- 1Department of Respiratory and Critical Care Medicine, West China Hospital, Sichuan University, Chengdu, China
- 2Centre for Heart Lung Innovation, University of British Columbia and St. Paul’s Hospital, Vancouver, BC, Canada
Background: The toll-like receptor 2 (TLR2)-mediated immune response is critical for host defense against Mycobacterium tuberculosis. There is evidence that TLR10, a TLR2 signaling modulator, may be involved in progression of tuberculosis (TB).
Methods: Using a self-validating case–control design, we tested for an association between seven TLR10 polymorphisms and susceptibility to TB in three independent series with two distinct populations. Single-nucleotide polymorphism (SNP) genotypes were determined by the SNPscanTM method. Three genetic models (additive, dominant, and recessive) as well as multiple-SNP score analyses were used to evaluate the risk of TB associated with the TLR10 SNPs.
Results: By comparing TB patients with healthy controls, we observed two SNPs (rs11466617 and rs4129009) that were associated with decreased risk of TB in the Tibetan population, but did not in the Chinese Han population. Further analysis demonstrated that the rs11466617 Chengdu cohort genotype served as a protective factor against the progression of latent TB infection (LTBI) to active TB under the recessive model. None of the SNPs were significantly different in comparisons of TB-uninfected people with LTBI individuals. Additionally, when the underlying four TB-associated loci were considered together in a multiple-SNP score analysis, we observed an allele dose-dependent decrease in TB risk in Tibetans.
Conclusion: Variants of TLR10 may show an ethnic specificity on susceptibility to TB in Tibetan individuals. rs11466617 affected the susceptibility to progress from LTBI to active TB disease, but was not associated with the establishment of LTBI after M. tuberculosis exposure. More studies are needed to verify this genetic epidemiological result and unravel the role of TLR10 SNPs in the pathogenesis of TB.
Introduction
Tuberculosis (TB), a chronic pulmonary disease caused by Mycobacterium tuberculosis (Mtb) infection, remains a leading global health threat with an estimated 10.4 million incident cases and 1.8 million deaths worldwide in 2015 (1). It is estimated that one third of the world’s population is infected with Mtb, yet only approximately 5–15% of infected individuals develop active TB and the rest remain asymptomatic, which is termed latent TB infection (LTBI) (2). A complex interaction occurs between Mtb and the host immune system, which begins with the inhalation of Mtb-containing aerosol and ultimately leads to the replication of Mtb. This pathway results in tissue inflammation and damage that trigger clinical symptoms (3). Patients who are immunocompromised due to HIV coinfection or diabetes are predisposed to active TB (4), emphasizing the important role of host immunity in the progression of this disease. In addition to environmental risk factors, genetic polymorphisms can influence the expression and/or activity of proteins involved in host immunity, which may result in susceptibility to TB and, to some extent, explain the TB patients without identifiable risk factors (5, 6). Numerous candidate genes have been associated with risk of TB, including members of the toll-like receptor (TLR) family (TLR1, TLR2, TLR4, TLR6, TLR9, TLR10, TIRAP) (7–9), which are widely expressed on various immune cells including monocytes, macrophages, dendritic cells, and lymphocytes and may modulate activation via TLR ligands (10, 11).
TLR10 is a TLR family member first discovered by Chuang et al. (12), which has 50 and 49% amino-acid sequence homology with TLR1 and TLR6, respectively. TLR10 remains an orphan receptor without a confirmed ligand, signaling pathway, or biological function. However, it is widely expressed in lymphoid tissues including the spleen, lymph node, thymus, and tonsils as well as a number of leukocyte subtypes, especially B cells (13). This expression pattern suggests that TLR10 has an immune function similar to other TLRs. Recently, a number of studies indicated that TLR10 serves as a modulatory pattern-recognition receptor with mainly inhibitory properties on TLR2-derived immune responses (14, 15), which are involved in the progression of TB (16, 17).
A previous study reported an association between TLR10 single-nucleotide polymorphisms (SNPs) and risk of TB in humans (18). However, SNPs located in non-coding regions, which may affect expression of the TLR10 gene and thus influence disease susceptibility (19), were not investigated. It is also unclear whether these SNPs could mediate progression of LTBI to active TB. Therefore, to give a comprehensive view of the association between TLR10 SNPs and risk of TB, we performed a self-validating association study based on three independent case–control series.
