- 1Bio-X Institutes, Key Laboratory for the Genetics of Developmental and Neuropsychiatric Disorders, Shanghai Jiao Tong University, Shanghai, China
- 2Shanghai Key Laboratory of Psychotic Disorders, and Brain Science and Technology Research Center, Shanghai Jiao Tong University, Shanghai, China
- 3School of Mental Health, Jining Medical University, Jining, Shandong, China
- 4Shanghai Center for Women and Children’s Health, Shanghai, China
This study aimed to explore the main influencing factors of suicide risk among Chinese students and establish an early warning model to provide interventions for high-risk students. We conducted surveys of students in their first and third years from a cohort study at Jining Medical College. Logistic regression models were used to screen the early warning factors, and four machine learning models were used to establish early warning models. There were 8 factors related to suicide risk that were eventually obtained through screening, including age, having a rough father, and CES-D, OHQ, ASLEC-4, BFI-Neuroticism, BFI-Openness, and MMC-AF-C scores. A random forest model with SMOTE was adopted, and it verified that these 8 early warning signs, for suicide risk can effectively predict suicide risk within 2 years with an AUC score of 0.947. Among the factors, we constructed a model that indicated that different personality traits affected suicide risk by different paths. Moreover, the factors obtained by screening can be used to identify college students in the same year with a high risk of suicide, with an AUC score that reached 0.953. Based on this study, we suggested some interventions to prevent students going high suicide risk.
Introduction
Suicide is a behavioral expression of serious psychological distress that causes serious public health concerns. Data from the World Health Organization (WHO) show that more than 700 000 people die due to suicide every year (WHO, 2022). For every person who dies due to suicide, there are many more people who attempt suicide (WHO, 2021). Globally, suicidal ideation (SI) and suicide attempt (SA) are more common in the younger population (!!! INVALID CITATION !!! (Eskin et al., 2005; Eskin et al., 2011)). Studies have shown that suicide is one of the main causes of death among adolescents and young adults, including among those in China (Benton et al., 2021).
The rates of suicide mortality vary among countries, and suicide behavior is influenced by ethnicity (Nock et al., 2008; Värnik, 2012). A study on American college students found that approximately 6.3% of college students had SI (Eisenberg et al., 2013). In a survey of suicide among college students in multiple countries, the proportion of Chinese college students who had SI was 22.9%, and the proportion with SA was 6.3% (Eskin et al., 2016). A study involving more than 8,000 Chinese colleges reported that the prevalence of SA was 6.8% (Shen et al., 2020). A meta-analysis involving 160339 Chinese college students calculated that the prevalence of SI was 10.72% (Li et al., 2014). Although the exact number varies among studies, the high frequency of suicide-related manifestations among Chinese college students should be a concern.
In a longitudinal study, it was shown that subjects who had SI during adolescence were twice as likely to have a Diagnostic and Statistical Manual of Mental Disorders (DSM) Axis I disorder and were nearly 12 times more likely to have attempted suicide by the age of 30 (Reinherz et al., 2006). It was also reported that these young patients have caused some pressure on local hospitals and medical systems (Eskin et al., 2016). Considering these facts, it is critical to pay attention to suicide in college students.
Many studies have focused on the risk factors for suicide to better understand suicide (Garlow et al., 2008; Breslin et al., 2020; Costanza et al., 2021). It has been reported that the strong risk factors for suicidal behavior (SB) in college students include psychological distress or depression (Garlow et al., 2008), low social support, affective dysregulation, alcohol use disorder (Arria et al., 2009), and depressogenic cognitive style (Hiramura et al., 2008). In addition, there are also reports indicating that adverse life events, family history, a history of sexual abuse, troubled relationships, impulsivity and difficulties with sexual identity can be risk factors for suicide (Nemeroff et al., 2001; Cooper et al., 2002; Arria et al., 2009; Costanza et al., 2021). The importance of the genetics of suicide was also emphasized in some studies, such as rs7989250, rs589046 and rs199633759, which suggesting the indispensable role of heritability (Otsuka et al., 2019; Erlangsen et al., 2020; Kimbrel et al., 2022; Mullins et al., 2022).
Of all the suicide studies, less attention has been given to early warning signs and identifying suicide in Chinese college students, and potentially important factors such as personality, happiness and attribution method have not been systematically investigated. Previous studies have mainly focused on SI and SA which may overlook some subjects who are at potential risk of suicide and the Suicidal Behaviors Questionnaire-Revised (SBQ-R) could help in this point, which is a well-validated approach to examine students’ suicide risk (Becker et al., 2018).
Therefore, in this work, we aimed to study the suicide situation of Chinese college students and identify the risk factors that affect suicide in Chinese college students, which, in addition to conventional factors, also include subjective well-being and attribution style, to better investigate this issue. This study also tried to establish a suitable early warning model and an identification model for suicide risk via a proper machine learning model.
Materials and methods
Subjects and data collection
Data in this study were collected from a cohort study of Jining Medical College in Shandong, China. The participants in this study were 3,630 Chinese college students from 28 provinces and 263 cities. The freshmen who enrolled in 2016 were selected to complete a basic demographic survey, family situation survey, and other relevant questionnaire surveys, which were used to investigate and evaluate the psychological status of the individuals. In their third year of school, we again conducted this data collection procedure and collected questionnaires about their suicide risk.
In both the baseline and follow-up groups, the exclusion criteria included a questionnaire completion time <600 s, feedback questions with unserious responses (efficacy, understanding, carefulness, significance), and K-means clustering analysis filtering. Furthermore, answers with inconsistencies regarding SI and SA were dropped.
All participants signed the informed consent form, and the study was appraised and approved by the Ethics Committee of Jining Medical University.
Measurements
We used the questionnaire survey to collect information including basic demographic information, family economic and educational situations, mental illness situation of relatives, subjective well-being, life events and attributional causality (details are provided in Table 1 and Table 2). We then integrated these heterogeneous data to explore the factors that most influence college students’ suicide risk.
