- Department of Medicine, University of Maryland School of Medicine, Baltimore, MD, United States
Objectives: To identify previously unrecognized genetic variants and clinical variables associated with the ICD-10 (International Classification of Diseases 10)-based diagnosis of hypertrophic cardiomyopathy in the UK Biobank cohort.
Background: Hypertrophic cardiomyopathy (HCM) is the most common genetic cardiovascular disorder with more than 2000 known mutations in one of eight genes encoding sarcomeric proteins. However, there is considerable variation in disease manifestation, suggesting the role of additional unrecognized contributors, genetic and otherwise. There is substantial interest in the use of real-world data, such as electronic health records to better understand disease mechanisms and discover new treatment strategies, but whether ICD-10-based diagnosis can be used to study HCM genetics is unknown.
Methods: In a genome-wide association study (GWAS) using the UK Biobank, we analyzed the genomes of 363 individuals diagnosed with HCM based on ICD-10 coding compared to 7,260 age, ancestry, and sex-matched controls in a 1:20 case:control design. Genetic variants were analyzed by Plink’s firth logistic regression and assessed for association with HCM. We also examined 61 biomarkers and other diagnoses in the 363 HCM cases and matched controls.
Results: The prevalence of ICD-10-based diagnosis of HCM in the UK Biobank cohort was 1 in 1,342, suggesting disease assignment based on the two ICD-10 codes underestimates HCM prevalence. In addition, common cardiovascular comorbidities were more prevalent in ICD-10-based HCM cases in comparison to controls. We identified two novel, non-sarcomeric genetic variants in KMT2C rs78630626, and PARD3B rs188937806 that were associated with ICD-10 codes for HCM with genome-wide significance (p < 5 x 10−8). These are associated with an increased odds ratio (OR) of ∼3.8 for being diagnosed with HCM. Minor allele frequency (MAF) of each variant was >1%.
Discussion: Disease assignment based strictly on ICD-10 codes may underestimate HCM prevalence. Individuals with HCM were more frequently diagnosed with several comorbid conditions, such as hypertension, atherosclerotic heart disease, diabetes, and kidney failure, suggesting they may contribute to disease manifestation. This UK Biobank database-based GWAS identified common variants in KMT2C and PARD3B that are associated with HCM diagnosis, which may represent novel modifier genes. Our study demonstrates the feasibility and limitations of conducting phenotypic and genotypic characterization of HCM based on ICD-10 diagnosis in a large population-based cohort.
Introduction
Hypertrophic cardiomyopathy (HCM) is a genetic disorder of heart muscle characterized by thickened left ventricular wall and intrinsic cardiac hypertrophy and sarcomere disarray. HCM prevalence is estimated at approximately 1:625-1:344 individuals in the general adult population (Hada et al., 1987; Maron et al., 1995; Maron et al., 1999; Zou et al., 2004). HCM is known to be caused most often by rare pathogenic variants in one of eight genes for sarcomere proteins. However, clinical disease expression varies considerably, even among individuals with identical pathogenic variants. Factors that affect phenotypic expression are largely unknown, although it has been hypothesized that disease modifier genes may play a role in the development of HCM.
There is considerable interest in the use of real-world data, such as electronic health records to better understand disease mechanisms and to discover new treatment strategies (Hemingway et al., 2018). The UK Biobank is a large, ongoing prospective cohort study that recruited 502,682 UK participants between 2006-2010. UK Biobank has compiled extensive health-related records and genetic data from the participants (Bycroft et al., 2018; Ashvetiya et al., 2021; Scalsky et al., 2021). Here, using ICD-10 diagnostic codes as a “real world” surrogate for the definitive diagnosis for HCM, we carried out a GWAS using this database.
Our study identified common variants in KMT2C and PARD3B that are associated with HCM. These may represent novel modifier genes for the development of HCM. Compared to the control population, individuals with HCM were more frequently diagnosed with several comorbid conditions, such as hypertension, atherosclerotic heart disease, diabetes, and kidney failure, suggesting they may contribute to hypertrophic disease manifestation in genetically susceptible individuals. Our study demonstrates the feasibility and limitations of conducting phenotypic and genotypic characterization of hypertrophic cardiomyopathy based on ICD-10 diagnosis in a large population-based cohort.
