- 1Artificial Intelligence, Arcada University of Applied Sciences, Helsinki, Finland
- 2Data Science Graduate School, Seoul National University, Seoul, South Korea
- 3Philosophy Department, Pace University, New York, NY, United States
- 4University of Copenhagen, Copenhagen Emergency Medical Services, Copenhagen, Denmark
- 5Department of Medicine and Division of Infectious Diseases and Immunology, NYU Grossman School of Medicine, New York, NY, United States
- 6Montreal AI Ethics Institute, Canada and Union College, New York, NY, United States
- 7Petrie-Flom Center for Health Law Policy, Biotechnology, and Bioethics, Harvard Law School, Berkeley, CA, United States
- 8Center for Human-Compatible AI, University of California, Berkeley, CA, United States
- 9Faculty of Law, University of Cambridge, Cambridge, United Kingdom
- 10Center for the Study of Ethics in the Professions, Illinois Institute of Technology Chicago, Chicago, IL, United States
- 11Department of Food and Resource Economics, Faculty of Science University of Copenhagen, Copenhagen, Denmark
- 12Hautmedizin, Bad Soden, Germany
- 13CLAIM - Charité Lab for AI in Medicine, Charité Universitätsmedizin Berlin, Berlin, Germany
- 14QUEST Center for Transforming Biomedical Research, Berlin Institute of Health, Charité Universitätsmedizin Berlin, Berlin, Germany
- 15School of Computing and Digital Technology, Faculty of Computing, Engineering and the Built Environment, Birmingham City University, London, United Kingdom
- 16Center for Psychiatry Research, Department of Clinical Neuroscience, Karolinska Institutet, Stockholm, Sweden
- 17Industrial Engineering and Operation Research, University of California, Berkeley, CA, United States
- 18Frankfurt Big Data Lab, Goethe University, Frankfurt, Germany
- 19Parallel Computing Labs, Intel, Santa Clara, CA, United States
- 20Fitbiomics, New York, NY, United States
- 21Health Ethics and Policy Lab, Department of Health Sciences and Technology, ETH Zurich, Zürich, Switzerland
- 22Department of Mathematics, University of Oslo, Oslo, Norway
- 23Department of Economics and Management, Università degli studi di Pavia, Pavia, Italy
- 24École des médias, Université du Québec à Montréal and Philosophie, Collège André-Laurendeau, Québec, QC, Canada
- 25Department of Informatics, Umeå University, Umeå, Sweden
- 26Department of Computer Science (DIKU), University of Copenhagen (UCPH), Copenhagen, Denmark
- 27Department of Mathematics and Computer Science Eindhoven University of Technology, Eindhoven, Netherlands
- 28Observatoire Ethique and Intelligence Artificielle de l’Institut Sapiens, Paris-Cachan, France
- 29Section for Health Service Research and Section for General Practice, Department of Public Health, University of Copenhagen, Copenhagen, Denmark
- 30Cluster of Excellence "Machine Learning: New Perspectives for Science", University of Tuebingen, Tuebingen, Germany
- 31Institute of the Law and Regulation of Digitalization, Philipps-University Marburg, Philipps, Germany
- 32Inrupt, San Francisco, CA, United States
- 33University of Manchester and Wellcome Sanger Institute, Cambridge, United Kingdom
- 34Philosophy of Law, LUMSA University, Rome, Italy
- 35Law Department, Western University, London, ON, Canada
- 36Faculty of Laws, University College London, London, United Kingdom
- 37Independent AI Researcher (Law and Ethics) and Legal Tech Entrepreneur, Barcelona, Spain
- 38Department of Informatics, University of Oslo, Oslo, Norway
- 39Head of Community and Communications, Z-Inspection®Initiative, london
- 40Department of Physiology, Faculty of Medicine, University of Iceland, Reykjavik, Iceland
Artificial Intelligence (AI) has the potential to greatly improve the delivery of healthcare and other services that advance population health and wellbeing. However, the use of AI in healthcare also brings potential risks that may cause unintended harm. To guide future developments in AI, the High-Level Expert Group on AI set up by the European Commission (EC), recently published ethics guidelines for what it terms “trustworthy” AI. These guidelines are aimed at a variety of stakeholders, especially guiding practitioners toward more ethical and more robust applications of AI. In line with efforts of the EC, AI ethics scholarship focuses increasingly on converting abstract principles into actionable recommendations. However, the interpretation, relevance, and implementation of trustworthy AI depend on the domain and the context in which the AI system is used. The main contribution of this paper is to demonstrate how to use the general AI HLEG trustworthy AI guidelines in practice in the healthcare domain. To this end, we present a best practice of assessing the use of machine learning as a supportive tool to recognize cardiac arrest in emergency calls. The AI system under assessment is currently in use in the city of Copenhagen in Denmark. The assessment is accomplished by an independent team composed of philosophers, policy makers, social scientists, technical, legal, and medical experts. By leveraging an interdisciplinary team, we aim to expose the complex trade-offs and the necessity for such thorough human review when tackling socio-technical applications of AI in healthcare. For the assessment, we use a process to assess trustworthy AI, called 1Z-Inspection® to identify specific challenges and potential ethical trade-offs when we consider AI in practice.
Introduction
According to a recent literature review (Bærøe et al., 2020), Artificial Intelligence (AI) in healthcare is already being used: 1) in the assessment of the risk of disease onset and in estimating treatment success (before initiation); 2) in an attempt to manage or alleviate complications; 3) to assist with patient care during the active treatment or procedure phase; 4) in research aimed at elucidating the pathology or mechanism of and/or the ideal treatment for a disease.
For all of its potential, the use of AI in healthcare also brings major risks and potential unintended harm. Warning examples have shown that if ethical and social implications are disregarded, AI can inflict significant harm on the people it is intended to benefit (Obermeyer et al., 2019; Wiens et al., 2019; Gerke et al., 2020a; Gerke et al., 2020b; Grote and Berens, 2020; Larrazabal et al., 2020).
While there are some first uses of AI in healthcare, there is still a lack of many approved and validated products. Indeed, given that “the artificial intelligence industry is driven by strong economic and political interests,” the need for trustworthy adoption of AI in healthcare is crucial (Bærøe et al., 2020).
AI has the potential to “greatly improve the delivery of healthcare and other services that advance well-being, if it is validated by the authorities, accepted and supported by the Healthcare Professionals and Healthcare Organizations and trusted by patients” (MedTech Europe, 2019; Deloitte, 2020).
Trustworthy AI
In line with efforts of the European Commission (EC), AI ethics scholarship focuses increasingly on converting abstract principles into actionable recommendations (Kredo et al., 2016). However, the interpretation, relevance, and implementation of trustworthy AI depend on the domain and the context where the AI system is used. In order to bring some clarity and define a general framework for the use of AI Systems, the High-Level Expert Group on AI (AI HLEG), set up by the EC, published ethics guidelines for trustworthy AI in April 2019 (AI HLEG trustworthy AI guidelines) (AI HLEG, 2019). These guidelines are aimed at a variety of stakeholders, especially guiding practitioners toward more ethical and more robust applications of AI2.
According to AI HLEG, an AI to be trustworthy needs to be: lawful—respecting all applicable laws and regulations, robust—both from a technical and social perspective, and ethical—respecting ethical principles and values.
The AI HLEG defines four ethical principles rooted on fundamental rights (AI HLEG, 2019): 1) respect for human autonomy, 2) prevention of harm, 3) fairness, and 4) explicability.
Based on these four principles, the AI HLEG sets out seven requirements for AI systems to be deemed trustworthy and which assist the process of self-assessment. Each requirement is described below (AI HLEG, 2019)3:
• Human agency and oversight: all potential impacts that AI systems may have on fundamental rights should be accounted for and that the human role in the decision-making process is protected.
• Technical robustness and safety: AI systems should be secure and resilient in their operation in a way that minimizes potential harm, optimizes accuracy, and fosters confidence in their reliability;
• Privacy and data governance: given the vast quantities of data processed by AI systems, this principle impresses the importance of protecting the privacy, integrity, and quality of the data and protects human rights of access to it;
• Transparency: AI systems need to be understandable at a human level so that decisions made through AI can be traced back to their underlying data. If a decision cannot be explained it cannot easily be justified;
• Diversity, non-discrimination, and fairness: AI systems need to be inclusive and non-biased in their application. This is challenging when the data is not reflective of all the potential stakeholders of an AI system;
• Societal and environmental wellbeing: in acknowledging the potential power of AI systems, this principle emphasizes the need for wider social concerns, including the environment, democracy, and individuals to be taken into account; and
• Accountability: this principle, rooted in fairness, seeks to ensure clear lines of responsibility and accountability for the outcomes of AI systems, mechanisms for addressing trade-offs, and an environment in which concerns can be raised.
However, the interpretation, relevance, and implementation of trustworthy AI depends on the domain and the context where the AI system is used.
Challenges and Limitations
Although these requirements are a welcome first step toward enabling an assessment of the societal implication of the use of AI systems, there are some challenges in the practical application of requirements, namely:
- The AI HLEG trustworthy AI guidelines are not contextualized by the domain they are involved in. The meaning of some of the seven requirements is not anchored to the context (e.g., fairness, wellbeing, etc.).
- They mainly offer a static checklist (AI HLEG, 2020) and do not take into account changes of the AI over time.
- They do not distinguish different applicability of the AI HLEG trustworthy AI guidelines (e.g., during design vs. after production) as well as different stages of algorithmic development, starting from business and use-case development, design phase, training data procurement, building, testing, deployment, and monitoring (Morley et al., 2019).
- There are not available best practices to show how to implement such requirements and apply them in practice.
- The AI HLEG trustworthy AI guidelines do not explicitly address the lawful part of the assessment.
To help overcome some of these shortcomings, we created a holistic process to guide a trustworthy AI assessment. We present a case study to illustrate how it applies to a specific healthcare context.
Assessing Trustworthy AI in Healthcare
The paper’s main contribution is to demonstrate how to use the general AI HLEG trustworthy AI guidelines in practice for the domain of healthcare. To this end, we present a best practice of assessing the use of machine learning (ML) as a supportive tool to recognize cardiac arrest in emergency calls. The AI system under investigation has been used in the city of Copenhagen in Denmark since Fall 2020.
We use a process to assess trustworthy AI in practice, called Z-Inspection® (Zicari et al., 2021), which expands upon the “Framework for Trustworthy AI” as defined by the AI HLEG.
The Z-Inspection® is a holistic process based on the method of evaluating new technologies according to which ethical issues must be discussed through the elaboration of socio-technical scenarios. Echoing work in pragmatist ethics (Keulartz et al., 2002; Lucivero, 2016), this methodology makes it possible to implement the principles and requirements as defined in the AI HLEG trustworthy AI guidelines, while ensuring a satisfactory consideration of the specific issues of the cases studied. Socio-technical scenarios prove to be a particularly effective means of eliciting the reflections necessary to achieve the aims of the Z-Inspection®.
The Z-Inspection® process, in a nutshell, is depicted in Figure 1, and it is composed of three main phases: 1) the Set Up Phase, 2) the Assess Phase, and 3) the Resolve Phase.
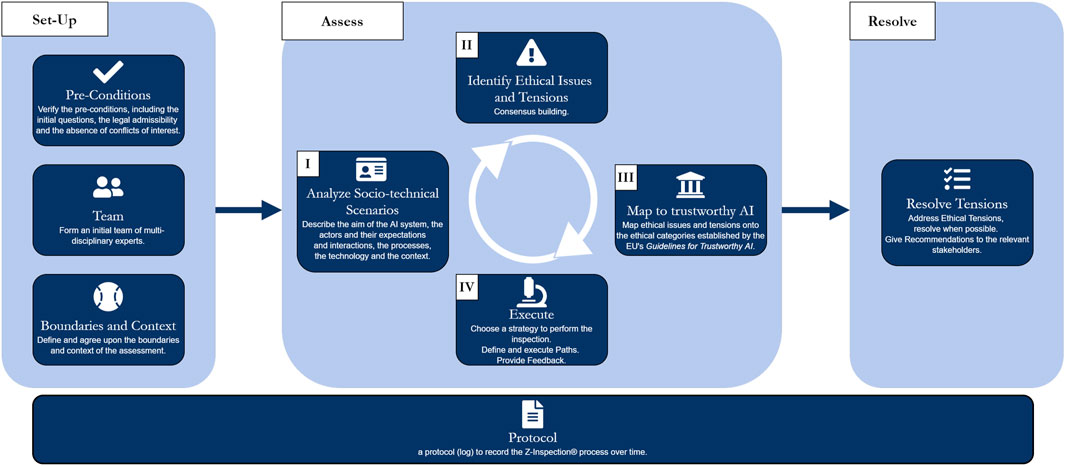
FIGURE 1. The Z-Inspection® process in a nutshell [with permission from (Zicari et al., 2021)].
Our approach is inspired by both theory and practice (“learning by doing”).
The work on Z-Inspection® started in late 2018. We have developed and tested the Z-Inspection® process by evaluating a non-invasive AI medical device designed to assist medical doctors in the diagnosis of cardiovascular diseases. The system we assessed was an AI medical device, [certified in Europe as CE class 1 according to the European Commission Medical Device Directives (MDD) (European Parliament and Council of European Union, 1993)] using machine learning to analyze sensor data (i.e., electrical signals of the heart) of patients to predict the risk of cardiovascular heart disease. Our team included ethicists, AI engineers, legal experts, medical doctors, and other domain experts.
The detailed process is described in (Zicari et al., 2021). Here we recall some of the key elements of the process. The Z-Inspection® is a general process to assess trustworthy AI in practice that can be used for a variety of domains where an AI system is under development and/or deployed. Here we focus on the healthcare domain, as it is pertinent to the use case that we are reporting in this paper.
The Set-Up Phase
The Set-Up phase starts by verifying that no conflict of interest exists, both direct and indirect, between independent experts and the primary stakeholders of the use case. This phase continues by creating a multi-disciplinary assessment team composed of a diverse range of experts. For this use case, the team included: philosophers, healthcare ethicists, healthcare domain experts (such as cardiologists, and other clinicians, cardiovascular and public health researchers), legal researchers, social scientists, AI engineers, and patient representatives. This is one of the most important aspects of our approach to ensure that a variety of viewpoints are expressed when assessing the trustworthiness of an AI system. The set-up phase also includes the definition of the boundaries of the assessment, taking into account that we do not assess the AI system in isolation but rather consider the social-technical interconnection with the ecosystem(s) where the AI is developed and/or deployed.
The Assess Phase
The Assess Phase is composed of four tasks:
I. The creation and analysis of Socio-Technical Scenarios for the AI system under assessment.
II. A list of ethical, technical, and legal “issues” is identified and described using an open vocabulary.
III. To reach consolidation, such “issues” are then mapped to some of the four ethical principles and the seven requirements defined in the EU framework for trustworthy AI.
