- Independent Researcher, Guntur, India
Aging is an inevitable biological phenomenon displayed by single cells and organs to entire organismal systems. Aging as a biological process is characterized as a progressive decline in intrinsic biological function. Understanding the causative mechanisms of aging has always captured the imagination of researchers since time immemorial. Although both biological and chronological aging are well defined and studied in terms of genetic, epigenetic, and lifestyle predispositions, the hallmarks of aging in terms of small molecules (i.e., endogenous metabolites to chemical exposures) are limited to obscure. On top of the endogenous metabolites leading to the onset and progression of healthy aging, human beings are constantly exposed to a natural and anthropogenic “chemical” environment round the clock, from conception till death, affecting one’s physiology, health and well-being, and disease predisposition. The research community has started gaining sizeable insights into deciphering the aging factors such as immunosenescence, nutrition, frailty, inflamm-aging, and diseases till date, without much input from their interaction with exogenous chemical exposures. The “exposome” around us, mostly, accelerates the process of aging by affecting the internal biological pathways and signaling mechanisms that result in the deterioration of human health. However, the entirety of exposome on human aging is far from established. This review intends to catalog the known and established associations of the exposome from past studies focusing on aging in humans and other model organisms. Further discussed are the current technologies and informatics tools that enable the study of aging exposotypes, and thus, provide a window of opportunities and challenges to study the “aging exposome” in granular details.
Introduction
The human exposome consists of the entirety of life-course environmental exposures and the lifestyle factors, a variable and dynamic entity which evolves throughout the lifetime of an individual and consists of every exposure to which an individual is subjected, from conception till death (Wild, 2005). Thus, the phenotype (P) is a sum of genotype (G) and its interaction with the environment (E), i.e., P = G + E. In the context of human health and disease the contribution of genotype for complex traits are low and variable, thus providing necessary impetus to research on the role of exposures on human health. These exposures around human civilization are not only natural but also engineered/anthropogenic factors that affect us every moment, either physically (i.e., noise) or chemically (i.e., pollutants). Xenobiotics such as pharmaceuticals and personal care products (PPCPs), pesticides and insecticides, plant-, bacteria- and food- derived compounds, food additives, surfactants, solvents, synthetic and industrial chemicals, and microbial compounds constitute a huge chunk of human chemical exposome (Misra, 2019). In a recent review (Vermeulen et al., 2020), the major categories of “chemical exposome” were identified as diet, drug use, smoking, alcohol use under lifestyle factors. Though most of the chemical exposures are unintentional, intentional exposures can happen too, whether be it for enjoyment (e.g., addictions such as smoking and alcoholism) or as side effects of a therapeutic treatment (e.g., chemotherapy, antibiotics etc.) (Sorrentino et al., 2014), the chemical exposures affect human life incessantly. Further, incidental exposures [e.g., polychlorinated biphenyls (PCBs), lead (Pb), nitrosamines, and pesticides] are also associated with chronic human diseases (Sorrentino et al., 2014). In a recent review, chemical exposures such as those from medication and environmental agents have been shown to be associated with metabolic/lifestyle diseases such as type 2 diabetes mellitus (T2DM) (Misra and Misra, 2020). In this review, the authors summarized that the disease predisposition was associated with exposure (levels) of POPs, phthalates, antibiotics, drugs, air pollution, pesticides, and heavy metals. An accumulating body of knowledge of the redox proteome and the redox interface of the genome and exposome further indicates that aging is a cumulative failure of the adaptive structures supporting genome–exposome interaction (Jones, 2015), given the continual interaction of exposome with the endogenous proteome, epigenome, genome (Go and Jones, 2014), and metabolome of human beings.
Aging in humans is defined as an inevitable progressive decline in physiology that leads to an increased risk of debility, disease, and eventual death. This physiological (and metabolic) decline is identified as the primary risk factor for important human diseases (López-Otín et al., 2013). The primary hallmarks (causes of damage) are identified as an unstable genome, shortening of telomeres, loss of proteostasis, and epigenetic modifications (López-Otín et al., 2013). The authors suggested other hallmarks as dysregulated nutrient sensing, functional imbalance in mitochondria, senescence, stem cell exhaustion, and dysregulated intercellular communication. Measurable biomarkers of aging common to mice and humans include senescence-associated beta-galactosidase (SA-β-gal) staining, leukocyte telomere length, interleukin 6 (IL-6), other SA-cytokines, tumor suppressor protein p16INK4a (an inhibitor of CDK4) mRNA in T lymphocytes, and DNA methylation (Sorrentino et al., 2014). Exposures to certain toxicants (named as “gerontogens”) enhance physiological aging (Sorrentino et al., 2014) as well. Exposure to unknown gerontogens explains much of the non-genetic variation in the rates of physiological aging processes (Sorrentino et al., 2014). A gradual slowing down of metabolism with age is also documented. A very recent review on the “aging metabolome” enumerated the set of biomarkers and hub metabolites of aging, indicating critical roles for nicotinamide adenine dinucleotide (NAD+), reduced nicotinamide dinucleotide phosphate (NADPH), α-ketoglutarate, and β−hydroxybutyrate with a central role of the TCA cycle in signaling and metabolic dysregulation associated with aging (Sharma and Ramanathan, 2020). As very recently commented by Di Ciaula and Portincasa, “successful aging could begin during in utero life” (Di Ciaula and Portincasa, 2020). However, there are currently no available literature where aging has been looked through the lenses of chemical exposures at an omics scale, i.e., an exposomal basis of aging. The connection between our chemical environment and its role in human aging is far from established, and we are just beginning to explore.
For the purpose of this review article, the term “environment” refers only to specify the “macroenvironment” (i.e., the habitat and dwelling space) and not the “microenvironment” such as those inside cells, organelles, and tissues etc. as noted elsewhere (Misra, 2019). Also, the term “exposome” is used in this review to exclusively include exogenous chemicals, and not the physical exposures such as noise, radiation, water, soil, education, societal, spiritual, occupational, and those different from the internal exposome (i.e., metabolism, physical activity, genetics, endocrine factors, inflammation, chronic diseases, stress etc.) (Misra, 2019).
Available literature was screened from 1980 to 2020 (till date of communication) at PubMed (Figure 1A), Google Scholar, Scopus and bioRxiv literature databases manually for information content with various key words and their combinations such as: “exposome,” “exposomics,” “chemical,” exposures with “aging” and “age.” The output was manually curated to include accessible scholarly articles that were in English. The limited resulting literature combining aging and exposure studies encompass biological to epidemiological studies that span in vitro cell lines, animal models such as mice, rat, and Caenorhabditis elegans based studies, to those investigated human cohorts, i.e., epidemiological studies. Also, to avoid the complexity of diet/nutrition (i.e., duration, type, diversity etc.) as an exposure which may include hundreds of epidemiological and intervention studies, I have excluded these studies, as the diets themselves remain chemically complex and uncharacterized in most studies.
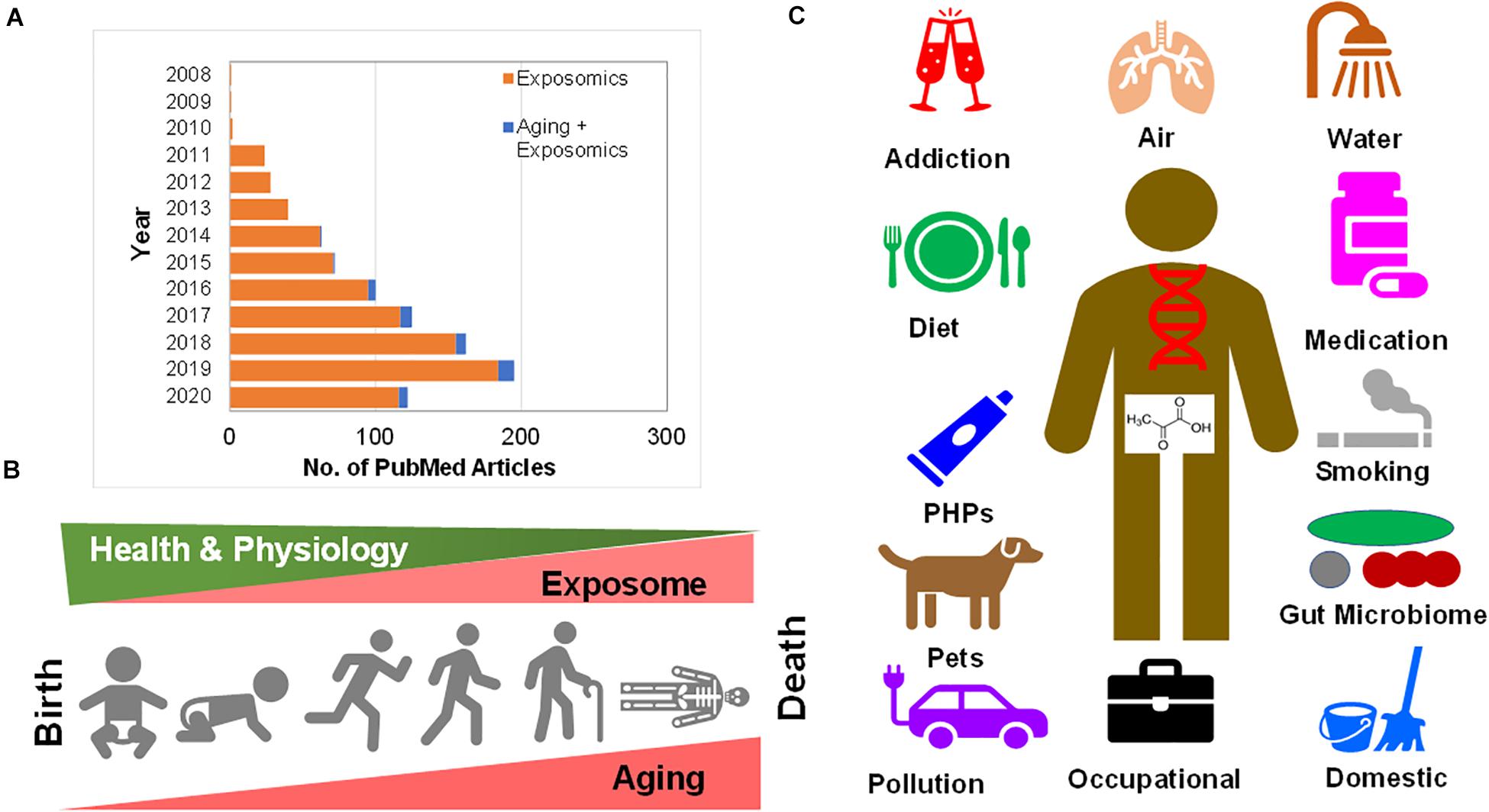
Figure 1. (A) Growth in research publications at PubMed pertaining to “exposomics” and “exposomics and aging” research. (B) Human aging from child birth (neonatal) through adolescence and the ultimate of life, death are riddled with exposure to chemicals surrounding us. As with the incremental aging one experiences physiological decline, possibly the cumulative exposome increases inside human body. (C) Human chemical exposure ranges from addiction (alcohol, drugs, cocaine, and smoking), to air pollution (PM, gases, industrial vapors), water (pollutions, pesticides, and PFAS), drugs (pharmaceuticals, and medications), the resident internal and external gut microbiome, domestic waste and indoor pollution (dust, cooking, and gases), occupational exposures (hospital disinfectants to asbestosis), pollution (fossil fuel burning to plastic), pets (carrying environmental pollution to microbiome), personal hygiene products (PHPs) (perfumes to soaps) to diet (and nutrition).
The Aging Exposome: Hallmarks of Aging Captured in Past Studies?
Environmental pollution is considered as a major exposure affecting human health. The major sources of pollutions are particulate matter (PM), polycyclic aromatic hydrocarbons (PAHs), volatile organic compounds (VOCs), nitrogen, sulfur dioxides, carbon monoxide, ozone, and heavy metals. A quick browsing of extant literature suggested that studies on the effects of physical exposures such as sun (light), sound, noise, ultraviolet (UV) light, temperature on skin aging out number, the chemical exposure based aging studies.
