- 1Department of Biochemistry and Molecular Biology, Medical College, Soochow University, Suzhou, China
- 2Department of Human Anatomy, Histology and Embryology, Medical College, Soochow University, Suzhou, China
- 3Department of Experimental Center, Medical College, Soochow University, Suzhou, China
Intrahepatic cholangiocarcinoma (iCCA) patients had no obvious symptoms at early stage and poor postoperative survival. Therefore, the establishment of an iCCA prognostic prediction model to carry out refined management of iCCA patients is expected to improve the survival of the iCCA patient population. In this paper, we analyzed the expression profiling data of patients from 32 iCCA tissues and eight paracancerous tissues in The Cancer Genome Atlas (TCGA) database. Perl software was used to separate M6A-related genes and lncRNAs from expression matrix files obtained from the TCGA database. The differentially expressed lncRNAs in the iCCA samples and the normal samples were screened out by differential analysis using the R package limma, and the m6A-related lncRNAs were further screened by Pearson correlation analysis. WGCNA clustering analysis constructs a random network to extract the module genes most related to iCCA, and take the intersection of differentially expressed lncRNAs related to m6A. Univariate Cox screening was performed for the intersection lncRNAs that had significant influence on the prognosis of iCCA patients, and further screening was performed by LASSO method and multivariate Cox regression analysis. Risk model was constructed and prognostic ability was evaluated according to risk score. In conclusion, we identified four m6A-related lncRNAs with potential prognostic value in iCCA, and established a novel m6A-related lncRNA-based prognostic model, which can be used as an independent prognostic factor to predict the prognosis of clinical patients.
Introduction
Cholangiocarcinoma (CCA) is a malignant tumor that occurs in the lining epithelium of the bile duct system. It is the most common biliary tract malignant tumor and the second most common primary liver malignant tumor, accounting for about 2% of all cancer patients worldwide. Cholangiocarcinoma can be further divided into intrahepatic Cholangiocarcinoma (iCCA), perihilar Cholangiocarcinoma (pCCA) and distal Cholangiocarcinoma (dCCA), the latter two can be collectively referred to as extrahepatic Cholangiocarcinoma (ECC) (Massarweh and El-Serag, 2017; Rizvi et al., 2018). Relevant studies show that China is one of the Asian countries with a high incidence of intrahepatic cholangiocarcinoma. Due to the insignificant early symptoms of intrahepatic cholangiocarcinoma and the lack of effective screening markers, patients are often diagnosed in the middle and late stages, missing the best opportunity for surgery, the treatment effect is not ideal, and the prognosis of the patient is poor (Shindoh and Vauthey, 2014).
Epigenetics is the regulation of gene expression in a manner independent of genome sequence and plays a very important role in various diseases and tumors (Feinberg, 2018). In general, epigenetic regulation refers to the diverse and reversible chemical modifications of DNA and histones. In addition to DNA and histones, intracellular RNAs (mRNA, lncRNA,snRNA,etc.) also have different types of post-transcriptional modifications, among which the most common is N6-adenosine methylation (m6A) (Roundtree and He, 2016). m6A methylation refers to the addition of a methyl group to the sixth nitrogen atom of adenine to form 6-methyladenine, which is about 0.1–0.4% in total RNA of adenosine is methylated by m6A (Zhang C. Y. et al., 2019). As the most abundant post-transcriptional modification at the RNA level (Meyer et al., 2012), m6A methylation modification is a dynamic, reversible modification process mediated by m6A WER (“ writers”, “Erasers” and “Reader”) (Panneerdoss et al., 2018). Among them, methyltransferases (writers), which are mainly responsible for adding methyl groups to specific sites in the mRNA of specific target genes, are composed of catalytic subunit methyltransferase-like 3 (METTL3), methyltransferase-like 14 (METTL14), Wilms’ tumor 1-associating protein (WTAP) and other proteins (Scholler et al., 2018). Demethylases (erasers), including proteins such as ALKBH5 and FTO, function to recognize and remove m6A methylation (Xu et al., 2020). In addition, YTHDF and YTHDC family members are generally considered to be m6A methylated readers, which can specifically recognize and bind m6A methylation sites and induce their corresponding functions (Yue et al., 2019). Growing evidence suggests that m6A modifications play important biological functions in mammals (Kato and Natarajan, 2019; Mayo et al., 2021). Long non-coding RNA (lncRNA) is a non-coding RNA with a length of more than 200 nucleotides, which generally does not encode proteins and polypeptides. Compared with mRNA, it has stronger spatiotemporal specificity and lower conservation between species (Rinn et al., 2007; Peng W. X. et al., 2017). LncRNAs can participate in the regulation of genomic imprinting, stem cell pluripotent differentiation, embryonic development, cardiac development, hematopoiesis and immune systems, and endocrine systems and other biological physiological processes (Bhan et al., 2017; Huang, 2018). And lncRNA can act as molecular scaffolds in the nucleus, assist alternative splicing, regulate chromosome structure, regulate translation in the cytoplasm, promote or inhibit mRNA degradation, adsorb miRNA, etc (Satpathy and Chang, 2015).
M6A modification affect the function of lncRNAs through multiple regulatory mechanisms. On the one hand, m6A modification acts on the RNA-DNA triple helix structure and regulates the relationship between lncRNA and specific DNA sites. On the other hand, m6A modification provides binding sites for methylated readers (readers) or regulates the structure of local RNAs, thereby inducing the binding of RNA-binding proteins (RBPs) and regulating the function of lncRNAs(Huang et al., 2020).
Weighted gene co-expression network analysis (WGCNA) is a comprehensive analysis technology based on biological network, which can identify a class of genes (or proteins) that are co-expressed, associate clustering modules with phenotypes through algorithms, and explore the core of the modules gene (or protein) (Langfelder and Horvath, 2008). The protein interaction network is composed of interactions between proteins, and participates in biological processes such as signal transmission, gene expression regulation, and material metabolism. It can be used for polygenic analysis of complex mechanisms and large-scale datasets, and can also be used to reveal associations between genes in different samples (Zhang et al., 2021).
