- 1Department of Systems Innovation, Graduate School of Engineering Science, Osaka University, Osaka, Japan
- 2ERATO ISHIGURO Symbiotic Human-Robot Interaction Project, Osaka University, Osaka, Japan
It is well-known that artificial agents, such as robots, can potentially exert social influence and alter human behavior in certain situations. However, it is still unclear what specific psychological factors enhance the social influence of artificial agents on human decision-making. In this study, we performed an experiment to investigate what psychological factors predict the degree to which human partners vs. artificial agents exert social influence on human beings. Participants were instructed to make a decision after a partner agent (human, computer, or android robot) answered the same question in a color perception task. Results indicated that the degree to which participants conformed to the partner agent positively correlated with their perceived interpersonal closeness toward the partner agent. Moreover, participants' responses and accompanying error rates did not differ as a function of agent type. The current findings contribute to the design of artificial agents with high social influence.
Introduction
Artificial agents, such as robots, have become increasingly sophisticated as a result of recent progress in Artificial Intelligence (AI) and robotics. Such artificial agents will continue to become even more embedded in human society as technology continues to advance. As the role of AI, robots, and other such technological advances within human society become increasingly pronounced, it is valuable to consider the impact that their social influence may have upon human behavior.
“Social influence,” which refers to implicit or explicit influences from surrounding individuals, has long been investigated in the field of social psychology (Cialdini and Goldstein, 2004). An example of social influence that has caused a considerable amount of interest in this field is the phenomenon of conformity. Many previous experiments have suggested that participants' responses conform to the behaviors and utterances of other individuals, even during the execution of extremely easy tasks, which they should be able to answer correctly without much effort in general (Asch, 1951; Germar et al., 2014). For example, Asch (1951) showed that when a participant was presented with three geometric lines and asked to answer which line was of equal length to a reference line, the participant was more likely to err when other participants—who were actually confederates—all chose the incorrect answer, despite the length of the incorrect line obviously differing from that of the reference line. Germar et al. (2014) conducted a similar study utilizing a color perception task wherein participants were asked to select the dominant color of a bi-colored picture. Although the dominant color was easily observable, participants were more likely to make an error when the confederates all chose the incorrect answer. In addition to research that has examined the influence of human agents, several studies have also investigated whether human beings will conform to suggestions or decisions of artificial agents (Beckner et al., 2016; Gaudiello et al., 2016; Shiomi and Hagita, 2016). Interestingly, some of these studies have suggested that artificial agents can indeed make humans conform in certain conditions (Gaudiello et al., 2016).
However, it is also known that the degree to which humans conform to artificial agents is rather weak compared to how they conform in response to human agents (Beckner et al., 2016; Shiomi and Hagita, 2016). Therefore, in order to enhance the social influence of artificial agents, it is necessary to investigate the psychological factors that are associated with social influence in human beings. One example of such a previous attempt is a study by Hertz and Wiese (2016), in which the authors hypothesized that the level of an agent's humanness may have an effect on conformity. To do so, they conducted an Asch line judgment task (Asch, 1951) with three types of agents—human, computer, and humanoid—all of which were presented to the participants via a screen. However, their results demonstrated that the humanness of the agent did not affect participants' level of conformity. Furthermore, Gaudiello et al. (2016) investigated whether negative attitudes toward robots and a desire for control were associated with conformity; again, these two variables were found to have no relation. With the exception of these studies, there are currently few promising indices for quantifying the psychological factors that may predict the social influence of an artificial agent.
One possible alternative to examining the psychological factors associated with an artificial agent's social influence is to examine the mental closeness between such agents and humans. It has previously been demonstrated that behavior synchrony between humans and agents can induce a human being to conform more with the agent's suggestions or requests (Paladino et al., 2010; Wiltermuth, 2012). Furthermore, research by Aron et al. (1992) has found that the degree of synchrony is closely linked to the Inclusion of Other in the Self (IOS) Scale, which is a measurement tool that evaluates perceived interpersonal closeness. However, their research did not examine a direct link between the IOS Scale and conformity. Given the link between behavior synchrony and conformity, we hypothesize that perceived interpersonal closeness (as measured via the IOS Scale) is a possible predictor of conformity.