In Figure 1B depicted is the progression of human aging from child birth (neonatal) through adolescence and the ultimate of life, death, that are riddled with exposure to chemicals surrounding us. As with incremental aging one experiences physiological decline, possibly as a result of the cumulative exposome that increases inside the human body. As this review was being submitted and peer-reviewed, an excellent new review was published that provided insights on molecular changes associated with exposures, causality, temporality in pathways leading to diseases, association of biological aging with socio-economic position, challenges with complex, multiple, and concurrent exposures and advancements in metabolomics and adductomics from a tool development perspective (Vineis et al., 2020). In the following sections, the existing literature on “aging exposome” have been thematically organized into several sub-sections purely based on the available content.
The Skin Aging Exposome
The exposome resulting in skin aging is often attributed to pollution, solar radiation, smoking, temperature, nutrition, stress, and lack of sleep (Krutmann et al., 2017). The solar spectrum, comprised of ultraviolet light, can cause skin damage, including skin cancers, where atmospheric factors such as air pollution (i.e., smog, ozone, PM, etc.) are associated with premature skin aging (McDaniel et al., 2018). This review also noted that skin damage brought upon by environmental exposure is attributed to an array of chemical reactions initiated by elevated cellular levels of reactive oxygen species (ROS) in skin cells, which further causes oxidative insult to biomolecules such as proteins, lipids, and nucleic acids. Generally speaking, air pollution was shown to be a major exposure to have a large impact on human skin aging.
In Figure 1C, summarized are the sources of human chemical exposures that range from addiction (alcohol, drugs, cocaine, and smoking), to air pollution (particulate matter-PM, gases, and industrial vapors), water [pollutions, pesticides, per- and polyfluoroalkyl substances (PFAS) etc.], drugs (pharmaceuticals and medications), the resident internal and external gut microbiome, domestic waste, and indoor pollution (dust, cooking, and gases), occupational exposures (hospital disinfectants to asbestosis), pollution (fossil fuel burning to plastics), pets (carrying environmental pollution to microbiome), personal hygiene products (PHPs) (perfumes to soaps) to diet (and nutrition).
Air Pollution Exposome in Aging
In 2018, the World Health Organization (WHO) recognized air pollution as the second-largest risk factor of non-communicable diseases, as more than 90% of global population breathe air quality that does not meet WHO definitions of clean air (Peeples, 2020). The US Environmental Protection Agency (EPA) defines particulate matter (PM), PM10 and PM2.5, as particles less than 10 and 2.5 μm in diameter, respectively (Peeples, 2020). Ambient atmospheric PM causes damage through generation of oxidative stress, that eventually leads to skin aging (Schroeder et al., 2006). More frequently, PM2.5 and PM10 have been more popularly studied in relation to human aging. In Table 1 listed are studies that associated various chemical exposures and their effect on aging in various human cohorts.
Air pollution exposure is also correlated with signs of skin aging such as pigment spots and wrinkles (Vierkötter et al., 2010). Increased soot and particles from traffic were associated with 20% higher pigment spots on forehead and cheeks (Vierkötter et al., 2010). Exposure to sun and smoking leads to enhanced risk for developing wrinkles than those in non-smokers and/or with reduced exposure to sun (Yin et al., 2001). Another study that explored the effect of PM2.5 (indoor air pollution) on two groups of individuals revealed that PM2.5 exposure levels were positively associated with skin aging, i.e., scores based on pigment spots on forehead and wrinkles on upper lip (Ding et al., 2017). Another study that investigated 522 participants of the Normative Aging cohort demonstrated that out of the PM2.5 component species, sulfate and ammonium were associated with at least a 0.36-year increase in Horvath DNAm-age (i.e., a measure of DNA methylation based age assessment) (Nwanaji-Enwerem et al., 2017). Other in vitro studies have shown that matrix metalloprotease I (MMP-1) expression was increased in fibroblasts upon exposure to either tobacco smoke extract or UVA. Moreover, PM, soot and nitrogen dioxide (NO2) have been associated with skin aging in several studies as reviewed elsewhere (Schikowski and Hüls, 2020). Further, epidemiological findings have suggested that the associations of PM can be altered by UV radiation, and provided evidence for an association of tropospheric ozone (O3) with wrinkle formation, independent of NO2, PM, and UV (Schikowski and Hüls, 2020). Currently, several research efforts are focussed at using untargeted chemical/exposomic profiling of the airborne organic exposome.
In the German KORA (Cooperative Health Research in the Region of Augsburg) F4 cohort, a follow-up study of the KORA S4 cohort (a “population-based” health survey conducted in the city of Augsburg and two surrounding counties between 1999 and 2001), it was shown that absorbance/black carbon (BC) and PM10 are associated with biological aging in men (Ward-Caviness et al., 2016). Further, in the Normative Aging Study conducted in the greater Boston, United States that consisted of male participants, revealed a positive association between BC and markers of inflammation in men with coronary heart disease (CHD) and diabetes (Fang et al., 2012). In the Washington Heights Inwood Community Aging Project (WHICAP), a prospective study of aging and dementia conducted with 4821 participants, higher concentrations of ambient air pollution [i.e., NO2, fine (PM2.5), and coarse (PM10)] were associated with rapid cognitive decline, and this association was higher in APOE-ε4 carriers (Kulick et al., 2020a). The same WHICAP cohort with 5,330 participants revealed that an increase in NO2 was associated with lower global cognitive score and its rapid decline (Kulick et al., 2020b). Moreover, aging and neurotoxicant induced neurodegeneration associated miRNAs include, miR-34 (targets genes such as P53, SIRT1, and PARKIN) miR-29 (targets VDAC1, P53, and BACE1) and miR-126 (targets Insulin/IGF-1/PI3K/AKT associated pathways) (Singh and Yadav, 2020), indicating miRNA-based regulation of neurotoxicant induced aging. Indoor pollution studied in two cohort of women from Northern and Southern China (30–90 years age, N = 1,262) revealed that cooking with solid fuels was associated with development of severe wrinkles on face and an increased risk of having fine wrinkles on back of the hands (Li et al., 2015). Cigarette smoking has been highly correlated with high rates of lung cancer from an analysis that leveraged associations between 2162 environmental exposures and lung cancer mortality rates in 2067 counties in the US (Juarez et al., 2017). A recent study that looked at the lung transcriptome in cigarette smoke-exposed mice predicted premature aging as indicated by gene expression patterns that showed strong upregulation of immunoglobulin genes in response to increased age and smoke exposure (Choukrallah et al., 2020). With increased global pollution (both indoor and outdoor), anthropogenic activities, and global climate change, skin aging might be accelerated as catalyzed by the deleterious chemical exposome.
Effect of Exposome on Aging: Telomere Length
Telomere length attrition is an important hallmark of cancer. Numerous reviews have shown that exposure to air pollution and low residential green space exposure are associated with shortened telomere length (Martens and Nawrot, 2016). Further, particulate air pollution exposure can affect telomere mitochondrial axis of aging to display chronic health effects of air pollution, the telomere maintenance (and length) was proposed as a proxy for assessing the exposome (Martens and Nawrot, 2016). The environmental and occupational exposures associated with shorter telomere length are traffic-related air pollution (i.e., PM, BC, benzene and toluene), polycyclic aromatic hydrocarbons (PAHs), N-nitrosamines, pesticides, Pb, and deleterious waste exposure (Zhang et al., 2013). However, arsenic (As), persistent organic pollutants (POPs) and short-term exposure to PM were associated with longer telomere length (Zhang et al., 2013). Residential green space, lower traffic exposure, and long-term lower exposure to PM were associated with longer telomeres in children, young adults, and older individuals (Martens and Nawrot, 2018). Occupational exposures such as toxic metals, PAHs, and PM were associated with decreased telomere lengths for a given age, and a more recent exposure was associated with longer telomeres (Martens and Nawrot, 2018). For instance, 5-μg/m3 increment in annual PM2.5 concentration was associated with a relative decrease of 16.8% in telomere length and a relative decrease of 25.7% in mtDNA (mitochondrial DNA) content in an elderly population (n = 166, non-smokers) (Pieters et al., 2016). PAH-exposure led to reduced telomere length in peripheral blood cells in male non-drinkers when compared for PAH-exposed workers and controls (Bin et al., 2010). Bisphenol A (BPA) levels were strongly associated with senescence, inflammation, and were negatively correlated with the length of telomeres in patients with T2DM (n = 30) (Soundararajan et al., 2019). In a multicentre European birth cohort study HELIX (Human Early Life Exposome), reduced exposures to air pollution during pregnancy and childhood were associated with longer telomeres in children in Europe (Clemente et al., 2019). In the same HELIX cohort it was shown in another study that early life tobacco exposures via maternal smoking (measured as maternal urinary cotinine levels) during pregnancy may induce biological aging from early age as indicated in the shortened telomere lengths in the off springs (Osorio-Yáñez et al., 2020). In another study (166 non-smoking elderly participants) the increases in PM2.5 concentrations were associated with decrease in telomere length and a relative decrease in mitochondrial DNA (mtDNA) (Pieters et al., 2016). In a birth cohort study (n = 641, mother-new-born pairs) revealed that mothers with increased residential PM2.5 exposure gave birth to new-borns with significantly lower telomere length (reduced by 9 and 13% in cord blood and placental telomeres, respectively) (Martens et al., 2017). Another recent study that probed the combined effect of PAHs and phthalates exposure on telomere length and lung function in a pilot study conducted during the winter of 2014 and summer of 2015 in Wuhan city, China, revealed that 8 urinary monohydroxylated-PAHs (OH-PAHs) showed an overall effect on telomere length or lung function (Hou et al., 2020). When a study consisting of coke-oven workers (n = 1,005, males) looked at the association of PAH exposure and mosaic loss of chromosome Y (mLOY), one of the most common structure somatic event contributing to disease and mortality, found that a 10-fold increase in urinary 1-hydroxynaphthalene (1-OHNa), 1-hydroxyphenanthrene (1-OHPh), 2-OHPh, 1-hydroxypyrene (1-OHP) resulted in increased incidence of mLOY in a dose-dependent linear association (Liu et al., 2020). In another study that monitored healthy women residing in the Cape Town region of South Africa revealed that levels of personal NO2 and benzene exposure was inversely associated with leukocyte telomere length, where the magnitude of effects corresponded to > 6 year increase in chronological age (Everson et al., 2020). For a more recent update on air pollution and skin aging the readers are highly suggested to consult a recent review (Schikowski and Hüls, 2020). Evidence from these aforementioned studies and those provided in Table 1 indicate that reductions in air pollution (i.e., traffic and fossil fuel burning related), and environmental exposures (i.e., BPA, PAHs) may promote molecular longevity, as exemplified by telomere length, starting with neonates.