IV. Execution of verification of claims is performed. A number of iterations of the four tasks may be necessary in order to arrive to a final consolidated rubrics of issues mapped into the trustworthy AI framework.
The Resolve Phase
In a nutshell, the resolve phase consists of giving recommendations to key stakeholders. It is crucial to monitor that the AI system that fulfilled the Trustworthy AI requirement at launch continues to do so over time. Therefore, when required, the resolve phase includes conducting a trustworthy monitoring over time of the AI system (we call it “ethical maintenance”). In Düdder et al. (2020), we have defined an AI ethical maintenance process based on an adapted version of the Reliability-Centered Maintenance (RCM) model (Moubray, 2001).
Using the process, we can identify possible ethical, as well as technical issues, of use of this AI system. In the rest of this paper, we report the results of the various tasks of the Z-Inspection® process applied to the specific use case presented below.
This paper is a first reflection of what we are learning by assessing this case. The assessment is ongoing. The final results of the assessment will be published in a follow up paper.
Assessing Trustworthy AI—Best Practice: Machine Learning as a Supportive Tool to Recognize Cardiac Arrest in Emergency Calls
The problem: Health-related emergency calls (112) are part of the Emergency Medical Dispatch Center (EMS) of the City of Copenhagen, triaged by medical dispatchers (i.e., medically trained dispatchers who answer the call, e.g., nurses and paramedics) and medical control by a physician on-site (Lippert, 2018).
In the last years, the Emergency Medical Dispatch Center of the City of Copenhagen has failed to identify approximately 25% of cases of out-of-hospital cardiac arrest (OHCA), the last quarter has only been recognized once the paramedics/ambulance arrives at the scene (Viereck et al., 2017; Blomberg et al., 2019; Drennan et al., 2021). Therefore, the Emergency Medical Dispatch Center of the City of Copenhagen loses the opportunity to provide the caller with instructions for cardiopulmonary resuscitation (CPR), and hence, impair survival rates. OHCA is a life-threatening condition that needs to be recognized rapidly by dispatchers, and recognition of OHCA by either a bystander or a dispatcher in the emergency medical dispatch center is a prerequisite for initiation of CPR.
A Cardiopulmonary Resuscitation (CPR) as defined by (Perkins et al., 2015) consists of compressions on the upper body to mechanically keep the blood flowing after the heart has stopped beating.
Previous research has identified barriers to the recognition of OHCA (Sasson et al., 2010; Møller et al., 2016; Viereck et al., 2017). Improving early recognition is a goal for both the American Heart Association and the Global Resuscitation Alliance (Callaway et al., 2015; Eisenberg et al., 2018; Nadarajan et al., 2018).
The AI solution: A team lead by Stig Nikolaj Blomberg (Emergency Medical Services Copenhagen, and Department of Clinical Medicine, University of Copenhagen, Denmark) worked together with a start-up company and examined whether a machine learning (ML) framework could be used to recognize out-of-hospital cardiac arrest (OHCA) by listening to the calls made to the Emergency Medical Dispatch Center of the City of Copenhagen. The company designed and implemented the AI system and trained and tested it by using the archive of audio files of emergency calls provided by Emergency Medical Services Copenhagen in the year 2014. The prime aim of this AI system is to assist medical dispatchers when answering 112 emergency calls to help them to early detect OHCA during the calls, and therefore possibly saving lives.
Status: The AI system was put into production during Fall 2020.
The research questions: Is the AI system trustworthy? Is the use of this AI system trustworthy?
Motivation
This is a self-assessment conducted jointly by a team of independent experts together with the prime stakeholder of this use case. The main motivation of this work is to study if the rate of lives saved could be increased by using AI, and at the same time to identify how trustworthy is the use of the AI system assessed here, and to provide recommendations to key stakeholders.
The Set Up
To perform the assessment, an initial team of interdisciplinary4 experts was formed. The composition of the team is a dynamic process and the choice of the experts, their skills, background, and roles have a significant ethical implication for the overall process. In our opinion, one cornerstone of being able to conduct an independent AI ethical assessment is the absence of conflict of interests,5 both direct and indirect. If conflicts of interests are revealed in the course of the inspection, they are recorded and shared with whatever entities solicited the inspection in the interest of transparency and integrity.
Next, we defined the boundaries and the context of the assessment. In our assessment process, an AI system is never analyzed in isolation but always taking into account what we call the “ecosystems.”
We define an ecosystem, as applied to our work, as a set of sectors and parts of society, level of social organization, and stakeholders within a political and economic context where the AI is playing a role (Whittlestone et al., 2019).
The Assess Phase
The Assess Phase is composed of four tasks: I. The analysis of the usage of the AI system. II. The identification of possible ethical issues, as well as technical and legal issues. III. Mapping of such issues to the trustworthy AI ethical values and requirements. IV. The verification of such requirements.
The basic idea is 1) to identify a list of ethical and other issues (called flags) which require inspection, then 2) map them to some or all of the seven requirements for trustworthy AI, and from this mapping, 3) create a plan of investigation that will provide feedback to re-evaluate the initial list of ethical issues and flags to produce a consolidated list.
We can visualize this part of the process as follows: the first part, 1) leaves space for the experts to explore and identify possible “issues” using an open vocabulary. They describe ethical issues and flags with their own words and bring in their own expertize and different background and viewpoints. The second part, 2) the mapping, forces the discussion to reach a consensus by using a closed vocabulary, i.e., the four ethical principles and the seven requirements for trustworthy AI. The third part, 3) depends on the status of the assessment. For post deployment, it helps verify claims (if any), or as a tool to support the design of the AI system.
This phase was undertaken by a general group of 30 participants proficient in technical and theoretical computer science, ethics, law, social science, and medical expertize specific to the particular use case. General meetings, reflecting the iterative structure of the Assess Phase, were envisioned via a tripartite structure: the first for the primary stakeholders of the original use case to motivate and present their work, the second for Z-Inspection® participants to ask substantive and critical questions of the primary stakeholders, and the third for participants to map these questions to the ethical categories in the EU’s Guidelines for Trustworthy AI. Following this mapping, the general group splintered into more specialized subgroups to continue the Z-inspection.
In this paper, we will cover tasks I, II and III. We plan to publish the results of task IV in a forthcoming paper.
The Analysis of the Usage of the AI System
The Assess Phase of the process begins with the analysis of socio-technical scenarios.
Scenarios of Use
In order to answer the above research questions, we created scenarios of use for this AI system and discussed them in several workshops with the experts together with the prime stakeholder. We report the essential parts in this section.
The basic idea is to analyze the AI system using socio-technical scenarios with relevant stakeholders, including domain, technical, legal, and ethics experts (Leikas et al., 2019). For this case, we decided not to include the vendor company who designed and implemented the AI system in the analysis, due to possible conflict of interests.
Socio-technical scenarios or usage scenarios are a useful tool to describe the aim of the system, the actors, their expectations, the goals of actors’ actions, the technology, and the context (Leikas et al., 2019). Socio-technical scenarios can also be used to broaden stakeholder understanding of one’s own role in understanding technology, as well as awareness of stakeholder interdependence. Scenarios can be used as a part of the assessment of an AI system already deployed (as in this case), or as a participatory design tool if the AI is in the design phase.
Our team of experts used socio-technical scenarios to be able to identify a list of potential ethical and, technical and legal issues that needed to be further deliberated. For that, we used discussion workshops, where expert groups worked together to systematically examine, and elaborate the various tasks with respect to different contexts of the AI. We then distributed the work to smaller working groups to continue the analysis. We present in the rest of this section a summary of the socio-technical scenarios that we have created for this use case.
Aim of the ML System
We started by analyzing the prime aim of this AI system, namely to assist medical dispatchers (also referred to as call takers) when answering 112 emergency calls to help them to early detect OHCA during the calls, and increase the potential for saving lives.
The system has been implemented because OHCA can be difficult for call takers to identify, possibly due to static, language barriers, unclear descriptions by callers, and misunderstandings, along with limited attention spans in calls.
For OHCA, a specific problem (compared with other 112 calls) is that the caller is never the patient—as they are unresponsive at that time of the call (Safar, 1988)—but a bystander (i.e., spouse or passer-by).
Identification of Actors
For this use case, we identified three classes of actors: primary, secondary, and tertiary.
We define primary actors as stakeholders in direct contact with the applied system.
The primary actors are Stig Nikolaj and his team (who specified the requirements for the design of the AI system and supplied the training and test data) are the prime stakeholder of the use case; the patients; the patients’ family members, the callers/bystanders; paramedics and the medically trained dispatchers who answer the call.
Secondary actors are stakeholders responsible for developing and implementing the system but not using it directly.
The secondary actors are: the AI vendor, a start-up company, independent from the owner of the case who designed, implemented, and deployed the AI system. The CEO of the Emergency Medical Services who gave permission to put the system into deployment.
Tertiary actors are part of the overall ecosystem where the AI system is used.
The tertiary actors are the Copenhagen Emergency Medical Services (EMS), which is an integrated part of the Health Care System for the Capital Region of Denmark, consisting of one hospital trust with six university hospitals in nine locations and one emergency medical service (Lippert, 2018).
Actors Expectations and Motivations
The actors listed above share one common goal: saving the patient’s life. Aside from this goal, the actors have some distinct expectations and motivations:
- Caller/bystander: receive easy to understand and follow instructions to help patient;
- Dispatcher/call taker: provide targeted support and instructions to caller based on correct information;
- Paramedics: receive correct information to be well prepared upon arrival to care for the patient;
- Patients’ family members: know that everything possible was done to save the patient’s life and that no error occurred in the process (human or machine); if the patient dies, they may look for someone to hold responsible (the dispatcher/paramedic/AI system?);
- AI vendor: profit, reputation, satisfied clients, avoid malfunctioning of the system leading to poor performance (e.g., death of the patient);
- Hospital system: improve efficiency and efficacy (i.e., number of lives saved due to the system), reputational gains; and
- Public Health System in Denmark: improve efficiency and efficacy (i.e., number of lives saved due to the AI system).
AI Pilot Testing
The system was introduced to the call takers by the primary investigator of research (i.e., the owner of the use case), who participated in four staff meetings, each of them consisting of an hour training session. During these sessions, the AI system was presented as well as the objectives of the research and the protocol the dispatchers should follow in case of an alert. There was a one-month pilot testing where none of the alerts were randomized. This was performed to allow most of the dispatchers to experience an alert prior to the randomization start. During this month, the primary investigator was present at the Emergency Medical Dispatch Center of the City of Copenhagen and available for dispatchers to address questions.
Develop of an Evidence Base
It is important at this point to review and create an evidence base that we will use to verify/support any claims made by the producer of the AI system and other relevant stakeholders.
For this case, we summarize here the most relevant findings.
OHCA is a major health care and socioeconomic problem with a total survival rate of generally below 10 percent (Berdowski et al., 2010; Gräsner et al., 2020; Virani et al., 2020). Time is of utmost importance when treating OHCA, with chances of survival decreasing rapidly in the first minutes after collapse. Efficient emergency medical services should detect cardiac arrest within the first minute (Perkins et al., 2015). Correct diagnosis and treatment are needed within minutes in order to increase the odds to attain a successful resuscitation. Every minute without resuscitation decreases the probability of survival by ∼10% and increases the risk of side-effects, such as brain damage (Murphy et al., 1994).
Detecting OHCA is the “king quality indicator” across medical services in Europe and the rest of the world (Wnent et al., 2015). One reason for this is that cardiac arrest is the most time critical incident, which an emergency medical service can respond to. If the emergency service performs substandard to these incidents, it would be a generally low quality proxy.
Therefore, recognition of OHCA is of the utmost importance as a prerequisite for the initiation of life-saving treatments such as CPR and defibrillation prior to the arrival of emergency medical services (Holmén Johan et al., 2020). However, there are also risks associated with administering CPR to a healthy person for a longer period (∼10 min), as it can impact their health negatively (Haley et al., 2011; Moriwaki et al., 2012). In cases of a misdiagnosis, it is highly likely that the patient will respond at this point.
The chance of survival decreases rapidly after the onset of OHCA until the initiation of resuscitation efforts (CPR or defibrillation), with models illustrating a decrease of roughly 10% per minute, leaving close to zero percent chance of survival 15 min after collapse. Due to the loss of circulation following OHCA, imminent treatment is of the essence since the chance of survival rapidly decreases with increased time from collapse to treatment (Cummins et al., 1991; Larsen et al., 1993; Hasselqvist-Ax et al., 2015; Monsieurs et al., 2015).
Survivors of OHCA may sustain brain injury due to inadequate cerebral perfusion during cardiac arrest. Anoxic brain damage after OHCA may result in a need for constant care or assistance with activities of daily living. Persons with anoxic brain damage may therefore require nursing home care after discharge (Middelkamp et al., 2007; Moulaert et al., 2009).
Context and Processes, Where the AI System is Used
We look now at the context and process where the AI system is used, including the interactions of actors with each other and with the ML.
The AI system is listening in to all calls made to the emergency medical services 112 emergency line. This includes calls for various other reasons (e.g., car accidents); OHCA is only responsible for ∼1% of all calls. With ∼65 dispatchers, every dispatcher only encounters 10–20 cases of cardiac arrest per year on average. It is reported that 1/2 of the human alerts were true cardiac arrests, 1/5 of the machine alerts were true cardiac arrests (Blomberg et al., 2021).
Figure 2 depicts a hypothetical case of a call where an actual cardiac arrest is occurring: The patient is suffering a cardiac arrest and is therefore lifeless. A bystander (e.g., spouse of the patient) calls the 112 emergency-line and he/she is connected to a dispatcher. The dispatcher is responsible for asking relevant questions to the caller; the ML system is listening in on the call but currently does not provide any questions to the caller or the dispatcher. Once the system suspects a cardiac arrest, it shows an “alert” to the dispatcher, who is then free to act upon this alert or ignore it.