Methods
Ethical Approval
The present study, which involved de-identified data obtained from the UK Biobank Resource under Application Number 49852, received the proper ethical oversight, including the determination by the University of Maryland, Baltimore Institutional Review Board that the study is not human research (IRB #: HF-00088022).
Study Population
We carried out a GWAS using the UK Biobank to interrogate the genome for statistically significant associations between single nucleotide polymorphisms (SNPs) and clinical manifestations of HCM. The UK Biobank is an ongoing prospective cohort that recruited over 502,682 UK participants between 2006 and 2010. Participants ranged in age from 37–73 years at the time of recruitment. Health-related records were collected from these participants, including clinical data such as biometrics, biomarkers, and diagnostic codes. The Biobank also contains genetic data, with over 820,000 genotyped SNPs and up to 90 million imputed variants available for most individuals.
Genome-Wide Association Study
Using data from the UK Biobank Resource on 487,310 subjects with imputed genotypes, we performed quality control by removing those with genetic relatedness exclusions (Data-Field 22018–UKB, https://biobank.ctsu.ox.ac.uk/crystal/field.cgi?id=22018; 1532 subjects), sex chromosome aneuploidy (Data-Field 22019—UKB, https://biobank.ctsu.ox.ac.uk/crystal/field.cgi?id=22019; 651 subjects), mismatch between self-reported sex and genetically determined sex (Data-Field 31—UKB, https://biobank.ctsu.ox.ac.uk/crystal/field.cgi?id=31; Data-Field 22001—UKB, https://biobank.ctsu.ox.ac.uk/crystal/field.cgi?id=22001; 372 subjects), recommended genomic analysis exclusions (Data-Field 22010—UKB, https://biobank.ctsu.ox.ac.uk/crystal/field.cgi?id=22010; 480 subjects), and outliers for heterozygosity or missing rate (Data-Field 22017—UKB, https://biobank.ctsu.ox.ac.uk/crystal/field.cgi?id=22077; 968 subjects).
Cases were defined as having using International Statistical Classification of Diseases and Related Health Problems, 10th edition (ICD-10) diagnostic codes for obstructive hypertrophic cardiomyopathy (I42.1) or other hypertrophic cardiomyopathy (I42.2) as their primary or secondary diagnosis at the time of this analysis (July 2020). The selected set of cases was purged of relatedness by removing one from each related pair in an iterative fashion until no related subjects remained. Relatedness was defined as a kinship coefficient greater than 0.044, which treats third-degree relationships (with a kinship coefficient of 0.0625) as related. Kinship coefficients for all subject pairs greater than 0.044 were provided by the UK Biobank as part of the standard data set. A pool of subjects for the control population was initially generated by removing the cases from the full set. Related subjects in the control population were then removed. For each case, twenty individuals from the control population were selected for comparison matching for age, sex, and ancestry. A total of 7,260 individuals were identified for inclusion as controls in the analysis. The twenty matching control subjects were selected for each case subject using incremental tolerance expansion for age and ancestry. The tolerance for age ranged from 0 (exact match) up to 7 years. Ancestry matching was performed with principal components (PCs) supplied by the UK Biobank. The mathematical distance in a graph plotting the PC1 x PC2 was used to test similarity in ancestry. The “distance” in ancestry tolerance ranged from 2 PC units to a maximum of 80 PC units with PC1 (Supplementary Figure S1), ranging from 0 to +400 and PC2 ranging from −300 to +100 units. Using these tolerances, 20 matching controls were found for every case.
The GWAS was performed with Plink’s firth logistic regression model adjusting for age, sex, and 5 PCs using data supplied by the UK Biobank Resource (Bycroft et al., 2018). We chose firth regression because it has been shown to provide the best combination of control for type I error and power for detection of low-frequency variants (Ma et al., 2013; Wang, 2014; Chang et al., 2015). The HCM cases were analyzed with the 40 million imputed genetic variants provided by the UK Biobank with imputation quality scores greater than 0.70. The analysis included covariates of sex, age, and principal components 1 through 5 to adjust for ancestry. Pre-calculated PC data for the first 40 principal components were supplied by the UK Biobank. Our preliminary analysis showed that only the first 5 PCs had significance with p-values less than 0.05. Thus, we used only the first 5 PCs in our GWAS.
