- 1Department of Respiratory Medicine, University General Hospital of Patras, Patras, Greece
- 2Department of Infectious Diseases-COVID-19 Unit, Laiko General Hospital, Athens, Greece
- 3Department of Respiratory Medicine, Corfu General Hospital, Corfu, Greece
- 4Laboratory of Molecular and Cellular Pneumonology, Department of Thoracic Medicine, Medical School, University of Crete, Heraklion, Greece
- 55th Department of Respiratory Medicine, Hospital for Thoracic Diseases, ‘SOTIRIA’, Athens, Greece
- 6Medical School, National and Kapodistrian University of Athens, Zografou, Greece
Introduction: Post-acute sequelae of COVID-19 seem to be an emerging global crisis. Machine learning radiographic models have great potential for meticulous evaluation of post-COVID-19 interstitial lung disease (ILD).
Methods: In this multicenter, retrospective study, we included consecutive patients that had been evaluated 3 months following severe acute respiratory syndrome coronavirus 2 infection between 01/02/2021 and 12/5/2022. High-resolution computed tomography was evaluated through Imbio Lung Texture Analysis 2.1.
Results: Two hundred thirty-two (n = 232) patients were analyzed. FVC% predicted was ≥80, between 60 and 79 and <60 in 74.2% (n = 172), 21.1% (n = 49), and 4.7% (n = 11) of the cohort, respectively. DLCO% predicted was ≥80, between 60 and 79 and <60 in 69.4% (n = 161), 15.5% (n = 36), and 15.1% (n = 35), respectively. Extent of ground glass opacities was ≥30% in 4.3% of patients (n = 10), between 5 and 29% in 48.7% of patients (n = 113) and <5% in 47.0% of patients (n = 109). The extent of reticulation was ≥30%, 5–29% and <5% in 1.3% (n = 3), 24.1% (n = 56), and 74.6% (n = 173) of the cohort, respectively. Patients (n = 13, 5.6%) with fibrotic lung disease and persistent functional impairment at the 6-month follow-up received antifibrotics and presented with an absolute change of +10.3 (p = 0.01) and +14.6 (p = 0.01) in FVC% predicted at 3 and 6 months after the initiation of antifibrotic.
Conclusion: Post-COVID-19-ILD represents an emerging entity. A substantial minority of patients presents with fibrotic lung disease and might experience benefit from antifibrotic initiation at the time point that fibrotic-like changes are “immature.” Machine learning radiographic models could be of major significance for accurate radiographic evaluation and subsequently for the guidance of therapeutic approaches.
Introduction
The global impact of the ongoing coronavirus disease 2019 (COVID-19) pandemic has been unparalleled. The disease course is variable, manifesting from asymptomatic to fatal forms, and long-term complications could have further devastating effects (1–3). Emerging evidence indicate that a substantial proportion of infected individuals may experience prolonged symptoms lasting for more than 6 months (4–6).
The National Institute for Health and Care Excellence (NICE) and the Centers for Disease Control and prevention (CDC) define long COVID as symptoms and sequalae that persist or develop after the 4-week acute phase of COVID-19 and that cannot be explained by an alternative diagnosis (7, 8). This term includes ongoing symptomatic COVID-19, which encompasses manifestations from 4 to 12 weeks post-infection, and post-COVID-19 syndrome, which refers to symptoms/clinical signs beyond 12 weeks following infection (8). The pathogenic process of this recently reported condition has not been elucidated; yet potential contributing mechanisms include viral toxicity inducing ACE2 downregulation (due to cellular internalization) thus leading to a pro-fibrotic microenvironment as well as immune dysregulation with emergence of autoimmunity phenomena leading to persistent inflammatory damage (9). Post-COVID clinical manifestations are multisystemic and the lasting symptom burden may lead to severe functional limitation and decrement in quality of life (9). Pulmonary sequalae range in a wide clinical, physiologic, and imaging spectrum and have been related to the severity of acute illness (9–11). The most commonly reported pulmonary manifestations are diffusion capacity decline, restrictive pattern with regards to functional impairment and ground glass opacities with or without fibrotic lesions in the context of radiographic signs (12, 13).
Meticulous radiographic evaluation might have a cardinal role for the guidance of therapeutic approaches in this new entity with unknown long-term effects. Toward this direction, we performed patients’ radiographic evaluation through a validated machine learning software system, denominated Imbio Lung Texture Analysis (14). Machine learning represents a subgroup of artificial intelligence. In this setting, computers extract patterns from appropriately classified input data and accordingly generate labels for new, unknown data (15). Machine learning and its subset named deep learning have demonstrated great potential in multiple medical imaging classification tasks including prediction of mortality in Idiopathic Pulmonary Fibrosis (16–20).
