- 1Department of Electrical and Computer Engineering, Aristotle University of Thessaloniki, Thessaloniki, Greece
- 2Third Neurological Clinic, G. Papanikolaou Hospital, Thessaloniki, Greece
- 3Department of Neurology, Hippokration Hospital, Thessaloniki, Greece
- 4Department of Neurology, Technical University Dresden, Dresden, Germany
- 5Faculdade de Motricidade Humana, Universidade de Lisboa, Lisbon, Portugal
- 6International Parkinson Excellence Research Centre, King's College Hospital NHS Foundation Trust, London, United Kingdom
- 7Department of Electrical and Computer Engineering, Khalifa University of Science and Technology, Abu Dhabi, United Arab Emirates
Parkinson's Disease (PD) is a neurodegenerative disorder with early non-motor/motor symptoms that may evade clinical detection for years after the disease onset due to their mildness and slow progression. Digital health tools that process densely sampled data streams from the daily human-mobile interaction can objectify the monitoring of behavioral patterns that change due to the appearance of early PD-related signs. In this context, touchscreens can capture micro-movements of fingers during natural typing; an unsupervised activity of high frequency that can reveal insights for users' fine-motor handling and identify motor impairment. Subjects' typing dynamics related to their fine-motor skills decline, unobtrusively captured from a mobile touchscreen, were recently explored in-the-clinic assessment to classify early PD patients and controls. In this study, estimation of individual fine motor impairment severity scores is employed to interpret the footprint of specific underlying symptoms [such as brady-/hypokinesia (B/H-K) and rigidity (R)] to keystroke dynamics that cause group-wise variations. Regression models are employed for each fine-motor symptom, by exploiting features from keystroke dynamics sequences from in-the-clinic data captured from 18 early PD patients and 15 healthy controls. Results show that R and B/H-K UPDRS Part III single items scores can be predicted with an accuracy of 78 and 70%, respectively. The generalization power of these trained regressors derived from in-the-clinic data was further tested in a PD screening problem using data harvested in-the-wild for a longitudinal period of time (mean ± std : 7 ± 14 weeks) via a dedicated smartphone application for unobtrusive sensing of their routine smartphone typing. From a pool of 210 active users, data from 13 self-reported PD patients and 35 controls were selected based on demographics matching with the ones in-the-clinic setting. The results have shown that the estimated index achieve {0.84 (R), 0.80 (B/H−K)} ROC AUC, respectively, with {sensitivity/specificity : 0.77/0.8 (R), 0.92/0.63 (B/H−K)}, on classifying PD and controls in-the-wild setting. Apparently, the proposed approach constitutes a step forward to unobtrusive remote screening and detection of specific early PD signs from mobile-based human-computer interaction, introduces an interpretable methodology for the medical community and contributes to the continuous improvement of deployed tools and technologies in-the-wild.
1. Introduction
Parkinson's Disease (PD) is the second most common neurodegenerative disorder after Alzheimer's Disease (Shulman et al., 2011) with a wide clinical spectrum of motor and non-motor symptoms (Chaudhuri et al., 2006), which are mild in the early stages and are causing progressive disability at the later ones. The underlying neuropathological process is preceding the onset of relevant PD motor symptoms up to decades, leaving the disease undiagnosed for years (Hawkes et al., 2010; Schrag et al., 2015). PD is creating significant impact on patients' quality of life, that, in part, is caused by a wide variety of motor impairments, such as brady-/hypokinesia (B/H-K) and rigidity (R), being, yet, less evident for the person concerned due to their mildness in the early stages of the disease. Furthermore, degradation in motor function is reflected to patient's motor behavioral patterns, e.g., fine motor movements, speed of reflex movements and intermittent tremor. Diagnosis of PD is made by a movement disorders specialist who assesses, usually clinically, the patient's overall condition using questionnaires and standardized scales, such as the Unified Parkinson's Disease Rating Scale (Fahn et al., 1987). UPRDS Part-III (Goetz et al., 2008) consists of 14 single items qualitatively measuring the range of PD motor symptomatology, evaluated by experts during the examination of specific tasks.
Objective and frequent evaluation with quantitative measures can assist the clinical decision making process on PD diagnosis and patients' monitoring. Nevertheless, in clinical examination, subject's self-reports are frequently involved as a source of information, subjected to the experience of the physician to assess the severity of the PD symptoms. Information and Communication Technology (ICT)-based solutions (Mellone et al., 2012) and plethora of related data can assist the relevant stakeholders to better understand the disease's impact on daily habits, even in the early stages, as well as patient's response to drug therapy. An emerging field of ICT where large-scale data streams are acquired from users' habitual patterns is human-mobile interaction. The latter can reveal everyday information that could be transformed to useful behavioral indices, built in a dynamic and personalized way across the time of interaction. Design of digital monitoring tools for PD with diagnostic value has been a research field with a great variety of applications, due to the wide spectrum of PD clinical symptoms. Efforts processing data streams as captured from ICT devices, have proven to be robust in distinguishing populations facing motor symptoms from healthy ones, in different sub-tasks in-the-clinic assessment, such as voice (Orozco-Arroyave et al., 2016), gait or tremor (Abdulhay et al., 2018).
Digital health solutions with potential transferability to real-life environments (in-the-wild) is a challenging step to capture both useful disease indicators and achieve long-term adherence. Bot et al. (2016) were the first ones that reported a large-scale smartphone-based PD-related study, namely mPower, with over 9,000 participants (both PD and healthy users), aiming remote PD screening by suggesting patients to perform designed digitized tests to assess motor-functionalities and self-reported questionnaires. However, drop out rates highlighted that the specific tests are not viable for long term user engagement. Moreover, Zhan et al. (2018) have recently used a mobile application to assess longitudinal PD patients via tests on five scheduled scenarios, and by using sensorial data analysis, they proposed an aggregated index that was correlated with the total UPDRS Part III score. Although both aforementioned studies paved the way for smartphone-based PD assessment, they included the requirement of users' active interaction with the mobile application. This requirement, however, is a non-resilient factor for avoiding drop outs and subjects are possibly subjected to Hawthorne effect (Monahan and Fisher, 2010). Non-obtrusive and passive sensing of data could overcome the latter barriers in designing such monitoring tools. Such an example is a recent study (Arroyo-Gallego et al., 2018) designed to unobtrusively perform data collection from keystroke typing on physical keyboard on subjects' PCs, in order to detect subjects with PD by using a machine learning approach. In fact, a numerical index was produced that related hold time keystrokes to the total UPDRS Part-III score. A possible drawback of this approach (Giancardo et al., 2016), was the use of the total UPDRS Part-III score as the regression target as it encapsulates both relevant (e.g., B/H-K) and irrelevant (e.g., voice degradation, gait) items with keystroke typing and fine-motor movement. The produced index performed 0.83 Area Under Curve (AUC) of the Receiving Operating Characteristic (ROC) with 0.77/0.72 sensitivity/specificity in classifying PD patients and healthy users, when they were typing in-the-clinic setting, and 0.76 AUC and sensitivity/specificity of 0.73/0.69 during the remote at-home-setting assessment.
Fine-motor skills decline can also be detected from typing patterns on mobile touchscreen as derived from recent similar works on typing pattern analysis on mobile touchscreen (Arroyo-Gallego et al., 2017; Iakovakis et al., 2018), during in-the-clinic experiments. In our latest study, we proposed a feature vector representation of enriched keystroke information and a two-stage machine learning-based pipeline to process multiple typing sessions as captured from mobile touchscreen, performing 0.92 AUC with 0.82/0.81 sensitivity/specificity on early PD and healthy subject's classification. Subjects typed multiple typing sessions during in-the-clinic medical examination, the derived typing sequences were analyzed and the study findings resulted in four keystroke features with high discriminative power, and a plausible connection with symptoms.