The LncSEA database integrates the collection of lncRNA functional information from more than 20 published databases, including lncRNA functional collections including downstream regulation, as well as TF ChIP-seq, DNase-seq, ATAC-seq, and H3K27ac ChIP-seq. This database focuses on the published information of various human lncRNAs, and can perform annotation and enrichment analysis on the lncRNA collections submitted by users, providing more than 40,000 reference lncRNA collections, including 18 types and 66 subtypes, including more than 50,000 lncRNAs(Chen et al., 2021).
The Cox proportional hazards model is essentially a regression model commonly used in statistics in medical research to study the association between a patient’s survival time and one or more predictor variables. It is suitable for both quantitative predictors and categorical variables (Cox, 1972). Univariate Cox analysis is usually used to remove collinearity, but it may lead to synergistic effects brought by other variables, so multivariate Cox regression is performed to correct other factors, which is often used for survival analysis data modeling, etc. Thus, an m6A-related prognostic model may be helpful in the understanding and management of iCCA (Huang and Liu, 2006; Li B. et al., 2016). Here, we investigated the prognostic and immunologic significance of m6Arelated lncRNAs and developed an m6A-related lncRNA prognostic model to predict survival outcomes in patients with iCCA.
Materials and methods
Data sources
Using The Cancer Genome Atlas (TCGA-GDC, Data release 32.0)which was updated on 29 March2022, we obtained transcriptome-sequencing (RNA-seq) information from 32 iCCA samples and eight adjacent non-tumor samples with corresponding clinical data. Perl (Strawberry Perl v5.30.2) was used to extract the gene expression information of lncRNAs, and Python (Python 3.6) was used to match 23 m6A-related genes (METTL3, METTL14, METTL16, WTAPI, VIRMA, ZC3H13, RBM15, RBM15B, YTHDC1, YTHDC2, YTHDF1, YTHD F2, YTHDF3, HNRNPC, FMR1, LRPPRC, HNRNPA2B1, IGFBP1, IGFBP2, IGFBP3, RBMX, FTO, ALKBH5) expression matrix (Deng et al., 2018; Chen et al., 2019).
Differential lncRNAs analysis and m6A-related lncRNAs identification
Using the limma R package, the sorted lncRNAs expression matrix was used for gene difference analysis (|log2FC|>1, p< 0.05), and these up-regulated or down-regulated genes were plotted by plotting volcano plot and heatmaps shows up. Pearson correlation analysis was used to identify m6A-related lncRNAs(|Pearson R| > 0.35, p < 0.025) (Xu et al., 2021).
Construction of a weighted gene functional co-expression network
The gene co-expression network analysis was constructed using the WGCNA package of the R. In order to ensure that it conforms to the scale-free network distribution, the “pickSoftTreshold” function in the WGCNA package calculates the correlation coefficient of the β value and the mean value of gene connectivity, and selects an appropriate soft threshold β to make the network more flexible. Then, a one-step method is used to construct the related gene network, the adjacency matrix is converted into a topological overlap matrix TOM, and a hierarchical clustering tree of a gene is generated by hierarchical clustering. Calculate gene significance (GS) and module significance (MS) to measure the significance of genes and clinical traits, and analyze significant associations between modules and models.
Network module establishment and functional annotation of lncRNAs
The most significant associations with cancer traits in the WGCNA modules were selected for study and compared with the association results using Python. Retaining the part of the most significant modules of WGCNA that coincides with the correlation results, and finally obtaining the correlation network of m6A-related lncRNAs. Use Gephi (v0.9.2) software to draw the network, export the network nodes, modules and in- and out-degree information, and import the lncRNAs with higher weights into the lncSEA (http://bio.liclab.net/LncSEA/index.php) for Annotation Analysis.
Prognostic analysis
Univariate Cox regression analysis was performed for all lncRNAs in the network (KM < 0.04, p < 0.05), and then the LASSO regression method was used to further screen m6A-related prognostic lncRNAs. Perform multivariate Cox regression analysis for prognostic modeling and draw forest plots. Using the Kaplan-Meier method of the “survival” package to perform survival analysis on the screened genes, and draw survival curves. According to the median prognostic risk value, the samples of patients with intrahepatic cholangiocarcinoma were divided into low-risk group and high-risk group, and the risk level between the low-risk group and the high-risk group, as well as the survival status and survival time of the patients were drawn as a scatterplot, and then use the Kaplan-Meier method to draw survival curves for the risk model. Compare risk values to various clinical traits and perform independent prognostic analyses to test the prognostic power of risk models. The Roc curve was drawn to represent the accuracy of predicting patient survival through the model, and the accuracy was judged by the area under the curve.
Statistical analysis
In this study, the R (version 4.2.0) and RStudio software were utilized to carry out the statistical analysis and figure preparation. The quantitative data from the control and the experimental groups were compared by using the student t test. Pearson correlation was used to calculate the correlation coefficients. p-values less than 0.05 were defined as statistically significant.
Results
Screening of differentially expressed and m6A-related lncRNAs in iCCA
In order to screen and identify m6A-related lncRNAs, we downloaded the transcriptome and clinical data of iCCA samples and adjacent normal samples from the TCGA database (matched to the expression matrix containing 22 m6A regulators and lncRNAs). The limma was used to analyze the differentially expressed lncRNAs in normal and iCCA samples, and finally 3,099 differential lncRNAs were obtained (|log2 FC|>1, p< 0.05). Differentially expressed genes are plotted on a volcano plot (Figure 1A), where red represents up-regulated genes and green represents down-regulated genes. At the same time, a heatmap (Figure 1B) is drawn, where red represents gene up-regulation and green represents gene down-regulation. Pearson correlation analysis was used to identify 2,848 lncRNAs associated with m6A (|Pearson R|>0.35, p< 0.025).
