A Simple-to-Use Web-Based Calculator for Survival Prediction in Acute Respiratory Distress Syndrome
- Department of Emergency, The First Affiliated Hospital of Nanchang University, Nanchang, China
Background: The aim of this study was to construct and validate a simple-to-use model to predict the survival of patients with acute respiratory distress syndrome.
Methods: A total of 197 patients with acute respiratory distress syndrome were selected from the Dryad Digital Repository. All eligible individuals were randomly stratified into the training set (n=133) and the validation set (n=64) as 2: 1 ratio. LASSO regression analysis was used to select the optimal predictors, and receiver operating characteristic and calibration curves were used to evaluate accuracy and discrimination of the model. Clinical usefulness of the model was also assessed using decision curve analysis and Kaplan-Meier analysis.
Results: Age, albumin, platelet count, PaO2/FiO2, lactate dehydrogenase, high-resolution computed tomography score, and etiology were identified as independent prognostic factors based on LASSO regression analysis; these factors were integrated for the construction of the nomogram. Results of calibration plots, decision curve analysis, and receiver operating characteristic analysis showed that this model has good predictive ability of patient survival in acute respiratory distress syndrome. Moreover, a significant difference in the 28-day survival was shown between the patients stratified into different risk groups (P < 0.001). For convenient application, we also established a web-based calculator (https://huangl.shinyapps.io/ARDSprognosis/).
Conclusions: We satisfactorily constructed a simple-to-use model based on seven relevant factors to predict survival and prognosis of patients with acute respiratory distress syndrome. This model can aid personalized treatment and clinical decision-making.
Introduction
Acute respiratory distress syndrome (ARDS) is a clinically and pathophysiologically complex syndrome characterized by rapid progression and devastating hypoxemic respiratory failure (1). Many risk factors, such as sepsis, pneumonia, pancreatitis, and major trauma, are associated with the development of ARDS (2). Although there has been some progress in ARDS treatment in the last several decades, the prognosis of patients with ARDS are still not satisfactory. The in-hospital mortality rate of ARDS patients remains between 34 and 60% (3). At present, the treatment of ARDS predominantly includes mechanical ventilation therapy (4). Therefore, identification of novel and effective treatment strategies is crucial for patients with ARDS. Moreover, a simple-to-use clinical prediction model is also required to provide adequate care to patients with ARDS.
The severity of ARDS is often assessed using the PaO2/FiO2 ratio, although this variable has a low-to-moderate prognostic value (5). Recently, several biomarkers including inflammation cytokines, epithelial or endothelial damage, and coagulation have been established to evaluated prognosis and therapeutic response of patients with ARDS. For example, a meta-analysis reported that elevated plasma levels of angiopoietin-2 strongly correlate with diagnosis and mortality in populations at high risk of ARDS (6). Moreover, various clinical biomarkers including lung inflammatory mediators (soluble suppression of tumorigenicity-2 and interleukin-6) (7) and products of epithelial and endothelial injury (the soluble form of the receptor for advanced glycation end products) (8, 9) were developed to monitor pathophysiologic changes and outcomes of ARDS. Unfortunately, although several lung-specific biomarkers have been validated to assess ARDS; however, none of them have been applied into clinical practice. Currently, there is no favorable prognosis prediction model for ARDS.
Nomograms (visualized graphs of a predictive model) are widely applied for prognosis and prediction of various diseases (10, 11). To date, no nomogram and corresponding web-based calculator has been developed to predict the prognosis of ARDS patients. Therefore, a refined model is needed to predict the prognosis of ARDS and guide clinical treatment. In this study, we aimed to construct a web-based calculator to predict the 28-day survival of patients with ARDS using several clinical parameters that are routinely used and readily available. This simple-to-use calculator might serve as an early warning and prediction system for patients with ARDS.
Methods
Patients
A total of 197 patients with ARDS were extracted from the Dryad Digital Repository (http://www.datadryad.org/), which was shared by Anan et al. (12). All ARDS patients were diagnosed according to the Berlin definition (5). Patients with chronic interstitial lung disease (idiopathic pulmonary fibrosis), vasculitis or alveolar hemorrhage, hypersensitivity pneumonitis were excluded. All eligible patients were randomly stratified into two groups in a 2:1 ratio (training set and validation set, respectively). The extracted clinical data included age, gender, white cell count (WBC), C-reactive protein (CRP), lactate dehydrogenase (LDH), albumin (Alb), platelet count (PLT), PEEP, SOFA score, high-resolution computed tomography (HRCT) score, McCabe score, PaO2/FiO2, ARDS etiology, survival time, and survival status. Institutional ethical approval was not necessary because all the data were obtained from an online database.
Development of the Nomogram
To obtain the subset of predictors, the LASSO regression analysis was used to select the optimal predictors from the risk factors in the training cohort. The “glmnet” package was used to perform the LASSO regression analysis (13, 14). Finally, using the selected predictors from the LASSO regression, a nomogram was developed using the “rms,” “survival,” “foreign,” and “openxlsx” R packages (15–18). A dynamic web-based calculator was constructed using “DynNom” package (19).
Validation of the Nomogram
To validate the constructed nomogram, the corresponding calibration map and receiver operating characteristic (ROC) analysis were performed in the training and validation sets to assess the prognostic accuracy of the nomogram by using the “rms,” “survival,” “foreign,” and “timeROC” R packages (20). In addition, decision curve analysis (DCA) was performed to quantify the clinical applicability of the nomogram.
