- 1Digital Wellness Lab, Division of Adolescent/Young Adult Medicine, Boston Children’s Hospital, Boston, MA, United States
- 2Harvard Medical School, Boston, MA, United States
- 3Harvard T.H. Chan School of Public Health, Boston, MA, United States
Concern has been raised over parallel increases in youth depression and online interactive media use over the past two decades. The aim of this study was to determine whether online interactions are associated with users’ affective states. Using ecological momentary assessment, we measured depressed adolescents’ momentary affect during and residual feelings following online interactions with offline friends and family, online friends, and acquaintances/strangers. We found that depressed adolescents use texting services and social networking sites to interact online, most frequently with offline friends and family, followed by online friends. Results of generalized estimating equations showed associations between negative affect and digital interactions with offline friends and family. Participants were less likely to report feeling better after interacting with online friends than after interacting with any other relationship type. Our findings highlight the heterogeneity of depressed adolescents’ online interactions and suggest that their affective experience varies depending on the nature of the relationships they have with those with whom they interact.
Introduction
Media Use and Psychological Well-Being
Increases in daily interactions on digital platforms and in the prevalence of depression have raised concerns about possible associations between media use and adolescents’ mental health. On average, teenagers spend seven and half hours per day online (Rideout and Robb 2019), and nearly two-thirds report spending time online with friends (Anderson and Jiang 2018a). In 2019, 3.8 million teenagers reported experiencing at least one major depressive episode (Substance Abuse and Mental Health Services Administration 2020). Youth suffering from depressive symptoms spend more time using media than their peers (Primack et al., 2011), highlighting the importance of investigating the media use patterns of this especially vulnerable population to better understand how contemporary uses of media serve as risk or protective factors for psychological well-being.
For almost two decades, researchers have examined the association between depression and the use of Social Networking Sites (SNS), finding inconsistent evidence of negative, positive and mixed effects (Best, Manktelow, and Taylor 2014; Hoge, Bickham, and Cantor 2017; Vidal et al., 2020) with a recent meta-analysis finding a small, but significant association between SNS use and increase in depressive symptoms (Odgers and Jensen 2020). Prior literature often associates increases in depressive symptoms with increases in media use or exposure. For example, some suggest based on social comparison theory that the viewing of TV shows and movies, and exposure to SNS provide opportunities for social comparison, leading to dissatisfaction with oneself and increases in negative affect (Nesi and Prinstein 2015; van den Berg et al., 2007; Vogel et al., 2015; Yoon et al., 2019). Researchers acknowledge, however, that depression may precede and motivate SNS use, or that vulnerable youth may engage with interactive media in maladaptive ways that have been associated with increases in depressive symptoms (Raudsepp and Kais 2019). Conversely, youth suffering from depressive symptoms may turn to interactive media as a respite to cope with negative emotions. They may seek out humorous or uplifting content to mitigate negative feelings, increase positive affect, and potentially alleviate depressive symptoms (Morgan and Jorm 2008; Rideout, Fox, and Trust 2018). Perhaps more effectively, youth may use social media or texting to connect with friends, family, and peers with similar experiences (Lerman et al., 2017), seeking online social connection and support that can serve as a protective factor against depressive symptoms (Frison and Eggermont 2015; Seabrook, Kern, and Rickard 2016).
Employing a person-specific, experience sampling method, recent research has found varying associations between social media use and affect across individuals, with no associations in some adolescents and associations with positive or negative affect in others (Beyens et al., 2020; Valkenburg et al., 2021). Variations found in associations between social media use and affect may be attributable to differential susceptibility of individual adolescents and to differences in individuals’ use of social media. Those with whom an adolescent is interacting and the nature of the interaction can influence the user’s affective state, including their depressive symptoms. More detailed research is necessary to reveal what characteristics of individual youth and what features of online interactions influence associations between social media use and mental health.
Differences in User Experiences and Effects on Mental Health
Two proposed hypotheses, the rich-get-richer hypothesis and the social compensation hypothesis (Kraut et al., 2002), propose different mechanisms by which personality and social behaviors shape the online interactions young people experience and their subsequent impact. The rich-get-richer hypothesis proposes that extroverted, socially adept individuals who have established social networks and strong social ties use online communication to increase social interactions and benefit from associated improved well-being. The social compensation hypothesis suggests that socially anxious or introverted youth who have difficulty forming real-life relationships with peers benefit by using online communication to compensate for a lack of offline social networks. While the majority of research tends to support the rich-get-rich hypothesis (e.g., Khan et al., 2016), some findings indicate that social compensation may be more applicable to youth experiencing symptoms of depression (Desjarlais and Willoughby 2010; Valkenburg and Peter 2007). Considering that social anxiety and loneliness are linked to depression (Qualter et al., 2010; Stein et al., 2001) and that depressed youth have higher levels of online media use (Primack et al., 2011), it is possible that youth with depression are using social media to compensate for limitations in their face-to-face social resources. Further research is needed to better understand how depressed adolescents use online communication to facilitate social interactions and whether and to what extent they use interactive media to cope with depressive symptoms.
The extent to which adolescents develop new relationships or foster existing ones online may contribute to the impact of their interactive media use on their affect and mental health. According to a 2015 Pew Research Center report (Lenhart 2015), adolescents are increasingly establishing online friendships that do not transition out of the digital space. Depressed and other at-risk youth, in particular, seek community and social support via online platforms (Frison and Eggermont 2015). This is in part because perceived social support, including online relationships, can provide protection against depressive symptoms (Frison and Eggermont 2016). Despite this protective quality and shift in the dynamics of social relationships to the online environment, few studies have investigated how these types of online relationships influence adolescent mental well-being. One study examined conjoint, exclusively online, and exclusively offline relationships, finding a positive association between exclusively online relationships and increased self-esteem among shy adolescents (Zalk et al., 2014). Another found that online only friends offer protective benefits against suicidal ideation (Massing-Schaffer et al., 2020). While this research demonstrates that disparate, online relationships may influence mental health and well-being in different ways, more detailed and precise measurement than self-report and focus groups is needed to map the directionality, severity, and timing of fluctuations in individuals' affective states in relation to their social media use.