We have also assessed other psychological measures to explore whether there is any other predictor of conformity, —namely, the Dyadic Trust Scale (Larzelere and Huston, 1980) and the Godspeed Questionnaire (Bartneck et al., 2009). The former scale assesses trust in the context of interpersonal relationships, whereas the latter questionnaire assesses how participants perceive the robot's anthropomorphism, animacy, likeability, intelligence, and safety. Although Hertz and Wiese (2016) maintain that it is unlikely that anthropomorphism has a connection with social influence, it is possible that social influence may be related to some other aspects by which robots are perceived by humans.
In this study, we attempted to reveal the key psychological factors enhancing social influence from artificial agents. To do so, we conducted an Asch-like experiment analogous to that of Germar et al. (2014), wherein participants were instructed to identify the dominant color of a bi-colored image together with an agent (a human confederate, a computer, or an android). The agent occasionally made purposeful errors (e.g., answering “blue” when the image obviously contained more orange), which allowed us to examine whether or not participants' answers conformed to that of their partner agents. Following the experiment, participants were administered the two aforementioned questionnaires in order to determine what factors predicted their conformity. Utilizing a stepwise regression, we examined which psychological factors best predicted the likelihood that participants would exhibit conformity in such situations.
Materials and Methods
Participants
Recruitment of the participants was done by posting a registration form on a social networking service. A total of 44 participants took part in the experiment (26 males, 18 females; mean age: 20.5 years, standard deviation: 1.5). Of these, 15 participants interacted with an android (Android Condition), 15 with a computer (Computer Condition), and 14 with a human (Human Condition). All participants provided written informed consent prior to the experiment, which was approved by the Graduate School of Engineering Science of Osaka University.
Measures
We assessed three psychological measurements, Inclusion of Other in the Self Scale (IOS), Dyadic Trust Scale, and Godspeed Questionnaire. IOS is a single item measurement. The interpersonal closeness is expressed by the degree of overlap across two circles, which represent self and other. Participant is requested to choose one of the seven figures presented with different degree of overlaps. The Dyadic Trust Scale was assessed by seven point Likert scale, and its Cronbach's alpha was α = 0.81. The Godspeed Questionnaire consists of five categories and their Cronbach's alpha values for this study were, anthropomorphism α = 0.88, animacy α = 0.81, likeability α = 0.80, intelligence α = 0.83, and safety α = 0.79. Each item was questioned by a five point Likert scale.
Stimuli
Bi-colored blue and orange pictures were prepared for the experiment, which consisted of randomly ordered blue and orange pixels. The proportion of orange pixels in the pictures was varied (46, 50, 54%). For each proportion, four different pictures were generated, thus making a total of 12 different pictures. These colors and proportions matched the pictures used in the previous study by Germar et al. (2014). The pictures were displayed on a large screen using software to control the precise duration and to randomize their order of appearance.
We prepared three agents for the experiment: android (Figure 1), computer (Figure 2), and human (Figure 3). For the Android Condition, we used Geminoid-F, a female-type android robot with an extremely human-like appearance. For the Computer Condition, a combination of a Bose Wireless Speaker and a Logitech Webcam were used. The webcam was set to act as the eye of the computer, although it actually did nothing. For the Human Condition, two female confederates, both with acting experience, were recruited. This condition was conducted across two separate days. On the first day, six participants interacted with one of the confederates, and on the second day, eight participants with the other confederate. The android and computer were programmed so that for each type of picture (four of each), they answered, “Orange is dominant” twice and “Blue is dominant” twice. A text-to-speech system was utilized for the vocalizations. The human confederates were instructed to respond to the question according to a secret cue imbedded on the screen which was given just before they were requested to answer the dominant color. The cues were given so that they matched the response programmed to the Android and Computer Conditions. After completion of the color perception task, participants were asked whether they noticed the cue or not. None of the participants detected the presence of the secret cues.
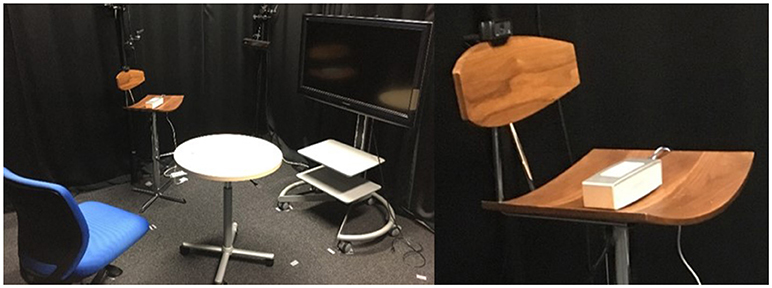
Figure 2. Computer condition. The speaker is set on a chair, and the camera is attached on the backrest.
