- Independent Researcher, Skopje, North Macedonia
Attention to addressing undernourishment in low-and middle-income countries has expanded notably since the beginning of the 21st century. Population growth increases the overall demand for food, while income growth affects consumption patterns. Using annual aggregate data from the World Bank in 2001–2020 and econometric approaches, this research investigates the changes in the growth rates in rural and urban populations and GDP per capita and the prevalence of undernourishment as % of the population in low-income countries, lower-middle-income countries, and upper-middle income countries. The main goal of the study is to convey a deeper understanding by quantifying the impacts of rural and urban population growth as well as GDP per capita growth on the prevalence of undernourishment. The robust regression models showed that the prevalence of undernourishment in these countries was strongly associated with rural and urban population growth. A positive impact of rural population growth on undernutrition was found in all three groups of countries, with the most significant positive effects found in upper-middle-income countries. The negative effect of urban population growth on undernourishment was largest for the upper middle-income countries. Furthermore, fully modified ordinary least squares results revealed that the changes in the prevalence of undernourishment are mostly associated with long-term changes in the rural and urban population growth. The Difference in Difference (DiD) estimation confirmed only the causal effect of rural population growth on the prevalence of undernourishment in the panel of these countries. The findings of this study have both methodological and policy implications.
Introduction
Population growth, increasing urbanization, modern technology, and climate change are transforming the world at a rapid pace. One of the biggest challenges today is ending hunger and poverty while making agriculture and food systems sustainable (Kamal, 2017). This challenge seems difficult due to the continued population growth, profound changes in food demand, and the threat of extensive migration of rural young people in search of a better life. Over the years, the concept of food security has evolved through many concepts, from the idea of existing food stocks in the 1970s to the concept of self-sufficiency in food production during the 1980s, and to access to food (at the individual as well as household level) in the 1990s. Thus, according to Reddy et al. (2016), the latest concept used by the global food security panelists is the following: “the right to adequate food.” While Reddy (2016) also agrees with the evolution of the concept of food security since the 1960s, he also adds that during the mentioned period there was an extensive food deficiency in the world, particularly in developing countries. In explaining the concept of food security by Reddy (2016) and Reddy et al. (2016), its main four components were accurately stated: food availability, accessibility, stability, and utilization. The availability of food is necessary when speaking about food security. In the past, the measurement of the food stability component became important since more and more food shocks occurred as a result of extreme weather conditions (such as droughts, floods, and heavy stormy hails), human displacements, and increased war refugees that produced food security risks at the local level for their vulnerable individuals and households. These shocks intensified the variability of food supply and prices, resulting in unstable food access to vulnerable segments of society, e.g., children, women, the poorest of the poor, landless workers, and also urban informal workers. Also, food utilization is becoming much more important nowadays as the ultimate goal of mankind is the proper utilization of available food for a healthy life. The most broadly recognized definition of food security claims that food security exists when “…all people, at all times, have physical and economic access to sufficient, safe and nutritious food to meet their dietary needs and food preferences for a healthy and active life” (Ickowitz et al., 2019, p. 10). When households and individuals are lacking the means to acquire food, then there is a condition of food insecurity. Food insecurity as the most fundamental form of human poverty is an inability to access food of enough quantity and quality to meet minimum dietary needs (Hashmi et al., 2018). Among the existential problems of today's civilization is survival, with the most important being food supply (Ðordević et al., 2022). According to the UN FAO official definition, hunger is a condition when a person does not eat enough food to meet his/her energy needs for a healthy and active life (e.g., 1,800 kcal per day), (Ðordević et al., 2022). Additionally to this official definition, hunger may be defined also as undernourishment in a situation where a person does not consume enough macronutrients (fats, proteins, carbohydrates) or micronutrients (vitamins and minerals). An increase in agricultural production and a decrease in the quantity of wasted or lost food are ways to lessen the proportion of malnourished populations as well as mortality due to undernourishment (Ðordević et al., 2022). Therefore, nowadays, together with the availability and stability of food supplies, access to food is one of the key linkages of food security.
Already more than half of the world's population lived in urban areas in 2011 and it is estimated that by 2050, two-thirds of the population in the world will reside in urban areas (Tuffrey and Espeut, 2015; van Zutphen et al., 2023), consuming 80% of the food in the world and producing 85% of global economic products (van Zutphen et al., 2023). Low-and high-income countries are projected to represent a relatively small proportion of the total urban population. Most of the projected growth in the world's total urban population between 2015 and 2050 will be due to middle-income countries (Tuffrey and Espeut, 2015). Therefore, middle-income countries and Asia are expected to account for most of the growth in the number of global urban populations between 2015 and 2050. Nevertheless, growing concerns about rapidly expanding population dynamics and increased risks as a result of climate change require additional information to advise policymakers and provide effective means to support the most vulnerable communities. As a consequence, vulnerable communities living in rural areas, characterized by low income and low education levels, in need of technical know-how as well as appropriate policy responses and safety nets, are those most affected by the consequences of the interaction between climate change and non-climatic impacts (FAO, 2016).
On a global level, the attention to addressing undernourishment in low-and middle-income countries (LMICs) expanded notably since the beginning of the 21st century (Hambloch et al., 2022). The percentage of the population that is undernourished in low-income countries since 2014 constantly has grown, especially in Sub-Saharan Africa, where the prevalence of undernourishment raised from 18% in 2014 to 23.2% in 2021 (Hambloch et al., 2022), and the rise in Sub-Saharan Africa is to some extent driven by population growth. Improvement toward lessening undernourishment has been especially restrained in West Africa, particularly in Nigeria, which accounts for a significant portion of global malnutrition (Adeyemi et al., 2022). According to official data, 850 million people in the world (about 11% of the world's total population) are chronically undernourished, and yearly 5,642 million children under 5, and 959,000 children between ages 5–14 die of starvation (Ðordević et al., 2022). Thus, India has the highest number of children as well as pregnant women suffering from malnutrition, and a great number of children have insufficient food for good health and condition (Reddy, 2016). Accordingly, the paradox with India is that India is self-sufficient in food but has the highest undernourished population in the world. In addition, Ðordević et al. (2022) emphasize that nowadays about two billion people in the world are considered undernourished, which is often attributed to “hidden malnutrition.” For instance, as a developing country, official data for Nigeria in a household expenditure survey released that, 56.65% of the total household expenditure in 2019 was allocated to food, in comparison with the U.S. consumers where only 8.6% of Americans' disposable personal income was spent on food in 2020 and with Europe where 13% of household spending were on food 2019 (Salam et al., 2022). These findings just clearly show that a greater percentage of disposable income in the developing world is spent on food. Only 72 developing countries out of 129 countries that were monitored by FAO achieved the Millennium Development Goal (MDG) hunger target (MDG 1c) by the year 2015 which required a reduction by half the proportion of the chronically undernourished from the baseline (1990/1992) (Reddy et al., 2016). Coming after the period, the Sustainable Development Goal (SDG) 2030 agenda has provided the global panels with a continued opportunity to end starvation and all forms of undernourishment, through integrating connected objectives into a more comprehensive, holistic and proactive development agenda (Reddy et al., 2016; Fanzo et al., 2020).
The research study aims to fill the knowledge gap in the vast body of research on food security, its theoretical and empirical dimensions, and trends over the last twenty years observed in low and middle-income countries. The specific objective is to conduct empirical research work on food security from the perspective of investigating population growth in urban and rural areas as well as economic development in these countries. Therefore, the main goal is to convey a deeper understanding by quantifying how much the change in both rural and urban population growth as well as GDP per capita growth within these countries contributed to the prevalence of undernourishment. The prevalence of undernourishment is one of the indicators of food access. The prevalence of undernourishment is indicative of chronic food deprivation. Furthermore, it is also a known indicator of “hunger.” Therefore, it shows the percentage of the population that does not consume an adequate amount of calories to maintain an active and healthy life (Reddy, 2016; Reddy et al., 2016).
With an understanding of the importance of economic development and urban-rural differences in population growth, the key motivation to work on this research study is to raise the general level of knowledge for the low-income and middle-income countries concerning their food crises. The findings of this study have a great contribution given the importance of the wellbeing of the people and improving the global security of food. For a society, hunger and malnutrition have obvious economic importance, which is mostly reflected through increased health care costs, reduced work capacity of their working-age population, etc. Hence, the results extend their importance to the level of adequate contribution as the causality in our results is ensured. Such causal assessments can shed light on whether urbanization or development generates greater benefits or costs, and thus provide a useful guide to which policies are better for enabling food security. Further, key research questions were arising during the research study, such as: Is it possible to reduce the number of undernourished in the absence of significant economic growth? Should the undernourishment problem be solved through increased food production and a shift in consumption? Might the population in rural areas within the LMICs be a powerful force for changing patterns of agricultural production, food security, and labor market as well as the process of economic development? This paper is organized as follows: Section 2 presents a literature review on undernourishment links with population growth and economic performances; Section 3 explains the data and method used. In Section 4 the research findings are presented. Section 5 discusses the research results and Section 6 concludes and provides policy recommendations.