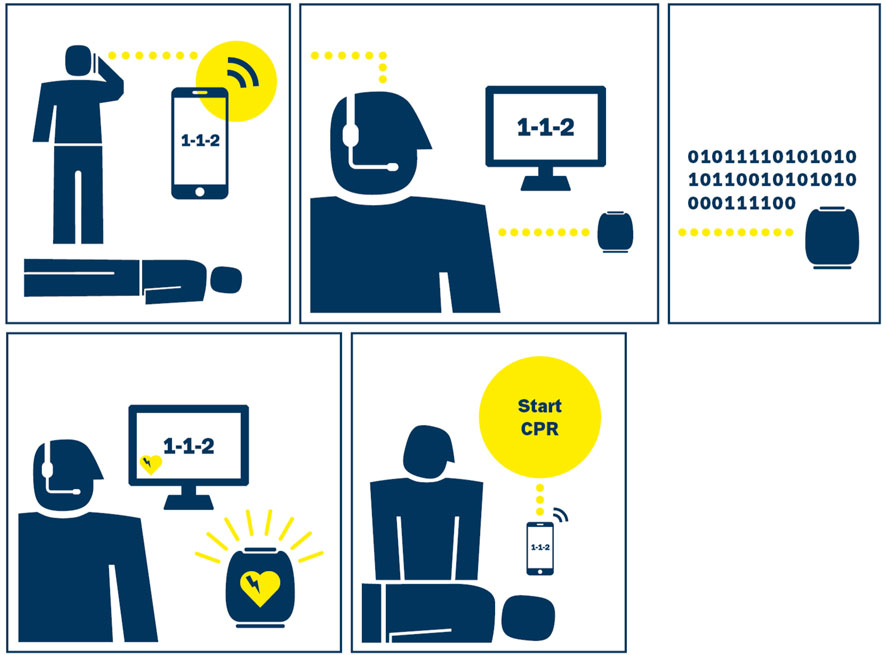
FIGURE 2. Ideal case of Interaction between Bystander, Dispatcher, and the ML System. (with permission from Blomberg et al., 2019).
If the dispatcher agrees with the system in the detection of a cardiac arrest, they instruct the caller to administer CPR to the patient (very time-sensitive) and dispatch an ambulance, including a doctor. They should then stay on the call until the ambulance arrives.
The Technology Used
The prime stakeholder commissioned an external start-up company to implement the AI system because they discovered that off-the-shelf solutions did not work, due to poor sound quality of the calls, and abnormal vocals (e.g., emotionally distressed, shouting, etc.). Also, at that time (2018), no Danish language model was readily available.
For this use case, the ML system was designed and implemented with the expectation to detect cardiac arrest in calls faster and more reliably than human operators. An initial confirmation of this assumption was reported in a retrospective study conducted by the prime stakeholders (Blomberg et al., 2019).
They used a language model for translating the audio to text based on a convolutional deep neural network (LeCun et al., 1989). The ML model was trained and tested on datasets of audio files of calls to the 112 emergency line made in 2014, provided by the prime stakeholder to the company. Only the audio was used, so other personal data was explicitly not used.
The text output of the language model was then fed to a classifier that predicted whether a cardiac arrest was happening or not (Figure 3). The AI system was applied directly on the audio stream where the only processing made was a short-term Fourier transformation (Havtorn et al., 2020), hence no explicit feature selection was made.
The predictive model, working only on the text output of the automatic speech recognition model, was predicted based on the raw textual output. When an emergency call was analyzed in real-time by the ML framework, the audio file was processed without any prior editing or transcription and transformed to a textual representation of the call, which was then analyzed and outputted as a prediction of cardiac arrest (Blomberg et al., 2021).
Using a Danish language model means that calls in other languages were interpreted in a way that the cardiac arrest model could not work with (i.e., trying to understand Danish words from English speech). In many cases, the model understood the calls anyways, but in some cases not. So far, there is no explanation why some calls were seemingly not understood.
There is no explanation of how the ML makes its predictions. The company that developed the AI system has some of their work in the open domain (Maaløe et al., 2019; Havtorn et al., 2020). However, the exact details on the ML system used for this use case are not publicly available.
The general principles used for this AI system are documented in the study by (Havtorn et al., 2020). The paper describes the AI model implemented for this use case. However, the paper presents the model trained using different data sets and therefore the results are not representative for this use case. The details of the implementation of the AI system for this case are proprietary, and therefore not known to our team.
Our expert team was informed by the prime stakeholder that the AI system does not have a CE-certification as a medical device.
AI Design Decisions
The assumption made by the designers of the AI system was that there are some patterns in the conversations that the AI system can detect and use to alert the call takers quicker than a human dispatcher, for example, from the words chosen and from the tone of a bystander.
The AI system analyses the conversation between the caller and the dispatcher. It has access to the full audio, including background noises. In its present implementation, if the AI system is suspecting a cardiac arrest, it presents an alert to the dispatcher. Currently, the system is only used for detecting cardiac arrest and does not propose questions to the dispatcher, based on the dispatcher’s previous conversations.
In a previous implementation of the AI system, background noises caused the AI system to generate many false positives (which would allocate resources to the wrong patients and thereby delaying treatment for others that are in greater need). Also, listening for agonal breathing has resulted in many false positives. Busses passing outside, or chairs being dragged on the floor, resulting in similar noises.
Agonal breathing (short, labored, gasping breaths that occur because oxygen cannot reach the brain) (Roppolo et al., 2009) is defined as “an abnormal breathing pattern originating from lower brainstem neurons and characterized by labored breaths, gasping, and, often, myoclonus and grunting.” (NCBI, 2021).
In some calls, the dispatcher asked the caller to put the phone to the patient’s mouth to listen for breathing. However, this is more an exception, and in the experience of the key stakeholders rarely produces any results. When one can hear the agonal breathing, it is quite distinct in the background. While agonal breathing is highly predictive of OHCA, it can be perceived by a layperson as normal breathing, leading to the misunderstanding that the patient is alive and therefore not having OHCA.
Therefore, for the final version of the AI system, one key design decision that the prime stakeholder took together with the software developers of the vendor company was to censor the ML model to disregard background noises in order to avoid too many false positives, even though some noises gave a good indication of a current cardiac arrest.
In its present implementation, the AI model therefore only listens to the words spoken by the caller and the dispatcher. The AI is converting the audio files into text files representing words. The call is transcribed, but the model is more complicated than just words. It is looking for patterns in questions and answers. For example, if the caller replies yes to a question of unconsciousness, then the probability of cardiac arrest goes up. If then the caller mentions blue lips, the probability goes up. If both are positive—patient unconscious and blue lips –, then the alert goes off, as described in an interview with our expert team by the prime stakeholder.
The medical dispatchers were involved in designing the alert that the system shows if it has detected signals indicating cardiac arrest. The dispatchers were consulted during several workshops conducted by the prime stakeholder. Callers and patients have not been involved in the system design with the reasoning that patients are clinically dead and callers are not concerned with how the system presents itself to the dispatcher.
AI Design Trade-Offs
Design choice depends on the perspective used by the prime stakeholders. There was a conscious key choice during system design to focus on high sensitivity over high specificity, as the prime stakeholder considered potential harm by a false negative much higher than the potential harm of a false positive. However, there was a trade-off as to not create too many false positives that undermine the credibility of the system and also waste of resources, with the unintended consequence that if there are not enough resources, then other patients can be harmed due to the false-positive result.
Clinical Studies
The primary stakeholders performed two studies: 1) A retrospective study performed before they deployed the AI system in production (Blomberg et al., 2019); and later on, 2) a randomized clinical trial (Blomberg et al., 2021) whose results were published after the AI system was already in production. For both studies, the same model was used—i.e., there were no changes to architecture or retraining between the studies.
Retrospective Study
In the retrospective study, the authors examined whether the ML system could recognize OHCA by analyzing the audio files of 108,607 emergency calls made in 2014 to the emergency medical dispatcher center. The performance of the ML system was compared to the actual recognition and time-to-recognition of cardiac arrest by medical dispatchers.
Out of 108,607 emergency calls, 0.8% (918) of the calls were OHCA calls eligible for analysis. Compared with medical dispatchers, the ML system had a lower positive predictive value than dispatchers (20.9 vs. 33.0%, p < 0.0001). Time-to-recognition was shorter for the ML system compared to the dispatchers (median 44 vs. 54 s, p < 0.001) (Blomberg et al., 2019).
Many times, the ML system was only slightly faster than the human, but in some calls, it was minutes faster than the dispatcher and well within the 1 min detection limit, making a huge practical difference in those cases.
The AI model for this use case was found to be more sensitive than the dispatcher but less specific. There were also cases where the model missed cardiac arrest, while the dispatcher did not (Blomberg et al., 2019). In some cases, this might come from the language barrier, as the system was only trained on Danish data, but the dispatchers understand more languages (i.e., English, German).
False negatives were identified with the help of the Danish Cardiac Arrest Register (Dansk Hjertestopregister, 2020). The register collects all emergency cases where either a bystander or ambulance personnel are applying CPR or defibrillation. The data is collected by ambulance personnel.
Randomized Clinical Trial
In the randomized clinical trial of 5242 emergency calls, the ML model was listening to calls and could alert the medical dispatchers in cases of suspected cardiac arrest. This way, it was possible to check how fast the machine could make a prediction compared to the human dispatchers. The dispatchers were instructed how to interact with the system: if they saw the alert symbol, they were instructed to repeat the questions for patient consciousness and if the patient was breathing.
In this randomized clinical trial, an extensive study of calls was done in relation to the retrospective study to compare if the dispatcher was trying to persuade the caller to perform CPR in these cases.
In some cases, the patient collapsed during the call. The patient was reported as being alive at the beginning of the conversation, but once the ambulance arrived, they had to administer CPR.
Sometimes the machine predicted cardiac arrest while the patient was not suffering any symptoms, but when the ambulance arrived at the call site, the patient did suffer a cardiac arrest.
In this clinical trial, the humans and the AI system missed the same patients. However, the AI system missed fewer.
During the interview with the prime stakeholders, we were told that benchmarking dispatchers was not done as this was not part of the task, but also due to not wanting to jeopardize the cooperation between the researchers and the medical dispatchers. While it might be possible to use anonymized data, with 65 dispatchers and ∼1,000 cardiac arrests per year, the sample is also very small. Finding reasons on why some dispatchers might perform worse than others was also not a goal of this trial.
Real time performance on actual emergency calls is comparable to the one reported in the retrospective study (Blomberg et al., 2021).
The result of this clinical trial was that “there was no significant improvement in recognition of out-of-hospital cardiac arrest during calls on which the model alerted dispatchers vs. those on which it did not; however, the machine learning model had higher sensitivity that dispatchers alone” (Blomberg et al., 2021).
The authors concluded that “these findings suggest that while a machine learning model recognized a significantly greater number of out-of-hospital cardiac arrests than dispatchers alone, this did not translate into improved cardiac arrest recognition by dispatchers” (Blomberg et al., 2021).
Intellectual Property
For this use case, the AI software (i.e., the AI model) is proprietary to the company that implemented it, but the data used for training and testing the ML model belongs to the prime stakeholder of the use case.
Due to the proprietary model and the personal data used, we were not able to reproduce the architecture and train the AI from scratch to conduct an independent evaluation of its performance. However, the authors of the randomized clinical trial provided an aggregated and anonymized dataset for analysis. This dataset did not contain audio data, but information on e.g., call length, sex and age of the caller, the recognition time of both dispatcher and AI system and whether the suspected OHCA could be confirmed later. With this data we could reproduce and confirm the findings of the randomized clinical trial (Blomberg et al., 2021).
The Legal Framework
The ML model in this use case is used by medical personnel to guide them in making an evaluation of the patient so that they can act accordingly. This process is not fully described by the Danish Health Act Sundhedsloven6, but it is described to some extent by the Patient Danish Authority (STPS)7. As the use of AI in health services is fairly new, the Danish authorities have apparently not yet decided how this new technology shall be regulated. In particular, it is very likely that the AI system should be classified as a medical device and thus being subject to medical device requirements. However, in the present state of the assessment we did not fully assess whether the prime stakeholder has obtained all authorizations needed. Since the AI system processes personal data, the General Data Protection Regulation (GDPR) (European Parliament and Council of European Union, 2016) applies, and the prime stakeholder must comply with its requirements. We do not assess here whether the AI system complies with the law. According to the prime stakeholder, the applicable laws have been followed.
The Identification of Possible Ethical and Technical Issues
In this step of the Assess Phase, we identified possible ethical and technical and legal issues for the use of the AI within the given boundaries and context (see list of actors above). For some ethical issues, a tension may occur. We use the definition of tension from Whittlestone et al. (2019), which refers to different ways in which values can be in conflict—i.e., tensions between the pursuit of different values in technological applications rather than an abstract tension between the values themselves.
The scenarios representing different usage situations of the system were discussed with a number of experts and when necessary other stakeholders.
The experts examined phase by phase according to the trustworthy ethical values requirements in order to define potential ethical issues and cross-checked against each phase of the scenario to identify possible ethical issues arising, discussed them, described them and reported at each step and documented.
In this part of the process, we left space for the experts to explore and identify possible “issues” using an open vocabulary. They described the ethical issues and what we call “flags” in their own words, bringing their own expertize and different background and view points. With a flag, we denote any other issues, i.e., that could refer to technical and ethical and/or any combination of.
The process used to reach consensus is made transparent so that it is possible to go back and re-assess possible relevant changes in the ecosystems.
Describe Ethical Issues and Tensions: Methodology
To describe and classify the ethical issues and flags, identify ethical tensions (if any) and describe them, a selected number of experts in our team were asked, with interdisciplinary skills, e.g., experts in ethics, philosophy, policy, law, domain experts, ML. Such a variety of backgrounds is necessary to identify all aspects of the ethical implications of using AI. While the interdisciplinary nature of the team is essential, it can pose a challenge on how to reach a consensus among the various experts.
Discussion—Initial Findings
By analyzing the scenario of usage for this use case, the following initial preliminary issues were observed and described. As mentioned, in this phase, the issues are described using an open vocabulary.
A selection of the initial findings is presented in the rest of this section.
Tensions in the Evidence Base
There is a tension between the conclusions from the retrospective study (Blomberg et al., 2019), indicating that the ML framework performed better than emergency medical dispatchers for identifying OHCA in emergency phone calls—and therefore with the expectation that the ML could play an important role as a decision support tool for emergency medical dispatchers-, and the results of a randomized control trial performed later (September 2018–January 2020) (Blomberg et al., 2021), which did not show any benefits in using the AI system in practice.
We were told in an interview with the prime stakeholder that patients were not study participants in this randomized control trial. The rationale that was given to us is that if they should have studied patient outcomes, the trial should have either be a multicenter study or continue for approximate eight years.
Possible Lack of Trust
For our assessment, it is important to find out whether and how the ML system influences the interaction between the human actors, i.e., how it influences the conversation between the caller/bystander and the dispatcher, the duration of the call, and the outcome, and why during the clinical trial the use of the AI system did not translate into improved cardiac arrest recognition by dispatchers (Blomberg et al., 2021).
Some possible hypotheses that need to be verified are listed in the following.
The dispatcher possibly did not trust the cardiac arrest alert. It might depend on how the system was introduced—how the well-known cognitive biases were presented/labeled—if the use of the system was labeled as a learning opportunity for the dispatcher, and not as a failure detection aid, that would disclose the incompetence of the dispatcher.
We reported this. To assess this, it would be desirable to look for potential patterns of, for example, cognitive bias in the dispatchers and provide specific feedback to the dispatcher.
Another hypothesis is that the case aims for performance (or accuracy), so if the machine works well enough, the alerts will be accepted. In this case, the trust might be increased by how the system is presented if the people who implement it confer the idea of a “growth mindset” that dispatchers could identify with, that might really improve uptake and trust.