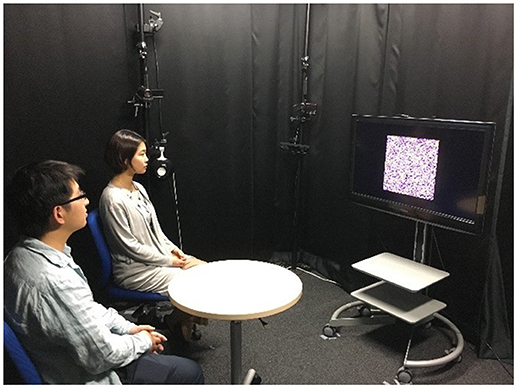
Figure 3. Human condition. The participant is on the left, and the female confederate is on the right. Written informed consent was obtained from the depicted individuals for the publication of these images.
The human confederate and android robot kept their gaze toward the screen throughout the experiment without making any eye contact with the participants. Facial and body movements were limited to eye blinks and slight neck motion only, maintaining a basic appearance. The utterances of the agents were uniformed to “Blue/Orange is dominant.”
Experimental Procedure
Following the informed consent, participants completed the color perception task, which consisted of four sessions per participant: two sessions with an agent and two sessions alone. The experiment was conducted with one participant and one agent at a time.
For the Android Condition and the Computer Condition, a single participant was invited into the experiment room. The agents were introduced as having AI with color perception abilities. For the Human Condition, the participant and confederate both entered the room together, with the confederate acting the role of another participant who did not know what was going to occur. The participant (and the confederate) was then instructed to view 12 bi-colored pictures for each session and to state the dominant color for each when requested. When the task was conducted together with the agent, a fixation cross was shown, the participant was told to concentrate on the screen and to closely examine the bi-color picture because it would only be displayed for 1.5 s. When the program asked, “What do you think the dominant color is?” the agent answered first. The program then asked the participant to answer by saying “How about you?” After the participant had responded, the program asked participants to direct their attention to the screen for the next trial by stating, “The next picture will now be displayed. Please look at the screen.” Then, the above sequence was restarted, beginning with the fixation cross, until the cycle had been repeated 12 times.
When participants conducted the task alone, a similar program was used with a few modifications. After the picture had been displayed, two checkboxes—one labeled “orange” and the other “blue,” and a button labeled “NEXT”—were displayed. Using a mouse, the participant was first instructed to mark the checkbox that he or she considered to be the dominant color and to then press the “NEXT” button to initiate the sequence once more. This cycle was also repeated 12 times.
To facilitate participants' understanding of the task, the experimenter walked through the above programs with the participants step-by-step prior to beginning the experimental trials. Once participants understood the procedure, the experimental sessions (with the agent and alone) were executed in alternating order, with the order of sessions counterbalanced across participants. After all four sessions, participants were administered the questionnaires (IOS Scale, Godspeed Questionnaire).
Data Analysis
We evaluated the difference in responses made by the participants during the task. When the ambiguous picture (orange proportion 50%) was shown, we counted the number of times that participants matched their answer with that of the agent and compared the difference of responses they made across the two colors. We also compared the number of errors they made to ascertain whether social influence was present even in tasks where it was difficult to make an error. Here, we defined an error as the number of times participants' responses were congruent with the agents' incorrect answers when viewing the unambiguous pictures (orange proportion 46 or 54%). We then calculated the total number of times participants matched the agents' answers when observing the ambiguous pictures, as well as the errors, and examined the relationship of this number to the two scales: IOS Scale, and Godspeed Questionnaire.
Results
Figure 4 shows participants' responses when they viewed the ambiguous pictures. A 2 (Agent's Response: Blue vs. Orange) × 3 (Agent: Android vs. Computer vs. Human) ANOVA revealed significant difference in participants' response across colors the agent mentioned. When the agent responded “orange,” participants were significantly more likely to respond “orange” compared to when the agent responded “blue” [F(2, 41) = 6.71, p < 0.05]. No main effect was found for agent type and interaction between color and agent.