This multicenter study aimed to present functional and radiographic features of patients with post-COVID-19-interstitial lung disease (ILD), in a quantitative, precise and unbiased fashion using cutting-edge technology, highlighting the importance of screening patients with long-COVID-19 clinical, and functional and radiological impairment.
Materials and methods
Trial design and oversight
In this multicenter, investigator-initiated, retrospective, observational cohort study, we included consecutive patients that had been evaluated 3 months following severe acute respiratory syndrome coronavirus 2 (SARS-CoV-2) infection between 01/02/2021 and 12/05/2022. Trial sites were five referral ILD centers in Greece. Patients with positive polymerase chain reaction test for SARS-CoV-2, treated both in outpatient and inpatient setting were included. Patients with less than 18 years of age were excluded from the analysis.
We recorded PaO2/FiO2 during hospitalization, demographics, comorbidities, as well as forced vital capacity (FVC), forced expiratory volume in 1 s (FEV1), diffusing capacity of the lung for carbon monoxide (DLCO) and High-Resolution Computed Tomography (HRCT) findings 3 months following COVID-19 infection.
High-Resolution Computed Tomography scans were evaluated through the validated machine learning software, named Imbio Lung Texture Analysis version 2.1 (14). Imbio Lung Texture Analysis had the following technical requirements for HRCT analysis: Minimal movement and acceptable position, slice thickness <2.0 mm, revolution time <1 s, pixel spacing <2.0 mm, and slice spacing <2.0 mm. Subsequently, Imbio Lung Texture Analysis version 2.1 provided a report with% of each lobe and % of lung as total that was characterized as: Normal, ground glass, reticular, honeycombing or hyperlucent. A succinct rating scale for % disease extent was applied, providing 1% step evaluation for disease extent.
The trial was conducted in accordance with the International Conference on Harmonization E6 guidelines for Good Clinical Practice, the Declaration of Helsinki and the local regulations. Management was based on a common algorithm. Radiographic findings of the patients were initially meticulously evaluated and split into two groups: (1) Fibrotic-like lesions and (2) inflammatory abnormalities only. Subsequently, patients were further divided based on their functional status. Retrospective data collection and analysis was approved by our institutional review board (protocol number: 16574/29-6-2022).
Outcome measures
Outcome measures included: (1) The frequency of functional impairment as indicated by decline in FVC% predicted, FEV1% predicted or DLCO% predicted, (2) the frequency of specific radiographic findings following Imbio Lung Texture Analysis, and (3) the effectiveness of antifibrotics as indicated by change in FVC% predicted, DLCO% predicted at 3, 6, and 12 months following treatment initiation and changes in HRCT.
Statistical analysis
Continuous data were reported as mean ± standard deviation (SD) or medians with 95% Confidence Interval (95% CI) following Kolmogorov–Smirnov test for normality. Frequency tables and graphs were drawn. ANOVA repeated measures was used to investigate differences in FVC% predicted and DLCO% predicted at different time points. P-values < 0.05 were considered statistically significant.
Results
Baseline characteristics
Two hundred thirty-two (n = 232) patients were included in the analysis. Baseline characteristics are summarized in Table 1. Median age (95% CI) was 61.0 (58.0–63.0) years. Most patients were male (n = 160, 68.9%), while the most common comorbid condition was arterial hypertension (n = 88, 37.9%). Six patients (2.6%) had been vaccinated against COVID-19 prior hospitalization. One hundred seventy-eight (n = 178, 76.7%) patients reported history of hospitalization for COVID-19 and 54 (23.3%) patients were treated as outpatients. With regards to hospitalized patients, median value of the worst PaO2/FiO2 (95% CI) during hospitalization was 160.0 (130.2–180.0).
Three-month follow-up
Functional and radiographic features at the 3-month follow-up are summarized in Table 2. FVC% predicted was ≥80, between 60 and 79 and <60 in 74.2% (n = 172), 21.1% (n = 49), and 4.7% (n = 11) of the cohort, respectively (Figure 1A). FEV1% predicted was ≥80 in 78.5% (n = 182), 60–79 in 16.8% (n = 39) and <60 in 4.7% (n = 11) of patients (Figure 1B). DLCO% predicted was ≥80, between 60 and 79 and <60 in 69.4% (n = 161), 15.5% (n = 36), and 15.1% (n = 35), respectively (Figure 1C).
