- 1Department of Neurology, The Third Affiliated Hospital of Sun Yat-sen University, Guangzhou, China
- 2Department of Hematology, The Third Affiliated Hospital of Sun Yat-sen University, Guangzhou, China
Objective: The triglyceride-glucose (TyG) index is a reliable surrogate of insulin resistance and a marker for ischemic stroke (IS) incident. Whether the TyG index predicts stroke outcome remains uncertain. This study investigated the prognostic value of the TyG index in critically ill stroke patients.
Methods: This was a retrospective observational study that included stroke patients, and all data were extracted from the eICU Collaborative Research Database. The TyG index was calculated as the ln [fasting glucose level (mg/dL) × triglyceride level (mg/dL)/2]. Outcomes included the hospital and intensive care unit (ICU) death. Multivariate logistic regression was used to determine independent risk factors. The smoothing curves and forest plots were illustrated.
Results: A total of 4,570 eligible subjects were enrolled. The mean level of TyG index was 9.1 ± 0.7. The hospital and ICU mortality rate were 10.3 and 5.0%, respectively. TyG index as a continuous variable was associated hospital mortality in univariate analysis (OR 1.723, 95% CI 1.524–1.948, P < 0.001), adjusted model 1 (OR 1.861, 95% CI 1637–2.116, P < 0.001), and adjusted model 2 (OR 2.543, 95% CI 1.588–4.073, P < 0.001). TyG was also associated ICU mortality in univariate analysis (OR 2.146, 95% CI 1.826–2.523, P < 0.001), adjusted model 1 (OR 2.183, 95% CI 1.847–2.580, P < 0.001), and adjusted model 2 (OR 2.672, 95% CI 1.376–5.188, P < 0.001). The smoothing curves observed a continuous linear association after adjusting all covariates both in hospital and ICU mortality. Subgroup analysis demonstrated TyG index was associated with increased risk of hospital and ICU death in critically ill IS (P < 0.05), but not in hemorrhage stroke (P > 0.05).
Conclusion: The TyG index is a potential predictor for hospital and ICU mortality in critically ill stroke patients, especially in IS patients.
Introduction
Stroke is a leading cause of mortality and disability worldwide. According to global burden of disease (GBD) 2016 stroke surveys, it was estimated that there were 5.5 million deaths due to stroke, and the global lifetime risk of stroke from the age of 25 years onward was ~25% (1, 2). The economic costs of treatment and post-stroke care are substantial, while the global burden of stroke has been increasing. Furthermore, an increasing number of stroke patients, who have higher hospital mortality, are being admitted to an intensive care unit (ICU) for management of severe neurological impairment and post-stroke complications (3, 4). Therefore, it is still important to identify the controllable risk factors targeted by preventive strategies and health-care management that lead to a larger decrease in stroke mortality.
The triglyceride-glucose (TyG) index combines both levels of fasting plasma glucose and triglyceride, and it has been reported to be significantly correlated with insulin resistance (IR) and to be a reliable surrogate marker of IR (5). Previous studies have indicated that the TyG index is associated with cardiovascular disease morbidity and mortality in the general population and different types of patient cohorts (6–9). Lately, the TyG index has been proposed as a direct marker for the risk of incident ischemic stroke (IS) in general population (10). To date, no relevant study has focused on the impact of the TyG index on mortality in patients with critically ill stroke including IS and hemorrhage stroke (HS). Therefore, this study aimed to specifically investigate the association between TyG index and mortality of stroke in a large observational multicentre study on eICU database.
Materials and Methods
Data Source
Data were collected from the eICU Collaborative Research Database v2.0. The database was a multi-center ICU database for over 200,000 admissions in 2014 and 2015 at 208 United States hospitals (11). The eICU database included hourly physiological readings from bedside monitors, records of demographic characteristics, severity of illness measures, diagnoses, treatment, and other clinical data collected during routine medical care. The use of this database has been approved by the institutional review boards of Massachusetts Institute of Technology (Cambridge, MA, USA). One author (Bingjun Zhang) obtained the access and was responsible for the data extraction (certification number: 36492956).
Participants
Patients with a primary diagnosis of brain stroke, recorded on patient dataset, were potentially eligible. The stroke patients can be grouped into 4 categories: IS group, HS group, IS with HS group, and unknown/others group. HS group included subarachnoid hemorrhage and intracerebral hemorrhage patients.
Demographic and Laboratory Variables
The following data were extracted from the eICU database: gender, age, ethnicity, admission height, admission weight, stroke types, plasma glucose values, blood lipids values, acute physiology, and chronic health evaluation (APACHE) IV score, the status of discharge hospital or ICU, in-hospital length of stay (LOS), ICU LOS, comorbidities, mechanical ventilation. The APACHE IV system is a tool used to risk-adjust ICU patients for ICU performance benchmarking (12), and it provides estimates of the probability that a patient dies given data from the first 24 h after the ICU admission (11).
If glucose, lipids, and some variables were measured for several times after the ICU entry, data of the first time were used. The TyG index was calculated as the ln [fasting glucose level (mg/dL) × triglyceride level (mg/dL)/2].
The primary outcome was hospital mortality, with ICU mortality as a secondary outcome.
Variables with more than 10% missing values were excluded from the analysis. Single imputation of EM algorithm was performed for variables with missing values of <10%.
