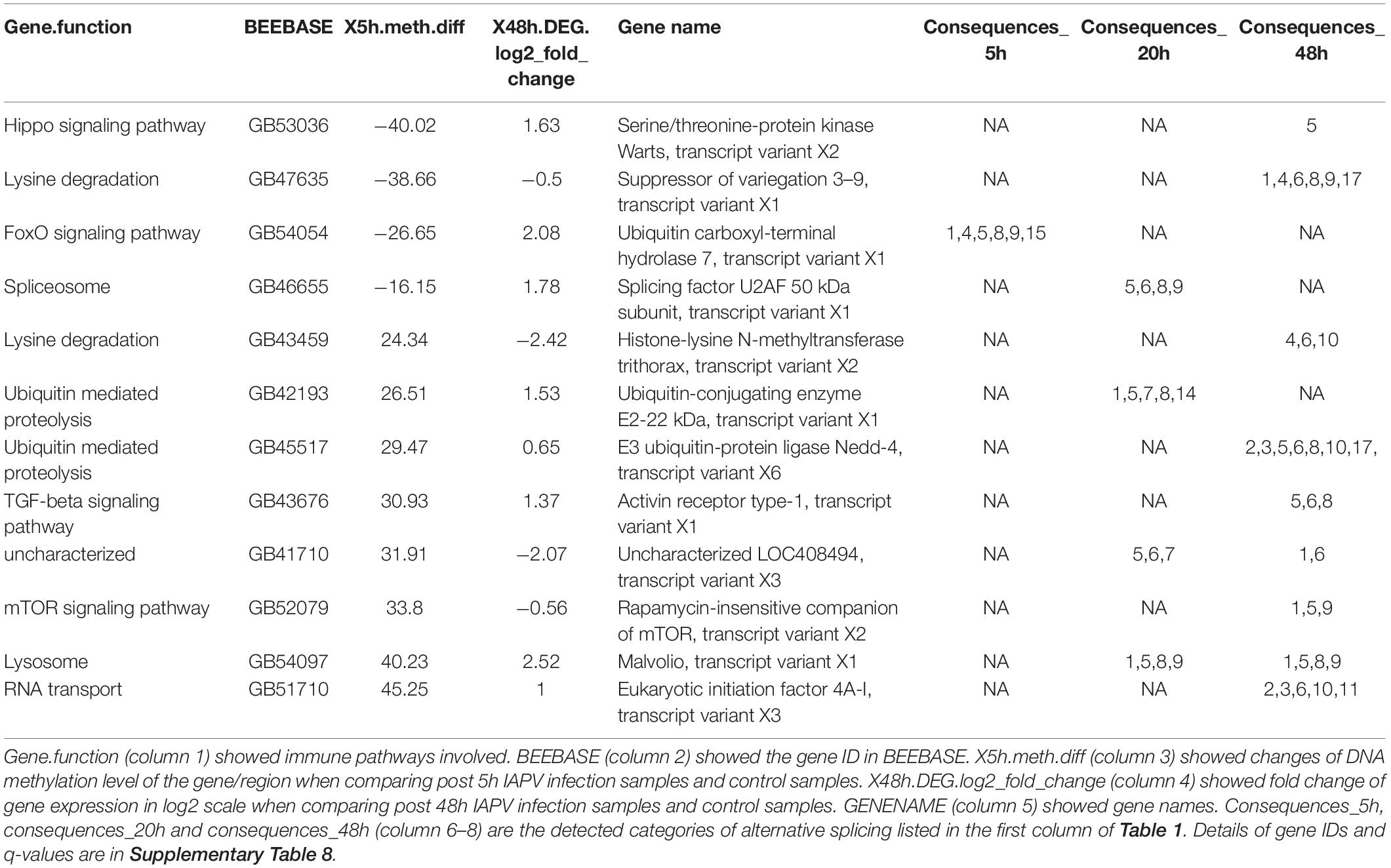
Table 2. A list of twelve immune genes that were hypo- or hyper-methylated first post 5 h of viral infection, then gene expression and splicing patterns changed significantly post 48 h of viral infection (q < 0.05).
Comparative Methylomic Analyses of Immune Genes With Other Pathogen Infection
The comparison with a previously published list of 186 immune genes from Evans et al. (2006), showed that 5 (GB45248, GB46478, GB55483, GB51399, and GB41850) and 1 (GB46478) genes overlapped in our 5h and 48h DMR lists, respectively (Supplementary Table 3). There was no significant directional overlap of the list of immune genes and our hyper- or hypo-regulated DMRs at 5, 20, and 48 h (Supplementary Tables 3, 4). Comparison of our data with IAPV-induced gene expression changes in adult honey bees in Galbraith et al. (2015) revealed 19, 1 (GB51998), and 1 genes (GB42022) overlapped, indicating no significant directional overlap of our hyper- or hypo-regulated DMRs at 5, 20, and 48 h (Supplementary Tables 3, 4). When compared to immune genes associated with anti-viral responses in Drosophila (Xu et al., 2012), 20, 1 (GB17743), and 1 genes (GB15242) overlapped in our 5, 20, and 48 h DMR lists (Supplementary Table 5).
Motif
To reveal the potential regulation of CpG methylation, we examined the 6-mer motif of each CpG site, including the 2 nucleotides upstream and downstream of each CpG site. Analysis of 6-mer motifs from 5 h DMRs showed no common pattern of the motif (Supplementary Figure 11). Similarly, analysis of motifs from 20 h DMRs containing CpG sites revealed little signal beyond the selected central CpG sites in the middle of 6-mer motifs. However, DMRs from 48 h post- IAPV infection tend to have a T or C following the central CpG (Supplementary Figure 11).
Discussion
To test our hypothesis that viral infections not only affects gene expression profiles but also DNA methylomic marks in honey bee pupae, we compared the complete transcriptome and methylome responses across three distinct phases (5, 20, and 48 h) of a lethal IAPV infection. While methylation is most affected early during the infection, differential gene expression increases over time and severity of the infection. Many up- and down-regulated genes are involved in RNA processing and translations, proteolysis, and metabolic processes (Figure 3). A collection of genes have differential transcript usage or alternative splicing post IAPV infections (Figure 5). When compared to differentially expressed genes from adult honey bee and fruit fly post viral infection, we have discovered significant overlap of our IAPV-responsive genes with immune genes from these other studies (Evans et al., 2006).
The pupal stage is a critical phase for metamorphosis and an important temporal window for Varroa mite parasitism. Our study, which incorporates and confirms the separate observations of adult or larval honey bees (Cox-Foster et al., 2007; Cornman et al., 2013; Chen et al., 2014; Galbraith et al., 2015), includes new observations that provide a more complete understanding of how the pupae respond to lethal viral attack. Previous studies showed that honey bee pupae can respond to disease with increased immune gene expression to decrease the success of parasites and pathogens (Kuster et al., 2014; Brutscher et al., 2017). Our results reveal that rapid epigenetic changes in response to viral infection precede and presumably trigger profound and widespread transcriptome responses in honey bees. A combination of both transcriptomics and methylomics at multiple time points can reveal the role of and the relation between 5 mC methylation and gene expression profiles under viral infection of insects (Huang et al., 2019).