Motivated by the aforementioned, the current study steps further by analyzing keystroke information with respect to specific motor symptoms that are possibly causing the variations to PD patients' typing patterns. The analysis direction is aiming to increase the interpretation of the produced indexes, by targeting the UPDRS Part III single item scores that are related to the PD fine motor symptoms. The first part of this study is the exploitation of the best performing features from mobile touchscreen typing in the binary setting (control or PD patient), as predictors of specific UPDRS Part III single items, in order to predict each symptoms' severity in the standardized medical scale, so to be easily interpreted by medical experts. From a methodological point of view, by employing regression models, numerical indexes were produced, which describe the severity of the motor symptomatology on typing kinetics, and tested with a leave-one-subject-out (LOSO) validation of symptom's severity estimation, using keystroke features as dependent variables; the target variables were the UPDRS Part III single item scores of each symptom. The conceptualization of this analysis direction was reinforced from the correlation results between both the employed features and the specific UPDRS Part III single items, and the variation that the symptoms had plausibly caused to keystroke distribution coming from PD patients.
Furthermore, the second contribution of the present work is the testing of the generalization power of these trained regressors from the in-the-clinic to the in-the-wild data analysis. Mobile touchscreen typing data were unobtrusively captured from users via a dedicated smartphone application. More specifically, the employment of the developed models in-the-wild setting was used to investigate further their diagnostic performance and their time response to a longitudinal manner. Multi modal data were collected through a developed research data donation application (i-Prognosis, 2017), and a third-party keyboard was included to capture keystroke dynamics from routine typing. The variance and noise induced to the data from the daily activities in the uncontrolled setting, is a real-life challenge when screening in an unobtrusive manner. However, touchscreen typing is a high-frequent activity and usually is an activity with cognitive attention; factors that contribute to the retainment of user's specific patterns in keystroke dynamics across time.
The present work is in line with the efforts toward predictive analytics approaches, both in-the-clinic and in-the-wild data analysis, in capturing PD-related early signs. This could potentially add in building an effective PD prediction system, taking into consideration the pragmatic conditions of everyday living, for automatic remote inference and recommendation of PD diagnosis and management.
2. Materials and Methods
Based on the findings of our previous work (Iakovakis et al., 2018), this study exploits the most discriminative features as the representation of typing patterns on mobile touchscreen, and make use of regression models to estimate individual UPDRS Part III single items scores relevant to fine-motor impairment. As it is depicted in Figure 1, the selected features are used as the independent variables for estimating the symptoms' severity which are the UPDRS Part III single items scores, used as the target variables for the training of the different regression models. A LOSO evaluation with nested cross validation for regressors' optimization scheme is used in the development set (DSet) in-the-clinic (Figure 1A), to identify the models and symptoms that can be predicted, and the generalization of the methods' is tested in-the-wild scenario (Figure 1B). The estimators are evaluated in their diagnostic properties using ROC based performance in the binary setting of classifying PD and healthy users. The goal of this employment is to investigate the diagnostic properties of the method as well as the transferability potential.
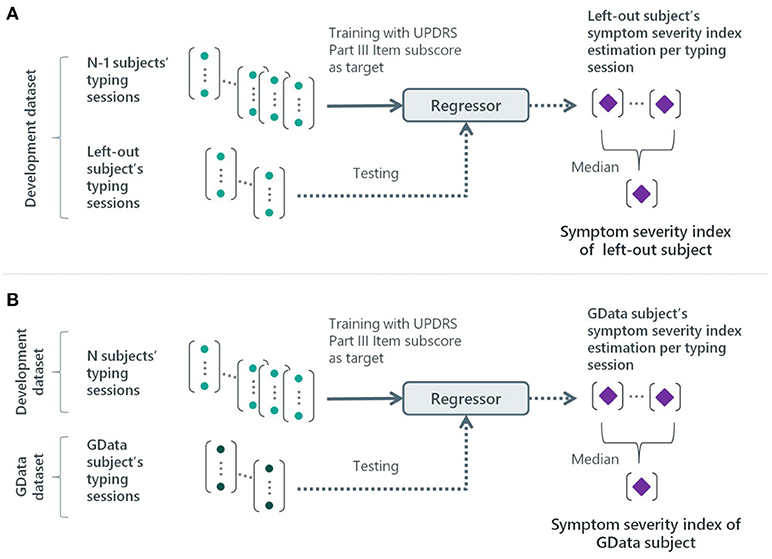
Figure 1. Schematic representation of the methodological steps referring to: (A) the DSet keystroke data from touchscreen typing captured in-the-clinic setting and (B) the extension of the best resulted models from the DSet to the GData. LOSO-based training/testing is used to evaluate which regressor can achieve better estimation of motor symptoms via the use of keystroke dynamics features as inputs and UPDRS Part III single item scores as targets. The regressors that efficiently capture the scale of symptoms severity are used in-the-wild setting for subjects characterization as PD or control.
2.1. Data Collection
2.1.1. In-the-Clinic Data
The DSet consisted of data acquired from 33 subjects who provided data on a day of visit at the clinic. The collection protocol included a typing experiment of multiple text excerpts on smartphones and a clinical evaluation. These data were logged in a spreadsheet file and mapped to the subjects coded IDs by the neurologist. The specific UPDRS Part III single item scores used in the regression analysis were item 31/ 21/ 22/ 23 for Bradykinesia-Hypokinesia/ Tremor/ Rigidity/ Finger Taps, respectively. The DSet study protocol was approved by the Aristotle University of Thessaloniki, Greece (Bioethics Committee of Medical School, approval no. 359/3.4.17). Written informed consent was obtained from all subjects prior to their participation in the study and the procedures carried out were according to institutional and international guidelines on research studies involving adult human beings. Subjects held the right to withdraw from the procedure at any time, without providing any justification. Recruitment and study procedures were carried out according to institutional and international guidelines on research involving adult human beings. PD patients under medication (14) has mean/std Levadopa equivalent dose of 237/156, and were asked to refrain from taking it for at least 8 h before their visit. More detailed information about the dataset acquisition and study cohort can be found in Iakovakis et al. (2018).
2.1.2. In-the-Wild Data
The data captured in-the-wild (GData) were collected by the i-PROGNOSIS remote data collection study (GData study). Subjects from four countries across EU contributed pseudo-anonymised multi modal data remotely (e.g., voice, handling) via downloading the mobile application from Google Play store and enrolling in the study. The application provides information regarding the study details on its first launch and gives subjects the option to communicate with medical representatives in each country in case of additional questions. An electronic informed consent was obtained from all subjects enrolled, within the smartphone application, by digitally signing a dated consent form. Due to the remote nature of the study, obtaining written consent was impractical. Subjects held the right to withdraw from the procedure at any time via the available option within the application and even request the deletion of the collected data. Subjects of GData have the option to use the third-party keyboard included in the application named iPrognosis App, as to capture the keystroke dynamics during their routine typing activities. All the experimental and ethical protocols were approved by Ethik-Kommission an der Technischen Universität Dresden, Dresden, Germany (EK 44022017), Greece, Bioethics Committee of the Aristotle University of Thessaloniki Medical School, Thessaloniki, Greece (359/3.4.17), Portugal Conselho de Ética, Faculdade de Motricidade Humana, Lisbon, Portugal (CEFMH 17/2017), and United Kingdom London, Dulwich Research Ethics Committee (17/LO/0909).
Two hundred and ten total users provided 42,812 typing sessions (mean/std 204/460 typing sessions per user). However, only subjects who self-reported to be aged between 48 and 80 years old were included in the analysis in order to be in the same age group with the subjects in the DSet, resulting in a total of 48 subjects.