Literature review
This Section presents the literature review done in the area of food security-population growth and economic development. It explains the background of the undernourishment determinants. Therefore, it examines the literature to determine how relevant determinants such as urban and rural population growth as well as economic development and their mutual interaction affect food security. The debate on the urban-rural population growth in low-income and middle-income countries and their effect on undernourishment appears to remain far from resolved. By considering this gap in the scientific literature, this study proposes an original research approach to partially fill this gap to the extent possible with some key aspects of evaluation. Urbanization, population growth, and income growth are driving strong demand for food at a time when agriculture is facing unprecedented natural-resource limitations and climate change (Kamal, 2017).
The economic consequences of population growth, specifically, have been a long-lasting subject matter of debate. In 1798, Thomas Malthus, among the first of the population pessimists, claimed that there will be an exhaustion of the world's resources and the reason for this would be the pace of population growth (Bloom and Canning, 2006). Therefore, the thinking that food production would expand more slowly than population growth held sweep well into the 20th century. Furthermore, Bloom and Canning (2006) also emphasized Simon's view, accordingly the prices of natural resources decline as growing populations are making the technological advances necessary to make a response to increasing demand. Thus, for instance, the Green Revolution, where new, high-yielding crops drastically increased food production in much of the developing world, happened partly as a response to population growth. The period of the Green Revolution (1960–2000) marked a remarkable period of increased global food security. The period was characterized by a huge increase in world food production and distribution, particularly of grains such as wheat, rice, and maize, as a result of the intensification of rural agriculture (Armanda et al., 2019).
The concept of effective demand is extremely important to understanding the link between food security, population and development (Villarreal and Stloukal, 2005). Even though population optimists did not accept the automatic link between population growth and economic progress, they somehow were assured that advantageous policies could help shift increases in population into greater prosperity. The main factor in the development of population-food security links is the problem of access to food in terms of purchasing power, market, and regional distribution, and reducing food insecurity thus means eliminating poverty. According to Villarreal and Stloukal (2005), the patterns of food consumption are determined primarily by two major factors: population growth and growth in GDP per capita. With a world population that significantly will increase during the 21st century and with more urban than the rural population, the total demand for food will be greater and different than ever. The second question of great importance is the incidence of poverty and hunger and its association with food security. As stated by Reddy (2016), income inequalities and poverty can be the causes of undernourishment. Therefore, the very important question is whether it could be possible to reduce half the proportion of the world population living in absolute poverty. Much of the food insecurity in the world is chronic and poverty-based, consequently reducing poverty indirectly is the most effective way to make progress in poverty mitigation (Samia et al., 2021). According to these scholars, poverty is a cause and a result of a lack of food security as well as an absence of economic strengthening. Thus, reducing poverty should assure appropriate access to food (Samia et al., 2021). To a great extent, reducing poverty will be the result of development in the different regions of the world. The economic and financial crises that affected the world, especially in developing countries, caused many households to take decisions to minimize their expenses, even for basic needs, such as food quantity and quality (Salam et al., 2022). Consequently, in a period of inflation and unemployment, consumers are more likely to change the distribution of their expenditures; accordingly, there is a strong shift toward the consumption of cheaper articles with lesser nutritional value considering the level of income and family size (Salam et al., 2022).
As stated by Fanzo et al. (2020), food security and nutrition have been important characteristics of the international development agenda for decades. Nevertheless, over time, development priorities and challenges fluctuated, and the required investment has not been assisted. A wide consent emerged that ensures food security and brings an end to famine and undernourishment in all its forms when there will be prosperous economies, human and world health, and sustainable development (Fanzo et al., 2020). For economic growth to be successful in reducing poverty, it should be inclusive and move at a rate that is higher than the population growth rate. The fact that agriculture is the most important economic sector in most poor countries implies that efforts to fight extreme poverty should be aimed at increasing agricultural production and productivity (Ayoo, 2022). Promoting inclusive economic growth is vital not only in raising incomes but also in ensuring that the benefits of economic growth are widely shared. Some ways to promote inclusive economic growth are investing in infrastructure and technology; trade liberalization and expansion of export markets; providing incentives for small and medium-sized businesses; providing fiscal stimulation for the economy; ensuring macroeconomic stability; and improving public governance (Ayoo, 2022). Implementing these measures in an integrated way can have positive effects on the economy, incentivize the private sector, create much-needed employment opportunities and reduce the levels of poverty.
The topic of rural-urban population growth has much in common with food security. The process of urbanization is a demographic transition from rural to urban living, as well as an economic transition from an agriculture-based economy to an industry, technology, and services-based one (Tuffrey and Espeut, 2015). In addition, urbanization also has intense social, political, and environmental effects. By 2050, it is expected that approximately about 68% of the population in the world will live in cities, and consequently, agriculture will need to produce nearly 50% more food than in 2012 to meet the needs of an estimated 9.73 billion people then (Armanda et al., 2019; Fanzo et al., 2020). Some of the poorest countries, especially in Sub-Saharan Africa will experience high and sustained growth rates, and on the other hand, the population growth in Asia is decelerating, during which some countries are facing negative population growth and aging populations as a threat to their sustained economic growth (Fanzo et al., 2020). Therefore, food systems will have to feed this growing and changing population utilizing the same available resources, but with more pressure from climate change and environmental degradation. While rural poverty is anticipated to decrease more rapidly than urban poverty as a result of rural-urban migration, there is large poverty in poorly planned urban slums which most often go unnoticed (Fanzo et al., 2020).
Food insecurity is strongly associated with poverty; around the world, rural poverty continues to exist more deeply and comprehensively than urban poverty (Tacoli et al., 2013). However, with the rapid urbanization in LMICs, more and more poverty is located in urban areas, and this will continue as almost all global population growth over the next two decades is expected to be in cities and towns in Asia and Africa (Tacoli et al., 2013). Overall, the data indicate a change toward a higher burden of malnutrition in all its forms in urban compared to rural areas. Thus, by 2035, half of individuals in extreme poverty with a daily income of < USD 1.25 will reside in urban areas, which means an increase in the number of people who cannot afford a healthy diet (van Zutphen et al., 2023). Labor-intensive, small-scale subsistence farming is the main source of income for the poor and thus a reason for food insecurity in rural parts of developing countries (Samia et al., 2021). Rapid urbanization has resulted in more than half of the global population living in cities, which means food security is a challenge for many people. Since urban consumers depend on being able to buy food, the food security of low-income urban populations is vulnerable to increases in food prices or unforeseen variations in food prices (Vågsholm et al., 2020).
Thus, periods of food insecurity are more likely to be associated with periods of low earnings, particularly for casual workers, fluctuations in food prices and high costs of other non-food things such as housing, heating and health, which are intensified by extreme weather conditions (Tacoli et al., 2013). To some degree, this is the case also in rural areas, and increasingly so, although rural food insecurity is still more in many cases connected to seasonal cycles. But as claimed by Tacoli et al. (2013) extreme weather events remain a major threat to low-income urban inhabitants, especially those living in non-formal settlements where environmental dangers tend to be more frequent than in rural areas. Rates of undernutrition among women and children are generally lower in urban areas than in rural areas; but in any case, urban regions are characterized by a particular set of vulnerabilities, especially for the poorest segments of urban populations (Tuffrey and Espeut, 2015). The determinants of nutrition in urban areas may differ from those in rural areas in terms of their importance and relationship with other circumstances (Darrouzet-Nardi and Masters, 2015), e.g., urban households do not have to rely as much on precarious natural resources and situations for their living as rural farmers do; or, urban areas may also provide more employment opportunities for men and women, including food service and retail opportunities. As rates of urbanization increase worldwide and there is continued pressure to decentralize decision-making to levels closer to the people being served, cities are taking on an increasingly important role in the global malnutrition crisis and providing points of transformation for the food system (van Zutphen et al., 2023). Although urbanization has become an unchanging phenomenon, some argue that solving the city's problems requires addressing the root causes of the problem, such as improving the socioeconomic conditions of the urban poor (Kuddus et al., 2020). Furthermore, Kuddus et al. (2020) add that until conditions in rural areas improve, the population will continue to migrate to urban areas. Therefore, given the challenges that rural development brings, the root causes are unlikely to be addressed nearby.