Heavy Metals Accelerate Human Aging
Several heavy metals and their derivatives are implicated in accelerating human aging. Of the limited number of studies available, lead (Pb), aluminum (Al), and other heavy metals are reported to be associated with human aging. Pb, is known to affect the aging brain, and is associated with neurodegenerative diseases such as Alzheimer’s disease, and Parkinson’s disease, in addition to its ability to impact the epigenome (i.e., DNA methylation, histone modifications) and miRNA expression across diverse organisms (Eid and Zawia, 2016). Various studies suggest the role of Al in hastening brain aging resulting in increased incidence of specific age-associated neurological ailments (Bondy, 2014). Another study conducted in rats showed that neonatal exposure to Pb resulted in an enhanced expression of the β amyloid (Aβ) precursor protein (APP) gene, increased levels of APP and its proteolytic product, the Aβ peptide, and oxidative DNA damage in the aging brain (Basha, 2005; Bolin et al., 2006). A study on primate brains exposed to Pb as infants revealed that early life exposure to Pb reprogrammed the gene expression resulting in both up and down-regulation of genes through alternate epigenetic pathways contributing to an enhanced neurodegeneration in old age (Bihaqi et al., 2011). Another study conducted on 777 male participants (between August 1991 and October 1996) as part of the Department of Veterans Affairs Normative Aging Study revealed a positive association between patellar bone Pb levels and uric acid levels where 6.7% participants developed gouty arthritis (Shadick et al., 2000). Another study conducted on the Normative Aging Study of the Department of Veterans Affairs (n = 744 men) revealed that the creatinine clearance rate was negatively associated with increasing blood Pb levels (Payton et al., 1994). Another recent study that performed metabolomics on plasma samples from 399 men with measured Pb exposure in the VA Normative Aging Study cohort revealed that out of 858 metabolite quantified, 154 (17.9%) metabolites showed significant association with blood Pb levels (Kelly et al., 2020), where benzoate metabolism associated with long-term Pb exposure as assessed by toenails. In another recent study on 683 elderly men in the Normative Aging Study (conducted between 1999 and 2013), looking at DNAm PhenoAge acceleration (DNAmPhenoAccel), a new epigenetic biomarker of phenotypic age revealed that Pb and calcium (Ca) were significantly associated with DNAmPhenoAccel, whereas PM2.5, Pb, and silicon (Si) were predictors for DNAmPhenoAccel (Wang et al., 2020b). In a study on Canadian Study of Health and Aging (CSHA), a prospective cohort of 10, 263 subjects followed-up from 1991-1992 to 2001–2002 were probed for the association between Al in drinking water and Alzheimer’s disease but did not find a clear association between them (Van Dyke et al., 2020). In summary, based on the limited observations to discern the effect of heavy metals as an exposure on human aging needs more mechanistic studies on human metabolism, ethnicities, and through multiple model systems to ascertain the exact role heavy metals play in human health.
Addiction (Alcohol, Smoking, and Drugs) and Other Exposures Affecting Human Aging
Addictions are another group of exposures that are chemically diverse, such as ethanol, cocaine, and nicotine. Smoking history decreases a human lifespan on average by 7 years (Bernhard et al., 2007), as smokes from cigarettes are known to contain > 4,000 toxic chemical compounds. Alcohol consumption has been associated with the higher DNA formamidopyrimidine DNA glycosylase (FPG)-sensitive sites, and only in the younger group of healthy women (i.e., > 40 years age) (Gonçalves Mota et al., 2017) (P < 0.05). Smoking as an exposure was associated with increasing telangiectasia and elastosis of skin in a study on 966 individuals investigated for environmental and genetic risk factors of skin cancer (Kennedy et al., 2003). Smoking habit was shown to be a significant independent predictor of skin aging in a Spanish population (n = 1,474 participants, 18–60 years old) (Buendía-Eisman et al., 2020). For occupational exposures, solvents used in carpentry can affect the aging process via changes in cognitive status when compared to unexposed individuals. Older subjects (i.e., carpenters) that were > 60 years old showed significantly high deterioration in visual memory and perceptual speed (Nilson, 2002). Among other life exposures, in older subjects (n = 1,819) exposed to hospital procedures such as general anaesthesia and surgery revealed a subtle decline in cognition (Schulte et al., 2018). Even biological exposures such as viral infections, i.e., human immunodeficiency virus (HIV) and cytomegalovirus (CMV) affect an aging immune system (Leng and Margolick, 2020). In summary, exposures that negatively affect aging can be chemically diverse, ranging from those originating with recreation or addition to those associated with a disease or its drug treatment.
Chemoprotection From Aging
There are also exposure chemicals that are known to be protective against progressive human aging- and used either as desirable or undesirable substances. For instance, rats fed a diet with 0.06 or 0.6 ppm of selenium (Se) as sodium selenite enriched diet, revealed that Se increased running in unexposed animals as they aged, and protected them against methylmercury (MeHg) (provided in drinking water for 16 months at 0.5, 5.0, or 15 ppm doses of Hg) induced neurotoxicity (Heath et al., 2010). Also, crocin, from saffron plant (a dietary component in the form of a spice), displayed ROS amelioration, and protected cellular squalene from UVA-induced peroxidation (Fagot et al., 2018).
In Figure 2, summarized are human chemical exposures that have been associated with aging- heavy metals/ions, particulate matter (PM) of various sizes (PM2.5, PM10), BC, pesticides, PDBEs, phthalates, BPA, drugs (methamphetamine, paracetamol), metalloids (methylmercury, arsenate) and chemicals from addiction (ethanol, nicotine, and cocaine).
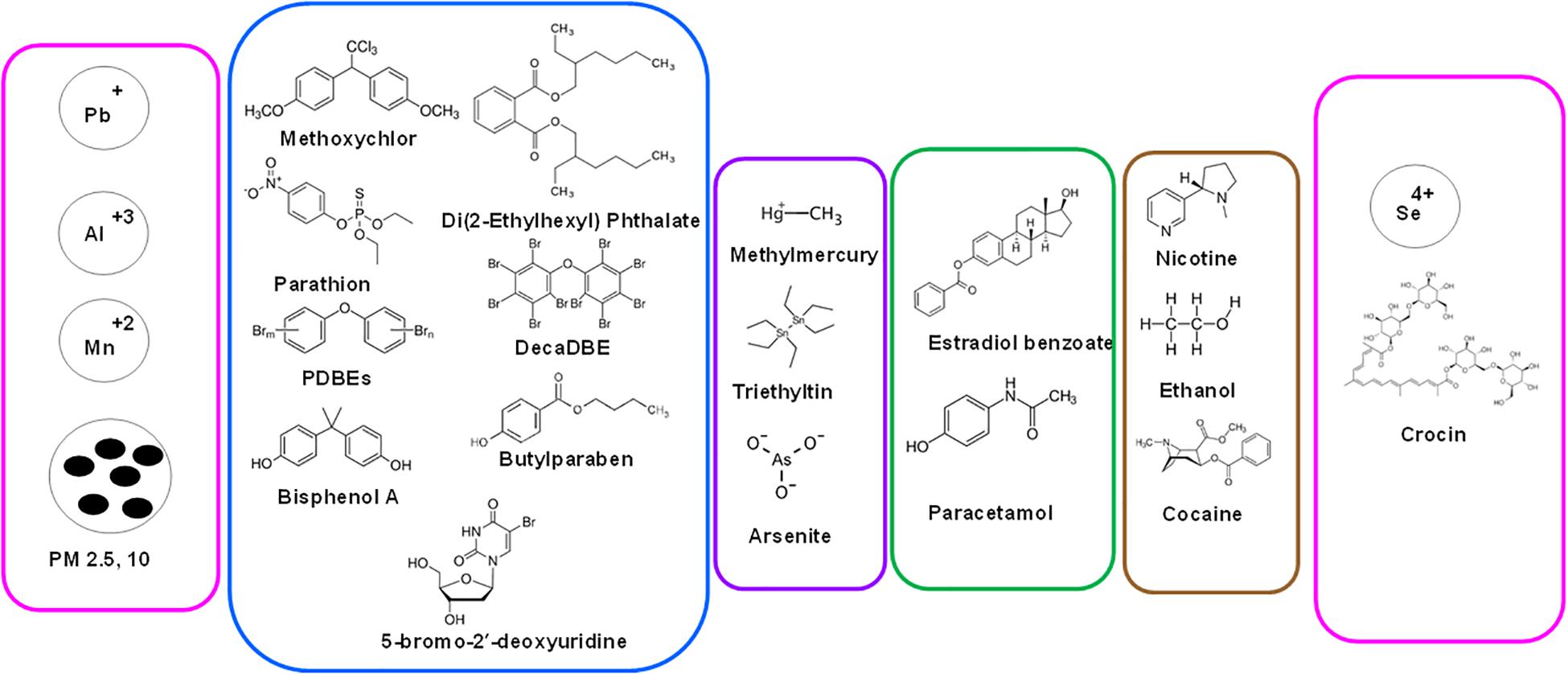
Figure 2. Range of human chemical exposures that are associated with aging. From heavy metals/ions to particulate matter (PM) of various sizes (PM2.5, PM10), carbon black (BC), pesticides, PDBEs, phthalates, BPA, drugs (methamphetamine, paracetamol) to metalloids (methylmercury, arsenate) to chemicals from addiction (ethanol, nicotine, cocaine). Shown also are selenium and crocin (from Saffron plant) that exert protective effect on aging.
Evidence From Exposome-Aging Association Studies Conducted in Animal Models
Experimental animal model systems have always provided meaningful mechanistic insights into the processes of biological aging as well as for understanding of the physiological responses of an exposure to aging in humans. Simple exposure to low-dose estradiol during development and to the endocrine disruptor chemical (EDC), BPA result in increased susceptibility to prostatic intraepithelial neoplasia (PIN) lesions with aging upon cumulative adult exposure to estradiol (Prins et al., 2007). Very recently, using a rat model of exposure to an EDC, it was demonstrated that early-life chemical exposure caused metabolic dysregulation in adulthood as evident from the reprogrammed histone signatures in the developing liver (Treviño et al., 2020). In this study it was demonstrated that EDC exposure hijacked age-related epigenomic plasticity to accelerate epigenomic aging. Moreover, it was Horvath who first proposed the “epigenetic clock” (i.e., DNA methylation (DNAm)-based estimates of age), that provided an precise age prediction based on the total methylation levels of multiple CpG loci (Horvath et al., 2015). Among other models, a recent study conducted in zebrafish (Danio rerio) model for cannabidiol (CBD) exposure revealed significantly enhanced survival and reduced size of female fish and reduced sperm concentration in male fish (Pandelides et al., 2020). Table 2 provides a list of studies that investigated exposures and their effects on aging in animal models and through in vitro studies. The following sections cover the findings from multiple model systems in age- chemical exposure association studies.
In vitro Cellular Model Systems
Mammalian cell culture models offer a time-proven quick in vitro, high throughput, inexpensive assay and screening capabilities for bioactivity screening of exposures, and have helped obtain enormous insights into in vivo metabolism of chemical exposures, though with all sorts of limitations of the system. Diverse studies have been performed to study the mechanisms of action and toxicity of chemical exposures, though studies w.r.t aging are scarce. In one such exposure study in in vitro cell models, when 5 μM of fatty acid hydroperoxides (OFA) were exposed to cells for 40 passages, age-related decrease in endothelial barrier function (EBF) was observed, predisposing the artery to infiltration by blood components and eventual atherogenesis (Boissonneault et al., 1990).
Caenorhabditis elegans Models
The model organism, Caenorhabditis elegans has been widely used to screen the effects of diverse chemical exposures over the years for the amenability and simplicity of this model system among other reasons. The C. elegans model has lend itself as a top model in developmental toxicity studies (Boyd et al., 2012) and has helped provide mechanistic insights on toxicity of POPs and EDCs (Chen et al., 2019). With C. elegans already a well-established model of aging (Johnson, 2008; Tissenbaum, 2015; Denzel et al., 2019), it offer the benefits of using this model system for studying individual/multiple chemical exposures w.r.t to aging research. Assays performed using chemotherapeutic agents and environmental compounds from ToxCast Phase I library helped compare results against the mammalian in vitro end point data from ToxRef database (DB) with regards to germline function (Allard et al., 2013). Further, several medium-throughput (feeding, growth, reproduction, and locomotion) and two high-throughput (growth and reproduction) assays have been developed in C. elegans to decipher the effects of potential neurotoxicants (Boyd et al., 2010b). Moreover, several chemical exposures such as chlorpyrifos (Roh and Choi, 2008), dibutyl phthalate (DBP), a likely endocrine disruptor and frequently used plasticizer, and the pesticides 2-(thiocyanomethylthio) benzothiazole (TCMTB) and permethrin (Shin et al., 2019), 1-alkyl-3-methylimidazolium chloride ionic liquids (Swatloski et al., 2004), heavy metal cadmium (chloride) (Boyd et al., 2010a) and arsenite (Wang et al., 2007), chemical repellents such as quinine, denatonium, detergents, other heavy metals (Hilliard et al., 2005), natural estrogens, 17β-estradiol (E2), and environmental pollutants such as bisphenol A (BPA) and tributyltin chloride (TBTCL) (Hoshi et al., 2003), triclosan (TCS) and triclocarban (TCC) (Lenz et al., 2017), nanopolystyrene (Qiu et al., 2020) among others. BPA exposure was shown to increase the generation of hydrogen peroxide-related ROS and superoxide anions, further accelerating the aging process by inducing oxidative stress (Tan et al., 2015). Two studies that probed arsenite exposure on C. elegans revealed a reduced amount of defecation, increased intestinal lipofuscin. and lipid peroxidation with age alongside increased intracellular ROS levels (Yu et al., 2011). Further, co-exposure to ROS scavengers prevented the lifespan-extending capabilities of As (i.e., mitohormesis) (Schmeisser et al., 2013). These studies indicate that C. elegans will continue to lead the path in studying both exposomics and aging in the same model.