2.1.3. Data Capturing
The application for capturing keystroke-related data includes a custom software keyboard developed for the Android Operating System (OS) by three authors (DI, SH, and VH). The users have to enable the software keyboard after the application installation and set it as the default input method. Subjects of the in-the-clinic assessment performed the typing task using the custom keyboard and a common smartphone provided by the authors, whereas in-the-wild subjects used the keyboard with their own mobile devices. In the background, a class of the software keyboard captured the timestamps of press and release touch events for each key tap, as well as the normalized pressure (0.000–1.000) on each press event as outputted by the OS. Each key tap was also flagged as a long-press event, corresponding to deliberate special keyboard actions, or not. The characters typed were not captured, as the context of what is being typed is not required for the analysis, rendering our data collection process privacy-aware. For each typing session (keyboard shown and afterwards hidden, with at least one key tap in the meantime), sequences of captured data were stored in JSON format and were indexed as database entry in a local SQLite database, available only to the application. The application periodically transmitted database entries to a remote cloud server (Microsoft Azure) when the user's device was connected to Wi-Fi and charging. Each entry was accompanied by a unique coded ID of the user to ensure privacy.
2.2. Feature Extraction and Regression Analysis
2.2.1. Keystroke Dynamic Features
The derivatives of time-stamp sequences of touchscreen key press and release produce the so-called hold time (HT) and flight time sequences (FT). The HT sequences, containing values of HTs n = 1,2,…,N, i.e., the differences between the time-stamp a key was released and the time-stamp the key was pressed, are pre-processed, in order to discard deliberate long-presses. Similarly, the FT sequences, containing values of FTs , n = 1,2,…,N-1, i.e., the differences between the time-stamp a key is pressed and the time-stamp the previous key was released, were also pre-processed to minimize the effect of typing dexterity and subjective factors. In particular, the filtering process included an upper bound of 3 s for sequence elements, a normalization procedure per typing session and a conditional filtering step to the produced normalized FT (NFT) sequences, as follows:
Each typing session is represented by a feature vector consisting of mean of mean μ of Hold time (HT), mean of standard deviation (σ) of HT as long as skewness S of normalized flight times, as derived by aggregating non-overlapping windows of 15 (s) of keystroke sequences:
The aforementioned typing features are drawn from our previous study (Iakovakis et al., 2018), in which statistical representations of sequences of HT, FT, and normalized pressure (NP) values were examined in-the-clinic data. However, in some cases, operational system implementations do not provide the value of NP, therefore the NP-related feature was omitted from in-the-wild data analysis, as no consistency in same smartphone devices was secured across the GData users.
2.2.2. Regression
For each of the UPDRS Part III items related to upper-extremity fine-motor symptoms, scores can be directly associated with the symptom severity, a regression model (transformation) was trained/tested, under a leave-one-subject-out (LOSO) scheme with inner cross validation for regressors' parameters optimization, to estimate the score of the corresponding item (target) on a typing session level. The total 275 typing sessions of DSet were assigned with the quantized target score of UPDRS Part III single items for each subject. By design, UPDRS scores (integer between 0 and 4) were quantized. Quantization levels span the severity of the underlying symptom with the lowest value (0) denoting a normal behavior, values between (0 and 2) exhibiting mild symptoms, and values from 3 up to 4 severe impairment or inability. Regression can granularize the target domain and provide an index of higher resolution, based on the continuous input predictors (i.e., the keystroke dynamics features of X). The UPDRS single items scores under investigation are B/H-K (B), tremor of right/left hand (Tr/Tl), rigidity (R) of right/left hand (Rr/Rl) and alternative finger tapping of right/left (AFTr/AFTl). In general, regression models involve: (a) the unknown parameters, denoted as β, (b) the independent variables, X and the dependent variable, Y. In our case, the regression analysis aims to investigate if the regressors fi can approximate the scale of Yi-th symptom's severity on each subject, i.e.,
An inner cross validation loop is used to optimize the β parameters of the different models under test. The models that were evaluated for the regression training were Support-Vector-Regression (Smola and Schölkopf, 2004), Lasso and Ridge Regression (Tibshirani, 1996), Random Forests (Liaw and Wiener, 2002), and Bagging of Linear Regressors (Breiman, 1996). We evaluated the accuracy of each regressor on a subject level to measure the ability to capture the scale of the severity, as long as the test error, by employing the Pearson's correlation coefficient and the mean absolute error (MAE). The regression analysis was applied in-the-clinic data and the learned functions fi that can explain a significant part of the underlying symptom and the mean absolute test error is below 0.5 (the half distance between the quantized scores), were further evaluated in-the-wild setting, as explained in the succeeding section.
2.3. Regression Models Exploitation In-the-Wild
Each subject Kd ∈ G, where G is denoted the set of GData subjects, has produced a sequence of sj typing sessions, and a corresponding feature vector Xsj where j ∈ {1, .., nd} is the total number of sessions for subject Kd.
2.3.1. Session Level Analysis
The feature extraction pipeline of typing session as captured in-the-wild (GData), depicted in Figure 2A, includes a post-hoc filtering component that discards recordings with less than eight keystrokes, to foster the statistical validity of the subsequent feature extraction. Therefore, each typing session is subjected to a windowing process that discards windows with less than four keystrokes to be consistent with the DSet processing pipeline. The values of Xsj are computed via aggregation across valid windows. Each session is represented by a feature vector for the subject Kd ∈ G. Three features are extracted from each valid typing session from GData and the learned mappings (fi) are applied to each session.
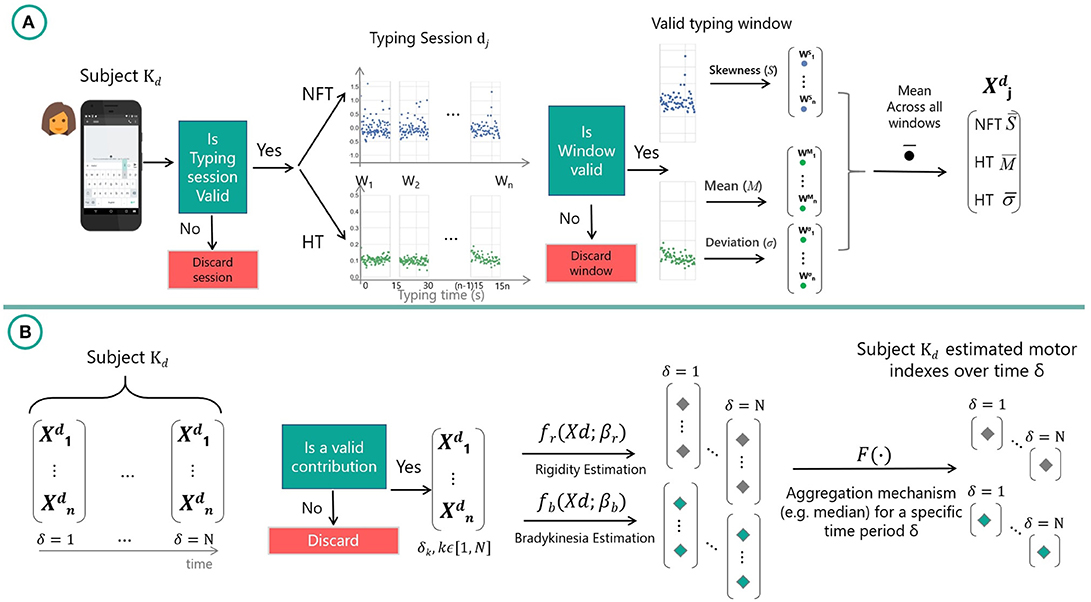
Figure 2. Procedural pipeline for processing GData (A) per typing session and (B) per subject. Typing sessions with at least eight keystrokes are considered valid for processing, whereas the rest are omitted. The keystroke dynamics consist of hold time (HT) and flight time (FT) sequences and are both split by non-overlapping 15 s windows (Wj). Only windows with at least four keystrokes within the 15 s interval are used further in order to extract features by computing the mean feature distribution for the valid corresponding windows. Each subject Kd has contributed typing features that are grouped by a time window δ, which is considered valid if contains more than 10 sessions. Each session is transformed with a learned mapping fk, k ∈ B/H − K, R which was previously trained on a the DSet, computes a single numerical score from each typing session. An aggregation mechanism F(·) is applied to each time window δ to characterize subject's time window contribution over time.