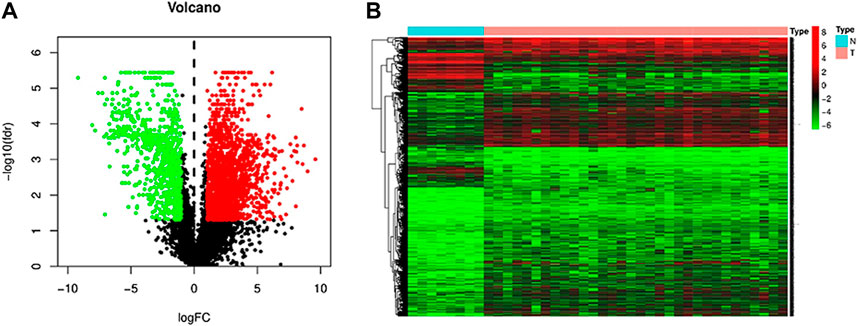
FIGURE 1. Differentially expressed lncRNAs in iCCA patients and adjacent normal patients. (A) Volcano map of differentially expressed lncRNAs (red dots represent up-regulated genes, green dots represent down-regulated genes). (B) Cluster heat map of hetero-expressed gene.
Construction of weighted gene co-expression network analysis
WGCNA analysis was performed using m6A regulators and expression matrix of lncRNAs. After setting the height to 2000, one outlier sample was removed, and 32 iCCA samples and seven normal samples were retained for subsequent analysis (Figure 2A).The pick Soft Threshold function is used to weight the parameter β. The results show that when β = 4, the scale-free topology fitting index R2 reaches 0.9. At this time, the average network connection corresponding to this threshold is close to the scale-free network (Figure 2B). Obtain the dissimilarity matrix dissTOM between genes, perform hierarchical clustering on the dissimilarity matrix to obtain a gene clustering tree, and combine high similarity modules to obtain 15 modules, that is, 22 m6A regulators and 13,975 lncRNAs are finally divided into 15 co-expression network modules, successfully constructed a scale-free network and completed the division of gene modules (Figure 2C). According to the heat map results of the correlation between modules and traits in WGCNA, we selected the blue module with the highest correlation coefficient (r = 0.97, p< 0.01) for follow-up research (Figure 2D).
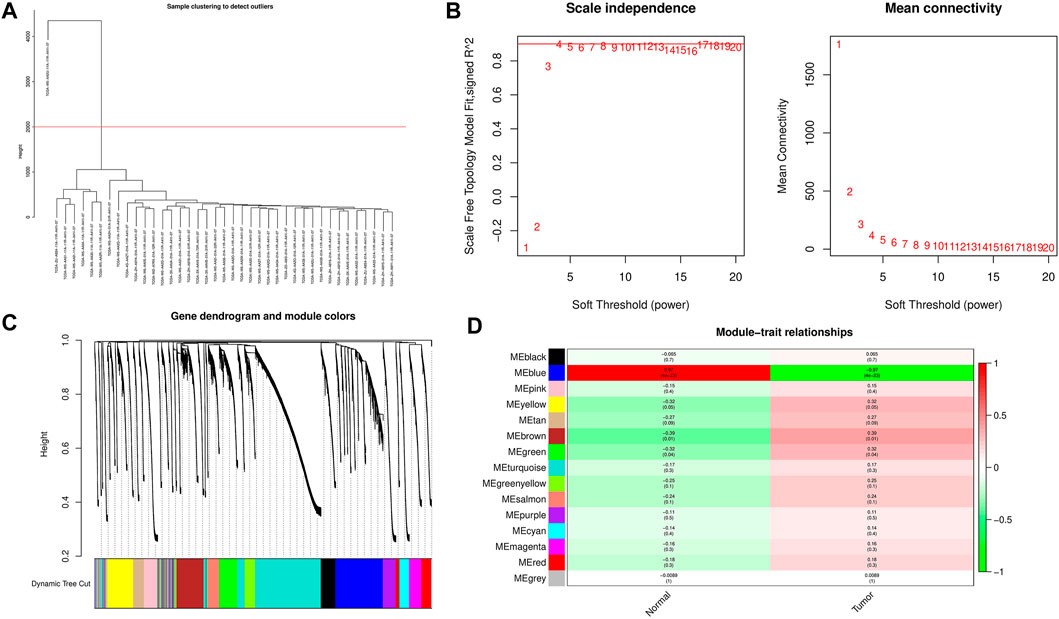
FIGURE 2. Construction of co-expression modules related to iCCA. (A) Set the height to 2000 to remove outliers. (B) Determine the best soft threshold (In the process of module selection, the adjacency matrix is converted into a topology matrix, and the optimal soft threshold β = 4 is determined). (C) Cluster tree of coexpressed gene modules (D) The correlation between the gene module and clinical information (The redder the color, the higher the correlation; the figure in the figure is the Pearson correlation coefficient, in brackets. The number is the corresponding p value).
Network module establishment and functional annotation of lncRNAs
Comparing the node-node information in the blue module with the node-node information of the correlation, and taking the intersection, a total of 723 nodes are obtained, corresponding to 1,560 edges, that is, the correlation co-expression network. By mapping the network using the Force Atlas layout of Gephi software, the network was divided into five modules, and the labels of the m6A regulators were abbreviated and displayed, resulting in nine m6A regulators in the WGCNA highly correlated modules (Figure 3). The network map of Gephi is derived, and the information of m6A regulators is selected (Table 1). The co-expression of these nine m6A regulators regulates a large number of lncRNAs. 207 lncRNAs with a weight of nine were imported into the LncSEA platform for annotation, and a total of five results were obtained, namely 1) RNA Protein interaction (Figure 4A); 2) RNA interaction (Figure 4B); 3) survival (Figure 4C); 4) RNA Histone modification (Figure 4D); 5) Cancer functional state (Figure 4E).
