- 1National Centre for Social Research, Institute of Social Research, Athens, Greece
- 2National Documentation Centre, Athens, Greece
- 3Department of Political Science and History, Centre for Political Research, Panteion University, Athens, Greece
This study highlights the role of Research Data Repositories (RDRs) in the concept of data reuse by examining a use case on migration research, a domain that requires up-to-date and accurate data for research and policy purposes. The survey from which the data for the use case was derived aimed to investigate the alignment of humanitarian assistance and social protection in Greece during the post-2015 refugee crisis. Through our analysis, we try to formulate a new corpus of variables and information that can create a value chain for research and policy purposes related to migration research, as well as to draw useful conclusions from this use case study in relation to the concept of data reuse. We address several issues related to data reuse, such as its definition, the role of research data repositories and research infrastructures in data reuse, as well as the limitations and advantages of reuse. We also present some specific features of the SoDaNet RDR, which hosts the primary data. We argue that comprehensive documentation of data adds value to the data and, through reuse, this value can be recycled to the RDR and, therefore, to potential new reusers.
1 Introduction
The development of Research Data Repositories (RDRs) and Research Infrastructures (RIs) have contributed favorably to data being shared and accessed more openly. The most important arguments for data sharing and reuse are the promotion of research and innovation, the transparency of research results, as well as the reallocation of public goods back to the public, which provides the resources and investments for the production of research data in the first place (Pasquetto et al., 2017).
Migration research holds an important place in the global research ecosystem, bringing together scientists from different disciplines who exchange and influence methodologies and means among scientific communities to conduct responsible research. Migration is a global phenomenon, but data describing this phenomenon are not available with the same granularity, frequency, and reliability for all parts of the world. There are more data gaps in developing countries and hard-to-reach populations (i.e., undocumented migrants, missing migrants, etc.). However, the recent refugee crisis in Europe highlighted the need for more up-to-date data within EU institutions and national governments to enable urgent responses to displaced populations and the rising need for financial and humanitarian capacity (European Commission, Directorate-General for Research and Innovation, 2022). Migration research composes an interdisciplinary complex field within which reliable data occupies a vital place. This study attempts to argue that data reusability, which is strongly dependent on data sharing, can significantly contribute to the development of migration research and, at the same time, positively impact and enhance responsible and transparent research to the benefit of science and society. The study of reusability, as conceived by the authors, can successfully fulfill these objectives in certified environments such as trusted repositories, which ensure the concept of data fairness and overall data management, including a prior research process that includes integrated operations for any interested user beyond the initial research team. Despite the dominant assumption that data sharing and open data are sine qua non-conditions in the research milieu and data world, these are not ends in themselves (Borgman, 2017; Pasquetto et al., 2017). A growing body of social science research reveals that sharing data is a complex sociotechnical process, making it hard to predict when, how, why, whether, and by whom scientific data will be reused (Borgman et al., 2015; Mosconi et al., 2019). In this regard, data reuse can significantly contribute to migration studies and research. Challenges and opportunities related to data reuse via RDRs will be analyzed within the frame of this study.
2 Data reuse
2.1 From data sharing to data reuse
One of the greatest achievements of the scientific community in recent years is undoubtedly fostering a culture of sharing and disseminating data generated by the various research projects to the wider scientific community. This emerging “culture of sharing” has the reuse of scientific data as its ultimate goal. But why is reusing data so important? There is extensive literature on the benefits of data sharing and the subsequent reuse of data. Piwowar et al. (2007, 2011) briefly present the economic benefits of investing in data-archiving infrastructure and the return it ultimately has in terms of scientific output, while Pronk (2019) stresses the importance of time efficiency gain in the reuse of data. Both the time and resources saved are tangible benefits of reusing research data. The process of collecting, processing, maintaining, and disseminating data requires a considerable investment of both time and financial resources. Therefore, reusing existing data saves resources and accelerates scientific production. It is the secondary use that acts as a “safety net” for the integrity of scientific data and the knowledge produced. Secondary users can verify or contradict the initial outputs (Sieber, 1991), the importance of which was demonstrated vividly during the “reproducibility crisis” of the last decade (Baker, 2016). At the same time, reuse exposes data to new techniques, models, and tools; different assumptions and approaches can be applied to the same datasets and combined with other datasets, highlighting new methodologies and providing new insights (Leonelli, 2013). Other beneficial aspects of data reuse are ensuring the “continuity” of research, boosting visibility, and promoting a culture of interdisciplinarity and collaboration. Furthermore, long-term preservation of data in certified repositories helps to conserve data integrity (Tenopir et al., 2011).
Meanwhile, an important factor in making data available to the community is the individual incentives of researchers, the most important being the increase in citation rates for data producers (Piwowar et al., 2011; Drachen et al., 2016), if not in the short-term, then in the long-term, as more recent studies show (Christensen et al., 2019). Other incentives are related to scientific ethics, the value of collaboration, reciprocity in scientific practice, and a “coercive environment” (Borgman, 2010), with the emergence of Open Science and FAIR data principles and the impositions of funding agencies and publishers worldwide.
1. Τhe Open Science movement, which aims to make scientific processes more transparent by creating a more reproducible and robust science and making the results of these processes more accessible (Spellman et al., 2018; Vicente-Saez and Martinez-Fuentes, 2018), is a factor that can really play a decisive role in accelerating the production of scientific knowledge (Molloy, 2011; Woelfle et al., 2011).
2. The FAIR data principles are the effort to make research data Findable, Accessible, Interoperable, and therefore Reusable (Wilkinson et al., 2016).
3. The respective policies and mandates adopted by national and supranational organizations related to research funding are important forms of pressure toward increasing data-sharing practices (Fry et al., 2008). For example, the European Commission, through the “Directive on Open Data and the Reuse of Public-sector Information” (European Commission, 2019) and the “European Strategy for data” (European Commission, 2020), demonstrates the EU’s commitment to and support for the principles of Open Science and FAIR data, therefore encouraging data reuse among researchers in crucial aspects of contemporary societies such as populations mobility and migration.
4. The publishers of various scientific journals. Following long years in which research data played a secondary role in the enterprise of scientific publishing (Vision, 2010), scientific journals are striving to overcome the various constraints they have had in terms of the data-sharing culture (Candela et al., 2015) and beginning to embrace the Open Science principles. As expected, this transition has different “accelerators” and drivers and is influenced by certain factors, such as the scientific disciplines that the journal operates in, impact factor indexes, etc. (Vasilevsky et al., 2017; Resnik et al., 2019; Rousi and Laakso, 2020).
The above factors do not influence the totality of scientific production simultaneously and with the same intensity. For example, in the social sciences, there seem to be different “temporalities” in the degree of adoption of Open Science principles (Breznau, 2021; Tedersoo et al., 2021). In any case, it is a protracted process that influences all scientific disciplines.
2.2 Data reuse constraints
However, even if data sharing has become a common practice for the majority of the scientific community, there are several issues that impede data reuse, as illustrated by the generally low rates of data reuse. Most of the insight into the constraints on the reuse of publicly available data comes from studies and research on secondary users and reuse scenarios (Curty, 2015; Faniel et al., 2015; Yoon, 2016; Cheng and Chiu, 2017; Gregory, 2020). As the categorizations that can be constructed from the wealth of all this research data and the subjective perspectives are innumerable, we will present a schema of two broad categories that emerge in relation to the process of finding and reusing data within repositories related to the social sciences and broader concerns that may arise for secondary users beyond the narrowly technical repository context.
1. Repository-specific restrictions, such as difficulties in accessing data, inability to locate licenses, non-interoperable file formats, the complete absence of incorrect or incomplete documentation, absence of accompanying files, non-user-friendly platforms, data errors, problems with data curation, etc.
2. Non-repository specific restrictions, such as researchers’ reluctance due to ethical reasons, concerns about misinterpretations and misuse, difficulties with reusability due to the initial research planning, data producers’ credibility, lack of skills or training, etc.
The relevance of the above constraints is certainly related to the intended secondary use. For example, if one tries to do a replication analysis and the replication code of the analysis is missing or incorrect, then the intended secondary use will be unsuccessful.