Statistical Analysis
The raw data were expressed as mean ± standard deviation when normally distributed, while expressed as median (interquartile range) when non-normally distributed. Differences between two groups were analyzed using chi-square tests for categorical variables and t-tests for continuous variables. The Kaplan–Meier method and the log-rank test were used to estimate survival. All statistical analyses were performed using R software (Version 3.6.2; http://www.Rproject.org). A two-sided P-value < 0.05 was considered to indicate statistical significance.
Results
Baseline Characteristics
In total, 197 eligible ARDS patients with integrated information were randomly stratified into two independent cohorts (training set, n = 133; validation set, n = 64). Patients' baseline clinical characteristics are shown in Table 1. A total of 123 male patients and 74 female patients were enrolled in this study. The average age of the patients was 73.94 ± 11.92 years. After 28 days of follow-up, 69 (35.0%) patients died during the entire study population.
Construction of the Model
A total of 13 parameters were used for LASSO regression, and seven parameters were selected as the optimal predictors by LASSO (Figures 1A,B). The seven retained variables were then used to construct the predictive model. The risk-score for each individual was calculated based on the model coefficients combined with the corresponding value of the identified seven clinical parameters. Thereafter, the patients were classified into low- and high-risk groups in both cohorts according to the median risk-score. Figures 1C,D show the risk-score distribution and the survival status of individual in the high- and low-risk cluster. The variables including Age, Alb, PLT, PaO2/FiO2, LDH, HRCT, and etiology were incorporated into the nomogram (Figure 2). To facilitate the clinical application of our findings, we developed a web-based calculator (https://huangl.shinyapps.io/ARDSprognosis/) to predict prognosis of ARDS patients according to the nomogram (Figures 3A,B). The estimated 28-day survival probabilities could be obtained by drawing a perpendicular line from the total point axis to the outcome axis.
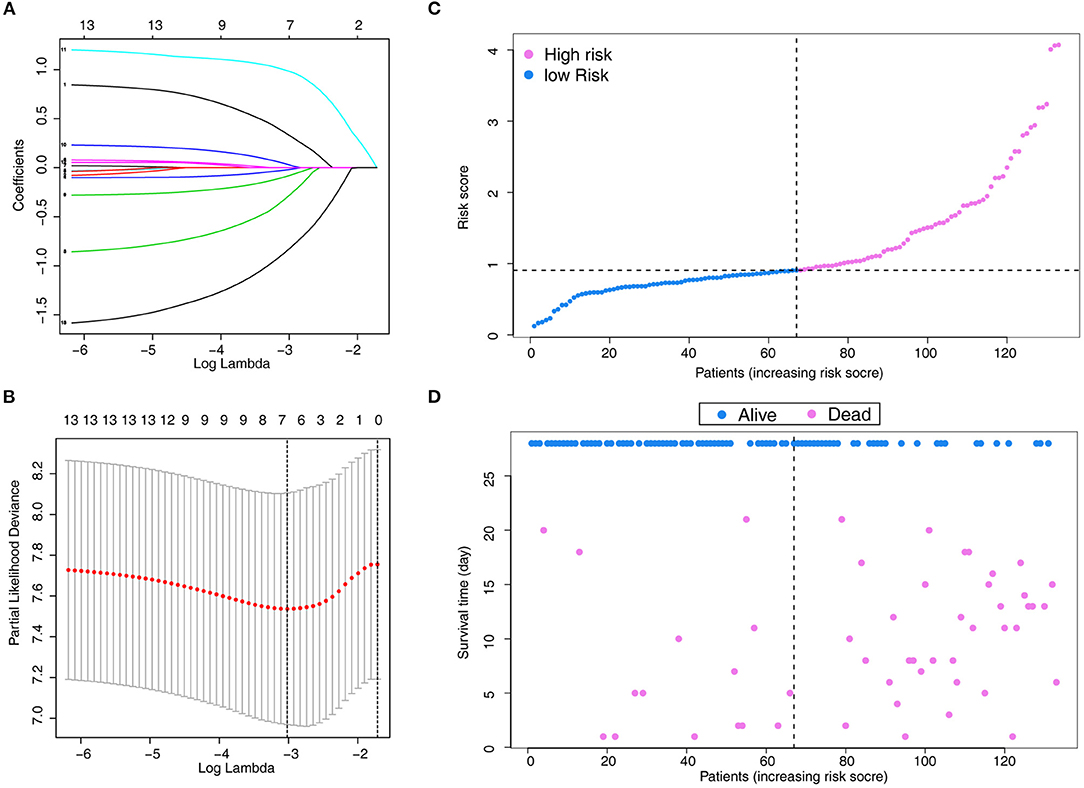
Figure 1. Parameter selection using LASSO regression. (A) LASSO coefficient profiles of the 13 features. A coefficient profile plot is produced against the log(λ) sequence. (B) Variables selected through LASSO with 10-fold cross-validation. (C) Distribution of the risk score. (D) Relationships between survival status and survival times of ARDS patients ranked by risk score. The black dotted line represents the optimum cut-off point dividing patients into low- and high-risk groups. LASSO, least absolute shrinkage and selection operator.