Statistical Analyses
Categorical variables were expressed in absolute number with percentage and analyzed by chi-square or Fisher exact test. Continuous variables were first assessed for normality. Normal data were expressed in mean with standard deviation (SD) and compared using Student t-test or one-way ANOVA. Non-normal data were expressed in median with interquartile range (IQR) and were compared using Wilcoxon rank-sum test. Variables with two-tailed p value < 0.05 were considered to be statistically significant and were included in the regression model. Multivariate-adjusted odds ratios (OR) and 95% confidence intervals (CI) for the study outcomes and TyG index (1 unit and quartile) were calculated by logistic regression analysis. The multivariate model 1 included gender, age, ethnicity, while model 2 included model 1 plus stroke types, coronary heart disease, diabetes mellitus, heart failure, plasma glucose, total cholesterol (TC), low-density lipoprotein-cholesterol (LDL-C), triglyceride, mechanical ventilation, APACHE IV score, LOS. All of the analyses were performed using the SPSS version 26 (Chicago, Illinois). All p values were two-tailed and a p value < 0.05 was considered statistically significant. The smoothing curves and forest plots were illustrated by EmpowerStats (X&Y Solutions, Inc., Boston, MA).
Results
The initial search identified 200,859 ICU admissions from the eICU database. A total of 6,849 subjects with a primary diagnosis of brain stroke were identified. A total of 2,279 subjects were excluded because they did not have mortality values. The final cohort included 4,570 patients, including 4,101 (89.7%) survivors before hospital discharge and 4,341 (95.0%) survivors before ICU discharge. The study patients had an average age of 66.3 ± 14.2 years and 2,358 (51.6%) patients were male. The mean level of TyG index was 9.1 ± 0.7. The hospital and ICU mortality rate were 10.3% and 5.0%, respectively.
Baseline Characteristics
The baseline characteristics between survivors and non-survivors groups are described in Table 1. Hospital non-survivor group had higher age, plasma glucose, triglyceride, ICU LOS than hospital survivor group (P < 0.05), while lower TC and LDL-C (P < 0.05). There was no significant difference on sex, ethnicity, height, weight, HDL-C, hypertension, atrial fibrillation, hospital LOS (P > 0.05). Furthermore, hospital non-survivor group had more frequent diabetes mellitus, coronary heart disease, heart failure, mechanical ventilation than hospital survivor group (P < 0.05). Stroke type was significantly different between the two groups (P < 0.05). Patients in the hospital non-survivor group had higher APACHE IV score (70.8 ± 25.0 vs. 47.7 ± 19.0, P < 0.001) and TyG index (9.3 ± 0.7 vs. 9.0 ± 0.7, P < 0.001) than those survivor group.
In addition, there was no significant difference on age and TC between ICU survivors and ICU non-survivors groups (P > 0.05). ICU non-survivor group had lower hospital LOS than ICU survivor group (P < 0.001). Patients in the ICU non-survivor group had higher APACHE IV score (70.8 ± 25.0 vs. 47.7 ± 19.0, P < 0.001) and TyG index (9.3 ± 0.7 vs. 9.0 ± 0.7, P < 0.001) than those survivor group. Other results in ICU groups were consistent with those in-hospital groups.
TyG Index and Mortality of Critically Ill Stroke
Univariate and multivariate logistic regression revealed the association between TyG index and critically ill stroke mortality (Table 2). In univariate analysis, TyG index as a continuous variable was associated hospital mortality (OR 1.723, 95% CI 1.524–1.948, P < 0.001). Several risk factors including important clinical and significant variables in the univariate model were included in the multivariate model for adjustment, and the TyG index also remained to be an independent predictor of hospital mortality in adjusted model 1 (OR 1.861, 95% CI 1637–2.116, P < 0.001) and model 2 (OR 2.543, 95% CI 1.588–4.073, P < 0.001). Furthermore, TyG index was also associated ICU mortality in univariate analysis (OR 2.146, 95% CI 1.826–2.523, P < 0.001), adjusted model 1 (OR 2.183, 95% CI 1.847–2.580, P < 0.001), and adjusted model 2 (OR 2.672, 95% CI 1.376–5.188, P < 0.001).
In addition, all participants were stratified into four groups based on the quartile of TyG index. The mean levels of TyG index were 8.3 ± 0.3, 8.8 ± 0.1, 9.2 ± 0.1, and 10.0 ± 0.1, respectively. The hospital and ICU mortality significantly increased with increasing quartiles of the TyG index (P < 0.001) (Figure 1). When dividing TyG index into quartiles, we observed a quartile increment in TyG index was associated with the increased hospital and ICU mortality of critically ill stroke in univariate, adjusted model 1 and adjusted model 2 logistic regression analysis, with a significant trend across the quartiles (P for trend < 0.001, Table 2).