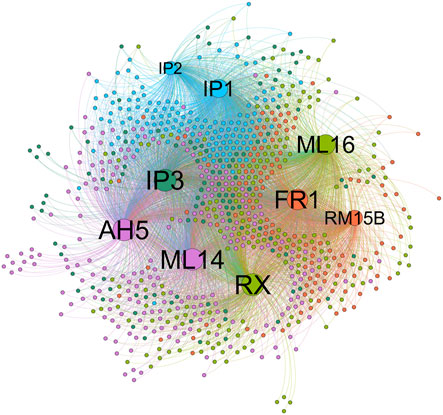
FIGURE 3. Correlation coexpression network graph (Use Gephi software’s Force Atlas layout to map the network).
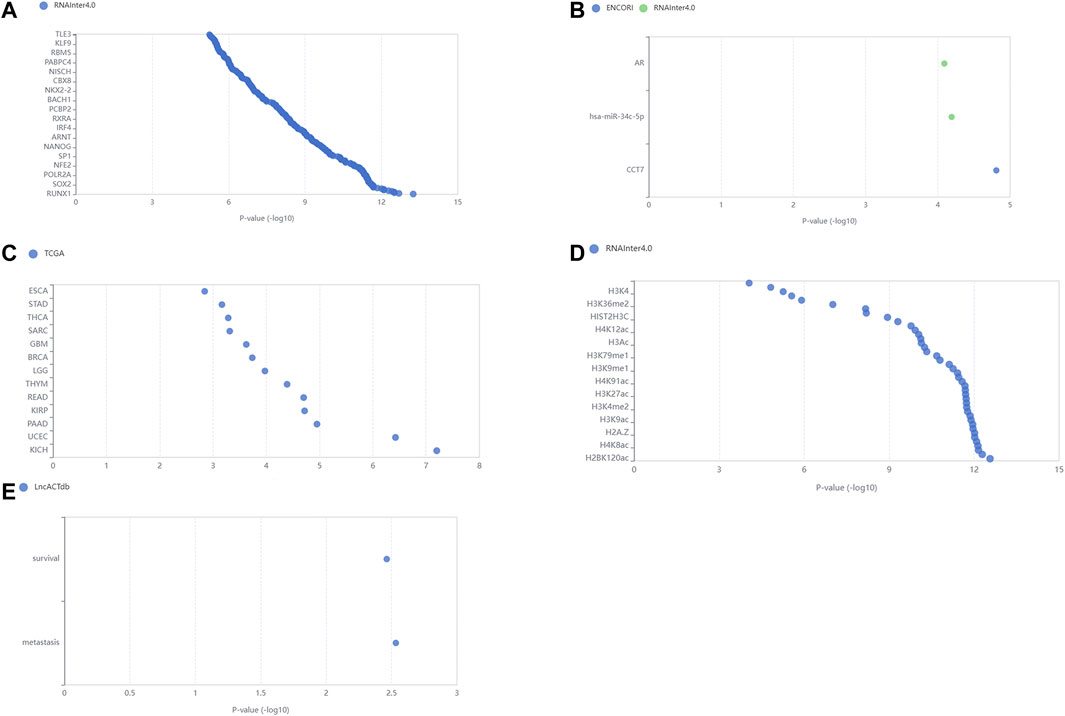
FIGURE 4. LncSEA platform annotation analysis results. These lncRNAs are closely associated with (A) RNA Protein interaction; (B) RNA interaction; (C) survival; (D) RNA Histone modification; (E) Cancer functional state.
Cox analysis and risk model establishment
The lncRNAs related to the above nine m6A regulators were screened by the single-factor Cox method (Table2), and the four m6A-related lncRNAs related to prognosis were screened by the LASSO method (Figure 5A). On this basis, further multivariate Cox regression analysis was performed, and it was found that four lncRNA, AC015917.2, AC010735.2, LINC02160, and AC009063.3, may have a significant impact on the prognosis of patients with iCCA (Figure 5B). Construction of a multivariate Cox model of prognostic risk in patients with iCCA using four lncRNAs (Table 3). According to the multivariate COX regression analysis, the regression coefficient of each lncRNA was calculated as the hazard ratio (HR), and the risk score formula was obtained as the prognostic model:
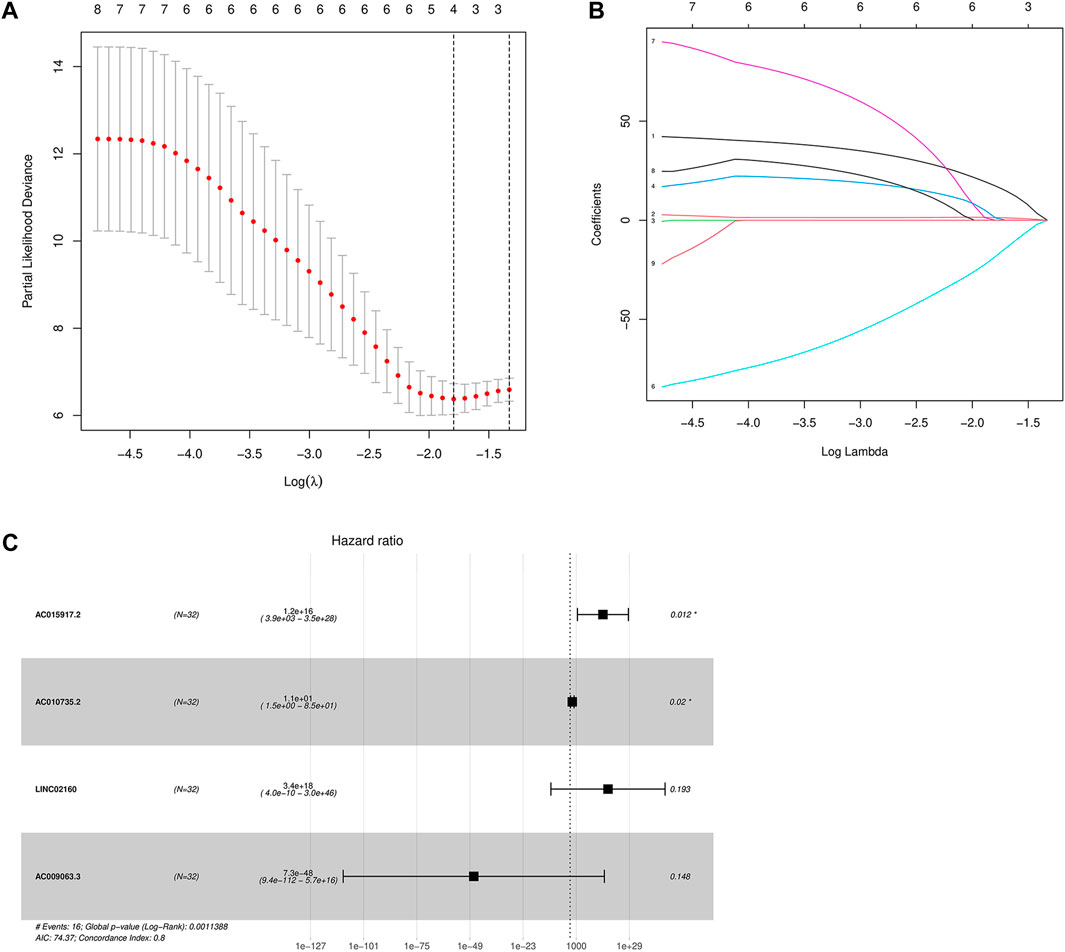
FIGURE 5. (A,B): LASSO Cox regression analysis determined four m6A-related lncRNAs; (C) Multivariate Cox forest diagram.