Materials and Methods
Study Design and Subjects
All protocols for this study were reviewed and approved by the Institutional Review Board of the West China Hospital of Sichuan University in 2013 (Protocol no. 131). We evaluated associations between TLR10 SNPs and TB by analyzing the genotype distributions of cases and controls in three independent series: Aba Tibetan Autonomous Prefecture cohort (ATAPC), Chengdu cohort (CC), and Chengdu LTBI cohort (CLC). Subjects in ATAPC and CC consist of two subgroups: TB patient group and healthy control (HC) group. A total of 1,216 self-identified Tibetan individuals (613 TB patients and 603 controls) in ATAPC were enrolled from the People’s Hospital of Aba Tibetan Autonomous Prefecture, located in northern Sichuan province, China, which is a Tibetan-predominant region. In addition, 1,185 Chinese Han subjects (580 TB patients and 605 controls) in CC were selected from our previous TB genetic association study conducted at the West China Hospital in Chengdu, Sichuan, China (20). The diagnosis of TB was based on the findings from Mtb, clinical symptoms, radiological evidence, and the response to anti-TB treatment, as described previously (20). The control groups in ATAPC and CC consisted of unrelated healthy individuals having routine-scheduled physical exams in the same region with no history of TB, who were gender and age matched to the cases.
We also performed a case–control study in CLC, which included three subgroups: 209 TB patients, 200 LTBI individuals, and 204 HCs, to investigate whether the TLR10 SNPs were associated with Mtb infection and/or the progression of LTBI to active TB. As with CC, the subjects in CLC were recruited from the West China Hospital in Chengdu. TB patients in this study were bacteriology-confirmed cases having positive of culture and/or acid-fast bacilli (AFB) and/or polymerase chain reaction of Mtb DNA in sputum or body sites, with a typical clinical complaint, e.g., fever, cough, sweating, hemoptysis, or weight loss. Both LTBI and HC subjects were unrelated and asymptomatic contacts of TB patients with normal radiological manifestations and negative sputum smear and culture. A contact was defined as someone who shared the same airspace with a sputum-positive TB patient for at least 15 h per week for >1 week or a total of 180 h during the date of onset of cough until 2 weeks after the initiation of appropriate anti-TB treatment (21). LTBI was defined as a positive result for the QuantiFERON-TB Gold in-tube (QFT-GIT) assay and HC was defined as a negative result for the same assay. Individuals demonstrating the presence of coinfection with HIV, cancer, diabetes, immune system diseases, chronic respiratory diseases, or on steroids for inflammatory conditions were excluded from this study. Written informed consent was obtained from all the study participants. The inclusion and exclusion criteria of each study are detailed in Table S1 in Supplementary Material.
Single-Nucleotide Polymorphism Selection
Single-nucleotide polymorphisms located within the region 3,000 base pairs upstream and 300 base pairs downstream of TLR10 in Chinese Han in Beijing were obtained from the International HapMap Project (accessed on May 1, 2016).1 We used Haploview 4.2 (Broad Institute of MIT and Harvard, Boston, MA, USA) to capture tagSNPs using common genetic variation [minor allele frequency (MAF) ≥5%] with strong coverage [linkage disequilibrium (LD) r2 ≥ 0.8]. Seven tagSNPs (rs10004195, rs11096956, rs11466617, rs11466655, rs4129009, rs11096957, and rs11466653) were retained for analysis in this study.
DNA Isolation and Genotyping
Genomic DNA was extracted from peripheral venous blood using the AxyPrep DNA Blood kit (Axygen Scientific Inc., Union City, CA, USA). The tagSNP genotyping was implemented using a custom-by-design 2 × 48-Plex SNPscan™ Kit (cat. #G0104, Genesky Biotechnologies Inc., Shanghai, China), which was based on double-ligation and multiplex fluorescence polymerase chain reaction (22). The details of the genotyping assays are described in the Supplementary Materials. Quality control measures included blinded repeat genotyping of 5% of the samples for each SNP.
Interferon-γ Release Assay
A commercial interferon-γ (IFN-γ) release assay QFT-GIT (Cellestis, Carnegie, Australia) was performed according to the manufacturer’s instructions by experienced medical technologists. Test results were interpreted according to the cut-off values provided by the manufacturer.
Statistical Analyses
Statistical analyses were performed using SPSS version 17.0 (SPSS Inc., Chicago, IL, USA). Goodness-of-fit χ2 tests were used to evaluate Hardy–Weinberg equilibrium (HWE) in the healthy subjects. Age and gender were compared between study groups using the independent t-test, χ2 test or one-way ANOVA. Three genetic models (additive, dominant, and recessive) were used to assess the genotype distribution difference between cases and controls by multivariate logistic regression with age and gender as covariates. Detailed descriptions of these three genetic models are shown in the Supplementary Materials. We calculated the power of our study design using Power and Sample Size Calculation software.2 We used the Haploview software package (version 4.2) for analyzing the LD patterns. A multiple-SNP score analysis was also performed as described previously (23), in which we evaluated the trend for association according to the number of relevant alleles the subjects carried. We used Bonferroni correction to adjust the P-values. A two-sided value of P < 0.0071 (0.05/7) was considered statistically significant.