2.3.2. Subject Level Analysis
From a subject's perspective, an aggregation mechanism F(·) over the estimates fi(Xsj) that belongs to a period of time δ, is used to characterize the subject's distribution during this time period (see Figure 2B). Each time window δ can contain a different number of estimates associated with a time-stamp t. Subject's contribution with less than 10 valid feature vectors during the period δ is omitted from the analysis, in order for the δ to contain enough number of typing sessions. In the current context of analysis, we examine the median as the aggregation mechanism F(·) to get the most representative sample from the distribution. Moreover, two time windows δ ∈ {1, 52}weeks are used for validating the discrimination power of the estimators. In particular, the time frame of a week (δ = 1) is chosen to include all patterns and habits that can vary during a week (micro-level), whereas all 52 weeks (δ = 52) are set as the global time frame of the analysis for a macro-level perspective.
2.3.3. PD Classification Performance Evaluation
The motor estimates resulted from the aforementioned subject-level analysis, are evaluated in the binary classification performance (PD vs. control), by estimating the area under curve (AUC) of the ROC curve. ROC based performance of the indexes discrimination power is computed with Confidence Interval (CI) with 1,000 bootstraps. Additionally, the sensitivity/specificity metrics, corresponding to the optimal ROC-based cut-off point (decision threshold), are estimated by maximizing the Youden Index (Fluss et al., 2005), under the assumption that costs for false positives and false negatives are equal.
2.3.4. Statistical Analysis
Logistic regression tests using the subject status (PD or control) as dependent variable and symptoms predictions, sex, age, years of education, and usability of smartphones as independent variables, are performed for statistical significance evaluation of the variables discrimination power. The two groups (PD and controls) of the GData setting are tested in terms of demographics using a two-sided Mann–Whitney U-test. Moreover, a two-sided Kolmogorov–Smirnov test for the null hypothesis that two samples are drawn from the same continuous distribution is used to examine the statistically significant difference of the raw keystroke dynamics between PD and controls. Statistically significant difference is set at the level of p < 0.05.
3. Results
3.1. Keystroke Dynamics Distributions
In Figure 3, the raw keystroke dynamics variables under investigation are compared with respect to their distribution as drawn from the DSet and the GData settings. From this figure, a clear similarity in the trend between the two settings can be observed. Moreover, both HT and FT values, are statistically different (p < 0.001) for group-wise comparisons of PD and controls in the two settings. This justifies the initial assumption of the robustness of the proposed approach to transfer knowledge from analysis in-the-clinic to the one in-the-wild, as described below.
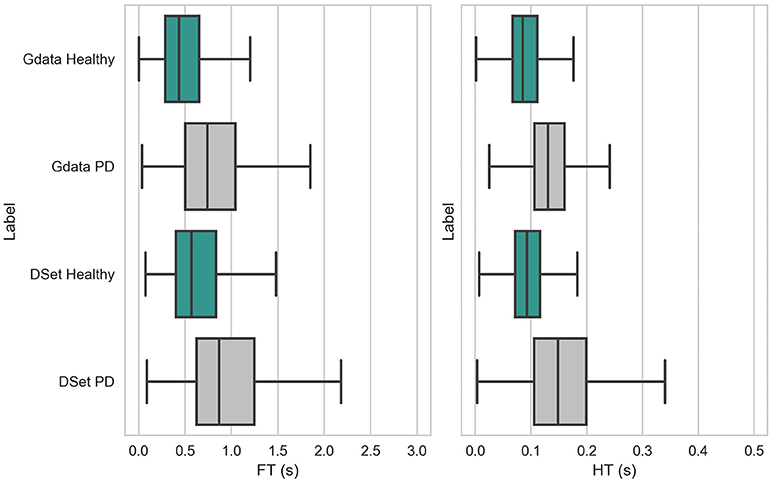
Figure 3. Box plots of keystroke dynamic variables (FT and HT) distributions when comparing the two data capturing settings (DSet and GData).
3.2. In-the-Clinic Setting
From the LOSO analysis results it was found that the best performing regression models achieved 0.83 (0.39), 0.69 (0.41), and 0.68 (0.55) Pearson's correlation coefficient (MAE) for predicting dominant-hand R, B/H-K and right-handed finger tapping, respectively. As MAE was greater than 0.5 for the case of right-handed finger tapping, overlapping the quantized levels of UPDRS Part III scores, dominant hand R and B/H-K were only involved in the consequent analysis. The predictions of the latter two symptoms and the UPDRS Part III single item scores are visualized in Figure 4. In particular, the dominant-hand R and B/H-K median predicted scores are depicted along with the medical scores, using error-bars of 0.5 height. The produced indexes achieve 78 and 70% in predicting the quantized UPDRS medical scores on R and B/H-K scores during the LOSO experiment, respectively. The rest UPDRS single items (Tl/r, Rl, AFTl) related to motor activity can not be predicted from the keystroke features. This is due to the low Pearson's correlation coefficient values (< 0.35), which are probably caused by the use of dominant hand during typing (all of them were right-handed) and the possible subtle relation of finger movement coordination with hand tremor (see also section 4).
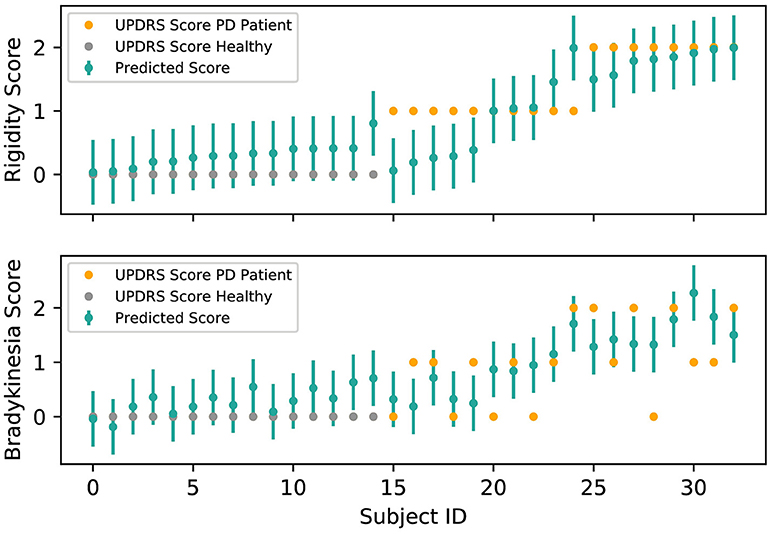
Figure 4. Regression estimates (green dots) of LOSO experiment using keystroke dynamic features and UPDRS Part III single items scores. The median of the predicted values distribution across the typing session predictions are also plotted per subject. Moreover, error bars of height ±0.5 are superimposed to show if the symptom estimation lies within the span of the physician's score.