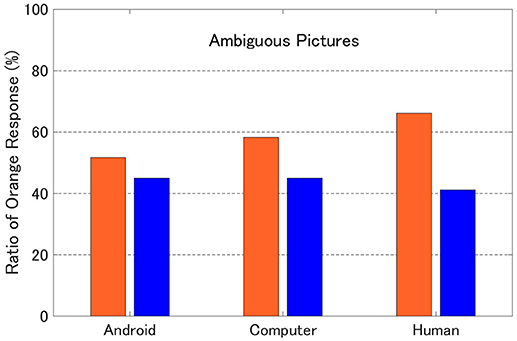
Figure 4. Participants' responses to ambiguous pictures. The vertical axis indicates the ratio of the number of times participants answered “orange.” The horizontal axis corresponds to the type of agent. The blue bar represents when the agent responded “blue” and the orange bar when they answered “orange”.
Figure 5 displays the error rate for unambiguous trials. A 2 (Situation: with Agent, Alone) × 3 (Agent: Android, Computer, Human) mixed ANOVA revealed a main effect of Agent vs. Alone [F(2, 41) = 9.9, p < 0.01], indicating that participants were more likely to make errors when conducting the task with an agent rather than when conducting it alone. There was no main effect for type of agent or any interactions across factors.
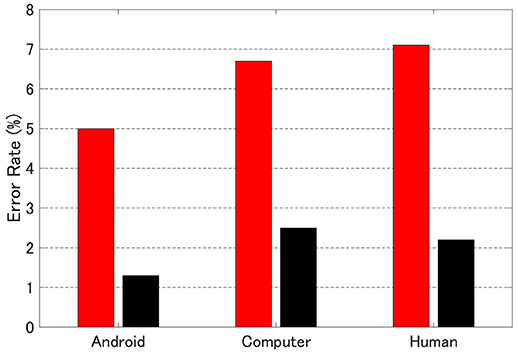
Figure 5. Error rate across conditions (agent type, with/without agent). Here, error rate is defined by the number of times participants failed to distinguish the dominant color of the bi-color image, which had either a 46 or a 54% ratio of orange pixels. The horizontal axis shows the type of agent. The red bar shows the error rate when conducting the session with an agent, and the black bar the rate when conducted alone.
Last, we examined which scale best predicted the number of congruent responses by conducting a stepwise regression, in which the IOS Scale, Dyadic Trust Scale, and Godspeed Questionnaire were included as independent variables, and the number of congruent responses was included as the dependent variable. Results revealed that the model using the IOS Scale [F(2, 42) = 8.59, β = 0.52, p < 0.01] best predicted the number of congruent responses (Table 1).
Discussion
The present study found that perceived interpersonal closeness (as measured by the IOS Scale) was the main psychological factor predicting the degree to which participants conformed to the agents' answers. This result is consistent with previous research demonstrating a relationship between perceived closeness toward others and behavior synchrony, as well as between conformity and synchrony (Paladino et al., 2010). Importantly, our study revealed a direct relationship between perceived interpersonal closeness and conformity, thus adding the final piece to the puzzle.
Furthermore, our results demonstrated that participants were equally likely to conform to the agents' response regardless of the type of agent. When ambiguous pictures were shown, participants' responses were biased accordingly to the agents' response (Figure 4), and when the agent purposely made errors, participants were more likely to make errors as well during the unambiguous trials (Figure 5). These results suggest that explicit humanness has little or no connection with social influence. These results further confirm Hertz and Wiese's (2016) finding that the humanness of the agents does not affect the level of conformity. In contrast to the study by Hertz and Wiese, though, the type of robot used and the manner by which agents were displayed differed in the current study. In Hertz and Wiese's study, a robot with a mechanical appearance was used, and all agents were displayed on a screen rather than sharing the same space with the participant. Our results thus extend their study by revealing that even in close proximity with the agent, conformity does not depend on humanness. Indeed, it was surprising that the effect of the computer—an agent with a minimalistic appearance—did not differ from that of the other two far more sophisticated agents. Given that speaker-type agents are becoming more popular in the current era (e.g., Amazon Echo, Google Home, Apple HomePod), it is worthwhile to investigate the extent to which these agents can influence users' behavior.
In the present study, although significant difference was observed, the error rate when participants were alone being ~2%, while with an agent it was ~6%, indicating that social influence did not exert a very powerful effect. Recent study on conformity and robots by Salomons et al. have shown that when robots make a mistake in the decision making task, humans are less likely to conform with the robots' response (Salomons et al., 2018). In the present experiment, the agents made obvious errors multiple times. It may be that these continuous errors lead the participants to conform less to the agents' responses.