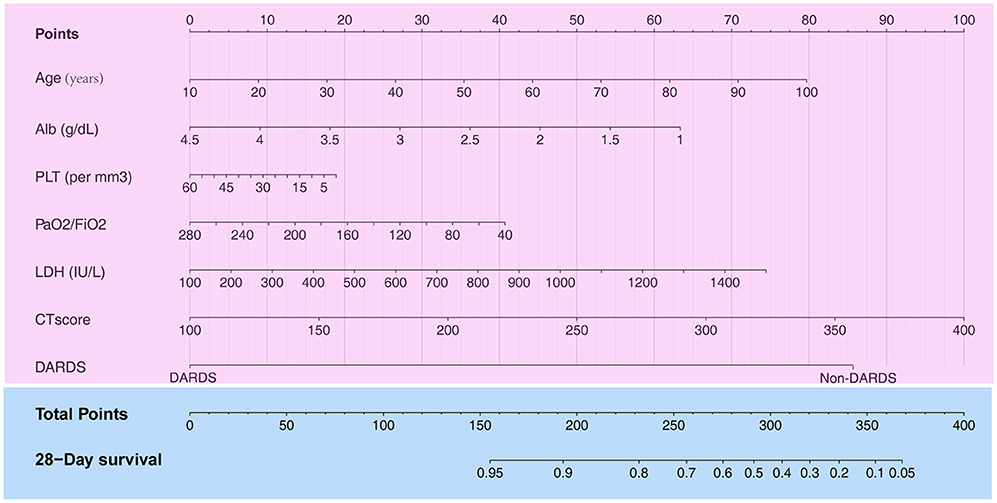
Figure 2. Construction of a nomogram with clinical indices to predict ARDS-related survival (based on the training set). The score for each value is assigned by drawing a line upward to the points line, and the sum of the seven scores is plotted on the Total points line. ARDS, acute respiratory distress syndrome.
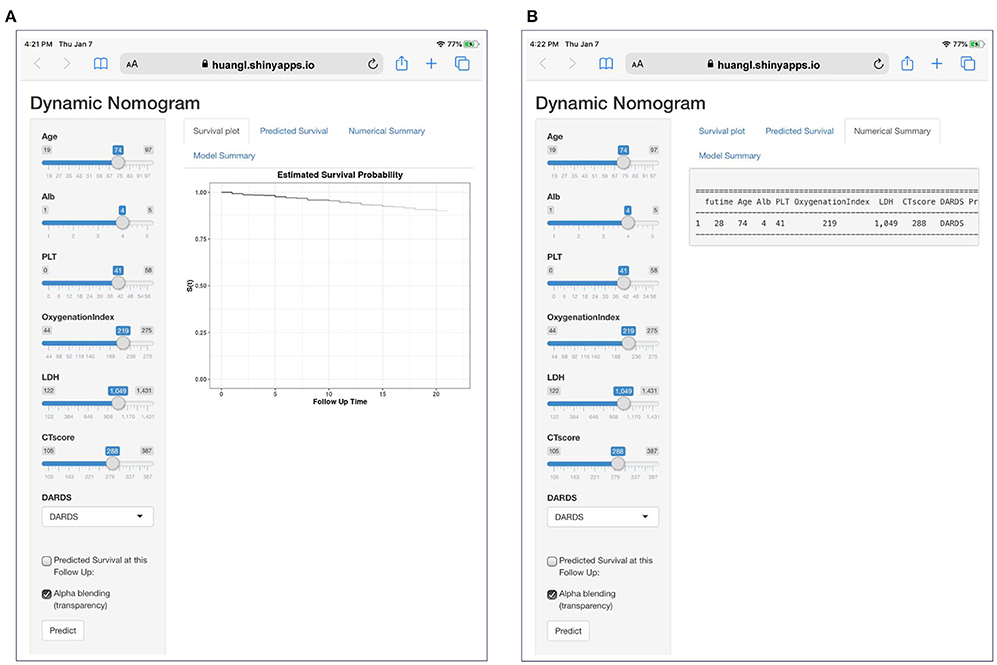
Figure 3. A dynamic web-based calculator to predict ARDS-related survival (https://huangl.shinyapps.io/ARDSprognosis/). (A) Web survival rate calculator. (B) 95% confidence interval of the web survival rate calculator.
Performance of the Model
The Kaplan–Meier survival curves revealed significantly poor overall survival in the high-risk group (p = 3.872e-04; Figure 4A). Thereafter, we performed ROC analysis to assess the discriminability of the model. The area under the ROC curve (AUC) indicative of the 28-day survival prediction was 0.75 (Figure 4B), which implied an efficacious performance of the model to predict prognosis. The calibration plots based on the training set showed that the model could accurately predict the 28-day survival (Figure 4C). The results of DCA also exhibited that the model could help clinicians to obtain maximum benefit when making clinical decisions (Figure 4D).