Rat Models
As a simpler mammalian model system, rats have been used extensively in research encompassing chemical exposures over the past few decades. Rats showed long-term effects on emotional behavior that led to depressive symptoms when exposed to prenatal high doses of both cocaine and nicotine (Sobrian et al., 2003). Alcohol (ethanol) exposure in rats (during gestation to parturition) showed a peripheral auditory disorder, i.e., congenital sensorineural hearing loss and age-related deterioration of auditory acuity (Church et al., 1996). Rats exposed to endocrine disruptor chemicals (EDCs) such as estradiol benzoate (EB) and MXC (methoxychlor) in early life affected neuroendocrine gene expression, and caused advancement of reproductive senescence (Gore et al., 2011). Exposure to a mixture of EDCs such as, phthalates, pesticides, UV-filters, BPA, butylparaben, paracetamol in pre-pubertal female rats led to a significant reduction in primordial follicle numbers, reduced plasma levels of prolactin, higher incidence of irregular oestrous cycles, and reduced ovary weights (Johansson et al., 2016). Exposure of estradiol benzoate in young female rats led to premature occurrence of vaginal opening, decreased number of corpora lutea, and an increased number of large follicles (Rodriguez et al., 1993).
Several other studies have focussed on the exposure of rats to heavy metals and organometallics. Pregnant female rats (prenatal) exposed to methylmercury (CH3.Hg) slowed the acquisition of choice in older rats (Newland, 2004), whereas a temporal reduction in the reinforcement rate began to appear in low- and high-dose rats (Newland and Rasmussen, 2000). Rats exposed to triethyltin (TET) revealed reduced levels of acquisition and retention of the water maze task as well as morphological damages (Barone et al., 1995). Al exposure resulted in decreased learning performance of the task in both adult and old rats, and an age-related effect on water maze performance (Roig et al., 2006). Rats exposed to synthetic analog of thymidine, 5-bromo-2′-deoxyuridine (BrdUrd) resulted in the following: mean life span decreased significantly, where in female rats the opening of the vagina was delayed, doubled the events of chromosomal aberrations in blood lymphocytes and showed dose-related increase in tumor incidence (Anisimov and Osipova, 1992). Thus, the rat models continue to provide mechanistic insights in response to chemical exposures in laboratory set ups.
Mice Models
Mice models have been used to measure the effects of chemical exposures with respect to aging. Mice orally exposed to di(2-Ethylhexyl) phthalate showed significantly decreased levels of inhibin B, enhanced the BAX/BCL2 ratio in primordial follicles resulting in reduced total number of follicles (Hannon et al., 2016). Mice exposed to decaBDE, a specific polybrominated diphenyl ethers (PBDEs) class chemical, demonstrated a reduction in performance such as light–dark discrimination and learning tasks in older decaBDE treated mice (Rice et al., 2009). Ethyl alcohol was shown to induce severe retention deficit and long-term retention in aging mice (Dumas and Rabe, 1994). Exposure to Pb in female pregnant mice resulted in up-regulation of transcripts related to the immune response, metal-binding, metabolism and transcription/transduction coupling (Dosunmu et al., 2012). Piperine, an alkaloid nutrient component of Piper nigrum, when exposed to a D-galactose-induced aging mouse model ameliorated the D-galactose induced cholinergic malfunction, extensive oxidative stress, neuroinflammation, and hyperphosphorylation of tau protein in hippocampus of these animals (Wang et al., 2020a). Based on the limited studies, one can clearly conclude that the mice models can offer a system to screen single or multiple chemical exposures in various strains that are yet to be explored.
Tools and Techniques to Capture the Exposotypes
Analytical Platforms to Capture Exposome
Generally speaking, past studies have typically focused on measuring the chemical space (i.e., metabolome and now, exposome) in human samples and habitats using spectrometry tools such as mass-spectrometry (MS) hyphenated with diverse chromatography approaches as mentioned elsewhere (Misra, 2019). Primarily these include, gas chromatography (GC)-MS, liquid chromatography (LC)-MS, capillary electrophoresis (CE)-MS etc. or without chromatography, i.e., direct injection (DI)-MS, flow injection analysis (FIA)-MS, imaging-MS and spectroscopy approaches such as nuclear magnetic resonance spectroscopy (NMR), Raman spectroscopy, infra-red (IR) spectroscopy etc. Other MS-based technologies are equipped to provide increased overall measurement dynamic range of environmental chemicals from polar to highly non-polar chemicals from a wide variety of matrices (Misra, 2019).
Software Tools, Databases, and Resources to Annotate Exposome
Over the past decade, there have been explosions in cheminformatics approaches enabling deep data mining efforts in the exploration of human exposome. Both, spectral databases and chemical structure/formula databases have enabled identification of novel compounds in diverse samples/matrices of environmental, biological, and human origin. Improvement of chemical structure databases have enabled development of bioinformatics tools for chemical dereplication and/or annotation of true “unknown unknown” structures. Currently, Mass Bank of North America (MoNA) has collected ∼6.5 million spectra (of which 1.3 million are MS/MS spectra) and the METLIN DB now has data for over 800,000 MS/MS spectral data collected at multiple energies that are accessible. From a data stand point, there are still gaps in the community standards (for exposomics data sharing and archiving), and lacks an active or implemented Minimum Information About an Exposomics Experiment (MIAEEE) (Misra, 2016). In Table 3, listed are current software tools, resources, and DBs to analyze the captured exposome from various analytical platforms.
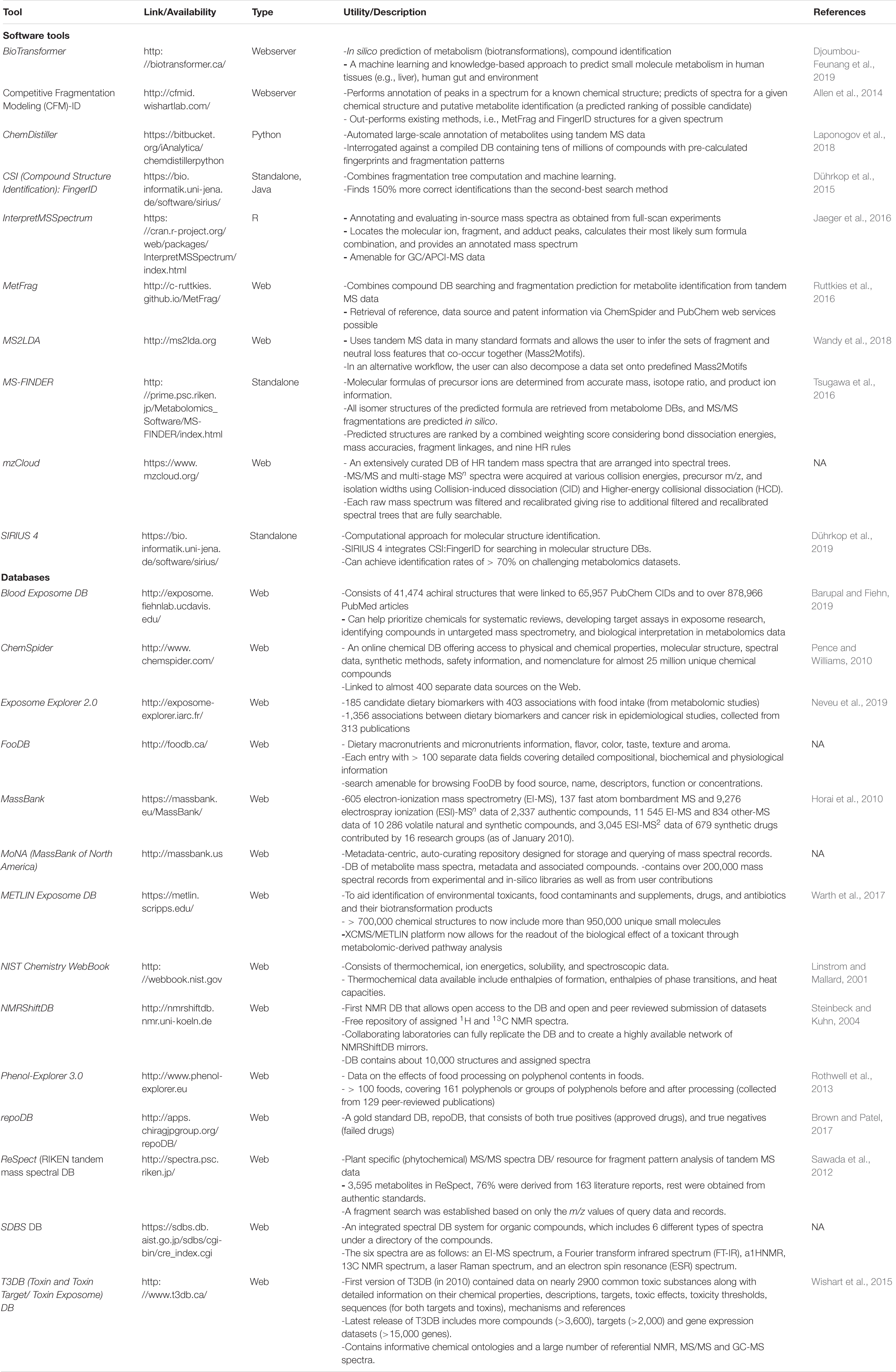
Table 3. List of software tools, resources and chemical and spectral databases for exposomics research.
Software tools such as BioTransformer can help to predict in silico metabolism of a metabolite/environmental chemical by following human metabolic pathway reactions that are gut, tissue or environment specific (Djoumbou-Feunang et al., 2019). Competitive Fragmentation Modeling (CFM)-ID can aid in annotation of spectral peaks, in prediction of spectra of a given chemical structure, and in putative identification of metabolites from unknown spectra (Allen et al., 2014). ChemDistiller can help annotate metabolites using tandem MS data against a compiled DB of millions of compounds (Laponogov et al., 2018). CSI (Compound Structure Identification): FingerID combined fragmentation tree computation and machine learning to help resolve unknown compound identification challenges (Dührkop et al., 2015). InterpretMSSpectrum is a tool suitable for annotating and evaluating in source full-scan mass spectra from gas chromatography-atmospheric pressure chemical ionization-mass spectrometry (GC/APCI-MS) data (Jaeger et al., 2016). MetFrag can perform compound DB searching and fragmentation prediction for metabolite identification of tandem MS spectral data (Ruttkies et al., 2016). MS2LDA uses tandem MS data for inferencing sets of fragment and neutral loss features that co-occur in spectral data for aiding in unknown interpretation (Wandy et al., 2018). Using tools such as MS-FINDER one can determine molecular formulas of precursor ions from accurate mass, isotope ratio etc. where the predicted formulae are retrieved from metabolome DBs and follow the nine hydrogen rearrangement (HR) rules (Tsugawa et al., 2016). mzCloud is a curated DB of HR tandem MS data arranged as spectral trees, where tandem MS and multistage MSn data were acquired at various collision energies. SIRIUS 4 is another computational approach for molecular structure identification (Dührkop et al., 2019).