The present study also provides suggestions for how we may be able to increase the error rate, that is, by increasing perceived interpersonal closeness and/or establishing behavior synchrony prior to the color perception task. Previous studies have already pointed out that humans display behavior synchrony not only toward other humans (Nakano and Kitazawa, 2010), but also toward an android (Tatsukawa et al., 2016). Furthermore, such studies have shown that adding a modality (e.g., gaze, touch) that biases humans' attitudes toward the android can change the degree of synchrony (Tatsukawa et al., 2016). Thus, if we introduce other modalities prior to the present experiment, we may be able to establish stronger behavior synchrony and, in turn, observe greater congruence with the agents' answers. Similarly, we can also try including an interaction that enhances participants' feelings of a strong bond with the android, with the aim of increasing perceived interpersonal closeness. Bickmore and Picard (2005) have developed a number of strategies for establishing relationships in the context of human-computer interactions, and these strategies may also be useful with regard to human-robot interactions. We will make further endeavors to confirm these hypotheses in the future.
Inducing errors may take some special treatments. However, implication gained from one of the recent study suggests that when it comes to leading someone to a correct answer, the agent's utterance may have a strong influence. Ullrich et al. replicated Asch's experiment paradigm, replacing one of the four confederates with a robot (Ullrich et al., 2018). They have shown that when only the robot responded with the correct answer (the other three confederates choosing the incorrect answer), the participants' correct choice rate was higher compared with when only one human confederate chose the correct answer. This implies that robots can be more trustworthy than humans in certain conditions. It may be worthwhile examining what type of agent is most influential in leading to correct answers, and what psychological factor best predicts the strength of such influence.
Finally, the present findings can be beneficial for developing a robot that is capable of carrying out social tasks more effectively. One possible application can be seen in a study by Watanabe et al. (2014), who conducted a field experiment in a department store to ascertain how people perceived their conversations with an android. Interestingly, their findings showed that people felt the android to be a humanlike entity, even if they interacted in a limited capacity. Thus, if such androids can be made to exert a stronger social influence, we may be able to strengthen the degree to which they are perceived as social entities, consequently enabling people to accept robots as our social peers.
In conclusion, the present study investigated the relationship between participants' degree of conformity and two psychological scales: the IOS Scale, and Godspeed Questionnaire. We conducted an Asch-like experiment and found that agents, regardless of their type, can exert social influence to some degree. More importantly, perceived interpersonal closeness was found to positively correlate with the level of congruence between the participants' responses and agents' responses. In future research, we will seek to expand upon these findings and devise a way to strengthen the social influence that a robot can exert upon human beings.
Author Contributions
KT, HT, YY, and HI designed the research. KT performed the research. KT conducted the analyses. KT, HT, and YY wrote the manuscript.
Funding
This work was supported by the Japan Science and Technology Agency (JST); the Exploratory Research for Advanced Technology (ERATO); the ISHIGURO symbiotic human-robot interaction project (JPMJER1401); the Japanese Ministry of Education, Culture, Sports, Science, and Technology (MEXT); and a Grant-in-Aid for Scientific Research on Innovative Areas titled Cognitive Interaction Design, A Model-based Understanding of Communication and its Application to Artifact Design (No. 4601) (No. 15H01618).
Conflict of Interest Statement
The authors declare that the research was conducted in the absence of any commercial or financial relationships that could be construed as a potential conflict of interest.
References
Aron, A., Aron, E. N., and Smollan, D. (1992). Inclusion of Other in the Self Scale and the structure of interpersonal closeness. J. Pers. Soc. Psychol. 63, 596–612. doi: 10.1037/0022-3514.63.4.596
Asch, S. E. (1951). “Effects of group pressure upon the modification and distortion of judgments,” in Groups, Leadership, and Men, ed H. Guetzkow (Pittsburgh, PA: Carnegie Press), 222–236.