The right-to-food-based approach to food security proposes that human dignity, recognition of rights, transparency and government responsibility, citizen empowerment, food, and wellbeing should be considered in welfare programs (Righettini and Bordin, 2022). The right-to-food approach requires governments to adopt specific programs and fulfill exact commitments to fight poverty. According to this approach, the right to food is not only a means to attain food security and preferably it should be a wider, more encompassing, and specific goal. The debate about the interrelationship between food access and poverty reduction interventions during the 1980s meant whether food security was looked at as an integral part of social security, which was understood as the reduction of poverty and a deficient standard of living by social means, regardless of whether these conditions are the results of chronic impoverishment or temporary difficulties (Righettini and Bordin, 2022). Since the 1990s, the food security literature has highlighted that measurements can vary in their performance in different population groups and societies and that good practice and policy instruments are difficult to transfer across different contexts (Righettini and Bordin, 2022). One of the major issues in food security interventions is that it is not simple to indicate the target, e.g., households under the poverty line (Righettini and Bordin, 2022).
According to the paradigm of political economy, food and nutrition are considered purely composed of nutrients, disregarding the political-economic and socio-cultural circumstances that determine the economic and cultural value of food production (Hambloch et al., 2022). Within the scope of this paradigm, the delivery of private sectors and the whole food and nutrition systems as well as increasing nutrient-consuming efforts was significantly supported by many people. These views have been criticized for avoiding the complexity of the underlying drivers of nutrition and the determinants of undernourishment (Hambloch et al., 2022).
In contrast to these criticisms, there has been a growing consensus that the apparent causes of malnutrition are not technical per se, but instead reflect a composite lack of equity and power relations in food systems, i.e., transformation of food system perspective. According to this perspective, the demands in the sustainable food system discussion suggest that a fundamental process of transformation toward healthier, equitable and socially just ways of environmental development is needed (Hambloch et al., 2022). In addition, transformation should not only include a technological change agenda, but more importantly a social and political agenda.
Furthermore, the agricultural intensification approach is closely related to the perspective of the transformation of the food system. Donors, development experts, and national policymakers often look to agricultural intensification as a way to increase rural incomes and stimulate development (Ickowitz et al., 2019). Simply put, agricultural intensification refers to any process of acquiring more production with fewer means, but mostly its meaning is explained as increasing yields per unit of land. Proponents of a “second Green Revolution” are particularly dominant in the national and international discourse on agricultural development in Africa where supporters of the “African Green Revolution” proclaim that intensification is important not only for food production but also as an essential driver of economic development (Ickowitz et al., 2019). Consequently, economic development, population growth and increasing rates of urbanization create an importance that requires rapid action to accurately understand the role of food systems in sustainable food production, consumption, diets and nutrition in urban environments (van Zutphen et al., 2023).
Therefore, the major hypothesis for this research study is: There is a cointegration relationship and causal relationship between economic development and rural-urban population growth rate with the prevalence of undernourishment as a proxy indicator for food security in low-and middle-income countries of the world.
Data and methods
This Section covers detailed information about the dataset collected for this study, as well as the methods and techniques applied to make the data ready for analysis. Furthermore, the three methods used to analyze the results of the models and the reasons for using these methods are discussed. This research study paper is based on a macro analytical approach. Using annual aggregate data from the World Bank from 2001 to 2020, this research investigates the changes in rural population growth rate (RPG), urban population growth rate (UPG) and GDP per capita growth rate (GDPPCG), and its implications to the prevalence of undernourishment as the proportion of the population (UNDERNUR) in low-and middle-income countries. The estimation of the country's prevalence of undernourishment as the proportion of the population is based on the availability of the relevant data in the World Bank development database (World Bank, 2022). This indicator is also available from the Food and Agriculture Organization (FAO), (FAO, 2023). In addition, the annual time series data of the country's GDP per capita growth rate as well as rural and urban population growth rates were retrieved from the World Bank database (World Bank, 2022). Therefore, given the available official data, it is confirmed that our empirical research opens a reflection on comparative research in the aspect of the population-development-food security nexus. Figures 1–4 present the prevalence of undernourishment (% of the population), GDP per capita growth rate, and urban and rural population growth rates from 2001 to 2020 in low-income countries, lower-middle-income countries, and upper-middle-income countries, respectively.
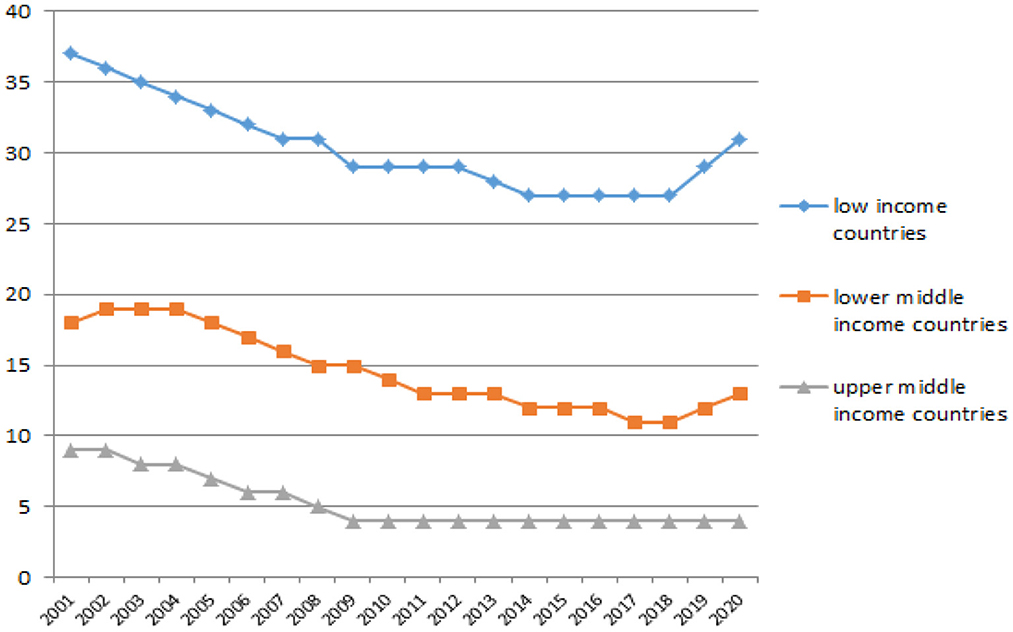
Figure 1. Prevalence of undernourishment (% of population). Source: author's design based on World Bank data.
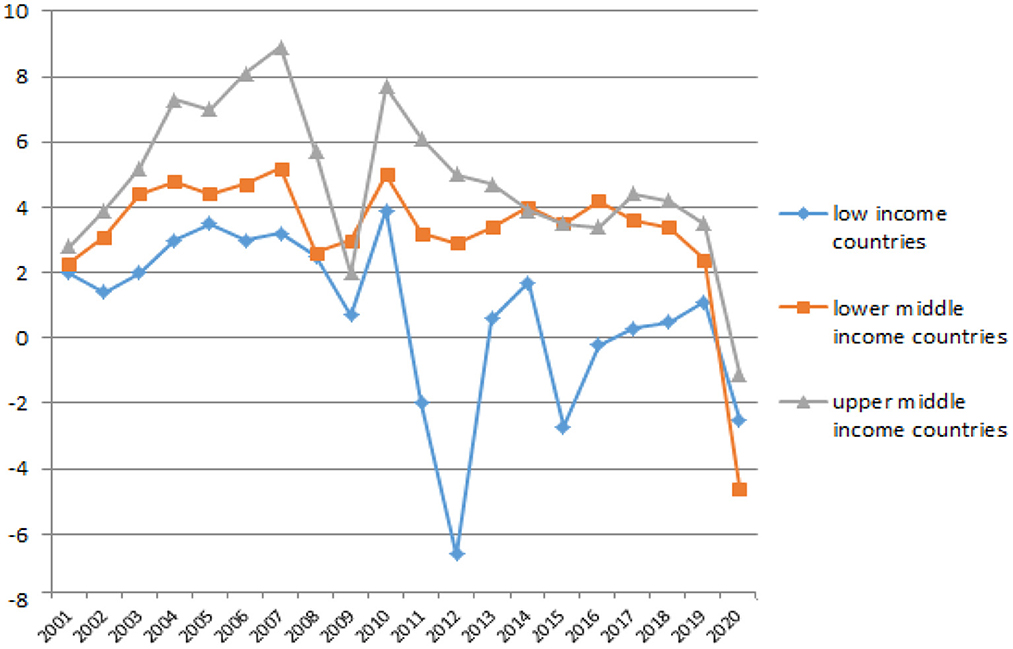
Figure 2. Annual growth rate of GDP per capita (%). Source: author's design based on World Bank data.