Information on the social demographic questionnaire is shown in Table 1. Dichotomous variables were encoded using 0 and 1. Regarding the categorical variables, sleep quality was denoted as “1 = very bad”, “2 = bad”, “3 = normal”, “4 = good”, and “5 = very good”. Exercise amount was evaluated and recoded as “1 = < 0.5 h”, “2 = 0.5–11 h”, “3 = 1–2 h”, and “4 = > 2 h”. Parents’ education levels were recoded as “1 = primary school and below”, “2 = junior high school”, “3 = technical secondary school or high school”, “4 = college or university”, and “5 graduate school and above”. Parental character traits were encoded used five variables with 0 and 1. The five traits were coded with five variables, with 1 as having the trait and 0 as not having the trait. The five traits were serious, bright and cheerful disposition, moderate, rough and reticence. Living expenses were evaluated and recoded as “1 = < 500 yuan”, “2 = 500-1,000 yuan”, “3 = 1,000-1,500 yuan”, “4 = 1,500-2,000 yuan”, and “5 = > 2,000 yuan”. Whether immediate family members (parents, siblings, grandparents) had a mental illness was represented by 1 for illness and 0 for no illness.
The questionnaires included the Positive and Negative Affect Scale (PANAS), Center for Epidemiological Survey Depression scale (CES-D) (Li and Hicks, 2010), Oxford Happiness Questionnaire (OHQ) (Hills and Argyle, 2002), Satisfaction with Life Scale (SWLS) (Diener et al., 1985), Adolescent Self-Rating Life Events Checklist (ASLEC) (Li et al., 2020), Social Support Scale for University Students (SSSUS) (Ye and Dai, 2008), Utrecht Work Engagement Scale-Student (UWES-S), General Self-Efficacy Scale (GSES) (Luszczynska et al., 2005), Learning Scales-Academic Self-Efficacy Scale (PALS-ASEC) (Dever and Kim, 2016), Big Five Inventory (BFI), Multidimensional-Multi-attributional Causality Scale (MMCS) (Lefcourt, 1981), Patterns of Adaptive Children’s Perceived Self-Efficacy Scales-Self-Efficacy for Self-Regulated Learning scale (CPSE-SESRL) (Pastorelli et al., 2001) and Suicidal Behaviors Questionnaire-Revised (SBQ-R) (Osman et al., 2001). Moreover, four feedback questions and time elapsed were designed to select reliable data.
Data analysis methods
Quantitative data were described by means (Xs) and standard deviations (SDs). Chi-square and t tests were used to compare the differences between the case group and the control group. Univariate and multivariable logistic regression (LR) models were used to establish the warning model of suicide risk. Principal component analysis (PCA) was used to further simplify the exact important factors. Mediation analysis was used to test the associations between factors. The synthetic minority oversampling technique (SMOTE) was used to address the class imbalance issue (Blagus and Lusa, 2013; Ryu et al., 2019). LR, linear support vector machine (SVM), Gaussian kernel SVM, and random forest (RF) models were used to evaluate the prediction results. For model development, 10-fold cross-validation was used to avoid overfitting and to increase the generalization of the model. In 10-fold cross-validation, data in the training set were partitioned into 10 equally sized folds, and each fold was used once as a validation set, while the other 9 folds were used for training. The area under the receiver operating characteristic (AUROC) curve was adopted to further evaluate the predictive characteristics of the acquired warning model. Statistical analysis software, including SPSS 22.0 and R studio, was used to analyze the data.
Results
A total of 3,630 students completed the online questionnaires, and 2020 students were ultimately included in our study (Extended Data Figure 1). The surveyed students completed the SBQ-R in their third year of school and were divided into the high-risk group (total score of 7 or greater) and the low-risk group (total score of less than 7), which is generally used (Osman et al., 2001; Becker et al., 2018; Dizon et al., 2022).
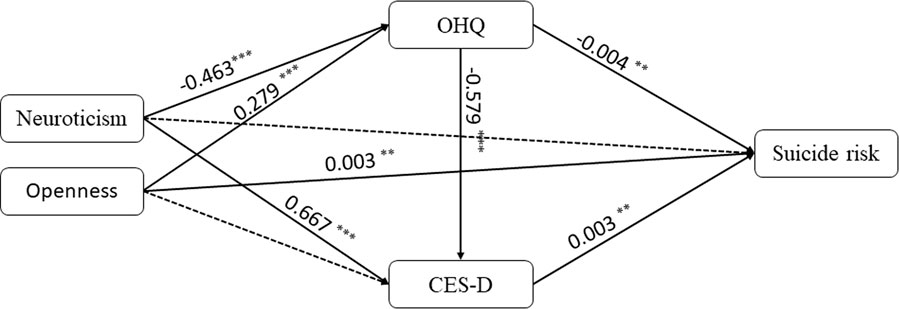
FIGURE 1. Path diagram for the mediation analysis modeling of the BFI-Neuroticism, BFI-Openness, OHQ, CES-D, and suicide risk. BFI, Big Five Inventory; CES-D, the Center for Epidemiological survey, depression scale; OHQ, Oxford Happiness Questionnaire.
We first compared the baseline variables of the two groups of students, as shown in Table 1. There were no significant differences (p > 0.05) between the two groups in some variables, including sex, weight, height, BMI, register, exercise status, father’s education level, mother’s education level, family economic condition, and family living expenses. However, we found that the average age of the high-risk group was significantly younger than that of the low-risk group (p = 0.029). The low-risk group showed a poor sleep status compared to the high-risk group (p < 0.001). There were statistically significant differences in three other variables: father’s character traits (p < 0.001), mother’s personality traits (p = 0.001), and relatives suffering from mental illness (p = 0.003). In total, two groups of major confounding variables were controlled, and they could be used to conduct on baseline data comparisons between the groups.
We found that there were significant differences between the low-risk group and the high-risk group in the scores (or subscale scores) of negative affect (NA), positive affect (PA), and the CES-D, OHQ, SWLS, ASLEC, SSSUS, BFI, GSES, PALS-ASEC, CPSE-SESRL, MMCS, and UWES-S (Table 2).
To simplify the early warning signs, we then used univariate logistic regression on all significantly different factors between the two groups for further factor screening, and a total of 34 factors were found to be significantly related to the suicide risk of college students (Extended Data Table 1). The 34 significant factors in univariate logistic regression were then included in the multivariable logistic regression analysis. The logistic regression (LR) stepwise multivariable logistic regression analysis method was applied to select the early warning predictors of suicide risk. According to different inclusion criteria (Sle) and exclusion criteria (Sls), the likelihood ratio forward entry method was adopted to carry out stepwise multivariate logistic regression analysis. Table 3 demonstrates the results of optimal multivariable LR. The final 8 early warning signs, were age, having a rough father, and the CES-D, OHQ, ASLEC-4, BFI-Neuroticism, BFI-Openness, and MMC-AF-C scores.