2.3 Different types of reuse
As van de Sandt et al. (2019) have noted, there is a lack of a strict definition of the term “reuse”, as well as the term “secondary use”, which is used in several studies as a synonym for reuse (Zimmerman, 2003; Sun and Khoo, 2017). The different definitions (Castle, 2003; Zimmerman, 2003; Francis and Francis, 2017; Sun and Khoo, 2017) highlight new dimensions of reuse, sometimes using a broader and therefore more inclusive concept and sometimes a more strictly defined one that excludes different types of reuse. For example, Zimmerman’s (2008: 634) definition of secondary analysis as “the use of data collected for one purpose to study a new problem” excludes secondary uses such as replication analyses because replication analyses do not study a “new problem” but aim to verify or refute the initial analysis. These definitions and implicit conceptualizations can, if analyzed into their individual components, yield reuse categorizations with the main variables being: (1) what is the character of data being reused, (2) who is the reuser, (3) what is the purpose of reuse, and (4) at what time point in the lifecycle of the primary data does the reuse take place (van de Sandt et al., 2019).
In our case, we will borrow Schöch’s (2017) categorization that distinguishes reuse from other similar and related concepts, as translated and edited by van de Sandt et al. (2019). This categorization (Figure 1) essentially uses three variables: (1) “same/other question,” (2) “same/other data,” and (3) “same/other method,” by assigning each of the categories a different term. Our case falls into the sixth category, that of “data reuse,” where we have:
1. A different research question
2. The same data used to answer the initial research questions
3. A different method of analysis
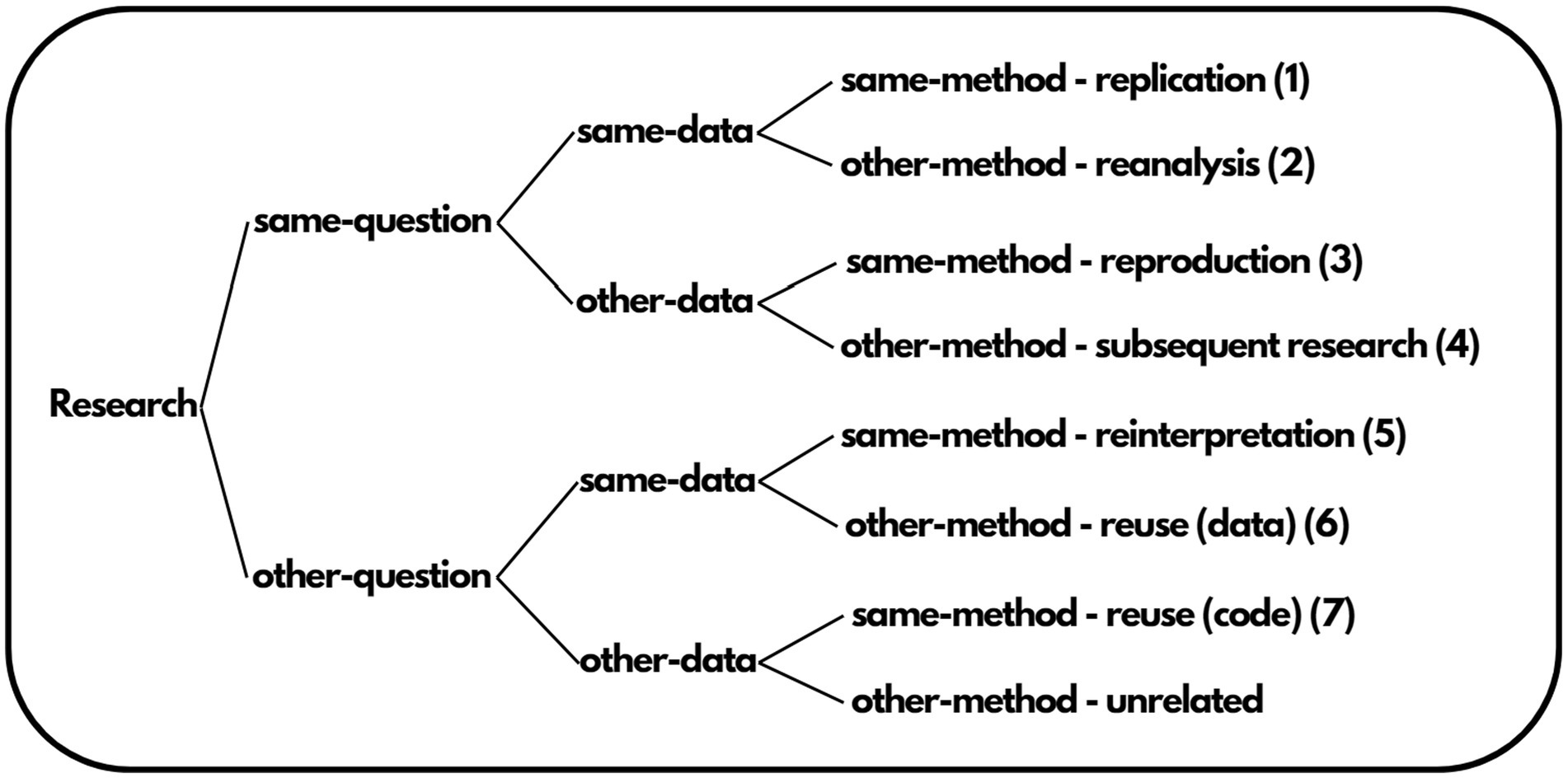
Figure 1. Schöch’s (2017) categorization distinguishes reuse from other similar and related concepts, as translated and edited by van de Sandt et al. (2019).
Therefore, we define data reuse as the process of utilizing data that are already available and have been collected to answer the research questions addressed for the primary research collection to investigate new, uncovered aspects of the primary data collection by using a different method of analysis.
2.4 The role of RDRs and research infrastructures in data reuse
In the last two decades, with the massive expansion of the internet and the transition from the old formats of scientific publications to the new digital forms of e-publishing, which also brought significant advantages (Correia and Teixeira, 2005), we have witnessed the development of online platforms for the storage and dissemination of scientific publications. What started initially with scientific publications continued with the development of platforms dedicated to hosting scientific data. RDRs are at the heart of scientific practice as they preserve, store, document, enhance, and disseminate data by acting simultaneously as intermediaries between data producers and users and depositaries of knowledge. According to a taxonomy proposed by Pampel et al. (2013), there are four RDR types:
1. Institutional RDRs,
2. Disciplinary RDRs,
3. Multidisciplinary RDRs, and
4. Project Specific RDRs.
As potential secondary users could find data from sources other than RDRs, an important role of RDRs lies in the systematic way they deliver information about data to users. In the process of depositing data into an RDR, whether done by the RDR staff or by the users themselves, specific documentation protocols are followed to ensure the availability of the necessary information about the data to ensure reliable documentation. Particularly in the era of the prevalence of FAIR Data principles (Wilkinson et al., 2016), these protocols can be quite rigorous, as efforts are directed toward ensuring sufficient documentation so that the data can eventually be meaningful to users. These documentation protocols vary between RDRs and are dependent on the business model of each institution, the established practices of the discipline, the availability of resources, etc. There are RDRs that only ensure storage of and accessibility to data, while some others perform several actions, such as the production of accompanying documents (e.g., codebook), data cleaning, logical, structural, and consistency checks, data anonymization procedures, identifier assignments, and data processing. All actions that take place during the documentation process are considered an added value to the hosted data (Daniels et al., 2012). It is widely accepted that the more actions are taken on the part of the documenter toward the completeness of the documentation, the more the added value is attributed to the data, thus assisting secondary users.