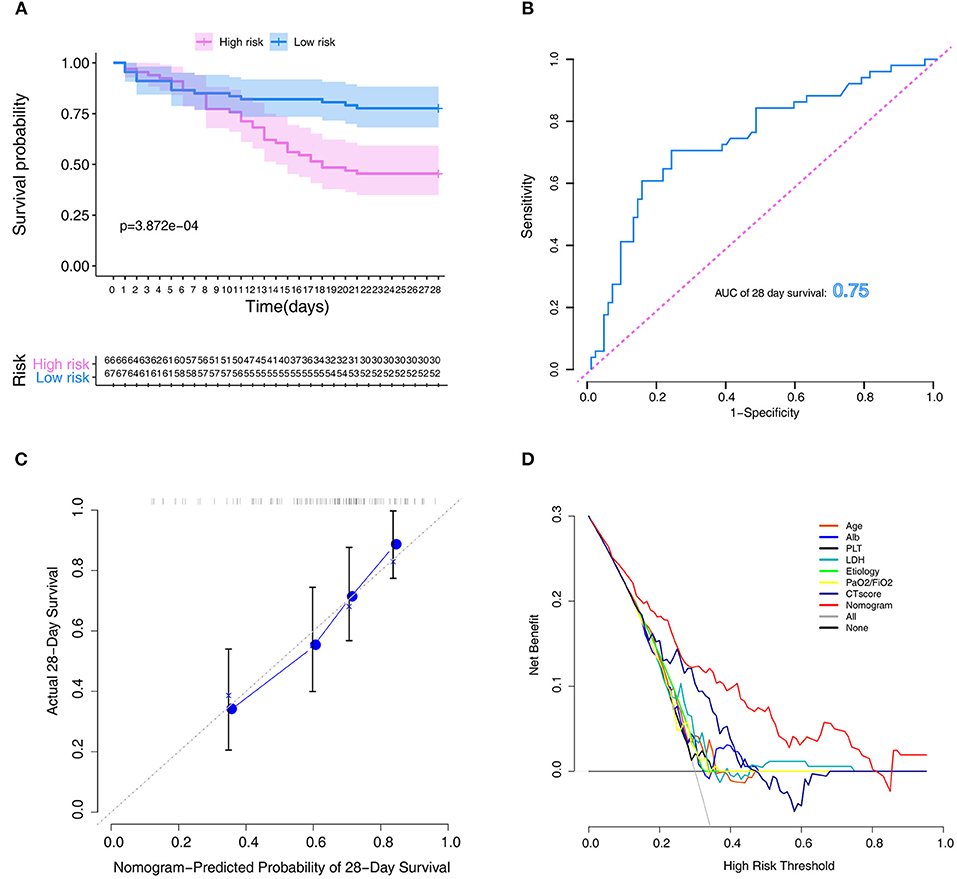
Figure 4. Assessment of the model in the training set. (A) Kaplan–Meier survival analysis between the high- and low-risk groups. (B) ROC curves of predictive models at 28 days. (C) Calibration plot for the training set that show the predicted and observed (with 95% confidence intervals) overall survival rates at 28 days. Model performance is shown by the plot, relative to the 45-degree line, which represents perfect prediction. (D) Decision curve of the model. The gray line represents the treat-all-patients scheme. The dotted line represents the treat-none scheme. The red line represents prediction nomogram scheme in training dataset. The X axis represents threshold probability. The Y axis represents net benefit. ROC, receiver operator characteristic.
To further study the predictive value of each parameter included in the model, we performed ROC analysis for each of them (Figures 5A–G). The AUC values of all parameters were lower than that of the complete nomogram model. These results demonstrated that the model had superior predictive performance and clinical value than any single factor.
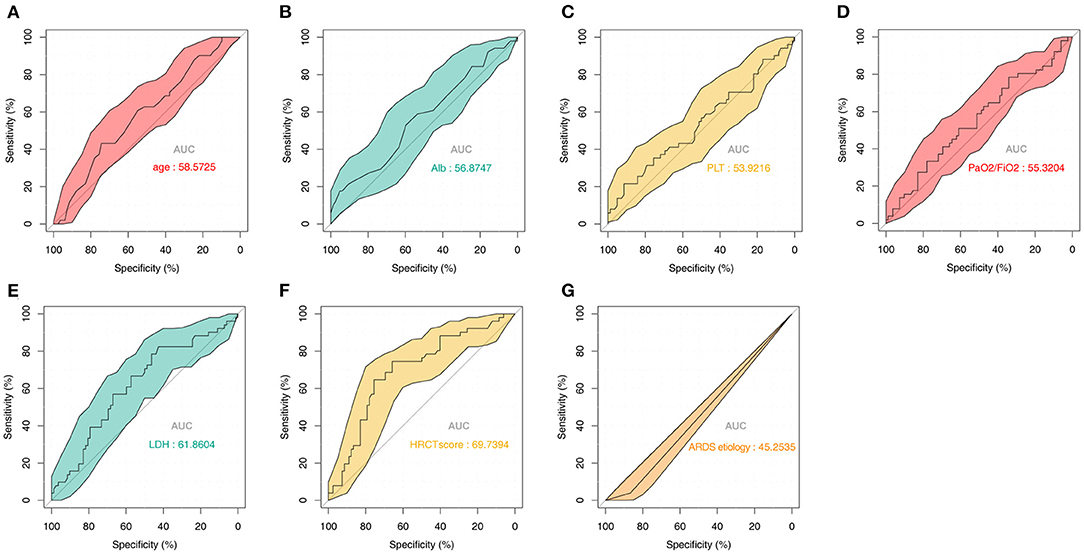
Figure 5. Predictive value of the seven parameters included in the model from the training set. ROC analysis of (A) Age, (B) Alb, (C) PLT, (D) PaO2/FiO2, (E) LDH, (F) HRCT score, and (G) ARDS etiology. ROC, receiver operator characteristic; Alb, albumin; PLT, platelet; LDH, lactate dehydrogenase; HRCT, high-resolution computed tomography; ARDS, acute respiratory distress syndrome.
Performance Validation of the Model
To verify the reliability of the constructed novel model, risk-scores were calculated in the validation set with the same formula that was used for calculating the risk-scores of patients in the training set. In the validation set, the distribution of risk-scores and the survival status (Figures 6A,B) had a trend similar to that in the training set between high- and low-risk groups. Also, survival analysis indicated that low-risk patients had significantly favor prognosis than high-risk patients (Figure 6C). ROC curves were used to assess the prognostic value of the risk-scores; the analysis results suggested that risk-scores could accurately predict the survival rate in patients (AUC = 0.776, Figure 6D). The calibration plot in the validation set also showed that the model could accurately predict the 28-day survival (Figure 6E).