PCA revealed that the first four components had 70.7% variance of the 8 risk factors, and it is clearer to identify the exact important factors of suicide risk. The first factor mainly reflected the personality and subjective feeling dimension, the second factor indicated the age dimension, and the third and fourth factors both identified the mixed dimensions of having a father with a rough character, age, and sense of loss.
We noted that the first component included personality and subjective feeling information and then verified the association between the factors. We hypothesized that the OHQ and CES-D scores would be affected by neuroticism and openness and that other confounders would not be affected. The structural equation model was used to test the relationship among the BFI-Neuroticism, BFI-Openness, OHQ, and CES-D scores and suicide risk, while the other confounders were controlled. The indices indicated that the model fit (p < 0.001, CFI = 0.968, RMSEA = 0.068, SRMR = 0.027). The results showed that neuroticism could indirectly affect suicide risk according to the OHQ and CES-D scores, while no significant direct path existed (Figure 1). Openness could affect suicide risk both directly and indirectly. Different personalities affected suicide risk by different paths.
Next, we tested the effectiveness of the warning model for the 8 factors associated with suicide risk. The LR model, SVM (linear) model, SVM (Gaussian) model, and RF model were used to verify the prediction effectiveness under tenfold cross-validation. As indicated in Table 4, the accuracy, sensitivity, specificity, positive predictive value (PPV), and negative predictive value (NPV) for all four methods were over 76%, 67%, 85%, 82%, and 72%, respectively. The AUC in the LR, SVM (linear), SVM (radial), and RF models reached 0.770, 0.767, 0.870, and 0.947, respectively. We further assessed the predictive effect of the model with the first three components. The indices for the 4 models we tested decreased by varying degrees. The RF model still showed excellent performance, with the AUC reaching 0.925 (Table 4).
Considering that the suicide questionnaire has a certain influence on the minds of college students, we hoped to identify the suicide risk of college students by screening early warning signs, to avoid the psychological implications of suicide on college students and the subsequent adverse effects caused by such psychological implications. The four prediction models were again employed for 7 factors, and the results are shown in Table 4. The best identification effect was achieved by using the RF model, with an AUC of 0.953, and the accuracy, sensitivity, specificity, PPV, and NPV reached 95.3%, 93.5%, 97.1%, 97.0%, and 93.7%, respectively. The AUC value of the other three models also exceeded 0.82, which indicated that the 8 factors were capable of identifying suicide risk in college students.
Discussion
We collected information on comprehensive factors that were conjectured to be associated with suicide risk and identified 8 important warning signs that can effectively predict suicide risk in college students. Some of the factors were consistent with previous studies, and some new points are worth noting (Figure 1).
Our results showed that age was a protective factor against suicide risk among college students. Older students had a low risk of suicide. Studies have reported that older age was associated with less neuroticism and openness, which could be one of the reasons why suicide risk was lower in older students (Carciofo et al., 2016). Some studies divided people into various groups by age, while our study divided students using a cross-section (Ivey-Stephenson et al., 2022; Paul and Fancourt, 2022). The age difference between students in the low- and high-risk groups of suicide risk was significant but not much, which indicated that people should take more care of younger students in the same grade. The influence of family members has also been considered by many existing researchers (Svob et al., 2018; Kim, 2019). In this study, we discovered that between the low suicide risk group and the high suicide risk group, there was a significant difference in parental personality traits. Follow-up regression analysis confirmed that having a father with a rough personality would have a significant impact on the suicide risk of college students. Studies have reported that parental relationships and parental company have a certain influence on the SI of teenagers and college students (Saffer et al., 2015; Fu et al., 2017). Our research emphasizes the importance of parental personality traits in the process of getting along with their children. All the results indicated that the impact of parents on suicide risk is substantial, especially for college students, as they are in the transition stage from adolescence to young adulthood and can therefore be greatly influenced by their parents Figure 2.
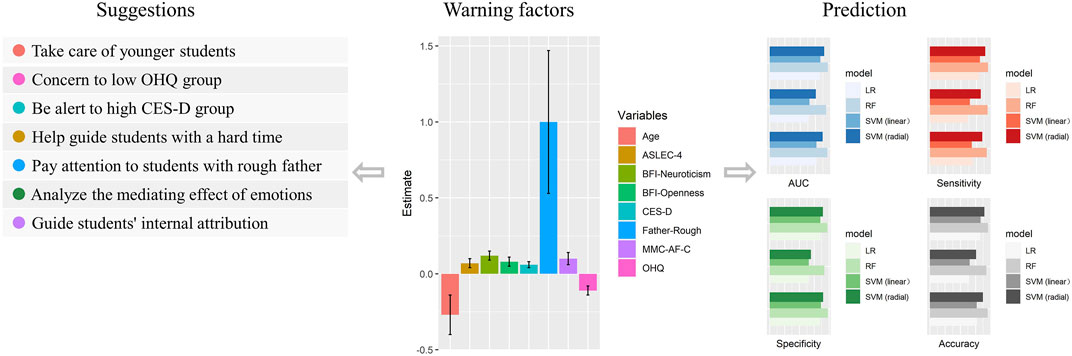
FIGURE 2. Overall schematic diagram of the warning factors, suggestions and model performance. CES–D, the Center for Epidemiological survey, depression scale; OHQ, Oxford Happiness Questionnaire; ASLEC, Adolescent Self–Rating Life Events Checklist; BFI, Big Five Inventory; MMC–AF–C, Multidimensional–Multiattributional Causality Affiliation–Context subscale; LR, logistic regression; RF, random forest; SVM, support vector machine; AUC, area under receiver operating characteristic curve. ASLEC–4 represents: sense of loss factor.
The OHQ score was associated with suicide risk and is related to subjective well-being (SWB). SWB assessments are generally composed of multiple measuring scales, including the SWLS, PANAS, and OHQ (Hou et al., 2020). All three aspects of SWB were significantly associated with suicide risk in the univariate logistic regression results. Evidence has shown that SWB is negatively correlated with depression and that depression is always assciated with suicide. We found that SWB was important in early warning signs of suicide risk, and only the OHQ score was left in the final model. This study revealed that higher happiness levels, as a protective factor, were reflected in the suicide risk assessment. This is also consistent with the conclusions of other studies (Hsu et al., 2019; Hsu et al., 2020). The importance of the OHQ score indicates that not only are students with disease symptoms a focus group, but students with low happiness should also be given attention in school.