2.5 The case of SoDaNet repository
The Social Data Network (SoDaNet) is the Greek research infrastructure for the social sciences. It is a network of seven university social science departments and one research center.1 Since 2015, SoDaNet has been a member of CESSDA ERIC2 (Consortium of European Social Data Archives European Research Infrastructures Consortium), linking Greece to the European Research Area. One of the key services offered by the SoDaNet infrastructure is its repository services, which are available to both its members and the wider social science community in Greece. Recently, in an effort to improve the services offered by the infrastructure, the SoDaNet RDR adopted the Dataverse software as its main repository software (Linardis et al., 2022). As the Dataverse software is open source, it was modified to meet the repository needs at the national, European (due to its participation in CESSDA ERIC), and international levels to ensure all state-of-the-art standards and good documentation practices, such as the application of FAIR principles to the data and metadata hosted in the infrastructure (Kondyli and Klironomos, 2022). The documentation services and, by extension, the added value (Linardis and Ioannidis, 2022) given to the data include:
1. Conducting logical, structural, consistency, and anonymization checks in data and all accompanying resources.
2. Documentation of the data and accompanying resources based on DDI3 2.5 metadata standards.
3. Documentation of data files at variable, question, and variable group levels based on DDI 2.5 metadata standards for quantitative data files.
4. Storage and provision for backup for documentation and resources.
5. Determination of access and license levels per resource and data project.
6. Attribution of DOI (Digital Object Identifiers) persistent identifiers.
7. Publication of data projects in the infrastructure information system.
8. Activation of the OAI-PMH (Open Archives Initiative—Protocol for Metadata Harvesting) to enable the exposure of metadata to other portals (e.g., CESSDA Data Catalog, re3data, and EOSC portal).
These ensure that the documentation is as complete as possible, allowing for greater visibility and facilitating secondary use (Vardigan et al., 2008). At the same time, improvements have also been made to the types of data available in the RDR beyond the quantitative data that were the predominant data type in the past. More specifically, the following types of data projects can now be documented:
1. Quantitative Data
2. Qualitative Data
3. Mixed Methods Data
4. Corpora (textual data)
5. Statistical (meta)data
6. Replicas of Analyses
7. Cubes
8. Indices and Classifications
The last three categories comprise our main area of interest in the present case, as they are particularly relevant to data reuse.
1. Replicas of quantitative analyses are often a necessary accompanying document to a publication in the context of the transparency and validity of the results of scientific publication. The producer of the replica provides the secondary users with all the necessary data and code to seamlessly reproduce the entire quantitative statistical analysis that was conducted. Apart from the necessary metadata, the documentation of a replica in the RDR requires the code of the analysis along with the data used. Secondarily, since the replica refers to a publication, it may also contain various other accompanying files, such as tables, graphs, and maps.
2. Cubes are essentially multidimensional aggregated tabular data derived from primary data. A cube data project in the SoDaNet RDR is usually a collection of tables on a topic (e.g., election data for a particular election). The primary data that are used to create these cubes may or may not be available via the RDR. To document a cube, in addition to the necessary information that must be provided about its topics, it is necessary to refer to the primary sources from which the data originated and to define the dimensions of the cube (dimensional variables) as well as the measurement variables. This type of data project allows users to compile data tables from various data sources, data files, and variables of interest. These tables will help them analyze and present their results in a transparent way by documenting the whole process and offering the tables for secondary use in return.
3. Indices and Classifications refer to variable processing to create an index or a classification. The documentation of an index or a classification includes:
4. a. Metadata. Both target and source variables are specified along with their description, categories, types, and names. The sources (data files) from which the source variables were derived are also mentioned.
5. b. Resources. The algorithm with the calculation of the target variable from the source variables is required. Secondarily, a datafile to which the algorithm can be directly applied as an example is also required.
In this case of data project, we also refer to secondary use, as users can construct their own indices and classifications from existing data, document them, and then give them back to the RDR for reuse.
Figure 2 attempts to illustrate the extended research data life cycle from the researcher’s perspective. To publish the data, the producer of data chooses a certified RDR and submits it there. The RDR staff then takes all necessary steps to make the data discoverable, accessible, interoperable, and potentially reusable by the community, thus adding value to them. Secondary users who gain access to the data then start their own cycle of returning added value to the data. This secondary added value, as we call it, can take the form of primary data and/or other types of processing such as indices, classifications, tables, cubes, analysis replicas, etc.
3 Reusing data to answer different research questions: a case study
The following case study is an attempt to highlight the potential of data reuse to answer new research questions while at the same time extracting useful conclusions about the constraints that may exist in the data reuse process. We reused quantitative data on migration from the research project “Social Protection Responses to Forced Displacement” available in the SoDaNet RDR.
3.1 Identity and scope of the initial research
“Social Protection Responses to Forced Displacement” is a comparative survey in Cameroon, Colombia, and Greece that aims to better understand effective mechanisms for linking social protection programs and humanitarian assistance. The project was led by ODI4 in close collaboration with the Centre for Applied Social Sciences Research and Training (CASS-RT) in Cameroon, the School of Government at the University of Los Andes in Colombia, and the National Centre for Social Research (EKKE) in Greece. This study is part of the program “Building the Evidence on Protracted Forced Displacement: A Multi-Stakeholder Partnership”, funded by the United Kingdom’s Foreign, Commonwealth and Development Office (FCDO), managed by the World Bank Group (WBG) in partnership with the United Nations High Commissioner for Refugees (UNHCR), and implemented between 2020 and 2022. Our study, within the framework of this article, refers only to the outcomes of the project implemented in Greece, taking into account that, to the best of our knowledge, there is no comparability between the countries as of yet. The main objective was to investigate how and why different linkages can be considered by combining research theory, the evidence base, and operational guidance on how social protection systems and humanitarian systems can work together to meet the needs of those affected by displacement crises, including not only the displaced but vulnerable households in their host communities as well (Tramountanis et al., 2022).
Specifically, for the survey in Greece, a mixed approach of quantitative and qualitative methods was used to mobilize different sources of information, from targeted population samples to key informants. Research findings concluded in specific policy recommendations. Both approaches, quantitative and qualitative, led to similar results, and the qualitative approach enriched the findings of the quantitative approach with testimonies and deeper information. The quantitative survey data include a sample of the three sub-groups as follows: 752 cases of host population, 312 cases of refugees, and 432 cases of asylum seekers, which amount to a total of 1,496 respondents in both regions, Attica and Ioannina. The quantitative research was conducted through two research tools: the Computer-Assisted Telephone Interviews (CATI) was chosen as a method for the host population to harness the existing knowledge and experience EKKE has in this specific method, while the displaced population was interviewed via Tablet Assisted Personal Interviewing (TAPI), taking into consideration the possibilities of access to this population (hot spots-hubs in terms of protected and closed centers for displaced populations in both areas). The working hypothesis was formulated around four main questions to find the most appropriate linkages between social protection and humanitarian assistance for an improved operation of the two parallel systems during the first years of the so-called refugee crisis in Europe, and more specifically in Greece, due to the numbers of displaced populations moving toward the continent. The questions aimed to extract information concerning:
a. To what extent and in what ways humanitarian assistance has been linked with social protection in different contexts.
b. Factors and processes that led to the adoption of these approaches.
c. How assistance provision affects basic needs and wellbeing outcomes in displacement settings.
d. Insights for linking social protection and humanitarian assistance in different displacement contexts.
In addition to answering the new research questions on the same data, which are presented in Section 3.2 that follows, we are contributing back to the RDR and the wider community the secondary added value that we generated, namely:
1. One index that was created to answer the new research questions
2. A replication of our analysis
Both are available in the SoDaNet RDR. You can find all the links to data in the “Data Availability Statement” at the end of the article.
3.2 New research questions
Beyond the core research questions for which it was designed, the research project “Humanitarian assistance and social protection responses to the forcibly displaced in Greece” (2022) provides answers to additional pre-existing research questions that were not included in the original design or questions that arise from the findings of the research. These questions include the concept of ‘awareness’ as it relates to social protection programs.
It is of great importance that all potential beneficiaries of social protection have all the necessary information about available social protection programs. Toward this aim, a feature that can measure the “awareness” of the existence of such social programs could satisfy this interest.
In addition, the correlation between awareness and quality of life, as assessed by the respondents themselves, is part of the research interest, as is finding out whether the degree of awareness differs across different types of migration. So, the research questions that arise are:
RQ1: Does the awareness of social protection programs correlate with the quality of life?
RQ2: Does the degree of awareness differ across different types of migration?