Capturing Differences Through Ecological Momentary Assessment
Given the heterogeneity of individual digital experiences and fluctuations in depressive affect, ecological momentary assessment (EMA), a method that collects real-time momentary data from participants in their natural environments, may be especially effective at assessing associations between media use and mental health (Bickham, Hswen, and Rich 2015; Shiffman et al., 1997; Solhan et al., 2009). Prior studies using this method have found that Facebook use predicted negative shifts in momentary affect and life-satisfaction (Kross et al., 2013), and have found positive associations between major depressive disorder and exposure to popular music (Primack et al., 2011). These findings, however, were based on a single social media platform or general media use, which may obscure mental health effects that are influenced by specific types of user experiences (e.g., whom a user is communicating with). Similarly, recent studies have used EMA to assess the relationship between mental health symptoms and the amount of time spent daily on technology (George et al., 2018; Jensen et al., 2019), but do not examine characteristics of media use that may be driving their findings. Given that recent work using experience sampling and an N = 1 approach (Beyens et al., 2020; Valkenburg et al., 2021) has identified varying associations between mental health and social media across individuals, and that different types of online relationships may afford varying protection against depressive symptoms, it is important to explore with whom young people are communicating online and how interacting with different types of people can shape the effects of social media use.
EMA can ask detailed questions in real time, such as how and with whom users are communicating, and the qualities of their current affect and residual feeling following an event. Such ephemeral details are critically important to social media users’ experiences and immediate affective state, but are easily lost to social desirability or recall bias by methods that do not collect data in the moment. To the best of our knowledge, no previous studies have used EMA to examine individuals’ interactive media use with different types of interaction partners and affective states among adolescents at risk for depression.
Current Study
This study explores depressed adolescents’ online social media interactions with different types of communication partners in relation to their positive and negative affective states. Sampling random moments throughout their day, we observed individuals’ digital interactions with offline friends and family, online friends, and acquaintances and strangers and assessed their momentary affect in relation to their communication partners. Our aim was to address the following research questions:
• With whom do depressed adolescents interact online and through what platforms?
• Do adolescents’ affective states vary with their relationship to the person with whom they connect online?
• What changes in their affective states do adolescents report after they have interacted with different types of people?
Methods
Participants and Recruitment
Adolescents aged 14–19 years were recruited from an urban adolescent medicine practice in Boston, MA, from August 2016 through March 2018. Using a recruitment protocol established previously by the practice, age-eligible patients who were not in the clinic for sensitive or confidential procedures were approached. To ensure patient privacy, these patients were asked to read a brief description of the study that detailed patient eligibility criteria, including the wording “currently experiencing symptoms of sadness,” and were asked if the criteria applied to them. Interested patients who self-identified as sad reviewed and signed an informed consent if they were 18 or 19 years of age; for patients under the age of 18 years, parental/legal guardian consent was obtained. Potential participants completed the Patient Health Questionnaire-9 (PHQ-9) depression screener (Kroenke, Spitzer, and Williams 2001) (a second PHQ-9 was completed after the EMA procedure, but this score was not used for this study). Patients who reported at least mild depressive symptoms (a PHQ-9 score of 5 or higher) were invited to participate in the study. Of 66 screened patients, 8 did not meet eligibility criteria. For patients who reported any non-zero response on the suicidality question (n = 12), a safety procedure was followed that included paging an on-call mental health provider. Informed consent and all study procedures were approved by the Boston Children’s Hospital IRB.
Procedure
Enrollment session. Enrolled participants downloaded MetricWire app (MetricWire 2020) onto their smartphones to deliver the EMA procedure and administer a baseline questionnaire. Three participants did not own a smartphone and were loaned an iPod Touch (with all non-study functionality disabled) for the duration of the study. The 189-item baseline questionnaire collected demographic information, as well as additional measures not used in the current study. Participants were compensated $10 in cash for completing the enrollment survey.
EMA procedure. The day following the enrollment session, participants began a 7-day data collection procedure in which they completed up to 5 EMAs a day, delivered at random times to their phones with a minimum of 30 min between each EMA signal. Metricwire was programmed to deliver EMA signals between the hours of 8AM-10PM for participants not attending school and from 6AM to 8AM and 3–10PM for those who were, in order to avoid disrupting their school day. If participants did not respond to a signal within 10 min, a reminder push notification was automatically sent. Following standard EMA protocol (Shrier et al., 2007), if participants did not complete the EMA within 5 min of the reminder, the EMA expired and was no longer available to the participant. Participants received up to $30 compensation in the form of a gift card based on the percentage of EMAs they completed.
EMA Measures. Three subscales of the Positive and Negative Affect Schedule expanded form (PANAS-X) were used to assess participants’ momentary affect (Watson and Clark 1994). The PANAS-X assesses general positive affect and negative affect via 10 items each, and sadness with 5 items. Positive affect includes items such as attentiveness and enthusiasm; negative affect includes irritability and being upset; and sadness, which is most like momentary symptoms of depression, includes being sad, lonely, and blue. When signaled, participants rated the extent to which they felt each affect item in the moment on a 5-point Likert scale from “very slightly or not at all” to “extremely.” In this study, the three subscales demonstrated a high level of internal reliability (Cronbach’s Alpha for positive affect, negative affect, and sadness = 0.85, 0.95, 0.93, respectively).
Participants were next asked to indicate which types of media they were using when signaled (TV, video game console, computer/laptop, cell phone, tablet, stereo/radio, or none of the above). For each device selected, participants were asked to select what they were doing on the device (playing a video game, using social media, checking an online discussion board, messaging, video chatting, voice calling, watching a video, listening to music/radio, schoolwork, shopping, other- write in option) and whether they were communicating with someone while on it. If participants indicated they were using social media, they were asked to identify the platform they were using. When participants indicated yes to communicating with another user, they were asked whether the person with whom they were interacting was a friend whom they only know online (online friend), friend whom they also know offline (offline friend), a family member, an acquaintance, or a stranger. For purposes of analyses, we created categories of relationships that existed entirely online (online friends), were established offline (offline friends/family), and were more distant (acquaintances/strangers). Finally, participants were asked how they felt after their interaction (better, same, or worse).