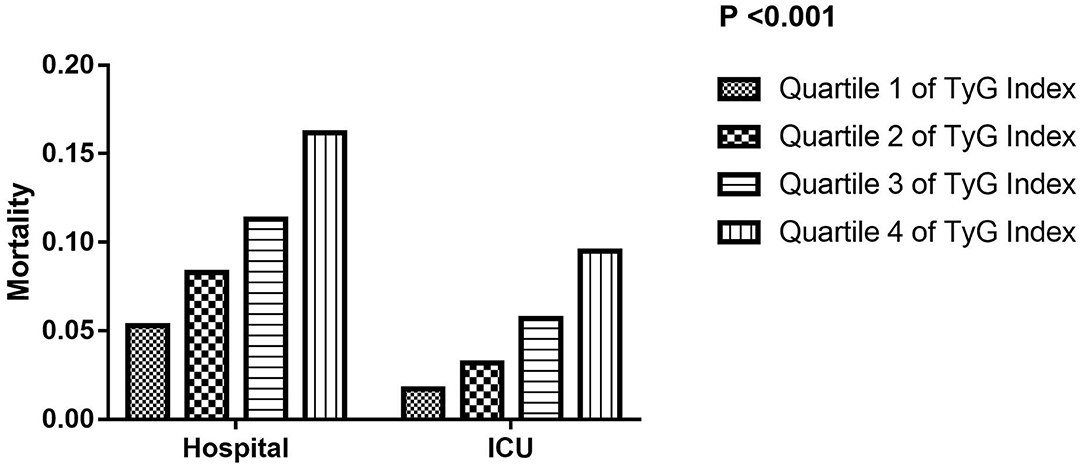
Figure 1. The hospital and ICU mortality according to TyG index quartiles. ICU, intensive care unit; TyG, triglyceride-glucose.
To further explore the relationship between TyG index and the mortality of critically ill stroke, we plotted the smoothing curves of TyG index against the hospital and ICU mortality of critically ill stroke (Figure 2). In this plot, we observed a continuous linear association after adjusting all covariates both in hospital (Figure 2A) and ICU (Figure 2B) mortality. This finding was consistent with the stepwise increased OR in the analysis of multivariate logistic regression.
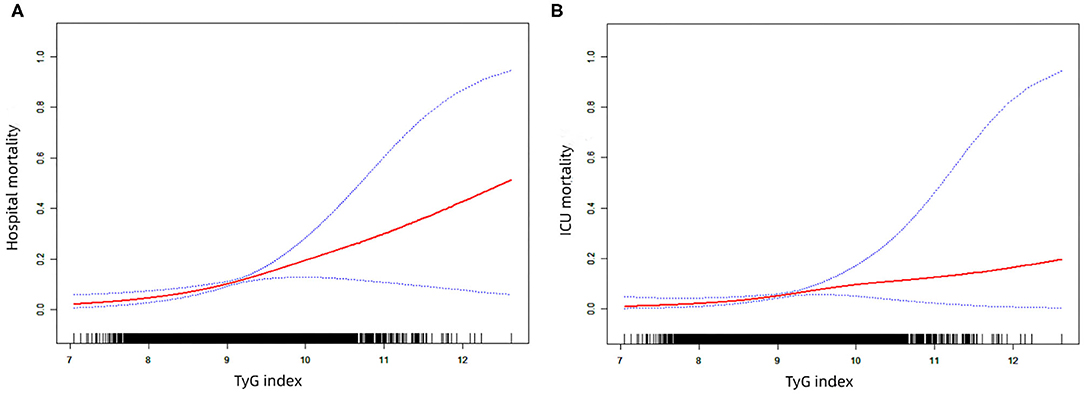
Figure 2. The smoothing curves of the hospital (A) and ICU (B) mortality of critically ill stroke against TyG index. ICU, intensive care unit; TyG triglyceride-glucose.
Relationship of TyG Index to Mortality According to Stroke Types
The stroke patients were grouped into 4 types: IS group, HS group, IS with HS group, and unknown/others group. Multivariate logistic regression models were analyzed to identify the association between TyG index and mortality according to the stroke type and TyG index. We found that a higher TyG index was significantly associated with the increased hospital and ICU mortality in IS group and unknown/others group (Figures 3, 4, adjusted P < 0.05). However, the similar result did not occur in HS group and IS with HS group (Figures 3, 4, adjusted P > 0.05).
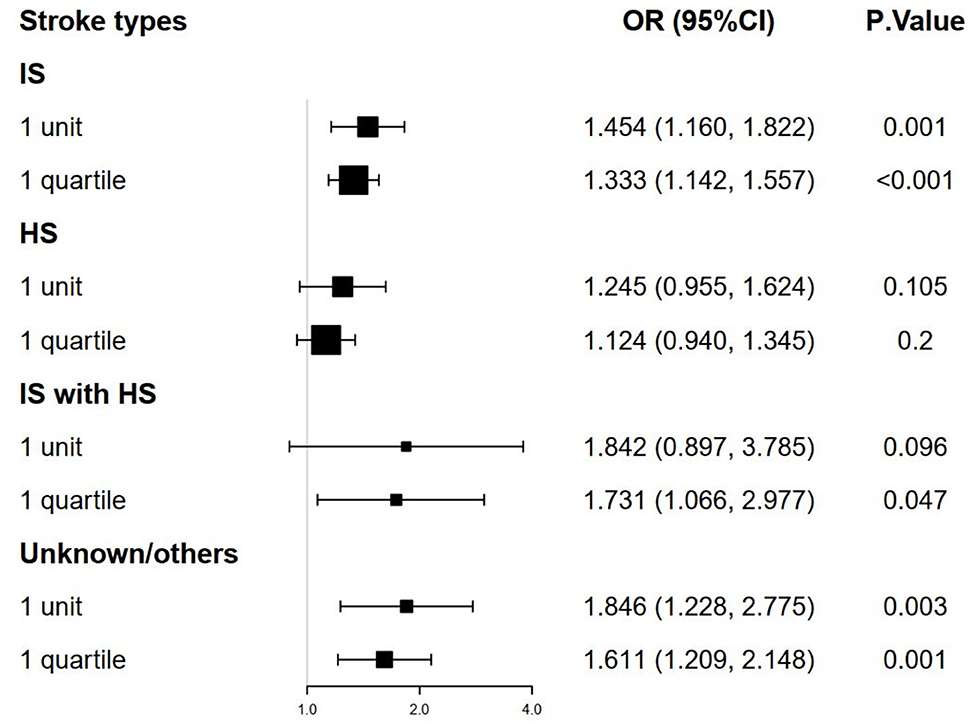
Figure 3. The forest plot of the hospital mortality of critically ill stroke according to stroke types and TyG index. TyG, triglyceride-glucose.