Results
Demographic Data
The demographic characteristics in each cohort are shown in Table 1. There were no significant differences between cases and controls except for age in CLC. The TB patients in CLC were younger than LTBI individuals or HCs.
Genotyping Results and Distribution in Each Group
The genotype call rates of all SNPs were 99.99% and the consistency of repeat genotyping results for 5% of the samples was 100%. The SNP location, functional consequence, and the MAF in the controls of each cohort as well as Chinese Han in Beijing based on the 1000 Genomes Project database are presented in Table 2. Five SNPs are located in exons (four missense and one synonymous) as well as an upstream variant (rs10004195) and an intron variant (rs11466617). The MAFs of these SNPs in each cohort were similar to those in the 1000 Genomes Project database. The genotype distributions in each cohort are shown in Table 3. All the SNP genotype distributions were consistent with HWE in controls.
Analyses of Single TLR10 tagSNPs and Tuberculosis (TB) Risk
Association Analysis in Aba Tibetan Autonomous Prefecture Cohort
As shown in Table 4, two SNPs (rs11466617 and rs4129009) showed statistically significant differences after Bonferroni correction (P < 0.0071) in Tibetans by comparing the genotype distributions of TB patients with HCs. The rs11466617 variant was associated with decreased susceptibility to TB under three genetic models (additive: P = 4.2 × 10−5, OR = 0.691, 95% CI = 0.578–0.826; dominant: P = 2.3 × 10−4, OR = 0.696, 95% CI = 0.555–0.873; recessive: P = 0.003, OR = 0.434, 95% CI = 0.280–0.671). Likewise, rs4129009 had a higher MAF in the HCs in comparison with the TB patient group (additive: P = 0.002, OR = 0.748, 95% CI = 0.622–0.903). In contrast, no significant differences were found for the other five SNPs under any models.
Association Analysis in Chengdu Cohort
The analyses of association between TLR10 SNPs and TB in CC are shown in Table 5. We tested the same SNPs, with the aim of enhancing the reliability of the associations by attempting to replicate them in different populations. Five SNPs showed a value of P < 0.05 under some of the genetic models, e.g., rs10004195 (additive: P = 0.043), rs11096956 (additive: P = 0.049), rs11466617 (additive: P = 0.049), and rs11096957 (additive: P = 0.027, dominant: P = 0.021) However, we did not find any statistically significant locus related to an individual’s susceptibility to TB after Bonferroni correction.
Association Analysis in Chengdu LTBI Cohort
Due to a poor verification in the CC, we further investigated the association between TLR10 SNPs and TB risk by comparing the genotype distributions in the TB-uninfected group, the LTBI group, and the active TB group. We noted that the rs11466617 CC genotype exhibited a significantly higher frequency in the LTBI group, as compared with the active TB patients under the recessive model (shown in Table 6; P = 0.004, OR = 0.389, 95% CI = 0.204–0.744). There were no other SNPs that showed significant differences after Bonferroni correction under any genetic model either in comparison of the TB-uninfected with LTBI individuals or in comparison of LTBI individuals with active TB patients.
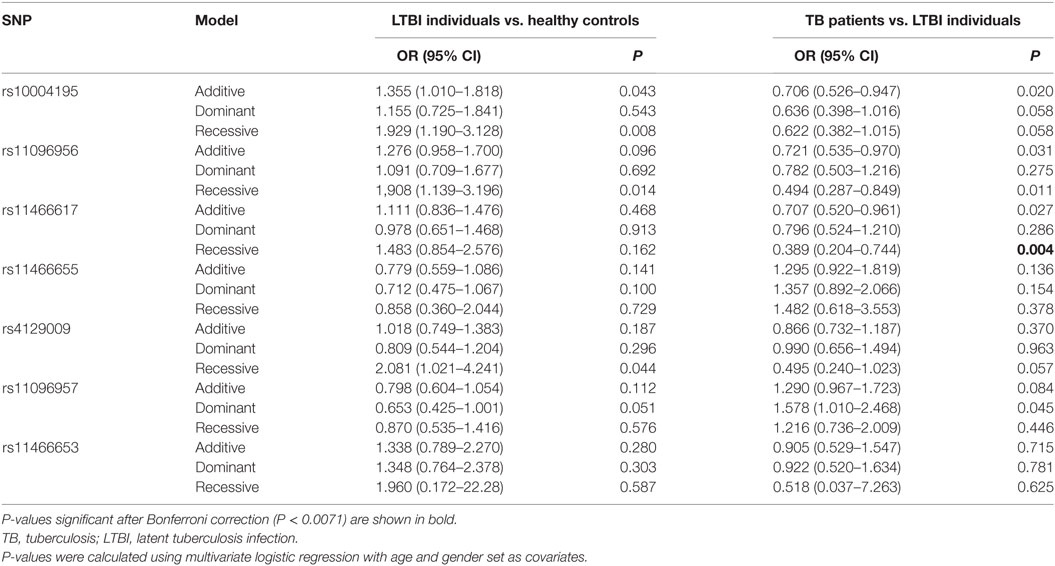
Table 6. Association between TLR10 tagSNPs and risk of TB and latent infection in the Chengdu latent tuberculosis infection cohort.