3.3 Methodology
To investigate the research questions, appropriate indices should be created and used accordingly. “Quality of life” assessment is an element of the research tool for which no additional process is needed. The same applies to migration types. On the contrary, as far as awareness of social protection programs is concerned, it seems useful to create a ‘construct’ based on a number of questionnaire items that structure the broader concept of awareness.
The four questions related to awareness of social protection programs are listed in Table 1. Two questions are single-select items, while the other two are multi-select. For the multi-select questions, the sum of the choices divided by the total number of choices creates a (sub-) variable that values from zero to one, while for the single-select questions, a positive response (in terms of awareness) is scored as one and a negative response is scored as zero. In total, the four (sub-) variables are summed to form the construct of “awareness,” which as a variable assigns values from zero to four, with higher values indicating greater awareness of the research unit about social protection programs.
To answer the research questions based on the types of available data, appropriate descriptive measures (median, quartiles, skewness, and kurtosis coefficients) for awareness are presented. Kendall’s rank correlation coefficient tau between awareness and quality of life assessment is calculated. The Kruskal–Wallis one-way analysis of variance is also implemented to determine whether migration type differentiates awareness of social protection programs. Depending on the results from the Kruskal–Wallis test, pairwise post-hoc tests (Benjamini–Hochberg correction) are calculated. Appropriate charts that reproduce the observed results are presented.
3.4 Analysis
3.4.1 Correlation of “awareness of social protection programs” with life satisfaction
The construction for the data collected takes a minimum value of zero and a maximum value of 1.51, with higher values indicating increased awareness. The median value of awareness is 0.35; 25% of the awareness values are less than 0.18, while the largest 25% of the values are above 0.65. The interquartile range is 0.47. Awareness is right skewed, g_1 = 0.54, and has a flattened shape (g_2 = −0.52, Figure 3).
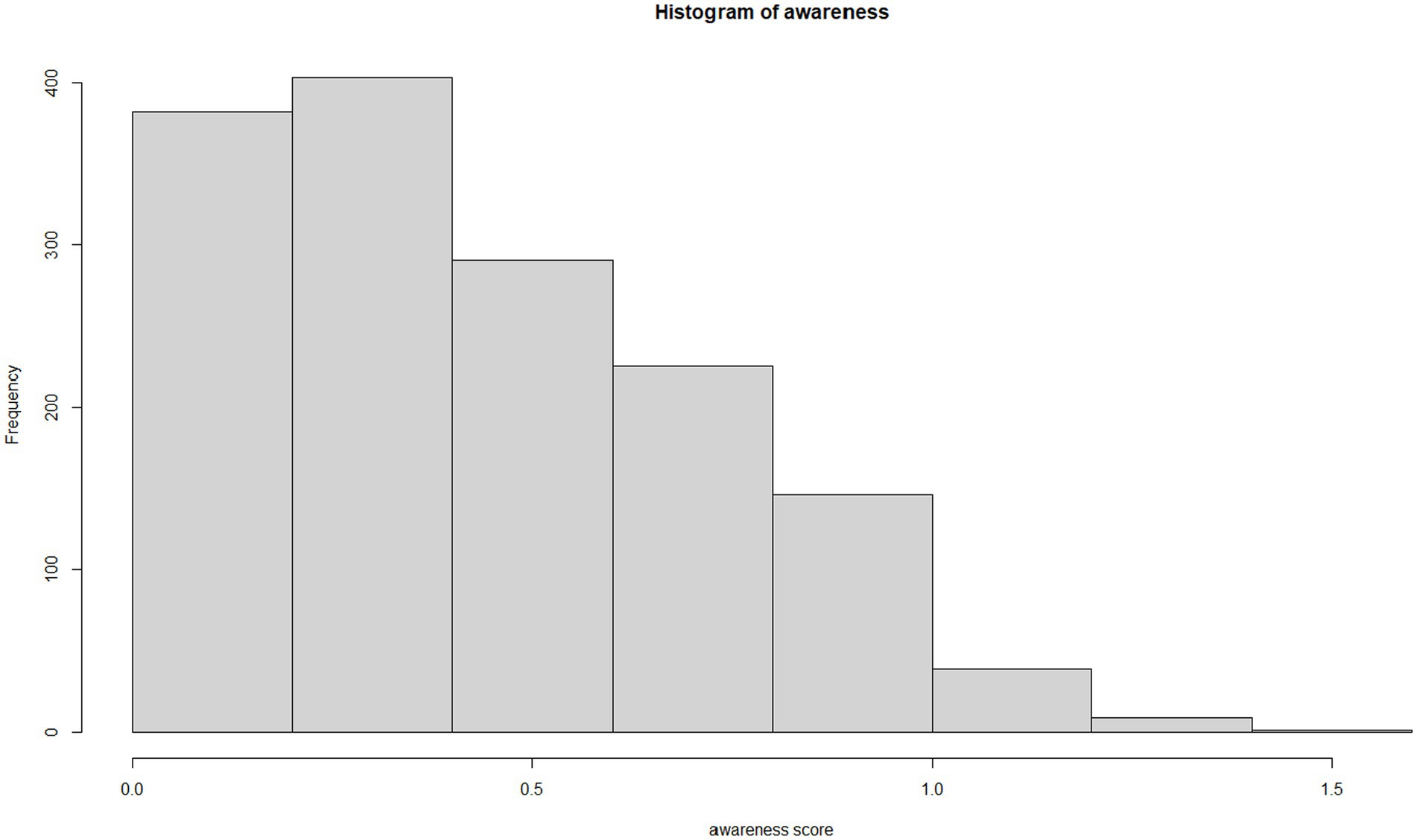
Figure 3. Representation of the distribution of awareness scores. Awareness ranges from 0 to 1.51 and has a right-skewed distribution. Values near zero indicate lower awareness of social protection programs.
To explore the central idea, a plot of awareness values versus life satisfaction is constructed (to visually capture the relationship between the variables, Figure 4), and an appropriate correlation coefficient (Kendall’s rank correlation tau) is calculated.
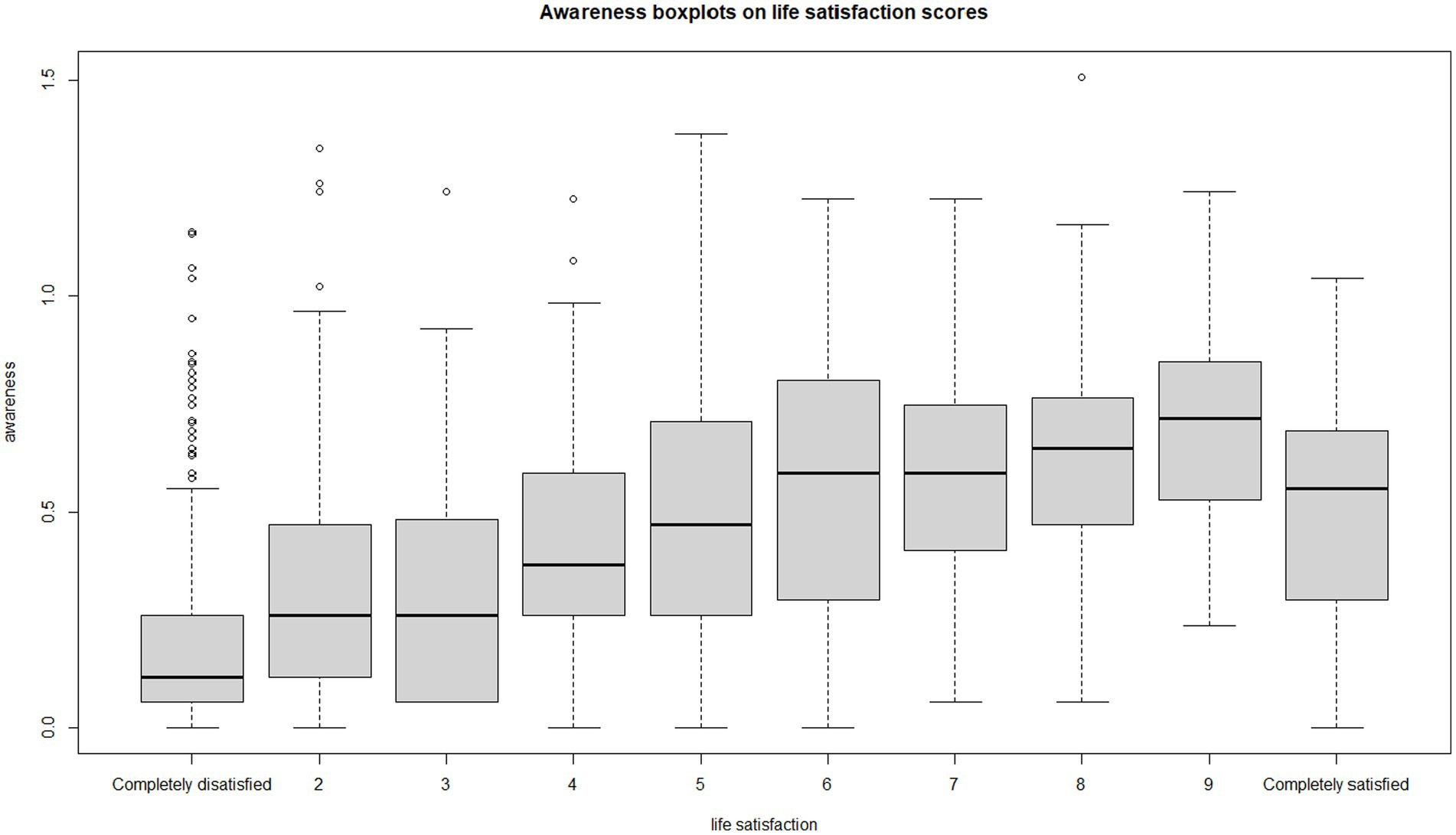
Figure 4. Boxplots of awareness scores by life satisfaction levels. Median awareness value increases as life satisfaction also increases.