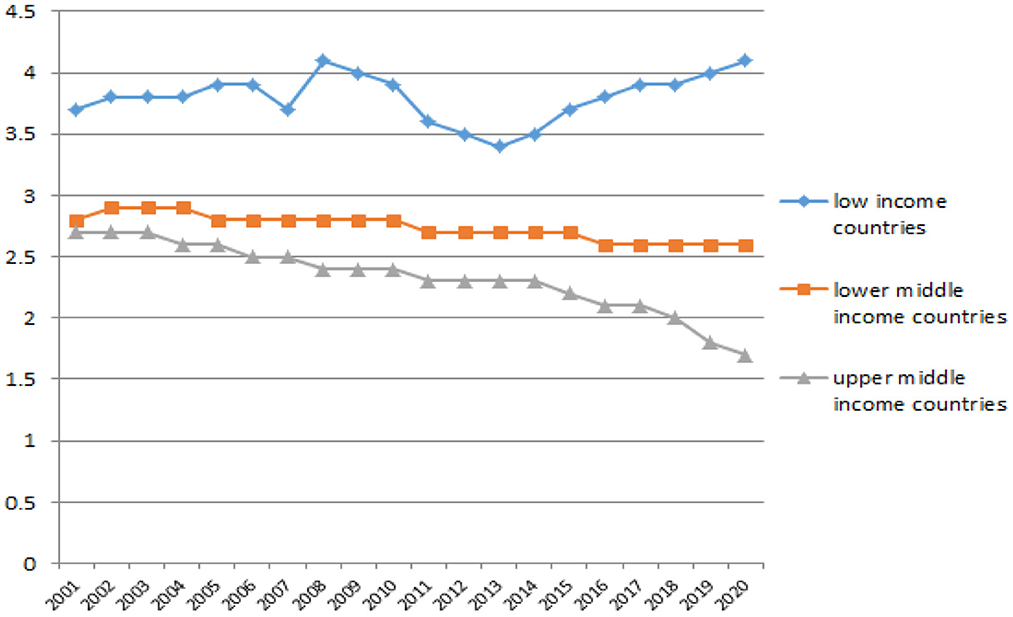
Figure 3. Annual growth rate of urban population (%). Source: author's design based on World Bank data.
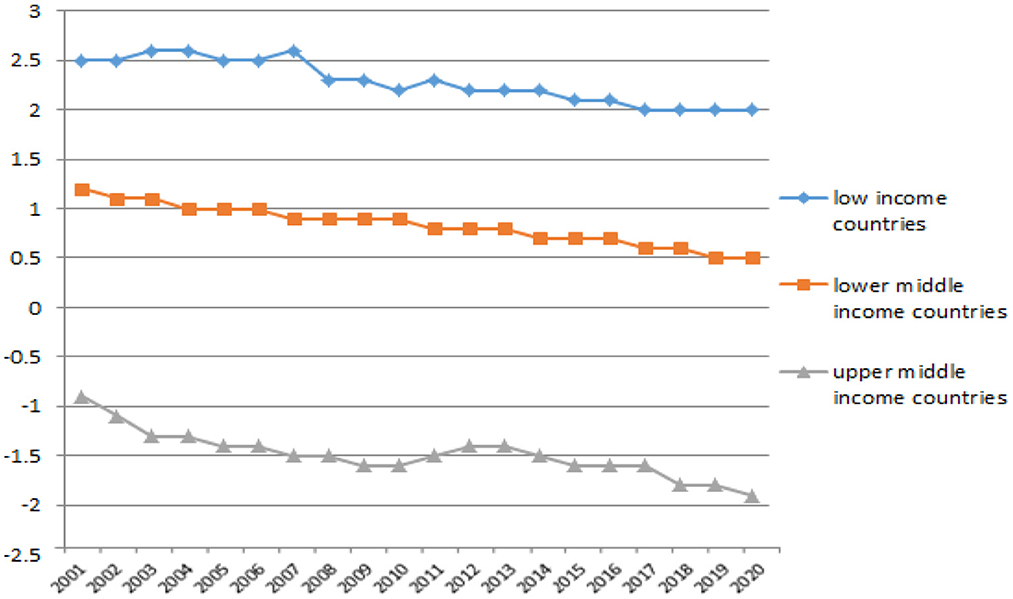
Figure 4. Annual growth rate of rural population (%). Source: author's design based on World Bank data.
To reveal a possible long-term relationship, a cointegration, or a causal relationship between a dependent variable and regressors, and to come up with a relevant conclusion, separately, three econometric approaches have been employed–robust ordinary least squares (OLS) method with MM-estimation, fully modified ordinary least squares (FMOLS) cointegration approach, and the difference in difference (DiD) estimation. Therefore, using separately three econometric approaches the study will determine whether significant and causal differences in outcome variables are evident between these groups of countries. In addition to the other methods, e.g., FMOLS, the difference-in-differences (DiD) approach explicitly was used also to provide a credible estimation focusing on the effects of the changes of the treatment on the outcome variable. Therefore, the challenge for causal inference with DiD is to come up with a reliable estimate of what the outcomes for the treatment group would have been in the absence of the treatment (Athey and Imben, 2017). Additionally, because robust OLS and FMOLS methods make assumptions of linearity and because our Difference in Difference (DiD) approach assumes linearity in the model (Athey and Imben, 2017), the DiD approach was used to estimate the average treatment effect as the difference between the change in average outcomes over time for the treatment group, with the subtraction of the change in average outcomes over time for the control group.
It is known that OLS estimators are sensitive to the presence of observations that lie outside the average for the regression model. The sensitivity of standard regression methods to these outlier observations could result in coefficient estimates that do not accurately reflect the underlying statistical relationship. Therefore, robust least squares refer to different types of regression methods created to be robust, or less sensitive to outliers. There are three different methods for robust least squares: M-estimation, S-estimation and MM estimation (IHS Global Inc., 2020). M-estimation addresses dependent variable outliers when the value of the dependent variable varies remarkably from the regression model. S-estimation addresses outliers in independent variables, i.e., when there are large residuals. MM estimation is a combination of S-estimation and M-estimation. The procedure begins by performing an S-estimation of the coefficients and scale and then uses the estimates acquired from the S-estimation as the starting point in iterating to find a solution for M-estimation. The second stage of M-estimation uses the Bisquare function with a default tuning parameter value. The summary statistics for MM-estimation are obtained from the second-stage M-estimation procedure. Since MM estimation is a combination of the other two methods, it addresses outliers in the dependent as well as independent variables. MM estimation was applied for all three groups of countries: low-income countries, lower-middle-income countries and upper-middle-income countries, For MM estimation, identical choices for all groups of countries in terms of the objective specification, scale estimator, and covariance type have been used. Computing the coefficient covariance matrix with all three methods taken from Huber provides unbiased estimates of the covariance matrix. In our case, Hubert Type II was used. Its form is presented in eq. (1):
with
where
where ψc(·) = and xij is the value of the j-th regressor for observation i, (IHS Global Inc., 2020). The estimation of long-run relationships has been the focal point of broad research work in time series econometrics (Breitung and Pesaran, 2005). Pedroni (2004) has considered the building of statistics which will be appropriate in the presence of heterogeneity in the dynamics and endogeneity of the regressors. Accordingly, the basic approach of Pedroni (2004) should first estimate the hypothesized cointegrating relationship for each unit separately, and then pool the resulting residuals in creating the panel test for the null of no cointegration. The research of cointegrating relationships has been an especially active field of investigation. Engle and Granger noted that a linear combination of two or more I(1) series may be stationary, or I(0), in which case it could be said that the series are cointegrated (IHS Global Inc., 2017). This kind of linear combination defines a cointegrating equation with cointegrating vectors of weights, indicating the long-run relationship between the variables. Within our research study, a fully modified OLS” (FM-OLS) weighted approach was applied. Thus, a conveniently pooled OLS estimator for heterogeneous cointegrated panels is described where the long-run variances differ over cross-sections. To obtain the residuals, it is needed to estimate the long-run variance and regressors equations (IHS Global Inc., 2020); (see eq. 3–4),
Here β0 is the preceding estimate of the long-run coefficient. The structure of the weighted variables is given in eq. (5):
The estimator and asymptotic covariance are presented in eq. (6–7), respectively, (Pedroni, 2000; IHS Markit, 2022):
DiD estimation (difference-in-difference) is a popular method of causal conclusion that enables the estimation of the average effect of a treatment or a policy on an outcome by comparing the difference in the outcome variable before and after treatment (Athey and Imben, 2017; Goodman-Bacon, 2021; IHS Markit, 2022). DiD is a seemingly-experimental model that uses longitudinal data from treatment and control groups to obtain an appropriate counterfactual to estimate the causal effect of the treatment (Bhavani et al., 2022). Therefore, DiD is usually used to assess the effect of a specific intervention or treatment by comparing changes in outcomes over time, thus focusing on changes rather than absolute levels. Even though DiD may be estimated on cross-sectional data, most research in econometrics has focused on the estimation of DiD models in panel data samples. The DiD estimation uses cross-section cluster-robust standard errors. On a single treatment date, D* some of the subjects in the study are given the treatment. The remaining part of subjects never receives treatment. The treated subjects belong to group T, and those not treated subjects belong to group NT. Besides data on whether a subject is treated, there is also time series data on an outcome variable, Y with periods before and after the treatment date, for all subjects. To estimate the average effect of treatment on Y, there should be a comparison of the difference in the mean of Y between the treated and never treated subjects before and after the treatment date. Thus, the ATET can be estimated using a fixed effects model with fixed effects in cross-section for given time dimensions, and a dummy variable appropriate to whether the observation is treated in the treated group, individual or otherwise, (eq. 8), (IHS Markit, 2022).