Draw a forest diagram (Figure 5C). According to the risk model, the risk value of all patients with intrahepatic cholangiocarcinoma can be obtained.
Survival analysis and independent prognostic analysis
The four long-chain non-coding expression matrices obtained in the model were combined with the survival time and survival status of the clinical data, and the survival curve was drawn (Figure 6(A-D)). It was found that p = 0.0017 for AC015917.2, p = 0.0195, p = 0.0324 for LINC02160, and p = 0.0088 for AC009063.3, indicating that these four lncRNAs may have an impact on the prognosis of iCCA patients. The median of the patient’s risk value was used as the threshold, and the patients were divided into high-risk group and low-risk group (Figure 7A). Status and survival time were analyzed. (Figure 7B). After dividing the samples into high and low risk groups according to the median prognostic risk level, the survival analysis result p = 0.006 (Figure 7C). It showed that the OS of the low-risk group was longer than that of the high-risk group, which preliminarily verified the prognostic ability of this model. Univariate and multivariate independent prognostic analyses were performed on the above four m6A-related lncRNAs respectively (Figures 7D, E), indicating that the risk model of the four m6A-related lncRNAs was independent of other clinicopathological parameters (such as gender, stage, TNM stage). The Roc curve showed the risk model AUC = 0.912, which suggests that the model is far superior to other clinical traits in predicting patient surviva (Figure 7F).
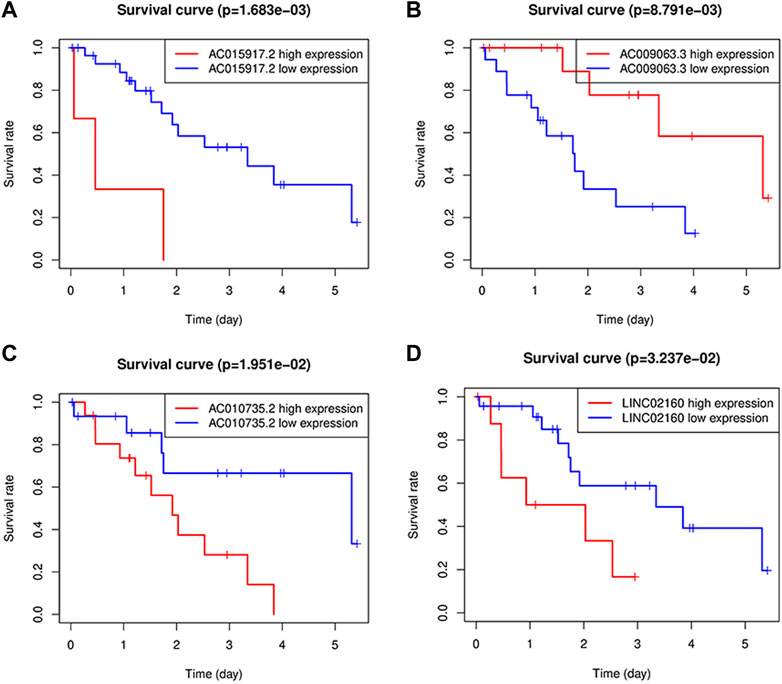
FIGURE 6. Kaplan-Meier survival analysis of four lncRNAs; (A-D): Survival curve, where AC015917.2 AC010735.2, LINC02160 high expression, AC009063.3 patients with low expression of iCCA OS is poor.