But it could be that dispatchers did not sufficiently pay attention to the output of the machine. It relates to the principle of human agency and oversight in trustworthy AI mentioned in the rest of this section. Why exactly is this?
There seems to be a tension concerning the role of dispatchers in designing the algorithmic output.
Perhaps certain sounds should also be used to ensure that the dispatcher perceived the urgency of the algorithmic output? One additional idea is that the look and functionality of the alert does not perform as it should, perhaps because the dispatchers have been part of designing it themselves?
What makes them knowledgeable about how to get them to react in the way desired? Perhaps they are biased against a design that would make them feel the pressure to follow the machine?
Additional Tensions
If one of the reasons why dispatchers are not following the system to the desired degree is that they find the AI system to have too many false positives, then this issue relates to the challenge of achieving a satisfactory interaction outcome between dispatchers and system.
Another tension concerns whether dispatchers should be allowed to overrule a positive prediction made by the system and not just merely overrule a negative prediction by the system. In particular, what exactly is the right interplay or form of interaction between system and human, given the goals of using the system and the documented performance of human and system?
Medical Benefits—Risks Vs. Benefits
Possible Risks and Harm: False Positives and False Negatives
One of the biggest risks for this use case is where a correct dispatcher would be overruled by an incorrect AI system.
The AI system does not predict “no cardiac arrest,” but only positive predictions are shown. Hence, if a dispatcher suspects a cardiac arrest, the machine does not change this, but the dispatcher would not necessarily be affirmed. However, the dispatcher’s actions might trick the machine into believing it is a cardiac arrest, as the conversation might take a turn and start sounding like cardiac arrest to the machine.
We could not find a justification for choosing a certain balance between sensitivity and specificity.
If specificity is too low, CPR is started on people who do not need it and administered CPR over a longer period of time can lead to rib cage fractures, for example. However, it is unlikely that CPR would be performed on a conscious patient for a longer time, as the patient probably would fight back against it.
If sensitivity is too low, cardiac arrests may not be detected. This results in no CPR being administered and the patient remains dead. In this context “too low” is when the AI system performs poorer than the dispatchers, hence will not be of any help. The AI system is evaluated against human performance, as this system is only useful if it can assist humans; otherwise, it is just a distraction.
The idea that it is a serious defect if the machine does not confirm a correct positive call by a dispatcher points to an ethical tension concerning the machine-dispatcher interaction.
While it seems to be a great harm if a dispatcher did not follow her judgment due to a lack of confirmation from the machine, it should also be considered whether this is any worse than having a dispatcher wrongly ignoring a true positive call by a machine, and if so, why?
From the point of view of the person suffering a cardiac arrest, the harm of either mistake would be the same. In fact, given that the machine has, for example, a 10% higher sensitivity than dispatchers, it can be expected that allowing dispatchers to ignore positive calls from the machine will result in more deaths overall as compared to making it compulsive for dispatchers to follow the machine’s advice.
Thus, there is a tension between allowing dispatchers to ignore machine advice, perhaps to maintain full human control and responsibility for the decision-making, and saving all the lives that one could save by making dispatchers obliged to follow the advice of the machine.
Ethical Tension Related to the Design of the AI System
A number of questions were raised during the analysis of the use of the AI system.
AI Human Interactions Design Limitations
Currently, there is no structured way for feedback from the ambulance medics to the dispatchers.
We noted that there is no learning possibility in the current system—compared with other contexts such as aviation security, where “individuals’ attitudes (as opposed to personalities) are relatively malleable to training interventions and predict performance” (Sexton et al., 2000).
For our assessment, it is important to verify: Is it possible that by improving the set of questions, it will also be possible to improve the ML classifier? This question would ask for biological descriptors—such as does he look pale, can he move, etc. It would make sense for the dispatcher to ask questions that are tailored to aid the ML classifier to reduce the risk of false alerts/non-alerts.
An additional serious challenge is that AI is based only on conversations and language with all connected risks of emotional language miscomprehension of dialect or not a native speaker.
Lack of Explainability
Our team of experts did not sign a Non Disclosure Agreement (NDA) with the vendor company, and that means that the AI system is considered a “black box,” with no details of the implementation of the AI algorithms and the AI model. To avoid possible conflict of interests, no direct communication between our team of experts and the vendor company was (and is) taking place.
The prime stakeholder cooperates with the vendor company, and they have declared no conflict of interest with them.
The main issue here is that it is not apparent to the dispatchers how the AI system comes to its conclusions. It is not transparent to the dispatcher whether it is advisable to follow the system or not. Moreover, it is not transparent to the caller that an AI system is used in the process.
If transparency, at least in part, concerns the ability to understand why and how the AI system comes to produce a certain output given a certain input, then transparency about the values that have guided and justified the trade-offs would seem relevant. There is increasing awareness of the many ways in which the design of an AI system involves value-based judgments (Biddle, 2020). Examples of this type of judgment include when designers of the system decide how to balance the costs of false positives and false negatives, but also trade-offs between accuracy and explainability, and between different formal and potentially conflicting definitions of algorithmic fairness, such as equality of error rates and equality of predictive value across socially salient groups would ideally be explicated.
Diversity, Non-Discrimination, and Fairness: Possible Bias, Lack of Fairness
It was reported in one of the workshops that if the caller was not with the patient, such as in another room or in a car on their way to the patient, the AI system had more false negatives. The same was found for people not speaking Danish or with a heavy dialect.
For this use case, concepts such as “bias” and “fairness” are domain-specific and should be considered at various levels of abstractions (e.g., from the viewpoint of the healthcare actors down to the level of the ML model).
We look at possible bias in the use of the AI system. The AI system was only trained on Danish data, but the callers spoke more languages (i.e., English, German). Here, there is a risk of bias, as the system brings disadvantages for some groups, such as non-Danish speaking callers, callers speaking dialects, etc.
A serious challenge is that AI is based only on conversations and language with all connected risks of emotional language miscomprehension of dialect or non-native speakers. There is a risk that the AI system does not work equally well with all ethnic groups. It works best with Danish-speaking callers. It actually has a lower degree of being able to handle caller diversity than the dispatchers, who sometimes speak several languages. Thus, ethnic minorities would be discriminated against.
When we looked at the data used to train the ML model, we observed that the dataset used to train the ML system was created by collecting data from the Copenhagen Emergency Medical Services from 2014.
The AI system was tested with data from calls between September 1, 2018, and December 31, 2019. It appears to be biased toward older males, with no data on race and ethnicity.
We suspect this methodology to present risks of unwanted discrimination against minorities and under-represented races and sex. Multiple factors could bias the system, such as accent or words used. Predictions for individuals outside of training distributions would likely be less accurate, and dispatchers would misuse this information.
At the same time, older males are the most frequent “consumers” of health care when it comes to cardiac arrest, so is this really a bias? And even if this is defined as a bias, we might need to acknowledge why such a bias emerged in the first place. Likely, the calls that were used in training the ML were with older males.
In general, AI encodes the same biases present in society, whether through algorithmic design or biased data sets (Owens and Walker, 2020).
Risk of De-Skilling
For this use case, a problem is the responsibility and liability of the dispatcher. What are the possible legal liability implications for ignoring an alert coming from a ML system?
The consequences of refuse or acceptance of an alert are central. There is a need of justification of choice: in this field, the risk of de-skilling is possible (technological delegation also in order not to be considered reliable for ignoring/refusing it); we also need to think about the cultural level of a dispatcher and the ethical awareness of the consequences of they choice: how could they decide against the machine? Sometimes it could be easier to accept than to ignore/refuse for many reasons.
Risk of Alert Fatigue
In the randomized clinical trial (Blomberg et al., 2021), it was reported that less than one in five alerts were true positives. Such low sensitivity might lead to alert fatigue, and in turn, ignoring true alerts. However, the dispatcher is always ultimately liable, meaning if they ignore a true positive, they will need to provide rationale.
The alert fatigue is important and needs to be investigated because one wants to make sure that the AI fits neatly in the medical workflow and actually improves patient outcomes. If it turns out that the dispatcher is not following it because of alert fatigue, this would be a problem (also likely from a liability perspective).
A follow-up question would be what the interaction between the human and the AI system should be. It may be (depending on data of human factors testing in the real world) that a fully autonomous AI will be safer than having too many other human decisions involved (that said, it may be that in this particular situation, there shouldn’t be a discretion not to follow an alert; of course under the condition that the AI is highly accurate).
Does the dispatcher need to know how the ML works and the ways it can make mistakes?
Ignoring the alert is a feasible option—if the dispatcher can produce a good reason for ignoring it. In order to provide such a rationale, the dispatchers ought to be educated in the inner working of a ML-model, and how it might produce false alerts.
It is questionable, however, whether it is realistic to assume that the dispatcher will actually ignore the system.
Human Agency and Oversight
The requirement for human agency and oversight seeks to ensure that AI is used to put people in a position to make more informed decisions, not necessarily to make the decisions for them. It specifically recognizes a “right not to be subject to a decision based solely on automated processing when this […] significantly affects them” (AI HLEG, 2019, p. 16).
For this case, the issue of the dispatcher having to distinguish if the alert is valid or not is a major challenge. Support is needed to extract important signals during the call that can be difficult for a human to discern on their own. However, this use case also surfaced many other issues with an AI support system. Is it possible for those who are impacted by the decisions made by AI to challenge them? Is there sufficient human oversight (Hickman and Petrin, 2020)?
It seems that the dispatchers’ agency and autonomous decision-making are reduced by the system. The assumption is that the dispatchers do not primarily rely on their own decision-making but take the system into consideration. However, they do not know what criteria the system uses for its suggestions/advice/decisions.
This is a case of agency in AI where agents and those being impacted (i.e., the wider public) should be better informed about what criteria are used to determine the AI output. In this way, the AI acts as an educator to improve the dispatcher’s abilities.
Insofar, this is supposed to be a shared control system, in which part of the responsibility is conferred to the system, without the dispatchers knowing details about the decision-making criteria or the reliability of the system. The inclusion of the ML system clearly decreases the dispatchers’ autonomy.
This may reduce the dispatchers’ engagement in the process and diminish their sense of agency. What is the basis for dispatchers to decide whether to follow the system’s suggestion or not? Are there any criteria? It could be useful to build a heuristic tool that informs the dispatcher when and when not to rely on the system, for example, to put a disclaimer in place in certain situations (see above).
Furthermore, the question needs to be addressed of how a balance between ML system and dispatcher input in the shared decision-making process can be achieved. Who is it who oversees the process? Is the process controlled by the advice given by some supervisor to dispatchers of whether or not they are supposed to follow the system’s output?
Alternatively, dispatchers could decide not to follow the system’s advice, which would make it obsolete.
Privacy and Data Governance
A data protection impact assessment in accordance with Article 35 GDPR must be carried out for this AI system. According to the General Data Protection Regulation (GDPR), an informed and explicit consent is required for the processing of sensitive data, such as health data. This requirement can be waived, e.g., if the vital interests of the data subject are affected and she or he is incapable to give consent. From a data protection perspective, GDPR requirements are extensive and evolving (European Parliament and Council of European Union, 2016). Specific concerns for this use case include the caller’s lack of awareness (and therefore consent) that an AI system is included in the process and that the call is used for analysis and research.
The possibility of a right to explanation under the GDPR may also pose difficulties to the extent that human understanding of the AI process is limited. A proper legal review will be needed to assess the compliance of the system with the GDPR’s requirements. The benefits that arise from AI are rooted in the processing of large quantities of data. However, this usage evokes inherent conflicts with the protection of privacy and integrity of and access to data. The AI HLEG trustworthy AI guidelines call for data governance policies and systems that “cover [s] the quality and integrity of the data used, its relevance in light of the domain in which the AI systems will be deployed, its access protocols and the capability to process data in a manner that protects privacy”. This raises the challenge of ensuring the use of AI is both transparent and overseen while also ensuring individual privacy (Abbott, 2020).
The goal of the GDPR is the protection of fundamental rights and freedoms of natural persons (Art. 1). These are determined in accordance with the Charter of Fundamental Rights of the European Union and the European Convention on Human Rights. This also includes the right to non-discrimination pursuant to Article 21 Charter of Fundamental Rights. This is relevant for this use case, as the system had more false negatives for people not speaking Danish or with a heavy dialect.
From a data protection perspective, the prime stakeholder of the use case is in charge of fulfilling the legal requirements. From a risk-based perspective, it would be desirable if the developers of the system would also be responsible as they implemented the AI system. But the responsibility of the vendors or developers of a system is not a requirement of the GDPR.
Possible Accountability Issues
For this use case, the AI HLEG trustworthy AI guidelines require “that mechanisms be put in place to ensure responsibility and accountability for AI systems” and emphasizes the importance of redress when unjust adverse impact occurs (AI HLEG, 2019, p. 19f). In matters of human health, particularly those with life or death consequences, as in this use case, the potential harm can be substantial both in non-monetary and in monetary terms. Mechanisms that allow for redress in case of the occurrence of any harm or adverse impact are therefore particularly important.
Accountability, in the form that the AI HLEG trustworthy AI guidelines address them, is arguably, for the most part, non-legal in nature. For instance, accountability in this sense may refer to auditability that enables affected parties to assess algorithms, data and design processes; minimization and reporting of negative impacts; consideration and balancing of trade-offs when there are tensions between various ethical principles; and redress other than liability in the technical sense. Nevertheless, in practice, there will almost inevitably be certain interactions between non-legal “ethical” principles and legal principles, and it is difficult to completely separate the two. In particular, the use case discussed herein raises important medical liability questions (some of which have already been alluded to above).
Due to the diffusion of responsibility that is typical for AI technology, however, the operation of such mechanisms is more complex than in usual medical liability cases. For this use case, different actors (such as the institution using the AI, the manufacturers of the AI, or those in charge of oversight of the AI) could potentially be responsible for the harm. It is therefore very difficult for any injured person to prove specific causation contributions or to show that an AI system was “defective”. In fact, such proof would require knowledge of the details of the AI algorithms’ and the AI models’ implementation—which are, however, proprietary of the company who implemented them (on intellectual property issues, see above).
Accordingly, it is also difficult to put mechanisms in place to provide information to (end-)users and third parties about opportunities for redress, as required by the AI HLEG trustworthy AI guidelines (AI HLEG, 2019, p. 31). As long as the algorithm is unknown, nothing more than general guidelines can be disclosed to these parties. Still, the parties involved in designing, developing, deploying, implementing, and using the AI system should consider how—in line with the AI HLEG trustworthy AI guidelines—they can enhance the accountability factors mentioned above. This could include facilitating audit processes, if appropriate via evaluation by internal and external auditors, and creating avenues for redress apart from the pre-existing legal avenues available to those negatively affected by AI.