There are several chemical and spectral DBs that aid in annotation of known exposomic chemicals, metabolites, as well as can help decipher the molecular formulae and structure of unknown chemicals. Leading the pack is the Blood Exposome DB that consists of 65,957 PubChem chemical IDs extracted from 878,966 PubMed article constituting all chemicals ever associated with blood as a biological matrix (including plasma, serum, and blood cell-types) (Barupal and Fiehn, 2019). ChemSpider is a resource linked to > 400 data sources on the web is an enormous chemical DB offering information on physio-chemical properties, molecular structure, spectral data, safety data and nomenclature for 25 million unique chemicals (Pence and Williams, 2010). Exposome Explorer 2.0 is a DB of exposomal chemicals, i.e., 185 dietary biomarkers with 403 associations obtained from food intake, and 1,356 associations between dietary biomarkers and cancer risk from epidemiological studies (Neveu et al., 2019). FooDB is a DB with information of dietary micro and macronutrients, with > 100 separate data fields for each chemical entry covering a chemical’s compositional, biochemical and physiological information. MassBank is a spectral DB with 100s of EI-MS, ESI-MS/MS, FAB-MS spectra for authentic metabolites, volatile and synthetic compounds, drugs etc. (Horai et al., 2010). MoNA is a mass spectral DB with 200,000 mass spectral records from experimental and in-silico libraries as well as from user contributions. METLIN Exposome DB can aid in identification of environmental toxicants, food contaminants, supplements, drugs, and their biotransformation products from > 950,000 unique chemicals. NIST Chemistry Webbook is a DB with thermochemical, ion energetics, solubility and spectrometric data (Linstrom and Mallard, 2001). NMRShiftDB is the first ever free repository of assigned 1H and 13C NMR spectra that contains > 10,000 structures and assigned spectra (Steinbeck and Kuhn, 2004). Phenol-explorer 3.0 is a DB with information on effects of food processing on polyphenol contents for > 100 foods, covering 161 polyphenols (Rothwell et al., 2013). RepoDB consists of information on approved and failed drugs as highly curated DB (Brown and Patel, 2017). ReSpect (RIKEN tandem mass spectral DB is a phytochemical tandem MS spectral DB that allows fragment pattern analysis from raw spectra based on the information content of the DB that has spectra from 3595 metabolites obtained from authentic standards (Sawada et al., 2012). SDBS DB is a spectral DB for organic compounds that has 6 different spectra types, i.e., EI-MS, FTIR, 1HNMR, 13CNMR, laser Raman and EST spectra. T3DB (Toxin and Toxin Target/Toxin Exposome DB) consists of compounds (>3,600), targets (>2,000) and gene expression datasets (>15,000 genes) and chemical ontologies and a large number of referential NMR, MS/MS and GC-MS spectra. For common toxic substances (Wishart et al., 2015). Other resources include such as the NORMAN Suspect List Exchange (NORMAN-SLE), that was established in 2015 for NORMAN members (and others) to find suspect lists relevant for their environmental monitoring1. Figure 3 displays the combined approach of using high throughput analytical platforms (i.e., LC-MS/MS, GC-MS, NMR, other spectroscopy) to enable capture the aging “chemical exposome,” that further uses the power of computation in exploiting the software tools, DBs and resources available to aid in identification of the known and unknown chemical exposures.
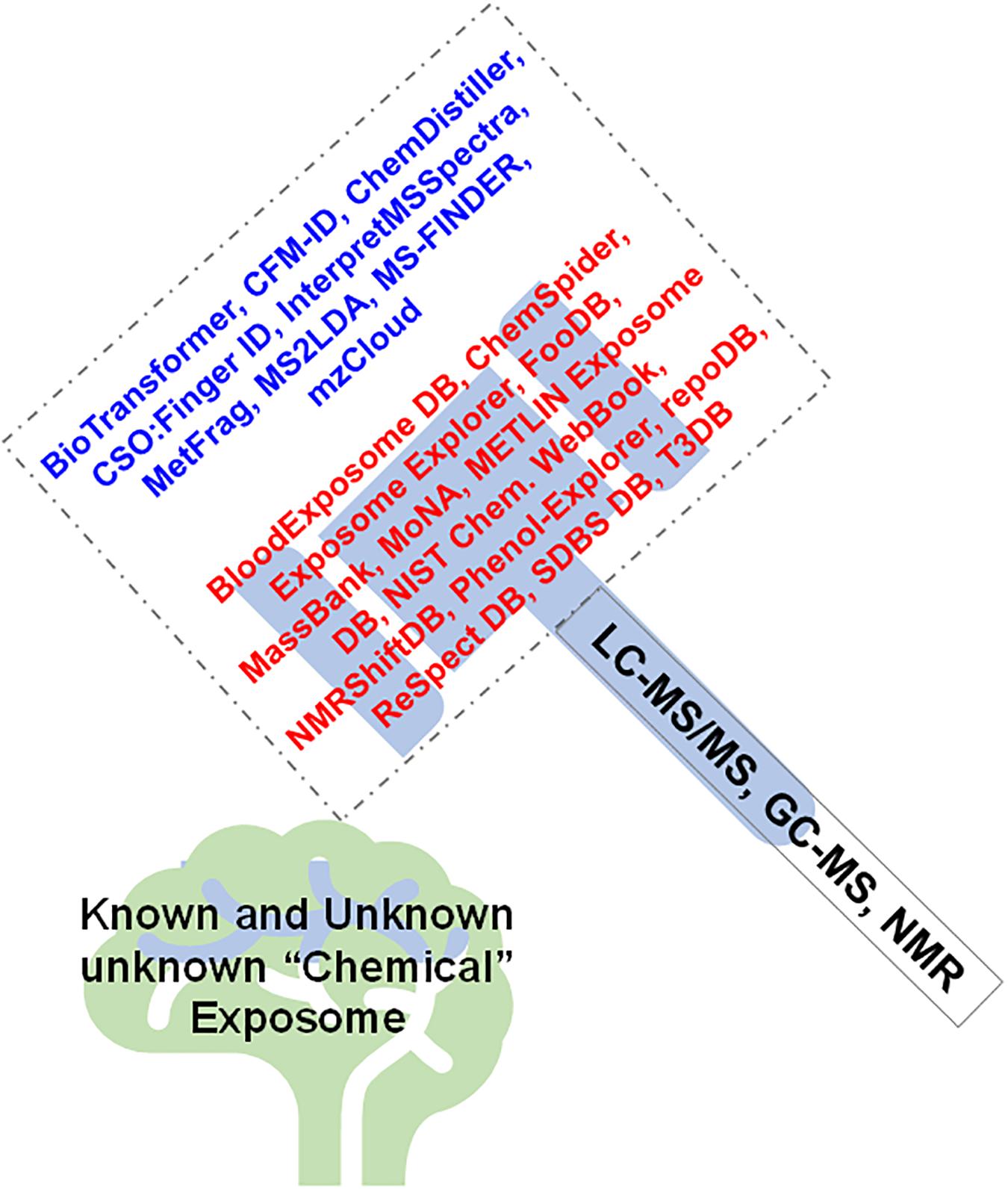
Figure 3. Mass spectrometry and spectroscopy provide the necessary analytical tools that aid in capturing the aging “exposome,” which when processed by the software tools, resources, and databases enable to “crack” (identify) the known and unknown chemical exposome.
Opportunities and Challenges in Studying the Aging Exposome: Unanswered Questions and Future Scope
A glance at the above literature from the past limited studies on the aging-exposome association, with scattered evidence from both animal models and human cohorts raise a handful of questions that warrants answers. Some of these questions are provided below:
1. How does one translate the exposome studies in cell lines, C. elegans, and mice models to human applications, given limitations of the models and at the same time challenges associated with human studies (i.e., an exposomal intervention study other than diet)?
2. What contributes to aging more- the genome or the exposome, both, or is it more dependent on the exposure in question?
3. If there is a need for exposome DB, then beyond the blood exposome DB, one needs to explore organ system (i.e., liver, kidney, gut exposome DBs) and model based exposome DBs to further our understanding of the human systemic exposome in the context of aging?
4. Can the international efforts in studying human aging from various dimensions (biochemical, ethnicities, pathophysiological, brain to gut microbiome studies) come together to collectively explore the “universal exposome signature of human aging”?
5. Do we need different and custom statistical models to associate aging with exposome (i.e., as exposures follow different regulations from genes, proteins, and metabolites that are endogenous) given that the exposome is cumulative, simultaneous, and interactive?
6. How would one decompose the biomolecular signatures of aging from those that are exposome mediated processes in human and other experimental models?
7. What could be the impact of the internal gut microbiome and external skin microbiome on human aging and how to best assess these across human populations?
8. What would the human aging exposome convey toward understanding of human health (both, wellbeing and disease) such as metabolic syndromes, cancer, cardiovascular, and neurodegenerative diseases?
9. How does the aging exposome research reach the stakeholders, governance, policy makers, health practitioners, and general population to realize the goals of this as global translational research endeavor?
10. Are we at a stage to sample personalized exposome or focus on community level stressors such as population, time, space, and region specific exposomes wrt. to aging research?
11. How would the findings from human aging exposome be used to change the human environment to help slower the process of biological or healthy aging?
12. Does the exposome affect males and females differently in humans and animal models, i.e., sex-specificity of the exposome?
13. Does the exposome of aging promises to deliver exposomal biomarkers of aging, independent of endogenous metabolism and genetics?
14. How can one leverage the exposomal accelerators of aging for identifying therapeutic targets in the scale of an individual (precision medicine) and at the population scale?
15. Does specific human genotypes and phenotypes predispose individuals to exposome- mediated aging to varied degrees?
Undoubtedly, exposomics allows one to study interactions between chronic stress and environmental chemicals that disrupt stress response pathways (i.e., “stressogens”) (Smith et al., 2015). In the future, multiple human cohorts and animal model based aging studies can be revisited form a systems biology stand point to capture the entirety of exposome and associate it with human aging. Clearly, in the future to capture the holistic exposome, one needs to obtain data from questionnaires (i.e., occupation, smoking history, habits, diseases, dietary choices), GIS-based environmental models (i.e., air pollution, heavy metals), images/pictures (i.e., usage of PHPs, food, cleaning products), mobile devices (i.e., smart phones to environmental sensors, wearable devices) among others to be able to obtain biomarkers across multiple time points and tissues following diverse approaches (Siroux et al., 2016). Approaches such as targeted and non-targeted exposomics efforts that look into dose-dependency and time-specific response to the exposome will be meaningful to understand the fate of a given chemical exposure, its transformation and degradation products during the course of human aging. Its also true that in spite of the availability of evidence on the role of chemical exposures affecting the process of aging, the precise cellular mechanisms and players such as proteins, lipids, nucleotides and carbohydrates as interacting partners of the exposome that negatively affect metabolism at an omics scale are largely understudied. Further, technologies and methods to capture these in vivo interactions are far from established.
Concluding Remarks
In summary, one can safely claim that the vast majority of the studies in the past with “exposure” and “aging” have studied one exposure at a time against a specific aging type, i.e., effect of PM2.5 on skin aging or BPA exposure in rats. The number of studies that have looked at exposures in aging animals, and human population to decipher the association of exposures with aging are very limited. Top most exposures that are studied include air pollution (PM2.5), heavy metals, EDCs, organometallics, addiction compounds (alcohol, nicotine, cocaine), and drugs/medications, thus indicating that there is a myriad of unexplored opportunities in investigating the under studied exposures in relationship to human aging. However, the challenge would be to capture the near-entirety of the human chemical exposome via multiple analytical platforms and data-centric approaches that can complement the enormous challenges associated with capturing the human exposome. We are definitely on right track with high resolution and high throughput analytical platforms from mass-spectrometry and various spectroscopy methods to capture the chemical exposome, and a myriad of annotation tools that with newer in silico approaches and tools lend themselves for identification of new exposomal signatures. The past studies have taken a reductionist approach that may help only understand the role of a single variable, but those will inadequately capture the complexity of the exposome and its interactions. However, to capture such enormity of exposome, one needs multiple longitudinal studies, over ethnically diverse cohorts and experimental model systems for validation with large statistical power. There is a clear need for studying aging in not only entire systems level of an organism, but also for each organ and cell-type in the longer run for obtaining mechanistic insights. Connecting the exposome with aging, in a real word scenario where an individual is subjected to multiple exposures at any point of time, is challenging (i.e., for decomposing the effect of single exposures on aging) yet not impossible (i.e., with evidence and insights from animal model studies). Gained insights from animal model studies, cell cultures, and epidemiological studies need to be contextualized for realizing the goals of associating the chemical exposome with human aging in our engineered environment. Resolving the true “unknown unknowns” [i.e., defined as truly novel compounds that are not represented in spectral DBs and would need the use of computer-aided structure elucidation methods (Wishart, 2009)] from the captured exposome that associate with human aging is going to be critical in understanding the effect of modern human lifestyle on accelerated aging.