Accounting for Multiple Communication Partners and Feeling Responses
Individual moments in which communication occurred were categorized according to the type of communication partner: online friends, offline friends/family, acquaintances/strangers, or a mix of these relationship types (e.g., texting on a phone with online friends and an offline family member during the same moment). The majority of EMAs fell into one of these categories. However, because participants could select more than one media type or platform through which they were communicating per EMA survey, some EMAs (n = 23, 11.8%) fell into multiple categories. We resolved these responses by characterizing the moment according to the most frequently reported relationship type, given that a higher occurrence of interactions with a specific type of person may be more strongly associated with the participants’ affective state. For example, a participant might have reported that at the moment they were signaled, they were texting on their phone with an offline friend, responding on social media to an offline friend, and using their computer to message with a relative stranger. This moment would be categorized as offline friend/family because two out of three communications were with an offline friend. There were 5 EMAs (3%) that fell into equal categories of relationship types and were, therefore, categorized as “mixed.” Similarly, since multiple interactions could be reported per EMA, it was possible for participants to report a different affective reaction to each. In 1.2% of EMA responses (n = 2), participants reported multiple affective states and were characterized by the most frequently reported affect. In 5 surveys (3%), participants reported multiple affective states with equal frequency. These moments were excluded from analysis.
Analysis
Generalized Estimating Equations (GEE) were used to determine whether momentary reports of positive affect, negative affect, or sadness differ when adolescents are interacting online with different types of communication partners (online friends, offline friends/family, acquaintances/strangers, or a mix of these relationship types). Separate analyses were conducted for each type of relationship so that results compare affect during moments with the included communication partner type with affect during moments with any other partner type. A similar approach was used to ascertain if participants were more likely to report feeling better (vs. feeling no change) after an interaction with each type of communication partner, but using a logit link function (rather than an identity function) to account for the binary nature of the dependent variable. GEE is a modeling approach that allows for the specification of a working correlation structure and can, therefore, account for different types of relationships among data points and has regularly been used with EMA data (Schwartz and Stone 1998; Shiffman, Stone, and Hufford 2008) including those studying adolescent and adult affect and health behaviors (Shrier, Ross, and Blood 2014; Paganini, Peterson, and Mills 2019). The quasilikelihood under the independence model criterion (QIC) is a goodness of fit statistic that can be calculated for GEE models and used to select the most appropriate correlation structure. QICs from identically specified GEE models using different correlation structures can be compared and the model having the lowest QIC considered the best (Cui 2007). Using this procedure we chose to use the identity structure (in which the data are assumed to be uncorrelated) as it resulted in the lowest QIC across our models. When modeled with the exchangeable correlation matrix that assumes equal correlations across observations within participant, the estimated correlations ranged from approximately 0.01 to .23. These are below the point at which the inefficiency of regression coefficients becomes most apparent (0.4) (Ballinger 2004). Analyses were conducted via IBM SPSS Statistics for Windows, Version 24.0 and controlled for participant age, gender, race/ethnicity, PHQ-9 score pre EMA data collection, and EMA response rate.
Results
Sample Characteristics. Fifty-eight participants were enrolled in the study. Of those, 56 provided usable data. The sample was predominately female (67.3%), and racially/ethnically diverse with an approximately even split between White, Black, and Hispanic participants (Table 1). Participants had a mean PHQ-9 score of 11.27 (moderately depressed) and responded to 49% of the EMA signals.
Descriptions of Interaction Moments. In 57.1% (n = 558) of EMAs, participants reported using media, and of those, 35.1% (n = 196) reported communication with another person using interactive media. Of EMAs reporting communication, 45.4% were with offline friends and family members, 24.5% were with online friends, 5.6% were with acquaintances/strangers, and 24.5% were with a mix of these relationship types. In 34.2% of EMA responses, participants reported using more than one type of interactive media.
Of the 56 participants in the study, 25.0% reported zero moments of communicating with another media user. For participants who reported at least one moment of communication, the majority (48.2%) reported communicating with a variety of relationship types, while 21.4% reported only communicating with offline friends or family, 3.6% communicated with online only friends, and 1.8% reported only communicating with acquaintances/strangers.
When conversing with acquaintances/strangers, 43.6% of interactions happened on social media platforms accessed on their phones, with most of these moments happening on Snapchat (52.9%) and Instagram (35.3%). The second most common form of interaction with acquaintances/strangers happened via text messaging on participants’ phones (25.6%). In comparison, when interacting with online friends and offline friends/family, participants reported most frequently doing so through text messaging on their phone (39.1 and 41.2%, respectively), followed by social media platforms on their phone (31.2 and 25%, respectively).
In terms of social media platforms, 51.1% of interactions with online friends happened via Snapchat, followed by nearly equal reports of Facebook (25.6%) and Instagram (23.2%). When communicating with offline friends/family, Snapchat was also the most frequently reported platform (50.0%), followed by Facebook (31.5%).
Affect During Interactions with Different Relationship Types. Table 2 presents the GEE results of analyses examining the associations between momentary affect and relationship type. Participants reported higher levels of negative affect during moments when they were communicating with an offline friend or family member vs moments of communication with other relationship types. However, no moment of communication with any relationship type was related to either momentary positive affect or sadness.
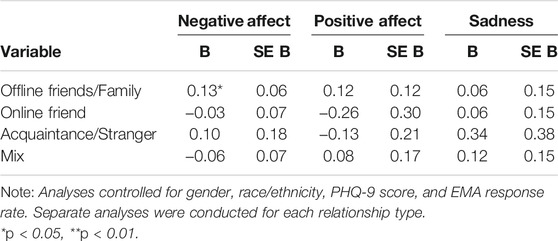
TABLE 2. Generalized Estimating Equation (GEE) model predicting momentary affect by relationship type.