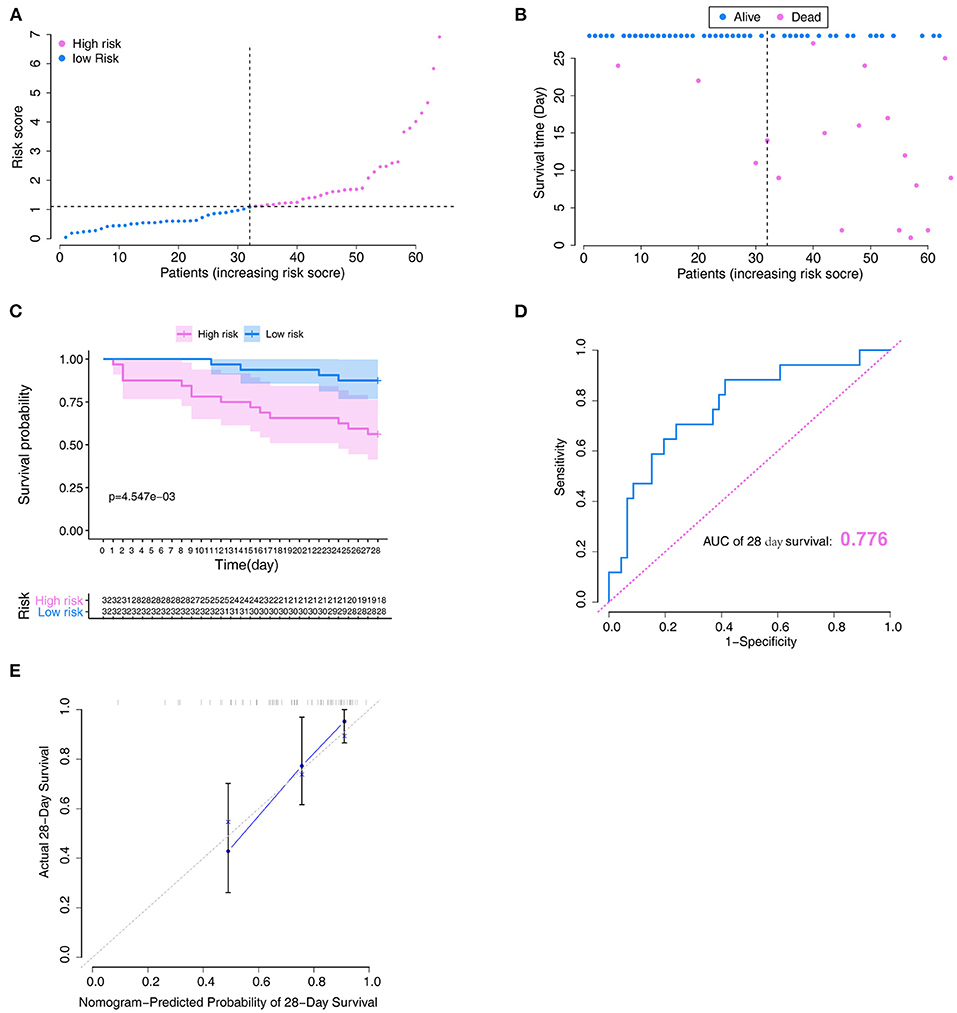
Figure 6. Verification of the model in the validation set. (A) Distribution of the risk score. (B) Relationships between survival status and survival times of ARDS patients ranked by risk score. The black dotted line represents the optimum cut-off point dividing patients into low- and high-risk groups. (C) Kaplan–Meier survival analysis between high- and low-risk groups. (D) ROC curves of predictive models at 28 days. (E) Calibration plot for the training set that show the predicted and observed (with 95% confidence intervals) overall survival rates at 28 days. Model performance is shown by the plot, relative to the 45-degree line, which represents perfect prediction. ROC, receiver operator characteristic.
Discussion
ARDS, one of the main critical diseases encountered in intensive care units, is a clinically and pathophysiologically complex syndrome of acute lung inflammation. Despite substantial progress in respiratory support strategies for critically ill patients, including the incorporation of a small tidal volume (21), high positive end-expiratory pressure (22), prone position ventilation (23), lung recruitment (24), use of neuromuscular blockers (25), high-frequency oscillatory ventilation (26, 27), and extracorporeal membrane oxygenation (28, 29), the mortality rate among patients with ARDS remains unacceptably high (30). However, to our knowledge, no study has previously developed a nomogram to predict the prognosis of patients with ARDS.
Herein, we first developed a nomogram using simple and easily available variables to evaluate the 28-day survival probabilities of ARDS patients whose information were extracted from an online database. Thereafter, we tested the performance of the nomogram in training and validation cohorts. Seven risk factors were identified in this model: age, Alb, PLT, PaO2/FiO2, LDH, CT score, and ARDS etiologies. Additionally, our results showed that PaO2/FiO2, and CT score could, albeit less accurately, predict the survival probability of ARDS patients compared to our novel model. These results suggest that the nomogram could be used as a cost-effective tool to predict the prognosis of ARDS and assist with clinical decision-making.
In 2012, the Berlin ARDS Society defined the severity of ARDS according to the oxygenation index (5). The oxygenation index (PaO2/FiO2) was helpful to categorize ARDS patients with different severity, and the mortality was reported to be higher in more severe stages of ARDS (mild, moderate, or severe) (5, 31). However, these severity categories have a low-to-moderate prognostic value to predict respiratory failure (32). Kamo and colleagues (33) reported that the severity stratification of the Berlin ARDS criteria may have a low capacity to differentiate between mild and moderate ARDS. In this study, the results of ROC curve analysis also indicated that the oxygenation index had low prognostic power (AUC, 55.3204%), which was consistent with previous studies.