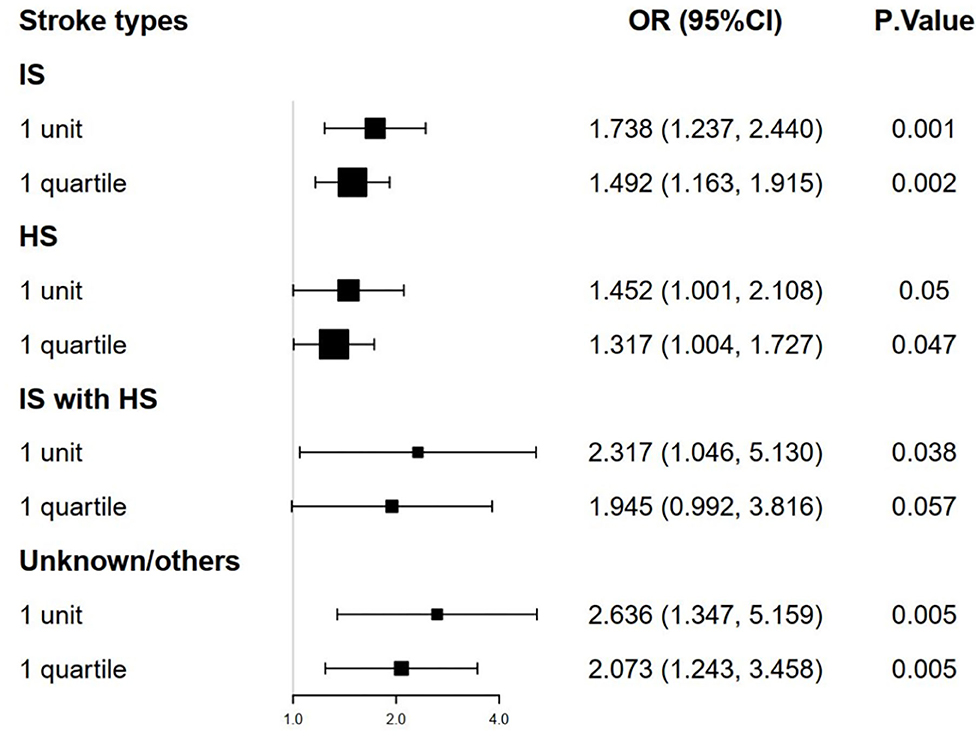
Figure 4. The forest plot of the ICU mortality of critically ill stroke according to stroke types and TyG index. ICU, intensive care unit; TyG triglyceride-glucose.
Discussion
To the best of our knowledge, this is the first study to evaluate the association of the TyG index with hospital and ICU mortality in critically ill stroke patients. The main findings are as follows: (1) the TyG index is an independent predictor for hospital and ICU mortality in patients with critically ill stroke; (2) the hospital and ICU mortality correlated proportionally with the increment of TyG index, implicating the linearity of TyG index as an indicator of critically ill stroke; (3) subgroup analysis demonstrated TyG index was associated with increased risk of hospital and ICU death in critically ill IS, but not in HS.
The TyG index, as the product of fasting plasma glucose and triglyceride, is a novel index that has been well-recognized as a simple and reliable surrogate of IR (13). The homeostatic model assessment of IR (HOMA-IR) has been traditionally used to estimate IR (14). However, insulin levels must be required to calculate the HOMA-IR index. Compared with the inconvenient HOMA-IR, the TyG index does not require levels of insulin and may apply to all of the patients and healthy population. Recent studies indicated that the TyG index has been shown to be superior to HOMA-IR in predicting IR (15). Furthermore, several studies conducted in Asia and Europe validated the strong association between TyG and incidence of diabetes mellitus (13, 16). Won et al. reported the TyG index was independently associated with arterial stiffness in a relatively healthy Korean population (17). Other studies demonstrated the TyG index was an independent predictor of coronary artery calcification progression and risk of cardiovascular diseases (8, 18). In addition, previous studies suggested the TyG index predicted severity and outcomes in patients with acute coronary syndrome (6, 19, 20). Wang et al. reported the TyG index predicted future cardiovascular events in patients with diabetes and acute coronary syndrome independently of known cardiovascular risk factors (7). However, in a previous study based on a Caucasian population, the TyG index displayed an insignificant association with stroke (21). Later, another study based on the same Caucasian cohort, identified metabolic health and obesity states groups based on TyG index was significantly associated with the risk of IS (22). Additionally, a recently published epidemiological investigation expanded the use of TyG index as a direct marker for the risk of IS (10). Zhou et al. demonstrated that the baseline TyG index was significantly associated with an increased risk of 12-month all cause mortality without accelerating poor functional outcome in IS patients (23). However, no relevant study focus on the association between TyG index and outcome in patients with critically ill stroke. The present study investigated the relationship between the TyG index and the hospital mortality in critically ill stroke on eICU database. We found that the TyG index was significantly associated with mortality in ICU stroke after adjusting for confounding factors. Furthermore, the TyG index had a continuous linear correlation with the mortality of ICU stroke.