UK Biobank contains a wealth of baseline clinical information of participants, including comorbidities, height, weight, body mass index, and basic laboratory values (Bycroft et al., 2018). We examined ICD-10 codes (Table 1), ABO blood type, 5 biometric markers, and 61 biomarkers in 363 HCM cases and 7260 controls (Table 2; Supplementary Table S1). The significance of the association of these conditions and variables with HCM patients in comparison to age, sex, ancestry-matched controls were determined by two-tailed Fisher’s exact test.
Identification of Hypertrophic Cardiomyopathy Genetic Variants
Variants of interest met thresholds of a minor allele frequency (MAF) of 0.5% or greater and a p-value < 5 x 10−8. Identified variants were also assessed for phenotypic associations via pheweb.org, a GWAS dataset for electronic health record-derived disease associations from the white British participants in the UK Biobank. PheWeb utilizes a generalized mixed model association test that uses the saddle point approximation to account for case-control imbalance and imputed using the Haplotype Reference Consortium Panel (Michigan Genomics Initiative, 2017).
Results
A total of 363 individuals (119 females and 244 males) were identified as HCM cases based on ICD-10 diagnostic codes for “obstructive hypertrophic cardiomyopathy” (I42.1) or “other hypertrophic cardiomyopathy” (I42.2) as a primary or secondary diagnosis at the time of this analysis (as of July 2020). This represents a prevalence rate of 1 in 1,342, suggesting disease assignment based on the two ICD-10 codes, while specific, underestimates the HCM prevalence. The majority of cases were self-described as having British ancestry (80.7%). Additionally, 3.3% reported Irish ancestry, 3% reported African ancestry, and 1.9% reported Caribbean ancestry. Baseline characteristics are displayed in Table 3.
Common co-diagnosis of HCM cases and controls are shown in Table 1. The 363 cases who were diagnosed with HCM were more likely to be also diagnosed with ventricular tachycardia (ICD-10 Code I47.2) compared to age, sex, ancestry-matched controls (11.6% versus 0.25%, p-value < 0.0001 by two-tailed Fisher’s exact test). Cases were also more likely to be diagnosed with syncope and collapse (R55; 16% vs. 3%, p < 0.0001), dyspnea (R06.0; 13% vs. 2%, p < 0.0001), chest pain (R07.4; 28% vs. 6%, p < 0.0001), and atrial fibrillation and flutter (I48; 40% vs. 6%; p < 0.0001), consistent with the notion that HCM is associated with increased risks of developing these complications. Not surprising for an inherited condition, 28% reported family history of heart disease (Z82.4) compared to 4% for controls (p < 0.0001).
Interestingly, the HCM cases were also more likely to be also diagnosed with chronic ischemic heart disease (I125.9; 19% vs. 5%, p < 0.0001), asthma (J45.9; 14% vs. 6%, p < 0.0001), non-insulin-dependent diabetes mellitus (E11.9; 13% vs. 7%, p = 0.0005), atherosclerotic heart disease (I25.1; 23% vs. 7%, p < 0.0001), pure hypercholesterolemia (E78.0; 34% vs. 12%, p < 0.0001), and essential hypertension (I10; 61% vs. 27%, p < 0.0001).
Overall, individuals with HCM were more likely to be also diagnosed with acute renal failure compared to controls (11% vs. 2%, p-value < 0.0001) (Table 1). Consistent with this, mean values for blood urea (35.8 vs. 33.5 mg/dl, p-value 1.4 x 10−4), creatinine (0.825 vs. 0.764 mg/dl, p-value 6.8 x 10−4), uric acid (6.0 versus 5.54, p-value 2.2 x 10−8), and cystatin C (1.01 vs. 0.932 mg/L, p-value 8.1 x 10−9) were higher in HCM cases than controls, suggesting subtle but consistent diminished renal function in HCM cases (Table 2). There was also a trend toward higher levels of urine microalbumin (112 vs. 36.5 mg/L). Although HCM cases were more likely to be diagnosed with type 2 diabetes mellitus (Table 1), there was no difference in mean serum glucose or hemoglobin A1c levels (Table 2). Lastly, while HCM cases were more likely to be diagnosed with hypercholesterolemia (Table 1), mean total cholesterol and low-density lipoprotein (LDL) levels were statistically significantly lower in HCM cases compared to controls (Table 2).