3.3. In-the-Wild Setting
As it was mentioned in the section 2.1, GData subjects have matched demographic characteristics of the ones participated in DSet, in order to avoid any inhomogeneity across the two data settings, after appropriate subject filtering process. Moreover, results from the statistical analysis tests, tabulated in Table 1, show that data from a PD patient' and a healthy controls' group, are matched in terms of demographics. Furthermore, ROC curves of the the two estimated indexes estimated for each subject are depicted in Figure 5, considering as time-frame δ = 52 weeks. In particular, estimation of R achieves 0.84 (0.75/0.93 is the 95%CI) AUC with 0.77/0.8 sensitivity/specificity and 0.79 accuracy, where the estimation of B/H-K achieves 0.80 (0.7/0.92 is the 95%CI) AUC with 0.92/0.63 sensitivity/specificity and 0.70 accuracy in the GData cohort (more diagnostic properties are tabulated in Table 2). In addition, when assessing diagnostic properties of subjects' contribution in time frame (δ = 1week), the indexes achieve lower discrimination performance with 0.80 AUC for R with 0.82/0.65 sensitivity/specificity and 0.78 AUC with 0.86/0.60 sensitivity/specificity for B/H-K. Finally, statistical significance discriminative performance of the estimated indexes was found (p < 0.001) with logistic regression models including gender, age, years of education, and mobile usability time as co-variates, achieving p < 0.001, where the other dependent variables (see section 2.3.4) did not show any statistical significance.
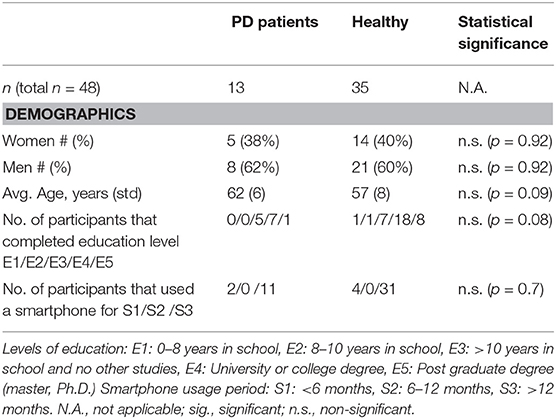
Table 1. Summary of GData study cohort (48 subjects) demographic and clinical characteristics with respect to each group (PD patients and Healthy).
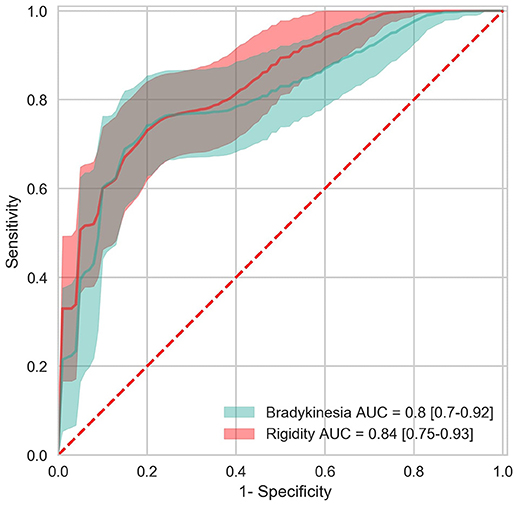
Figure 5. Classification performance of the median predicted estimates of R and B/H-K during the time frame of δ = 52. ROC curves demonstrating the classification performance of the proposed models for estimating individual motor symptoms on the GData (13 PD patients/35 controls). Solid lines represent the mean ROC curve, while shadowed areas delimit the 95% confidence intervals, computed over 1,000 bootstraps. From the ROC curves the corresponding AUC values are for the R 0.84 (0.75/0.93 is the 95%CI) and for the B/H-K is 0.80 (0.7/0.92 is the 95%CI), respectively.
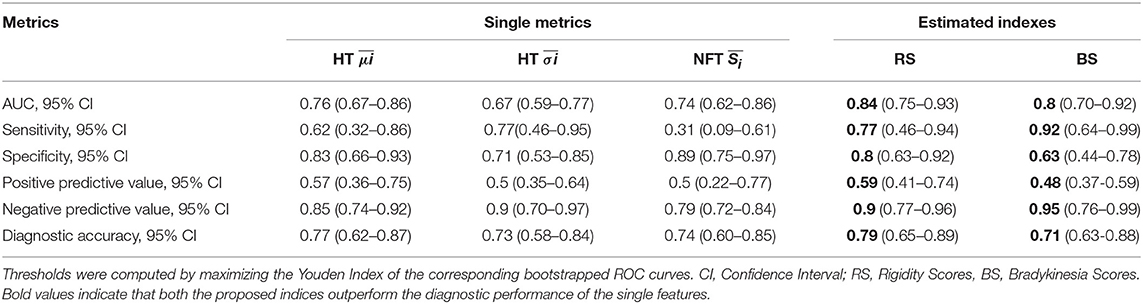
Table 2. Diagnostic properties of the typing features and the symptoms estimated scores as calculated in the GData cohort.
4. Discussion
Digital Health is an emerging field that could enhance disease detection and management via the realization of objective and accessible tools that could quantify behavioral characteristics. Unobtrusive capturing of data via the natural interaction with digital devices is a key factor of digital tools' design to meet the need of long-adherence. User's habitual patterns are influenced by motor symptoms, even in the early stage of PD where the motor manifestation is subtle. The underlying behavior can be detected via algorithmic transformation of high frequency sampled data streams to useful medical indicators, that can be interpreted from physicians and assist the longitudinal process of passive monitoring, diagnosis, and treatment. The current study design aims to amalgamate the aforementioned requirements, while the results contribute to the interpretation and the real life transferability of the developed methods, by exploiting keystroke dynamics during routine typing on mobile touchscreen, a fine-motor activity which is of high frequency due to the booming of mobile technology (Sarwar and Soomro, 2013). A machine learning-based estimation of dominant-hand rigidity and bradykinesia/hypokinesia severity is employed using captured keystroke typing features.
The individual items of R and B/H-K, related to fine-motor skills decline in PD, are used as regression targets to provide a granular data driven estimation about the specific fine motor symptom, which is more interpretable than a high-level label for the subject (e.g., a binary label or total UPDRS Part III single item score). The regression results show that dominant-hand R and B/H-K predictions achieve a low test error and can sufficiently capture the severity of symptom. Hand tremor and non-dominant hand UPDRS Part III single item scores, however, could not be predicted during the proposed analysis, which resulted in low correlation coefficients (<0.35). The latter can be explained by the nature of the finger typing information, which can be disentangled to the finger reflexes of pressing the keys (can expressed via HT) and the finger movements across the digital screen (FT); actions that can be influenced by R and B/H-K but not directly from tremor. In addition, PD patients were at the early stage of the disease and their UPDRS Part III single item scores were in the lower scales 0 − 2 for both symptoms. The latter reinforces the added value of the results toward capturing PD specific fine-motor impairment at the early stage of the disease, where the symptoms are mild. Moreover, the expansion of the developed in-the-clinic method to the uncontrolled setting in-the-wild constitutes a step toward remote passive monitoring of users' fine-motor symptoms. The unobtrusive capturing of GData containing more than 40,000 typing sessions (mean/std: 204/406 per subject) from 210 total users (mean/std weeks of each subject's data contribution: 7/14), highlights the positive effect of the unobtrusive data collection in the long-term adherence. This overcomes the dropouts seen in recent smartphone-based studies for PD, which require active user's involvement (Bot et al., 2016; Zhan et al., 2018).