Societal and Environmental Well-Being
We consider here broader implications, such as additional costs that could arise from an increase in false positives by the AI system, resulting in unnecessary call taker assisted CPRs, and dispatching ambulances when they are not necessary, and trade-offs, by detracting resources from other areas.
Map Ethical Issues and Flags to Trustworthy AI Areas of Investigation
Our group faced a counterintuitive problem as we began analyzing ethical issues. It was not difficult in locating issues and responding to them. Instead, the challenge was to stop responding. The diversity of our group members opened so many angles and subjects of interest that our project was threatened by too much success: left to our own devices, we would have discussed interminably.
To convert our work from theoretical discussion into practical and applicable results, we took two steps. First, we limited the set of ethical principles and approaches that we would employ. Concretely, we opted for the EC Ethics Guidelines for Trustworthy AI because it is a widely recognized set of principles. We also selected the list of frequent AI ethical tensions cataloged by the Nuffield Foundation (Whittlestone et al., 2019), because they are so well explained and accessible. Second, we forced consensus by having each participant commit their personal thoughts to a short rubric. It required that each ethical dilemma and tension be narrated in our own individual words and then mapped onto the Ethics Guidelines. We found that the structured approach helped funnel our thinking into a single, coherent set of results that we could apply to the case. One drawback of this modular approach is that it does sacrifice some ethical nuance. However, the benefit of a common ethical language and structure for thought is that a sizable group of experts from diverse backgrounds can efficiently work toward a single and useful set of results.
To avoid decision-bias, we distributed the work into four independent Working Groups (WG), created according to the skills and expertize of the various team members. The four working groups are: WG Ethics, WG Law/Healthcare, WG Healthcare and WG ML.
Distinct subgroups adopted different strategies for arriving at an internal consensus, in order to be mindful of relevant cognitive biases for different modes of expertize. The expectation was that distinct paths to consensus may be more or less suited to providing external validity checks on the judgments of particular Z-Inspection® participants. As an example of this procedure, the ethics and law subgroup first selected two volunteers to lead their internal discussions. The wider group of four participants then held 2–3 separate calls to go through the ethical issues already flagged during the Assess Phase by all participants. The subgroup discussed these to see if they were covered by previous work (e.g., GDPR) or not, as well as what assurance(s) had already been given by the team on, for example, data storage and protection. The ethical issues were then given distinctive titles, descriptions, and narratives as needed to make sure they did not overlap with each other. The two subgroup leaders then gave these updated descriptions to the Z-Inspection® lead, where they were joined with the descriptions provided by other subgroups.
While this use case directly refers to the use of ML as a supportive tool to recognize cardiac arrest in emergency calls, there are various ways in which the findings of this qualitative analysis could be applicable to other contexts. First, the general framework for achieving trustworthy AI sets out in the HLEG AI guidelines proved to be an adequate starting point for a specific case study discussion in the healthcare domain. Second, the ethical principles of the HLEG AI guidelines need some context-specific specifications. Third, this contextualization and specification can successfully be undertaken by an interdisciplinary group of researchers that together is able to not only bring in the relevant scientific, medical, ethical, legal, and technological expertize but also to highlight the various facets of the ethical principles as they play out in the respective case.
This layered approach allowed to minimize (cognitive) biases in our assessment approach. Since several groups worked independently, it was not possible that one view would influence all participants, as it would potentially happen in one meeting with all experts.
Each working group worked independently, narrated each discussed ethical dilemma and tension, and flags (i.e., other issues) in their own words, and mapped each one onto ethics principles and tensions. Concretely this meant taking the four pillars of the AI HLEG trustworthy AI guidelines (Respect for Human Autonomy, Prevention of Harm, Fairness, Explicability) and selecting the one the WG found the most apt. Each of those pillars has a number of requirements, from which the WG selected, and then each requirement contains sub-requirements, which were also selected. The list of requirements and sub-requirements is listed below (AI HLEG, 2019):
REQUIREMENT #1 Human Agency and Oversight
Sub-requirements:
Human Agency and Autonomy
Human Oversight
REQUIREMENT #2 Technical Robustness and Safety
Sub-requirements: Resilience to Attack and Security
General Safety
Accuracy
Reliability,
Fall-back plans and Reproducibility
REQUIREMENT #3 Privacy and Data Governance
Sub-requirements:
Privacy
Data Governance
REQUIREMENT #4 Transparency
Sub-requirements:
Traceability
Explainability
Communication
REQUIREMENT #5 Diversity, Non-Discrimination and Fairness
Sub-requirements:
Avoidance of Unfair Bias
Accessibility and Universal Design
Stakeholder Participation
REQUIREMENT #6 Societal and Environmental Well-Being
Sub-requirements: Environmental Well-Being
Impact on Work and Skills
Impact on Society at Large or Democracy
REQUIREMENT #7 Accountability
Sub-requirements:
Auditability
Risk Management
To help the process, especially as a help to experts who might not have sufficient knowledge in ethics, we used a sample of catalog of predefined ethical tensions. We have chosen the catalog defined by the Nuffield Foundations (Whittlestone et al., 2019), indicated in the box below.
Sample Catalog of Ethical Tensions (Whittlestone et al., 2019).Quality of services vs. privacy;
Personalization vs. solidarity;
Convenience vs. dignity;
Privacy vs. transparency;
Accuracy vs. explainability;
Accuracy vs. fairness;
Satisfaction of preferences vs. equality;
Efficiency vs. safety and sustainability.
When a specific “issue” did not correspond to one or more of the predefined ethical tensions, experts described them with their own words. The results of four WGs are then “merged” into one consolidated mapping using a consensus workshop. We present here the consolidated mapping for this use case.
Ethical Issues
ID Ethical Issue: E1, Dispatcher Accept/Reject Prompt
Description
It is unclear whether the dispatcher should be advised or controlled by the AI, and it is unclear how the ultimate decision is made.
Map to Ethical Pillars/Requirements/Sub-Requirements (Closed Vocabulary)
Respect for Human Autonomy > Human Agency and Oversight > Human Agency and Autonomy.
Narrative Response
Importantly, any use of an AIsystem in the healthcare system needs to be accompanied by a clear definition of its use. In the current setting, it is unclear how the decision support tool, should be used by the dispatchers. Should they defer to the tool’s decision (especially since the performance seems to surpass human capabilities)? And if they do not defer to the tool, do they need to justify the decision? We also need to take into account that the dispatchers in Denmark are highly trained professionals that will not easily defer to an automated tool without a certain level of clinical validation and trust in the system. Despite the fact that the dispatchers are the primary users, they were not involved in the system design.
ID Ethical Issue: E2, Caller’s and Patient’s Personally Identifying Information
Description
To what extent is the caller’s personally identifying information protected, and who has access to information about the caller?
Map to Ethical Pillars/Requirements/Sub-Requirements (Closed Vocabulary)
Prevention of Harm > Privacy and Data Governance > Privacy Prevention of Harm > Privacy and Data Governance > Data Governance.
Narrative Response
The main issue here is whether and how the data can be identified and traced back to particular stakeholders. The study participants claimed to follow the GDPR standards put in place by the EU, which in this case did help specify the respective roles of the dispatcher, caller, and AI system. However, these descriptions must be augmented by protections that further specify how data will be used and stored, for how long this will occur before its disposal, and what form(s) of anonymization will be maintained so that only trusted, legitimized parties can access the identifying information directly.
ID Ethical Issue: E3, Informed Consent/Research Ethics Committee
Description
It is unclear whether the study participants should be the medical dispatchers, the patients, or both.
Map to Ethical Pillars/Requirements/Sub-Requirements (Closed Vocabulary)
Respect for Human Autonomy > Fundamental Rights > Human Agency and Autonomy.
Narrative Response
There is a question of whether a research ethics board should have reviewed the study; the need for an ethical approval was waived here by the research ethics committee in the Capital Region of Denmark. Written informed consent was only obtained by the medical dispatchers. However, there is the question of whether there should have been a formal ethical review and a community consultation process, or a form of surrogate or deferred consent, to address the ethical implications regarding trial patients, as is common in comparable studies reviewed by institutional review boards in the United States and United Kingdom.
ID Ethical Issue: E4, Fairness in the Training Data
Description
The training data is likely not sufficient to account for relevant differences in languages, accents, and voice patterns, potentially generating unfair outcomes.
Map to Ethical Pillars/Requirements/Sub-Requirements (Closed Vocabulary)
Fairness > Diversity, Non-Discrimination and Fairness > Avoidance of Unfair Bias.
Narrative Response
There is likely empirical bias since the tool was developed in a predominantly white Danish patient group. It is unclear how the tool would perform in patients with accents, different ages, sex, and other specific subgroups. There is also a concern that this tool is not evaluated for fairness with respect to outcomes in a variety of populations. Given the reliance on transcripts, non-native speakers of Danish may not have the same outcome. It was reported that Swedish and English speakers were well represented but would need to ensure a broad training set. It would also be important to see if analyses show any bias in results regarding age, gender, race, nationality, and other sub-groups. The concern is that the training data may not have a diverse enough representation.
ID Ethical Issue: E5, Potential Harm Resulting From Tool Performance
Description
The tool’s characteristic performance, such as a higher rate of false positives compared to human dispatchers, could adversely affect health outcomes for patients.
Map to Ethical Pillars/Requirements/Sub-Requirements (Closed Vocabulary)
Prevention of Harm > Technical Robustness and Safety > Accuracy.
Narrative Response
The algorithm did not appear to reduce the effectiveness of emergency dispatchers but also did not significantly improve it. The algorithm, in general, has a higher sensitivity but also leads to more false positives. There should be a firm decision on thresholds for false positive vs. false negatives. The risk of not doing CPR if someone needs CPR exceeds the risk of doing CPR if not needed. On the other hand, excessive false positives put a strain on healthcare resources by sending out ambulances and staff to false alarms. This potentially harms other patients in need of this resource. The gold standard to assess whether the tool is helpful for the given use case is to analyze its impact on outcome. Given, however, the low likelihood of survival from out of hospital cardiac arrest, there wasn’t an analysis attempting to assess the impact on survival, as it would take years in a unicentric study.
ID Ethical Issue: E6, The AI tool is not Interpretable
Description
The system outputs cannot be interpreted, leading to challenges when dispatcher and tool are in disagreement.
Map to Ethical Pillars/Requirements/Sub-Requirements (Closed Vocabulary)
Explicability > Transparency > Explainability.
Narrative Response
The tool lacks explainability, which might lead to several challenges. First, outcomes are based on a transcription of the conversation between dispatcher and caller. It is not clear what is used from these transcripts to trigger an alert. This lack of transparency may have contributed to the noted lack of trust among the dispatchers, as well as the limited training of the users. Second, there is a lack of transparency regarding whether and which value judgments went into the design of the model. Such value judgments are important because explaining the output is partly a matter of accounting for the design decisions that humans have made.
ID Ethical Issue: E7, Cybersecurity
Description
The model may suffer from security vulnerabilities due to cyber attacks.
Map to Ethical Pillars/Requirements/Sub-Requirements (Closed Vocabulary)
Prevention of Harm -> Technical Robustness and Safety -> Resilience to Attack and Security.
Narrative Response
The data should also be adequately protected against potential cyber-attacks. In particular, since the model is not interpretable, it seems hard to determine resistance to adversarial attack scenarios, such as the importance of age, gender, accents, bystander’s type, etc.
ID Ethical Issue: E8, Utility of the System
Description
The added value of the system to particular stakeholders is not clear.
Map to Ethical Pillars/Requirements/Sub-Requirements (Closed Vocabulary)
Prevention of Harm > Societal and Environmental Wellbeing > Impact on Society at Large or Democracy.
Narrative Response
The AI system did not significantly improve the dispatcher’s ability to recognize cardiac arrests. AIs should improve medical practice rather than disrupting it or making it more complicated. Where should the line be drawn? How much improvement is needed to conclude that an AI system should be deployed in clinical practice? Will it be cost-effective (worth the electric bill, energy, and compute power) to run the ML model? What would it take to guarantee this?
ID Ethical Issue: E9, Design of Clinical Trials
Description
The trials conducted did not include a diverse group of patients or dispatchers.
Map to Ethical Pillars/Requirements/Sub-Requirements (Closed Vocabulary)
Fairness > Diversity, Non-Discrimination and Fairness > Stakeholder Participation.
Narrative Response
Clinical trials are rare in the field of AI and are certainly welcomed. The design of the trial needs to be carefully considered and thoroughly thought through in consideration of stakeholder priorities, concerns, and active participation.
ID Ethical Issue: E10, Unclear Tool Definition and Safety Assessment
Description
It is unclear whether the tool is a medical device or not and whether its safety was sufficiently assessed by the involved ethics committees and authorities.
Map to Ethical Pillars/Requirements/Sub-Requirements (Closed Vocabulary)
Prevention of Harm > Technical robustness and safety > General safety.
Narrative Response
It is unclear whether the tool is a medical device or not. Thus, it is also unclear whether the clinical studies should have fallen under medical device regulation. It is thus also unclear whether the Danish authorities and the involved ethics committees assessed the safety of the tool sufficiently.
This is the list of the consolidated tensions.
Tensions
We define “true dilemma” as a tension between values that is general to any AI system faced with the problem at hand. We define “dilemma in practice” as a tension specific to the affordances and features of this particular system. We define “false dilemma” as an apparent tension between values that, in fact, could be readily resolved through a canonical technical procedure that is known to work in this domain (Whittlestone et al., 2019).
ID Ethical Tension (Open Vocabulary): ET1
Kind of tension: True Dilemma.
Trade-off: Autonomy vs. Accuracy.
Description: Autonomous AI system may or may not be more accurate than with the interaction of a human dispatcher (support system).
ID Ethical Tension (Open Vocabulary): ET2
Kind of tension: True dilemma.
Trade-off: Privacy vs. Accuracy.
Description: The more data, the better the AI system will likely perform. However, there is a challenge to adequately protect and maintain the privacy of the individuals.
ID Ethical Tension (Open Vocabulary): ET3
Kind of tension: Dilemma in practice.
Trade-off: Autonomy vs. Quality of Services.
Description: There is a question of who is the participant - the dispatcher and/or caller/patient. If it is the caller/patient, their autonomy should be respected and informed consent be obtained according to best practices for emergency medicine.
ID Ethical Tension (Open Vocabulary): ET4
Kind of tension: True dilemma.
Trade-off: Fairness vs. Accuracy.
Description: The algorithm is accurate on average but may systematically discriminate against specific minorities of callers and/or dispatchers due to ethnic and gender bias in the training data.
ID Ethical Tension (Open Vocabulary): ET5
Kind of tension: True dilemma.
Trade-off: Safety vs. Efficiency.
Description: There is a risk of incorrect diagnosis and intervention arising from false positives, relative to that provided by human dispatchers.
ID Ethical Tension (Open Vocabulary): ET6
Kind of tension: True dilemma.
Trade-off: Accuracy vs. Explainability.