CT or other lung imaging techniques have been used as diagnostic tools to optimize lung assessment and ventilator management in patients with ARDS; however, it is still controversial whether CT findings can predict ARDS outcomes (34–36). HRCT scores have been reported to correlate with the pathological stage of diffuse alveolar damage (37). Ichikado and colleagues (38) noted that HRCT score was one of the independent predictors of death and ventilator dependency in ARDS patients. Simultaneously, HRCT score was also found to be associated with multiorgan failure and ventilator-associated complications (38). In the present study, to increase model accuracy, HRCT score was incorporated into the nomogram. To evaluate the performance of HRCT score as a prognostic biomarker for the survival of ARDS patients, we performed ROC analysis. Our results showed that the model fit was significantly better than that of the one-factor HRCT model.
APACHE II score can be used as indicators to evaluate the prognosis among critically ill patients; it has been used worldwide to measure ICU performance (39). As APACHE II score included age and other factors in the calculation process, and repeated operations would be generated if the model was built again, APACHE II was not included in the LASSO regression analysis. The APACHE II score is calculated based on acute physiological parameters and chronic health conditions, all of which have significant effects on the predictive prognosis of ICU patients (40). Hwang and colleagues (41) revealed that APACHE II score was a mortality predictor for ARDS patients, but that the accuracy was not high. Lesur and colleagues (42) reported that APACHE II score may be less predictive value when applied for ARDS patients, and that in those patients, it might be less accurate than other indicators, such as age. In the present study, it was also found that the prediction accuracy of this model was better than APACHE II score when compared to the results of precious study (AUC = 0.623) (41).
Certain drugs have also been reported to have the potential to cause ARDS. It has been proved that molecular targeted therapy, such as methotrexate and certain herbal medicines, can cause severe respiratory failure or ARDS (43–45). However, only few studies have focused on the prognostic role of different etiologies of ARDS. In the present study, our results indicated that there is a lower risk of death if ARDS is caused by drugs. However, these discrepancies may be partly related to differences in the dose and duration of drug treatments.
Our study has some limitations. Firstly, the model was developed mainly based on the seven variables. As these factors were unstable throughout the whole follow-up period, which may partly influence the precision of the model. Secondly, only 197 patients were included in this study; further studies with bigger sample sizes are needed. Thirdly, the lack of external validation may limit the extrapolation of the nomogram.
To summarize, we identified eight variables and developed a novel model to predict prognosis in patients with ARDS. These results may help to further improve clinical decision-making and individualized treatment of ARDS patients. Also, this model could distinguish patients with high-risk of ARDS, and further help to perform a careful follow-up among those patients.
Data Availability Statement
The raw data supporting the conclusions of this article will be made available by the authors, without undue reservation.
Ethics Statement
Ethical review and approval was not required for the study on human participants in accordance with the local legislation and institutional requirements. Written informed consent for participation was not required for this study in accordance with the national legislation and the institutional requirements.
Author Contributions
YL and LH designed the study. YL and JL performed the data analysis statistical analysis. YL prepared the manuscript. LH contributed funding for the project. All authors read and approved the final manuscript.
Funding
This study was supported by Key R & D Program of Jiangxi Province (No. 20202BBG73011).
Conflict of Interest
The authors declare that the research was conducted in the absence of any commercial or financial relationships that could be construed as a potential conflict of interest.
Acknowledgments
We thank the Anan et al. for-data collection and sharing.
Abbreviations
Alb, albumin; PLT, platelet count; WBC, white cell count; CRP, C reactive protein; SOFA, sequential organ failure assessment; APACHE, acute physiology and chronic health evaluation; LDH, lactate dehydrogenase; HRCT, high-resolution computed tomography; DARDS, drug-associated ARDS.
References
1. Urner M, Jüni P, Hansen B, Wettstein MS, Ferguson ND, Fan E. Time-varying intensity of mechanical ventilation and mortality in patients with acute respiratory failure: a registry-based, prospective cohort study. Lancet Respir Med. (2020) 8:905–13. doi: 10.1016/S2213-2600(20)30325-8
2. Suresh R, Kupfer Y, Tessler S. Acute respiratory distress syndrome. N Engl J Med. (2000) 343:660–1. doi: 10.1056/NEJM200008313430914
3. Ware LB, Matthay MA. The acute respiratory distress syndrome. N Engl J Med. (2000) 342:1334–49. doi: 10.1056/NEJM200005043421806