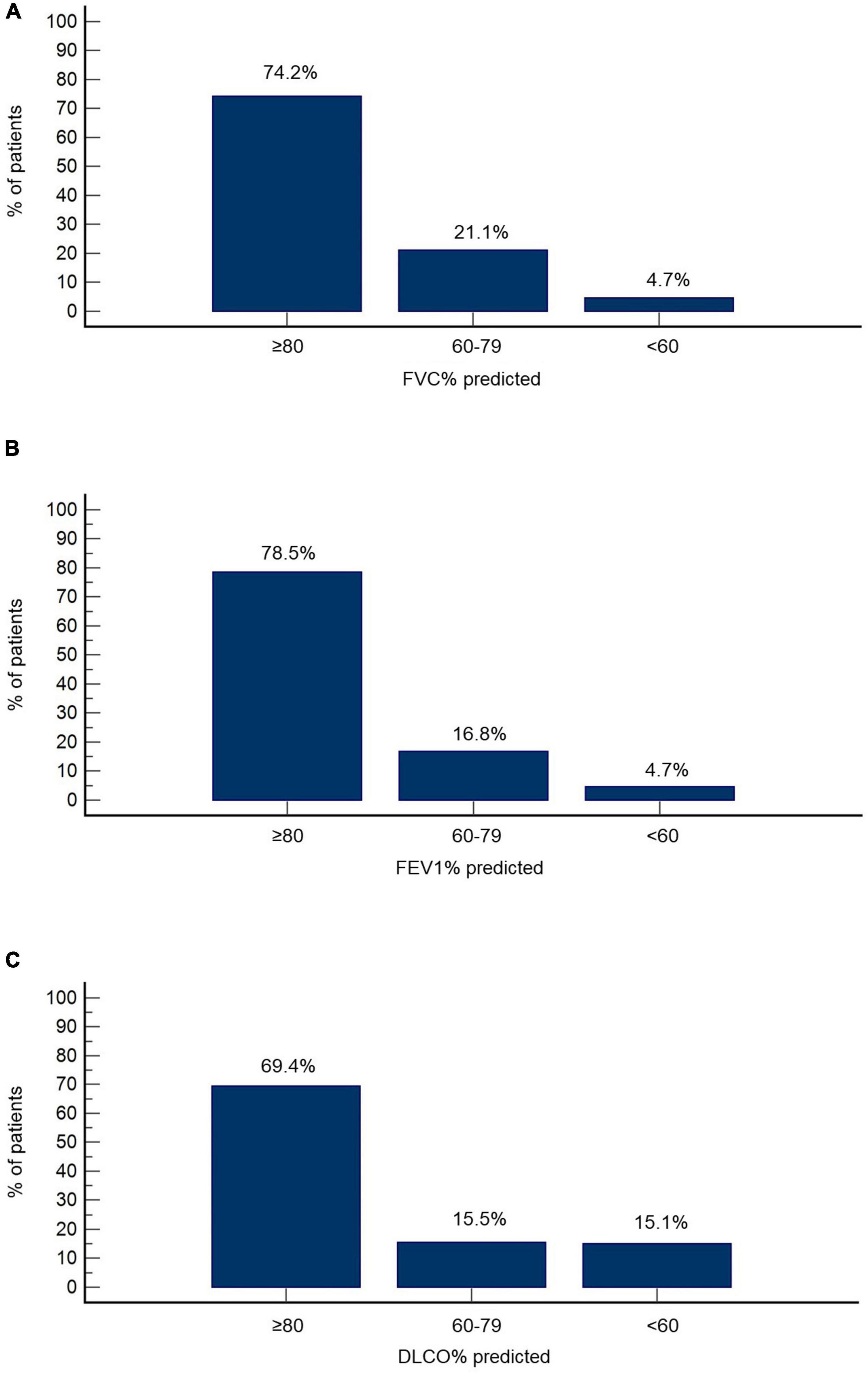
Figure 1. Functional features at the 3-month follow-up. Percentage of patients with reduced FVC% predicted (A), FEV1% predicted (B), and DLCO% predicted (C) is presented.
Imbio Lung Texture Analysis 2.1 demonstrated extent of ground glass opacities ≥30% in 4.3% of patients (n = 10), extent between 5 and 29% in 48.7% of patients (n = 113) and <5% in 47.0% of patients (n = 109) (Figure 2A). Accordingly, the extent of reticulation was ≥30, 5–29, and <5% in 1.3% (n = 3), 24.1% (n = 56), and 74.6% (n = 173) of the cohort, respectively (Figure 2B). Honeycombing was identified in 14 patients (6.0%). Representative images from Imbio Lung Texture Analysis 2.1 are presented in Figure 3.