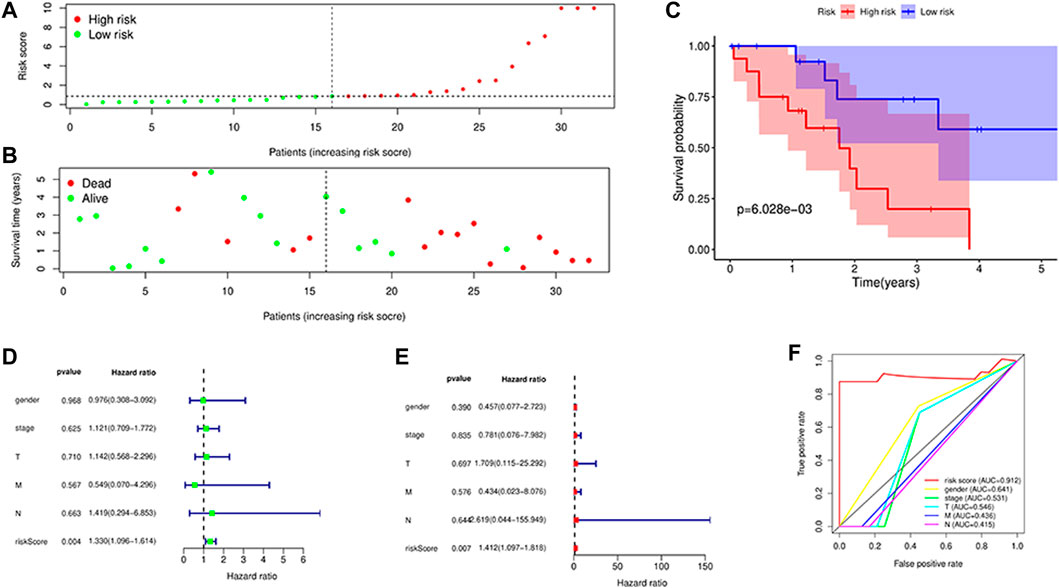
FIGURE 7. Survival analysis. (A,B): Distributions of risk scores and survival status, Survival was longer in the low-risk group than in the high-risk group; (C): Kaplan–Meier curves for the High risk and low risk patients. The survival curve showed low survival in the high-risk group; (D,E): Univariate (D) and multivariate (E) Cox regression analysis in the simultaneously demonstrated the independent prognostic value of the risk score; (F): ROC curves for predicting risk score, gender,stage,T, M, N stage.
Discussion
Cholangiocarcinoma is a common primary liver malignancy worldwide, and its incidence is increasing year by year, seriously endangering the life and health of patients (Florio et al., 2020). Because its early pathogenesis is complex and difficult to detect, patients are often in the middle and late stages once diagnosed, and the prognosis of patients is poor. As one of the common subtypes of cholangiocarcinoma, iCCA also has the characteristics of high metastasis and high invasion (Moeini et al., 2016). Therefore, further search for biological prognostic markers of iCCA is conducive to further understanding the pathogenesis of iCCA and providing new ideas for the development of new treatment methods.
With the in-depth study of lncRNAs, we have gained new understanding of cancer. In cancer, abnormal expression of lncRNAs determines disease progression, explains patient resistance, and can predict patient prognosis (Bester et al., 2018; Zhang W. et al., 2019). Current evidence suggests that H19 is overexpressed in prostate cancer, glioblastoma, and breast cancer and is associated with cancer progression (Bauderlique-Le Roy et al., 2015; Jiang et al., 2016; Peng F. et al., 2017). High expression of HIF2PUT is a marker of aggressive osteosarcoma, and overexpression of HIF2PUT is associated with an extremely poor prognosis in patients (Li W. et al., 2016). At the same time, more and more evidences indicate that m6A is related to tumor proliferation, differentiation, invasion and metastasis (Cui et al., 2017; Kwok et al., 2017). And it has been deeply studied in a variety of solid tumors (Chen et al., 2019). Therefore, the study of m6A related lncRNA is of great significance for the occurrence, development and treatment of iCCA.
In this study, the gene expression map data of iCCA in the TCGA database was used to separate the mRNA and lncRNAs in the gene matrix file by using Perl software, and the lncRNAs gene expression matrix file of iCCA was obtained, and aggregated by WGCNA. Class analysis to further screen out differentially expressed lncRNAs in normal and iCCA patients. After correlation analysis of m6A-related genes, lncRNAs with differential expression in m6A-related genes were further obtained. By drawing a Venn diagram to take the intersection of the two, taking the lncRNAs with higher weights in the network, and analyzing them in the LncSEA database, it is found that the overexpression of lncRNAs co-expressed with m6A is likely to cause the deterioration of cholangiocarcinoma. The above lncRNAs were screened by univariate Cox regression, LASSO method screening and multivariate Cox regression method, and obtained four lncRNAs related to the prognosis of patients with intrahepatic cholangiocarcinoma, namely AC015917.2, AC010735.2, LINC02160, AC009063.3. Based on this, a prognostic model was established, and then the prognosis was predicted according to the risk score. The risk score calculated by these four m6A-related lncRNAs as risk signals can relatively accurately predict the prognosis of patients with intrahepatic cholangiocarcinoma, and is an independent prognosis factor for patients with iCCA.
In conclusion, we identified four m6A-related lncRNAs with potential prognostic value in iCCA and developed a prognostic. They may be applied in the determination of the treatment efficacy in patients with iCCA. However, this study also has certain limitations. The data analyzed are all from the TCGA database, and the data analysis results may be biased. A large amount of relevant data information of iCCA patients is still needed to verify the predictive power of this prognostic model. Therefore, in the follow-up study, we will continue to collect the relevant data of iCCA patients and their survival information, and combine the conclusions of this paper to conduct further clinical validation of the screened related genes and the constructed prognostic model.
Data availability statement
Publicly available datasets were analyzed in this study. This data can be found here: https://portal.gdc.cancer.gov/repository.
Author contributions
GS, JW, MW, and XZ conceived and design the work and wrote the article. GS, JW, WW, MC, XL, YZ, and YF acquired and analysed the data. YF, MW, and XZ provided the comments. All of the authors have read and approved the manuscript.
Funding
This work was supported by grants from the Chinese National Natural Science Foundation Grant (81872417), the Open Project of the State Key Laboratory of Genetic Engineering, Fudan University (SKLGE 1906), and the Priority Academic Program Development of Jiangsu Higher Education Institutions of China (PAPD).
Conflict of interest
The authors declare that the research was conducted in the ;absence of any commercial or financial relationships that could be construed as a potential conflict of interest.