The mechanism underlying the relationship between the TyG index and stroke is not fully elucidated. The formula of TyG index is composed of glucose and triglyceride, while recent evidence has proved that glucose and triglyceride disorders are the risk factors of stroke (24, 25). Hyperglycemia in stroke patients is associated with increased infarct volume, higher risk of secondary hemorrhagic transformation, and reduced recanalization rates after intravenous thrombolysis and endovascular thrombectomy (26, 27). Hyperglycemia on admission is strongly predictive of unfavorable outcome and high mortality (28). Under experimental stroke conditions, hyperglycemia promotes tissue acidosis, the production of reactive oxygen and nitrogen species, inflammatory cell infiltration, and the imbalance of matrix metalloprotease-9 that increase infarct size, brain swelling, hemorrhagic transformation, blood–brain barrier disruption and results in more severe neurological deficits (26, 29). A number of studies have indicated that hypertriglyceridemia is associated with early neurological deterioration and mortality in patients with stroke (30). Hypertriglyceridemia is related to the development of atherosclerosis and the loss of reserve capacity of cerebral vessels (31). Triglyceride, which comprises large amounts of arachidonic acid, can be involved in generating oxidative stress and thrombus (32). Hyperglycemia and dyslipidemia are two basic hallmarks of IR, while IR may play a vital role in the development of hyperglycemia and dyslipidemia (23). The TyG index has been deemed as a useful atherogenic indicator linked to IR and metabolic syndrome. IR may be the mechanism in increasing mortality in stroke. Firstly, IR may increase proinflammatory cytokines and enhance prothrombotic responses, thus exacerbating damage in the brain after stroke (33, 34). Secondly, IR may cause sympathetic activity and catabolism in muscles, thus enhancing muscle loss and leading to poor functional outcomes (35). Thirdly, IR may increase platelet adhesion, enhance atherosclerosis progression, facilitate plaque instability, and therefore contribute to severity of stroke, via promoting apoptosis of vascular smooth muscle cells macrophages, and endothelial cells (36, 37). Fourthly, IR may augment the role of modifiable risk factors of stroke, such as hypertension, atrial fibrillation (10). Although the present study had showed the association between TyG index and post-stroke outcomes, the underlying molecular mechanisms involved in this association should be further investigated in the future study.
Stroke accounts for almost 5% of all disability-adjusted life-years and 10% of all deaths worldwide (2). From different epidemiological surveys, stroke mortality in different periods varies from 5 to 72% (38, 39). Stroke mortality has been declining since the early twentieth century (40). The reasons for this are not completely understood, although the decline is welcome (40). However, due to the increasing complexity of stroke treatment and severe conditions, an increasing proportion of acute stroke patients are being admitted to an ICU (41, 42). A study including 4,958 consecutive stroke patients reported 347 (7.0%) patients required ICU admission at any time point during their index hospitalization (4). In-hospital mortality of ICU stroke reported in the literature varies widely, highly depending on the patient characteristics. A previous small study found the mortality was 38.7% (43/111) in stroke patients requiring ICU admission (41). An United States study including 448 ICU stroke patients provided hospital mortality was 30% (43). Recently, a prospective observation trial reported the mortality was 7.5% in critically ill stroke patients (44). These patients with high hospital mortality mainly associated with older age, poor neurological severity at admission, high APACHE score, impaired consciousness, intracranial hemorrhage, and need for mechanical ventilation (41, 42). Moreover, functional outcomes in survivors appear to be poor (45). The current study based on eICU database, indicated the hospital and ICU mortality were 10.3 and 5.0%, respectively. Furthermore, we found a new risk factor, the TyG index, for the mortality of critically ill stroke.
In subgroup analysis, we demonstrated TyG index was associated with increased risk of hospital and ICU death in critically ill IS, but not in HS. In agreement with previous GBD 2016 report, we did not estimate the mortality due to subarachnoid hemorrhage and intracerebral hemorrhage separately (1). Of the total number of prevalent strokes, over 80% were IS. However, the number of global deaths due to IS was slightly lower than the number due to HS deaths (1). In contrast, a previous neurology ICU study demonstrated HS was more frequent than IS (71.9 vs. 28.1%) (46). Several studies, including our present study, described TyG index was a useful marker in IS. However, to our knowledge, no relevant study has evaluated the relationship between the TyG index and HS. Although previous studies had focused on the relationship between IR and HS, the results had been discrepancy. The Rotterdam study and the Uppsala study had previously examined the association between IR and risk of HS, finding virtually no evidence of an association (47, 48). Later, a large United States stroke cohort showed IR may be a protective effect on HS (49). In the current study, we did not observe a significant association of the TyG index with all-cause death in critically ill HS in either unadjusted or adjusted analysis.
Although our study based on a large multicentre critical care database, it still has some limitations. First, this was a retrospective analysis derived from an observational study, which could not definitively establish causality. Second, the eICU v2.0 did not contain the data on head imaging, neurological severity scores, and follow-up outcomes after discharge. Residual confounding could exist. Third, the baseline levels of plasma glucose and triglyceride could be affected by the use of antidiabetic and lipid-lowering drugs before ICU admission. The TyG index might have changed during hospital; therefore, it is unknown whether the change in the TyG index could have predicted the mortality. Fourth, we did not measure HOMA-IR because the examination of insulin levels is not included in the eICU v2.0. Last, the data were from the United States, and thus the results may not apply fully to ICUs elsewhere with different practices or resources.