Bartneck, C., Kulić, D., Croft, E., and Zoghbi, S. (2009). Measurement instruments for the anthropomorphism, animacy, likeability, perceived intelligence, and perceived safety of robots. Int. J. Soc. Robot. 1, 71–81. doi: 10.1007/s12369-008-0001-3
Beckner, C., Rácz, P., Hay, J., Brandstetter, J., and Bartneck, C. (2016). Participants conform to humans but not to humanoid robots in an English past tense formation task. J. Lang. Soc. Psychol. 35, 158–179. doi: 10.1177/0261927X15584682
Bickmore, T. W., and Picard, R. W. (2005). Establishing and maintaining long-term human-computer relationships. ACM Trans. Comput. Hum. Interact. 12, 293–327. doi: 10.1145/1067860.1067867
Cialdini, R. B., and Goldstein, N. J. (2004). Social influence: compliance and conformity. Annu. Rev. Psychol. 55, 591–621. doi: 10.1146/annurev.psych.55.090902.142015
Gaudiello, I., Zibetti, E., Lefort, S., Chetouani, M., and Ivaldi, S. (2016). Trust as indicator of robot functional and social acceptance. An experimental study on user conformation to iCub answers. Comput. Hum. Behav. 61, 633–655. doi: 10.1016/j.chb.2016.03.057
Germar, M., Schlemmer, A., Krug, K., Voss, A., and Mojzisch, A. (2014). Social influence and perceptual decision making: a diffusion model analysis. Pers. Soc. Psychol. Bull. 40, 217–231. doi: 10.1177/0146167213508985
Hertz, N., and Wiese, E. (2016). Influence of agent type and task ambiguity on conformity in social decision making. Proc. Hum. Fact. Ergon. Soc. Annu. Meet. 60, 313–317. doi: 10.1177/1541931213601071
Larzelere, R. E., and Huston, T. L. (1980). The dyadic trust scale: toward understanding interpersonal trust in close relationships. J. Marr. Fam. 42, 595–604. doi: 10.2307/351903
Nakano, T., and Kitazawa, S. (2010). Eyeblink entrainment at breakpoints of speech. Exp. Brain Res. 205, 577–581. doi: 10.1007/s00221-010-2387-z
Paladino, M. P., Mazzurega, M., Pavani, F., and Schubert, T. W. (2010). Synchronous multisensory stimulation blurs self-other boundaries. Psychol. Sci. 21, 1202–1207. doi: 10.1177/0956797610379234
Salomons, N., van der Linden, M., Strohkorb Sebo, S., and Scassellati, B. (2018). “Humans conform to robots: disambiguating trust, truth, and conformity,” in Proceedings of the 2018 ACM/IEEE International Conference on Human-Robot Interaction (Chicago, IL), 187–195.
Shiomi, M., and Hagita, N. (2016). “Do synchronized multiple robots exert peer pressure?,” in Proceedings of the Fourth International Conference on Human Agent Interaction, (Singapore: Biopolis), 27–33.
Tatsukawa, K., Nakano, T., Ishiguro, H., and Yoshikawa, Y. (2016). Eyeblink synchrony in multimodal human-android interaction. Sci. Rep. 6:39718. doi: 10.1038/srep39718
Ullrich, D., Butz, A., and Diefenbach, S. (2018). “Who do you follow?: social Robots' impact on human judgment,” in Companion of the 2018 ACM/IEEE International Conference on Human-Robot Interaction (ACM), 265–266.
Watanabe, M., Ogawa, K., and Ishiguro, H. (2014). “Field study: can androids be a social entity in the real world?,” in Proceedings of the 2014 ACM/IEEE International Conference on Human-Robot Interaction (Bielefeld), 316–317.
Keywords: agents, robot, social influence, conformity, interpersonal closeness
Citation: Tatsukawa K, Takahashi H, Yoshikawa Y and Ishiguro H (2018) Interpersonal Closeness Correlates With Social Influence on Color Perception Task Using Human and Artificial Agents. Front. ICT 5:24. doi: 10.3389/fict.2018.00024
Received: 18 April 2018; Accepted: 29 August 2018;
Published: 26 September 2018.
Edited by:
Stefan Kopp, Bielefeld University, GermanyReviewed by:
Isabelle Hupont, UMR7222 Institut des Systèmes Intelligents et Robotiques (ISIR), FranceAnna Pribilova, Slovak University of Technology in Bratislava, Slovakia
Copyright © 2018 Tatsukawa, Takahashi, Yoshikawa and Ishiguro. This is an open-access article distributed under the terms of the Creative Commons Attribution License (CC BY). The use, distribution or reproduction in other forums is permitted, provided the original author(s) and the copyright owner(s) are credited and that the original publication in this journal is cited, in accordance with accepted academic practice. No use, distribution or reproduction is permitted which does not comply with these terms.
*Correspondence: Kyohei Tatsukawa, dGF0c3VrYXdhLmt5b3VoZWlAaXJsLnN5cy5lcy5vc2FrYS11LmFjLmpw