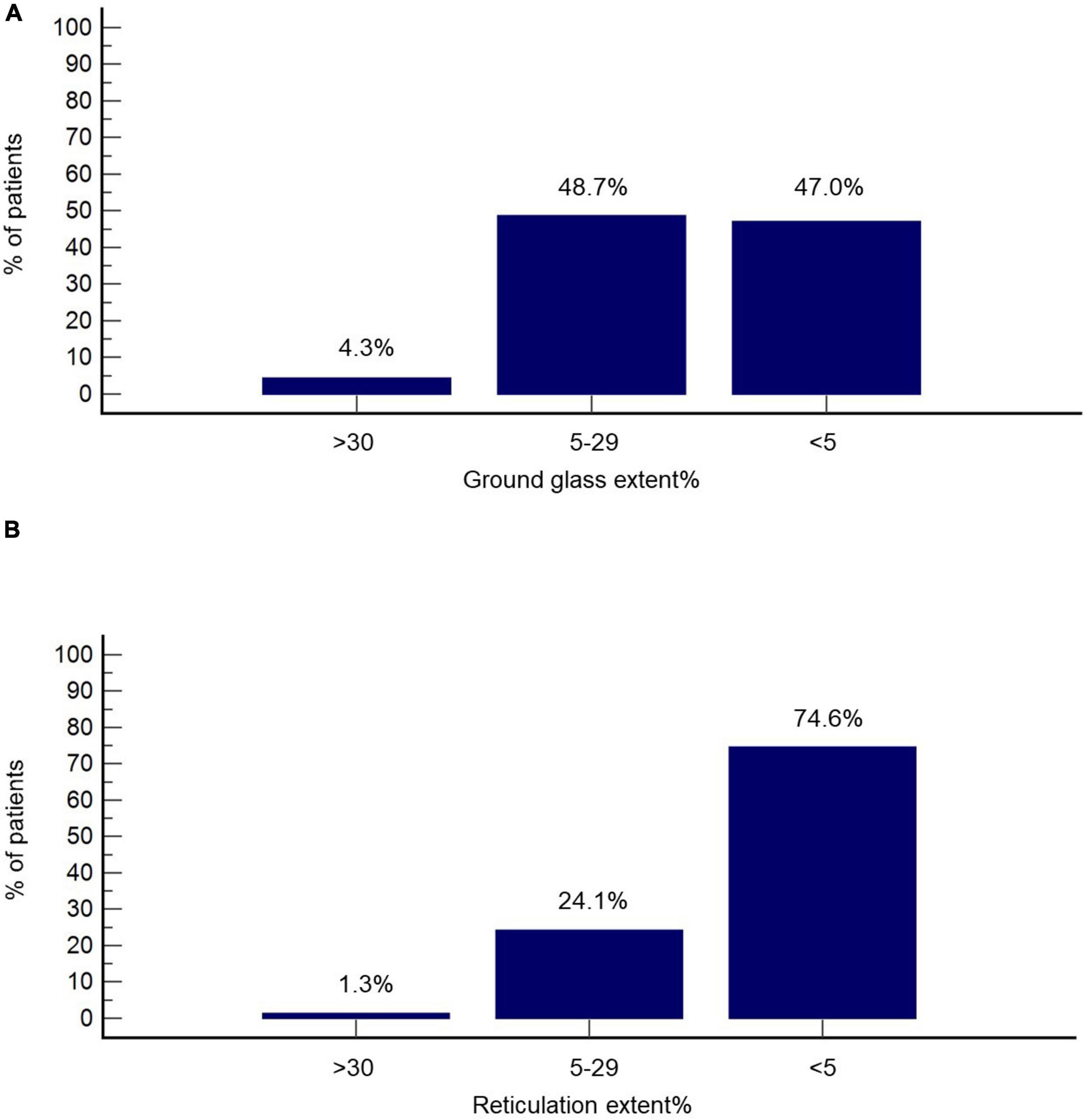
Figure 2. Radiographic features at the 3-month follow-up based on Imbio Lung Texture Analysis 2.1. Percentage of patients with% extent ground glass (A) and % extent reticulation (B) above 30, between 5 and 29, as well as below 5 is presented.