Feelings After Interactions with Different Relationship Types. Of the moments in which participants reported how they felt after communicating with another media user (n = 162), 48.1% of the time participants reported feeling better, 46.9% of the time participants reported feeling the same, and 4.9% of the time participants reported feeling worse. When communicating with offline friends and family, participants reported feeling better 57.3% of the time, feeling the same 38.7% of the time, and feeling worse 4% of the time, vs. 27.5% better, 70% the same, 2.5% worse when communicating with online friends, and 44.4% better, 33.3% the same, and 22.2% worse when communicating with acquaintances/strangers (communication with acquaintances/strangers happened infrequently, 11 EMA responses). Because of the very few moments of feeling worse, logistic GEE were used to determine whether interacting with certain relationship types was related to feeling better compared to feeling the same following the interactions. Results are presented in Table 3. When participants were interacting with online friends vs. moments of communication with other relationship types, they were less likely to report feeling better after the interaction.
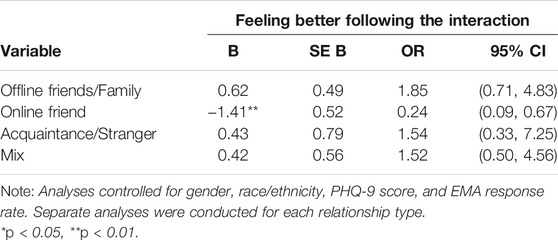
TABLE 3. Generalized Estimating Equation (GEE) model predicting feeling better after interaction by relationship type.
Discussion
We examined depressed adolescents’ online interactions in real time to determine whether and how their momentary affect and residual feelings vary with the nature of the relationships they have with their communication partner. We found that, among the participants who reported online interactions, nearly half of the interactions occurred with offline friends and family, a quarter were with online friends, and the other quarter were with a mix of relationship types. Reports of communication with acquaintances and strangers were infrequent (5.6%). The two platforms most frequently used for online interaction were texting and SNS. We found a positive association between communications with offline friends/family and negative affect, suggesting that adolescents are experiencing negative emotions more often during moments of online interaction with people with whom they have pre-existing social ties than with other types of communication partners. We also found that when compared to all other interactions, adolescents were less likely to report feeling better after interacting with online friends. These results suggest that when interacting online, young people’s affective experience varies depending on the relationships they have with their communication partners.
Interaction With Offline Friends and Family
For adolescents experiencing depressive symptoms, digitally communicating with offline friends and family was associated with higher negative affect, differing from prior literature with a healthy sample suggesting that digital interactions with offline friends and/or family may increase social connection and build stronger relationships (Burke, Kraut, and Marlow 2011; Reich, Subrahmanyam, and Espinoza 2012). Negative affect may have been influenced by social pressures experienced by adolescents interacting online, including peer rejection, normative conformity, and “teen drama” (Nesi, Choukas-Bradley, and Prinstein 2018), which are more likely to occur in established relationships. Pew Research Center (Anderson and Jiang 2018a) reported that teens spent more time online with their friends than in face-to-face interactions with them. As time spent online increases, so does the potential for peer rejection, a possible contributor to adolescent depression (Platt, Kadosh, and Lau 2013). Fearful of being rejected, adolescents may feel added pressure to normatively conform, including posting only content that makes them look good (Anderson and Jiang 2018b), or oversharing content with peers that is exaggerated or risky (Radovic et al., 2017). For depressed adolescents, observing that others seem to be doing better than they are, and hiding their own vulnerabilities or “acting out” in response to them, may increase negative affect.
When teens are exposed to online drama among their friends, they can experience increased negative affect. Online drama is often related to negative interpersonal interactions (Marwick and boyd 2014) and is defined by teens as “conflict between peers” (Lenhart 2015), with 45% feeling overwhelmed by drama on social media (Anderson and Jiang 2018b). Early research has shown that depressed youth experience dysregulated emotions during stressful peer interactions (Rudolph, Hammen, and Burge 1994), and others have found that adolescent girls, in particular, are more susceptible to depressive symptoms due to increased levels of interpersonal stress (Shih et al., 2006). Since our sample was predominantly female, their experience of online drama among friends could explain our findings. Additional research that directly tests this relationship would further advance our understanding of the type of online interactions that can contribute to depression, anxiety and other mental health issues.
If interactions with offline friends and family were contributing to increased negative affect among our participants, we would expect corroborating results from our measure assessing their feelings immediately following the interactions. This was not the case. Very rarely did adolescents report feeling worse after communicating with offline friends and family (4% of the time). Overall, they reported low rates of feeling worse (4.9% of the time), potentially countering the idea that their depression was contributing to maladaptive behavior, which worsened their depression. In fact, participants reported nearly equal, and cumulatively predominant frequencies of feeling better (48.1%) or not different at all (46.9%) after communicating online. Given that depressed adolescents tend to seek social support from friends and family when distressed, our results may indicate that their negative affect preceded, and possibly motivated, their online interaction with established friends or family.
Previous research has found that when depressed adolescents are asked about the impact of using social media on their depressive symptoms, 30% said it makes them feel better, 22% said it makes them feel worse, and 47% report no difference (Rideout, Fox, and Trust 2018). In context with these self-reported results, our findings seem to point toward a conclusion that depressed teens regularly perceive online communication as having a positive impact on their emotional well-being. However, our respondents reported either negative or no impact on their mood after more than half of their online interactions. Such variability opens the door to new questions about what specific types of interactions may lead to an improvement in users’ affective states. Additional research is necessary to determine how to use interactive media and with whom to interact that may help depressed adolescents feel better in the moment, and whether or not these momentary affective states translate into long term effects on mood.
Since social support from close ties is effective at reducing depressive symptoms (Fredrick et al., 2018), depressed adolescents’ tendency to seek connection with offline friends and family when experiencing higher levels of negative affect is to be expected. However, we found that interacting online with offline friends and family did not increase the likelihood of feeling better following the interaction. This finding, in addition to the lack of positive association between interactions with offline friends and family and positive affect, do not support the rich-get-richer hypothesis, which predicts that reaching out to established strong social ties would lead to improved affect. It is possible that online media interactions with offline family and friends may not be robust enough to provide the support depressed young people are seeking or that expectations of support from friends and family are not met in the online environment because they are attenuated in comparison to face-to-face support. These results are similar to our previous findings in which young adolescents’ cell phone use (primarily texting) was associated with increased symptoms of depression (Bickham, Hswen, and Rich 2015). More research is needed to explore the directionality of our finding and to determine whether and how online interactions with offline family and friends might help adolescents cope with depression.