Alternative splicing in response to viral infection can affect subsequent gene expression through several mechanisms (De Maio et al., 2016). Our results of differential transcript usage or alternative splicing indicated the viral infection can cause large changes in alternative splicing of the bee hosts, which brings a new perspective on molecular mechanisms of IAPV virus pathogenesis. It is known that RNA virus can alter host mRNA splicing (Dubois et al., 2014; Chan et al., 2019). Previous research revealed that DNA methylation is linked to regulate alternative splicing in honeybees via RNA interference knocking down DNMT3 (Li-Byarlay et al., 2013).
The epigenetic changes we report here are interesting because the 5 mC methylome profile indicated a large amount of DMRs after 5 h IAPV infection. However, DMR number dramatically dropped after 20 h and 48 h of infection. This phenomenon may be explained by the cell death and apoptosis after severe infections after 20 and 48 h. We propose a possible explanation is that 5 mC changes as a primary host-defense mechanism responding to viral infection at the onset. During the early stage of viral infection, 5 mC methylation may be the first to react leading to molecular changes genome-wide, potentially interacting with the transcription process such as transcription factor binding. The mechanism on how to induce abnormal methylation at the molecular and cellular levels by viral infection is yet to be determined. A few potential explanations are that pathogenic infection may lead to cellular proliferation and inaccuracy in epigenetic processes, or molecular signaling pathways involved in the infection response affect these epigenetic processes. Previous studies showed that viral infection can change 5 mC methylation in human cancer (Kusano et al., 2006; Toyota and Yamamoto, 2011). Other studies also showed that changes in epigenetics marks can affect immune responses, cell-cycle checkpoints, cell death, and cell fate (Toyota and Yamamoto, 2011; Turner and Diaz-Munoz, 2018).
In honey bees, some evidence links 5 mC methylation marks to gene regulation (Kucharski et al., 2008; Li-Byarlay et al., 2013; Wang and Li-Byarlay, 2015; Li-Byarlay, 2016; Glastad et al., 2019) but limited reports showed 5 mC methylation are associated with change of gene expressions (Herb et al., 2012). The data here indicates that 38 of the initially differentially methylated genes subsequently exhibit significant changes of gene expression (Table 1), and 12 of these genes displayed significant changes in their splicing events. In general, the epigenetic control of 5 mC methylation and gene expression has been reported for plants and mammals (Allis and Jenuwein, 2016; Saripalli et al., 2020), but the function of 5 mC methylation in the regulation of genes in insects is still not clear (Li-Byarlay et al., 2013; Wang and Li-Byarlay, 2015). One hypothesis is that DNA methylation is involved in cell signaling or involved in the regulation of co-transcription via transcription factors (Toyota et al., 2009; Shukla et al., 2011; Marina et al., 2016).
DNA methyl-transferases 1 and 3 are critical enzymes for a functional methylation system. Our data show the IAPV infection reduced the gene expression of these two key enzymes over time. This finding is consistent with previous reports showing that viral infection can change the expression of DNMTs in animal and cell studies (Fang et al., 2012; Tian et al., 2013). The significant fold change of these enzymes is moderate but sufficient to cause dramatic changes in the global DNA methylome, as indicated in previous studies (Wang et al., 2006; Kucharski et al., 2008; Li-Byarlay et al., 2013). The DNA methylation changes in turn can have further consequences for widespread gene expression patterns. We found more DMR post 5 h IAPV infection, when the DNMTs are not yet differently expressed. Possible explanation is that the initial viral infection induced the methylation changes at the initial stage by other potential molecular mechanism such as histone modifications (Paschos and Allday, 2010; Dantas Machado et al., 2015). DNMTs may be regulated to restore homeostasis which may explain why fewer DMRs at the later stages (Matilainen et al., 2017).
At every time-point, we identified numerous differentially expressed genes that confirmed the profound consequences of IAPV infection (Boncristiani et al., 2013). Such effects can certainly be caused by cell expansion or loss, rather than direct effects on gene activity. However, the systemic consequences for the health and function of the organism may be similar: the level of immunity might drop due to declining cell numbers or lowered gene activity per cell. Molecular pathways from differentially expressed and methylated genes indicated that the MAPK signaling pathway, Jak/STAT signaling pathway, Hippo signaling pathway, mTOR signaling pathway, TGF-beta signaling pathway, ubiquitin mediated proteolysis, and spliceosome are critical to respond to viral infections. Both MAPK and Jak/STAT signaling pathway are immune pathways with antiviral immune response in honey bees and dipterans (Dostert et al., 2005; Lemaitre and Hoffmann, 2007; Cirimotich et al., 2010; Chen et al., 2014; Galbraith et al., 2015; Brutscher et al., 2015; Grozinger and Flenniken, 2019). The Hippo signaling pathway is highly conserved from insects to mammals, which regulates cell death and differentiation (Munoz-Wolf and Lavelle, 2017). Previous study also showed differentially expressed genes regulated by IAPV in honey bee brood are involved in the mTOR, TGF-beta pathways, and ubiquitin mediated proteolysis (Chen et al., 2014).
In addition, our data showed that IAPV infection can induce Defensin-1, a key antimicrobial peptide responding to viral infections (Kuster et al., 2014; Brutscher et al., 2015). P450 genes are known as responding to oxidative stress in Drosophila during larval development (Li et al., 2008). In honey bees, P450s are involved in xenobiotic detoxification (Johnson et al., 2009; Berenbaum and Johnson, 2015). From a wider perspective, Chikungunya virus infection specifically activates the MAPK signaling pathways in humans (Varghese et al., 2016) and is a stress-responsive part of the intestinal innate immunity of C. elegans (Sakaguchi et al., 2004). Recent report showed that MAPK may play a role in regulating certain P450 genes (Yang et al., 2020). On the other hand, IAPV infection presumably causes major deregulation of the cellular homeostasis through the cooption of the cellular machinery to replicate itself (Boncristiani et al., 2013). Thus, deregulation of ribosome biosynthesis (Figure 3) and major energetic pathways is not surprising. Interestingly, transcriptomic analyses of Colony Collapse Disorder, which had been associated with IAPV (Cox-Foster et al., 2007), have shown similar immune responses (Johnson et al., 2009; Aufauvre et al., 2014). The methylomic profile of pupae 48 h after IAPV infection indicated genes involved in anti-parasitoid immune response (Howell et al., 2012; Haddad et al., 2016) to be affected. One of these genes that overlaps with a previous genomic study of honey bee immune genes (Evans et al., 2006) is Peroxin 23, a protein involved in peroxisomal protein import and associated with autophagosome formation in Drosophila muscles and central nervous system (Hazelett et al., 2012; Zirin et al., 2015). Compared to a previous report of the 5 mC methylomic profiles of honey bee adults infected with IAPV (Galbraith et al., 2015), our analysis revealed only one overlapping gene (GB51998), which is related to ATP binding, circadian regulation of gene expression systems (Claridge-Chang et al., 2001), and muscle morphogenesis and function in Drosophila (Schnorrer et al., 2010).