From the calculation of Kendall’s rank correlation coefficient tau between awareness and life satisfaction for the entirety of the data available, one can claim that there is a statistically significant (different from zero), moderate, positive correlation (tau = 0.38, p-value < 0.001) between awareness and life satisfaction. Otherwise, the more one knows about social protection programs, the higher one rates his/her life satisfaction.
A multiple boxplot demonstrates how the awareness score changes according to the quality-of-life assessment. The almost linear relationship between the two characteristics is evident (Figure 4).
3.4.2 Association of awareness with migration type
The Kruskal–Wallis H test was conducted to study differences in awareness between different types of migration. Statistical differences (chi-squared = 302.46, p < 0.001, df = 4) were found between the five types of immigration (“Any other type of immigrant,” “Asylum seeker,” “Naturalized Greek citizen,” “Recognized refugee,” and “Returned Greek citizen who lived outside of Greece for some time”).
Post-hoc pairwise tests (with Benjamini and Hochberg correction) indicate that the above-observed differences are mainly due to differences between the two migration groups: “Any other type of immigrant,” “Naturalized Greek citizen,” and “Returned Greek citizen who lived outside of Greece for some time” on the one hand and “Asylum seeker” and “Recognized refugee” on the other. There are statistically significant differences between the types of the two groups but not between the types within the groups. The corresponding multiple scatter plot demonstrates how the awareness score varies according to the type of migration (see Table 2; Figure 5).
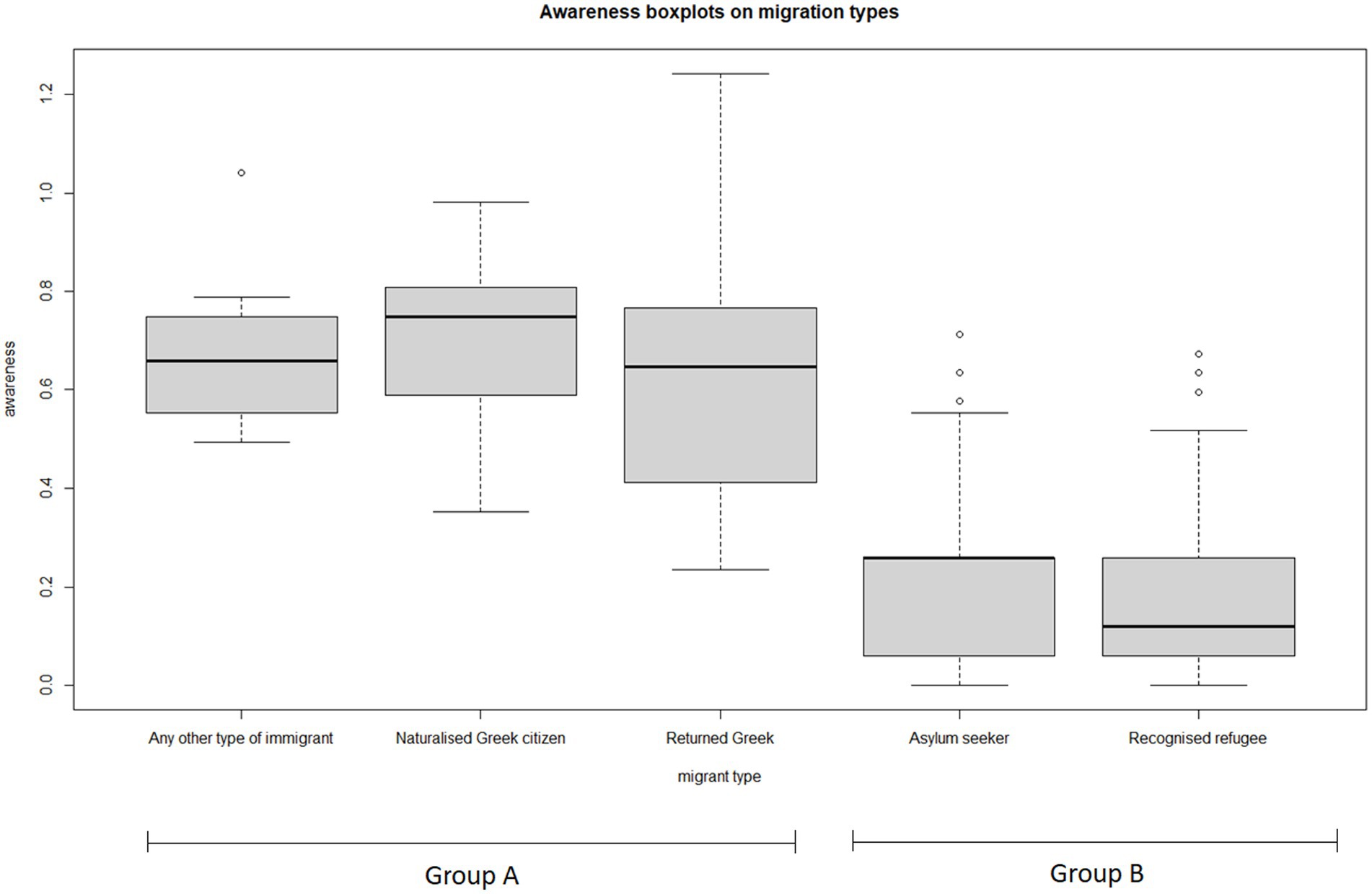
Figure 5. Boxplots of awareness scores by migration type. Median awareness values are close for migration types within groups “Naturalized Greek,” “Returned Greek,” and “Any other type of immigrant” (Group A) and “Asylum seeker” and “Recognized refugee” (Group B). Awareness median values are different between migration types from different groups.
4 Discussion
The main goal of this study is not to focus on exploring characteristics related to the intensifying phenomenon of migration but rather on the expanded range of possibilities offered by the secondary analysis of primary information. However, no one can ignore the findings themselves, which stem from the reuse of data from the research project “Social Protection Responses to Forced Displacement.” Specifically, demonstrated awareness regarding social protection programs for migrants in Greece can be generally characterized as low. The awareness index revolves around low values, with few research units showing comparatively higher scores. Overall, awareness is very low, and this constitutes a significant initial result for the wellbeing of migrants in Greece. If reinforced by the now confirmed belief in the related degree of satisfactory living, then the resulting conclusions gain particular value for all stakeholders involved in designing and implementing policies regarding migration. Even more demanding is the need to link the research findings with state and non-state entities related to migration since it is quite obvious that although immediate and comprehensive intervention is necessary in awareness issues regarding social protection programs for migrants, even more attention and emphasis should be given to two specific groups of migrants—asylum seekers and recognized refugees—whose awareness level is truly far from the desired awareness level.