where g is constant for an individual i, and g is associated with treatment (g = 1) and for non-treatment (g = 0), Dg, t has a value of 1 when a treatment observation is treated. In this identification, the ATET is equivalent to the value of δ. The estimate of δ is the same as the result obtained in the panel two-way fixed effects regression, i.e., eq. (8). As the TWFE is estimated using OLS regression, all assumptions needed for an OLS model are needed for DiD estimation with TWFE as well. The computation of the ATET may be placed in an OLS regression structure, as presented in the model in eq. (9):
where D is a dummy variable identical to 1 if the observation lies after D*, and T is a dummy variable identical to 1 if the observation is in the treated group (IHS Markit, 2022). In addition, it is obvious to note that the ATET is equal to the value of β3, which quantifies the marginal effect of being a part of the treatment group after D*. For the estimates of δ to be unbiased for the ATET, there is a key DiD requirement for an additional parallel trends assumption. Treatment and control groups have parallel trends in outcomes (Bhavani et al., 2022). The parallel trends assumption is important and requires that in the absence of treatment, the difference between the treatment group and the control group is constant over time (Bhavani et al., 2022; IHS Markit, 2022). For this reason, the DiD approach is suitable for impact assessment and the results will be robust. This means that when comparing a treatment group to a never-treated or always-treated control group, unbiased estimation seeks that the change in outcomes for the control group before and after the treatment date be a good measure of the unobserved untreated change in the treatment group (IHS Markit, 2022).
Research findings
Before continuing with the estimation results, the orders of integration of our series were studied as well. The specification of the integration orders was performed by a cross-sectionally independent unit root test. The test was performed directly by the ADF-Fisher Chi-square and ADF- Choi Z-stat tests on the levels of the series. Since the null hypothesis of this test is the presence of a unit root, tests that have p-values close to zero will reject the null and identify the series as I(0). In other ways, tests with p-values significantly away from zero will fail to reject the null and identify the series as I(1). Table 1 sums up the results of this test. Table 1 shows that each variable's null hypothesis for the unit root tests in levels failed to reject the null at the 5% level of significance, and for that reason, the variables were identified as non-stationary time series in levels. Only the variable undernourishment in upper-middle income countries and GDP per capita growth in low-income countries were identified as stationary time series at levels at the 1 and 10% levels of significance, respectively.
Three separate econometric approaches (robust OLS method; cointegration panel FMOLS method; and DiD- the difference in difference approach) have been used to test for the effects of urban and rural population growth as well as economic development through a proxy GDP per capita growth on the prevalence of undernourishment as the proxy measure indicator of food security. Given that the prevalence of undernourishment within the population depends on the available foods that can be purchased in markets and their ability to be afforded, it was hypothesized that the percentage of undernourishment in the country is quite a good indicator of the existence of food security. Determining the significance and direction of these multiple impacts is important to understand the differences and synergies that different factors have on food security and that is at the core of the debate and discussion in the next Section of this study. The results of this research provide valuable information on the link between urban and rural population growth, GDP per capita growth and the prevalence of undernourishment trends in three groups of countries in the world: low-income countries, lower-middle-income countries and upper-middle-income countries. The sample in each applied method consisted of annual data for the three groups of countries for the years 2001–2020.
Referring to the MM-robust results (Table 2), it is noted that the results from the MM-estimation are generally with roughly comparable coefficient standard errors across the models of the three groups of countries. The MM-estimate of the scale for the robust model in the upper middle-income countries is considerably larger than that obtained from the MM-estimation of the model of the low-income countries as well as lower middle-income countries. Largely, goodness-of-fit measures and statistics as well as test results are rather similar to the MM-estimations, with the highest values for the lower-middle-income countries and lowest values for the upper-middle-income countries. The findings with the MM robust method have shown a positive significant effect at a 1% level of rural population growth (RPG) on the prevalence of undernourishment variation in low-income countries and with a 10% level of significance in lower-middle-income countries. The effect of RPG was found to be significant for the prevalence of undernourishment in upper-middle-income countries at 5 % but with negative effects. Thus, the results from the upper middle-income countries showed the largest effect of urban population growth (UPG) on undernourishment (UNDERNUR). Most importantly, this research study has found that the positive effect of urban population growth (UPG) on undernourishment (UNDERNUR) was largest for the upper middle-income countries at 1% and then for low-income countries at 5%. The effect of UPG in the lower middle-income countries on UNDERNUR was insignificant at even 10%. The MM-estimator produces a quite large negative impact of GDP per capita growth (GDPPCG) on undernourishment (UNDERNUR) at 1% only for the lower middle-income countries. Accordingly, the effect of GDP per capita growth (GDPPCG) on UNDERNUR was statistically insignificant for low-income countries and upper-middle-income countries, as well as a negative sign.
The analysis of long-run cointegrating relationships has received considerable attention in modern time series analysis. Furthermore, panel cointegration analysis was applied to the study of the prevalence of undernourishment by estimating the cointegrating relationship of the first difference of rural and urban population growth as well as GDP per capita growth. The weighted estimation method of the panel FMOLS estimator was considered for the cointegrating vectors as can be seen in Table 3. The fixed effect specification is assumed with UNDERNUR as the dependent variable and UPG, RPG and GDP per capita growth as the cointegrating regressors. To tackle the fixed effect, a Constant (Level) is specified in the Trend specification. A panel is said to be balanced when every cross-section is observed for the same period (Startz, 2015). Within the FMOLS panel model (Table 3) the data are balanced since there are observations for all four variables from 2001 to 2020 for each three groups of countries. Table 3 shows that the panel estimates are based on a weighted estimation using the constant C as a deterministic regressor which is included in the regression equation but excluded from the cointegrating equation. The individual deterministic coefficients obtained from the FMOLS regression equation show that they have the lowest value for upper middle-income countries (5.0467) and the highest value for low-income countries (29.711). The value of deterministic coefficients for the lower middle-income counties is (14.285). The coefficient covariance matrix computation uses an estimator of the long-run covariance estimates using a Bartlett kernel by putting a user-specified bandwidth value at 6. The coefficients of rural population growth and urban population growth are significantly associated with the prevalence of undernourishment and both at a 1% level. Rural population growth has a negative effect and urban population growth has a positive impact on the prevalence of undernourishment (Table 3).