Conclusions
In this multicentre critically ill stroke cohort, we observed the association of the TyG index with hospital and ICU mortality in stroke patients. For the first time, this study demonstrated that the TyG index is a potential predictor for hospital and ICU mortality in critically ill stroke patients, especially in IS patients. Furthermore, the TyG index has a linear correlation with the mortality of ICU stroke. Most importantly, these findings suggested that the TyG index may be a useful indicator for risk stratification and prognosis in patients with critically ill stroke. Further prospective studies are required to confirm our findings.
Data Availability Statement
Data were fully available at https://eicu-crd.mit.edu/. Source code for data extraction can be found at https://github.com/tyg999/TyG-stroke.
Ethics Statement
The establishment of this database was approved by the Massachusetts Institute of Technology (Cambridge, MA), and consent was obtained for the original data collection. Therefore, the ethical approval statement and the need for informed consent were waived for this manuscript.
Author Contributions
BZ, LL, HR, and ZL designed research. QZ and DY performed experiments and analyzed data. BZ, YY, and XM wrote the main manuscript text and prepared figures. BZ and XM edited and revised manuscript. All authors reviewed and approved the manuscript.
Funding
This study was supported by the National Natural Science Foundation of China (81971110 and 81671178), The Guangdong Basic and Applied Basic Research Foundation (2020A1515010056), and The Third Affiliated Hospital of Sun Yat-Sen University, Clinical Research Program (QHJH201907).
Conflict of Interest
The authors declare that the research was conducted in the absence of any commercial or financial relationships that could be construed as a potential conflict of interest.
Acknowledgments
We thank all the investigators and subjects who participated in this project.
References
1. GBDS Stroke Collaborators. Global, regional, and national burden of stroke, 1990-2016: a systematic analysis for the global burden of disease study 2016. Lancet Neurol. (2019) 18:439–58. doi: 10.1016/S1474-4422(19)30034-1
2. Feigin VL, Nguyen G, Cercy K, Johnson CO, Alam T, Parmar PG, et al. Global, regional, and country-specific lifetime risks of stroke, 1990 and 2016. N. Engl. J. Med. (2018) 379:2429–37. doi: 10.1056/NEJMoa1804492
3. Smith M, Citerio G. Focus on clinical neuroscience. Intensive Care Med. (2016) 42:460–2. doi: 10.1007/s00134-015-4207-1
4. Alonso A, Ebert AD, Kern R, Rapp S, Hennerici MG, Fatar M. Outcome predictors of acute stroke patients in need of intensive care treatment. Cerebrovasc. Dis. (2015) 40:10–7. doi: 10.1159/000430871
5. Unger G, Benozzi SF, Perruzza F, Pennacchiotti GL. Triglycerides and glucose index: a useful indicator of insulin resistance. Endocr. Nutr. (2014) 61:533–40. doi: 10.1016/j.endoen.2014.11.006
6. Ma X, Dong L, Shao Q, Cheng Y, Lv S, Sun Y, et al. Triglyceride glucose index for predicting cardiovascular outcomes after percutaneous coronary intervention in patients with type 2 diabetes mellitus and acute coronary syndrome. Cardiovas. Diabetol. (2020) 19:31. doi: 10.1186/s12933-020-01006-7
7. Wang L, Cong HL, Zhang JX, Hu YC, Wei A, Zhang YY, et al. Triglyceride-glucose index predicts adverse cardiovascular events in patients with diabetes and acute coronary syndrome. Cardiovas. Diabetol. (2020) 19:80. doi: 10.1186/s12933-020-01054-z
8. Won KB, Park EJ, Han D, Lee JH, Choi SY, Chun EJ, et al. Triglyceride glucose index is an independent predictor for the progression of coronary artery calcification in the absence of heavy coronary artery calcification at baseline. Cardiovas. Diabetol. (2020) 19:34. doi: 10.1186/s12933-020-01008-5
9. Li S, Guo B, Chen H, Shi Z, Li Y, Tian Q, et al. The role of the triglyceride (triacylglycerol) glucose index in the development of cardiovascular events: a retrospective cohort analysis. Sci. Rep. (2019) 9:7320. doi: 10.1038/s41598-019-43776-5
10. Shi W, Xing L, Jing L, Tian Y, Yan H, Sun Q, et al. Value of triglyceride-glucose index for the estimation of ischemic stroke risk: insights from a general population. Nutr. Metab. Cardiovasc. Dis. (2020) 30:245–53. doi: 10.1016/j.numecd.2019.09.015
11. Pollard TJ, Johnson AEW, Raffa JD, Celi LA, Mark RG, Badawi O. The eICU collaborative research database, a freely available multi-center database for critical care research. Sci. Data. (2018) 5:180178. doi: 10.1038/sdata.2018.178
12. Zimmerman JE, Kramer AA, McNair DS, Malila FM. Acute physiology and chronic health evaluation (APACHE) IV: hospital mortality assessment for today's critically ill patients. Crit. Care Med. (2006) 34:1297–310. doi: 10.1097/01.CCM.0000215112.84523.F0