4. Kim JS, Kim YJ, Kim M, Ryoo SM, Sohn CH, Ahn S, et al. Impact of lung compliance on neurological outcome in patients with acute respiratory distress syndrome following out-of-hospital cardiac arrest. J Clin Med. (2020) 9:527. doi: 10.3390/jcm9020527
5. Ranieri VM, Rubenfeld GD, Thompson BT, Ferguson ND, Caldwell E, Fan E, et al. Acute respiratory distress syndrome: the Berlin Definition. JAMA. (2012) 307:2526–33. doi: 10.1001/jama.2012.5669
6. Terpstra ML, Aman J, van Nieuw Amerongen GP, Groeneveld AB. Plasma biomarkers for acute respiratory distress syndrome: a systematic review and meta-analysis*. Crit Care Med. (2014) 42:691–700. doi: 10.1097/01.ccm.0000435669.60811.24
7. Yehya N, Thomas NJ, Meyer NJ, Christie JD, Berg RA, Margulies SS. Circulating markers of endothelial and alveolar epithelial dysfunction are associated with mortality in pediatric acute respiratory distress syndrome. Intensive Care Med. (2016) 42:1137–45. doi: 10.1007/s00134-016-4352-1
8. Levitt JE, Gould MK, Ware LB, Matthay MA. The pathogenetic and prognostic value of biologic markers in acute lung injury. J Intensive Care Med. (2009) 24:151–67. doi: 10.1177/0885066609332603
9. Jones TK, Feng R, Kerchberger VE, Reilly JP, Anderson BJ, Shashaty MGS, et al. Plasma sRAGE acts as a genetically regulated causal intermediate in sepsis-associated acute respiratory distress syndrome. Am J Respir Crit Care Med. (2020) 201:47–56. doi: 10.1164/rccm.201810-2033OC
10. Graesslin O, Abdulkarim BS, Coutant C, Huguet F, Gabos Z, Hsu L, et al. Nomogram to predict subsequent brain metastasis in patients with metastatic breast cancer. J Clin Oncol. (2010) 28:2032–7. doi: 10.1200/JCO.2009.24.6314
11. Wang J, He L, Tang Y, Li D, Yang Y, Zeng Z. Development and validation of a nomogram with an epigenetic signature for predicting survival in patients with lung adenocarcinoma. Aging. (2020) 12:23200–16. doi: 10.18632/aging.104090
12. Anan K, Ichikado K, Kawamura K, Johkoh T, Fujimoto K, Suga M. Clinical characteristics and prognosis of drug-associated acute respiratory distress syndrome compared with non-drug-associated acute respiratory distress syndrome: a single-centre retrospective study in Japan. BMJ Open. (2017) 7:e015330. doi: 10.1136/bmjopen-2016-015330
13. Friedman J, Hastie T, Tibshirani R. Regularization paths for generalized linear models via coordinate descent. J Stat Softw. (2010) 33:1–22. doi: 10.18637/jss.v033.i01
14. Simon N, Friedman J, Hastie T, Tibshirani R. Regularization paths for cox's proportional hazards model via coordinate descent. J Stat Softw. (2011) 39:1–13. doi: 10.18637/jss.v039.i05
17. R Core Team. Foreign: Read Data Stored by ‘Minitab’, ‘S’,‘SAS’, ‘SPSS’, ‘Stata’, ‘Systat’, ‘Weka’, ‘dBase’, R Package Version 0.8-76. R Core Team (2020).
18. Schauberger P, Walker A. Openxlsx: Read, Write and Edit xlsx Files. R Package Version 4.1.5. (2020).
19. Jalali A, Roshan D, Alvarez-Iglesias A, Newell J. DynNom: Visualising Statistical Models Using Dynamic Nomograms. R Package Version 5.0.1. (2019). doi: 10.1371/journal.pone.0225253
20. Kamarudin AN, Cox T, Kolamunnage-Dona R. Time-dependent ROC curve analysis inmedical research: current methods and applications. BMC Med Res Methodol. (2017) 17:53. doi: 10.1186/s12874-017-0332-6
21. Brower RG, Matthay MA, Morris A, Schoenfeld D, Thompson BT, Wheeler A. Ventilation with lower tidal volumes as compared with traditional tidal volumes for acute lung injury and the acute respiratory distress syndrome. N Engl J Med. (2000) 342:1301–8. doi: 10.1056/NEJM200005043421801
22. Brower RG, Lanken PN, MacIntyre N, Matthay MA, Morris A, Ancukiewicz M, et al. Higher versus lower positive end-expiratory pressures in patients with the acute respiratory distress syndrome. N Engl J Med. (2004) 351:327–36. doi: 10.1056/NEJMoa032193
23. Guérin C, Reignier J, Richard JC, Beuret P, Gacouin A, Boulain T, et al. Prone positioning in severe acute respiratory distress syndrome. N Engl J Med. (2013) 368:2159–68. doi: 10.1056/NEJMoa1214103
24. Meade MO, Cook DJ, Guyatt GH, Slutsky AS, Arabi YM, Cooper DJ, et al. Ventilation strategy using low tidal volumes, recruitment maneuvers, and high positive end-expiratory pressure for acute lung injury and acute respiratory distress syndrome: a randomized controlled trial. JAMA. (2008) 299:637–45. doi: 10.1001/jama.299.6.637
25. Papazian L, Forel JM, Gacouin A, Penot-Ragon C, Perrin G, Loundou A, et al. Neuromuscular blockers in early acute respiratory distress syndrome. N Engl J Med. (2010) 363:1107–16. doi: 10.1056/NEJMoa1005372
26. Young NH, Andrews PJ. High-frequency oscillation as a rescue strategy for brain-injured adult patients with acute lung injury and acute respiratory distress syndrome. Neurocrit Care. (2011) 15:623–33. doi: 10.1007/s12028-011-9550-7
27. Ferguson ND, Cook DJ, Guyatt GH, Mehta S, Hand L, Austin P, et al. High-frequency oscillation in early acute respiratory distress syndrome. N Engl J Med. (2013) 368:795–805. doi: 10.1056/NEJMoa1215554
28. Zampieri FG, Mendes PV, Ranzani OT, Taniguchi LU, Pontes Azevedo LC, Vieira Costa EL, et al. Extracorporeal membrane oxygenation for severe respiratory failure in adult patients: a systematic review and meta-analysis of current evidence. J Crit Care. (2013) 28:998–1005. doi: 10.1016/j.jcrc.2013.07.047