Interaction With Online Friends
Although the majority of digital interaction moments in our study happened with offline family and friends, one-fourth occurred with online friends, highlighting the increasing number of youth building personal relationships with people they have only “met” online (Lenhart 2015). However, our participants were less likely to report feeling better after interactions with online friends. While outreach to online friends was predicted by the social compensation theory, the lack of positive association between interactions with this relationship type and increased positive affect or feeling better after those interactions that we found suggests that these interactions may not provide effective social compensation for depressed adolescents. This result may be explained by the types of people adolescents chose to befriend online and the interactions that occur within those relationships. Adolescents usually develop online relationships with others who share mutual interests and/or similar personal experiences (Mazur and Richards 2011). Social ties built on casual connections may be less likely to result in strongly positive or negative experiences, thereby leaving participants feeling neither considerably better nor worse after the interaction. More work is necessary to determine the extent to which online friends can serve as effective support for depressed youth.
Interaction With Acquaintances and Strangers
Instances of interactions with acquaintances and strangers did not occur often enough in our study to make generalizations about their impact on mental health. EMA does, however, allow us to describe what moments of interaction with acquaintances and strangers look like for depressed adolescents. It is not surprising that interactions with acquaintances and strangers occurred most frequently on image-based platforms (i.e., Snapchat and Instagram) that feature settings that make communicating with acquaintances and strangers more accessible (Snap, Inc. n.d.; Koch 2016). Texting was the second most frequently used means of online interaction with acquaintances and strangers, indicating that adolescents in our study were willing to exchange their telephone number, a vulnerable personal identifier, with people they do not personally know well (Chen 2019).
There has been long-standing debate about the benefits of applications that allow or encourage adolescents to engage with strangers (Bindley 2020). While some argue that interacting online with unknown partners allows for exploration of self-identity in relative anonymity, others have examined online sexual harassment, cyberbullying and other risky online behaviors, and found them detrimental to psychological well-being (Allen et al. 2014; Uhls, 2017). Conflicting findings require further exploration of potential positive and negative mental health implications of adolescents’ interactions with acquaintances and strangers.
Heterogeneity of Adolescents’ Digital Interactions
Participants reported interacting on multiple device/platforms per single EMA in roughly 34% of responses, highlighting the complexity of a single moment of adolescent online interaction. The ubiquity and mobility of devices allowing simultaneous use of multiple communication platforms increases the heterogeneity and often contradictory nature of these experiences, with media multitasking potentially exacerbating depression and anxiety (Becker, Alzahabi, and Hopwood 2013). Using multiple channels to communicate varying messages to different people nearly simultaneously may alter the influence of each interaction on mental health. Research is needed to determine the types of online interactions that provide robust social support and best foster positive affect and residual positive feelings among young people suffering from depression.
Limitations
Due to its random sampling of moments in a participant’s day, EMA does not capture all online interactions or other experiences that can powerfully influence an adolescent’s affective state. The single item we used to assess feelings after online interactions included only 3 levels and may not have been precise enough or the interval not long enough to capture a change in participants’ affect during the interaction. Although not surprising given the moderate depression experienced by our sample, the EMA response rate (49%) was lower than other studies using EMA to assess adolescents (52–80%) (Bickham et al., 2013; Shrier et al., 2007; Whalen et al., 2001). Given that our convenience sample is small, primarily female, and includes adolescents with at least some level of depression, results should be considered as applicable to the participants in our study alone and caution should be taken when generalizing to any other population.
Conclusion
This study highlights the nuanced heterogeneity of adolescent online interactions. Our findings of the affective experiences of depressed adolescents emphasize the importance of contextualizing online interactions with the intentions and expectations of the adolescent in reaching out and the nature of their relationships with those with whom they interact. It is through understanding the complexities of these interactions that we can begin to provide guidance toward reducing mental illness and promoting digital wellness.
Data Availability Statement
The raw data supporting the conclusions of this article will be made available by the authors, without undue reservation.
Ethics Statement
The studies involving human participants were reviewed and approved by Boston Children’s Hospital Institutional Review Board. Written informed consent to participate in this study was obtained from the participant's legal guardian/next of kin or from the participant when they were 18 years old or older.
Author Contributions
DB and MR conceived and designed this study. SM and DB analyzed the data. All authors contributed to the manuscript and approved the submitted version.
Funding
This study was supported by a Boston Children's Hospital Office of Faculty Development Career Development Fellowship awarded to DB.
Conflict of Interest
The authors declare that the research was conducted in the absence of any commercial or financial relationships that could be construed as a potential conflict of interest.
Publisher’s Note
All claims expressed in this article are solely those of the authors and do not necessarily represent those of their affiliated organizations or those of the publisher, the editors, and the reviewers. Any product that may be evaluated in this article, or claim that may be made by its manufacturer, is not guaranteed or endorsed by the publisher.
Acknowledgments
We thank Jill R. Kavanaugh for bibliographic and editing assistance.
References
Allen, K. A., Ryan, T., GrayGray, D. L, Dennis, M. Mc, McInerney, D. M., and Waters, L. (2014). Social Media Use and Social Connectedness in Adolescents: The Positives and the Potential Pitfalls. Aust. Educ. Developmental Psychol. 31 (1), 18–31. doi:10.1017/edp.2014.2
Anderson, Monica., and Jiang, Jingjing. (2018a). Teens, Social Media & Technology 2018. Pew Research Center Available At: https://www.pewresearch.org/internet/2018/05/31/teens-social-media-technology-2018/.
Anderson, Monica., and Jiang, Jingjing. (2018b). Teens' Social Media Habits and Experiences.. Washington, DC. Pew Research Center. Available At: https://www.pewresearch.org/internet/2018/11/28/teens-social-media-habits-and-experiences/.