13. Du T, Yuan G, Zhang M, Zhou X, Sun X, Yu X. Clinical usefulness of lipid ratios, visceral adiposity indicators, and the triglycerides and glucose index as risk markers of insulin resistance. Cardiovas. Diabetol. (2014) 13:146. doi: 10.1186/s12933-014-0146-3
14. Wallace TM, Matthews DR. The assessment of insulin resistance in man. Diab. Med. (2002) 19:527–34. doi: 10.1046/j.1464-5491.2002.00745.x
15. Vasques AC, Novaes FS, de Oliveira Mda S, Souza JR, Yamanaka A, Pareja JC, et al. TyG index performs better than HOMA in a Brazilian population: a hyperglycemic clamp validated study. Diabetes Res. Clin. Pract. (2011) 93:e98–e100. doi: 10.1016/j.diabres.2011.05.030
16. Lee JW, Lim NK, Park HY. The product of fasting plasma glucose and triglycerides improves risk prediction of type 2 diabetes in middle-aged Koreans. BMC Endocr. Disord. (2018) 18:33. doi: 10.1186/s12902-018-0259-x
17. Won KB, Park GM, Lee SE, Cho IJ, Kim HC, Lee BK, et al. Relationship of insulin resistance estimated by triglyceride glucose index to arterial stiffness. Lipids Health Dis. (2018) 17:268. doi: 10.1186/s12944-018-0914-2
18. Park K, Ahn CW, Lee SB, Kang S, Nam JS, Lee BK, et al. Elevated TyG index predicts progression of coronary artery calcification. Diabetes Care. (2019) 42:1569–73. doi: 10.2337/dc18-1920
19. Mao Q, Zhou D, Li Y, Wang Y, Xu SC, Zhao XH. The triglyceride-glucose index predicts coronary artery disease severity and cardiovascular outcomes in patients with non-ST-segment elevation acute coronary syndrome. Dis. Markers. (2019) 2019:6891537. doi: 10.1155/2019/6891537
20. Jin JL, Cao YX, Wu LG, You XD, Guo YL, Wu NQ, et al. Triglyceride glucose index for predicting cardiovascular outcomes in patients with coronary artery disease. J. Thorac. Dis. (2018) 10:6137–46. doi: 10.21037/jtd.2018.10.79
21. Sánchez-Íñigo L, Navarro-González D, Fernández-Montero A, Pastrana-Delgado J, Martínez JA. The TyG index may predict the development of cardiovascular events. Eur. J. Clin. Invest. (2016) 46:189–97. doi: 10.1111/eci.12583
22. Sánchez-Iñigo L, Navarro-González D, Fernández-Montero A, Pastrana-Delgado J, Martínez JA. Risk of incident ischemic stroke according to the metabolic health and obesity states in the vascular-metabolic CUN cohort. Int. J. Stroke. (2017) 12:187–91. doi: 10.1177/1747493016672083
23. Zhou Y, Pan Y, Yan H, Wang Y, Li Z, Zhao X, et al. Triglyceride glucose index and prognosis of patients with ischemic stroke. Front. Neurol. (2020) 11:456. doi: 10.3389/fneur.2020.00456
24. Budoff M. Triglycerides and triglyceride-rich lipoproteins in the causal pathway of cardiovascular disease. Am. J. Cardiol. (2016) 118:138–45. doi: 10.1016/j.amjcard.2016.04.004
25. Yao M, Ni J, Zhou L, Peng B, Zhu Y, Cui L. Elevated fasting blood glucose is predictive of poor outcome in non-diabetic stroke patients: a sub-group analysis of SMART. PLoS ONE. (2016) 11:e0160674. doi: 10.1371/journal.pone.0160674
26. Chamorro Á, Brown S, Amaro S, Hill MD, Muir KW, Dippel DWJ, et al. Glucose modifies the effect of endovascular thrombectomy in patients with acute stroke. Stroke. (2019) 50:690–6. doi: 10.1161/STROKEAHA.118.023769
27. Capes SE, Hunt D, Malmberg K, Pathak P, Gerstein HC. Stress hyperglycemia and prognosis of stroke in nondiabetic and diabetic patients: a systematic overview. Stroke. (2001) 32:2426–32. doi: 10.1161/hs1001.096194
28. Bruno A, Levine SR, Frankel MR, Brott TG, Lin Y, Tilley BC, et al. Admission glucose level and clinical outcomes in the NINDS rt-PA Stroke Trial. Neurology. (2002) 59:669–74. doi: 10.1212/WNL.59.5.669
29. Suh SW, Shin BS, Ma H, Van Hoecke M, Brennan AM, Yenari MA, et al. Glucose and NADPH oxidase drive neuronal superoxide formation in stroke. Ann. Neurol. (2008) 64:654–63. doi: 10.1002/ana.21511
30. Deng Q, Li S, Zhang H, Wang H, Gu Z, Zuo L, et al. Association of serum lipids with clinical outcome in acute ischaemic stroke: a systematic review and meta-analysis. J. Clin. Neurosci. (2019) 59:236–44. doi: 10.1016/j.jocn.2018.09.003
31. Choi KH, Park MS, Kim JT, Chang J, Nam TS, Choi SM, et al. Serum triglyceride level is an important predictor of early prognosis in patients with acute ischemic stroke. J. Neurol. Sci. (2012) 319:111–6. doi: 10.1016/j.jns.2012.04.018
32. Bakker SJ, RG IJ, Teerlink T, Westerhoff HV, Gans RO, Heine RJ. Cytosolic triglycerides and oxidative stress in central obesity: the missing link between excessive atherosclerosis, endothelial dysfunction, and beta-cell failure? Atherosclerosis. (2000) 148:17–21. doi: 10.1016/S0021-9150(99)00329-9