Author Contributions
BBM conceived the idea, prepared, and wrote the original review.
Conflict of Interest
The author declares that the research was conducted in the absence of any commercial or financial relationships that could be construed as a potential conflict of interest.
Acknowledgments
BBM acknowledges the reviewers for their critique and help in improving this article.
Abbreviations
CE-MS, capillary electrophoresis mass spectrometry; DB, database; EPA, U.S. Environmental Protection Agency; GC-MS, gas chromatography-mass spectrometry; HMDB, Human Metabolome Database; HRMS, high resolution mass spectrometry; LC-MS, liquid chromatography mass spectrometry; MS, mass spectrometry; MS/MS, tandem mass spectrometry; NMR, nuclear magnetic resonance spectroscopy; VOC, volatile organic carbon.
Footnotes
References
Allard, P., Kleinstreuer, N. C., Knudsen, T. B., and Colaiácovo, M. P. (2013). A C. elegans Screening Platform for the Rapid Assessment of Chemical Disruption of Germline Function. Environ. Health Perspect. 121, 717–724. doi: 10.1289/ehp.1206301
Allen, F., Pon, A., Wilson, M., Greiner, R., and Wishart, D. (2014). CFM-ID: a web server for annotation, spectrum prediction and metabolite identification from tandem mass spectra. Nucleic Acids Res. 42, W94–W99. doi: 10.1093/nar/gku436
Anisimov, V. N., and Osipova, G. Y. (1992). Effect of neonatal exposure to 5-bromo-2′-deoxyuridine on life span, estrus function and tumor development in rats-an argument in favor of the mutation theory of aging? Mutat. Res. 275, 97–110. doi: 10.1016/0921-8734(92)90013-F
Barone, S., Stanton, M. E., and Mundy, W. R. (1995). Neurotoxic effects of neonatal triethyltin (TET) exposure are exacerbated with aging. Neurobiol. Aging 16, 723–735. doi: 10.1016/0197-4580(95)00089-W
Barupal, D. K., and Fiehn, O. (2019). Generating the Blood Exposome Database Using a Comprehensive Text Mining and Database Fusion Approach. Environ. Health Perspect. 127:097008. doi: 10.1289/EHP4713
Basha, M. R. (2005). The Fetal Basis of Amyloidogenesis: Exposure to Lead and Latent Overexpression of Amyloid Precursor Protein and -Amyloid in the Aging Brain. J. Neurosci. 25, 823–829. doi: 10.1523/JNEUROSCI.4335-04.2005
Bernhard, D., Moser, C., Backovic, A., and Wick, G. (2007). Cigarette smoke–an aging accelerator? Exp. Gerontol. 42, 160–165. doi: 10.1016/j.exger.2006.09.016
Bihaqi, S. W., Huang, H., Wu, J., and Zawia, N. H. (2011). Infant Exposure to Lead (Pb) and Epigenetic Modifications in the Aging Primate Brain: Implications for Alzheimer’s Disease. J. Alzheimer’s Dis. 27, 819–833. doi: 10.3233/JAD-2011-111013
Bin, P., Leng, S., Cheng, J., Pan, Z., Duan, H., Dai, Y., et al. (2010). Association between telomere length and occupational polycyclic aromatic hydrocarbons exposure. Zhonghua Yu Fang Yi Xue Za Zhi 44, 535–538.
Boissonneault, G. A., Hennig, B., Wang, Y., and Wood, C. L. (1990). Aging and endothelial barrier function in culture: Effects of chronic exposure to fatty acid hydroperoxides and vitamin E. Mech. Ageing Dev. 56, 1–9. doi: 10.1016/0047-6374(90)90110-2
Bolin, C. M., Basha, R., Cox, D., Zawia, N. H., Maloney, B., Lahiri, D. K., et al. (2006). Exposure to lead (Pb) and the developmental origin of oxidative DNA damage in the aging brain. FASEB J. 20, 788–790. doi: 10.1096/fj.05-5091fje
Bondy, S. C. (2014). Prolonged exposure to low levels of aluminum leads to changes associated with brain aging and neurodegeneration. Toxicology 315, 1–7. doi: 10.1016/j.tox.2013.10.008
Boyd, W. A., McBride, S. J., Rice, J. R., Snyder, D. W., and Freedman, J. H. (2010a). A high-throughput method for assessing chemical toxicity using a Caenorhabditis elegans reproduction assay. Toxicol. Appl. Pharmacol. 245, 153–159. doi: 10.1016/j.taap.2010.02.014
Boyd, W. A., Smith, M. V., and Freedman, J. H. (2012). Caenorhabditis elegans as a model in developmental toxicology. Methods Mol. Biol. 889, 15–24. doi: 10.1007/978-1-61779-867-2_3
Boyd, W. A., Smith, M. V., Kissling, G. E., and Freedman, J. H. (2010b). Medium- and high-throughput screening of neurotoxicants using C. elegans. Neurotoxicol. Teratol. 32, 68–73. doi: 10.1016/j.ntt.2008.12.004
Brown, A. S., and Patel, C. J. (2017). A standard database for drug repositioning. Sci. Data 4:170029. doi: 10.1038/sdata.2017.29
Buendía-Eisman, A., Prieto, L., Abarquero, M., and Arias-Santiago, S. (2020). Study of the Exposome Ageing-related Factors in the Spanish Population. Acta Derm. Venereol. 100:adv00153. doi: 10.2340/00015555-3500
Chen, H., Wang, C., Li, H., Ma, R., Yu, Z., Li, L., et al. (2019). A review of toxicity induced by persistent organic pollutants (POPs) and endocrine-disrupting chemicals (EDCs) in the nematode Caenorhabditis elegans. J. Environ. Manage. 237, 519–525. doi: 10.1016/j.jenvman.2019.02.102
Choukrallah, M. A., Hoeng, J., Peitsch, M. C., and Martin, F. (2020). Lung transcriptomic clock predicts premature aging in cigarette smoke-exposed mice. BMC Genom. 21:291. doi: 10.1186/s12864-020-6712-z
Church, M. W., Abel, E. L., Kaltenbach, J. A., and Overbeck, G. W. (1996). Effects of Prenatal Alcohol Exposure and Aging on Auditory Function in the Rat: Preliminary Results. Alcohol. Clin. Exp. Res. 20, 172–179. doi: 10.1111/j.1530-0277.1996.tb01061.x
Clemente, D. B. P., Vrijheid, M., Martens, D. S., Bustamante, M., Chatzi, L., Danileviciute, A., et al. (2019). Prenatal and Childhood Traffic-Related Air Pollution Exposure and Telomere Length in European Children: The HELIX Project. Environ. Health Perspect. 127:087001. doi: 10.1289/EHP4148
Denzel, M. S., Lapierre, L. R., and Mack, H. I. D. (2019). Emerging topics in C. elegans aging research: Transcriptional regulation, stress response and epigenetics. Mech. Ageing Dev. 177, 4–21. doi: 10.1016/j.mad.2018.08.001
Di Ciaula, A., and Portincasa, P. (2020). The environment as a determinant of successful aging or frailty. Mech. Ageing Dev. 188:111244. doi: 10.1016/j.mad.2020.111244
Ding, A., Yang, Y., Zhao, Z., Hüls, A., Vierkötter, A., Yuan, Z., et al. (2017). Indoor PM2.5 exposure affects skin aging manifestation in a Chinese population. Sci. Rep. 7:15329. doi: 10.1038/s41598-017-15295-8
Djoumbou-Feunang, Y., Fiamoncini, J., Gil-de-la-Fuente, A., Greiner, R., Manach, C., and Wishart, D. S. (2019). BioTransformer: a comprehensive computational tool for small molecule metabolism prediction and metabolite identification. J. Cheminform. 11:2. doi: 10.1186/s13321-018-0324-5
Dosunmu, R., Alashwal, H., and Zawia, N. H. (2012). Genome-wide expression and methylation profiling in the aged rodent brain due to early-life Pb exposure and its relevance to aging. Mech. Ageing Dev. 133, 435–443. doi: 10.1016/j.mad.2012.05.003
Dührkop, K., Fleischauer, M., Ludwig, M., Aksenov, A. A., Melnik, A. V., Meusel, M., et al. (2019). SIRIUS 4: a rapid tool for turning tandem mass spectra into metabolite structure information. Nat. Methods 16, 299–302. doi: 10.1038/s41592-019-0344-8
Dührkop, K., Shen, H., Meusel, M., Rousu, J., and Böcker, S. (2015). Searching molecular structure databases with tandem mass spectra using CSI:FingerID. Proc. Natl. Acad. Sci. 112, 12580–12585. doi: 10.1073/pnas.1509788112
Dumas, R. M., and Rabe, A. (1994). Augmented memory loss in aging mice after one embryonic exposure to alcohol. Neurotoxicol. Teratol. 16, 605–612. doi: 10.1016/0892-0362(94)90038-8
Eid, A., and Zawia, N. (2016). Consequences of lead exposure, and it’s emerging role as an epigenetic modifier in the aging brain. Neurotoxicology 56, 254–261. doi: 10.1016/j.neuro.2016.04.006
Everson, F., Martens, D. S., Nawrot, T. S., Goswami, N., Mthethwa, M., Webster, I., et al. (2020). Personal exposure to NO2 and benzene in the Cape Town region of South Africa is associated with shorter leukocyte telomere length in women. Environ. Res. 182:108993. doi: 10.1016/j.envres.2019.108993
Fagot, D., Pham, D. M., Laboureau, J., Planel, E., Guerin, L., Nègre, C., et al. (2018). Crocin, a natural molecule with potentially beneficial effects against skin ageing. Int. J. Cosmet. Sci. 40, 388–400. doi: 10.1111/ics.12472
Fang, S. C., Mehta, A. J., Alexeeff, S. E., Gryparis, A., Coull, B., Vokonas, P., et al. (2012). Residential Black Carbon Exposure and Circulating Markers of Systemic Inflammation in Elderly Males: The Normative Aging Study. Environ. Health Perspect. 120, 674–680. doi: 10.1289/ehp.1103982
Go, Y. M., and Jones, D. P. (2014). Redox biology: Interface of the exposome with the proteome, epigenome and genome. Redox Biol. 2, 358–360. doi: 10.1016/j.redox.2013.12.032
Gonçalves Mota, M. P., Santos, Z., Soares, J., Pereira, A., Fonseca, S., Peixoto, F., et al. (2017). Oxidative Stress Function in Women over 40 Years of Age, Considering Their Lifestyle. Front. Endocrinol 8:48. doi: 10.3389/fendo.2017.00048
Gore, A. C., Walker, D. M., Zama, A. M., Armenti, A. E., and Uzumcu, M. (2011). Early Life Exposure to Endocrine-Disrupting Chemicals Causes Lifelong Molecular Reprogramming of the Hypothalamus and Premature Reproductive Aging. Mol. Endocrinol. 25, 2157–2168. doi: 10.1210/me.2011-1210
Hannon, P. R., Niermann, S., and Flaws, J. A. (2016). Acute Exposure to Di(2-Ethylhexyl) Phthalate in Adulthood Causes Adverse Reproductive Outcomes Later in Life and Accelerates Reproductive Aging in Female Mice. Toxicol. Sci. 150, 97–108. doi: 10.1093/toxsci/kfv317
Heath, J. C., Banna, K. M., Reed, M. N., Pesek, E. F., Cole, N., Li, J., et al. (2010). Dietary selenium protects against selected signs of aging and methylmercury exposure. Neurotoxicology 31, 169–179. doi: 10.1016/j.neuro.2010.01.003
Hilliard, M. A., Apicella, A. J., Kerr, R., Suzuki, H., Bazzicalupo, P., and Schafer, W. R. (2005). In vivo imaging of C. elegans ASH neurons: cellular response and adaptation to chemical repellents. EMBO J. 24, 63–72. doi: 10.1038/sj.emboj.7600493
Horai, H., Arita, M., Kanaya, S., Nihei, Y., Ikeda, T., Suwa, K., et al. (2010). MassBank: a public repository for sharing mass spectral data for life sciences. J. Mass Spectrom. 45, 703–714. doi: 10.1002/jms.1777
Horvath, S., Garagnani, P., Bacalini, M. G., Pirazzini, C., Salvioli, S., Gentilini, D., et al. (2015). Accelerated epigenetic aging in Down syndrome. Aging Cell 14, 491–495. doi: 10.1111/acel.12325
Hoshi, H., Kamata, Y., and Uemura, T. (2003). Effects of 17β-Estradiol, Bisphenol A and Tributyltin Chloride on Germ Cells of Caenorhabditis elegans. J. Vet. Med. Sci. 65, 881–885. doi: 10.1292/jvms.65.881
Hou, J., Yin, W., Li, P., Hu, C., Xu, T., Cheng, J., et al. (2020). Joint effect of polycyclic aromatic hydrocarbons and phthalates exposure on telomere length and lung function. J. Hazard. Mater. 386:121663. doi: 10.1016/j.jhazmat.2019.121663