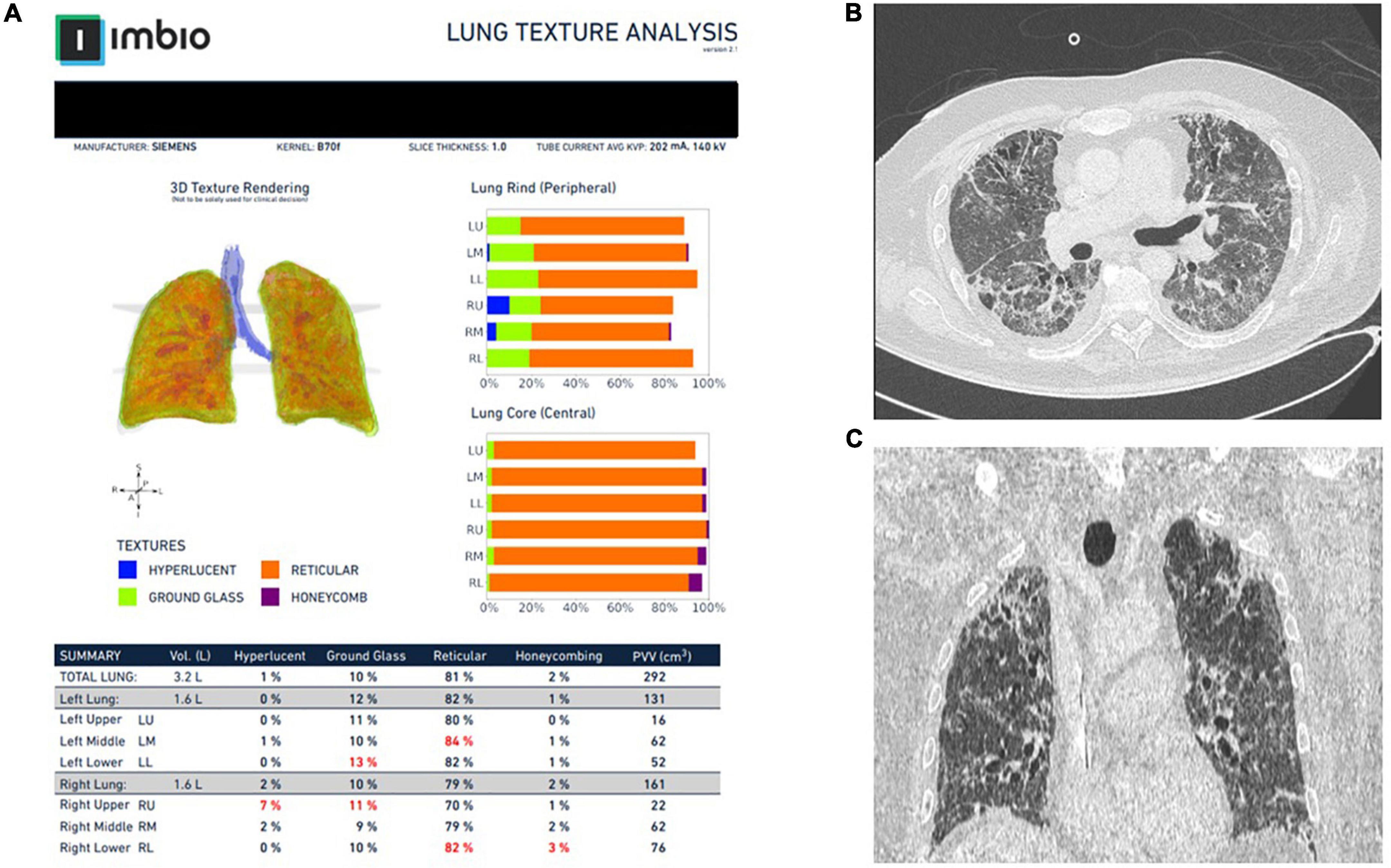
Figure 3. Representative images from Imbio Lung Texture Analysis 2.1 of a patient with post-COVID-19-ILD (A). Notice that almost 80% of the lung parenchyma presents with reticular abnormalities that are inconspicuous (at least to that extent) to the bear eye of the operator (B,C).
Post-COVID-19 interstitial lung disease
Management of patients with post-COVID-19 interstitial lung disease was based on a common algorithm as shown in Figure 4A. Patients with reticulation >5% or honeycombing (n = 71, 30.6%) were meticulously evaluated at 6-months. Among them, patients with either of the following: FVC% predicted <70, DLCO% predicted <50 or 6-min walking distance <350 m (n = 16, 6.9%) had been offered a 6-week course of oral prednisolone with gradual tapering at the time point of the 3-month follow-up visit. The rest patients were meticulously evaluated as shown in Figure 4B and to this end none of them presented with disease progression.