Analyses of Multiple-SNP Score on TB Risk
Given that the TLR10 gene variants were shown to have a dose-dependent effect on cytokine production, we also performed a multiple-SNP score analysis on TB risk in the three case–control studies. We first pooled the subjects from ATAPC and CC together and obtained four SNPs (rs10004195 rs11096956, rs11466617, rs4129009) with a significant difference (P < 0.0071, data not shown). As the LD between these tagSNPs was low (r2 < 0.5, as shown in Figure 1), we hypothesized that these polymorphisms influence susceptibility to TB risk independently of one another. As shown in Table 7, there was a correlation between the number of TLR10 protective alleles carried and decreasing TB risk in the analysis in ATAPC (P = 0.002 and 1.94 × 10−4 for trend). However, this dose-dependent protective effect was not observed in CC or CLC.
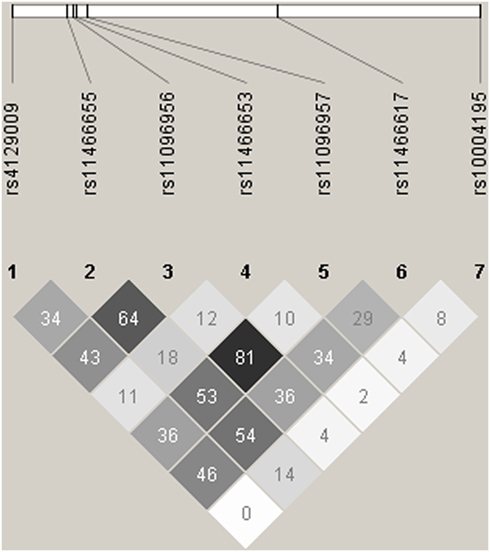
Figure 1. Linkage disequilibrium (LD) plots for LTR10. The LD plots were generated by Haploview 4.2. Polymorphisms are identified by their dbSNP rs numbers, and their relative positions are marked by vertical lines within the white horizontal bar. The numbers within squares indicate the r2 value, expressed as a percentile.
Power Analysis
In order to assess whether the power of study with the current simple size was sufficient, we calculated the power of all the SNPs under an allelic model. As shown in Figure S1 in Supplementary Material, the frequency of the SNPs in each group was sufficient to provide >90% power to detect a common risk allele with an OR of 1.6, except for rs11466653 in CLC.
Discussion
Strong evidence supports a critical role for genetic factors in susceptibility to TB infection and progression (24). In this self-validating case–control association study, we used tagSNPs that captured all the common variations in TLR10 to investigate the role of genetic variation in susceptibility to TB. We found two tagSNPs (rs11466617 and rs4129009) that were associated with TB susceptibility, most notably the C-allele of rs11466617 that exhibited a strong association with decreased TB risk in the Tibetan population. However, these two associations were not validated in the study of the Chinese Han population. We also observed that the CC genotype of rs11466617 was associated with resistance to activation from LTBI, but not associated with TB infection in the Han Chinese population. Additionally, we identified a dose-dependent protective effect in TB susceptibility among individuals carrying the “protective” alleles of TLR10.
Toll-like receptor family members play critical roles in the defense against Mtb infection, especially TLR2 that can trigger an immune response against Mtb by recognizing Mtb components and inducing pro-inflammatory signals (25), including the MyD88- and TRIF-dependent pathway (26, 27). However, very few functional studies have implicated TLR10 in the response to Mtb infection either in vivo or in vitro. Evidence shows that TLR10 in cooperation with TLR2 can recruit the proximal adaptor MyD88 to the complex, but fails to trigger the MyD88- and TRIF-dependent signaling, suggesting TLR10 functions as a negative regulator (15, 28), which may be involved in the progression of TB. Furthermore, this inhibitory property of TLR10 in immune regulation upon stimulation with TLR2 ligands is affected by TLR10 polymorphisms (14). We found that two SNPs of TLR10 were associated with decreased risk of TB in the Tibetan but not in the Chinese Han population, suggesting racial differences may impact on the risk of TB associated with these SNPs. Another underlying reason to explain this inconsistent result could be environmental factors. In particular, the Tibetans recruited in the present study lived in a region with higher altitude, which is considered to be a risk factor for TB (29). Therefore, a proportion of subjects in ATAPC may not suffer active TB disease if living in the region where the CC was conducted. Moreover, we used Bonferroni correction, in other words regarded a P < 0.0071 as the threshold for significance, to limit the type-1 error. However, reducing the chance of a type-1 error in this way may increase the possibility of a type-2 error. In the present study, we observed that a few SNPs showed a borderline P-value in CC, including rs11466617, the most significant locus in ATAPC.