4.1 Our data reuse case
Despite the dominant assumption that data sharing and open data are sine qua non-conditions in the research milieu and data world, these are not ends in themselves (Pasquetto et al., 2017). A growing body of social science research reveals that sharing data is a complex sociotechnical process, making it hard to predict when, how, why, whether, and by whom scientific data will be reused (Borgman et al., 2015; Mosconi et al., 2019). Considering that the decision to reuse the data is ultimately up to the user, and it is not a cost-free decision, it will require a significant investment of time, taking into account the type of data, the subject of the research, and all the relevant information available on the data. RDRs mediate the process by adding value to the data through data documentation. Complete and high-quality documentation from the side of the RDRs will be of great time benefit to the reuser, providing them with a fairly accurate overview of the data and assisting them in deciding whether to reuse it or not. For example, having a questionnaire as an accompanying file in survey documentation with distinct sections and groupings of questions will help the reuser avoid having to perform clustering analyses of variables to identify variables that describe a similar concept. However, as we observed in our case, no matter how comprehensive the documentation is, the reuser will have to process and analyze the data to make a conclusive dataset. In our case, even though the dataset is quite extensive, we did not answer exactly the research questions we initially had in mind, and we have had to slightly modify our research questions to obtain satisfactory analyses and, ultimately, answers. We suggest that this was mainly for two reasons:
1. When designing a survey, the questions and thus the resulting variables will obviously yield fairly good results to the research questions posed by the research project itself. However, it is possible that this may not allow space for analyses that use the same data but different questions and different methods. They could be quite useful for other categories of reuse, as described in Schöch’s (2017) scheme, if that is in the reuser’s interests. Alternatively, the secondary user should amend the research questions or even completely revise them.
2. According to the documentation of the data in the RDR as well as the report of the research project that produced the data, we realize that there was a part of the research that was conducted through personal interviews with individuals. These qualitative data are not available from the RDR, as they refer to vulnerable groups (in this case, refugees and migrants). So, our analysis misses a certain amount of data that is available. In theory, if all the data were available, we might not have needed to make adjustments to our research questions, or we might have set different ones from the very beginning. Evidently, this problem is not the RDR's responsibility, but neither is it the responsibility of the data producer. Although there is considerable literature on the importance of reusing qualitative data (Corti, 2007; Moore, 2007; Bishop, 2009; Hammersley, 2010), the practice of sharing and reusing qualitative method data is much less frequent compared to quantitative methods. Despite an increasing trend in the re-use of qualitative data (Yoon, 2014; Bishop and Kuula-Luumi, 2017; Mannheimer et al., 2018; Alexander et al., 2019; Chauvette et al., 2019) due to efforts to establish dedicated RDRs for qualitative and mixed methods data (Antonio et al., 2019) as well as to introduce and develop new tools and technologies (Elman and Kapiszewski, 2018), qualitative data often cannot be made public, especially in our case, which raises issues of ethics and accountable conduct of research when it comes to vulnerable populations. As a generalization, we argue that this issue characterizes mixed-methods research on migration and human mobility, as in many cases there are ethical issues that discourage or even prevent the reuse of such data.
4.2 The urgency and limitations of comprehensive documentation
In this study, we have often alluded to the necessity and urgency of a comprehensive and rigorous documentation of research data. Documentation of research data adds value to the data because it makes data reusable that might otherwise not be reused by anyone without in-depth knowledge of the methods and conditions under which they were produced. As Blank and Rasmussen (2004) put it: “the value of information lies in use.” However, RDRs and RIs also serve another very important purpose: they function as a “memory of science” by preserving and storing the scientific knowledge of the present to contribute to future questions or simply by providing future researchers with “reliable evidence for examining the past” (Jimerson, 2003). Comprehensive documentation that follows the state-of-the-art and good practices of today is likely to remain relevant in future by extending the life cycle of the data.
Comprehensive documentation of research data is an area in which RDRs often have limited control, as it is the producers of the data themselves who are familiar with the data. Even in cases where documentation by RDR staff takes place, the volume of information available depends on what the data producer has submitted. Therefore, a rigorous policy and supervision of the process by the RDR would be advisable. However, despite the RDR’s internal rigorous policies, or even the external impositions set by various initiatives such as the Open Science or FAIR Data principles, compliance is ultimately subject to the necessities and capacity of the individual RDR/RI. In addition, comprehensive documentation is a fairly costly process in terms of human and material resources because apart from the labor itself, it also requires the development and maintenance of the infrastructure that makes supporting such a level of service possible in a context—at least in the case of small RDRs/RIs—of chronic underfunding and differently directed demands and prioritizations by the national, regional, or institutional frameworks.
4.3 Adding value to the data
Beyond the added value that documentation brings to data, which can be defined as all the activities that an RDR performs after receiving the data to ensure its availability for reuse in the context of the data life cycle, we argue that there can also be a secondary added value—as we call it—related to reusers as data (re)producers. If the reusers determine that the data are relevant and fit for reuse, they can develop their own processings and extend the primary data’s intended scope. These processings can vary from using the data to support an argument in a presentation to using a different research method to answer novel questions, or even producing composite indicators. Since these new processings are reusable, they are subject to their own life cycle. Admittedly, not all data processings and syntheses are useful for everyone, and there is a risk of “noise” in the results delivered to the potential reuser, who operates a simple search in the RDM. We suggest that this contingency is technically manageable by RDMs and RIs if the newly acquired relevant data go through a documentation process that clearly identifies their provenance and the processing operations they have undergone. In addition, the whole process creates a chain of added value for the data, which is now accessible for reprocessing, validation, and criticism.
5 Conclusion
Migration will remain on social and political agendas globally, especially in the EU context, for the years ahead. Crises of human displacement caused by socioeconomic factors and natural disasters will impact and transform European societies. Researchers seek to investigate and contribute to the changing landscape, aware of the existence of accurate data and sources. A solution that can balance human resources, financial resources, and high-quality data can be found in RDRs and RIs. Seeing as the ultimate objective of data sharing and movements such as Open Data is the reuse of data by the research community, we believe that the role of RDRs and RIs is to add value to the research data by providing comprehensive documentation. Only in this way can data become meaningfully reusable, both in the present and in the, perhaps, unknown future, to provide multiple benefits to the scientific community throughout their lifecycle. If research data are the “prime currency” of science (Curty et al., 2017), then RDRs and RIs take on the pivotal—for the economy of science—role of “financial institutions”, hoarding, maintaining, disseminating, and re-circulating the “currency” to the wider community. Thus, the reuse of research data is as important for the scientific economy as the circulation of money is for the real economy. The more reuse that takes place, the more research questions are answered and the more the added value on the existing data. RDRs and RIs do not benefit from having data that cannot be reused; quite the opposite is true. They only need to direct their actions toward the ultimate purpose of reuse. In this form of the “circular economy” of data, there should be no “losers and winners” or “recessions and capital depreciation”: both producers and secondary users, and certainly the totality of scientific knowledge, can benefit from the reuse of data.
Data availability statement
The original contributions presented in the study are included in the article/supplementary material. The datasets [generated/analyzed] for this study can be found at https://datacatalogue.sodanet.gr.Dataset Analyzed1. Primary Data used for our case study: [Social Protection responses to Forced Displacement]. Τramountanis, Angelo; Linardis, Apostolos. (2023) SoDaNet Data Catalogue. Version 1. https://doi.org/10.17903/FK2/GBISOHDatasets Generated2. Replication of Analysis: [Replication Data for: Data reusability for migration research: a case study from SoDaNet Research Infrastructure] Kondyli, Dimitra; Nisiotis, C.S.; Klironomos, Nicolas. (2023). SoDaNet Data Catalogue. Version 1. https://doi.org/10.17903/FK2/CCWUGK3. Index: [Awareness Index] Kondyli, Dimitra; Nisiotis, C.S.; Klironomos, Nicolas. (2023)SoDaNet Data Catalogue. Version 1. https://doi.org/10.17903/FK2/Q9E8BXFurther inquiries can be directed to the corresponding author.