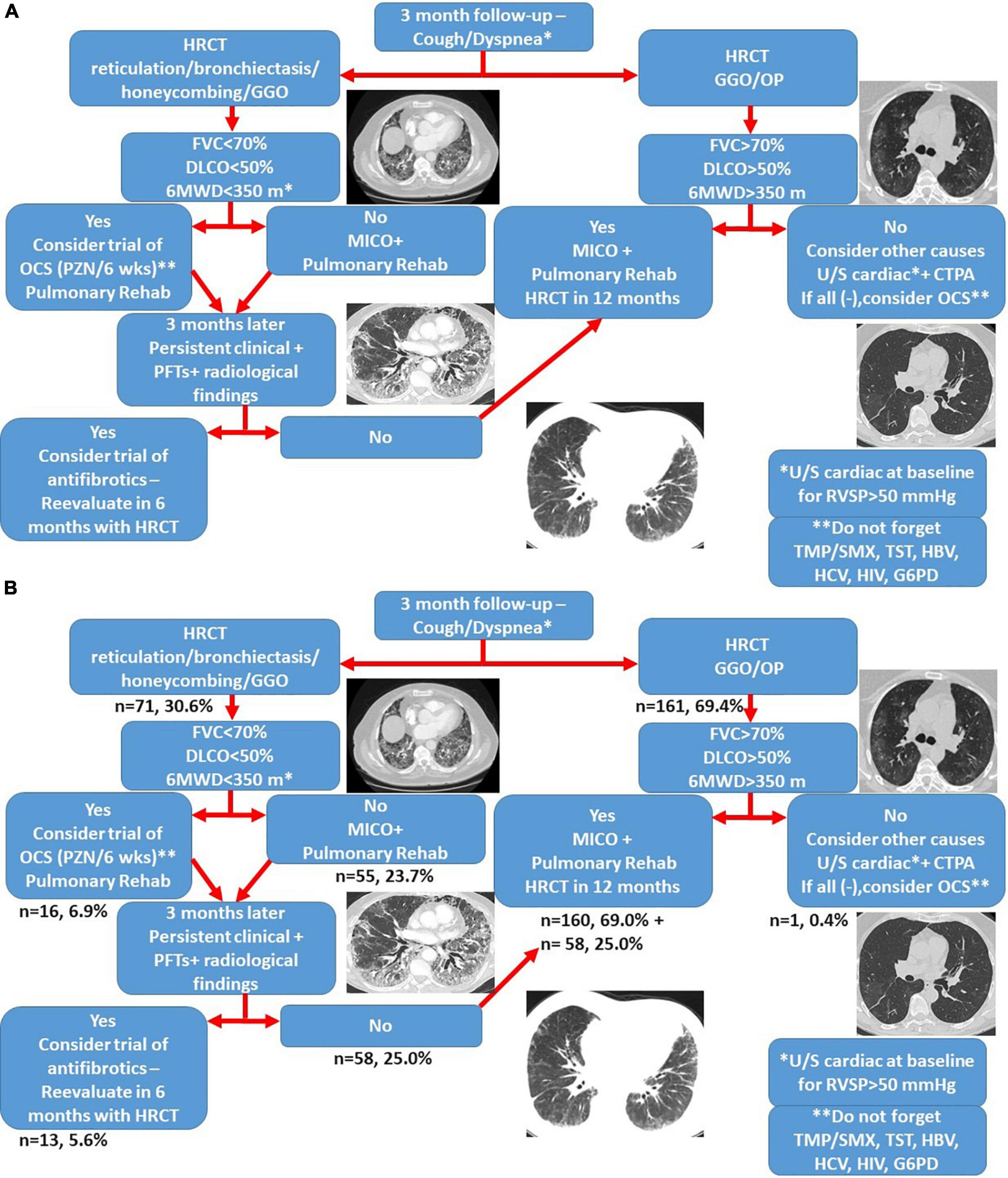
Figure 4. Treatment algorithm for patients with post-COVID-19 interstitial lung disease (A). The proportion of patients that belongs to each group is shown in panel (B). CTPA, computed tomography pulmonary angiogram; GGO, ground glass opacities; G6PD, glucose-6-phosphate dehydrogenase; HBV, hepatitis B virus; HCV, hepatitis C virus; HIV, human immunodeficiency virus; MICO, masterful inactivity with cat-like observation; OCS, oral corticosteroids; OP, organizing pneumonia; TMP/SMX, trimethoprim-sulfamethoxazole; TST, tuberculin skin test; 6MWD: 6 min walking distance.