It is widely accepted that the pathogenesis of TB can be thought of as a two-stage process: LTBI, the establishment of a productive infection but remaining asymptomatic, and active TB disease which is characterized by growth of Mtb in sputum and cultivation in culture or positive AFB smear, plus characteristic radiological signs and hallmark symptoms (30). Early observations detected considerable interindividual variability in the establishment of TB infection after exposure to Mtb in sailors who shared similar known environmental factors, suggesting genetic factors may influence this stage (31). On the other hand, only 5–15% of infected individuals develop active TB, indicating that genetic factors are associated with an individual’s susceptibility to trigger an active TB disease (2). However, the mechanisms involved in the host–pathogen interaction in each stage are extremely complex and not fully understood (32). It is reasonable to assume that different genetic polymorphisms may influence different stages. Given this hypothesis, we performed an association study in CLC in which we distinguished the different stages of TB, to investigate the role of TLR10 SNPs in the pathogenesis of TB, and to further explore the inconsistent results observed in CC. We demonstrated that rs11466617 only affected the status of Mtb-infected individuals with respect to LTBI vs. active TB. The mechanism underlying this association may partly relate to the mediator function of TLR10 in macrophages after Mtb has been engulfed (33), as well as a potential mechanism of apoptosis by TLR2 signaling (34). It can also explain the inconsistent result we observed in ATAPC and CC: more uninfected individuals in the control group of CC may have led to the negative result in this cohort, while the potentially higher frequency of LTBI in control group of ATAPC, based on the higher TB prevalence in Tibetan (29), may partly contribute to the significant result in ATAPC.
As the evidence from a functional study of TLR10 showed gene variant dosage effects on cytokine production (14), we also carried out a multi-SNP score test in three cohorts. We found that the TLR10 SNPs showed a dose-dependent protective effect on TB risk in Tibetans. Therefore, further study investigating the mechanism of TLR10 in the pathogenesis of TB could build on this observation.
Studies exploring the functional effects of TLR10 SNPs and their role in susceptibility to various diseases have been increasing. We searched the PubMed database using the key words “polymorphism” “SNP” “mutation” “variation” combined with “TLR10.” By screening of abstracts and full texts, we found a total of 56 relevant studies that investigated TLR10 SNPs in relation to phenotypes including infectious disease, carcinoma, allergic, and autoimmune disease as well as altered immunoreactions in vitro (see Table S2 in Supplementary Material). We also noted that three studies, using various designs, demonstrated that TLR10 SNPs were associated with susceptibility to TB. Bulat-Kardum et al. found that the rs11096957 AA genotype was associated with a predisposition to TB in the Caucasian population (8). However, in the present study, we did not observe association between rs11096957 and risk of TB. This inconsistent result is likely due to the ethnic difference, as the frequency of the A allele was much higher in the Caucasian population (0.6) than in the Han Chinese population (0.37), based on the 1000 Genomes Project data. Ma et al. demonstrated that nonsynonymous polymorphisms of TLR10 were significantly associated with TB in African and European Americans (18). The present study identified that rs4129009, which causes an amino-acid change in the TIR domain of the intracellular portion of the protein and results in increased cytokine responses (35), showed decreased risk of TB in Tibetans. Moreover, Uren et al. observed that an SNP (rs12233670) upstream of TLR10 was associated with TB risk by using genome-wide association data and case–control analysis of South Africans (36). Although we did not genotype this SNP, another upstream SNP (rs10004195) that is in perfect LD (r2 = 1) with rs12233670 was tested in our study. However, this locus was not associated with risk of TB in present study. Differences in ethnicity and study design may explain the inconsistent result.
Our study has several strengths. (a) This investigation represents the first exploration of the association between TLR10 genetic variants and TB risk in a Chinese population, with two ethnic groups and an additional study of TB infection status. In this study, we used the genetic database of Chinese Beijing Han to conduct tagSNP selection, as Chinese Han and Tibetans share a similar genetic background (37). The SNPs we studied cover the vast majority of the functional SNPs of TLR10 investigated in previous studies (Table S2 in Supplementary Material). (b) The sample size in the present study was sufficient to provide >90% power to detect a common risk allele with an OR of 1.6, except for rs11466653 in CLC (see Figure S1 in Supplementary Material). (c) Furthermore, in order to avoid the possibility of obtaining a false positive result, we used Bonferroni correction to adjust the P-values. We also limited potential confounding factors by excluding coinfection with HIV, comorbidities such as cancer, diabetes, immune system diseases, and chronic respiratory diseases as well as steroid use for inflammatory conditions.