GWAS of variants with MAF of ≥0.5% revealed two variants that were associated with the HCM diagnosis with a p-value < 5 x 10−8 (Figures 1A,B). First, rs78630626 (MAF 1.08%), an intron in the KMT2C gene, encoding lysine methyltransferase 2C, was associated with HCM with the odds ratios of 3.79 and a p-value of 2.41 x 10−10 (Figures 1A,C). Second, rs188937806 (MAF 1.63%), in an intron in the PARD3B gene, encoding Par-3 family cell polarity regulator beta, was associated with HCM with the odds ratio of 3.79 and a p-value of 1.33 x 10−8 (Figures 1A,D). Of note, no common variants in known sarcomere genes were found to be associated with HCM in this population. Quantile-quantile plots (QQ Plots) are provided in Supplementary Figure S2 to illustrate that the GWAS quality was well controlled.
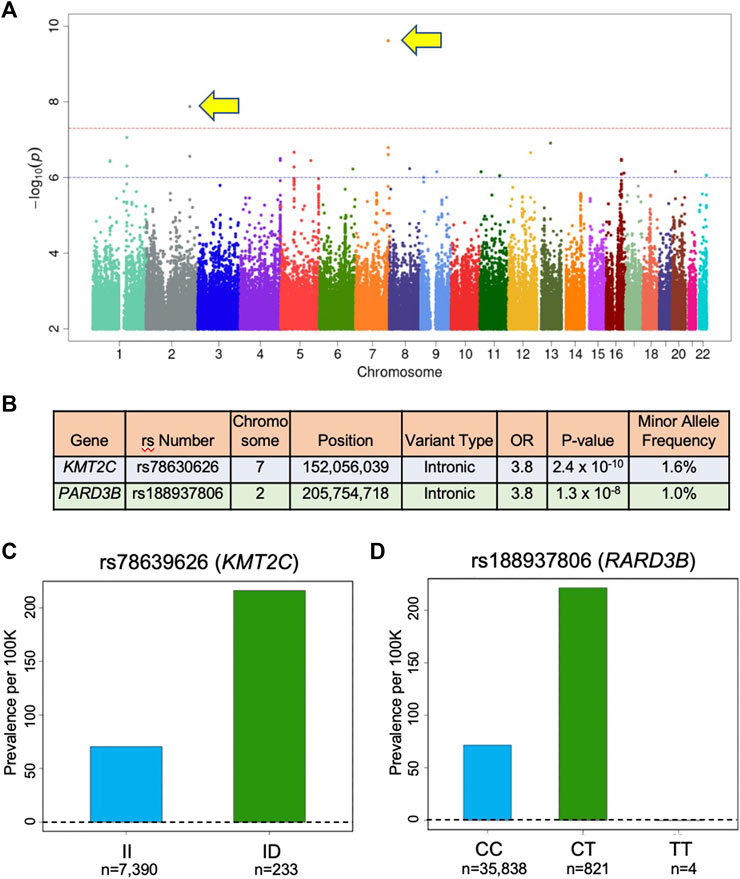
FIGURE 1. Variants associated with HCM in UK Biobank. (A) Manhattan plot of GWAS results (MAF > 0.5) for HCM identifies 2 SNPs associated with HCM diadnosis that are above the red line (p = 5 x 10−8) indicating genome-wide significance. In this plot, significance is displayed on the y-axis as −log10 of the p-value, with results ordered along the x-axis by chromosomes (each colored bar represents a different chromosome). (B) Association results for the SNPs identified in (A). OR is odds ratio and indicates that subjects in the UK Biobank carrying one copy of the minor allele are 3.8 times more likely to have the HCM diagnosis compared to non-carriers. (C) Prevalence of HCM in the UK Biobank cohort increases with KMT2C variant rs78630626 status (70 per 100,000 for wild-type; 216 per 100,000 for heterozygotes; p-value 1.4 x 10−10; OR per D allele = 3.82). (D) Prevalence of HCM in the UK Biobank cohort increases with PARD3B variant rs188937806 (71 per 100,00 for wild-type; 242 per 100,000 for heterozygotes; 0 per 100,000 for homozygotes; p-value 7.4 x 10−9; OR per T allele = 3.85). KMT2X: ltsine methyltransferase 2C; PARD3B: par-3 family cell polarity regulator beta.