There are many studies on suicide clarifying the relationship between depression and SA and SI (Konick and Gutierrez, 2005; Garlow et al., 2008; Lewis et al., 2014). In our research, the mental state of depression was also found to be linked to the risk of suicide. A higher depressive mood can be an early warning sign of college students’ suicide risk, which is also in line with reports on other ethnic groups (AbdElmageed and Mohammed Hussein, 2022; Mullins et al., 2022; Qi and Li, 2022). The loss factor of the ASLEC described the negative life events of the loss of relatives, friends, and property over the last 6 months. Such negative life events increased the risk of suicide. Some studies reported that other factors of the ASLEC were significantly associated with suicide (or suicide-related symptoms) (Shao et al., 2021).
It was noted in this study that the neuroticism and openness of college students were also strong early warning signs for suicide risk. The results were different among different groups in other studies (Heisel et al., 2006; Bluml et al., 2013; Jo et al., 2021). People with a neurotic personality are generally considered to have difficulty handling emotions such as anxiety, hostility, depression, self-awareness, impulsivity, and vulnerability. For college students, their emotions might be unstable and uncontrollable, and college students with a neurotic personality may be more likely to have SI or SA because they have more difficulty in controlling their emotions. People with an openness personality might be open to new experiences, which might lead to a light decision to attempt suicide. College students with such personalities should be given special attention.
Openness, as a strong early warning sign of suicide risk in college students, also differed among different groups. A study of individuals with posttraumatic stress disorder (PTSD) showed that openness predicted lower suicide attempts (Yoo et al., 2018). Another interesting result in this study was that the PTSD group with much higher suicidality had higher scores in openness and neuroticism compared to the control group, while the logistic regressions showed that openness predicted lower SA and neuroticism with no significant result (Yoo et al., 2018). Our results showed a similar result: neuroticism scores were higher in the high-risk group, and openness scores were lower. Logistic regression showed that both neuroticism and openness predicted higher suicide risk. Further PCA showed that openness and the OHQ score had the opposite effect on suicide risk in multivariable logistic regression.
Based on these results, we verified that there was a masking effect between suicide risk and openness, mediated by OHQ and CES-D scores. This result indicated that among different groups, human personality traits played a different role in predicting suicide risk and could be affected by some confounding factors. The masking effect has been less discussed in other studies. This result suggested that the classification of the individuals involved in the study should be given attention.
Another point of concern is that attribution to the interpersonal context can lead to a greater risk of suicide, which means that if a college student attributes their problems or the difficulties encountered in interpersonal relationships to extraversion and attributes these occurrences to environmental factors beyond their control, they would be prone to a greater risk of suicide. We believe that this finding is meaningful for college students. Researchers have paid extensive attention to the role of interpersonal factors in suicide and interpersonal factors related to personality traits (Maxwell, 2005; Van Orden et al., 2010; Baertschi et al., 2017; Baertschi et al., 2018). Our results are useful for further understanding the attribution component of interpersonal relationships. Lectures on the attribution method may be of great help in protecting college students from suicide risk, and instructors are advised to remind students to become accustomed to looking for problems related to their own perspectives.
In this study, based on the 8 early warning signs, the students’ risk of suicide 2 years after completing the survey can be effectively predicted. In the RF model, the AUC value reached 0.947, which means that we established an effective early warning model to divide students into two groups: the low-risk and high-risk groups. This can benefit schools in taking preventative guidance measures for students at risk of suicide, helping them deal with psychological pressure and avoiding the further aggravation of suicide risk in appropriate ways.
We noted that among college students, the high-risk group was commonly unbalanced, with a small percentage. We verified that the SMOTE technique is an effective and valuable method used in suicide prediction, which has been less used in previous studies.
The models using the first three components of PCA showed poor performance in three models: the LR (AUC = 0.641), linear-SVM (0.651), and radial-SMV (0.757) models. However, the RF model provided a valuable result (AUC = 0.925), which indicated that the three components had given enough information to predict suicide risk and that researchers should better use and explore them.
Furthermore, we tested the identification effect of suicide risk in the same year based on the 8 factors. It was believed that it was beneficial to use the SBQ-R in the survey of suicide risk, and it can comprehensively investigate the situation related to suicide among students (Becker et al., 2018). The questionnaire methodology may, however, exert a certain influence on college students because it directly involves negative implications such as suicide itself. Therefore, we suggest using the 8 factors selected in this study to identify the suicide risk of college students instead of the SBQ-R, unless necessary. Models based on the 8 factors demonstrated effective discriminatory power. The AUC values of the four models all exceeded 0.82, and the AUC value of the RF model reached 0.953. This will assist schools in identifying whether college students are at high risk of suicide. Based on some studies, we note that the fear of the suicide survey’s implication for college students might not be entirely founded (Baertschi et al., 2019; Rudd and Bryan, 2022). Talking about suicidality does not encourage suicidality; in contrast, talking about it openly allows an individual to be able to express himself or herself without the fear of being judged. We should continue to pay attention to this effect among Chinese college students.
Our study also suggested a potential link for some genetic level explanations. A number of association studies on suicide have confirmed that some SNPS are loci that affect suicide (Otsuka et al., 2019; Erlangsen et al., 2020; Kimbrel et al., 2022). However, this attempt to explain the genetic factors affecting suicide from a single phenotype is insufficient. Our study suggests that some individual symptoms linked with suicide might help profile the genetic risk of suicide. It is reported that hedonic wellbeing was associated with some loci of FSHR on chromosome 2 and TRIM26 on chromosome 6 (Jamshidi et al., 2022). The rs3131073 was associated with some related conditions including positive-affect, wellbeing spectrum and depression (Howard et al., 2019; Jamshidi et al., 2022). The neuroticism has also identified positive correlative with anxiety disorder and MDD, while revealing a significant genetic overlap between depression and neuroticism (Kendler et al., 2006; Navrady et al., 2018; Forstner et al., 2021). To synthesize the characteristics associated with suicide, a comprehensive consideration of its genetic predisposition may lead to a deeper understanding of the genetic dimension of suicide.