29. Peek GJ, Clemens F, Elbourne D, Firmin R, Hardy P, Hibbert C, et al. CESAR: conventional ventilatory support vs extracorporeal membrane oxygenation for severe adult respiratory failure. BMC Health Serv Res. (2006) 6:163. doi: 10.1186/1472-6963-6-163
30. Chen X, Wu S, Tang L, Ma L, Wang F, Feng H, et al. Mesenchymal stem cells overexpressing heme oxygenase-1 ameliorate lipopolysaccharide-induced acute lung injury in rats. J Cell Physiol. (2019) 234:7301–19. doi: 10.1002/jcp.27488
31. Ferguson ND, Fan E, Camporota L, Antonelli M, Anzueto A, Beale R, et al. The Berlin definition of ARDS: an expanded rationale, justification, and supplementary material. Intensive Care Med. (2012) 38:1573–82. doi: 10.1007/s00134-012-2682-1
32. Hernu R, Wallet F, Thiollière F, Martin O, Richard JC, Schmitt Z, et al. An attempt to validate the modification of the American-European consensus definition of acute lung injury/acute respiratory distress syndrome by the Berlin definition in a University hospital. Intensive Care Med. (2013) 39:2161–70. doi: 10.1007/s00134-013-3122-6
33. Kamo T, Tasaka S, Suzuki T, Asakura T, Suzuki S, Yagi K, et al. Prognostic values of the Berlin definition criteria, blood lactate level, and fibroproliferative changes on high-resolution computed tomography in ARDS patients. BMC Pulm Med. (2019) 19:37. doi: 10.1186/s12890-019-0803-0
34. Chiumello D, Taccone P, Berto V, Marino A, Migliara G, Lazzerini M, et al. Long-term outcomes in survivors of acute respiratory distress syndrome ventilated in supine or prone position. Intensive Care Med. (2012) 38:221–9. doi: 10.1007/s00134-011-2445-4
35. Chung JH, Kradin RL, Greene RE, Shepard JA, Digumarthy SR. CT predictors of mortality in pathology confirmed ARDS. Eur Radiol. (2011) 21:730–7. doi: 10.1007/s00330-010-1979-0
36. Chiumello D, Froio S, Bouhemad B, Camporota L, Coppola S. Clinical review: lung imaging in acute respiratory distress syndrome patients—an update. Crit Care. (2013) 17:243. doi: 10.1186/cc13114
37. Anan K, Kawamura K, Suga M, Ichikado K. Clinical differences between pulmonary and extrapulmonary acute respiratory distress syndrome: a retrospective cohort study of prospectively collected data in Japan. J Thorac Dis. (2018) 10:5796–803. doi: 10.21037/jtd.2018.09.73
38. Ichikado K, Muranaka H, Gushima Y, Kotani T, Nader HM, Fujimoto K, et al. Fibroproliferative changes on high-resolution CT in the acute respiratory distress syndrome predict mortality and ventilator dependency: a prospective observational cohort study. BMJ Open. (2012) 2:e000545. doi: 10.1136/bmjopen-2011-000545
39. Giamarellos-Bourboulis EJ, Norrby-Teglund A, Mylona V, Savva A, Tsangaris I, Dimopoulou I, et al. Risk assessment in sepsis: a new prognostication rule by APACHE II score and serum soluble urokinase plasminogen activator receptor. Crit Care. (2012) 16:R149. doi: 10.1186/cc11463
40. Hsu YT, He YT, Ting CK, Tsou MY, Tang GJ, Pu C. Administrative and claims data help predict patient mortality in intensive care units by logistic regression: a nationwide database study. Biomed Res Int. (2020) 2020:9076739. doi: 10.1155/2020/9076739
41. Hwang H, Choi SM, Lee J, Park YS, Lee CH, Yoo CG, et al. Validation of age, PaO(2)/FlO(2) and plateau pressure score in Korean patients with acute respiratory distress syndrome: a retrospective cohort study. Respir Res. (2020) 21:94. doi: 10.1186/s12931-020-01357-5
42. Lesur O, Langevin S, Berthiaume Y, Légaré M, Skrobik Y, Bellemare JF, et al. Outcome value of Clara cell protein in serum of patients with acute respiratory distress syndrome. Intensive Care Med. (2006) 32:1167–74. doi: 10.1007/s00134-006-0235-1
43. Wolkove N, Baltzan M. Amiodarone pulmonary toxicity. Can Respir J. (2009) 16:43–8. doi: 10.1155/2009/282540
44. Imokawa S, Colby TV, Leslie KO, Helmers RA. Methotrexate pneumonitis: review of the literature and histopathological findings in nine patients. Eur Respir J. (2000) 15:373–81. doi: 10.1034/j.1399-3003.2000.15b25.x
Keywords: acute respiratory distress syndrome, LASSO regression, nomogram, model, survival
Citation: Liu Y, Liu J and Huang L (2021) A Simple-to-Use Web-Based Calculator for Survival Prediction in Acute Respiratory Distress Syndrome. Front. Med. 8:604694. doi: 10.3389/fmed.2021.604694
Received: 10 September 2020; Accepted: 29 January 2021;
Published: 16 February 2021.
Edited by:
Rifat Hamoudi, University of Sharjah, United Arab EmiratesReviewed by:
Rui-Qing Zhai, Harbin Medical University, ChinaTingfan Wu, GE Healthcare (China), China
Nao Yan, Wuhan University, China
Copyright © 2021 Liu, Liu and Huang. This is an open-access article distributed under the terms of the Creative Commons Attribution License (CC BY). The use, distribution or reproduction in other forums is permitted, provided the original author(s) and the copyright owner(s) are credited and that the original publication in this journal is cited, in accordance with accepted academic practice. No use, distribution or reproduction is permitted which does not comply with these terms.
*Correspondence: Liang Huang, huangliang6312@sina.com
†These authors have contributed equally to this work