Publisher’s note
All claims expressed in this article are solely those of the authors and do not necessarily represent those of their affiliated organizations, or those of the publisher, the editors and the reviewers. Any product that may be evaluated in this article, or claim that may be made by its manufacturer, is not guaranteed or endorsed by the publisher.
References
Bauderlique-Le Roy, H., Vennin, C., Brocqueville, G., Spruyt, N., Adriaenssens, E., and Bourette, R. P. (2015). Enrichment of human stem-like prostate cells with s-SHIP promoter activity uncovers a role in stemness for the long noncoding RNA H19. Stem Cells Dev. 24, 1252–1262. doi:10.1089/scd.2014.0386
Bester, A. C., Lee, J. D., Chavez, A., Lee, Y. R., Nachmani, D., Vora, S., PANDOLFI, P. P., et al. 2018. An integrated genome-wide CRISPRa approach to functionalize lncRNAs in drug resistance. Cell, 173, 649-+.doi:10.1016/j.cell.2018.03.052
Bhan, A., Soleimani, M., and Mandal, S. S. (2017). Long noncoding RNA and cancer: A new paradigm. Cancer Res. 77, 3965–3981. doi:10.1158/0008-5472.Can-16-2634
Chen, J. X., Zhang, J., Gao, Y., Li, Y. Y., Feng, C. C., Song, C., et al. (2021). LncSEA: A platform for long non-coding RNA related sets and enrichment analysis. Nucleic Acids Res. 49, D969–D980. doi:10.1093/nar/gkaa806
Chen, X. Y., Zhang, J., and Zhu, J. S. (2019). The role of m(6)A RNA methylation in human cancer. Mol. Cancer 18. doi:10.1186/s12943-019-1033-z
Cox, D. R. (1972). Regression models and life-tables. J. R. Stat. Soc. Ser. B-Statistical Methodol. 34, 187. doi:10.1111/j.2517-6161.1972.tb00899.x
Cui, Q., Shi, H. L., Ye, P., Li, L., Qu, Q. H., Sun, G. Q., et al. (2017). m(6)A RNA methylation regulates the self-renewal and tumorigenesis of glioblastoma stem cells. Cell Rep. 18, 2622–2634. doi:10.1016/j.celrep.2017.02.059
Deng, X. L., Su, R., Weng, H. Y., Huang, H. L., Li, Z. J., and Chen, J. J. (2018). RNA N-6-methyladenosine modification in cancers: Current status and perspectives. Cell Res. 28, 507–517. doi:10.1038/s41422-018-0034-6
Feinberg, A. P. (2018). The Key role of epigenetics in human disease prevention and mitigation. N. Engl. J. Med. 378, 1323–1334. doi:10.1056/NEJMra1402513
Florio, A. A., Ferlay, J., Znaor, A., Ruggieri, D., Alvarez, C. S., Laversanne, M., et al. (2020). Global trends in intrahepatic and extrahepatic cholangiocarcinoma incidence from 1993 to 2012. Cancer 126, 2666–2678. doi:10.1002/cncr.32803
Huang, H. L., Weng, H. Y., and Chen, J. J. (2020). m(6)A modification in coding and non-coding RNAs: Roles and therapeutic implications in cancer. Cancer Cell 37, 270–288. doi:10.1016/j.ccell.2020.02.004
Huang, J. H. Z., and Liu, L. X. (2006). Polynomial spline estimation and inference of proportional hazards regression models with flexible relative risk form. Biometrics 62, 793–802. doi:10.1111/j.1541-0420.2005.00519.x
Huang, Y. (2018). The novel regulatory role of lncRNA-miRNA-mRNA axis in cardiovascular diseases. J. Cell. Mol. Med. 22, 5768–5775. doi:10.1111/jcmm.13866
Jiang, X. C., Yan, Y. K., Hu, M. H., Chen, X. D., Wang, Y. X., Dai, Y., et al. (2016). Increased level of H19 long noncoding RNA promotes invasion, angiogenesis, and sternness of glioblastoma cells. J. Neurosurg. 124, 129–136. doi:10.3171/2014.12.Jns1426
Kato, M., and Natarajan, R. (2019). Epigenetics and epigenomics in diabetic kidney disease and metabolic memory. Nat. Rev. Nephrol. 15, 327–345. doi:10.1038/s41581-019-0135-6
Kwok, C. T., Marshall, A. D., Rasko, J. E. J., and Wong, J. J. L. (2017). Genetic alterations of m(6)A regulators predict poorer survival in acute myeloid leukemia. J. Hematol. Oncol. 10. doi:10.1186/s13045-017-0410-6
Langfelder, P., and Horvath, S. (2008). Wgcna: an R package for weighted correlation network analysis. Bmc Bioinforma. 9. doi:10.1186/1471-2105-9-559
Li, B., Cairns, J. A., Robb, M. L., Johnson, R. J., Watson, C. J. E., Forsythe, J. L., et al. (2016a). Predicting patient survival after deceased donor kidney transplantation using flexible parametric modelling. Bmc Nephrol. 17. doi:10.1186/s12882-016-0264-0
Li, W., He, X. J., Xue, R. L., Zhang, Y., Zhang, X. Q., Lu, J. R., et al. (2016b). Combined over-expression of the hypoxia-inducible factor 2 alpha gene and its long non-coding RNA predicts unfavorable prognosis of patients with osteosarcoma. Pathology Res. Pract. 212, 861–866. doi:10.1016/j.prp.2016.06.013
Massarweh, N. N., and El-Serag, H. B. (2017). Epidemiology of hepatocellular carcinoma and intrahepatic cholangiocarcinoma. Cancer control. 24. doi:10.1177/1073274817729245
Mayo, S., Benito-Leon, J., Pena-Bautista, C., Baquero, M., and Chafer-Pericas, C. (2021). Recent evidence in epigenomics and proteomics biomarkers for early and minimally invasive diagnosis of alzheimer's and Parkinson's diseases. Curr. Neuropharmacol. 19, 1273–1303. doi:10.2174/1570159x19666201223154009