The list of identified DMRs linked to genes associated with ATP binding, phagosome, fatty acid degradation, RNA transport, and RNA degradation. Lipid metabolism, signaling, and biosynthesis are related to virus-host interactions (Chukkapalli et al., 2012). Fatty acid degradation and metabolism also responded to cold stress in other insects and might indicate increased energetic demands of stressed organisms (Vermeulen et al., 2013). Alternatively, IAPV may remodel the host cells by hijacking the lipid signaling pathways and synthesis process for its own replication, similar to virus manipulation of host RNA replication and transport mechanisms (Nagy and Pogany, 2012; Boncristiani et al., 2013).
Our study only represents a first step in the understanding of the intricate interplay of viruses and their honey bee host’s physiology. Future research needs to characterize the temporal transcriptome trends in more detail and tissue-specificity. Pupae are particularly relevant but also challenging because the ongoing metamorphosis entails drastic hormonal and presumably transcriptional changes even in the absence of disease. The discovered functional pathways need to be functionally investigated, particularly the putative 5 mC methylation control of immune genes.
Materials and Methods
Bee Samples and Virus Preparation
All honey bee samples were acquired from unselected experimental hives in the research apiary of the University of North Carolina Greensboro. A previously prepared and characterized IAPV solution in PBS was used for inoculations (Boncristiani et al., 2013). White-eyed pupae were either injected with PBS solution as control treatment or with 104 genome copies of IAPV in 1.0 μl of the viral suspension per pupa. Pupae were maintained on folder filter papers in a sterile lab incubator at 32°C and 60% R.H. After 5, 20, and 48 h of injections, each individual pupal sample was flash-frozen in liquid nitrogen and then stored in a −80°C freezer. Figure 1 illustrates the experimental design.
RNA-Seq Library Preparation and Sequencing
Treatment control (PBS-injection) and IAPV-infected pupae were compared across three time points (5, 20, and 48 h). Three pupae were collected for each treatment and each time point for a total of 18 samples. Dual extraction of nucleic acid (RNA and DNA) from each pupa was carried out using the MasterPure dual extraction kit (Epicenter, MC85200). Briefly, whole pupae were individually homogenized and lysed first by using a micropestle. The lysed cells were mixed with extraction buffer and centrifuged to remove proteins and undesired debris of the cell. Subsequent RNA and DNA fractions were treated with DNase and RNase accordingly. RNA-seq library preparation and sequencing were performed by the Genomic Sciences Laboratory of the North Carolina State University. Total RNA from each pupa was used to generate tagged Illumina libraries, using the NEBNext® RNA Library Prep Kit (New England Biolab). All RNA-seq libraries were sequenced in two flow cells on the Illumina Next-Seq 500 (paired-end 150 bp length reads).
BS-Seq Library Preparation and Sequencing
Bisulfite (BS) conversion of the genomic DNA from the above-mentioned dual extraction of each sample was carried out by using the EZ DNA Methylation-Lightning Kit (Zymo Research, D5030, Irvine, CA, United States). BS-converted DNA (100 ng) of each sample was used for preparing the BS-seq library via the TruSeq DNA Methylation Kit (Illumina, EGMK91324, San Diego, CA, United States). The quality of the libraries was checked using Qubit assays (Q32850, Life Technologies) and with the 2100 Bioanalyzer (Agilent Technologies, Inc.). The pooled 18 libraries were run on two replicate lanes of the Illumina NextSeq 500 (150 bp paired-end reads) by the Genomic Sciences Laboratory at NCSU.
Bioinformatic Analyses
Overall quality control analysis of all resulting data was carried out by using FastQC.1 Raw data were trimmed by Trimmomatic (java -jar PE -phred33 ILLUMINACLIP:TruSeq3-PE.fa:2:30:10 MINLEN:36). TopHat2 v.2.0.12 (Kim et al., 2013) was used to align trimmed RNA-seq reads to the Apis mellifera 4.5 reference genome. General alignment parameters were chosen to allow three mismatches per segment, 12 mismatches per read, and gaps of up to 3 bp (tophat –read-mismatches 12 –segment-mismatches 3 –read-gap-length 3 –read-edit-dist 15 –b2-sensitive) to minimize the possibility that small sequence differences of our samples with the reference genome would bias expression estimates. These settings resulted in the successful alignment of 35% of raw reads (117–143 million per sample). Reconstructed transcripts were aligned with Cufflinks (v2.2.1) to the Apis mellifera OGS 3.2 allowing gaps of up to 1 bp (cufflinks –overlap-radius 1 –library-type fr-firststrand). To annotate promoters and transcription starts, we employed the “cuffcompare” command using OGS3.2 gff file (cuffcompare -s -CG -r).
Cuffdiff2 (v.2.1.1) (Kim et al., 2013) was used to test for differential expression between IAPV and control groups (n = 3 pupae treated as biological replicates at each of the 3 different time points) with the multiple mapping correction and a false discovery rate threshold set at 0.05. All three IAPV groups (IAPV_5h, IAPV_20h, and IAPV_48h) were compared to their corresponding sham-control groups (PBS_5h, PBS_20h, PBS_48h) to identify differentially expressed genes (DEGs). The data were explored and visualized with CummeRbundv.2.0.02 and custom R scripts (Rsoftwarev.2.15.0).3 Differentially expressed genes with similar expression temporal dynamics were analyzed by cufflinks in R. Total of output of gene numbers is set to be 10 (for example, consider gene GB49890 (cyp6as5), we can find nine other genes in the database with similar expression patterns, then plot the expression patterns in Supplementary Figure 7).
Whole genome BS-Seq analysis was carried out by trimming the sequences using trim_galore (version 0.4.1)4 (trim_galore./file1_R1_001.fastq.gz./file1_R2_001.fastq.gz -q 20 –paired –phred33 -o). The Bismark tool (Krueger and Andrews, 2011) (bismark –bowtie2 –path_to_bowtie -p 8 path_to_genome_files/–1/file1 –2/file2) was employed for whole genome alignment. Brief procedures included Genome Conversion, Genome Alignment, Deduplication, and Methylation calls. Differentially methylated regions and genes (DMRs) were produced by methylKit in R (version 1.3.8) with percent methylation difference larger than 10% and q < 0.01 for the difference (Akalin et al., 2012).