Jaeger, C., Hoffmann, F., Schmitt, C. A., and Lisec, J. (2016). Automated Annotation and Evaluation of In-Source Mass Spectra in GC/Atmospheric Pressure Chemical Ionization-MS-Based Metabolomics. Anal. Chem. 88, 9386–9390. doi: 10.1021/acs.analchem.6b02743
Johansson, H. K. L., Jacobsen, P. R., Hass, U., Svingen, T., Vinggaard, A. M., Isling, L. K., et al. (2016). Perinatal exposure to mixtures of endocrine disrupting chemicals reduces female rat follicle reserves and accelerates reproductive aging. Reprod. Toxicol. 61, 186–194. doi: 10.1016/j.reprotox.2016.03.045
Johnson, T. E. (2008). Caenorhabditis elegans 2007: The premier model for the study of aging. Exp. Gerontol. 43, 1–4. doi: 10.1016/j.exger.2007.09.008
Juarez, P. D., Hood, D. B., Rogers, G. L., Baktash, S. H., Saxton, A. M., Matthews-Juarez, P., et al. (2017). A novel approach to analyzing lung cancer mortality disparities: Using the exposome and a graph-theoretical toolchain. Environ. Dis. 2, 33–44. doi: 10.4103/ed.ed_8_17
Kelly, R. S., Bayne, H., Spiro, A., Vokonas, P., Sparrow, D., Weiss, S. T., et al. (2020). Metabolomic signatures of lead exposure in the VA Normative Aging Study. Environ. Res. 190:110022. doi: 10.1016/j.envres.2020.110022
Kennedy, C., Bastiaens, M. T., Willemze, R., Bouwes Bavinck, J. N., Bajdik, C. D., and Westendorp, R. G. J. (2003). Effect of Smoking and Sun on the Aging Skin. J. Invest. Dermatol. 120, 548–554. doi: 10.1046/j.1523-1747.2003.12092.x
Kim, R., Rotnitzky, A., Sparrow, D., Weiss, S. T., Wager, C., Hu, H., et al. (1996). A longitudinal study of low-level lead exposure and impairment of renal function: the normative aging study. JAMA 275, 1177–1181. doi: 10.1001/jama.1996.03530390043032
Krutmann, J., Bouloc, A., Sore, G., Bernard, B. A., and Passeron, T. (2017). The skin aging exposome. J. Dermatol. Sci. 85, 152–161. doi: 10.1016/j.jdermsci.2016.09.015
Kulick, E. R., Elkind, M. S. V., Boehme, A. K., Joyce, N. R., Schupf, N., Kaufman, J. D., et al. (2020a). Long-term exposure to ambient air pollution, APOE-ε4 status, and cognitive decline in a cohort of older adults in northern Manhattan. Environ. Int. 136:105440. doi: 10.1016/j.envint.2019.105440
Kulick, E. R., Wellenius, G. A., Boehme, A. K., Joyce, N. R., Schupf, N., Kaufman, J. D., et al. (2020b). Long-term exposure to air pollution and trajectories of cognitive decline among older adults. Neurology 94, E1782–E1792. doi: 10.1212/WNL.0000000000009314
Laponogov, I., Sadawi, N., Galea, D., Mirnezami, R., and Veselkov, K. A. (2018). ChemDistiller: an engine for metabolite annotation in mass spectrometry. Bioinformatics 34, 2096–2102. doi: 10.1093/bioinformatics/bty080
Leng, S. X., and Margolick, J. B. (2020). Aging, sex, inflammation, frailty, and CMV and HIV infections. Cell. Immunol. 348:104024. doi: 10.1016/j.cellimm.2019.104024
Lenz, K. A., Pattison, C., and Ma, H. (2017). Triclosan (TCS) and triclocarban (TCC) induce systemic toxic effects in a model organism the nematode Caenorhabditis elegans. Environ. Pollut. 231, 462–470. doi: 10.1016/j.envpol.2017.08.036
Li, M., Vierkötter, A., Schikowski, T., Hüls, A., Ding, A., Matsui, M. S., et al. (2015). Epidemiological evidence that indoor air pollution from cooking with solid fuels accelerates skin aging in Chinese women. J. Dermatol. Sci. 79, 148–154. doi: 10.1016/j.jdermsci.2015.04.001
Linstrom, P. J., and Mallard, W. G. (2001). The NIST Chemistry WebBook: A Chemical Data Resource on the Internet †. J. Chem. Eng. Data 46, 1059–1063. doi: 10.1021/je000236i
Liu, Y., Bai, Y., Wu, X., Li, G., Wei, W., Fu, W., et al. (2020). Polycyclic aromatic hydrocarbons exposure and their joint effects with age, smoking, and TCL1A variants on mosaic loss of chromosome Y among coke-oven workers. Environ. Pollut. 258:113655. doi: 10.1016/j.envpol.2019.113655
López-Otín, C., Blasco, M. A., Partridge, L., Serrano, M., and Kroemer, G. (2013). The Hallmarks of Aging. Cell 153, 1194–1217. doi: 10.1016/j.cell.2013.05.039
Martens, D. S., and Nawrot, T. S. (2016). Air Pollution Stress and the Aging Phenotype: The Telomere Connection. Curr. Environ. Heal. Rep. 3, 258–269. doi: 10.1007/s40572-016-0098-8
Martens, D. S., and Nawrot, T. S. (2018). Ageing at the level of telomeres in association to residential landscape and air pollution at home and work: a review of the current evidence. Toxicol. Lett. 298, 42–52. doi: 10.1016/j.toxlet.2018.06.1213
Martens, D. S., Cox, B., Janssen, B. G., Clemente, D. B. P., Gasparrini, A., Vanpoucke, C., et al. (2017). Prenatal Air Pollution and Newborns’ Predisposition to Accelerated Biological Aging. JAMA Pediatr. 171:1160. doi: 10.1001/jamapediatrics.2017.3024
McDaniel, D., Farris, P., and Valacchi, G. (2018). Atmospheric skin aging-Contributors and inhibitors. J. Cosmet. Dermatol. 17, 124–137. doi: 10.1111/jocd.12518
Misra, B. B. (2016). Bring Out Your Data: A call for community standards and the archiving of exposomics datasets or MIAEE (Minimum Information About an Exposomics Experiment). Anal. Sci. 1:1.
Misra, B. B. (2019). Metabolomics Tools to Study Links Between Pollution and Human Health: an Exposomics Perspective. Curr. Pollut. Rep. 5, 93–111.
Misra, B. B., and Misra, A. (2020). The chemical exposome of type 2 diabetes mellitus: Opportunities and challenges in the omics era. Diab. Metab. Syndr. Clin. Res. Rev. 14, 23–38.
Neveu, V., Nicolas, G., Salek, R. M., Wishart, D. S., and Scalbert, A. (2019). Exposome-Explorer 2.0: an update incorporating candidate dietary biomarkers and dietary associations with cancer risk. Nucleic Acids Res. 48, D908–D912. doi: 10.1093/nar/gkz1009
Newland, M. (2004). Gestational exposure to methylmercury retards choice in transition in aging rats. Neurotoxicol. Teratol. 26, 179–194. doi: 10.1016/j.ntt.2003.12.004
Newland, M. C., and Rasmussen, E. B. (2000). Aging unmasks adverse effects of gestational exposure to methylmercury in rats. Neurotoxicol. Teratol. 22, 819–828. doi: 10.1016/S0892-0362(00)00107-0
Nilson, L. N. (2002). Influence of solvent exposure and aging on cognitive functioning: an 18 year follow up of formerly exposed floor layers and their controls. Occup. Environ. Med. 59, 49–57. doi: 10.1136/oem.59.1.49
Nwanaji-Enwerem, J. C., Dai, L., Colicino, E., Oulhote, Y., Di, Q., Kloog, I., et al. (2017). Associations between long-term exposure to PM2.5 component species and blood DNA methylation age in the elderly: The VA normative aging study. Environ. Int. 102, 57–65. doi: 10.1016/j.envint.2016.12.024
Osorio-Yáñez, C., Clemente, D. B. P., Maitre, L., Vives-Usano, M., Bustamante, M., Martinez, D., et al. (2020). Early life tobacco exposure and children’s telomere length: The HELIX project. Sci. Tot. Environ. 711:135028. doi: 10.1016/j.scitotenv.2019.135028
Pandelides, Z., Thornton, C., Faruque, A. S., Whitehead, A. P., Willett, K. L., and Ashpole, N. M. (2020). Developmental exposure to cannabidiol (CBD) alters longevity and health span of zebrafish (Danio rerio). GeroScience 42, 785–800. doi: 10.1007/s11357-020-00182-4
Payton, M., Hu, H., Sparrow, D., and Weiss, S. T. (1994). Low-level Lead Exposure and Renal Function in the Normative Aging Study. Am. J. Epidemiol. 140, 821–829. doi: 10.1093/oxfordjournals.aje.a117330
Peeples, L. (2020). News Feature: How air pollution threatens brain health. Proc. Natl. Acad. Sci. 117:202008940. doi: 10.1073/pnas.2008940117
Pence, H. E., and Williams, A. (2010). ChemSpider: An Online Chemical Information Resource. J. Chem. Educ. 87, 1123–1124. doi: 10.1021/ed100697w
Pieters, N., Janssen, B. G., Dewitte, H., Cox, B., Cuypers, A., Lefebvre, W., et al. (2016). Biomolecular Markers within the Core Axis of Aging and Particulate Air Pollution Exposure in the Elderly: A Cross-Sectional Study. Environ. Health Perspect. 124, 943–950. doi: 10.1289/ehp.1509728
Prins, G., Birch, L., Tang, W., and Ho, S. (2007). Developmental estrogen exposures predispose to prostate carcinogenesis with aging? Reprod. Toxicol. 23, 374–382. doi: 10.1016/j.reprotox.2006.10.001
Qiu, Y., Luo, L., Yang, Y., Kong, Y., Li, Y., and Wang, D. (2020). Potential toxicity of nanopolystyrene on lifespan and aging process of nematode Caenorhabditis elegans. Sci. Tot. Environ. 705:135918. doi: 10.1016/j.scitotenv.2019.135918
Rice, D. C., Thompson, W. D., Reeve, E. A., Onos, K. D., Assadollahzadeh, M., and Markowski, V. P. (2009). Behavioral Changes in Aging but Not Young Mice after Neonatal Exposure to the Polybrominated Flame Retardant DecaBDE. Environ. Health Perspect. 117, 1903–1911. doi: 10.1289/ehp.11814
Rodriguez, P., Fernández-Galaz, C., and Tejero, A. (1993). Controlled Neonatal Exposure to Estrogens: A Suitable Tool for Reproductive Aging Studies in the Female Rat1. Biol. Reprod. 49, 387–392. doi: 10.1095/biolreprod49.2.387
Roh, J. Y., and Choi, J. (2008). Ecotoxicological evaluation of chlorpyrifos exposure on the nematode Caenorhabditis elegans. Ecotoxicol. Environ. Saf. 71, 483–489. doi: 10.1016/j.ecoenv.2007.11.007
Roig, J., Fuentes, S., Teresacolomina, M., Vicens, P., and Domingo, J. (2006). Aluminum, restraint stress and aging: Behavioral effects in rats after 1 and 2 years of aluminum exposure. Toxicology 218, 112–124. doi: 10.1016/j.tox.2005.10.006
Rothwell, J. A., Perez-Jimenez, J., Neveu, V., Medina-Remon, A., M’Hiri, N., Garcia-Lobato, P., et al. (2013). Phenol-Explorer 3.0: a major update of the Phenol-Explorer database to incorporate data on the effects of food processing on polyphenol content. Database 2013, bat070–bat070. doi: 10.1093/database/bat070
Ruttkies, C., Schymanski, E. L., Wolf, S., Hollender, J., and Neumann, S. (2016). MetFrag relaunched: incorporating strategies beyond in silico fragmentation. J. Cheminform. 8:3. doi: 10.1186/s13321-016-0115-9
Sawada, Y., Nakabayashi, R., Yamada, Y., Suzuki, M., Sato, M., Sakata, A., et al. (2012). RIKEN tandem mass spectral database (ReSpect) for phytochemicals: A plant-specific MS/MS-based data resource and database. Phytochemistry 82, 38–45. doi: 10.1016/j.phytochem.2012.07.007
Schikowski, T., and Hüls, A. (2020). Air Pollution and Skin Aging. Curr. Environ. Heal. Rep. 7, 58–64. doi: 10.1007/s40572-020-00262-9
Schmeisser, S., Schmeisser, K., Weimer, S., Groth, M., Priebe, S., Fazius, E., et al. (2013). Mitochondrial hormesis links low-dose arsenite exposure to lifespan extension. Aging Cell 12, 508–517. doi: 10.1111/acel.12076
Schroeder, P., Schieke, S. M., and Morita, A. (2006). “Premature skin aging by infrared radiation, tobacco smoke and ozone,” in Skin Aging, eds Edn, eds B. A. Gilchrest and J. Krutmann (Berlin: Springer), 45–53.