Discussion
The approach used in this paper has several limitations. As with any GWAS study, the discovery of novel loci associated with hypertrophic cardiomyopathy does not prove functional causality, and the findings described herein need to be validated by analysis of other databases. Moreover, there are certain limitations inherent to a population study based on ICD-10 codes in comparison to a study dedicated specifically to hypertrophic cardiomyopathy. For example, ICD-10-based studies are limited by the fact that, as in many real-world situations, many diseases and medical conditions are underdiagnosed. Indeed, a total of 363 individuals were identified as HCM cases based on ICD-10 diagnostic codes for “obstructive hypertrophic cardiomyopathy” (I42.1) or “other hypertrophic cardiomyopathy” (I42.2) as a primary or secondary diagnosis at the time of this analysis, represents prevalence rate of 1 in 1,342, indicating disease assignment based on the two ICD-10 codes underestimates the HCM prevalence.
Sarcomeric HCM is an archetypal single gene disorder with autosomal dominant inheritance. However, recent findings indicate that the majority of those diagnosed with HCM do not carry a mutation in sarcomeric genes (sarcomere-negative HCM) (Neubauer et al., 2019). Our GWAS of the UK Biobank cohort identified two variants, one in KMT2C and another PARD3B that are associated with HCM. Our findings for PARD3B are consistent with data published in the PheWeb database (Michigan Genomics Initiative, 2017). The variants we report are common (MAF > 1%), suggesting that they may be genetic contributors to the HCM phenotype, which is increasingly recognized as polygenic and multifactorial (Watkins, 2021). Of note, our GWAS did not reveal association with common variants in any of the sarcomere genes. Presently, we don’t know whether any of the carriers of KMT2C and PARD3B also harbor rare sarcomere mutations. We attempted to examine the rare sarcomere HCM mutations in the UK Biobank, but at the time of this study, only 40% of the subjects were sequenced. Consequently, there is not enough overlap between carriers of rare pathogenic variants in the sarcomere genes and the reported SNPs in KTM2C and PARD3B to make a meaningful conclusion. Alternatively, we acknowledge hat association of KMT2C and PARD3B variants with the ICD-10 codes for HCM diagnosis may not be due to direct association with HCM per se but rather a phenotype resembling HCM (Marian and Braunwald, 2017).
UK Biobank contains a wealth of baseline clinical information of participants, including comorbidities, height, weight, body mass index, and basic laboratory values (Supplementary Table S1) (Bycroft et al., 2018). Several inferences can be made from this information. For example, HCM diagnosis in this cohort is associated with the traditional cardiac risk factors: atherosclerotic heart disease, hypertension, noninsulin-dependent diabetes mellitus, and hypercholesterolemia as well as a host of novel associations. While additional studies are needed to confirm these associations, our findings suggest a possible role of these factors in disease expression of HCM in susceptible individuals. Taken together, our study supports the emerging picture that sarcomere-negative HCM is polygenic and multifactorial (Watkins, 2021). Additionally, we note that despite higher rates of diagnoses of hypertension, noninsulin-dependent diabetes mellitus, and hypercholesterolemia in HCM cases, mean blood pressure, mean hemoglobin A1C and lipid levels are not higher in HCM cases in comparison to age, sex-matched controls (Table 2). This suggests there may be explanations other than biological, such as ascertainment bias in those already diagnosed with HCM. Finally, we found additional biometrics and biomarkers associated with HCM in the UK Biobank (Supplementary Table S1). While we do not yet have a full understanding of these findings, we have included them for future analysis.
Another interesting finding is that in our cohort, HCM cases are associated with a surprisingly high prevalence of atrial fibrillation and flutter (40%), compared to 20% in a case series of 1,558 HCM patients over a 10-year period (Rowin et al., 2017). The reason for this discrepancy is unclear, but we hypothesize that the HCM cases in a cross-sectional population-based cohort like UK Biobank may be different from HCM cases in disease-centric registries.