The diagnostic properties of the produced indexes achieved up to 0.8/0.77 sensitivity/specificity on classifying PD and healthy subjects in the wild setting, when aggregating for the whole time period of data contribution, which matches the satisfactory performance seen in-the-clinic setting analyses, i.e., 0.81/0.82 in Iakovakis et al. (2018) and 0.81/0.81 in Arroyo-Gallego et al. (2017). The results are also compliant with the findings of Arroyo-Gallego et al. (2018), who suggests that keystroke dynamics on physical keyboard can be used for remote PD screening with sensitivity/specificity of 0.73/0.69. Estimated indexes were also aggregated across the time frames of hour, weekday, and week, as to compute the variance and consistency of the indices over time. Figure 6 exemplifies the longitudinal estimates of the median of indices coming from a PD patient and a healthy GData user for hour/weekday and for six continuous weeks of data contribution. Estimated indexes of both subjects have a constant behavior over the time frames of week and weekday, whereas intra day data are more variant.
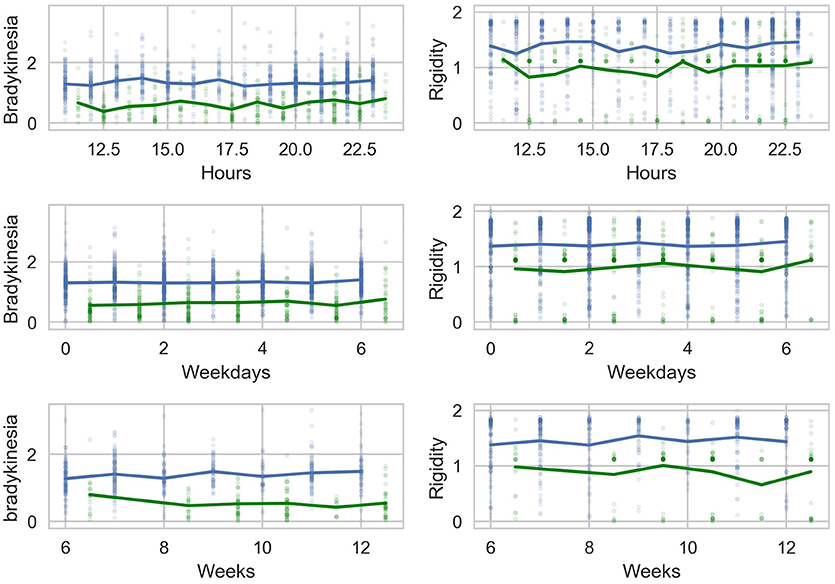
Figure 6. Responses of the estimated indices across time using different time resolution (hours, weekdays, and weeks) of two cases, i.e., a PD (blue graph) and a control (green graph). Blue/green line represents the median of the estimated indices, whereas blue/green dots are estimations for a single typing session regarding the PD patient/control contribution for each period of time.
Additionally, in Figure 7 group-wise comparisons of the time response of the indexes are depicted, with an obvious discrimination of the two groups across different time frame resolutions. The corresponding standard deviation of PD/controls; 0.37/0.34 for hours 0.27/0.3 for weekday and 0.24/0.3 for week. The latter denote more variant behavior during the day rather than the weekday and week for both groups. In fact, intra-day variations of controls' fine-motor skills have been previously reported (Van Vugt et al., 2013) to be affected by the circadian rhythm, which can also be considered as a factor that might influence the findings here, due to its relation with fine motor movements during smartphone interaction. Also, dopamine plays a substantial role on circadian regulation and timing behavior (Agostino et al., 2011), whereas recent works (Videnovic and Golombek, 2017) present increasing evidence to disruption of circadian function in PD, where a dopamine-based therapy may increase the circadian oscillations. Healthy population tends to have the peak of their motor coordination and fast reflexes between the time zone of 14:00 and 16:00 (Bass, 2012; Smolensky and Lamberg, 2015), which may explain the groups' median indexes divergence during that specific period of time, as it can be seen in Figure 7. Though circadian rhythm in PD is a novel area of research and recent studies state it as a new therapeutic target (Videnovic and Willis, 2016), applications of digital health with interpretability can enhance the understanding of the underlying patterns of the human behavior, setting the direction for the future work.
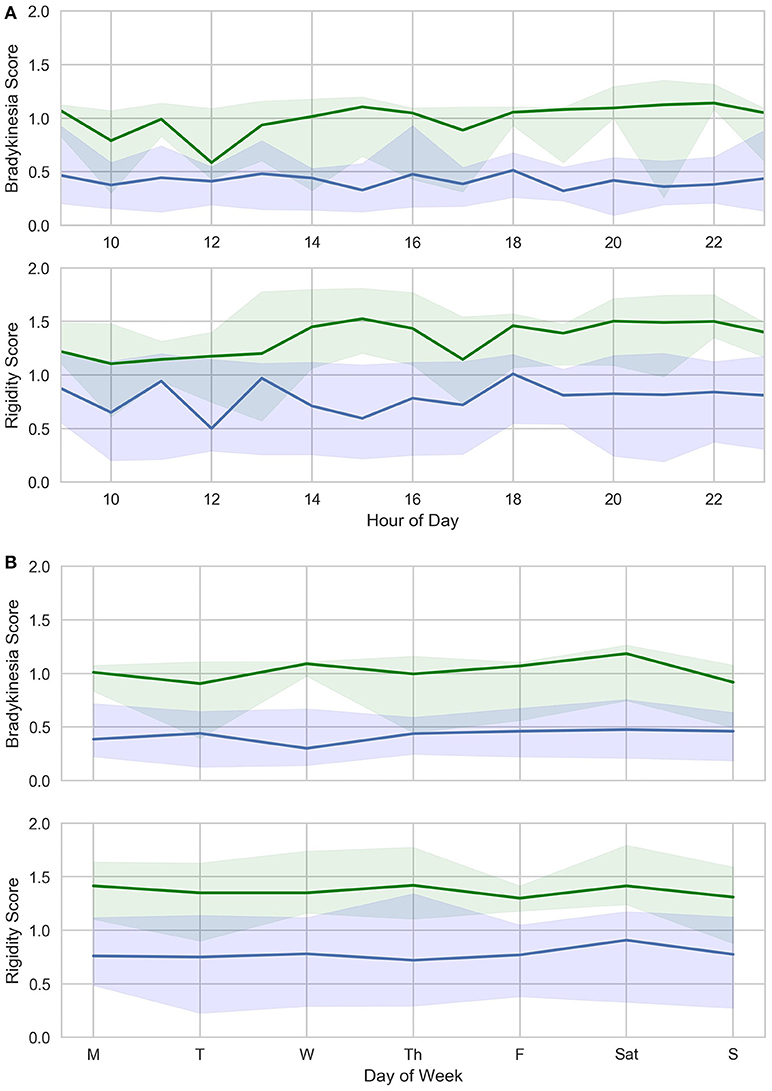
Figure 7. Response of the estimated indices across time using δ of (A) daily hours and (B) weekdays, for all subjects grouped according to their health status, i.e., PD (green graph) and controls (blue graph). The solid lines represent the group medians, whereas the shadowed areas denote the upper(75th)/lower(25th) quartiles range, respectively.