Author contributions
DK: Conceptualization, Funding acquisition, Investigation, Methodology, Project administration, Resources, Supervision, Validation, Writing – original draft, Writing – review & editing. CSN: Data curation, Formal analysis, Methodology, Resources, Validation, Visualization, Writing – original draft, Writing – review & editing, Conceptualization, Supervision. NK: Conceptualization, Data curation, Formal analysis, Investigation, Methodology, Project administration, Resources, Validation, Visualization, Writing – original draft, Writing – review & editing.
Funding
The author(s) declare financial support was received for the research, authorship, and/or publication of this article. The data used for the article are stored at the EKKE’s RDR of SoDaNet RI for social sciences, which has received funding for its construction phase within the frame of the Action “SoDaNet in Action” funded by the Greek Business Program Competitiveness Entrepreneurship and Innovation and co-financed by the European Regional Development Fund (ERDF).
Conflict of interest
The authors declare that the research was conducted in the absence of any commercial or financial relationships that could be construed as a potential conflict of interest.
The handling editor HQ declared a shared research group with the author DK at the time of review.
Publisher’s note
All claims expressed in this article are solely those of the authors and do not necessarily represent those of their affiliated organizations, or those of the publisher, the editors and the reviewers. Any product that may be evaluated in this article, or claim that may be made by its manufacturer, is not guaranteed or endorsed by the publisher.
Footnotes
1. ^For more details please visit: https://sodanet.gr.
2. ^For more details please visit: https://www.cessda.eu.
3. ^Data Documentation Initiative, from more details please visit: https://ddialliance.org.
4. ^ODI, an independent, global think tank. For more information: https://odi.org/en/about/.
References
Alexander, S. M., Jones, K., Bennett, N. J., Budden, A., Cox, M., Crosas, M., et al. (2019). Qualitative data sharing and synthesis for sustainability science. Nat. Sustain. 3, 81–88. doi: 10.1038/s41893-019-0434-8
Antonio, M. G., Schick-Makaroff, K., Doiron, J. M., Sheilds, L., White, L., and Molzahn, A. (2019). Qualitative data management and analysis within a data repository. West. J. Nurs. Res. 42, 640–648. doi: 10.1177/0193945919881706
Baker, M. (2016). 1,500 scientists lift the lid on reproducibility. Nature 533, 452–454. doi: 10.1038/533452a
Bishop, L. (2009). Ethical sharing and reuse of qualitative data. Aust. J. Soc. Issues 44, 255–272. doi: 10.1002/j.1839-4655.2009.tb00145.x
Bishop, L., and Kuula-Luumi, A. (2017). Revisiting qualitative data reuse: a decade on. SAGE Open 7:215824401668513. doi: 10.1177/2158244016685136
Blank, G., and Rasmussen, K. B. (2004). The data documentation initiative. Soc. Sci. Comput. Rev. 22, 307–318. doi: 10.1177/0894439304263144
Borgman, C. L. (2010) Research data: who will share what, with whom, when, and why? Presented at the China-North America library conference, Beijing. Available at: http://www.nlc.gov.cn/yjfw/zm/index_en.html
Borgman, C. L. (2017) Big data, little data, no data: scholarship in the networked world. Cambridge, MA: The MIT Press.
Borgman, C. L., Darch, P. T., Sands, A. E., Pasquetto, I. V., Golshan, M. S., Wallis, J. C., et al. (2015). Knowledge infrastructures in science: data, diversity, and digital libraries. Int. J. Digit. Libr. 16, 207–227. doi: 10.1007/s00799-015-0157-z
Candela, L., Castelli, D., Manghi, P., and Tani, A. (2015). Data journals: a survey. J. Assoc. Inf. Sci. Technol. 66, 1747–1762. doi: 10.1002/asi.23358
Castle, J. E. (2003). Maximizing research opportunities: secondary data analysis. J. Neurosci. Nurs. 35, 287–290. doi: 10.1097/01376517-200310000-00008
Chauvette, A., Schick-Makaroff, K., and Molzahn, A. E. (2019). Open data in qualitative research. Int. J. Qual. Methods 18:160940691882386. doi: 10.1177/1609406918823863
Cheng, W., and Chiu, M. P. (2017). How do medical researchers use open health data? A case study on data reuse behavior of using NHIRD in Taiwan. Proc. Assoc. Inf. Sci. Technol. 54, 637–639. doi: 10.1002/pra2.2017.14505401097
Christensen, G., Dafoe, A., Miguel, E., Moore, D. A., and Rose, A. K. (2019). A study of the impact of data sharing on article citations using journal policies as a natural experiment. PLoS One 14:e0225883. doi: 10.1371/journal.pone.0225883
Correia, A. M., and Teixeira, J. (2005). Reforming scholarly publishing and knowledge communication: from the advent of the scholarly journal to the challenges of open access. Online Inf. Rev. 29, 349–364. doi: 10.1108/14684520510617802
Corti, L. (2007). Re-using archived qualitative data – where, how, why? Arch. Sci. 7, 37–54. doi: 10.1007/s10502-006-9038-y
Curty, R. G. (2015) "Beyond “Data Thrifting”: An Investigation of Factors Influencing Research Data Reuse In the Social Sciences" [Dissertation] [Syracuse (NY)]: Syracuse University. Available at: https://surface.syr.edu/etd/266
Curty, R. G., Crowston, K., Specht, A., Grant, B. W., and Dalton, E. D. (2017). Attitudes and norms affecting scientists’ data reuse. PLoS One 12:e0189288. doi: 10.1371/journal.pone.0189288
Daniels, M., Faniel, I., Fear, K., and Yakel, E. (2012) ‘Managing fixity and fluidity in data repositories’, proceedings of the 2012 iConference [preprint]. doi: 10.1145/2132176.2132212
Drachen, T. M., Ellegaard, O., Larsen, A. V., and Fabricius Dorch, S. B. (2016). Sharing data increases citations. LIBER Q. 26, 67–82. doi: 10.18352/lq.10149
Elman, C., and Kapiszewski, D. (2018). The qualitative data repository’s annotation for transparent inquiry (ATI) initiative. PS Polit. Sci. Polit. 51, 3–6. doi: 10.1017/s1049096517001755
European Commission . (2019) Directive (EU) 2019/1024 of the European Parliament and of the Council of 20 June 2019 on open data and the re-use of public sector information (recast). Available at: https://eur-lex.europa.eu/legal-content/EN/TXT/?qid=1561563110433&uri=CELEX:32019L1024
European Commission . (2020) A European Strategy for Data. Available at: https://digital-strategy.ec.europa.eu/en/policies/strategy-data#:~:text=The%20Data%20Act%20is%20a,the%20European%20economy%20and%20society
European Commission, Directorate-General for Research and Innovation (2022) Science, research and innovation performance of the EU 2022: Building a sustainable future in uncertain times. Publications Office of the European Union. Available at: https://data.europa.eu/doi/10.2777/78826
Faniel, I. M., Kriesberg, A., and Yakel, E. (2015). Social scientists’ satisfaction with data reuse. J. Assoc. Inf. Sci. Technol. 67, 1404–1416. doi: 10.1002/asi.23480
Francis, L. P., and Francis, J. G. (2017). Data reuse and the problem of group identity. Stud. Law Politics Soc. 73, 141–164. doi: 10.1108/s1059-433720170000073004
Fry, J., Lockyer, S., Oppenheim, C., Houghton, J., and Rasmussen, B. (2008) Identifying benefits arising from the curation and open sharing of research data produced by UK higher education and research institutes. JISC Report. Available at: http://repository.jisc.ac.uk/279/
Gregory, K. (2020). A dataset describing data discovery and reuse practices in research. Sci. Data 7:232. doi: 10.1038/s41597-020-0569-5
Hammersley, M. (2010). Can we re-use qualitative data via secondary analysis? Notes on some terminological and substantive issues. Sociol. Res. Online 15, 47–53. doi: 10.5153/sro.2076
Jimerson, R. C. (2003). Archives and memory. OCLC Syst. Serv. Int. Digit. Libr. Perspect. 19, 89–95. doi: 10.1108/10650750310490289
Kondyli, D., and Klironomos, N. (2022). “FAIR data: opportunities and challenges for research infrastructures and research communities” in Development of infrastructures for data production and management in the social sciences. eds. J. Kallas, et al. (Athens, GR: Alexandria), 231–251.