Some limitations in this study must be noted. First, rs11466617 and rs4129009 were associated with decreased risk of TB in Tibetans but these associations did not replicate in the verification cohort of Chinese Han. Second, we did not elucidate the mechanism of how these variations affect the host immune response and the progression of TB in the subjects, although we noted that rs11466617 may only affect an individual’s susceptibility to progress from LTBI to active TB disease. Third, there is no gold standard diagnostic test for LTBI to date, though INF-γ release assay has an acceptable specificity, positive and negative predictive values for LTBI detection (38).
Conclusion
In summary, the present study indicated that two SNPs (rs11466617 and rs4129009) as well as a dose-dependent effect of TLR10 SNPs were associated with a decreased susceptibility to TB in Tibetans, but not in Chinese Han. Moreover, individuals carrying the rs11466617 CC genotype showed decreased risk of progression to active TB from LTBI. However, no genotype of TLR10 was associated with the establishment of LTBI after Mtb exposure. More studies are required to validate these findings in different populations and to elucidate the mechanistic role of TLR10 variants in TB susceptibility.
Ethics Statement
All protocols for this study were reviewed and approved by the Institutional Review Board of the West China Hospital of Sichuan University in 2013 (Protocol no. 131). Written informed consent was obtained from all the study participants.
Author Contributions
YW, M-MZ, and W-WH carried out the molecular genetic studies, statistical analysis, and drafted the manuscript. AS and J-QH contributed to the study conception and design, analysis, and interpretation of the data, and helped revise the manuscript. S-QW, M-GW, and X-yT carried out the sample acquisition and genotyping assays.
Conflict of Interest Statement
The authors declare that the research was conducted in the absence of any commercial or financial relationships that could be construed as a potential conflict of interest.
Acknowledgments
We thank all study participants for their cooperation. We also thank Shanghai Genesky Biotechnologies Inc. for technical support.
Funding
This work was supported by the Research Fund for the Doctoral Program of Higher Education of China (RFDP) (grant number 20130181110068), the National Natural Science Foundation of China (grant number 81170042 and grant number 81370121), and the National Scientific and Technological Major Project of China (grant number 2012ZX10004-901).
Supplementary Material
The Supplementary Material for this article can be found online at https://www.frontiersin.org/articles/10.3389/fimmu.2018.00309/full#supplementary-material.
Figure S1. Statistical power of each SNP in the ATAPC (A), CC (B), and CLC (C) cohorts. Power was calculated by the Power and Sample Size Calculation software.
Footnotes
References
1. Global Tuberculosis Report 2016. Geneva: World Health Organization (2016). Available from: http://apps.who.int/iris/bitstream/10665/250441/1/9789241565394-eng.pdf?ua=1
2. Zumla A, Raviglione M, Hafner R, von Reyn CF. Tuberculosis. N Engl J Med (2013) 368(8):745–55. doi:10.1056/NEJMra1200894
3. Bocchino M, Galati D, Sanduzzi A, Colizzi V, Brunetti E, Mancino G. Role of mycobacteria-induced monocyte/macrophage apoptosis in the pathogenesis of human tuberculosis. Int J Tuberc Lung Dis (2005) 9(4):375–83.
4. Sester M, van Leth F, Bruchfeld J, Bumbacea D, Cirillo DM, Dilektasli AG, et al. Risk assessment of tuberculosis in immunocompromised patients. A TBNET study. Am J Respir Crit Care Med (2014) 190(10):1168–76. doi:10.1164/rccm.201405-0967OC
5. Garantziotis S, Hollingsworth JW, Zaas AK, Schwartz DA. The effect of toll-like receptors and toll-like receptor genetics in human disease. Annu Rev Med (2008) 59:343–59. doi:10.1146/annurev.med.59.061206.112455
6. Cheepsattayakorn A, Cheepsattayakorn R. Human genetic influence on susceptibility of tuberculosis: from infection to disease. J Med Assoc Thai (2009) 92(1):136–41.