Schulte, P. J., Roberts, R. O., Knopman, D. S., Petersen, R. C., Hanson, A. C., Schroeder, D. R., et al. (2018). Association between exposure to anaesthesia and surgery and long-term cognitive trajectories in older adults: report from the Mayo Clinic Study of Aging. Br. J. Anaesth. 121, 398–405. doi: 10.1016/j.bja.2018.05.060
Shadick, N. A., Kim, R., Weiss, S., Liang, M. H., Sparrow, D., and Hu, H. (2000). Effect of low level lead exposure on hyperuricemia and gout among middle aged and elderly men: the normative aging study. J. Rheumatol. 27, 1708–1712.
Sharma, R., and Ramanathan, A. (2020). The Aging Metabolome—Biomarkers to Hub Metabolites. Proteomics 20:1800407. doi: 10.1002/pmic.201800407
Shin, N., Cuenca, L., Karthikraj, R., Kannan, K., and Colaiácovo, M. P. (2019). Assessing effects of germline exposure to environmental toxicants by high-throughput screening in C. elegans. PLoS Genet. 15:e1007975. doi: 10.1371/journal.pgen.1007975
Singh, T., and Yadav, S. (2020). Role of microRNAs in neurodegeneration induced by environmental neurotoxicants and aging. Ageing Res. Rev. 60:101068. doi: 10.1016/j.arr.2020.101068
Siroux, V., Agier, L., and Slama, R. (2016). The exposome concept: a challenge and a potential driver for environmental health research. Eur. Respir. Rev. 25, 124–129. doi: 10.1183/16000617.0034-2016
Smith, M. T., de la Rosa, R., and Daniels, S. I. (2015). Using exposomics to assess cumulative risks and promote health. Environ. Mol. Mutagen 56, 715–723. doi: 10.1002/em.21985
Sobrian, S. K., Marr, L., and Ressman, K. (2003). Prenatal cocaine and/or nicotine exposure produces depression and anxiety in aging rats. Prog. Neuro Psychopharmacol. Biol. Psych. 27, 501–518. doi: 10.1016/S0278-5846(03)00042-3
Sorrentino, J. A., Sanoff, H. K., and Sharpless, N. E. (2014). Defining the toxicology of aging. Trends Mol. Med. 20, 375–384. doi: 10.1016/j.molmed.2014.04.004
Soundararajan, A., Prabu, P., Mohan, V., Gibert, Y., and Balasubramanyam, M. (2019). Novel insights of elevated systemic levels of bisphenol-A (BPA) linked to poor glycemic control, accelerated cellular senescence and insulin resistance in patients with type 2 diabetes. Mol. Cell. Biochem. 458, 171–183. doi: 10.1007/s11010-019-03540-9
Steinbeck, C., and Kuhn, S. (2004). NMRShiftDB – compound identification and structure elucidation support through a free community-built web database. Phytochemistry 65, 2711–2717. doi: 10.1016/j.phytochem.2004.08.027
Swatloski, R. P., Holbrey, J. D., Memon, S. B., Caldwell, G. A., Caldwell, K. A., and Rogers, R. D. (2004). Using Caenorhabditis elegans to probe toxicity of 1-alkyl-3-methylimidazolium chloride based ionic liquids. Chem. Commun. 4, 668–669. doi: 10.1039/b316491h
Tan, L., Wang, S., Wang, Y., He, M., and Liu, D. (2015). Bisphenol A exposure accelerated the aging process in the nematode Caenorhabditis elegans. Toxicol. Lett. 235, 75–83. doi: 10.1016/j.toxlet.2015.03.010
Tissenbaum, H. A. (2015). Using C. elegans for aging research. Invertebr. Reprod. Dev. 59, 59–63. doi: 10.1080/07924259.2014.940470
Treviño, L. S., Dong, J., Kaushal, A., Katz, T. A., Jangid, R. K., Robertson, M. J., et al. (2020). Epigenome environment interactions accelerate epigenomic aging and unlock metabolically restricted epigenetic reprogramming in adulthood. Nat. Commun. 11:2316. doi: 10.1038/s41467-020-15847-z
Tsugawa, H., Kind, T., Nakabayashi, R., Yukihira, D., Tanaka, W., Cajka, T., et al. (2016). Hydrogen Rearrangement Rules: Computational MS/MS Fragmentation and Structure Elucidation Using MS-FINDER Software. Anal. Chem. 88, 7946–7958. doi: 10.1021/acs.analchem.6b00770
Van Dyke, N., Yenugadhati, N., Birkett, N. J., Lindsay, J., Turner, M. C., Willhite, C. C., et al. (2020). Association between aluminum in drinking water and incident Alzheimer’s disease in the Canadian Study of Health and Aging cohort. Netherland: Elsevier.
Vermeulen, R., Schymanski, E. L., Barabási, A.-L., and Miller, G. W. (2020). The exposome and health: Where chemistry meets biology. Science 367, 392–396. doi: 10.1126/science.aay3164
Vierkötter, A., Schikowski, T., Ranft, U., Sugiri, D., Matsui, M., Krämer, U., et al. (2010). Airborne particle exposure and extrinsic skin aging. J. Invest. Dermatol. 130, 2719–2726. doi: 10.1038/jid.2010.204
Vineis, P., Robinson, O., Chadeau-Hyam, M., Dehghan, A., Mudway, I., and Dagnino, S. (2020). What is new in the exposome? Environ. Int. 143:105887. doi: 10.1016/j.envint.2020.105887
Wandy, J., Zhu, Y., van der Hooft, J. J. J., Daly, R., Barrett, M. P., and Rogers, S. (2018). Ms2lda.org: web-based topic modelling for substructure discovery in mass spectrometry. Bioinformatics 34, 317–318. doi: 10.1093/bioinformatics/btx582
Wang, C., Cai, Z., Wang, W., Wei, M., Si, X., Shang, Y., et al. (2020a). Piperine regulates glycogen synthase kinase-3β-related signaling and attenuates cognitive decline in D-galactose-induced aging mouse model. J. Nutr. Biochem. 75:108261. doi: 10.1016/j.jnutbio.2019.108261
Wang, C., Koutrakis, P., Gao, X., Baccarelli, A., and Schwartz, J. (2020b). Associations of annual ambient PM2.5 components with DNAm PhenoAge acceleration in elderly men: The Normative Aging Study. Environ. Pollut. 258:113690. doi: 10.1016/j.envpol.2019.113690
Wang, S., Zhao, Y., Wu, L., Tang, M., Su, C., Hei, T. K., et al. (2007). Induction of germline cell cycle arrest and apoptosis by sodium arsenite in Caenorhabditis elegans. Chem. Res. Toxicol. 20, 181–186. doi: 10.1021/tx0601962
Ward-Caviness, C. K., Nwanaji-Enwerem, J. C., Wolf, K., Wahl, S., Colicino, E., Trevisi, L., et al. (2016). Long-term exposure to air pollution is associated with biological aging. Oncotarget 7, 74510–74525. doi: 10.18632/oncotarget.12903
Warth, B., Spangler, S., Fang, M., Johnson, C. H., Forsberg, E. M., Granados, A., et al. (2017). Exposome-scale investigations guided by global metabolomics, pathway analysis, and cognitive computing. Anal. Chem. 89, 11505–11513. doi: 10.1021/acs.analchem.7b02759
Wild, C. P. (2005). Complementing the Genome with an “Exposome”: The Outstanding Challenge of Environmental Exposure Measurement in Molecular Epidemiology. Cancer Epidemiol. Biomarkers Prev. 14, 1847–1850. doi: 10.1158/1055-9965.EPI-05-0456
Wishart, D. S. (2009). Computational strategies for metabolite identification in metabolomics. Bioanalysis 1, 1579–1596. doi: 10.4155/bio.09.138
Wishart, D., Arndt, D., Pon, A., Sajed, T., Guo, A. C., Djoumbou, Y., et al. (2015). T3DB: the toxic exposome database. Nucleic Acids Res. 43, D928–D934. doi: 10.1093/nar/gku1004
Yin, L., Morita, A., and Tsuji, T. (2001). Skin aging induced by ultraviolet exposure and tobacco smoking: evidence from epidemiological and molecular studies. Photodermatol. Photoimmunol. Photomed. 17, 178–183. doi: 10.1034/j.1600-0781.2001.170407.x
Yu, F., Wang, B.-H., Feng, S.-P., Wang, J.-Y., Li, W.-G., and Wu, Y.-T. (2011). Development, characterization, and cross-species/genera transferability of SSR markers for rubber tree (Hevea brasiliensis). Plant Cell Rep. 30, 335–344.
Yu, C. W., How, C. M., and Liao, V. H. C. (2016). Arsenite exposure accelerates aging process regulated by the transcription factor DAF-16/FOXO in Caenorhabditis elegans. Chemosphere 150, 632–638. doi: 10.1016/j.chemosphere.2016.01.004
Keywords: exposome, metabolite, aging, mass spectrometry, informatics, pollution, model, telomere
Citation: Misra BB (2020) The Chemical Exposome of Human Aging. Front. Genet. 11:574936. doi: 10.3389/fgene.2020.574936
Received: 22 June 2020; Accepted: 08 October 2020;
Published: 23 November 2020.
Edited by:
Serena Dato, University of Calabria, ItalyReviewed by:
Michael Petrascheck, The Scripps Research Institute, United StatesSrinivas Ayyadevara, Central Arkansas Veterans Healthcare System Eugene J. Towbin Healthcare Center, United States
Copyright © 2020 Misra. This is an open-access article distributed under the terms of the Creative Commons Attribution License (CC BY). The use, distribution or reproduction in other forums is permitted, provided the original author(s) and the copyright owner(s) are credited and that the original publication in this journal is cited, in accordance with accepted academic practice. No use, distribution or reproduction is permitted which does not comply with these terms.
*Correspondence: Biswapriya B. Misra, YmJtaXNyYWNjYkBnbWFpbC5jb20=; orcid.org/0000-0003-2589-6539