At the time point of the 6-month follow-up visit, 13 patients (5.6%) presented with evidence of both “fibrotic-like” abnormalities and persistently decreased functional indices as shown in Figure 4B. Of note, none of these patients was vaccinated against COVID-19 (0/13, 0%). In these patients, antifibrotics were implemented. Antifibrotics were chosen based on patients’ comorbidities and preferences following discussion for the potential adverse events of each compound (pirfenidone: 10, nintedanib: 3). A statistically significant improvement was observed with regards to ground glass opacities in the follow-up HRCT 6 months after initiation of antifibrotics [25.0 (95% CI: 6.7–27.9) vs. 5.0 (95% CI: 0.5–8.8), p = 0.04]. A trend for improvement of reticular opacities was also observed [8.0 (95% CI: 2.5–15.0) vs. 2.0 (95% CI: 1.0–8.9), p = 0.13]. Patients presented with an improvement (absolute change) of 10.3 (p = 0.01) and 14.6 (p = 0.01) in FVC% predicted at 3 and 6 months after the initiation of antifibrotic (Figure 5A). An absolute change of +8.6 (p = 0.0003) and +15.1 (p = 0.002) was observed with regards to DLCO% predicted (Figure 6A). Subgroup analysis of patients with 1-year follow-up is presented in Figures 5, 6B.
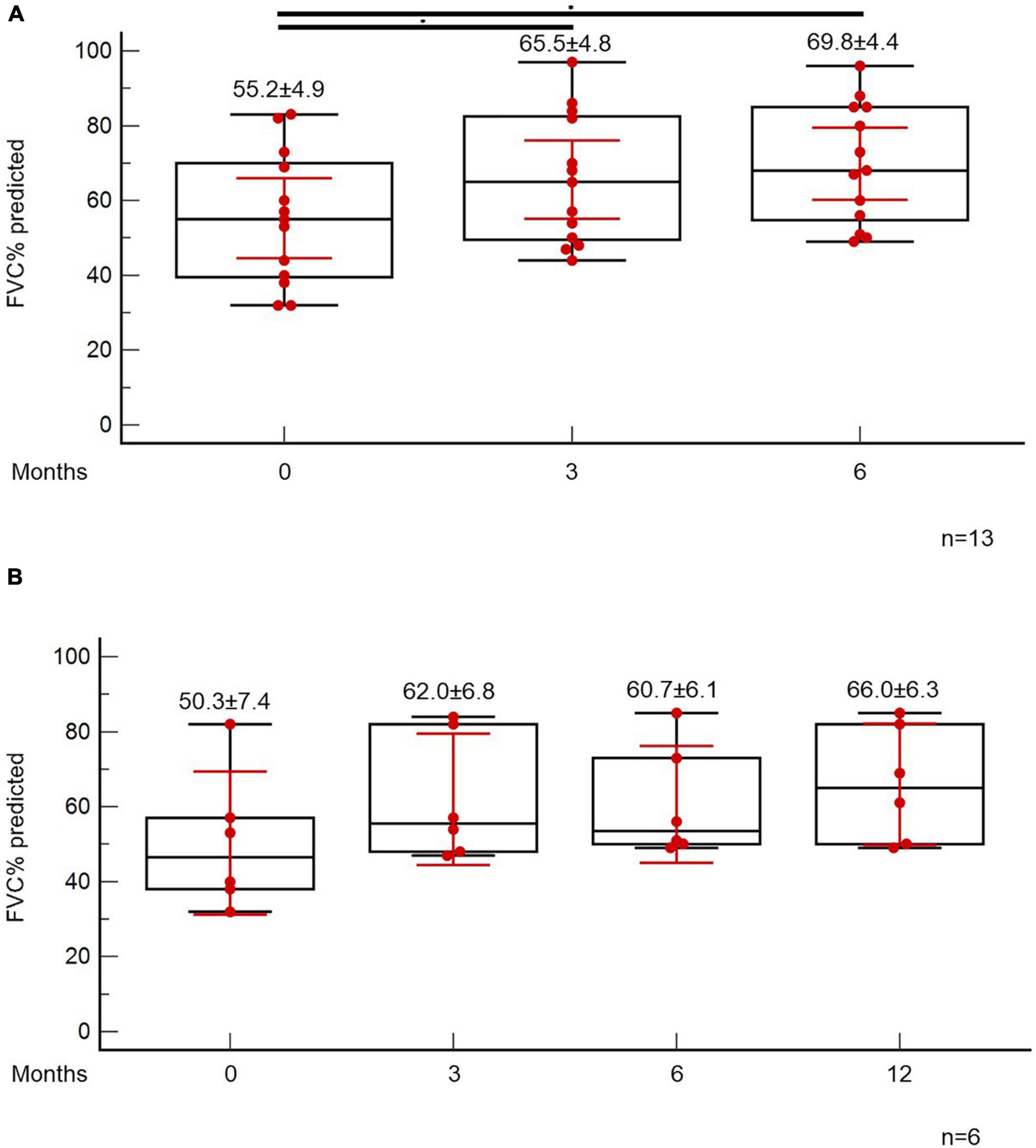
Figure 5. Patients with fibrotic-like changes and persistently decreased functional indices received antifibrotics. ANOVA repeated measures was used to investigate differences in FVC% predicted at different time points (A). Subgroup analysis of patients with 1-year follow-up is presented in panel (B).