7. Schurz H, Daya M, Moller M, Hoal EG, Salie M. TLR1, 2, 4, 6 and 9 variants associated with tuberculosis susceptibility: a systematic review and meta-analysis. PLoS One (2015) 10(10):e0139711. doi:10.1371/journal.pone.0139711
8. Bulat-Kardum LJ, Etokebe GE, Lederer P, Balen S, Dembic Z. Genetic polymorphisms in the toll-like receptor 10, interleukin (IL)17A and IL17F genes differently affect the risk for tuberculosis in Croatian population. Scand J Immunol (2015) 82(1):63–9. doi:10.1111/sji.12300
9. Liu Q, Li W, Li D, Feng Y, Tao C. TIRAP C539T polymorphism contributes to tuberculosis susceptibility: evidence from a meta-analysis. Infect Genet Evol (2014) 27:32–9. doi:10.1016/j.meegid.2014.06.025
10. Imanishi T, Hara H, Suzuki S, Suzuki N, Akira S, Saito T. Cutting edge: TLR2 directly triggers Th1 effector functions. J Immunol (2007) 178(11):6715–9. doi:10.4049/jimmunol.178.11.6715
11. Jimenez-Dalmaroni MJ, Gerswhin ME, Adamopoulos IE. The critical role of toll-like receptors––from microbial recognition to autoimmunity: a comprehensive review. Autoimmun Rev (2016) 15(1):1–8. doi:10.1016/j.autrev.2015.08.009
12. Chuang T, Ulevitch RJ. Identification of hTLR10: a novel human toll-like receptor preferentially expressed in immune cells. Biochim Biophys Acta (2001) 1518(1–2):157–61. doi:10.1016/S0167-4781(00)00289-X
13. Hess NJ, Jiang S, Li X, Guan Y, Tapping RI. TLR10 is a B cell intrinsic suppressor of adaptive immune responses. J Immunol (2017) 198(2):699–707. doi:10.4049/jimmunol.1601335
14. Oosting M, Cheng SC, Bolscher JM, Vestering-Stenger R, Plantinga TS, Verschueren IC, et al. Human TLR10 is an anti-inflammatory pattern-recognition receptor. Proc Natl Acad Sci U S A (2014) 111(42):E4478–84. doi:10.1073/pnas.1410293111
15. Jiang S, Li X, Hess NJ, Guan Y, Tapping RI. TLR10 is a negative regulator of both MyD88-dependent and -independent TLR signaling. J Immunol (2016) 196(9):3834–41. doi:10.4049/jimmunol.1502599
16. Pecora ND, Gehring AJ, Canaday DH, Boom WH, Harding CV. Mycobacterium tuberculosis LprA is a lipoprotein agonist of TLR2 that regulates innate immunity and APC function. J Immunol (2006) 177(1):422–9. doi:10.4049/jimmunol.177.1.422
17. Arji N, Busson M, Iraqi G, Bourkadi JE, Benjouad A, Bouayad A, et al. Genetic diversity of TLR2, TLR4, and VDR loci and pulmonary tuberculosis in Moroccan patients. J Infect Dev Ctries (2014) 8(4):430–40. doi:10.3855/jidc.3820
18. Ma X, Liu Y, Gowen BB, Graviss EA, Clark AG, Musser JM. Full-exon resequencing reveals toll-like receptor variants contribute to human susceptibility to tuberculosis disease. PLoS One (2007) 2(12):e1318. doi:10.1371/journal.pone.0001318
19. Deplancke B, Alpern D, Gardeux V. The genetics of transcription factor DNA binding variation. Cell (2016) 166(3):538–54. doi:10.1016/j.cell.2016.07.012
20. Liu Q, Wang J, Sandford AJ, Wu J, Wang Y, Wu S, et al. Association of CYBB polymorphisms with tuberculosis susceptibility in the Chinese Han population. Infect Genet Evol (2015) 33:169–75. doi:10.1016/j.meegid.2015.04.026
21. Fiske CT, Yan FX, Hirsch-Moverman Y, Sterling TR, Reichler MR, Tuberculosis Epidemiologic Studies Consortium Task Order 2 Team. Risk factors for treatment default in close contacts with latent tuberculous infection. Int J Tuberc Lung Dis (2014) 18(4):421–7. doi:10.5588/ijtld.13.0688
22. Du W, Cheng J, Ding H, Jiang Z, Guo Y, Yuan H. A rapid method for simultaneous multi-gene mutation screening in children with nonsyndromic hearing loss. Genomics (2014) 104(4):264–70. doi:10.1016/j.ygeno.2014.07.009
23. Mira MT, Alcais A, Nguyen VT, Moraes MO, Di Flumeri C, Vu HT, et al. Susceptibility to leprosy is associated with PARK2 and PACRG. Nature (2004) 427(6975):636–40. doi:10.1038/nature02326
24. van Tong H, Velavan TP, Thye T, Meyer CG. Human genetic factors in tuberculosis: an update. Trop Med Int Health (2017) 22(9):1063–71. doi:10.1111/tmi.12923