Ballinger, G. A. (2004). Using Generalized Estimating Equations for Longitudinal Data Analysis. Organizational Res. Methods 7 (2), 127–150. doi:10.1177/1094428104263672
Becker, M. W., Alzahabi, R., and Hopwood, C. J. (2013). Media Multitasking Is Associated with Symptoms of Depression and Social Anxiety. Cyberpsychology, Behav. Soc. Networking 16 (2), 132–135. doi:10.1089/cyber.2012.0291
Best, P., Manktelow, R., and Taylor, B. (2014). Online Communication, Social Media and Adolescent Wellbeing: A Systematic Narrative Review. Child. Youth Serv. Rev. 41 (June 2014), 27–36. doi:10.1016/j.childyouth.2014.03.001
Beyens, I., Pouwels, J. L., van Drielvan Driel, I. I., Keijsers, L., and Valkenburg, P. M. (2020). The Effect of Social media on Well-Being Differs from Adolescent to Adolescent. Sci. Rep. 10 (1), 10763. doi:10.1038/s41598-020-67727-710.1038/s41598-020-67727-7
Bickham, D. S., Blood, E. A., WallsShrier, C. E. Lydia. A., Shrier, L. A., and Rich, M. (2013). Characteristics of Screen Media Use Associated with Higher BMI in Young Adolescents. Pediatrics 131 (5), 935–941. doi:10.1542/peds.2012-1197
Bickham, D. S., Hswen, Y., and Rich., M. (2015). Media Use and Depression: Exposure, Household Rules, and Symptoms Among Young Adolescents in the USA. Int. J. Public Health 60 (2), 147–155. doi:10.1007/s00038-014-0647-6
Bindley, Katherine. (2020). Don’t Talk to Strangers? These Apps Encourage it. Wall Street J. February 24, 2020, Tech Available At: https://www.wsj.com/articles/dont-talk-to-strangers-these-apps-encourage-it-11582347631.
Burke, M., Kraut, R., and Marlow, C. (2011). Social Capital on Facebook. Proceedings of the Sigchi Conference on Human Factors in Computing Systems. Montréal Québec Canada, April 22 - 27, 2006, Vancouver, BC: Canada. doi:10.1145/1978942.1979023
Chen, Brian. X. (2019). I Shared My Phone Number. I Learned I Shouldn’t Have. The New York Times. Tech Fix Available At: https://www.nytimes.com/2019/08/15/technology/personaltech/i-shared-my-phone-number-i-learned-i-shouldnt-have.html.
Cui, J. (2007). QIC Program and Model Selection in GEE Analyses. Stata J. 7 (2), 209–220. doi:10.1177/1536867X0700700205
Desjarlais, M., and Willoughby, T. (2010). A Longitudinal Study of the Relation between Adolescent Boys and Girls' Computer Use with Friends and friendship Quality: Support for the Social Compensation or the Rich-Get-Richer Hypothesis? Comput. Hum. Behav. 26 (5), 896–905. doi:10.1016/j.chb.2010.02.004
Eline, F., and Steven, E. (2015). Exploring the Relationships between Different Types of Facebook Use, Perceived Online Social Support, and Adolescents’ Depressed Mood. Soc. Sci. Computer Rev. 34 (2), 153–171. doi:10.1177/0894439314567449
Fredrick, S. S., Demaray, M. K., Malecki, C. K., and Dorio, N. B. (2018). Can Social Support Buffer the Association between Depression and Suicidal Ideation in Adolescent Boys and Girls? Psychol. Schs. 55 (5), 490–505. doi:10.1002/pits.22125
Frison, E., and Eggermont, S. (2015). The Impact of Daily Stress on Adolescents' Depressed Mood: The Role of Social Support Seeking through Facebook. Comput. Hum. Behav. 44, 315–325. doi:10.1016/j.chb.2014.11.070
George, M. J., Russell, M. A. Joy. R. Piontak., Piontak, J. R., and Odgers, C. L. (2018). Concurrent and Subsequent Associations between Daily Digital Technology Use and High-Risk Adolescents' Mental Health Symptoms. Child. Dev. 89 (1), 78–88. doi:10.1111/cdev.12819
Hoge, E., Bickham, D., and Cantor, J. (2017). Digital Media, Anxiety, and Depression in Children. Pediatrics 140 (Suppl. 2), S76–S80. doi:10.1542/peds.2016-1758G
Jensen, M., GeorgeGeorgeRussell, M. J. Michael. R., Russell, M. R., and Odgers, C. L. (2019). Young Adolescents' Digital Technology Use and Mental Health Symptoms: Little Evidence of Longitudinal or Daily Linkages. Clin. Psychol. Sci. 7 (6), 1416–1433. doi:10.1177/2167702619859336
Khan, S., Gagné, M., Yang, L., and Shapka, J. (2016). Exploring the Relationship between Adolescents' Self-Concept and Their Offline and Online Social Worlds. Comput. Hum. Behav. 55 (B), 940–945. doi:10.1016/j.chb.2015.09.046
Koch, Lucy. (2016). Most Popular Social Networks of Teenagers in the United States from Fall 2012 to Fall 2019. Available At: https://www.emarketer.com/content/three-charts-on-tiktok-penetration-among-gen-z-users (Accessed October 30.
Kraut, R., Kiesler, S., Boneva, B., Cummings, J., Helgeson, V., and Crawford, A. (2002). Internet Paradox Revisited. J. Soc. Isssues 58 (1), 49–74. doi:10.1111/1540-4560.00248
Kroenke, K., Spitzer, R. L., and Williams, J. B. W. (2001). The PHQ-9. J. Gen. Intern. Med. 16 (9), 606–613. doi:10.1046/j.1525-1497.2001.016009606.x
Kross, E., Verduyn, P., Demiralp, E., Park, J., Lee, D. S., Lin, N., et al. (2013). Facebook Use Predicts Declines in Subjective Well-Being in Young Adults. PLOS One 8 (8), e69841. doi:10.1371/journal.pone.0069841
Lenhart, Amanda. (2015). Teens, Technology and Friendships. Pew Research Center Available At: https://www.pewresearch.org/internet/2015/08/06/teens-technology-and-friendships/.