Meyer, K. D., Saletore, Y., Zumbo, P., Elemento, O., Mason, C. E., and Jaffrey, S. R. (2012). Comprehensive analysis of mRNA methylation reveals enrichment in 3 ' UTRs and near stop codons. Cell 149, 1635–1646. doi:10.1016/j.cell.2012.05.003
Moeini, A., Sia, D., Bardeesy, N., Mazzaferro, V., and Llovet, J. M. (2016). Molecular pathogenesis and targeted therapies for intrahepatic cholangiocarcinoma. Clin. Cancer Res. 22, 291–300. doi:10.1158/1078-0432.Ccr-14-3296
Panneerdoss, S., Eedunuri, V. K., Yadav, P., Timilsina, S., Rajamanickam, S., Viswanadhapalli, S., et al. (2018). Cross-talk among writers, readers, and erasers of m(6)A regulates cancer growth and progression. Sci. Adv. 4. doi:10.1126/sciadv.aar8263
Peng, F., Li, T. T., Wang, K. L., Xiao, G. Q., Wang, J. H., Zhao, H. D., et al. (2017a). H19/let-7/LIN28 reciprocal negative regulatory circuit promotes breast cancer stem cell maintenance. Cell Death Dis. 8. doi:10.1038/cddis.2016.438
Peng, W. X., Koirala, P., and Mo, Y. Y. (2017b). LncRNA-mediated regulation of cell signaling in cancer. Oncogene 36, 5661–5667. doi:10.1038/onc.2017.184
Rinn, J. L., Kertesz, M., Wang, J. K., Squazzo, S. L., Xu, X., Brugmann, S. A., et al. (2007). Functional demarcation of active and silent chromatin domains in human HOX loci by Noncoding RNAs. Cell 129, 1311–1323. doi:10.1016/j.cell.2007.05.022
Rizvi, S., Khan, S. A., Hallemeier, C. L., Kelley, R. K., and Gores, G. J. (2018). Cholangiocarcinoma - evolving concepts and therapeutic strategies. Nat. Rev. Clin. Oncol. 15, 95–111. doi:10.1038/nrclinonc.2017.157
Roundtree, I. A., and He, C. (2016). RNA epigenetics - chemical messages for posttranscriptional gene regulation. Curr. Opin. Chem. Biol. 30, 46–51. doi:10.1016/j.cbpa.2015.10.024
Satpathy, A. T., and Chang, H. Y. (2015). Long noncoding RNA in hematopoiesis and immunity. Immunity 42, 792–804. doi:10.1016/j.immuni.2015.05.004
Scholler, E., Weichmann, F., Treiber, T., Ringle, S., Treiber, N., Flatley, A., et al. (2018). Interactions, localization, and phosphorylation of the m(6)A generating METTL3-METTL14-WTAP complex. Rna 24, 499–512. doi:10.1261/rna.064063.117
Shindoh, J., and Vauthey, J. N. (2014). Staging of biliary tract and primary liver tumors. Surg. Oncol. Clin. N. Am. 23, 313. doi:10.1016/j.soc.2013.11.003
Xu, F., Huang, X. L., Li, Y. Y., Chen, Y. S., and Lin, L. (2021). m(6)A-related lncRNAs are potential biomarkers for predicting prognoses and immune responses in patients with LUAD. Mol. Therapy-Nucleic Acids 24, 780–791. doi:10.1016/j.omtn.2021.04.003
Xu, K. W., Mo, Y. C., Li, D., Yu, Q. M., Wang, L., Lin, F. H., et al. (2020). N-6-methyladenosine demethylases Alkbh5/Fto regulate cerebral ischemia-reperfusion injury. Ther. Adv. Chronic Dis. 11. doi:10.1177/2040622320916024
Yue, H., Nie, X. J., Yan, Z. G., and Song, W. N. (2019). N6-methyladenosine regulatory machinery in plants: Composition, function and evolution. Plant Biotechnol. J. 17, 1194–1208. doi:10.1111/pbi.13149
Zhang, C. Y., Fu, J. R., and Zhou, Y. F. (2019a). A review in research progress concerning m6A methylation and immunoregulation. Front. Immunol. 10. doi:10.3389/fimmu.2019.00922
Zhang, K., Qin, X. Y., Wen, P. J., Wu, Y. H., and Zhuang, J. (2021). Systematic analysis of molecular mechanisms of heart failure through the pathway and network-based approach. Life Sci. 265. doi:10.1016/j.lfs.2020.118830
Keywords: intrahepatic cholangiocarcinoma, N6-methyladenosine, long non-coding RNA, gene co-expression network, prognostic signature
Citation: Shi G, Wang J, Wang W, Chen M, Liu X, Zheng Y, Fu Y, Wang M and Zhang X (2022) Prognostic analysis of m6A-related lncRNAs as potential biomarkers in intrahepatic cholangiocarcinom. Front. Genet. 13:982707. doi: 10.3389/fgene.2022.982707
Received: 30 June 2022; Accepted: 10 August 2022;
Published: 08 September 2022.
Edited by:
Jia Meng, Xi’an Jiaotong-Liverpool University, ChinaReviewed by:
Kunqi Chen, Fujian Medical University, ChinaBowen Song, University of Liverpool, United Kingdom
Copyright © 2022 Shi, Wang, Wang, Chen, Liu, Zheng, Fu, Wang and Zhang. This is an open-access article distributed under the terms of the Creative Commons Attribution License (CC BY). The use, distribution or reproduction in other forums is permitted, provided the original author(s) and the copyright owner(s) are credited and that the original publication in this journal is cited, in accordance with accepted academic practice. No use, distribution or reproduction is permitted which does not comply with these terms.
*Correspondence: Minghua Wang, mhwang@suda.edu.cn; Xiaojie Zhang, xiaojiezhang@suda.edu.cn
†These authors have contributed equally to this work and share first authorship