A Pedroni panel cointegration test was performed with an automatic selection for lag length. This test provides several Pedroni panel cointegration test statistics which evaluate the null of no cointegration against both the homogeneous and the heterogeneous alternatives (Table 4). The test showed that six of the eleven statistics reject the null hypothesis of no cointegration at the conventional level of 1%. Thus, the Pedroni test indicates cointegration regression using the FMOLS method. The results of the Pedroni panel cointegration test indicate that there is cointegration between rural population growth, urban population growth and GDP per capita growth with the prevalence of undernourishment (% of the population) in the panel sample for these three groups of countries. In addition, by performing Pairwise Granger Causality Tests, there was one-way direction causality from urban population growth to the prevalence of undernourishment at a 5% level of significance. Causality that runs one-way from the prevalence of undernourishment to GDP per capita growth was found at 5% as well. Granger causality and feedback are important aspects of applied time series and panel data analysis. In 1969, Granger developed a statistical concept of causality between two or more time series variables, according to which, a variable x “Granger-causes” a variable y, if the variable y can be better predicted using past values of both x and y than using only past values y (Xiao et al., 2021). The Granger testing started with the pair of UPG, RPG and GDPPCG Granger causes UNDERNUR. Subsequently, univariate tests were considered by modeling UNDERNUR as a function of UPG, RPG and GDPPCG separately. Throughout, it was allowed for a maximum of 2 lags of the dependent variable and the covariates. The null hypothesis that urban population growth does not Granger-cause undernourishment was rejected at the 5% level of significance.
Furthermore, using the common two-way fixed-effects (TWFE) method, a single treatment date approach was applied to the average treatment effect for the treated (ATET). The average impact of the treatment on Y (the prevalence of undernourishment) was estimated by comparing the difference in the mean of Y between the treated and never treated groups before and after the single treatment date. As in the previous models, the dependent variable consists of annual percentage rates of prevalence of undernourishment for low-income countries, lower middle-income countries and upper middle-income countries between 2001 and 2020 (Table 5). It is important to note that the estimation of the DiD method was only obtained for rural population growth as a treatment dummy variable, probably because of the panel structure of the available data in the sample. Results of urban population and GDP per capita growth as a treatment dummy variable were either not valid, as was the case for GDP per capita growth (GDPPCG), where probably there was a biased positive coefficient that may have sloped upward and biased the overall TWFE coefficient or statistical insignificance was found, as was the case with the urban population growth (UPG), i.e., no causality was detected. In addition, both variables (GDPPCG and UPG) could not be computed as an estimate for trend values, and as such, the results were not able to be adequately interpreted. In contrast, causality at the 1% level was found only in the impact of rural population growth on the prevalence of undernourishment. As can be seen from Table 5, the adjusted R-squared is 0.992. Therefore, the model can explain more than 99% of the variance in the UNDERNUR response variable. It is a great result. It is noted that the RPG coefficient is statistically significant as indicated by its p-value, which is < 0.01 and leads us to conclude that the model variable is significant and explains well the variance in UNDERNUR.
All over this period, several countries categorized within these three groups of countries at different times faced the increase or decrease in rural population growth. The data on the rural population growth in the series RPG, a binary series, is equal to 1 if an observation has an increase and zero if no increase has been noted, i.e., the same level as the previous year or a decrease as well as cross-section (countries) and date (year) series. To determine the effect of an increase in rural population growth on the prevalence of undernourishment, a simple difference-in-difference was performed with a single treatment time estimation (Table 5). The estimate of the effect of an increase in rural population growth on the prevalence of undernourishment is 0.90. Figure 5 and Table 6 present the aggregations by treatment date as well as parallel trends of always and never treated.
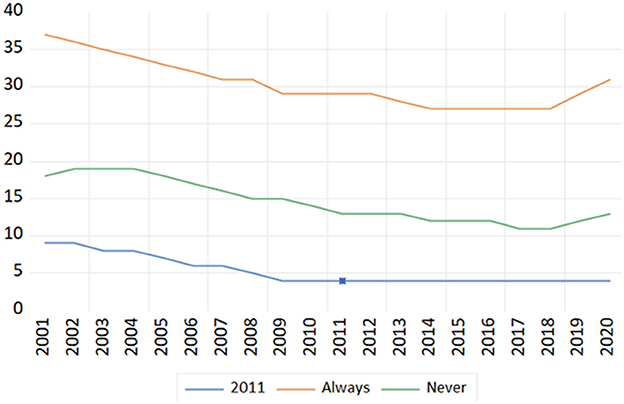
Figure 5. DiD estimation results: means of UNDERNUR treatment date. 2011 represents the average single treatment date of rural population growth (RPG) on the prevalence of undernourishment (UNDERNUR). Always and Never represent the always-treated and never-treated control group. Source: author's design based on World Bank data.
Discussion
The study is data-driven and after validating our models by performing a variety of diagnostic tests, this study provides empirical results that are very valuable for the scholars as well as policymakers and others concerned. The reports of the Pedroni cointegration test statistics and its p-values, as well as regression results concerning the DiD estimation, confirmed the long-term cointegration between all four variables included in the model. The causation was found only for urban population growth and rural population growth in the prevalence of undernourishment in the population within these three groups of countries, respectively, with the use of Granger univariate tests and DiD estimation per se. If we disregard the fact that no causal relationship was found only between GDP per capita and the prevalence of undernourishment, we can confirm as fairly relevant our main hypothesis that: There is a cointegration relationship and causation between economic development and rural-urban population growth with the prevalence of undernourishment as a proxy indicator for food security in low-and middle-income countries of the world.
The empirical results from MM robust estimator show us clear evidence that during the last two decades, the prevalence of undernourishment as a percent of the population in these three groups of countries has been characterized by a strong significant effect of the urban and rural population growth, respectively (Fanzo et al., 2020; Samia et al., 2021). More precisely, a positive impact of the rural population growth was found in all three groups of countries, with the most significant positive effect in upper-middle-income countries and then in low-income countries at 1% and with a positive effect in lower-middle-income countries at 10%. The robust regression results show that the negative effect of urban population growth on undernourishment was largest for the upper-middle-income countries and the positive impact of the urban population growth was revealed for low-income countries, both at 5%. The effect of urban population growth in the lower middle-income countries was insignificant. Only for the lower-middle-income countries was found a strong statistically negative effect of GDP per capita growth on undernourishment at 1% level. This is a good and relevant signal from our robust regression results and has a direct link with the findings of Tuffrey and Espeut (2015), which stressed that middle-income countries and Asia are expected to account for most of the growth in global urban populations between 2015 and 2050, and it is in line with the reasoning of Ayoo (2022) about promoting of inclusive economic growth. Consistent with the available theoretical literature, in general, our empirical findings are in line with the population-development-food security link of Villarreal and Stloukal (2005). Consequently, the patterns of future food consumption will be determined mainly by two key factors: population growth and growth in GDP per capita. In addition, the new patterns of development may have important implications for these societies. As stated by Samia et al. (2021), providing food security networks and related social assistance programs, improving farming production, fostering rural development, supporting agrarian research and investing in human capital and essential infrastructure components are the five basic options that could be considered to enhance economic development.
From the FMOLS results in Table 3, it can be confirmed that the regressors are with the expected signs and are highly significant. The cointegration that was found using the panel FMOLS model simply confirms the existence of a long-run relationship between these four variables. It should be noted that both coefficients of the rural and urban population growth represent the elasticity of the prevalence of undernourishment concerning each variable. The results suggest that the annual decrease in rural population growth leads to an increase in the prevalence of undernourishment in the population. Also, the annual increase in urban population growth leads to an increase in the prevalence of undernourishment in the population. In other words, looking at the coefficients of the regressors and their p-values from the panel cointegration FMOLS method in Table 3, it can be revealed that the changes in the explanation of the prevalence of undernourishment are under the long-term significant negative influence of the changes in the rural population growth and under the long-term positive impact of the changes in the urban population growth. There is a long-term relationship between rural population growth, urban population growth and GDP per capita growth with the prevalence of undernourishment (percent of the population) within these three groups of countries. From the causality results obtained, only relevant causation conclusions can be drawn between urban population growth and the prevalence of undernourishment in the population. Accordingly, this claim based on the causality test is much stronger. This tells us that urban population growth is a “Granger cause of the prevalence of undernourishment” because past urban population growth values explain the prevalence of undernourishment and also contain statistically significant information about the future values of the prevalence of undernourishment in the population within all three groups of countries. This finding also implies that past values of UPG contain information that helps to predict UNDERNUR over and above the information contained in past values of UNDERNUR.