As with any GWAS study, the discovery of novel loci associated with HCM does not prove a functional association. Nonetheless, our GWAS findings have biological plausibility. For example, KMT2C encodes a lysine N-methyltransferase 2C, which is critical for histone H3 lysine 4 (H3K4) methylation necessary for stabilization of gene expression patterns in adult cardiomyocytes and is markedly elevated in hypertrophied cardiomyocytes in transverse aortic constriction (TAC) model and diabetic mice (Stein et al., 2011; Jiang et al., 2017). PARD3B encodes Partitioning defective 3 homolog B, also known as PAR3 family cell polarity regulator beta, which is an adapter protein that may play a role in the formation or maintenance of epithelial tight junctions in kidney glomerulus (Koehler et al., 2016) and is found in the urine of preeclampsia patients (Zhao et al., 2011). Interestingly, mean measures of renal dysfunction (serum urea, creatinine, cystatin C and uric acid; and microalbumin) were all elevated in HCM cases, although high urine microalbumin in HCM cases did not reach statistical significance (p-value = 0.065) due to wide range of values and relatively few patients who had this measured. Nonetheless, since albuminuria associated is well-known to be associated with left ventricular hypertrophy due to long-standing hypertension (Pedrinelli et al., 1993; Tsioufis et al., 2002; Nabbaale et al., 2015), it is tempting to speculate that subclinical albuminuria, due to intrinsic renal dysfunction, hypertension, or diabetes, contributes to HCM disease manifestation in susceptible individuals.
Conclusion
Here, using ICD-10 diagnostic codes as a “real world” surrogate for the definitive diagnosis for HCM, we carried out a GWAS using the UK Biobank database and identified two relatively common genetic variants that are associated with the diagnosis of HCM. Both have biological plausibility as modifiers of HCM disease with KMT2C previously being associated with hypertrophied cardiomyocytes in a diabetic mouse model and PARD3B potentially playing a role in epithelial tight junctions. Compared to the controls, HCM cases were also more frequently diagnosed with several comorbid conditions, such as hypertension, atherosclerotic heart disease, diabetes, and kidney failure, suggesting they may contribute to hypertrophic disease manifestation in genetically susceptible individuals. While the findings must be validated in future studies, our approach supports the feasibility of using real-world data, such as those contained in electronic health records, to gain new insights into the pathophysiology of relatively rare conditions (Hemingway et al., 2018).
Data Availability Statement
The datasets presented in this study can be found in online repositories. The names of the repository/repositories and accession number(s) can be found in the article/Supplementary Material.
Author Contributions
AG developed and suggested the hypothesis of this manuscript and wrote the majority of the draft. Y-JC provided data analysis. LW provided subject matter expertise in hypertrophic cardiomyopathy to identify strengths and weaknesses of the methods of this study. JO’C provided conceptualization and data analysis, as needed. YWC provided conceptualization support. CW provided data analysis. JP and CH developed the methods, provided subject matter expertise in GWAS and omics and provided guidance to authors on the manuscript.
Conflict of Interest
The authors declare that the research was conducted in the absence of any commercial or financial relationships that could be construed as a potential conflict of interest.
Publisher’s Note
All claims expressed in this article are solely those of the authors and do not necessarily represent those of their affiliated organizations, or those of the publisher, the editors and the reviewers. Any product that may be evaluated in this article, or claim that may be made by its manufacturer, is not guaranteed or endorsed by the publisher.