The reference genome and official gene set were the same as described before. The sequencing raw data are published at the NCBI database (Sequence Read Archive (SRA) submission: SUB3404557, BioProject: PRJNA429508). All codes for the bioinformatic analyses are online.5
To determine overlap between DEG lists, either up- or down-regulated genes were compared between different time points. This directional overlap analysis avoids artifacts that can confound undirected DEG comparisons (Lawhorn et al., 2018). The analysis was compared focusing on DEGs with a more than 8-fold difference, and with DMRs (either hyper- or hypo-methylated). All overlap analyses were performed with hypergeometric tests to calculate the p-value for overlap based on the cumulative distribution function (CDF) of the hypergeometric distribution as published previously (Li-Byarlay et al., 2013). GO Mann-Whitney U tests were carried out on the gene expression data using codes published in Github.6 These analyses search for patterns of up- or down-regulation in genes associated with particular GO-terms based on their signed, uncorrected p-values regardless of significance thresholds (Wright et al., 2015).
For differentially transcript usage or spliced genes, transcript-level counts were quantified using Salmon1 v1.2.1 (Patro et al., 2017) against the A. mellifera HAv3.1 annotation. Viral RNAs were included in the transcriptome used for alignment but were omitted from isoform analysis. Salmon results were imported directly into the IsoformSwitchAnalyzeR R package (Vitting-Seerup and Sandelin, 2017; Vitting-Seerup and Sandelin, 2019), which normalizes transcript counts based on abundances and uses the TMM method to adjust effective library size. The version of IsoformSwitchAnalyzeR used was 1.11.3, obtained from GitHub commit 5a7ab4a due to a bug in version 1.11.2 on Bioconductor. Although CDS were annotated in the GFF file for A. mellifera, these caused problems in IsoformSwitchAnalyzeR due to inclusion of stop codons, as well as some transcripts having no annotated CDS or CDS that were obviously incorrect. Therefore, the analyzeORF function was used to predict open reading frames de novo from the transcript sequences, which in the vast majority of cases resulted in agreement with the published annotation. Nucleotide and amino acid sequences for all transcripts for significant genes were then exported for analysis with external tools: CPAT9 was used to determine whether a transcript was likely to be coding (Wang et al., 2013). It was run from the webserver using the Drosophila (dm3, BDGP release 5) assembly. A coding probability of 0.7 was used as the cutoff for classifying a transcript as coding or non-coding. Pfam10 (Punta et al., 2012) version 32.0 was used to predict protein domains, using pfam_scan v1.6 run on the Biocluster.
Statistical Analysis of Differential Transcript Usage
A design matrix was constructed in which the condition was the combination of treatment and time point (six conditions total), and DWV infection (determined by alignment of reads to the DWV mRNA) was included as a covariate. Sample degradation was excluded as a covariate as it introduced singularity into the model at 48 h. The contrasts tested were IAPV_5h – PBS_5h, IAPV_20h – PBS_20h, and IAPV_48h – PBS_48h. The preFilter function in IsoformSwitchAnalyzeR was used with default settings to filter transcripts and genes. Genes were removed if they only had one isoform or if they did not have at least 1 RPKM in at least one condition. Transcripts were removed if they had no expression in any condition. After filtering, 15,808 isoforms belonging to 4,667 genes remained.
Differential transcript usage (DTU) was assessed using DEXSeq from within IsoformSwitchAnalyzeR (Anders et al., 2012; Ritchie et al., 2015; Vitting-Seerup and Sandelin, 2019). Genes were only retained for further analysis if they had an FDR < 0.05 for DTU, and if isoform usage (proportion of counts from a gene belonging to a given isoform) changed by at least 0.1 for at least two isoforms in opposite directions (i.e., the isoforms “switched”).
Enrichment of Overlapping Genes Among Different Studies
To test whether our DEGs and DMRs had significant overlap with known immune genes, these lists were compared to published immune genes (Evans et al., 2006) using hypergeometric tests. Additionally, a directional overlap analysis was performed on our DEGs (separated by up- or down-regulation) with a previous RNA-seq study of IAPV infection in adult honey bee workers (Galbraith et al., 2015).
To identify potential motifs that are targeted for epigenetic modification after IAPV infection, differentially methylated regions (DMR) were analyzed post 5, 20, and 48 h IAPV infection generating 6-mer sequence logos with the online tool weblogo.7
Data Availability Statement
The sequencing raw data are published at the NCBI database (Sequence Read Archive (SRA) submission: SUB3404557, BioProject: PRJNA429508).
Author Contributions
HL-B, MS, DT, and OR conceptualized the scientific idea and aims. HB performed the viral infection experiments. HL-B prepared the samples and performed sequencing experiments and bioinformatics analyses. GH provided assistance on the computational techniques on High Performance Cluster. JH and LC provided additional analysis of GO and splicing. HL-B wrote the manuscript with all co-authors input. All authors contributed to the article and approved the submitted version.
Funding
The National Research Council Postdoctoral Fellowship, USDA NIFA Evans Allen fund (NI191445XXXXG002), NSF HBCU-UP grant (1900793), and Illumina Go Mini Challenge Grant supported HL-B. Major research funding was from the U.S. Army Research Laboratory grants #W911NF04D0003 and #W911NF1520045 to OR, DT, and MS.
Conflict of Interest
The authors declare that the research was conducted in the absence of any commercial or financial relationships that could be construed as a potential conflict of interest.
Acknowledgments
We thank Karl M. Glastad (University of Pennsylvania), Marce Lorenzen (NCSU), Andrew Petersen (NCSU), and HPCBio at University of Illinois on bioinformatics consulting, Karmi Oxman and Susan Balfe for the graphic designs, and Conrad Zagory, Jr. at CSU for critical reviews on the English writing.
Supplementary Material
The Supplementary Material for this article can be found online at: https://www.frontiersin.org/articles/10.3389/fgene.2020.566320/full#supplementary-material
Footnotes
- ^ http://www.bioinformatics.babraham.ac.uk/projects/fastqc/
- ^ http://compbio.mit.edu/cummeRbund/
- ^ http://www.r-project.org/
- ^ http://www.bioinformatics.babraham.ac.uk/projects/trim_galore/
- ^ https://www.researchgate.net/publication/343237015_RNAseq_and_BSseq_codes_for_public
- ^ https://github.com/z0on/GO_MWU
- ^ https://weblogo.berkeley.edu
References
Akalin, A., Kormaksson, M., Li, S., Garrett-Bakelman, F. E., Figueroa, M. E., Melnick, A., et al. (2012). methylKit: a comprehensive R package for the analysis of genome-wide DNA methylation profiles. Genome Biol. 13:R87.