This study also has certain limitations. The research subjects came from one college in China, and the results obtained need to be verified by a wider range of university student research. Additionally, the lack of suicide risk data in the students’ first year makes it difficult to verify the performance of identification in the first year. In this study, we note the importance of false alarms, which means that some students were not defined as being in the high-risk group for suicide but were alarmed by our system. The reason false alarms exist is that the data collected could not give a full view of high-suicide-risk students. False alarms remind us to include more aim indicators in data collection, such as impulsivity, near-infrared spectroscopy (NIRS), electroencephalogram (EEG), and some genetic indices (Lutz et al., 2017; Hirose et al., 2018; Costanza et al., 2021; Iznak et al., 2021; Koenig et al., 2021). Lifetime SA was significantly associated with both higher impulsivity and higher aggression (Costanza et al., 2021). In bipolar disorder patients, current suicide risk was noted to be significantly and positively associated with delayed activation timing of the NIRS signal in the prefrontal region (Hirose et al., 2018). EEG coherence was higher in the nonsuicidal self-injury (NSSI) and SA subgroups than in the NSSI subgroup, especially in the frontal–central–parietal regions. Some metabolic parameters and hormones are associated with suicide behaviors, which should be included in subsequent studies (O'Connor et al., 2020; Zhou et al., 2021). Thyroid stimulating hormone (TSH) could help to differentiate suicide attempters from nonsuicide attempters (Zhou et al., 2021). Cortisol was observed to be significantly associated with suicide attempts, while people younger than 40 years had a positive association, and those older than 40 years had a negative association with suicide attempts (O'Connor et al., 2020). Genome-wide significant SNPs of suicide were also needed to be noticed, such as rs589046, rs199633759, and rs77378519 (Otsuka et al., 2019; Erlangsen et al., 2020; Kimbrel et al., 2022).
In conclusion, we established a concise early suicide warning model and provided a simplified version of the suicide risk identification approach for college students’ suicide risk (Figure 1). The main factors in these models suggested that we should pay attention not only to strong internal and external factors but also to some predisposing factors that are not particularly valued by disease diagnosis, especially in the warning period of up to 2 years. We advocate for some suggestions, including improving students’ happiness, reducing their stress responses to life events, and guiding them to make positive attributions. We look forward to additional research examining the actual effects of these interventions on suicide risk among college students.
Data availability statement
The original contributions presented in the study are included in the article/Supplementary Material, further inquiries can be directed to the corresponding authors.
Ethics statement
The studies involving human participants were reviewed and approved by the Ethics Committee of the Jining Medical University. Written informed consent to participate in this study was provided by the participants’ legal guardian/next of kin. Written informed consent was obtained from the individual(s) for the publication of any potentially identifiable images or data included in this article.
Author contributions
KH conceived the hypothesis, developed the methodology, conducted the survey, collected the data, conducted the analysis, and drafted the manuscript. LJ and CC participated in data collection and critically reviewed manuscript drafts. BH and DR participated in the study design and critically reviewed manuscript drafts. FY participated in the study design, and reviewed manuscript drafts. LL reviewed the data analysis. YB, ZG, NW, MF, KS, CW, and FY participated in survey conduction and data collection. XW participated in the study design and reviewed manuscript drafts. XL and CL participated in survey conduction and data collection. ZZ participated in survey conduction and data collection. RZ participated in survey conduction, and data collection. ZY and YX participated in the study design and critically reviewed manuscript drafts. LH assessed the methodological quality of the included studies and critically reviewed manuscript drafts. YS assessed the methodological quality of the included studies and critically reviewed manuscript drafts. TY conceptualized the research question, conducted the survey, collected the data, and contributed to subsequent drafts of the manuscript. GH conceptualized the research question, supervised KH involvement, assessed the methodological quality, and critically reviewed manuscript drafts. All authors read and approved the final manuscript.
Funding
We appreciate the contribution of the members participating in this study. This project is supported by the National Key Research and Development Program (2016YFC0906400), Innovation Funding in Shanghai (20JC1418600, 18JC1413100), the National Nature Science Foundation of China (82071262, 81671326), Natural Science Foundation of Shanghai (20ZR1427200, 20511101900), Shanghai Municipal Science and Technology Major Project (2017SHZDZX01), the Shanghai Leading Academic Discipline Project (B205), the Technology Department of Sichuan Province (2022YFS0350), the Capacity Building Planning Program for Shanghai Women and Children’s Health Service and The collaborative Innovation Center Project Construction for Shanghai Women and Children’ Health.
Acknowledgments
We appreciate the contribution of the members participating in this study.
Conflict of interest
The authors declare that the research was conducted in the absence of any commercial or financial relationships that could be construed as a potential conflict of interest.
Publisher’s note
All claims expressed in this article are solely those of the authors and do not necessarily represent those of their affiliated organizations, or those of the publisher, the editors and the reviewers. Any product that may be evaluated in this article, or claim that may be made by its manufacturer, is not guaranteed or endorsed by the publisher.
Supplementary material
The Supplementary Material for this article can be found online at: https://www.frontiersin.org/articles/10.3389/fgene.2022.977007/full#supplementary-material
SUPPLEMENTARY FIGURE S1 | Study flowchart.