25. Underhill DM, Ozinsky A, Hajjar AM, Stevens A, Wilson CB, Bassetti M, et al. The toll-like receptor 2 is recruited to macrophage phagosomes and discriminates between pathogens. Nature (1999) 401(6755):811–5. doi:10.1038/44605
26. Rahman A, Sobia P, Gupta N, Kaer LV, Das G. Mycobacterium tuberculosis subverts the TLR-2-MyD88 pathway to facilitate its translocation into the cytosol. PLoS One (2014) 9(1):e86886. doi:10.1371/journal.pone.0086886
27. Kim TH, Shin SJ, Park YM, Jung ID, Ryu SW, Kim DJ, et al. Critical role of TRIF and MyD88 in Mycobacterium tuberculosis Hsp70-mediated activation of dendritic cells. Cytokine (2015) 71(2):139–44. doi:10.1016/j.cyto.2014.09.010
28. Guan Y, Ranoa DR, Jiang S, Mutha SK, Li X, Baudry J, et al. Human TLRs 10 and 1 share common mechanisms of innate immune sensing but not signaling. J Immunol (2010) 184(9):5094–103. doi:10.4049/jimmunol.0901888
29. Sun W, Gong J, Zhou J, Zhao Y, Tan J, Ibrahim AN, et al. A spatial, social and environmental study of tuberculosis in China using statistical and GIS technology. Int J Environ Res Public Health (2015) 12(2):1425–48. doi:10.3390/ijerph120201425
31. Lamar JE II, Malakooti MA. Tuberculosis outbreak investigation of a U.S. Navy amphibious ship crew and the Marine expeditionary unit aboard, 1998. Mil Med (2003) 168(7):523–7.
32. Wolff KA, de la Peña AH, Nguyen HT, Pham TH, Amzel LM, Gabelli SB, et al. A redox regulatory system critical for mycobacterial survival in macrophages and biofilm development. PLoS Pathog (2015) 11(4):e1004839. doi:10.1371/journal.ppat.1004839
33. Regan T, Nally K, Carmody R, Houston A, Shanahan F, Macsharry J, et al. Identification of TLR10 as a key mediator of the inflammatory response to Listeria monocytogenes in intestinal epithelial cells and macrophages. J Immunol (2013) 191(12):6084–92. doi:10.4049/jimmunol.1203245
34. Wang L, Zuo M, Chen H, Liu S, Wu X, Cui Z, et al. Mycobacterium tuberculosis lipoprotein MPT83 induces apoptosis of infected macrophages by activating the TLR2/p38/COX-2 signaling pathway. J Immunol (2017) 198(12):4772–80. doi:10.4049/jimmunol.1700030
35. Mikacenic C, Reiner AP, Holden TD, Nickerson DA, Wurfel MM. Variation in the TLR10/TLR1/TLR6 locus is the major genetic determinant of interindividual difference in TLR1/2-mediated responses. Genes Immun (2013) 14(1):52–7. doi:10.1038/gene.2012.53
36. Uren C, Henn BM, Franke A, Wittig M, van Helden PD, Hoal EG, et al. A post-GWAS analysis of predicted regulatory variants and tuberculosis susceptibility. PLoS One (2017) 12(4):e0174738. doi:10.1371/journal.pone.0174738
37. Xu S, Li S, Yang Y, Tan J, Lou H, Jin W, et al. A genome-wide search for signals of high-altitude adaptation in Tibetans. Mol Biol Evol (2011) 28(2):1003–11. doi:10.1093/molbev/msq277
Keywords: TLR10, tuberculosis, latent tuberculosis infection, polymorphisms, susceptibility
Citation: Wang Y, Zhang M-M, Huang W-W, Wu S-Q, Wang M-G, Tang X-Y, Sandford AJ and He J-Q (2018) Polymorphisms in Toll-Like Receptor 10 and Tuberculosis Susceptibility: Evidence from Three Independent Series. Front. Immunol. 9:309. doi: 10.3389/fimmu.2018.00309
Received: 12 October 2017; Accepted: 05 February 2018;
Published: 23 February 2018
Edited by:
Hao Shen, University of Pennsylvania, United StatesReviewed by:
Javeed Ali Shah, University of Washington, United StatesCarmen Judith Serrano, Instituto Mexicano del Seguro Social (IMSS), Mexico
Copyright: © 2018 Wang, Zhang, Huang, Wu, Wang, Tang, Sandford and He. This is an open-access article distributed under the terms of the Creative Commons Attribution License (CC BY). The use, distribution or reproduction in other forums is permitted, provided the original author(s) and the copyright owner are credited and that the original publication in this journal is cited, in accordance with accepted academic practice. No use, distribution or reproduction is permitted which does not comply with these terms.
*Correspondence: Jian-Qing He, jianqhe@gmail.com, jiangqing_he@scu.edu.cn
†These authors have contributed equally to this work.