Allis, C. D., and Jenuwein, T. (2016). The molecular hallmarks of epigenetic control. Nat. Rev. Genet. 17, 487–500. doi: 10.1038/nrg.2016.59
Anders, S., Reyes, A., and Huber, W. (2012). Detecting differential usage of exons from RNA-seq data. Genome Res. 22, 2008–2017. doi: 10.1101/gr.133744.111
Arvey, A., Tempera, I., Tsai, K., Chen, H.-S., Tikhmyanova, N., Klichinsky, M., et al. (2012). An atlas of the epstein-barr virus transcriptome and epigenome reveals host-virus regulatory interactions. Cell Host Microbe 12, 233–245. doi: 10.1016/j.chom.2012.06.008
Aufauvre, J., Misme-Aucouturier, B., Viguès, B., Texier, C., Delbac, F., and Blot, N. (2014). Transcriptome analyses of the honeybee response to Nosema ceranae and insecticides. PLoS One 9:e91686. doi: 10.1371/journal.pone.0091686
Bailey, L., Gibbs, A., and Woods, R. (1963). Two viruses from adult honey bees (Apis mellifera Linnaeus). Virology 21, 390–395. doi: 10.1016/0042-6822(63)90200-9
Berenbaum, M. R., and Johnson, R. M. (2015). Xenobiotic detoxification pathways in honey bees. Curr. Opin. Insect Sci. 10, 51–58. doi: 10.1016/j.cois.2015.03.005
Bewick, A. J., Vogel, K. J., Moore, A. J., and Schmitz, R. J. (2017). Evolution of DNA methylation across insects. Mol. Biol. Evol. 34, 654–665.
Boncristiani, H. F., Evans, J. D., Chen, Y., Pettis, J., Murphy, C., Lopez, D. L., et al. (2013). In-vitro infection of pupae with Israeli Acute Paralysis Virus suggests variation for susceptibility and disturbance of transcriptional homeostasis in honey bees (Apis mellifera). PLoS One 8:e73429. doi: 10.1371/journal.pone.0073429
Bonning, B. C., and Miller, W. A. (2010). Dicistroviruses. Annu. Rev. Entomol. 55, 129–150. doi: 10.1146/annurev-ento-112408-085457
Brutscher, L. M., Daughenbaugh, K. F., and Flenniken, M. L. (2015). Antiviral defense mechanisms in honey bees. Curr. Opin. Insect Sci. 10, 71–82. doi: 10.1016/j.cois.2015.04.016
Brutscher, L. M., Daughenbaugh, K. F., and Flenniken, M. L. (2017). Virus and dsRNA-triggered transcriptional responses reveal key components of honey bee antiviral defense. Sci. Rep. 7:6448.
Burggren, W. W. (2017). Epigenetics in insects: mechanisms, phenotypes and ecological and evolutionary implications. Adv. Insect Physiol. 53, 1–30. doi: 10.1016/bs.aiip.2017.04.001
Calderone, N. W. (2012). Insect pollinated crops, insect pollinators and US agriculture: trend analysis of aggregate data for the period 1992–2009. PLoS One 7:e37235. doi: 10.1371/journal.pone.0037235
Chan, C. Y., Low, J. Z. H., Gan, E. S., Ong, E. Z., Zhang, S. L.-X., Tan, H. C., et al. (2019). Antibody-dependent dengue virus entry modulates cell intrinsic responses for enhanced infection. mSphere 4:e00528-19.
Chen, Y. P., Pettis, J. S., Corona, M., Chen, W. P., Li, C. J., Spivak, M., et al. (2014). Israeli acute paralysis virus: epidemiology, pathogenesis and implications for honey bee health. PLoS Pathog. 10:e1004261. doi: 10.1371/journal.ppat.1004261
Chen, Y. P., and Siede, R. (2007). “Honey bee viruses,” in Advances in Virus Research, Vol. 70, eds K. Maramorosch, A. J. Shatkin, and F. A. Murphy (Salt Lake City, UT: Academic Press), 33–80.
Chukkapalli, V., Heaton, N. S., and Randall, G. (2012). Lipids at the interface of virus–host interactions. Curr. Opin. Microbiol. 15, 512–518. doi: 10.1016/j.mib.2012.05.013
Cirimotich, C. M., Dong, Y., Garver, L. S., Sim, S., and Dimopoulos, G. (2010). Mosquito immune defenses against Plasmodium infection. Dev. Comp. Immunol. 34, 387–395. doi: 10.1016/j.dci.2009.12.005
Claridge-Chang, A., Wijnen, H., Naef, F., Boothroyd, C., Rajewsky, N., and Young, M. W. (2001). Circadian regulation of gene expression systems in the Drosophila head. Neuron 32, 657–671. doi: 10.1016/s0896-6273(01)00515-3
Cornman, R. S., Boncristiani, H., Dainat, B., Chen, Y., Weaver, D., and Evans, J. D. (2013). Population-genomic variation within RNA viruses of the Western honey bee, Apis mellifera, inferred from deep sequencing. BMC genomics. 14:154. doi: 10.1186/1471-2164-14-154
Cornman, R. S., Tarpy, D. R., Chen, Y., Jeffreys, L., Lopez, D., Pettis, J. S., et al. (2012). Pathogen webs in collapsing honey bee colonies. PLoS One 7:e43562. doi: 10.1371/journal.pone.0043562
Cox-Foster, D. L., Conlan, S., Holmes, E. C., Palacios, G., Evans, J. D., Moran, N. A., et al. (2007). A metagenomic survey of microbes in honey bee colony collapse disorder. Science 318, 283–287. doi: 10.1126/science.1146498
Dantas Machado, A. C., Zhou, T., Rao, S., Goel, P., Rastogi, C., Lazarovici, A., et al. (2015). Evolving insights on how cytosine methylation affects protein–DNA binding. Brief. Funct. Genomics 14, 61–73. doi: 10.1093/bfgp/elu040
De Maio, F. A., Risso, G., Iglesias, N. G., Shah, P., Pozzi, B., Gebhard, L. G., et al. (2016). The dengue virus NS5 protein intrudes in the cellular spliceosome and modulates splicing. PLoS Pathog. 12:e1005841. doi: 10.1371/journal.ppat.1005841
De Monerri, N. C. S., and Kim, K. (2014). Pathogens hijack the epigenome: a new twist on host-pathogen interactions. Am. J. Pathol. 184, 897–911.
Di Prisco, G., Pennacchio, F., Caprio, E., Boncristiani, H. F. Jr., Evans, J. D., and Chen, Y. (2011). Varroa destructor is an effective vector of Israeli acute paralysis virus in the honeybee, Apis mellifera. J. Gen. Virol. 92, 151–155. doi: 10.1099/vir.0.023853-0
Dostert, C., Jouanguy, E., Irving, P., Troxler, L., Galiana-Arnoux, D., Hetru, C., et al. (2005). The Jak-STAT signaling pathway is required but not sufficient for the antiviral response of drosophila. Nat. Immunol. 6, 946–953. doi: 10.1038/ni1237
Dubois, J., Terrier, O., and Rosa-Calatrava, M. (2014). Influenza viruses and mRNA splicing: doing more with less. mBio 5:e00070-14.