References
AbdElmageed, R. M., and Mohammed Hussein, S. M. (2022). Risk of depression and suicide in diabetic patients. Cureus 14 (1), e20860. doi:10.7759/cureus.20860
Arria, A. M., O'Grady, K. E., Caldeira, K. M., Vincent, K. B., Wilcox, H. C., and Wish, E. D. (2009). Suicide ideation among college students: A multivariate analysis. Arch. Suicide Res. 13 (3), 230–246. doi:10.1080/13811110903044351
Baertschi, M., Costanza, A., Canuto, A., and Weber, K. (2019). The dimensionality of suicidal ideation and its clinical implications. Int. J. Methods Psychiatr. Res. 28 (1), e1755. ARTN e1755. doi:10.1002/mpr.1755
Baertschi, M., Costanza, A., Canuto, A., and Weber, K. (2018). The function of personality in suicidal ideation from the perspective of the interpersonal-psychological theory of suicide. Int. J. Environ. Res. Public Health 15 (4), E636. ARTN 636. doi:10.3390/ijerph15040636
Baertschi, M., Costanza, A., Richard-Lepouriel, H., Pompili, M., Sarasin, F., Weber, K., et al. (2017). The application of the interpersonal-psychological theory of suicide to a sample of Swiss patients attending a psychiatric emergency department for a non-lethal suicidal event. J. Affect. Disord. 210, 323–331. doi:10.1016/j.jad.2016.12.049
Becker, S. P., Dvorsky, M. R., Holdaway, A. S., and Luebbe, A. M. (2018). Sleep problems and suicidal behaviors in college students. J. Psychiatr. Res. 99, 122–128. doi:10.1016/j.jpsychires.2018.01.009
Benton, T. D., Muhrer, E., Jones, J. D., and Lewis, J. (2021). Dysregulation and suicide in children and adolescents. Child. Adolesc. Psychiatr. Clin. N. Am. 30 (2), 389–399. doi:10.1016/j.chc.2020.10.008
Blagus, R., and Lusa, L. (2013). SMOTE for high-dimensional class-imbalanced data. BMC Bioinforma. 14, 106. doi:10.1186/1471-2105-14-106
Bluml, V., Kapusta, N. D., Doering, S., Brahler, E., Wagner, B., and Kersting, A. (2013). Personality factors and suicide risk in a representative sample of the German general population. PLoS One 8 (10), e76646. doi:10.1371/journal.pone.0076646
Breslin, K., Balaban, J., and Shubkin, C. D. (2020). Adolescent suicide: What can pediatricians do? Curr. Opin. Pediatr. 32 (4), 595–600. doi:10.1097/Mop.0000000000000916
Carciofo, R., Yang, J., Song, N., Du, F., and Zhang, K. (2016). Psychometric evaluation of Chinese-language 44-item and 10-item Big five personality inventories, including correlations with chronotype, mindfulness and mind wandering. PLoS One 11 (2), e0149963. doi:10.1371/journal.pone.0149963
Cooper, J., Appleby, L., and Amos, T. (2002). Life events preceding suicide by young people. Soc. Psychiatry Psychiatr. Epidemiol. 37 (6), 271–275. doi:10.1007/s001270200019
Costanza, A., Rothen, S., Achab, S., Thorens, G., Baertschi, M., Weber, K., et al. (2021). Impulsivity and impulsivity-related endophenotypes in suicidal patients with substance use disorders: An exploratory study. Int. J. Ment. Health Addict. 19 (5), 1729–1744. doi:10.1007/s11469-020-00259-3
Dever, B. V., and Kim, S. Y. (2016). Measurement equivalence of the PALS academic self-efficacy scale. Eur. J. Psychol. Assess. 32 (1), 61–67. doi:10.1027/1015-5759/a000331
Diener, E., Emmons, R. A., Larsen, R. J., and Griffin, S. (1985). The satisfaction with life scale. J. Pers. Assess. 49 (1), 71–75. doi:10.1207/s15327752jpa4901_13
Dizon, J., Mendoza, N. B., Mordeno, I. G., and Nalipay, M. J. N. (2022). Validating the suicide behavior questionnaire-revised among Filipino adults during the COVID-19 pandemic. Death Stud. 17, 1–5. doi:10.1080/07481187.2022.2039327
Eisenberg, D., Hunt, J., and Speer, N. (2013). Mental health in American colleges and universities: Variation across student subgroups and across campuses. J. Nerv. Ment. Dis. 201 (1), 60–67. doi:10.1097/NMD.0b013e31827ab077
Erlangsen, A., Appadurai, V., Wang, Y. P., Turecki, G., Mors, O., Werge, T., et al. (2020). Genetics of suicide attempts in individuals with and without mental disorders: A population-based genome-wide association study. Mol. Psychiatry 25 (10), 2410–2421. doi:10.1038/s41380-018-0218-y
Eskin, M., Sun, J. M., Abuidhail, J., Yoshimasu, K., Kujan, O., Janghorbani, M., et al. (2016). Suicidal behavior and psychological distress in university students: A 12-nation study. Arch. Suicide Res. 20 (3), 369–388. doi:10.1080/13811118.2015.1054055
Forstner, A. J., Awasthi, S., Wolf, C., Maron, E., Erhardt, A., Czamara, D., et al. (2021). Genome-wide association study of panic disorder reveals genetic overlap with neuroticism and depression. Mol. Psychiatry 26 (8), 4179–4190. doi:10.1038/s41380-019-0590-2
Fu, M. C., Xue, Y., Zhou, W. H., and Yuan, T. F. (2017). Parental absence predicts suicide ideation through emotional disorders. Plos One 12 (12), e0188823. ARTN e0188823. doi:10.1371/journal.pone.0188823
Garlow, S. J., Rosenberg, J., Moore, J. D., Haas, A. P., Koestner, B., Hendin, H., et al. (2008). Depression, desperation, and suicidal ideation in college students: Results from the American foundation for suicide prevention college screening project at emory university. Depress. Anxiety 25 (6), 482–488. doi:10.1002/da.20321
Heisel, M. J., Duberstein, P. R., Conner, K. R., Franus, N., Beckman, A., and Conwell, Y. (2006). Personality and reports of suicide ideation among depressed adults 50 years of age or older. J. Affect. Disord. 90 (2-3), 175–180. doi:10.1016/j.jad.2005.11.005
Hills, P., and Argyle, M. (2002). The Oxford happiness questionnaire: A compact scale for the measurement of psychological well-being. Personality Individ. Differ. 33 (7), 1073–1082. Pii S0191-8869(01)00213-6. doi:10.1016/S0191-8869(01)00213-6
Hiramura, H., Shono, M., Tanaka, N., Nagata, T., and Kitamura, T. (2008). Prospective study on suicidal ideation among Japanese undergraduate students: Correlation with stressful life events, depression, and depressogenic cognitive patterns. Arch. Suicide Res. 12 (3), 238–250. doi:10.1080/13811110802100924