Lerman, B. I., Lewis, S. P., Lumley, M., Grogan, G. J., Hudson, C. C., and Johnson, E. (2017). Teen Depression Groups on Facebook: A Content Analysis. J. Adolesc. Res. 32 (6), 719–741. doi:10.1177/0743558416673717
Marwick, A., and boyd, d. (2014). 'It's Just Drama': Teen Perspectives on Conflict and Aggression in a Networked Era. J. Youth Stud. 17 (9), 1187–1204. doi:10.1080/13676261.2014.901493
Massing-Schaffer, M., Nesi, J., Telzer, E. H., Lindquist, K. A., and Prinstein, M. J. (2020). Adolescent Peer Experiences and Prospective Suicidal Ideation: The Protective Role of Online-Only Friendships. J. Clin. Child Adolesc. Psychol., 1–12. doi:10.1080/15374416.2020.1750019
Mazur, E., and Richards, L. (2011). Adolescents' and Emerging Adults' Social Networking Online: Homophily or Diversity? J. Appl. Developmental Psychol. 32 (4), 180–188. doi:10.1016/j.appdev.2011.03.001
MetricWire (2020). MetricWire. Available At: http://metricwire.com (Accessed October 30.
Morgan, A. J., and Jorm, A. F. (2008). Self-help Interventions for Depressive Disorders and Depressive Symptoms: a Systematic Review. Ann. Gen. Psychiatry 7 (1), 13. doi:10.1186/1744-859x-7-13
Nesi, J., Choukas-Bradley, S., and Prinstein, M. J. (2018). Transformation of Adolescent Peer Relations in the Social Media Context: Part 2-Application to Peer Group Processes and Future Directions for Research. Clin. Child. Fam. Psychol. Rev. 21 (3), 295–319. doi:10.1007/s10567-018-0262-9
Nesi, J., and Prinstein, M. J. (2015). Using Social Media for Social Comparison and Feedback-Seeking: Gender and Popularity Moderate Associations with Depressive Symptoms. J. Abnorm Child. Psychol. 43 (8), 1427–1438. doi:10.1007/s10802-015-0020-0
Odgers, C. L., and Jensen., M. R. (2020). Annual Research Review: Adolescent Mental Health in the Digital Age: Facts, Fears, and Future Directions. J. Child. Psychol. Psychiatr. 61 (3), 336–348. doi:10.1111/jcpp.13190
Paganini, C., Peterson, Gregory., and Mills, Jacqueline. 2019. "Negative Affect and Situational Antecedents of Eating in Disordered Eating and Normal Population an Ecological Momentary Assessment Study."Journal of Nutritional Biology.4(2):289-297. doi:10.18314/jnb.v4i2.1384
Platt, B., Kadosh, K. C., Lau, J. Y. F., and Lau, F. (2013). The Role of Peer Rejection in Adolescent Depression. Depress. Anxiety 30 (9), 809–821. doi:10.1002/da.22120
Primack, B. A., SilkSilk, J. S., Christian, R. D., William, G. S., Francesca Shadel, C. R., Shadel, W. G., et al. (2011). Using Ecological Momentary Assessment to Determine Media Use by Individuals with and without Major Depressive Disorder. Arch. Pediatr. Adolesc. Med. 165 (4), 360–365. doi:10.1001/archpediatrics.2011.27
Qualter, P., Brown, S. L. Penny. Munn., Munn, P., and Rotenberg, K. J. (2010). Childhood Loneliness as a Predictor of Adolescent Depressive Symptoms: an 8-year Longitudinal Study. Eur. Child. Adolesc. Psychiatry 19 (6), 493–501. doi:10.1007/s00787-009-0059-y
Radovic, A., Gmelin, T., Stein, B. D., and Miller, E. (2017). Depressed Adolescents' Positive and Negative Use of Social Media. J. Adolescence 55 (February 2017), 5–15. doi:10.1016/j.adolescence.2016.12.002
Raudsepp, L., and Kais, K. (2019). Longitudinal Associations between Problematic Social media Use and Depressive Symptoms in Adolescent Girls. Prev. Med. Rep. 15, 100925. doi:10.1016/j.pmedr.2019.100925
Reich, S. M., Subrahmanyam, K., and Espinoza., G. (2012). Friending, Iming, and Hanging Out Face-To-Face: Overlap in Adolescents' Online and Offline Social Networks. Developmental Psychol. 48 (2), 356–368. doi:10.1037/a0026980
Rideout, Vicky., and Robb, Michael. B. (2019). The Common Sense Census: Media Use by Tweens and Teens. Common Sense Media. San Francisco, CA. Available At: https://www.commonsensemedia.org/sites/default/files/uploads/research/2019-census-8-to-18-full-report-updated.pdf.
Rideout, Victoria., Fox, Susannah., and Well Being Trust, (2018). Digital Health Practices, Social Media Use, and Mental Well-Being Among Teens and Young Adults in the U.S." Articles, Abstracts, and Reports.1093 Available At: https://digitalcommons.psjhealth.org/publications/1093.
Rudolph, K. D., Hammen, C., and Burge, D. (1994). Interpersonal Functioning and Depressive Symptoms in Childhood: Addressing the Issues of Specificity and Comorbidity. J. Abnorm Child. Psychol. 22 (3), 355–371. doi:10.1007/BF02168079
Schwartz, J. E., and Stone, A. A. (1998). Strategies for Analyzing Ecological Momentary Assessment Data. Health Psychol. 17 (1), 6–16. doi:10.1037/0278-6133.17.1.6
Seabrook, E. M., Kern, M. L., and Rickard, N. S. (2016). Social Networking Sites, Depression, and Anxiety: A Systematic Review. JMIR Ment. Health 3 (4), e50. doi:10.2196/mental.5842
Shiffman, S., Hufford, M., Hickcox, M., Paty, J. A., Gnys, M., and Kassel, J. D. (1997). Remember that? A Comparison of Real-Time versus Retrospective Recall of Smoking Lapses. J. Consulting Clin. Psychol. 65 (2), 292–300. doi:10.1037/0022-006X.65.2.292.a
Shiffman, S., Stone, A. A., and Hufford, M. R. (2008). Ecological Momentary Assessment. Annu. Rev. Clin. Psychol. 4 (1), 1–32. doi:10.1146/annurev.clinpsy.3.022806.091415
Shih, J. H., Eberhart, N. K., Hammen, C. L., and Brennan, P. A. (2006). Differential Exposure and Reactivity to Interpersonal Stress Predict Sex Differences in Adolescent Depression. J. Clin. Child Adolesc. Psychol. 35 (1), 103–115. doi:10.1207/s15374424jccp3501_9
Shrier, L. A., Ross, C. S., and Blood, E. A. (2014). Momentary Positive and Negative Affect Preceding Marijuana Use Events in Youth. J. Stud. Alcohol. Drugs 75 (5), 781–789. doi:10.15288/jsad.2014.75.781
Shrier, L. A., Shih, M.-C., Hacker, L., and de Moor, C. (2007). A Momentary Sampling Study of the Affective Experience Following Coital Events in Adolescents. J. Adolesc. Health 40 (4), e1–e8. doi:10.1016/j.jadohealth.2006.10.014
Snap (2020). Snapchat Support: Privacy Settings. Available At: https://support.snapchat.com/en-GB/a/privacy-settings2 (Accessed October 30, 2020).