Edwards, T. M., and Myers, J. P. (2007). Environmental exposures and gene regulation in disease etiology. Environ. Health Perspect. 115, 1264–1270. doi: 10.1289/ehp.9951
Evans, J. D., Aronstein, K., Chen, Y. P., Hetru, C., Imler, J. L., Jiang, H., et al. (2006). Immune pathways and defence mechanisms in honey bees Apis mellifera. Insect Mol. Biol. 15, 645–656. doi: 10.1111/j.1365-2583.2006.00682.x
Fang, J., Hao, Q., Liu, L., Li, Y., Wu, J., Huo, X., et al. (2012). Epigenetic changes mediated by microRNA miR29 activate cyclooxygenase 2 and lambda-1 interferon production during viral infection. J. Virol. 86, 1010–1020. doi: 10.1128/jvi.06169-11
Galbraith, D. A., Yang, X., Niño, E. L., Yi, S., Grozinger, C., and Schneider, D. S. (2015). Parallel epigenomic and transcriptomic responses to viral infection in honey bees (Apis mellifera). PLoS Pathog. 11:e1004713. doi: 10.1371/journal.ppat.1004713
Gardy, J. L., and Loman, N. J. (2018). APPLICATIONS OF NEXT-GENERATION SEQUENCING Towards a genomics-informed, real-time, global pathogen surveillance system. Nat. Rev. Genet. 19, 9–20. doi: 10.1038/nrg.2017.88
Gisder, S., and Genersch, E. (2017). Viruses of commercialized insect pollinators. J. Invertebr. Pathol. 147, 51–59. doi: 10.1016/j.jip.2016.07.010
Glastad, K. M., Gokhale, K., Liebig, J., and Goodisman, M. A. (2016). The caste-and sex-specific DNA methylome of the termite Zootermopsis nevadensis. Sci. Rep. 6:37110.
Glastad, K. M., Goodisman, M. A., Yi, S. V., and Hunt, B. G. (2015). Effects of DNA methylation and chromatin state on rates of molecular evolution in insects. G3 6, 357–363. doi: 10.1534/g3.115.023499
Glastad, K. M., Hunt, B. G., and Goodisman, M. A. (2019). Epigenetics in insects: genome regulation and the generation of phenotypic diversity. Annu. Rev. Entomol. 64, 185–203. doi: 10.1146/annurev-ento-011118-111914
Glastad, K. M., Hunt, B. G., and Goodisman, M. A. D. (2014). Evolutionary insights into DNA methylation in insects. Curr. Opin. Insect Sci. 1, 25–30. doi: 10.1016/j.cois.2014.04.001
Goulson, D., Nicholls, E., Botias, C., and Rotheray, E. L. (2015). Bee declines driven by combined stress from parasites, pesticides, and lack of flowers. Science 347:1255957. doi: 10.1126/science.1255957
Grozinger, C. M., and Flenniken, M. L. (2019). Bee viruses: ecology, pathogenicity, and impacts. Annu. Rev. Entomol. 64, 205–226. doi: 10.1146/annurev-ento-011118-111942
Haddad, N., Mahmud Batainh, A., Suleiman Migdadi, O., Saini, D., Krishnamurthy, V., Parameswaran, S., et al. (2016). Next generation sequencing of Apis mellifera syriaca identifies genes for Varroa resistance and beneficial bee keeping traits. Insect Sci. 23, 579–590. doi: 10.1111/1744-7917.12205
Hazelett, D. J., Chang, J. C., Lakeland, D. L., and Morton, D. B. (2012). Comparison of parallel high-throughput RNA sequencing between knockout of TDP-43 and its overexpression reveals primarily nonreciprocal and nonoverlapping gene expression changes in the central nervous system of Drosophila. G3 2, 789–802. doi: 10.1534/g3.112.002998
Herb, B. R., Wolschin, F., Hansen, K. D., Aryee, M. J., Langmead, B., Irizarry, R., et al. (2012). Reversible switching between epigenetic states in honeybee behavioral subcastes. Nat. Neurosci. 15, 1371–1373. doi: 10.1038/nn.3218
Howell, L., Sampson, C. J., Xavier, M. J., Bolukbasi, E., Heck, M. M., and Williams, M. J. (2012). A directed miniscreen for genes involved in the Drosophila anti-parasitoid immune response. Immunogenetics 64, 155–161. doi: 10.1007/s00251-011-0571-3
Huang, H., Wu, P., Zhang, S., Shang, Q., Yin, H., Hou, Q., et al. (2019). DNA methylomes and transcriptomes analysis reveal implication of host DNA methylation machinery in BmNPV proliferation in Bombyx mori. BMC genomics. 20:736. doi: 10.1186/s12864-019-6146-7
Hunt, B. G., Brisson, J. A., Yi, S. V., and Goodisman, M. A. D. (2010). Functional conservation of DNA methylation in the pea aphid and the honeybee. Genome Biol. Evol. 2, 719–728. doi: 10.1093/gbe/evq057
Hunt, B. G., Glastad, K. M., Yi, S. V., and Goodisman, M. A. D. (2013). The function of intragenic DNA methylation: insights from insect epigenomes. Integr. Comp. Biol. 53, 319–328. doi: 10.1093/icb/ict003
Johnson, R. M., Evans, J. D., Robinson, G. E., and Berenbaum, M. R. (2009). Changes in transcript abundance relating to colony collapse disorder in honey bees (Apis mellifera). Proc. Natl. Acad. Sci. U. S. A. 106, 14790–14795. doi: 10.1073/pnas.0906970106
Kim, D., Pertea, G., Trapnell, C., Pimentel, H., Kelley, R., and Salzberg, S. L. (2013). TopHat2: accurate alignment of transcriptomes in the presence of insertions, deletions and gene fusions. Genome Biol. 14:R36.
Krueger, F., and Andrews, S. R. (2011). Bismark: a flexible aligner and methylation caller for Bisulfite-Seq applications. Bioinformatics 27, 1571–1572. doi: 10.1093/bioinformatics/btr167
Kucharski, R., Maleszka, J., Foret, S., and Maleszka, R. (2008). Nutritional control of reproductive status in honeybees via DNA methylation. Science 319, 1827–1830. doi: 10.1126/science.1153069
Kulhanek, K., Steinhauer, N., Rennich, K., Caron, D. M., Sagili, R. R., Pettis, J. S., et al. (2017). A national survey of managed honey bee 2015–2016 annual colony losses in the USA. J. Apic. Res. 56, 328–340. doi: 10.1080/00218839.2017.1344496
Kusano, M., Toyota, M., Suzuki, H., Akino, K., Aoki, F., Fujita, M., et al. (2006). Genetic, epigenetic, and clinicopathologic features of gastric carcinomas with the CpG island methylator phenotype and an association with Epstein–Barr virus. Cancer 106, 1467–1479. doi: 10.1002/cncr.21789
Kuss-Duerkop, S. K., Westrich, J. A., and Pyeon, D. (2018). DNA tumor virus regulation of host DNA methylation and its implications for immune evasion and oncogenesis. Viruses 10:82. doi: 10.3390/v10020082
Kuster, R. D., Boncristiani, H. F., and Rueppell, O. (2014). Immunogene and viral transcript dynamics during parasitic Varroa destructor mite infection of developing honey bee (Apis mellifera) pupae. J. Exp. Biol. 217, 1710–1718. doi: 10.1242/jeb.097766
Lawhorn, C. M., Schomaker, R., Rowell, J. T., and Rueppell, O. (2018). Simple comparative analyses of differentially expressed gene lists may overestimate gene overlap. J. Comput. Biol. 25, 606–612. doi: 10.1089/cmb.2017.0262
Lemaitre, B., and Hoffmann, J. (2007). The host defense of Drosophila melanogaster. Annu. Rev. Immunol. 25, 697–743.