Description: The tool lacks explainability but explainable AI systems may be less accurate than non-interpretable models.
ID Ethical Tension (Open Vocabulary): ET7
Kind of tension: True dilemma.
Trade-off: Security vs. Accessibility.
Description: The system should be transparent and available to various stakeholders, but also must have safeguards to resist external threats that may limit transparency conditions.
ID Ethical Tension (Open Vocabulary): ET8
Kind of tension: True dilemma.
Trade-off: Utility vs. Economic Interests.
Description: AI systems should be effective and improve medical interventions without unnecessary disruption (e.g., hospital workflow).
ID Ethical Tension (Open Vocabulary): ET9
Kind of tension: True dilemma.
Trade-off: Safety vs. Convenience.
Description: Clinical trials are rare in the AI field, but could ensure that the devices are safe and effective. However, there is a tension that clinical trials are time-consuming and costly and cannot be provided by manufacturers to the necessary degree.
Challenges of Mapping the HLEG AI Guidelines to this Specific Case
A challenge is related to making the abstract principles formulated in the guidelines applicable to the respective case study. This almost certainly will involve some narrowing down of the broad concepts (such as autonomy or privacy) reflected in the principles to conceptions that prove useful in the case study. While from a pragmatic point of view, this seems adequate, both for time constraints and in view of the interdisciplinary nature of the group of researchers, it certainly limits the philosophical, ethical, and legal bandwidth and depth of the discussion.
Despite the broad multidisciplinary expertize of our Z-Inspection® assessment team, it was surprising how challenging it was to map broad general guidelines to a concrete and specific use case. This highlights that all institutions tasked with assessing and regulating AI in healthcare products should exhibit two important characteristics: On the one hand, flexibility in assessing the solution at hand. It is likely that different solutions will have very different advantages and challenges. Hardcoding certain requirements into regulation is thus probably not a recommended way forward. On the other hand, this requires broad and wide expertize in all areas related to AI in healthcare.
The Resolve Phase: Verification of Requirements
As a next step, we will work on task IV verification of requirements of the Assess Phase. Here, the goal is to start from the list of consolidated ethical and technical and legal issues, to prioritize them by urgency, verify any claims, and as a result of this verification, give feedback to the expert teams so that they can possibly revise the final list of ethical issues and tensions, and then produce some recommendations.
To verify claims, we plan to use a mixed approach, consisting in adapting concepts from the Claims, Arguments, Evidence (CAE) framework and using the ALTAI web tool. CAE is often used as a framework in aviation, nuclear, and defense industries to reason about safety, security, reliability, and dependability. Recent work has begun applying CAE to the safety analysis of AI systems (Brundage et al., 2020; Zhao et al., 2020). We will adjust the concepts to apply to the seven requirements for trustworthy AI.
The ALTAI web tool (AI HLEG, 2020) is an interactive general self-assessment beta prototype tool based on the EU trustworthy AI framework. The tool is not specific to the domain of healthcare. It gives only general recommendations and produces a generic score. We are already experienced in using it. We will adapt the general recommendations resulting from the tool and take into account the results of the verification phase, the final list of ethical issues and tensions, and then produce specific recommendations relevant for the domain of healthcare, and for this case in particular.
The output of the assessment will be a report containing recommendations to the key stakeholders. Such recommendations should be considered a source of qualified information that help decision makers make good decisions, and that help the decision-making process for defining appropriate trade-offs. They would also help continue the discussion by engaging additional stakeholders in the decision-process.
We list here a preliminary list of recommendations for this use case.
Recommendation 1: It is important to ensure that dispatchers understand the model predictions so that they can identify errors and detect biases that could discriminate against certain populations. Here, the model is a statistical black-box, and the clinical trial conducted with the model showed an important lack of trust that had an impact on the outcome of the trial. An improvement to the model would include interpretable local approximations [such as SHAP (Lundberg and Lee, 2017)], which are easy for stakeholders to understand and provide different levels of interpretation for judging the relevance of an individual prediction. In our example, explanation may involve words that were more predictive, tone of voice, or breath sounds.
Recommendation 2: We believe that the team should either intentionally sample the entire training set in order to prevent discrimination, or define a heuristic that could inform dispatchers when to use and when not to use the model. Feeding the ML system with a data set that is built to more adequately represent the whole population would avoid bias toward older males and better take all genders, ages, and ethnicities into consideration and would make the system work better with dialects and non-native speakers. An approach like this would be in analogy to what has been recommended for facial recognition technology applications (Buolamwini and Gebru, 2018). Alternatively, the use of the ML system could be confined to those cases that are inside the training distribution, and in the other cases, signal a disclaimer to the dispatchers. This would allow the dispatchers to better identify the cases where to rely on their own decision-making and prevent them from being overturned by the system when the system lacks reliability. This approach would increase dispatcher autonomy and could improve the overall outcome.
Recommendation 3: Involve stakeholders. The group of (potential future) patients and (potential future) callers could be interested in how the system functions and is developed. User involvement/stakeholder involvement could be very helpful in the process of re-designing the AI system.
Recommendation 4: It is important to learn how the protocol (what questions, how many, etc.) does or does not influence the accuracy of the ML output. Further research work should be performed to answer this question. The goal should be to responsibly integrate the classifier into the context of the dispatcher calls rather than just have it passively observe the call and make “trustworthy” recommendations. This requires reimagining the context of the calls themselves (with new protocols, questions, etc.).
Recommendation 5: Although we did not assess the legal aspects of the AI system, we suggest to the prime stakeholder to verify with legal local competent authorities if the AI system needed a CE-certification as a medical device, according to the definition of current regulation Medical Device Directives (MDD), which was transposed into Danish Law. In the new forthcoming Medical Device Regulation (MDR) in the EU, which will apply from May 26, 2021, “software that is used for human beings for the medical purpose of prediction or prognosis of disease will be classified as a medical device.” Under the MDR, the AI system will be classified as medical device, and it would therefore need a CE-certification.
Human-Machine Interaction and Legal Perspective
Human-Machine Interaction
To put this into a broader context, one of the main problems debated in ethics, in the field of human-machine interaction, is the possible complete “replacement” of the human decision-making capacity. This is recognition of the principle of human dignity, in a human-centric approach, and the principle of non-maleficence (do no harm to humans) and beneficence (do good to humans) in ethics. Human involvement in design and construction is not enough to discharge this concern. Humans need to maintain a level of control and oversight over the AI, allowing it to cognitively assist human decisions, not become a substitute for them. Machines should not compete but complete human actions. The AI HLEG trustworthy AI guidelines requirement for human agency and oversight tacitly acknowledges this debate and seeks to ensure that AI is used to inform decision making, not make the decisions.
The AI HLEG trustworthy AI guidelines specifically recognize a “right not to be subject to a decision based solely on automated processing when this [...] significantly affects them” (AI HLEG, 2019, p. 16). In order to comply with this requirement, a certain level of human involvement is necessary. As such, the first issue to consider from a human agency and oversight perspective will be what the appropriate level of human involvement is.
Different levels of human oversight in AI have been categorized as human in the loop, human on the loop, or human in command. Human in command describes a high level of human involvement, with human in the loop and on the loop incrementally less. To the extent a high level of human involvement is deemed necessary from an ethical standpoint, this will necessarily reduce some of the benefits the AI system was intended to bring (Hickman and Petrin, 2020). On the other hand, minimal human oversight raises concerns regarding monitoring of the accuracy of the AI system and potential harm and liability that could result. There is a trade-off between the efficiency gains of the AI and the ability to oversee its decisions. The appropriate balance for this trade-off needs to be part of the assessment.
A second issue relating to human involvement is the impact of the presence of AI in the process and its perception by the humans charged with overseeing it (supervisors) and processing its output (dispatchers). For both supervisors and dispatchers, if there is a perception that the AI is not often wrong, heuristics suggest that they will be less likely to spot the anomalies when they arise. Conversely, the AI may frequently be wrong.
In the randomized clinical trial, less than one in five alerts were true positives, raising the possibility of alert fatigue, which could result in true alerts being ignored. These potential issues, and others like them, will need to be identified and methods to resolve them will need to be explored. Such resolutions could include, for example, (Blomberg et al., 2021) a heuristic tool that informs the dispatcher when and when not to rely on the system, for example, to put a disclaimer in place in certain situations.
Relatedly, a relational conception of human dignity, which is characterized by our social relations, requires that we should be aware of whether and when we are interacting with a machine or another human being and that we reserve the right to vest certain tasks to the human or the machine. In this ethical framework, the ethics of AI is the ethics of human beings: the machine cannot obscure the agency, which is human. Humans conceive, design, use AI, and humans should be kept at the center (human-centric approach).
The need to keep human oversight also remains essential in order to avoid the possible problem of technological delegation. An AI system that becomes optimal in suggesting “decisions” to humans (also in medicine, e.g., as in this case) poses the risk of decreasing human attention with the possible consequence of reducing human skills (the so-called phenomenon of de-skilling or de- professionalization), reducing responsibility. In this sense, it is important to frame a complementarity between man and machine, searching for ways of intelligent “support” that allows man to have “significant or meaningful human control”8 in terms of attention, contribution, supervision, control, and responsibility.
Legal Perspective
The general debate on AI in healthcare has identified a great variety of legal issues concerning, inter alia, intellectual property (IP), privacy and data protection (Ford and Price, 2016), product safety and cybersecurity (Tschider, 2018), liability risks due to negligence claims (Kerr et al., 2017; Price et al., 2021, 2019), and, more specifically, the applicability of medical device regulation (Gerke, Minssen, et al., 2020; Kiseleva, 2019). On a more general level, even tax, trade, and non-discrimination laws need to be observed (Puaschunder, 2019). The assessment of trustworthy AI cannot take all these manifold legal implications into account, and it therefore cannot replace a proper legal, due diligence review. However, it can and does provide a plausibility check with a focus on the most specific legal challenges. (See section on Possible Accountability Issues).
AI system (for an overview, see for example European Commission. Directorate General for Justice and Consumers (2019)). Such liability can be both civil and criminal in nature. Generally, regarding civil liability, this could be based on contractual and non-contractual theories, with the latter including general tort law and – if, which is not certain, an AI system is or will be classified as a product in the legal sense – product liability principles. The latter are governed both by harmonized EU principles and national/domestic law, that is in the present use case, presumably mainly Danish law. In the future, there will likely be new laws specifically geared towards liability for AI. Indeed, the European Parliament has recently drafted a resolution with recommendations to the Commission concerning civil liability relating to an liability for AI (European Parliament resolution of 20 October 2020 with recommendations to the Commission on a civil liability regime for artificial intelligence (2020/2014(INL)).
In terms of relevant parties, individuals and/or legal entities involved in designing, developing, importing, distributing, selling, and using AI, among other roles, are potentially exposed. Indeed, we could even imagine that not using AI may – if not now, then at least in the future – lead to liability (Price et al., 2019). More specifically, for the present use case, parties that are potentially exposed to liability include (in addition to the parties mentioned above) the hospital, dispatchers, and staff. Further, it is even possible to imagine scenarios where the person who called the emergency number or other bystanders might face liability for incorrectly or unnecessarily attempting resuscitation of patients. It is important to note that liability issues, as well as any other legal issues relevant to AI and its specific use cases, tend to be jurisdiction-specific, that is governed by local laws in the relevant country or countries to which there are pertinent connections (such as the AI is being used there, has caused harm, etc.). As mentioned above, it is therefore critical for those involved in the use case discussed herein to ensure that an in-depth legal analysis by lawyers qualified to advise on legal matters in the relevant jurisdiction(s) and with specialist knowledge in the various subject areas is conducted, and that any insights therefrom will be considered when implementing the AI system.
The previous discussion assumes that the AI system in this use case has met all legal requirements for introducing it to the market. Whether that is the case is governed by another set of specific rules. For this case, we assume that the Danish legal framework applies. It is based on the EU Commission's guidance MEDDEV 2.1/6 2012 - Qualification and Classification of stand alone software9 and the Danish executive order from 15/12/2008 about medical devices10 .
The Danish executive order implements the EU Council ‘Medical Device Directive 93/42/EEC’ into Danish law. The responsible authority is the Danish Medicines Agency (DKMA) under the Danish Health Authority. According to DKMA, no requirements in the Danish Executive order go beyond what is stated in the EU directive11.
The Danish executive order defines a medical device as “any instrument, apparatus, appliance, software, material or other article, whether used alone or in combination, including the software intended by its manufacturer to be used specifically for diagnostic or therapeutic purposes and necessary for its proper application, intended by the manufacturer to be used for human beings for the purpose of: a) diagnosis, prevention, monitoring, treatment or alleviation of disease (...)”12.
Importantly, as stated, whether a device is within the definition of the executive order depends on the intended purpose of the device, which is defined by the manufacturer. If so, the device “must fulfill the requirements in the applicable legislation and classified according to risk and CE marked”13. Some of these requirements are: a process for the development and design of safe products; clinical evaluation; risk analysis; labeling and information about the manufacturer; instructions in Danish; and an established market surveillance system.
Limitations
Among the various audit frameworks, ethics as a service approaches or impact assessment tools for AI systems, every one of them has its limitations and shortcomings. With Z-Inspection®, it is no different. Although the method has several great strengths (Zicari et al., 2021), among them the lack of conflicting interests on the side of the members, the interdisciplinary approach, the broad scope of the framework etc., it also has an important limitation:
The evaluation cannot guarantee that the organization administering the AI system in question necessarily sticks to the recommendations that are given. However, since participation in the inspection is voluntary, organizations come with a high openness for proposed changes.
A requirement for AI systems that is becoming more and more salient is that their computing power should be also estimated (Strubell et al., 2019). If we consider specifically this use case, during the assessment we had no access to information on the energy requirements during model training, and therefore we cannot give recommendations in this respect.
Conclusion and Future Work
The best practice defined in this paper illustrates that our holistic and multidisciplinary evaluation process can be used to evaluate the risks and identify the ethical tensions arising from the use of the AI system and can also be used to improve current or future versions of an AI system.
AI systems can raise ethical and societal concerns from direct stakeholders, such as patients in healthcare, and from indirect stakeholders such as politicians or general media. The nature of these concerns can vary and include a vast array of topics like data security, biases, cost-benefit-debates, technical dependencies, or technical supremacy. The interdisciplinary approach of the evaluation can help to identify these concerns in many different fields, already at very early development stages.
Evaluation of AI development with a holistic approach like Z-Inspection® creates benefits related to general acceptance or concerns inside and outside the institution that applies an AI project. The approach can improve the quality of the project’s processes and increase transparency about possible conflicts of interest. In general, the system becomes more comprehensible, which improves the quality of communication for any kind of stakeholder.
For the public, communicating the evaluation process itself can help reinforce trust in such a system by making its exact workings transparent, even to non-specialist project staff. This transparency helps funders, oversight boards, and executive teams explain their decisions about funding and governing decisions as well as the system’s operation.