Solhan, M. B., Trull, T. J., Jahng, S., and Wood, P. K. (2009). Clinical Assessment of Affective Instability: Comparing Ema Indices, Questionnaire Reports, and Retrospective Recall. Psychol. Assess. 21 (3), 425–436. doi:10.1037/a0016869
Stein, M. B., Fuetsch, M., Müller, N., Höfler, M., Lieb, R., and Wittchen, H.-U. (2001). Social Anxiety Disorder and the Risk of Depression. Arch. Gen. Psychiatry 58 (3), 251–256. doi:10.1001/archpsyc.58.3.251
Substance Abuse and Mental Health Services Administration (2020). Key Substance Use and Mental Health Indicators in the United States: Results from the 2019 National Survey on Drug Use and Health. Rockville, MD: Center for Behavioral Health Statistics and Quality, Substance Abuse and Mental Health Services Administration. Available At: https://store.samhsa.gov/product/key-substance-use-and-mental-health-indicators-in-the-united-states-results-from-the-2019-national-survey-on-Drug-Use-and-Health/PEP20-07-01-001.
Uhls, Y. T., Ellison, N. B., and Subrahmanyam., K. (2017). Benefits and Costs of Social Media in Adolescence. Pediatrics 140 (Suppl. 2), S67–S70. doi:10.1542/peds.2016-1758E
Valkenburg, P., Beyens, I., Pouwels, J. L., van Drielvan Driel, I. I., and Keijsers, L. (2021). Social Media Use and Adolescents' Self-Esteem: Heading for a Person-specific Media Effects Paradigm. J. Commun. 71 (1), 56–78. doi:10.1093/joc/jqaa03910.1093/joc/jqaa039
Valkenburg, P. M., and Peter., J. (2007). Preadolescents' and Adolescents' Online Communication and Their Closeness to Friends. Developmental Psychol. 43 (2), 267–277. doi:10.1037/0012-1649.43.2.267
van den Berg, P., Paxton, S. J., Keery, H., Wall, M., Guo, J., and Neumark-Sztainer, D. (2007). Body Dissatisfaction and Body Comparison with media Images in Males and Females. Body Image 4 (3), 257–268. doi:10.1016/j.bodyim.2007.04.003
van ZalkWalter, M. H. W., Van Zalk, N., Kerr, M., and Stattin, H. (2014). Influences between Online-Exclusive, Conjoint and Offline-Exclusive Friendship Networks: The Moderating Role of Shyness. Eur. J. Pers 28 (2), 134–146. doi:10.1002/per.1895
Vidal, C., Lhaksampa, T., Miller, L., and Platt, R. (2020). Social Media Use and Depression in Adolescents: A Scoping Review. Int. Rev. Psychiatry 32 (3), 235–253. doi:10.1080/09540261.2020.1720623
Vogel, E. A., Rose, J. P. Bradley. M. Okdie., Okdie, B. M., Eckles, K., and Franz, B. (2015). Who Compares and Despairs? the Effect of Social Comparison Orientation on Social media Use and its Outcomes. Personal. individual differences 86, 249–256. doi:10.1016/j.paid.2015.06.026
Watson, David., and LeeClark, Anna. (1994). The PANAS-X: Manual for the Positive and Negative Affect Schedule - Expanded Form. University of Iowa. San Francisco, CA. Available At: https://iro.uiowa.edu/discovery/fulldisplay/alma9983557488402771/01IOWA_INST:ResearchRepository.
Whalen, C. K., Jamner, L. D., Henker, B., and Delfino, R. J. (2001). Smoking and Moods in Adolescents with Depressive and Aggressive Dispositions: Evidence from Surveys and Electronic Diaries. Health Psychol. 20 (2), 99–111. doi:10.1037/0278-6133.20.2.99
Keywords: depression, ecological momentary assessment (EMA), affect, online interactions, social support, social media, social network site use
Citation: Moukalled SH, Bickham DS and Rich M (2021) Examining the Associations Between Online Interactions and Momentary Affect in Depressed Adolescents. Front. Hum. Dyn 3:624727. doi: 10.3389/fhumd.2021.624727
Received: 01 November 2020; Accepted: 01 November 2021;
Published: 30 November 2021.
Edited by:
Yalda Uhls, University of California, Los Angeles, United StatesReviewed by:
Lucía Magis Weinberg, University of California, Berkeley, United StatesKaveri Subrahmanyam, California State University, Los Angeles, United States
Jessica Maya Hernandez, University of California, Irvine, United States, in collaboration with reviewer KS
Copyright © 2021 Moukalled, Bickham and Rich. This is an open-access article distributed under the terms of the Creative Commons Attribution License (CC BY). The use, distribution or reproduction in other forums is permitted, provided the original author(s) and the copyright owner(s) are credited and that the original publication in this journal is cited, in accordance with accepted academic practice. No use, distribution or reproduction is permitted which does not comply with these terms.
*Correspondence: David S. Bickham, RGF2aWQuYmlja2hhbUBjaGlsZHJlbnMuaGFydmFyZC5lZHU=