Li, H. M., Buczkowski, G., Mittapalli, O., Xie, J., Wu, J., Westerman, R., et al. (2008). Transcriptomic profiles of Drosophila melanogaster third instar larval midgut and responses to oxidative stress. Insect Mol. Biol. 17, 325–339. doi: 10.1111/j.1365-2583.2008.00808.x
Li-Byarlay, H. (2016). The function of DNA methylation marks in social insects. Front. Ecol. Evol. 4:57. doi: 10.3389/fevo.2016.00057
Li-Byarlay, H., Li, Y., Stroud, H., Feng, S., Newman, T. C., Hou, K. K., et al. (2013). RNA interference knockdown of DNA methyl-transferase 3 affects gene alternative splicing in the honey bee. Proc. Natl. Acad. Sci. U.S.A. 110, 12750–12755. doi: 10.1073/pnas.1310735110
Lyko, F., Foret, S., Kucharski, R., Wolf, S., Falckenhayn, C., and Maleszka, R. (2010). The honey bee epigenomes: differential methylation of brain DNA in queens and workers. PLoS Biol. 8:e1000506. doi: 10.1371/journal.pbio.1000506
Maori, E., Lavi, S., Mozes-Koch, R., Gantman, Y., Peretz, Y., Edelbaum, O., et al. (2007). Isolation and characterization of Israeli acute paralysis virus, a dicistrovirus affecting honeybees in Israel: evidence for diversity due to intra- and inter-species recombination. J. Gen. Virol. 88(Pt 12), 3428–3438. doi: 10.1099/vir.0.83284-0
Maori, E., Paldi, N., Shafir, S., Kalev, H., Tsur, E., Glick, E., et al. (2009). IAPV, a bee-affecting virus associated with Colony Collapse Disorder can be silenced by dsRNA ingestion. Insect Mol. Biol. 18, 55–60. doi: 10.1111/j.1365-2583.2009.00847.x
Marina, R. J., Sturgill, D., Bailly, M. A., Thenoz, M., Varma, G., Prigge, M. F., et al. (2016). TET-catalyzed oxidation of intragenic 5-methylcytosine regulates CTCF-dependent alternative splicing. EMBO J. 35, 335–355. doi: 10.15252/embj.201593235
Matilainen, O., Quirós, P. M., and Auwerx, J. (2017). Mitochondria and epigenetics–crosstalk in homeostasis and stress. Trends Cell Biol. 27, 453–463. doi: 10.1016/j.tcb.2017.02.004
McMenamin, A. J., and Genersch, E. (2015). Honey bee colony losses and associated viruses. Curr. Opin. Insect Sci. 8, 121–129. doi: 10.1016/j.cois.2015.01.015
Merkling, S. H., Bronkhorst, A. W., Kramer, J. M., Overheul, G. J., Schenck, A., and Van Rij, R. P. (2015). The epigenetic regulator G9a mediates tolerance to RNA virus infection in Drosophila. PLoS Pathog. 11:e1004692. doi: 10.1371/journal.ppat.1004692
Mukherjee, K., Twyman, R. M., and Vilcinskas, A. (2015). Insects as models to study the epigenetic basis of disease. Prog. Biophys. Mol. Biol. 118, 69–78. doi: 10.1016/j.pbiomolbio.2015.02.009
Munoz-Wolf, N., and Lavelle, E. C. (2017). Hippo interferes with antiviral defences. Nat. Cell Biol. 19, 267–269. doi: 10.1038/ncb3502
Nagy, P. D., and Pogany, J. (2012). The dependence of viral RNA replication on co-opted host factors. Nat. Rev. Microbiol. 10, 137–149. doi: 10.1038/nrmicro2692
Opachaloemphan, C., Yan, H., Leibholz, A., Desplan, C., and Reinberg, D. (2018). Recent advances in behavioral (epi) genetics in eusocial insects. Annu. Rev. Genet. 52, 489–510. doi: 10.1146/annurev-genet-120116-024456
Paschos, K., and Allday, M. J. (2010). Epigenetic reprogramming of host genes in viral and microbial pathogenesis. Trends Microbiol. 18, 439–447. doi: 10.1016/j.tim.2010.07.003
Patro, R., Duggal, G., Love, M. I., Irizarry, R. A., and Kingsford, C. (2017). Salmon provides fast and bias-aware quantification of transcript expression. Nat. Methods 14, 417–419. doi: 10.1038/nmeth.4197
Pavet, V., Quintero, C., Cecchini, N. M., Rosa, A. L., and Alvarez, M. E. (2006). Arabidopsis displays centromeric DNA hypomethylation and cytological alterations of heterochromatin upon attack by Pseudomonas syringae. Mol. Plant Microbe Interact. 19, 577–587. doi: 10.1094/mpmi-19-0577
Punta, M., Coggill, P. C., Eberhardt, R. Y., Mistry, J., Tate, J., Boursnell, C., et al. (2012). The Pfam protein families database. Nucleic Acids Res. 40, D290–D301.
Ritchie, M. E., Phipson, B., Wu, D., Hu, Y., Law, C. W., Shi, W., et al. (2015). limma powers differential expression analyses for RNA-sequencing and microarray studies. Nucleic Acids Res. 43:e47. doi: 10.1093/nar/gkv007
Runckel, C., Flenniken, M. L., Engel, J. C., Ruby, J. G., Ganem, D., Andino, R., et al. (2011). Temporal analysis of the honey bee microbiome reveals four novel viruses and seasonal prevalence of known viruses, Nosema, and Crithidia. PLoS One 6:e20656. doi: 10.1371/journal.pone.0020656
Sakaguchi, A., Matsumoto, K., and Hisamoto, N. (2004). Roles of MAP kinase cascades in Caenorhabditis elegans. J. Biochem. 136, 7–11. doi: 10.1093/jb/mvh097
Saripalli, G., Sharma, C., Gautam, T., Singh, K., Jain, N., Prasad, P., et al. (2020). Complex relationship between DNA methylation and gene expression due to Lr28 in wheat-leaf rust pathosystem. Mol. Biol. Rep. 47, 1339–1360. doi: 10.1007/s11033-019-05236-1
Schnorrer, F., Schonbauer, C., Langer, C. C. H., Dietzl, G., Novatchkova, M., Schernhuber, K., et al. (2010). Systematic genetic analysis of muscle morphogenesis and function in Drosophila. Nature 464, 287–291. doi: 10.1038/nature08799
Shukla, S., Kavak, E., Gregory, M., Imashimizu, M., Shutinoski, B., Kashlev, M., et al. (2011). CTCF-promoted RNA polymerase II pausing links DNA methylation to splicing. Nature 479, 74–79. doi: 10.1038/nature10442
Tanca, A., Deligios, M., Addis, M. F., and Uzzau, S. (2013). High throughput genomic and proteomic technologies in the fight against infectious diseases. J. Infect. Dev. Ctries. 7, 182–190. doi: 10.3855/jidc.3027
Tantillo, G., Bottaro, M., Di Pinto, A., Martella, V., Di Pinto, P., and Terio, V. (2015). Virus infections of honeybees Apis mellifera. Ital. J. Food Saf. 4, 157–168.