33. Ozkul A, Turgut ET, Akyol A, Yenisey C, Kadikoylu G, Tataroglu C, et al. The relationship between insulin resistance and hypercoagulability in acute ischemic stroke. Eur. Neurol. (2010) 64:201–6. doi: 10.1159/000319196
34. Harada S, Fujita-Hamabe W, Tokuyama S. Ischemic stroke and glucose intolerance: a review of the evidence and exploration of novel therapeutic targets. J. Pharmacol. Sci. (2012) 118:1–13. doi: 10.1254/jphs.11R04CR
35. Narici MV, Maffulli N. Sarcopenia: characteristics, mechanisms and functional significance. Br. Med. Bull. (2010) 95:139–59. doi: 10.1093/bmb/ldq008
36. Bornfeldt KE, Tabas I. Insulin resistance, hyperglycemia, and atherosclerosis. Cell Metab. (2011) 14:575–85. doi: 10.1016/j.cmet.2011.07.015
37. Sung KC, Wild SH, Kwag HJ, Byrne CD. Fatty liver, insulin resistance, and features of metabolic syndrome: relationships with coronary artery calcium in 10,153 people. Diabetes Care. (2012) 35:2359–64. doi: 10.2337/dc12-0515
38. García-Berrocoso T, Giralt D, Bustamante A, Etgen T, Jensen JK, Sharma JC, et al. B-type natriuretic peptides and mortality after stroke: a systematic review and meta-analysis. Neurology. (2013) 81:1976–85. doi: 10.1212/01.wnl.0000436937.32410.32
39. Singh RJ, Chen S, Ganesh A, Hill MD. Long-term neurological, vascular, and mortality outcomes after stroke. Int. J. Stroke. (2018) 13:787–96. doi: 10.1177/1747493018798526
40. Seminog OO, Scarborough P, Wright FL, Rayner M, Goldacre MJ. Determinants of the decline in mortality from acute stroke in England: linked national database study of 795, 869 adults. BMJ. (2019) 365:l1778. doi: 10.1136/bmj.l1778
41. Sonneville R, Gimenez L, Labreuche J, Smonig R, Magalhaes E, Bouadma L, et al. What is the prognosis of acute stroke patients requiring ICU admission? Intensive Care Med. (2017) 43:271–2. doi: 10.1007/s00134-016-4553-7
42. van Valburg MK, Arbous MS, Georgieva M, Brealey DA, Singer M, Geerts BF. Clinical predictors of survival and functional outcome of stroke patients admitted to critical care. Crit. Care Med. (2018) 46:1085–92. doi: 10.1097/CCM.0000000000003127
43. Rincon F, Morino T, Behrens D, Akbar U, Schorr C, Lee E, et al. Association between out-of-hospital emergency department transfer and poor hospital outcome in critically ill stroke patients. J. Crit. Care. (2011) 26:620–5. doi: 10.1016/j.jcrc.2011.02.009
44. Suntrup-Krueger S, Schmidt S, Warnecke T, Steidl C, Muhle P, Schroeder JB, et al. Extubation readiness in critically Ill stroke patients. Stroke. (2019) 50:1981–8. doi: 10.1161/STROKEAHA.118.024643
45. Kirkman MA, Citerio G, Smith M. The intensive care management of acute ischemic stroke: an overview. Intensive Care Med. (2014) 40:640–53. doi: 10.1007/s00134-014-3266-z
46. Wang D, Guo Y, Zhang Y, Li Z, Li A, Luo Y. Epidemiology of acute kidney injury in patients with stroke: a retrospective analysis from the neurology ICU. Intern. Emerg. Med. (2018) 13:17–25. doi: 10.1007/s11739-017-1703-z
47. Wiberg B, Sundström J, Zethelius B, Lind L. Insulin sensitivity measured by the euglycaemic insulin clamp and proinsulin levels as predictors of stroke in elderly men. Diabetologia. (2009) 52:90–6. doi: 10.1007/s00125-008-1171-0
48. Wieberdink RG, Koudstaal PJ, Hofman A, Witteman JC, Breteler MM, Ikram MA. Insulin resistance and the risk of stroke and stroke subtypes in the nondiabetic elderly. Am. J. Epidemiol. (2012) 176:699–707. doi: 10.1093/aje/kws149
Keywords: triglyceride-glucose index, insulin resistance, stroke, mortality, ICU
Citation: Zhang B, Liu L, Ruan H, Zhu Q, Yu D, Yang Y, Men X and Lu Z (2020) Triglyceride-Glucose Index Linked to Hospital Mortality in Critically Ill Stroke: An Observational Multicentre Study on eICU Database. Front. Med. 7:591036. doi: 10.3389/fmed.2020.591036
Received: 03 August 2020; Accepted: 05 October 2020;
Published: 28 October 2020.
Edited by:
Tzvi Dwolatzky, Technion Israel Institute of Technology, IsraelReviewed by:
Zhongheng Zhang, Sir Run Run Shaw Hospital, ChinaLiping Liu, Capital Medical University, China
Copyright © 2020 Zhang, Liu, Ruan, Zhu, Yu, Yang, Men and Lu. This is an open-access article distributed under the terms of the Creative Commons Attribution License (CC BY). The use, distribution or reproduction in other forums is permitted, provided the original author(s) and the copyright owner(s) are credited and that the original publication in this journal is cited, in accordance with accepted academic practice. No use, distribution or reproduction is permitted which does not comply with these terms.
*Correspondence: Zhengqi Lu, bHpxMTgyOEBvdXRsb29rLmNvbQ==; Xuejiao Men, bXh1ZWppYW9AbWFpbC5zeXN1LmVkdS5jbg==
†These authors have contributed equally to this work