From a wider perspective, smartphone interaction has been a promising research direction (Pan et al., 2015) for detecting individual PD-related symptoms, such as gait difficulties and hand tremor through accelerometer recordings during the execution of specific scenarios using a smartphone. Furthermore, fusion of data associated with different PD symptoms captured via smartphone-based tests (Arora et al., 2015) and machine learning have been explored toward PD screening, resulting in classification performance of 0.96/0.97 sensitivity/specificity. Although the latter study provides proof-of-evidence for the feasibility of assessing a wide range of motor symptoms through smartphone interaction, data were recorded during guided scenarios, constraining the scalability of data collection due to the requirement of users' active participation. The novelty of the current study is that it sets up an interpretable framework for unobtrusive assessment of individual PD symptoms, which can be further used in combination with other data sources, e.g., background and privacy-aware capturing of accelerometer data for tremor assessment during typing or microphone data (voice) for dysarthrophonia assessment during phone calls, to broaden symptom assessment and pave the way for a holistic objective PD detection tool. Following the same approach proposed in this work, the additional data sources can potentially yield explainable symptom severity indicators, that if combined, can form a fused behavioral vector based on which the final decision of the subject's status against PD can be reached, in a similar way that diagnosis takes place in clinical practice. The fusion approach can include the feeding of the time-aggregated (e.g., every week) behavioral vectors to a decision system, similar to that of (Arora et al., 2015), allowing for high frequency monitoring of the time evolution of both the overall decision, as well as the individual PD symptoms indicators. This is the direction that the i-PROGNOSIS European research project (http://www.i-prognosis.eu) follows toward early PD screening in daily living, in the context of which this study has been carried out.
One possible limitation of our study is that patients under dopaminergic therapy that were included in the DSet data setting refrained from taking their medication at least 8 h before their participation in the experiment, instead of the 12 h that usually secures the “practically off” condition. The latter, combined with potential effects of long-duration response to Levodopa, may have improved the psycho-motor state of these patients and consequently, their typing cadence, leading to a reduced discrimination performance across classification methods tested. Nevertheless, the promising results of the symptoms' estimation in the DSet setting show limited “echoing” effects of dopaminergic therapy on certain study participants' fine motor skills. A second possible limitation of this study is the validity of the users' self-reported demographics in the GData data setting. This perhaps will induce noise in the data evaluation. However, using the time-frame of one week within the range of 52 weeks creates a longitudinal user profile at the micro and macro level of analysis, which reveals a data driven behavior that can be compared across different users. In this way, noticeable deviations could lead to group reorganization; yet, this was not the case here.
Considering the future adoption and extension of the current methods, effectiveness of the approach will not be affected by subjects' reorganization because symptoms severity estimators were trained/evaluated based on data captured from a medically valid cohort. However, reorganization could happen to the in-the-wild cohort, which was used for the reporting of diagnostic properties of the indices. The group reorganization will not severely affect the findings, since the diagnostic properties of the indices are reported with a confidence interval, the true value of the diagnostic performance will probably lie in the span of the reported confidence intervals in case of more participants join the study. Moreover, demographic characteristics did not show any statistical significance from the logistic regression tests. Scalability is the main cause the study is designed in this manner, and re-analyzing the data arising from a larger pool of subjects will yield to even more robust values for the diagnostic performance, which will further increase the medical interpretation of the proposed approach. Toward this, research plans include sampling of subjects for medical evaluation in order to fine-tune the time-aggregation function used in this study toward better modeling of time-related variations of the proposed indicators.
Regarding the transparency of the developed machine learning methods, the current approach aims to quantify the fine-motor skills of the user and transform daily behavior to indices that can be linked to scores of the physician's assessments. The produced indices can be also reverse-explained by the physician due to the initial compact feature representation and plausible correlations with standardized UPDRS items. The use of specific features in the pipeline, which are naturally linked to fine-motor impairment, further enhances the interpretability of the resulting estimations. Specifically, keystroke dynamics-related features inputted to the regression mechanisms are naturally affected by rigidity (muscle stiffness) and bradykinesia (slowness of movement), causing longer (mean of HT), more variant (standard deviation of HT) pressings of virtual keyboard keys and slower finger coordination across the screen (skewness of FT), during PD patients' typing, when compared to controls. The latter can be interpreted by the physician as an objective projection of the scale of symptoms' severity to the specific body part used to perform the task. In a nutshell, the developed approach aims to support physicians, not replace them, and accelerate the PD diagnosis, by providing objective tools for remote quantification of the symptoms footprint on the patient.
Conclusions
In this study, evidence of real-life usage of unobtrusive detection of fine-motor symptoms from undiagnosed population using prior information on symptoms severity estimation in-the-clinic setting was provided. The presented results validate the initial hypothesis that individual symptom severity can be approximated using keystroke dynamics information and based on this, PD can be detected via keystroke pattern analysis when data are captured in-the-wild. Separation between PD and healthy controls, purely based on smartphone keystroke dynamics is possible and these results could be seen constantly over a longer time frame. Furthermore, the severity expressed by the smartphone touchscreen typing corresponds well with the severity evaluated by the neurologists on which the training algorithms are based. Potential future extension of the method can include the use of deep learning (LeCun et al., 2015), accompanied with explainable methods (Gunning, 2017), which may reveal better representations of the raw keystroke dynamics and capture more efficiently the latent factors of the symptom's digital footprint, considering also other factors in the analysis, such as the circadian rhythm. Finally, embedding such analyses in the operational systems of smartphones could assist the mobile health booming, considering though, all ethical guidelines and data regulations regarding privacy and security, such as the General Data Protection Regulation (GDPR).
Data Availability Statement
All data generated and analyzed during the current study are available from the corresponding author on a reasonable request.
Author Contributions
SH, VC, SB, and ZK conceived the study protocol. DI, SH, and VC developed the keyboard and the algorithms, conducted the typing experiment. SB and ZK conducted the clinical evaluations. DI, SH, LH, and VC analyzed the data. SH, SB, ZK, LK, HR, SD, JD, DT, and KC developed the GData demographic information, participant information and e-consent process and handled the data governance procedures as well as the corresponding GData ethic approvals. All authors discussed the results and contributed to the manuscript.
Funding
The research leading to these results has received funding from the European Union's Horizon 2020 research and innovation programme under grant agreement No 690494—i-PROGNOSIS: Intelligent Parkinson early detection guiding novel supportive interventions.
Conflict of Interest Statement
The authors declare that the research was conducted in the absence of any commercial or financial relationships that could be construed as a potential conflict of interest.
Acknowledgments
The authors wish to acknowledge the donations of time and data from all of the i-PROGNOSIS study participants, engineering contributions from Ntakakis George, Fotis Karayiannis, and Kyritsis Konstantinos, for support of the iPrognosis app and data-collection system, proof reading by Anastasia Ntracha.
References
Abdulhay, E., Arunkumar, N., Narasimhan, K., Vellaiappan, E., and Venkatraman, V. (2018). Gait and tremor investigation using machine learning techniques for the diagnosis of parkinson disease. Future Gener. Comput. Syst. 83, 366–373. doi: 10.1016/j.future.2018.02.009
Agostino, P. V., Golombek, D. A., and Meck, W. H. (2011). Unwinding the molecular basis of interval and circadian timing. Front. Integr. Neurosci. 5:64. doi: 10.3389/fnint.2011.00064
Arora, S., Venkataraman, V., Zhan, A., Donohue, S., Biglan, K. M., Dorsey, E. R., et al. (2015). Detecting and monitoring the symptoms of parkinson's disease using smartphones: a pilot study. Parkinsonism Relat. Disord. 21, 650–653. doi: 10.1016/j.parkreldis.2015.02.026
Arroyo-Gallego, T., Ledesma-Carbayo, M. J., Butterworth, I., Matarazzo, M., Montero-Escribano, P., Puertas-Martín, V., et al. (2018). Detecting motor impairment in early parkinson's disease via natural typing interaction with keyboards: validation of the neuroqwerty approach in an uncontrolled at-home setting. J. Med. Intern. Res. 20:e89. doi: 10.2196/jmir.9462
Arroyo-Gallego, T., Ledesma-Carbayo, M. J., Sanchez-Ferro, A., Butterworth, I., Mendoza, C. S., Matarazzo, M., et al. (2017). Detection of motor impairment in parkinson's disease via mobile touchscreen typing. IEEE Trans. Biomed. Eng. 64, 1994–2002. doi: 10.1109/TBME.2017.2664802
Bot, B. M., Suver, C., Neto, E. C., Kellen, M., Klein, A., Bare, C., et al. (2016). The mpower study, parkinson disease mobile data collected using researchkit. Sci. Data 3:160011. doi: 10.1038/sdata.2016.11
Chaudhuri, K. R., Healy, D. G., and Schapira, A. H. (2006). Non-motor symptoms of parkinson's disease: diagnosis and management. Lancet Neurol. 5, 235–245. doi: 10.1016/S1474-4422(06)70373-8
Fahn, S. E., R, and of the UPDRS Development Committee, M. (1987). The unified parkinson's disease rating scale. Recent Dev. Parkinson's Dis. 2, 153–163, 293–304.