Supplementary Material
The Supplementary Material for this article can be found online at: https://www.frontiersin.org/articles/10.3389/fgene.2022.866042/full#supplementary-material
References
Ashvetiya, T., Fan, S. K., Chen, Y. J., Williams, C. H., O’Connell, J. R., Perry, J. A., et al. (2021). Identification of Novel Genetic Susceptibility Loci for Thoracic and Abdominal Aortic Aneurysms via Genome-Wide Association Study Using the UK Biobank Cohort. PLoS One 16 (9), e0247287. doi:10.1371/journal.pone.0247287
Bycroft, C., Freeman, C., Petkova, D., Band, G., Elliott, L. T., Sharp, K., et al. (2018). The UK Biobank Resource with Deep Phenotyping and Genomic Data. Nature 562 (7726), 203–209. doi:10.1038/s41586-018-0579-z
Chang, C. C., Chow, C. C., Tellier, L. C., Vattikuti, S., Purcell, S. M., and Lee, J. J. (2015). Second-Generation PLINK: Rising to the Challenge of Larger and Richer Datasets. GigaSci. 4 (1), 1–16. doi:10.1186/s13742-015-0047-8
Hada, Y., Sakamoto, T., Amano, K., Yamaguchi, T., Takenaka, K., Takahashi, H., et al. (1987). Prevalence of Hypertrophic Cardiomyopathy in a Population of Adult Japanese Workers as Detected by Echocardiographic Screening. Am. J. Cardiol. 59, 183–184. doi:10.1016/s0002-9149(87)80107-8
Hemingway, H., Asselbergs, F. W., Danesh, J., Dobson, R., Maniadakis, N., Maggioni, A., et al. (2018). Big Data from Electronic Health Records for Early and Late Translational Cardiovascular Research: Challenges and Potential. Eur. Heart J. 39 (16), 1481–1495. doi:10.1093/eurheartj/ehx487
Jiang, D.-S., Yi, X., Li, R., Su, Y.-S., Wang, J., Chen, M.-L., et al. (2017). The Histone Methyltransferase Mixed Lineage Leukemia (MLL) 3 May Play a Potential Role in Clinical Dilated Cardiomyopathy. Mol. Med. 23, 196–203. doi:10.2119/molmed.2017.00012
Koehler, S., Tellkamp, F., Niessen, C. M., Bloch, W., Kerjaschki, D., Schermer, B., et al. (2016). Par3A Is Dispensable for the Function of the Glomerular Filtration Barrier of the Kidney. Am. J. Physiol.-Renal Physiol. 311 (1), F112–F119. doi:10.1152/ajprenal.00171.2016
Ma, C., Blackwell, T., Boehnke, M., and Scott, L. J. (2013). Recommended Joint and Meta-Analysis Strategies for Case-Control Association Testing of Single Low-Count Variants. Genet. Epidemiol. 37 (6), 539–550. doi:10.1002/gepi.21742
Marian, A. J., and Braunwald, E. (2017). Hypertrophic Cardiomyopathy: Genetics, Pathogenesis, Clinical Manifestations, Diagnosis, and Therapy. Circ. Res. 121 (7), 749–770. doi:10.1161/circresaha.117.311059
Maron, B. J., Gardin, J. M., Flack, J. M., Gidding, S. S., Kurosaki, T. T., and Bild, D. E. (1995). Prevalence of Hypertrophic Cardiomyopathy in a General Population of Young Adults. Echocardiographic Analysis of 4111 Subjects in the CARDIA Study. Coronary Artery Risk Development in (Young) Adults. Circulation 92, 785–789. doi:10.1161/01.cir.92.4.785
Maron, B. J., Mathenge, R., Casey, S. A., Poliac, L. C., and Longe, T. F. (1999). Clinical Profile of Hypertrophic Cardiomyopathy Identified De Novo in Rural Communities. J. Am. Coll. Cardiol. 33, 1590–1595. doi:10.1016/s0735-1097(99)00039-x
Michigan Genomics Initiative (2017). UK Biobank ICD PheWeb. Retrieved from: https://pheweb.org/UKB-SAIGE/. (Accessed on September 20, 2017).