An important lesson from this use case is that there should be some requirement that independent experts can assess the system before its deployment. This seems to be relevant in order to determine its trustworthiness in the first place as a means toward sociotechnical validation of the AI system.
One way to understand this use case we have been looking at is to see the “medical discussions” as possible forms of validation metrics rather than simply mechanisms for “verifiable claims,” as OpenAI has recently argued (Brundage et al., 2020).
Instead of formal verification procedures concerned with matching the model to the proposed specification, empirical validation would investigate whether the specification itself is well-founded, treating the model parameters as “hypotheses” that must be tested against real-world conditions under controlled settings (Dobbe et al., 2019).
So the question for our inspection isn’t just making AI systems that are trustworthy, but making sure the proposed trustworthiness definition actually matches the expectations of affected parties, and drawing attention to the way particular use cases highlight discrepancies within and across stakeholder groups and point to a need for further validation and regulation through clinical standards.
While this use case directly refers to the use of machine learning as a supportive tool to recognize cardiac arrest in emergency calls, there are various ways in which the findings of this qualitative analysis could be applicable to other contexts. First, the general framework for achieving trustworthy AI set out in the HLEG AI guidelines proved to be an adequate starting point for a specific case study discussion in the healthcare domain. Second, the ethical principles of the HLEG AI guidelines need some context-specific specification. Third, this contextualization and specification can successfully be undertaken by an interdisciplinary group of researchers that together are able to not only bring in the relevant scientific, medical and technological expertize but also to highlight the various facets of the ethical principles as they play out in the respective case.
The Peril of Inaccurate Inspection
There is a danger that a false or inaccurate inspection will create natural skepticism by the recipient, or even harm them and, eventually, backfire on the inspection method. There are also legal issues (some of which are addressed in the Human-Machine interaction and Legal perspective Section). This is a well-known problem for all quality processes. We alleviated it using an open development and incremental improvement to establish a process and brand (“Z-Inspected”).
In Memoriam
Our team member, colleague and friend Naveed Mushtaq has passed away on December 27, 2020, after suffering a sudden cardiac arrest a few weeks before. This work is dedicated to him.
Data Availability Statement
Proprietary datasets were analyzed in this study. This data can be obtained on request by contacting the authors.
Author Contributions
Conception/design—all authors; JB, MC, MG, SG, TG, VM, ES, AS, JT, DV, MW were the leaders of the four working groups, and were responsible for producing the consolidated mapping of Ethical Issues and Tensions; EH, FM, LP, MP prepared the Supplementary Materials; paper preparation—RZ; paper editing—SG, WO, DV, MW, RW, JA, EC, AG, CH, TH, MO, HV; final approval—all authors.
Funding
SG was supported by a grant from the Collaborative Research Program for Biomedical Innovation Law, a scientifically independent collaborative research program supported by a Novo Nordisk Foundation grant (NNF17SA0027784). JA received funding from the European Union’s Horizon 2020 research and innovation program under grant agreement No. 777107 (PRECISE4Q). TH was supported by the Cluster of Excellence “Machine Learning—New Perspectives for Science” funded by the Deutsche Forschungsgemeinschaft (DFG, German Research Foundation) under Germany’s Excellence Strategy—Reference Number EXC 2064/1—Project ID 390727645. All other authors did not receive any funding (neither private nor public) to conduct this work.
Conflict of Interest
Author RW was employed by the company Fitbiomics. Author DO was employed by the company Inrupt.
The remaining authors declare that the research was conducted in the absence of any commercial or financial relationships that could be construed as a potential conflict of interest.
Acknowledgments
Many thanks to John Brodersen, Anne Riechert and Leonardo Espinosa-Leal for proving valuable comments on this manuscript. Thanks also to Irmhild van Halem, Timo Eichhorn, Todor Ivanov, Melissa McCullough, Karsten Tolle, Gemma Roig, and Norman Stürtz for their invaluable contribution to the definition of the Z-Inspection® process.
Footnotes
1Z-Inspection® is a registered trademark.
2Another relevant document at EU level is the European Group on Ethics in Science and New Technologies (EGE) at the European Commission, Statement on AI, Robotics and “Autonomous Systems,” Brussels, March 2018 https://ec.europa.eu/research/ege/pdf/ege_ai_statement_2018.pdf
3In the EGE document, the ethical principles proposed are: 1. Human Dignity (meaningful human control and awareness with the interaction with humans or machines); 2. Autonomy; 3. Responsibility; 4. Justice, Equity and Solidarity; 5. Democracy; 6. Rule of law and Accountability; 7. Security and Safety; 8. Data protection and Privacy; 9. Sustainability.
4To describe inclusion of different scientific disciplines in the same project, various terms exist, such as multidisciplinary, transdisciplinary, and interdisciplinary (Frodeman et al., 2012; Budtz Pedersen et al., 2015). Our approach is most accurately described as “interdisciplinary” since the research is developed in between disciplines about a research question formulated from within the research group.
5What exactly are conflicts of interest? While it is hard to find a universal definition, a common denominator is that conflicts of interest arise when personal interests interfere with requirements of institutional roles or professional responsibilities (Komesaroff et al., 2019). Here, interests can be seen as goals that are aligned with certain financial or non-financial values that have a particular, possibly detrimental effect on decision-making. Coexistence of conflicting interests results in incompatibility of two or more lines of actions. In modern research settings, dynamic and complex constellations of conflicting interests frequently occur (Hagendorff and Meding, 2020).
6https://www.retsinformation.dk/eli/lta/2019/903, LBK nr 903 af 26/08/2019
8The principle of Meaningful Human Control was first suggested in the field of weapon systems. This means that humans - and not computers and their algorithms - should ultimately remain in control, and thus be morally responsible. But now, it is also used with reference to human oversight.
9https://ec.europa.eu/docsroom/documents/17921.
10https://www.retsinformation.dk/eli/lta/2008/1263.
11https://laegemiddelstyrelsen.dk/en/devices/legislation-and-guidance/guidance/guidance-for-manufacturers-on-health-apps-and-software-as-medical-devices/#.
12https://laegemiddelstyrelsen.dk/en/devices/legislation-and-guidance/guidance/guidance-for-manufacturers-on-health-apps-and-software-as-medical-devices/.
13https://laegemiddelstyrelsen.dk/en/devices/legislation-and-guidance/guidance/guidance-for-manufacturers-on-health-apps-and-software-as-medical-devices/.
References
Abbott, R. (2020). The Reasonable Robot: Artificial Intelligence and the Law. Cambridge University Press. doi:10.1017/9781108631761
AI, HLEG (2019). High-Level Expert Group on Artificial Intelligence. Ethics guidelines for trustworthy AI [Text]. European Commission. Available at: https://ec.europa.eu/digital-single-market/en/news/ethics-guidelines-trustworthy-ai.
AI, HLEG (2020). High-Level Expert Group on Artificial Intelligence. Assessment List for Trustworthy Artificial Intelligence (ALTAI) for self-assessment [Text]. European Commission. Available at: https://ec.europa.eu/digital-single-market/en/news/assessment-list-trustworthy-artificial-intelligence-altai-self-assessment.
Bærøe, K., Miyata-Sturm, A., and Henden, E. (2020). How to Achieve Trustworthy Artificial Intelligence for Health. Bull. World Health Organ. 98 (4), 257–262. doi:10.2471/BLT.19.237289
Berdowski, J., Berg, R. A., Tijssen, J. G. P., and Koster, R. W. (2010). Global Incidences of Out-Of-Hospital Cardiac Arrest and Survival Rates: Systematic Review of 67 Prospective Studies. Resuscitation 81 (11), 1479–1487. doi:10.1016/j.resuscitation.2010.08.006
Biddle, J. B. (2020). On Predicting Recidivism: Epistemic Risk, Tradeoffs, and Values in Machine Learning. Can. J. Philos., 1–21. doi:10.1017/can.2020.27
Blomberg, S. N., Christensen, H. C., Lippert, F., Ersbøll, A. K., Torp-Petersen, C., Sayre, M. R., et al. (2021). Effect of Machine Learning on Dispatcher Recognition of Out-Of-Hospital Cardiac Arrest during Calls to Emergency Medical Services. JAMA Netw. Open 4 (1), e2032320. doi:10.1001/jamanetworkopen.2020.32320
Blomberg, S. N., Folke, F., Ersbøll, A. K., Christensen, H. C., Torp-Pedersen, C., Sayre, M. R., et al. (2019). Machine Learning as a Supportive Tool to Recognize Cardiac Arrest in Emergency Calls. Resuscitation 138, 322–329. doi:10.1016/j.resuscitation.2019.01.015
Brundage, M., Avin, S., Wang, J., Belfield, H., Krueger, G., Hadfield, G., et al. (2020). Toward Trustworthy AI Development: Mechanisms for Supporting Verifiable Claims. ArXiv:2004.07213 [Cs]. Available at: http://arxiv.org/abs/2004.07213. doi:10.5772/intechopen.90859
Budtz Pedersen, D., Stjernfelt, F., and Køppe, S. (2015). Kampen Om Disciplinerne: Viden Og Videnskabelighed I Humanistisk Forskning.
Buolamwini, J., and Gebru, T. (2018). Gender Shades: Intersectional Accuracy Disparities in Commercial Gender Classification. New York, NY: Conference on Fairness, Accountability and Transparency, 77–91. Available at: http://proceedings.mlr.press/v81/buolamwini18a.html.
Callaway, C. W., Donnino, M. W., Fink, E. L., Geocadin, R. G., Golan, E., Kern, K. B., et al. (2015). Part 8: Post-Cardiac Arrest Care. Circulation 132 (18_Suppl. l_2), S465–S482. doi:10.1161/CIR.0000000000000262
Cummins, R. O., Ornato, J. P., Thies, W. H., and Pepe, P. E. (1991). Improving Survival from Sudden Cardiac Arrest: The “Chain of Survival” Concept. A Statement for Health Professionals from the Advanced Cardiac Life Support Subcommittee and the Emergency Cardiac Care Committee, American Heart Association. Circulation 83 (5), 1832–1847. doi:10.1161/01.CIR.83.5.1832
Deloitte (2020). The Socio-Economic Impact of AI in Healthcare, 48. Available at: https://www.medtecheurope.org/resource-library/the-socio-economic-impact-of-ai-in-healthcare-addressing-barriers-to-adoption-for-new-healthcare-technologies-in-europe/.
(DHR) Dansk Hjertestopregister (2020). (n.d.). Dansk Hjertestopregister. Retrieved February 8, 2021, from Available at: https://hjertestopregister.dk/.
Dobbe, L., Rahman, R., Elmassry, M., Paz, P., and Nugent, K. (2019). Cardiogenic Pulmonary Edema. Am. J. Med. Sci. 358 (6), 389–397. doi:10.1016/j.amjms.2019.09.011
Drennan, I. R., Geri, G., Brooks, S., Couper, K., Hatanaka, T., Kudenchuk, P., et al. (2021). Diagnosis of Out-Of-Hospital Cardiac Arrest by Emergency Medical Dispatch: A Diagnostic Systematic Review. Resuscitation 159, 85–96. doi:10.1016/j.resuscitation.2020.11.025
Düdder, B., Möslein, F., Stürtz, N., Westerlund, M., and Zicari, R. V. (2020). “Ethical Maintenance of Artificial Intelligence Systems,” in Artificial Intelligence for Sustainable Value Creation. Editors M. Pagani, and R. Champion (Cheltenham, UK: Edward Elgar Publishing. To appear).
Eisenberg, M., Lippert, F. K., Castren, M., Moore, F., Ong, M., Rea, T., et al. (2018). The Global Resuscitation Alliance. doi:10.7591/9781501719783 https://www.globalresuscitationalliance.org/wp-content/pdf/acting_on_the_call.pdf. Acting on the Call, 2018 Update from the Global Resuscitation Alliance.
Europe, Med. Tech. (2019). Trustworthy Artificial Intelligence (AI) in Healthcare. Available at: https://www.medtecheurope.org/resource-library/trustworthy-ai-in-healthcare/.
European Commission (2019). Directorate General for Justice and ConsumersLiability for Artificial Intelligence and Other Emerging Digital Technologies. Brussels, Belgium: Publications OfficeAvailable at: https://data.europa.eu/doi/10.2838/25362.
European Parliament, & Council of European Union (1993). Council Directive 93/42/EEC of 14 June 1993 Concerning Medical Devices. Official J. Eur. Communities, L 169, 1–43.
European Parliament, & Council of European Union (2016). GDPR) Regulation (EU) 2016/679 of the European Parliament and of the Council of 27 April 2016 on the protection of Natural Persons with Regard to the Processing of Personal Data and on the Free Movement of Such Data, and Repealing Directive 95/46/EC (General Data Protection Regulation). Official J. Eur. Union, L 119, 1–88.
Ford, R. A., and Price, W. N. I. (2016). Privacy and Accountability in Black-Box Medicine. Mich. Telecommunications Tech. L. Rev. 23, 1.
Gerke, S., Babic, B., Evgeniou, T., and Cohen, I. G. (2020a). The Need for a System View to Regulate Artificial Intelligence/machine Learning-Based Software as Medical Device. Npj Digit. Med. 3 (1), 1–4. doi:10.1038/s41746-020-0262-2
Gerke, S., Minssen, T., and Cohen, G. (2020b). “Ethical and Legal Challenges of Artificial Intelligence-Driven Healthcare,” in Artificial Intelligence in Healthcare. Editors A. Bohr, and K. Memarzadeh (Academic Press), 295–336. doi:10.1016/B978-0-12-818438-7.00012-5
Gräsner, J.-T., Wnent, J., Herlitz, J., Perkins, G. D., Lefering, R., Tjelmeland, I., et al. (2020). Survival after Out-Of-Hospital Cardiac Arrest in Europe - Results of the EuReCa TWO Study. Resuscitation 148, 218–226. doi:10.1016/j.resuscitation.2019.12.042
Grote, T., and Berens, P. (2020). On the Ethics of Algorithmic Decision-Making in Healthcare. J. Med. Ethics 46 (3), 205–211. doi:10.1136/medethics-2019-105586
Hagendorff, T., and Meding, K. (2020). Ethical Considerations and Statistical Analysis of Industry Involvement in Machine Learning Research. ArXiv:2006.04541 [Cs]. Available at: http://arxiv.org/abs/2006.04541. doi:10.1007/s00146-020-01045-4
Haley, K. B., Lerner, E. B., Pirrallo, R. G., Croft, H., Johnson, A., and Uihlein, M. (2011). The Frequency and Consequences of Cardiopulmonary Resuscitation Performed by Bystanders on Patients Who Are Not in Cardiac Arrest. Prehosp. Emerg. Care 15 (2), 282–287. doi:10.3109/10903127.2010.541981
Hasselqvist-Ax, I., Riva, G., Herlitz, J., Rosenqvist, M., Hollenberg, J., Nordberg, P., et al. (2015). Early Cardiopulmonary Resuscitation in Out-Of-Hospital Cardiac Arrest. N. Engl. J. Med. 372 (24), 2307–2315. doi:10.1056/NEJMoa1405796
Havtorn, J. D., Latko, J., Edin, J., Borgholt, L., Maaløe, L., Belgrano, L., et al. (2020). MultiQT: Multimodal Learning for Real-Time Question Tracking in Speech. ArXiv:2005.00812 [Cs, Eess]. Available at: http://arxiv.org/abs/2005.00812.