Fluss, R., Faraggi, D., and Reiser, B. (2005). Estimation of the youden index and its associated cutoff point. Biometr. J. 47, 458–472. doi: 10.1002/bimj.200410135
Giancardo, L., Sánchez-Ferro, A., Arroyo-Gallego, T., Butterworth, I., Mendoza, C. S., Montero, P., et al. (2016). Computer keyboard interaction as an indicator of early parkinson's disease. Sci. Rep. 6:34468. doi: 10.1038/srep34468
Goetz, C. G., Tilley, B. C., Shaftman, S. R., Stebbins, G. T., Fahn, S., Martinez-Martin, P., et al. (2008). Movement disorder society-sponsored revision of the unified parkinson's disease rating scale (mds-updrs): scale presentation and clinimetric testing results. Mov. Disord. 23, 2129–2170. doi: 10.1002/mds.22340
Gunning, D. (2017). Explainable Artificial Intelligence (xai). Defense Advanced Research Projects Agency (DARPA), nd Web.
Hawkes, C. H., Del Tredici, K., and Braak, H. (2010). A timeline for Parkinson's disease. Parkinsonism Relat. Disord. 16, 79–84. doi: 10.1016/j.parkreldis.2009.08.007
Iakovakis, D., Hadjidimitriou, S., Charisis, V., Bostantzopoulou, S., Katsarou, Z., and Hadjileontiadis, L. J. (2018). Touchscreen typing-pattern analysis for detecting fine motor skills decline in early-stage parkinson's disease. Sci. Rep. 8:7663. doi: 10.1038/s41598-018-25999-0
i-Prognosis (2017). i-Prognosis. Available online at: https://play.google.com/store/apps/details?id=com.iprognosis.gdatasuite
LeCun, Y., Bengio, Y., and Hinton, G. (2015). Deep learning. Nature 521:436. doi: 10.1038/nature14539
Liaw, A., and Wiener, M. E. A. (2002). Classification and regression by randomforest. R News 2, 18–22. Available online at: http://cogns.northwestern.edu/cbmg/LiawAndWiener2002.pdf
Mellone, S., Tacconi, C., Schwickert, L., Klenk, J., Becker, C., and Chiari, L. (2012). Smartphone-based solutions for fall detection and prevention: the farseeing approach. Z. Gerontol. Geriatr. 45, 722–727. doi: 10.1007/s00391-012-0404-5
Monahan, T., and Fisher, J. A. (2010). Benefits of observer effects: lessons from the field. Qualit. Res. 10, 357–376. doi: 10.1177/1468794110362874
Orozco-Arroyave, J. R., Hönig, F., Arias-Londoño, J. D., Vargas-Bonilla, J. F., Daqrouq, K., Skodda, S., et al. (2016). Automatic detection of parkinson's disease in running speech spoken in three different languages. J. Acoust. Soc. Am. 139, 481–500. doi: 10.1121/1.4939739
Pan, D., Dhall, R., Lieberman, A., and Petitti, D. B. (2015). A mobile cloud-based Parkinson's disease assessment system for home-based monitoring. JMIR mHealth uHealth 3:e29. doi: 10.2196/mhealth.3956
Sarwar, M., and Soomro, T. R. (2013). Impact of smartphone's on society. Eur. J. Sci. Res. 98, 216–226. Available online at: https://pdfs.semanticscholar.org/2c28/0b6a690442a97a571e09b2404e2d21720db4.pdf
Schrag, A., Horsfall, L., Walters, K., Noyce, A., and Petersen, I. (2015). Prediagnostic presentations of Parkinson's disease in primary care: a case-control study. Lancet Neurol. 14, 57–64. doi: 10.1016/S1474-4422(14)70287-X
Shulman, J. M., De Jager, P. L., and Feany, M. B. (2011). Parkinson's disease: genetics and pathogenesis. Annu. Rev. Pathol. 6, 193–222. doi: 10.1146/annurev-pathol-011110-130242
Smola, A. J., and Schölkopf, B. (2004). A tutorial on support vector regression. Stat. Comput. 14, 199–222. doi: 10.1023/B:STCO.0000035301.49549.88
Smolensky, M., and Lamberg, L. (2015). The Body Clock Guide to Better Health: How to Use Your Body's Natural Clock to Fight Illness and Achieve Maximum Health. New York, NY: Henry Holt and Company.
Tibshirani, R. (1996). Regression shrinkage and selection via the lasso. J. R. Stat. Soc. Ser. B 267–288.
Van Vugt, F. T., Treutler, K., Altenmüller, E., and Jabusch, H.-C. (2013). The influence of chronotype on making music: circadian fluctuations in pianists' fine motor skills. Front. Hum. Neurosci. 7:347. doi: 10.3389/fnhum.2013.00347
Videnovic, A., and Golombek, D. (2017). Circadian dysregulation in Parkinson's disease. Neurobiol. Sleep Circ. Rhythms 2, 53–58. doi: 10.1016/j.nbscr.2016.11.001
Videnovic, A., and Willis, G. L. (2016). Circadian system:a novel diagnostic and therapeutic target in Parkinson's disease? Mov. Disord. 31, 260–269. doi: 10.1002/mds.26509
Keywords: fine motor skills, Parkinson's disease, keystroke dynamics, unobtrusive monitoring, data in-the-wild, machine learning, digital medicine
Citation: Iakovakis D, Hadjidimitriou S, Charisis V, Bostantjopoulou S, Katsarou Z, Klingelhoefer L, Reichmann H, Dias SB, Diniz JA, Trivedi D, Chaudhuri KR and Hadjileontiadis LJ (2018) Motor Impairment Estimates via Touchscreen Typing Dynamics Toward Parkinson's Disease Detection From Data Harvested In-the-Wild. Front. ICT 5:28. doi: 10.3389/fict.2018.00028
Received: 30 July 2018; Accepted: 27 September 2018;
Published: 08 November 2018.
Edited by:
Eugeniu Costetchi, Office des Publications de l'Union Européenne, LuxembourgReviewed by:
Haridimos Kondylakis, Foundation for Research and Technology (FORTH), GreeceVladimir Tihomir Trajkovik, Saints Cyril and Methodius University of Skopje, Macedonia
Copyright © 2018 Iakovakis, Hadjidimitriou, Charisis, Bostantjopoulou, Katsarou, Klingelhoefer, Reichmann, Dias, Diniz, Trivedi, Chaudhuri and Hadjileontiadis. This is an open-access article distributed under the terms of the Creative Commons Attribution License (CC BY). The use, distribution or reproduction in other forums is permitted, provided the original author(s) and the copyright owner(s) are credited and that the original publication in this journal is cited, in accordance with accepted academic practice. No use, distribution or reproduction is permitted which does not comply with these terms.
*Correspondence: Leontios J. Hadjileontiadis, bGVvbnRpb3NAYXV0aC5ncg==