Tian, F., Zhan, F., VanderKraats, N. D., Hiken, J. F., Edwards, J. R., Zhang, H., et al. (2013). DNMT gene expression and methylome in Marek’s disease resistant and susceptible chickens prior to and following infection by MDV. Epigenetics 8, 431–444. doi: 10.4161/epi.24361
Toyota, M., Suzuki, H., Yamashita, T., Hirata, K., Imai, K., Tokino, T., et al. (2009). Cancer epigenomics: implications of DNA methylation in personalized cancer therapy. Cancer Sci. 100, 787–791. doi: 10.1111/j.1349-7006.2009.01095.x
Toyota, M., and Yamamoto, E. (2011). DNA methylation changes in cancer. Prog. Mol. Biol. Transl. Sci. 101, 447–457.
Turner, M., and Diaz-Munoz, M. D. (2018). RNA-binding proteins control gene expression and cell fate in the immune system. Nat. Immunol. 19, 120–129. doi: 10.1038/s41590-017-0028-4
Varghese, F. S., Thaa, B., Amrun, S. N., Simarmata, D., Rausalu, K., Nyman, T. A., et al. (2016). The antiviral alkaloid berberine reduces chikungunya virus-induced mitogen-activated protein kinase signaling. J. Virol. 90, 9743–9757. doi: 10.1128/jvi.01382-16
Vermeulen, C. J., Sorensen, P., Gagalova, K. K., and Loeschcke, V. (2013). Transcriptomic analysis of inbreeding depression in cold-sensitive Drosophila melanogaster shows upregulation of the immune response. J. Evol. Biol. 26, 1890–1902. doi: 10.1111/jeb.12183
Vilcinskas, A. (2017). The impact of parasites on host insect epigenetics. Adv. Insect Physiol. 53, 145–165. doi: 10.1016/bs.aiip.2017.05.001
Vitting-Seerup, K., and Sandelin, A. (2017). The landscape of isoform switches in human cancers. Mol. Cancer Res. 15, 1206–1220. doi: 10.1158/1541-7786.mcr-16-0459
Vitting-Seerup, K., and Sandelin, A. (2019). IsoformSwitchAnalyzeR: analysis of changes in genome-wide patterns of alternative splicing and its functional consequences. Bioinformatics 35, 4469–4471. doi: 10.1093/bioinformatics/btz247
Wang, L., Park, H. J., Dasari, S., Wang, S., Kocher, J.-P., and Li, W. (2013). CPAT: coding-potential assessment tool using an alignment-free logistic regression model. Nucleic Acids Res. 41:e74. doi: 10.1093/nar/gkt006
Wang, Y., Jorda, M., Jones, P. L., Maleszka, R., Ling, X., Robertson, H. M., et al. (2006). Functional CpG methylation system in a social insect. Science 314, 645–647. doi: 10.1126/science.1135213
Wang, Y., and Li-Byarlay, H. (2015). Physiological and molecular mechanisms of nutrition in honey bees. Adv. Insect Physiol. 49, 25–58. doi: 10.1016/bs.aiip.2015.06.002
Wilson-Rich, N., Spivak, M., Fefferman, N. H., and Starks, P. T. (2009). Genetic, individual, and group facilitation of disease resistance in insect societies. Annu. Rev. Entomol. 54, 405–423. doi: 10.1146/annurev.ento.53.103106.093301
Wright, R. M., Aglyamova, G. V., Meyer, E., and Matz, M. V. (2015). Gene expression associated with white syndromes in a reef building coral, Acropora hyacinthus. BMC Genomics 16:371. doi: 10.1186/s12864-015-1540-2
Xu, J., Grant, G., Sabin, L. R., Gordesky-Gold, B., Yasunaga, A., Tudor, M., et al. (2012). Transcriptional pausing controls a rapid antiviral innate immune response in Drosophila. Cell Host Microbe 12, 531–543. doi: 10.1016/j.chom.2012.08.011
Yan, H., Simola, D. F., Bonasio, R., Liebig, J., Berger, S. L., and Reinberg, D. (2014). Eusocial insects as emerging models for behavioural epigenetics. Nat. Rev. Genet. 15, 677–688. doi: 10.1038/nrg3787
Yang, X., Deng, S., Wei, X., Yang, J., Zhao, Q., Yin, C., et al. (2020). MAPK-directed activation of the whitefly transcription factor CREB leads to P450-mediated imidacloprid resistance. Proc. Natl. Acad. Sci. U.S.A. 117, 10246–10253. doi: 10.1073/pnas.1913603117
Zemach, A., McDaniel, I. E., Silva, P., and Zilberman, D. (2010). Genome-wide evolutionary analysis of eukaryotic DNA methylation. Science 328, 916–919. doi: 10.1126/science.1186366
Keywords: alternative splicing, transcriptome, DNA methylation, immune genes, pupa, IAPV, gene expression, comparative genomics
Citation: Li-Byarlay H, Boncristiani H, Howell G, Herman J, Clark L, Strand MK, Tarpy D and Rueppell O (2020) Transcriptomic and Epigenomic Dynamics of Honey Bees in Response to Lethal Viral Infection. Front. Genet. 11:566320. doi: 10.3389/fgene.2020.566320
Received: 27 May 2020; Accepted: 17 August 2020;
Published: 24 September 2020.
Edited by:
Wei Guo, Chinese Academy of Sciences (CAS), ChinaReviewed by:
Sufang Zhang, Chinese Academy of Forestry, ChinaRichard Alan Katz, Fox Chase Cancer Center, United States
Copyright © 2020 Li-Byarlay, Boncristiani, Howell, Herman, Clark, Strand, Tarpy and Rueppell. This is an open-access article distributed under the terms of the Creative Commons Attribution License (CC BY). The use, distribution or reproduction in other forums is permitted, provided the original author(s) and the copyright owner(s) are credited and that the original publication in this journal is cited, in accordance with accepted academic practice. No use, distribution or reproduction is permitted which does not comply with these terms.
*Correspondence: Hongmei Li-Byarlay, aGxpLWJ5YXJsYXlAY2VudHJhbHN0YXRlLmVkdQ==
†Present address: Hongmei Li-Byarlay, Agricultural Research and Development Program, Central State University, Wilberforce, OH, United States; Humberto Boncristiani, Department of Entomology, University of Florida, Gainesville, FL, United States; Olav Rueppell, Department of Biological Sciences, University of Alberta, Edmonton, AB, Canada