Hickman, E., and Petrin, M. (2020). Trustworthy AI and Corporate Governance - the EU's Ethics Guidelines for Trustworthy Artificial Intelligence from a Company Law Perspective. SSRN J. doi:10.2139/ssrn.3607225
J. Keulartz, M. Korthals, M. Schermer, and T. Swierstra (2002). in Pragmatist Ethics for a Technological Culture (Springer Netherlands), Vol. 3. doi:10.1007/978-94-010-0301-8
Johan, Holmén., Johan, Herlitz., Sven‐Erik, Ricksten., Anneli, Strömsöe., Eva, Hagberg., Christer, Axelsson., et al. (2020). Shortening Ambulance Response Time Increases Survival in Out‐of‐Hospital Cardiac Arrest. J. Am. Heart Assoc. 9 (21), e017048. doi:10.1161/JAHA.120.017048
Kerr, I. R., Millar, J., and Corriveau, N. (2017). Robots and Artificial Intelligence in Health Care. SSRN J. doi:10.2139/ssrn.3395890
Kiseleva, A. (2019). AI as a Medical Device: Is it Enough To Ensure Performance Transparency And Accountability In Healthcare? (SSRN Scholarly Paper ID 3504829). Soc. Sci. Res. Netw. Available at: https://papers.ssrn.com/abstract=3504829.
Komesaroff, P. A., Kerridge, I., and Lipworth, W. (2019). Conflicts of Interest: New Thinking, New Processes. Intern. Med. J. 49 (5), 574–577. doi:10.1111/imj.14233
Kredo, T., Bernhardsson, S., Machingaidze, S., Young, T., Louw, Q., Ochodo, E., et al. (2016). Guide to Clinical Practice Guidelines: The Current State of Play. Int. J. Qual. Health Care 28 (1), 122–128. doi:10.1093/intqhc/mzv115
Larrazabal, A. J., Nieto, N., Peterson, V., Milone, D. H., and Ferrante, E. (2020). Gender Imbalance in Medical Imaging Datasets Produces Biased Classifiers for Computer-Aided Diagnosis. Proc. Natl. Acad. Sci. USA 117 (23), 12592–12594. doi:10.1073/pnas.1919012117
Larsen, M. P., Eisenberg, M. S., Cummins, R. O., and Hallstrom, A. P. (1993). Predicting Survival from Out-Of-Hospital Cardiac Arrest: A Graphic Model. Ann. Emerg. Med. 22 (11), 1652–1658. doi:10.1016/S0196-0644(05)81302-2
LeCun, Y., Boser, B., Denker, J. S., Henderson, D., Howard, R. E., Hubbard, W., et al. (1989). Backpropagation Applied to Handwritten Zip Code Recognition. Neural Comput. 1 (4), 541–551. doi:10.1162/neco.1989.1.4.541
Leikas, J., Koivisto, R., and Gotcheva, N. (2019). Ethical Framework for Designing Autonomous Intelligent Systems. JOItmC 5 (1), 18. doi:10.3390/joitmc5010018
Lippert, F. (2018). Emergency Medical Services Copenhagen—Implementation of a State-Of The-Art System. Available at: https://www.forum-rettungsdienst-bayern.de/images/praesentationen_2018/Lippert_EMS_Copenhagen_November_2018_Munich.pdf.
Lucivero, F. (2016). Ethical Assessments of Emerging Technologies: Appraising the Moral Plausibility of Technological Visions. 1st ed. Imprint: Springer International Publishing Springer. doi:10.1007/978-3-319-23282-9
Lundberg, S. M., and Lee, S.-I. (2017). A Unified Approach to Interpreting Model Predictions. Adv. Neural Inf. Process. Syst. 30, 4765–4774.
Maaløe, L., Fraccaro, M., Liévin, V., and Winther, O. (2019). BIVA: A Very Deep Hierarchy of Latent Variables for Generative Modeling. Adv. Neural Inf. Process. Syst. 32, 6551–6562.
Middelkamp, W., Moulaert, V. R., Verbunt, J. A., van Heugten, C. M., Bakx, W. G., and Wade, D. T. (2007). Life after Survival: Long-Term Daily Life Functioning and Quality of Life of Patients with Hypoxic Brain Injury as a Result of a Cardiac Arrest. Clin. Rehabil. 21 (5), 425–431. doi:10.1177/0269215507075307
Møller, T. P., Andréll, C., Viereck, S., Todorova, L., Friberg, H., and Lippert, F. K. (2016). Recognition of Out-Of-Hospital Cardiac Arrest by Medical Dispatchers in Emergency Medical Dispatch Centres in Two Countries. Resuscitation 109, 1–8. doi:10.1016/j.resuscitation.2016.09.012
Monsieurs, K. G., Nolan, J. P., Bossaert, L. L., Greif, R., Maconochie, I. K., Nikolaou, N. I., et al. (2015). European Resuscitation Council Guidelines for Resuscitation 2015: Section 1. Executive Summary. Resuscitation 95, 1–80. doi:10.1016/j.resuscitation.2015.07.038
Moriwaki, Y., Tahara, Y., Kosuge, T., Arata, S., Sugiyama, M., Iwashita, M., et al. (2012). Complications of Bystander Cardiopulmonary Resuscitation for Unconscious Patients without Cardiopulmonary Arrest. J. Emerg. Trauma Shock 5 (1), 3–6. doi:10.4103/0974-2700.93094
Morley, J., Floridi, L., Kinsey, L., and Elhalal, A. (2019). From what to How: An Initial Review of Publicly Available AI Ethics Tools, Methods and Research to Translate Principles into Practices. ArXiv:1905.06876 [Cs]. Available at: http://arxiv.org/abs/1905.06876.
Moulaert, V. R. M. P., Verbunt, J. A., van Heugten, C. M., and Wade, D. T. (2009). Cognitive Impairments in Survivors of Out-Of-Hospital Cardiac Arrest: A Systematic Review. Resuscitation 80 (3), 297–305. doi:10.1016/j.resuscitation.2008.10.034
Murphy, D. J., Burrows, D., Santilli, S., Kemp, A. W., Tenner, S., Kreling, B., et al. (1994). The Influence of the Probability of Survival on Patients' Preferences Regarding Cardiopulmonary Resuscitation. N. Engl. J. Med. 330 (8), 545–549. doi:10.1056/NEJM199402243300807
Nadarajan, G. D., Tiah, L., Ho, A. F. W., Azazh, A., Castren, M. K., Chong, S. L., et al. (2018). Global Resuscitation Alliance Utstein Recommendations for Developing Emergency Care Systems to Improve Cardiac Arrest Survival. Resuscitation 132, 85–89. doi:10.1016/j.resuscitation.2018.08.022
NCBI (2021). (n.d.). Agonal Respiration (Concept Id: C2315245). from Available at: https://www.ncbi.nlm.nih.gov/medgen/746160 (Retrieved February 4, 2021).
Obermeyer, Z., Powers, B., Vogeli, C., and Mullainathan, S. (2019). Dissecting Racial Bias in an Algorithm Used to Manage the Health of Populations. Science 366 (6464), 447–453. doi:10.1126/science.aax2342
Owens, K., and Walker, A. (2020). Those Designing Healthcare Algorithms Must Become Actively Anti-racist. Nat. Med. 26 (9), 1327–1328. doi:10.1038/s41591-020-1020-3
Perkins, G. D., Handley, A. J., Koster, R. W., Castrén, M., Smyth, M. A., Olasveengen, T., et al. (2015). European Resuscitation Council Guidelines for Resuscitation 2015. Resuscitation 95, 81–99. doi:10.1016/j.resuscitation.2015.07.015
Price, W. N., Gerke, S., and Cohen, I. G. (2021). How Much Can Potential Jurors Tell Us about Liability for Medical Artificial Intelligence?. J. Nucl. Med. 62 (1), 15–16. doi:10.2967/jnumed.120.257196
Price, W. N., Gerke, S., and Cohen, I. G. (2019). Potential Liability for Physicians Using Artificial Intelligence. JAMA 322 (18), 1765–1766. doi:10.1001/jama.2019.15064
Puaschunder, J. M. (2019). The Legal and International Situation of AI, Robotics and Big Data with Attention to Healthcare. SSRN J. doi:10.2139/ssrn.3472885
R. Frodeman, J. T. Klein, and C. Mitcham (2012). in The Oxford Handbook of Interdisciplinarity (Oxford University Press).
Roppolo, L. P., Westfall, A., Pepe, P. E., Nobel, L. L., Cowan, J., Kay, J. J., et al. (2009). Dispatcher Assessments for Agonal Breathing Improve Detection of Cardiac Arrest. Resuscitation 80 (7), 769–772. doi:10.1016/j.resuscitation.2009.04.013
Safar, P. (1988). Resuscitation from Clinical Death. Crit. Care Med. 16 (10), 923–941. doi:10.1097/00003246-198810000-00003
Sasson, C., Rogers, M. A., Dahl, J., and Kellermann, A. L. (2010). Predictors of Survival from Out-Of-Hospital Cardiac Arrest: a Systematic Review and Meta-Analysis. Circ. Cardiovasc. Qual. Outcomes 3 (1), 63–81. doi:10.1161/CIRCOUTCOMES.109.889576
Sexton, J. B., Thomas, E. J., and Helmreich, R. L. (2000). Error, Stress, and Teamwork in Medicine and Aviation: Cross Sectional Surveys. BMJ 320 (7237), 745–749. doi:10.1136/bmj.320.7237.745
Strubell, E., Ganesh, A., and McCallum, A. (2019). Energy and Policy Considerations for Deep Learning in NLP. ArXiv:1906.02243 [Cs]. Available at:. doi:10.18653/v1/p19-1355 http://arxiv.org/abs/1906.02243.
Tschider, C. A. (2018). Regulating the Internet of Things: Discrimination, Privacy, and Cybersecurity in the Artificial Intelligence Age. Denver L. Rev. 96, 87.
Viereck, S., Møller, T. P., Ersbøll, A. K., Bækgaard, J. S., Claesson, A., Hollenberg, J., et al. (2017). Recognising Out-Of-Hospital Cardiac Arrest during Emergency Calls Increases Bystander Cardiopulmonary Resuscitation and Survival. Resuscitation 115, 141–147. doi:10.1016/j.resuscitation.2017.04.006
Virani, S. S., Alonso, A., Benjamin, E. J., Bittencourt, M. S., Callaway, C. W., Carson, A. P., et al. (2020). Heart Disease and Stroke Statistics—2020 Update: A Report from the American Heart Association. Circulation 141 (9), e139–e596. doi:10.1161/CIR.0000000000000757
Whittlestone, J., Nyrup, R., Alexandrova, A., Dihal, K., and Cave, S. (2019). Ethical and Societal Implications of Algorithms, Data, and Artificial Intelligence: A Roadmap for Research. London: Nuffield Foundation. doi:10.1145/3306618.3314289
Wiens, J., Saria, S., Sendak, M., Ghassemi, M., Liu, V. X., Doshi-Velez, F., et al. (2019). Do no Harm: A Roadmap for Responsible Machine Learning for Health Care. Nat. Med. 25 (9), 1337–1340. doi:10.1038/s41591-019-0548-6
Wnent, J., Masterson, S., Gräsner, J.-T., Böttiger, B. W., Herlitz, J., Koster, R. W., et al. (2015). EuReCa ONE - 27 Nations, ONE Europe, ONE Registry: a Prospective Observational Analysis over One Month in 27 Resuscitation Registries in Europe - the EuReCa ONE Study Protocol. Scand. J. Trauma Resusc Emerg. Med. 23 (1), 7. doi:10.1186/s13049-015-0093-3
Zhao, X., Banks, A., Sharp, J., Robu, V., Flynn, D., Fisher, M., et al. (2020). A Safety Framework for Critical Systems Utilising Deep Neural Networks. A Saf. Framework Crit. Syst. Utilising Deep Neural Networks 12234, 244–259. doi:10.1007/978-3-030-54549-9_16 ArXiv:2003.05311 [Cs].
Keywords: artificial intelligence, cardiac arrest, case study, ethical trade-off, explainable AI, healthcare, trust, trustworthy AI
Citation: Zicari RV, Brusseau J, Blomberg SN, Christensen HC, Coffee M, Ganapini MB, Gerke S, Gilbert TK, Hickman E, Hildt E, Holm S, Kühne U, Madai VI, Osika W, Spezzatti A, Schnebel E, Tithi JJ, Vetter D, Westerlund M, Wurth R, Amann J, Antun V, Beretta V, Bruneault F, Campano E, Düdder B, Gallucci A, Goffi E, Haase CB, Hagendorff T, Kringen P, Möslein F, Ottenheimer D, Ozols M, Palazzani L, Petrin M, Tafur K, Tørresen J, Volland H and Kararigas G (2021) On Assessing Trustworthy AI in Healthcare. Machine Learning as a Supportive Tool to Recognize Cardiac Arrest in Emergency Calls. Front. Hum. Dyn 3:673104. doi: 10.3389/fhumd.2021.673104
Received: 26 February 2021; Accepted: 19 May 2021;
Published: 08 July 2021.
Edited by:
Remo Pareschi, University of Molise, ItalyReviewed by:
Francesca Rossi, IBM Research, United StatesVictoria L. Lemieux, University of British Columbia, Canada
Copyright © 2021 Zicari, Brusseau, Blomberg, Christensen, Coffee, Ganapini, Gerke, Gilbert, Hickman, Hildt, Holm, Kühne, Madai, Osika, Spezzatti, Schnebel, Tithi, Vetter, Westerlund, Wurth, Amann, Antun, Beretta, Bruneault, Campano, Düdder, Gallucci, Goffi, Haase, Hagendorff, Kringen, Möslein, Ottenheimer, Ozols, Palazzani, Petrin, Tafur, Tørresen, Volland and Kararigas. This is an open-access article distributed under the terms of the Creative Commons Attribution License (CC BY). The use, distribution or reproduction in other forums is permitted, provided the original author(s) and the copyright owner(s) are credited and that the original publication in this journal is cited, in accordance with accepted academic practice. No use, distribution or reproduction is permitted which does not comply with these terms.
*Correspondence: Roberto V. Zicari, cm9iZXJ0b0B6aWNhcmkuZGU=