Hirose, T., Tsujii, N., Mikawa, W., and Shirakawa, O. (2018). Delayed hemodynamic responses associated with a history of suicide attempts in bipolar disorder: A multichannel near-infrared spectroscopy study. Psychiatry Res. Neuroimaging 280, 15–21. doi:10.1016/j.pscychresns.2018.08.003
Hou, B., Ji, L., Chen, Z., An, L., Zhang, N., Ren, D., et al. (2020). Role of rs454214 in personality mediated depression and subjective well-being. Sci. Rep. 10 (1), 5702. doi:10.1038/s41598-020-62486-x
Howard, D. M., Adams, M. J., Clarke, T. K., Hafferty, J. D., Gibson, J., Shirali, M., et al. (2019). Genome-wide meta-analysis of depression identifies 102 independent variants and highlights the importance of the prefrontal brain regions. Nat. Neurosci. 22(3), 343–352. doi:10.1038/s41593-018-0326-7
Hsu, C. Y., Chang, S. S., and Gunnell, D. (2020). Suicide and happiness: The association between two potential sustainable development goal indicators for mental health. J. Clin. Psychiatry 81 (6), 20l13339. doi:10.4088/JCP.20l13339
Hsu, C. Y., Chang, S. S., and Yip, P. S. F. (2019). Subjective wellbeing, suicide and socioeconomic factors: An ecological analysis in Hong Kong. Epidemiol. Psychiatr. Sci. 28 (1), 112–130. doi:10.1017/S2045796018000124
Ivey-Stephenson, A. Z., Crosby, A. E., Hoenig, J. M., Gyawali, S., Park-Lee, E., and Hedden, S. L. (2022). Suicidal thoughts and behaviors among adults aged ≥18 Years - United States, 2015-2019. MMWR. Surveill. Summ. 71 (1), 1–19. doi:10.15585/mmwr.ss7101a1
Iznak, A. F., Iznak, E. V., Damyanovich, E. V., and Oleichik, I. V. (2021). Differences of EEG frequency and spatial parameters in depressive female adolescents with suicidal attempts and non-suicidal self-injuries. Clin. EEG Neurosci. 52 (6), 406–413. Artn 1550059421991685. doi:10.1177/1550059421991685
Jamshidi, J., Schofield, P. R., Gatt, J. M., and Fullerton, J. M. (2022). Phenotypic and genetic analysis of a wellbeing factor score in the UK Biobank and the impact of childhood maltreatment and psychiatric illness. Transl. Psychiatry 12 (1), 113. ARTN 113. doi:10.1038/s41398-022-01874-5
Jo, A., Kim, H., Lee, J. Y., Kim, J. M., Jeong, M. H., Chung, Y. C., et al. (2021). The effects of patient personality traits and family cohesion on the treatment delay for patients with first-episode schizophrenia spectrum disorder. Early Interv. Psychiatry 15 (4), 889–895. doi:10.1111/eip.13029
Kendler, K. S., Gatz, M., Gardner, C. O., and Pedersen, N. L. (2006). Personality and major depression - a Swedish longitudinal, population-based twin study. Arch. Gen. Psychiatry 63(10), 1113–1120. doi:10.1001/archpsyc.63.10.1113
Kim, H. H. (2019). Parental overprotection and youth suicide behavior in low- and middle-income countries: A multilevel analysis of cross-national data. Int. J. Public Health 64 (2), 173–184. doi:10.1007/s00038-018-1169-4
Kimbrel, N. A., Ashley-Koch, A. E., Qin, X. J., Lindquist, J. H., Garrett, M. E., Dennis, M. F., et al. (2022). A genome-wide association study of suicide attempts in the million veterans program identifies evidence of pan-ancestry and ancestry-specific risk loci. Mol. Psychiatry 27 (4), 2264–2272. doi:10.1038/s41380-022-01472-3
Koenig, J., Hoper, S., van der Venne, P., Murner-Lavanchy, I., Resch, F., and Kaess, M. (2021). Resting state prefrontal cortex oxygenation in adolescent non-suicidal self-injury-A near-infrared spectroscopy study. Neuroimage. Clin. 31, 102704. ARTN 102704. doi:10.1016/j.nicl.2021.102704
Konick, L. C., and Gutierrez, P. M. (2005). Testing a model of suicide ideation in college students. Suicide Life. threat. Behav. 35 (2), 181–192. doi:10.1521/suli.35.2.181.62875
Lewis, A. J., Bertino, M. D., Bailey, C. M., Skewes, J., Lubman, D. I., and Toumbourou, J. W. (2014). Depression and suicidal behavior in adolescents: A multi-informant and multi-methods approach to diagnostic classification. Front. Psychol. 5, 766. doi:10.3389/fpsyg.2014.00766
Li, J., Chen, Y. P., Zhang, J., Lv, M. M., Valimaki, M., Li, Y. F., et al. (2020). The mediating role of resilience and self-esteem between life events and coping styles among rural left-behind adolescents in China: A cross-sectional study. Front. Psychiatry 11, 560556. doi:10.3389/fpsyt.2020.560556
Li, Z., and Hicks, M. H. (2010). The CES-D in Chinese American women: Construct validity, diagnostic validity for major depression, and cultural response bias. Psychiatry Res. 175 (3), 227–232. doi:10.1016/j.psychres.2009.03.007
Li, Z. Z., Li, Y. M., Lei, X. Y., Zhang, D., Liu, L., Tang, S. Y., et al. (2014). Prevalence of suicidal ideation in Chinese college students: A meta-analysis. Plos One 9 (10), e104368. ARTN e104368. doi:10.1371/journal.pone.0104368
Luszczynska, A., Gutierrez-Dona, B., and Schwarzer, R. (2005). General self-efficacy in various domains of human functioning: Evidence from five countries. Int. J. Psychol. 40 (2), 80–89. doi:10.1080/00207590444000041
Lutz, P. E., Mechawar, N., and Turecki, G. (2017). Neuropathology of suicide: Recent findings and future directions. Mol. Psychiatry 22 (10), 1395–1412. doi:10.1038/mp.2017.141
Mullins, N., Kang, J., Campos, A. I., Coleman, J. R. I., Edwards, A. C., Galfalvy, H., et al. (2022). Dissecting the shared genetic architecture of suicide attempt, psychiatric disorders, and known risk factors. Biol. Psychiatry 91 (3), 313–327. doi:10.1016/j.biopsych.2021.05.029
Navrady, L. B., Adams, M. J., Chan, S. W. Y., Ritchie, S. J., McIntosh, A. M., and Working, M. D. D. (2018). Genetic risk of major depressive disorder: The moderating and mediating effects of neuroticism and psychological resilience on clinical and self-reported depression. Psychol. Med. 48 (11), 1890–1899. doi:10.1017/S0033291717003415
Nemeroff, C. N., Compton, M. T., and Berger, J. (2001). “The depressed suicidal patient - assessment and treatment,”, Ann. N. Y. Acad. Sci., CLINICAL SCIENCE OF SUICIDE PREVENTION, 932, 1–23. doi:10.1111/j.1749-6632.2001.tb05795.x