Nabbaale, J., Kibirige, D., Ssekasanvu, E., Sebatta, E. S., Kayima, J., Lwabi, P., et al. (2015). Microalbuminuria and Left Ventricular Hypertrophy Among Newly Diagnosed Black African Hypertensive Patients: A Cross Sectional Study from a Tertiary Hospital in Uganda. BMC Res. Notes 8, 198. doi:10.1186/s13104-015-1156-2
Neubauer, S., Kolm, P., Ho, C. Y., Kwong, R. Y., Desai, M. Y., Dolman, S. F., et al. (2019). Distinct Subgroups in Hypertrophic Cardiomyopathy in the NHLBI HCM Registry. J. Am. Coll. Cardiol. 74 (19), 2333–2345. doi:10.1016/j.jacc.2019.08.1057
Pedrinelli, R., Di Bello, V., Catapano, G., Talarico, L., Materazzi, F., Santoro, G., et al. (1993). Microalbuminuria Is a Marker of Left Ventricular Hypertrophy but Not Hyperinsulinemia in Nondiabetic Atherosclerotic Patients. Arterioscler. Thromb. 13 (6), 900–906. doi:10.1161/01.atv.13.6.900
Rowin, E. J., Hausvater, A., Link, M. S., Abt, P., Gionfriddo, W., Wang, W., et al. (2017). Clinical Profile and Consequences of Atrial Fibrillation in Hypertrophic Cardiomyopathy. Circulation 136 (25), 2420–2436. doi:10.1161/circulationaha.117.029267
Scalsky, R. J., Chen, Y. J., Desai, K., O’Connell, J. R., Perry, J. A., and Hong, C. C. (2021). Baseline Cardiometabolic Profiles and SARS-CoV-2 Infection in the UK Biobank. PLoS One 16 (4), e0248602. doi:10.1371/journal.pone.0248602
Stein, A. B., Jones, T. A., Herron, T. J., Patel, S. R., Day, S. M., Noujaim, S. F., et al. (2011). Loss of H3K4 Methylation Destabilizes Gene Expression Patterns and Physiological Functions in Adult Murine Cardiomyocytes. J. Clin. Invest. 121 (7), 2641–2650. doi:10.1172/jci44641
Tsioufis, C., Stefanadis, C., Toutouza, M., Kallikazaros, I., Toutouzas, K., Tousoulis, D., et al. (2002). Microalbuminuria Is Associated with Unfavourable Cardiac Geometric Adaptations in Essential Hypertensive Subjects. J. Hum. Hypertens. 16 (4), 249–254. doi:10.1038/sj.jhh.1001379
Wang, X. (2014). Firth Logistic Regression for Rare Variant Association Tests. Front. Genet. 5 (7-8), 187. doi:10.3389/fgene.2014.00187
Watkins, H. (2021). Time to Think Differently About Sarcomere-Negative Hypertrophic Cardiomyopathy. Circulation 143 (25), 2415–2417. doi:10.1161/circulationaha.121.053527
Zhao, S., Gu, Y., Coates, G., Groome, L. J., Saleem, M. A., Mathieson, P. W., et al. (2011). Altered Nephrin and Podoplanin Distribution Is Associated with Disturbed Polarity Protein PARD-3 and PARD-6 Expressions in Podocytes from Preeclampsia. Reprod. Sci. 18 (8), 772–780. doi:10.1177/1933719111398145
Keywords: hypertrophic cardiomyopathy, genome-wide association study, genetic susceptibility loci, UK biobank, ICD-10
Citation: Gyftopoulos A, Chen Y-J, Wang L, Williams CH, Chun YW, O’Connell JR, Perry JA and Hong CC (2022) Identification of Novel Genetic Variants and Comorbidities Associated With ICD-10-Based Diagnosis of Hypertrophic Cardiomyopathy Using the UK Biobank Cohort. Front. Genet. 13:866042. doi: 10.3389/fgene.2022.866042
Received: 30 January 2022; Accepted: 13 April 2022;
Published: 24 May 2022.
Edited by:
Brian Hon-Yin Chung, The University of Hong Kong, Hong Kong SAR, ChinaReviewed by:
Christopher Chun Yu Mak, The University of Hong Kong, Hong Kong SAR, ChinaGane Ka-Shu Wong, University of Alberta, Canada
Copyright © 2022 Gyftopoulos, Chen, Wang, Williams, Chun, O’Connell, Perry and Hong. This is an open-access article distributed under the terms of the Creative Commons Attribution License (CC BY). The use, distribution or reproduction in other forums is permitted, provided the original author(s) and the copyright owner(s) are credited and that the original publication in this journal is cited, in accordance with accepted academic practice. No use, distribution or reproduction is permitted which does not comply with these terms.
*Correspondence: James A. Perry, anBlcnJ5QHNvbS51bWFyeWxhbmQuZWR1; Charles C. Hong, Y2hhcmxlcy5ob25nQHNvbS51bWFyeWxhbmQuZWR1