Indeed, these findings give strength to the results of the FMOLS estimation as it verifies the causation effect between the dependent variable and urban population growth. Hence, the levels of prevalence of malnutrition will continue to be sensitive to differences in the future rates of urban population growth as they were also from their past values. This finding is in direct line with the claims of Tacoli et al. (2013), Tuffrey and Espeut (2015), Fanzo et al. (2020), which emphasize that those living in non-formal settlements and the poorest segments of urban populations are under a higher burden of undernutrition. Such causal estimations from FMOLS allow us to make a valid assessment of which policies to consider because urbanization or development can generate greater costs to enable food security in these countries. Urbanization presents a set of challenges and opportunities for addressing under-nutrition in low-and middle-income countries. Thus, given the further expected population growth in urban areas in low-and middle-income countries, it is time for policies to address the challenges but also seize the opportunities of these key demographic, social, and economic changes (Tacoli et al., 2013).
The DiD estimation revealed causality at 1% only for the impact of rural population growth on the prevalence of undernourishment. It means that through this time rural population growth is a cause of the variation in the prevalence of undernourishment within the three groups of countries. The estimate of the impact of the increase in the population growth rate on the prevalence of undernourishment was 0.90. This overall finding reveals that raising the rural population growth in low-income countries, lower middle-income countries and upper-middle-income countries in the world in effect led to an increase in their prevalence of undernourishment. In other words, simply putting an increase of the rate of rural population growth in these three groups of countries by 1% led to an average increase in the prevalence of undernourishment by about 0.90 percent. This finding is consistent with the work that has already been done by Tacoli et al. (2013) and (Fanzo et al., 2020), which claim that food insecurity is under strong pressure and the influence of rural poverty. Therefore, implementing interventions to reduce poverty among the rural poor should imply well-founded information about the effectiveness and more broadly about the positive and negative, as well as desired and not desired impacts of interventions (Joffe, 2007).
Overall results, especially results obtained from FMOLS and DiD models, are in coalition with the reasoning of Samia et al. (2021). According to them, the pace of reducing poverty will be the result of development in these regions of the world. Since poverty is seen as a cause and a result of a lack of food and considering that a long-term relationship was found and cointegration as well as causation, it seems that the process of reducing poverty will have a long-lasting effect through both urban and rural population growth on the prevalence of undernourishment as long as food security will be assured. Given current estimates of a rising average prevalence of undernourishment over the next period, the likelihood of a very high effect by the rural population growth within these groups of countries is persistent. However, this situation could reflect the future government spending on innovative measures in response to undernourishment within these countries, e.g., through the perspectives of transformation of the food system and agricultural intensification (Ickowitz et al., 2019; Hambloch et al., 2022). Hence, Vågsholm et al. (2020) allude that novel ways of looking at food security and sustainability are needed. The novel ways of thinking about food and sustainability should include changing the perspectives as well as responsible production and consumption of food (Vågsholm et al., 2020).
In the context of estimating the impact of policies, when causal interpretations of estimates are more plausible, the inference of causality can enhance the credibility of policy analysis. Therefore, the greater sophistication in the use of methods like differences-in-differences (DiD) estimation provides important new tools to improve the estimation of causal effects (Athey and Imben, 2017). Thus, Difference-in-differences estimation is one of the ways to estimate the effects of new policies. For instance, the new laws regarding rural development can be estimated by comparing how the prevalence of undernourishment in these three groups of countries changes before and after their implementation. Hence, Kuddus et al. (2020) seek to address the root causes of the problem. Therefore, according to them, governments and development organizations should focus on adapting to the challenges of urbanization while striving to reduce unplanned urbanization and improve the conditions in rural areas. Finally, as stated by van Zutphen et al. (2023), urban planners and policymakers have a very important role in the global malnutrition crisis and in providing ways of transformation for the food system as well as promoting inclusive economic growth (Ayoo, 2022). Therefore, the structural and rural transformations underway in low-income countries can show how an agro-sector planning approach can leverage food systems to drive sustainable and inclusive rural development (Kamal, 2017). Environmental sustainability is considered one of the essential points of the SDG and also part of social and economic sustainability as well as food security. Accordingly, the pursuit of one goal must not be at the expense of other goals (Vågsholm et al., 2020).
Despite these three econometric approaches used, our study also discusses some limitations. Even though this does not diminish the evidential value of our analysis, further research may improve the empirical methodology that was proposed in this study. To further investigate the complex process of food security and its determinants, longer data panels with more environmental information would represent valuable research qualities. Due to the available data only up to 2020, the study was not able to provide a more complete investigation into how COVID-19 has affected food security on a country-by-country basis or around low-income or middle-income countries in general. Thus, the only thing that can be observed is the trend of these variables in 2020, when the COVID crisis started and it is shown in Figures 1–4.
Conclusions and policy recommendations
The topic of food security of rural populations in low and middle-income countries has gained more cross-sectorial importance in academic and policy circles, especially related to public health, economics, and social issues (Bellon et al., 2022). The findings of this research have important implications for academic research as well as for the governments, policy debate and people to recognize and categorize high-impact food security components, enabling proper and well-informed decision-making. Particularly, the findings of this study have both methodological and policy implications. Considering the growing number of empirical attempts to offer methodological frameworks to evaluate food security, following this direction, this study provides important implications for the research methods in the social sciences field. Moreover, the implemented econometric approaches provide a different comparison between low-income, lower-middle-income and upper-middle-income countries as well as common effective responses of the same type and at the same time. The determinants found to affect food security in this study can be used as test hypotheses for other food security research studies. Therefore, the findings conclusively demonstrate the significance of the differences in rural and urban population growth in food security.
The prevalence of undernourishment as a phenomenon related closely to the food security concept exists throughout the developing world. To reduce the rate of undernourishment, policies should be targeted at the poor. Understanding long-run and causal linkages between urban population growth, rural population growth, GDP per capita growth and prevalence of undernourishment plays a decisive role in policy formulation. Three most important policy implications arise from the findings of the empirical models: (1) government support programs to help target households in securing food, (2) policy implementation to improve market access in more distant areas of the country by investing in infrastructures or by attracting private investment in the agricultural sector, (3) the presence of informal institutions, such as social networks that provide financial support, support the formation of these networks, encourage the participation of households in agricultural cooperation, agricultural associations, or community projects. It also involves greater cooperative planning between rural and urban regions to improve food security, such as subsidies to farmers who provide locally produced, unprocessed and cheap food to urban centers. Therefore, policies should take into account the distribution of undernourishment across all socioeconomic groups within these countries. Further, addressing global food insecurity and undernourishment can be achieved only in the context of wider food systems and policymaking, especially in a world that will be increasingly influenced by interconnected and multispectral risks.
For future studies, researchers can draw on a more complete, the latest, and wider range of data that will include data with environmental features. Therefore, it would be necessary to collect additional information and apply different techniques to understand the impact of environmental indicators on undernourishment in these groups of countries, including using some other proxy-dependent/outcome variable for undernutrition. In addition, a more in-depth investigation of how different key socio-economic indicators affect food security can be conducted using country-specific data if such data is available. Furthermore, future research could also examine different algorithms, including unsupervised learning algorithms, as the main method for developing predictive models to perceive if results differ. However, future research studies will need a more comprehensive database as well as qualitative information to address these topics. Finally, the effect of these indicators on food security can be modeled also in terms of asset accumulation, simulation or even household spending, provided the data is of adequate quality.
Data availability statement
The original contributions presented in the study are included in the article/supplementary material, further inquiries can be directed to the corresponding author. The data were obtained from the World Bank database: http://data.worldbank.org/indicator.
Author contributions
The author is solely responsible as a full contributor for both theoretical and empirical parts and findings of this research work.
Conflict of interest
The author declares that the research was conducted in the absence of any commercial or financial relationships that could be construed as a potential conflict of interest.
Publisher's note
All claims expressed in this article are solely those of the authors and do not necessarily represent those of their affiliated organizations, or those of the publisher, the editors and the reviewers. Any product that may be evaluated in this article, or claim that may be made by its manufacturer, is not guaranteed or endorsed by the publisher.