Leonelli, S. (2013). Integrating data to acquire new knowledge: three modes of integration in plant science. Stud. Hist. Philos. Biol. Biomed. Sci. 44, 503–514. doi: 10.1016/j.shpsc.2013.03.020
Linardis, A., Alexandris, K., and Klironomos, N. (2022). “The new SoDaNet data catalogue. The transition from Nesstar to Dataverse” in Development of infrastructures for data production and management in the social sciences. eds. J. Kallas, et al. (Athens, GR: Alexandria), 147–183.
Linardis, A., and Ioannidis, A. (2022) “Extending the life-cycle of quantitative studies and data: the role of data repositories” In Development of infrastructures for data production and management in the social sciences, ed. J. Kallas et al. (Athens, GR: Alexandria), 43–73.
Mannheimer, S., Pienta, A., Kirilova, D., Elman, C., and Wutich, A. (2018). Qualitative data sharing: data repositories and academic libraries as key partners in addressing challenges. Am. Behav. Sci. 63, 643–664. doi: 10.1177/0002764218784991
Molloy, J. C. (2011). The open Knowledge Foundation: open data means better science. PLoS Biol. 9:e1001195. doi: 10.1371/journal.pbio.1001195
Mosconi, G., Li, Q., Randall, D., Karasti, H., Tolmie, P., Barutzky, J., et al. (2019). Three gaps in opening science. Comput. Support. Coop. Work 28, 749–789. doi: 10.1007/s10606-019-09354-z
Pampel, H., Vierkant, P., Scholze, F., Bertelmann, R., Kindling, M., Klump, J., et al. (2013). Making research data repositories visible: the re3data.org registry. PLoS One 8:e78080. doi: 10.1371/journal.pone.0078080
Pasquetto, I. V., Randles, B. M., and Borgman, C. L. (2017). On the reuse of scientific data. Data Sci. J. 16:8. doi: 10.5334/dsj-2017-008
Piwowar, H. A., Day, R. S., and Fridsma, D. B. (2007). Sharing detailed research data is associated with increased citation rate. PLoS One 2:e308. doi: 10.1371/journal.pone.0000308
Piwowar, H. A., Vision, T. J., and Whitlock, M. C. (2011). Data archiving is a good investment. Nature 473:285. doi: 10.1038/473285a
Pronk, T. E. (2019). The time efficiency gain in sharing and reuse of research data. Data Sci. J. 18:10. doi: 10.5334/dsj-2019-010
Resnik, D. B., Morales, M., Landrum, R., Shi, M., Minnier, J., Vasilevsky, N. A., et al. (2019). Effect of impact factor and discipline on journal data sharing policies. Account. Res. 26, 139–156. doi: 10.1080/08989621.2019.1591277
Rousi, A. M., and Laakso, M. (2020). Journal research data sharing policies: a study of highly-cited journals in neuroscience, physics, and operations research. Scientometrics 124, 131–152. doi: 10.1007/s11192-020-03467-9
Schöch, C. (2017). “Wiederholende Forschung in den digitalen Geisteswissenschaften” in DHd2017 Digitale Nachhaltigkeit. ed. v. M. Stolz (Bern: DHd-Verband)
Sieber, J. E. (1991). Openness in the social sciences: sharing data. Ethics Behav. 1, 69–86. doi: 10.1207/s15327019eb0102_1
Spellman, B. A., Gilbert, E. A., and Corker, K. S. (2018). Open Science. Stevens’ Handbook of Experimental Psychology and Cognitive Neuroscience. 5, 1–47.
Sun, G., and Khoo, C. S. G. (2017). Social science research data curation. Libellarium 9, 59–80. doi: 10.15291/libellarium.v9i2.291
Tedersoo, L., Küngas, R., Oras, E., Köster, K., Eenmaa, H., Leijen, Ä., et al. (2021). Data sharing practices and data availability upon request differ across scientific disciplines. Sci. Data 8:192. doi: 10.1038/s41597-021-00981-0
Tenopir, C., Allard, S., Douglass, K., Aydinoglu, A. U., Wu, L., Read, E., et al. (2011). Data sharing by scientists: practices and perceptions. PLoS One 6:e21101. doi: 10.1371/journal.pone.0021101
Tramountanis, A., Linardis, A., Mouriki, A., Gerakopoulou, P., Kondyli, D., Papaliou, O., et al. (2022) ‘Humanitarian assistance and social protection responses to the forcibly displaced in Greece’. Available at: https://odi.org/en/publications/humanitarian-assistance-and-social-protection-responses-to-the-forcibly-displaced-in-greece/
van de Sandt, S., Dallmeier-Tiessen, S., Lavasa, A., and Petras, V. (2019). The definition of reuse. Data Sci. J. 18:22. doi: 10.5334/dsj-2019-022
Vardigan, M., Heus, P., and Thomas, W. (2008). Data documentation initiative: toward a standard for the social sciences. Int. J. Digit. Curation 3, 107–113. doi: 10.2218/ijdc.v3i1.45
Vasilevsky, N. A., Minnier, J., Haendel, M. A., and Champieux, R. E. (2017). Reproducible and reusable research: are journal data sharing policies meeting the mark? PeerJ 5:e3208. doi: 10.7717/peerj.3208
Vicente-Saez, R., and Martinez-Fuentes, C. (2018). Open science now: a systematic literature review for an integrated definition. J. Bus. Res. 88, 428–436. doi: 10.1016/j.jbusres.2017.12.043
Vision, T. J. (2010). Open data and the social contract of scientific publishing. Bioscience 60, 330–331. doi: 10.1525/bio.2010.60.5.2
Wilkinson, M. D., Dumontier, M., Aalbersberg, I. J. J., Appleton, G., Axton, M., Baak, A., et al. (2016). The fair guiding principles for scientific data management and stewardship. Sci. Data 3:160018. doi: 10.1038/sdata.2016.18
Woelfle, M., Olliaro, P., and Todd, M. H. (2011). Open science is a research accelerator. Nat. Chem. 3, 745–748. doi: 10.1038/nchem.1149
Yoon, A. (2014). “Making a square fit into a circle”: researchers’ experiences reusing qualitative data. Proc. Am. Soc. Inf. Sci. Technol. 51, 1–4. doi: 10.1002/meet.2014.14505101140
Yoon, A. (2016). Red flags in data: learning from failed data reuse experiences. Proc. Assoc. Inf. Sci. Technol. 53, 1–6. doi: 10.1002/pra2.2016.14505301126
Zimmerman, A. S. (2003) ‘Data sharing and secondary use of scientific data: experiences of ecologists’, Dissertation, The University of Michigan, Michigan. Available at: http://hdl.handle.net/2027.42/39373.
Keywords: data reuse, secondary analysis, repositories, Open Science, FAIR data, use case, research infrastructure, migration
Citation: Kondyli D, Nisiotis C-S and Klironomos N (2024) Data reusability for migration research: a use case from SoDaNet data repository. Front. Hum. Dyn. 5:1310420. doi: 10.3389/fhumd.2023.1310420
Edited by:
Haodong Qi, Malmö University, SwedenReviewed by:
Charlotte Till, Arizona State University, United StatesOlympia Kaminioti, Ministry of Family and Social Cohesion, Greece
Copyright © 2024 Kondyli, Nisiotis and Klironomos. This is an open-access article distributed under the terms of the Creative Commons Attribution License (CC BY). The use, distribution or reproduction in other forums is permitted, provided the original author(s) and the copyright owner(s) are credited and that the original publication in this journal is cited, in accordance with accepted academic practice. No use, distribution or reproduction is permitted which does not comply with these terms.
*Correspondence: Dimitra Kondyli, ZGtvbmR5bGlAZWtrZS5ncg==
†These authors have contributed equally to this work and share first authorship