Nock, M. K., Borges, G., Bromet, E. J., Cha, C. B., Kessler, R. C., and Lee, S. (2008). Suicide and suicidal behavior. Epidemiol. Rev. 30, 133–154. doi:10.1093/epirev/mxn002
O'Connor, D. B., Gartland, N., and O'Connor, R. C. (2020). Stress, cortisol and suicide risk. Int. Rev. Neurobiol. 152, 101–130. doi:10.1016/bs.irn.2019.11.006
Osman, A., Bagge, C. L., Gutierrez, P. M., Konick, L. C., Kopper, B. A., and Barrios, F. X. (2001). The suicidal behaviors questionnaire-revised (SBQ-R): Validation with clinical and nonclinical samples. Assessment 8 (4), 443–454. doi:10.1177/107319110100800409
Otsuka, I., Akiyama, M., Shirakawa, O., Okazaki, S., Momozawa, Y., Kamatani, Y., et al. (2019). Genome-wide association studies identify polygenic effects for completed suicide in the Japanese population. Neuropsychopharmacology 44 (12), 2119–2124. doi:10.1038/s41386-019-0506-5
Pastorelli, C., Caprara, G. V., Barbaranelli, C., Rola, J., Rozsa, S., and Bandura, A. (2001). The structure of children's perceived self-efficacy: A cross-national study. Eur. J. Psychol. Assess. 17(2), 87–97. doi:10.1027//1015-5759.17.2.87
Paul, E., and Fancourt, D. (2022). Factors influencing self-harm thoughts and behaviours over the first year of the COVID-19 pandemic in the UK: Longitudinal analysis of 49 324 adults. Br. J. Psychiatry. 220 (1), 31–37. doi:10.1192/bjp.2021.130
Qi, H. J., and Li, L. F. (2022). Association of atopic dermatitis with depression and suicide: A two-sample mendelian randomization study. Biomed. Res. Int. 2022, 4084121. doi:10.1155/2022/4084121
Reinherz, H. Z., Tanner, J. L., Berger, S. R., Beardslee, W. R., and Fitzmaurice, G. M. (2006). Adolescent suicidal ideation as predictive of psychopathology, suicidal behavior, and compromised functioning at age 30. Am. J. Psychiatry 163(7), 1226–1232. doi:10.1176/appi.ajp.163.7.1226
Rudd, M. D., and Bryan, C. J. (2022). Finding effective and efficient ways to integrate research advances into the clinical suicide risk assessment interview. Front. Psychiatry 13, 846244. ARTN 846244. doi:10.3389/fpsyt.2022.846244
Ryu, S., Lee, H., Lee, D. K., Kim, S. W., and Kim, C. E. (2019). Detection of suicide attempters among suicide ideators using machine learning. Psychiatry Investig. 16 (8), 588–593. doi:10.30773/pi.2019.06.19
Saffer, B. Y., Glenn, C. R., and David Klonsky, E. (2015). Clarifying the relationship of parental bonding to suicide ideation and attempts. Suicide Life. threat. Behav. 45 (4), 518–528. doi:10.1111/sltb.12146
Shao, C., Wang, X., Ma, Q., Zhao, Y., and Yun, X. (2021). Analysis of risk factors of non-suicidal self-harm behavior in adolescents with depression. Ann. Palliat. Med. 10 (9), 9607–9613. doi:10.21037/apm-21-1951
Shen, Y. M., Meng, F. C., Xu, H. M., Li, X., Zhang, Y. R., Huang, C. X., et al. (2020). Internet addiction among college students in a Chinese population: Prevalence, correlates, and its relationship with suicide attempts. Depress. Anxiety 37 (8), 812–821. doi:10.1002/da.23036
Svob, C., Wickramaratne, P. J., Reich, L., Zhao, R., Talati, A., Gameroff, M. J., et al. (2018). Association of parent and offspring religiosity with offspring suicide ideation and attempts. JAMA Psychiatry 75 (10), 1062–1070. doi:10.1001/jamapsychiatry.2018.2060
Van Orden, K. A., Witte, T. K., Cukrowicz, K. C., Braithwaite, S. R., Selby, E. A., and Joiner, T. E. (2010). The interpersonal theory of suicide. Psychol. Rev. 117 (2), 575–600. doi:10.1037/a0018697
Värnik, P. (2012). Suicide in the World. Int. J. Environ. Res. Public Health 9 (3), 760–771. doi:10.3390/ijerph9030760
Ye, Y., and Dai, .X. (2008). Development of social support scale for university students. Chin. J. Clin. Psychol. 16 (5), 456–458.
Yoo, Y., Park, H. J., Park, S., Cho, M. J., Cho, S. J., Lee, J. Y., et al. (2018). Interpersonal trauma moderates the relationship between personality factors and suicidality of individuals with posttraumatic stress disorder. PLoS One 13 (1), e0191198. doi:10.1371/journal.pone.0191198
Zhou, Y. J., Ren, W. C., Sun, Q. Q., Yu, K. M., Lang, X. E., Li, Z. Z., et al. (2021). The association of clinical correlates, metabolic parameters, and thyroid hormones with suicide attempts in first-episode and drug-naive patients with major depressive disorder comorbid with anxiety: A large-scale cross-sectional study. Transl. Psychiatry 11 (1), 97. ARTN 97. doi:10.1038/s41398-021-01234-9
Keywords: college students, suicide risk, early warning, masking effect, affiliation attribution
Citation: Han K, Ji L, Chen C, Hou B, Ren D, Yuan F, Liu L, Bi Y, Guo Z, Wu N, Feng M, Su K, Wang C, Yang F, Wu X, Li X, Liu C, Zuo Z, Zhang R, Yi Z, Xu Y, He L, Shi Y, Yu T and He G (2022) College students’ screening early warning factors in identification of suicide risk. Front. Genet. 13:977007. doi: 10.3389/fgene.2022.977007
Received: 24 June 2022; Accepted: 31 October 2022;
Published: 10 November 2022.
Edited by:
Diego Quattrone, King’s College London, United KingdomReviewed by:
Zezhi Li, Guangzhou Medical University, ChinaAlessandra Costanza, Université de Genève, Switzerland
Copyright © 2022 Han, Ji, Chen, Hou, Ren, Yuan, Liu, Bi, Guo, Wu, Feng, Su, Wang, Yang, Wu, Li, Liu, Zuo, Zhang, Yi, Xu, He, Shi, Yu and He. This is an open-access article distributed under the terms of the Creative Commons Attribution License (CC BY). The use, distribution or reproduction in other forums is permitted, provided the original author(s) and the copyright owner(s) are credited and that the original publication in this journal is cited, in accordance with accepted academic practice. No use, distribution or reproduction is permitted which does not comply with these terms.
*Correspondence: Yi Shi, eWlzaGlAc2p0dS5lZHUuY24=; Tao Yu, eXV0YW9Ac2p0dS5lZHUuY24=; Guang He, aGVndWFuZ2Jpb3hAMTYzLmNvbQ==