References
Ðordević, V., Mirilović, M., Katanić, N., Janjić, J., Nedić, D., Šarčević, D., et al. (2022). Food production and security in the area of Serbia: historical background and current situation. Meat Technol. 63, 1–10. doi: 10.18485/meattech.2022.63.1.1
Adeyemi, O., van den Bold, M., Nisbett, N., and Covic, N. (2022). Changes in Nigeria's enabling environment for nutrition from 2008 to 2019 and challenges for reducing malnutrition. Food Secur. 15, 343–61. doi: 10.1007/s12571-022-01328-2
Armanda, D. T., Guinée, J. B., and Tukkera, A. (2019). The second green revolution: Innovative urban agriculture's contribution to food security and sustainability – a review. Global Food Secur. 22, 13–24. doi: 10.1016/j.gfs.2019.08.002
Athey, S., and Imben, G. W. (2017). The state of applied econometrics: causality and policy evaluation. J. Econ. Perspect. 31, 3–32. doi: 10.1257/jep.31.2.3
Ayoo, C. (2022). Poverty reduction strategies in developing countries. Rural Development - Education, Sustainability, Multifunctionality. doi: 10.5772/intechopen.101472
Bellon, M. R., Ntandou-Bouzitou, G., Lauderdale, J. E., and Caracciolo, F. (2022). Combining market and nonmarket food sources provides rural households with more options to achieve better diets in Southern Benin. Food Secur. 2022, 1–12. doi: 10.1007/s12571-022-01320-w
Bhavani, G., Sreenivasulu, M., Naik, R. V., Reddy, M. J. M., Darekar, A. S., and Reddy, A. A. (2022). Impact assessment of seed village programme by using difference in difference (DiD) approach in Telangana, India. Sustainability, 14:9543. doi: 10.3390/su14159543
Bloom, D., and Canning, D. (2006). Global Demography: Facts, Force and Future. MPRA Paper No. 2577. Available online at: https://mpra.ub.uni-muenchen.de/2577/
Breitung, J., and Pesaran, H. (2005). Unit Roots and Cointegration in Panels. Discussion Paper Series 1: Economic Studies No 42/2005. Frankfurt am: Deutsche Bundesbank. doi: 10.2139/ssrn.2785225
Darrouzet-Nardi, A. F., and Masters, W. A. (2015). Urbanization, market development and malnutrition in farm households: evidence from the demographic and health surveys, 1986–2011. Food Sec. 7, 521–533. doi: 10.1007/s12571-015-0470-9
Fanzo, J., Covic, N., Dobermann, A., Henson, S., Herrero, M, Pingali, P., et al. (2020). A research vision for food systems in the 2020s: defying the status quo. Global Food Secur. 26:100397. doi: 10.1016/j.gfs.2020.100397
FAO (2016). Diversification strategies and adaptation deficit: Evidence from rural communities in Niger, by Asfaw, S., Palma, A., and Lipper, L. ESA Working Paper No. 16-02. Rome, FAO.
FAO (2023). Sustainable Development Goals. Indicator 2.1.1- Prevalence of undernourishment. Available online at: https://www.fao.org/sustainable-development-goals/indicators/211/en/ (accessed 02 May, 2023).
Goodman-Bacon, A. (2021). Difference-in-differences with variation in treatment timing. J. Econom. 225, 254–277. doi: 10.1016/j.jeconom.2021.03.014
Hambloch, C., Mausch, K., Conti, C., and Hall, A. (2022). Simple solutions for complex problems? what is missing in agriculture for nutrition interventions. Food Secur. 15, 363–379. doi: 10.1007/s12571-022-01324-6
Hashmi, A. A., Sial, M. H., Akram, W., and Hashmi, M. H. (2018). Assessing food insecurity trends and determinants by using mix methods in Pakistan: evidence from household pooled data (2005-2014). Sarhad J. Agric. 35, 87–101. doi: 10.17582/journal.sja/2019/35.1.87.101
Ickowitz, A., Powell, B., Rowland, D., Jones, A., and Sunderland, T. (2019). Agricultural intensification, dietary diversity, and markets in the global food security narrative. Glob. Food Secur. 20, 9–16. doi: 10.1016/j.gfs.2018.11.002
Joffe, M. (2007). Health, livelihoods, and nutrition in low-income rural systems. Food Nutr. Bull. 28, 5227–5236. doi: 10.1177/15648265070282S202
Kamal, B. (2017). How to eradicate rural poverty, end urban malnutrition. Third World Econ. 648, 13–15.
Kuddus, M. A., Tynan, E., and McBryde, E. (2020). Urbanization: a problem for the rich and the poor?. Public Health Rev. 41, 1. doi: 10.1186/s40985-019-0116-0
Pedroni, P. (2000). “Fully modified OLS for heterogeneous cointegrated panels”. in Nonstationary Panels, Panel Cointegration and Dynamic Panels, eds. Baltagi, B. H. Amsterdam: Elsevier, 93–130. doi: 10.1016/S0731-9053(00)15004-2
Pedroni, P. (2004). Panel cointegration: asymptotic and finite sample properties of pooled time series tests with an application to the PPP hypothesis. Econ. Theory 20, 597–625. doi: 10.1017/S0266466604203073
Reddy, A. A. (2016). Food security indicators in India compared to similar countries. Curr. Sci. 111, 632–640. doi: 10.18520/cs/v111/i4/632-640
Reddy, A. A., Rani, C. R., Cadman, T., Kumar, S. N., and Reddy, A. N. (2016). Towards sustainable indicators of food and nutritional outcomes in India. World J. Sci. Technol. Sustain. Dev. 13, 128–142. doi: 10.1108/WJSTSD-10-2015-0049
Righettini, M. S., and Bordin, E. (2022). Exploring food security as a multidimensional topic: twenty years of scientifc publications and recent developments. Qual. Quant. 9, 1–20. doi: 10.1007/s11135-022-01452-3
Salam, N. G., Alase, G. A., Lamidi, S. O., and Joseph, T. E. (2022). Determinant of household food expenditure: Does Engel law hold in Nigeria. Afr. Econ. Manag. Rev. 2, 11–17. doi: 10.53790/aemr.v2i3.65
Samia, N., Naárné, T., and Vasa, L. (2021). The Role of food aid and livelihood interventions in mediating the relationship between household's resilience and economic empowerment. Econ. Ann. 194, 151–163. doi: 10.21003/ea.V194-19
Tacoli, C., Bukhari, B., and Fisher, S. (2013). Urban poverty, food security and climate change. Human settlements Working Paper No.37, Rural-Urban interactions and livelihood strategies. London, UK: International Institute for Environment and Development (IIED).
Tuffrey, V., and Espeut, D. (2015). Addressing under nutrition in the context of in low-and middle-income countries. Washington, DC: MQSUN Report.
Vågsholm, I., Arzoomand, N. S., and Boqvist, S. (2020). Food security, safety, and sustainability—getting the trade-offs right. Front. Sustain. Food Syst. 4:16. doi: 10.3389/fsufs.2020.00016
van Zutphen, K. G., Barjolle, D., van den Berg, S., Gavin-Smith, B., Kraemer, K., Musard, C., et al. (2023). “Secondary Cities as Catalysts for Nutritious Diets in Low- and Middle-Income Countries,” in: Science and Innovations for Food Systems Transformation. Eds. von Braun, J., Afsana, K., Fresco, L. O., Hassan, M. H. A. Cham: Springer. doi: 10.1007/978-3-031-15703-5_16
Villarreal, M., and Stloukal, L. (2005). Population, development and food security: an unresolved challenge for the twenty-first century. Genus 61, 215–246. doi: 10.2307/29789276
World Bank (2022). World Bank Development Indicators. Available online at: https://data.worldbank.org/indicator
Xiao, J., Juodis, A., Karavias, Y., and Sarafidis, V. (2021). Improved Tests for Granger Non-Causality in Panel Data. Discussion Papers 21-06. Department of Economics, University of Birmingham. Available online at: https://ideas.repec.org/s/bir/birmec.html
Keywords: robust least squares, low-income countries, lower-middle-income countries, upper-middle-income countries, GDP per capita growth, undernourishment, FMOLS, DiD
Citation: Miladinov G (2023) Impacts of population growth and economic development on food security in low-income and middle-income countries. Front. Hum. Dyn. 5:1121662. doi: 10.3389/fhumd.2023.1121662
Received: 12 December 2022; Accepted: 02 June 2023;
Published: 19 June 2023.
Edited by:
Shah Md Atiqul Haq, Shahjalal University of Science and Technology, BangladeshReviewed by:
Mohammad Ashraful Ferdous Chowdhury, King Fahd University of Petroleum and Minerals, Saudi ArabiaA. Amarender Reddy, National Institute of Agricultural Extension Management (MANAGE), India
Copyright © 2023 Miladinov. This is an open-access article distributed under the terms of the Creative Commons Attribution License (CC BY). The use, distribution or reproduction in other forums is permitted, provided the original author(s) and the copyright owner(s) are credited and that the original publication in this journal is cited, in accordance with accepted academic practice. No use, distribution or reproduction is permitted which does not comply with these terms.
*Correspondence: Goran Miladinov, bWlsYWRpbm92Z0Bhb2wuY29t