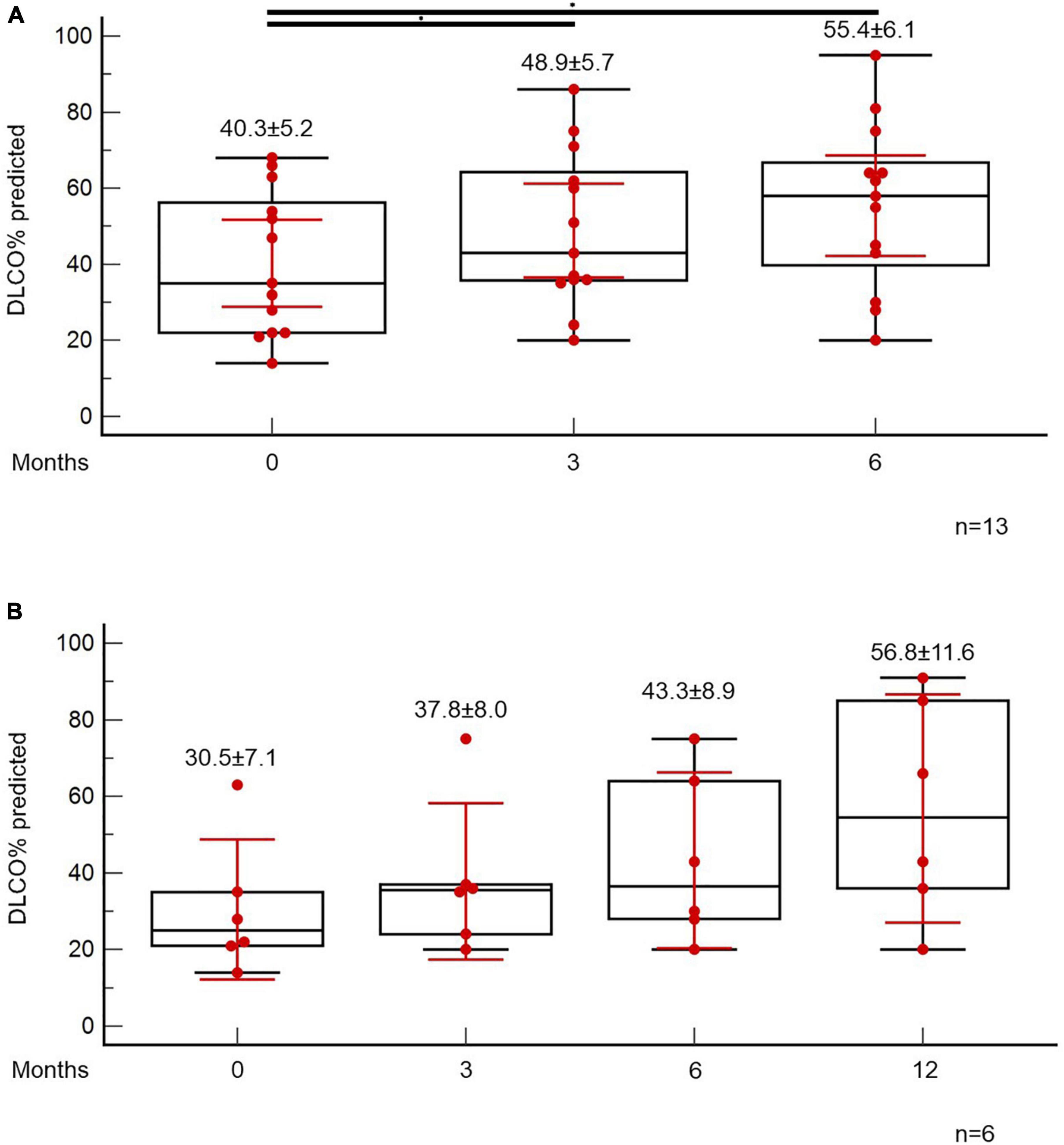
Figure 6. Patients with fibrotic-like changes and persistently decreased functional indices received antifibrotics. ANOVA repeated measures was used to investigate differences in DLCO% predicted at different time points (A). Subgroup analysis of patients with 1-year follow-up is presented in panel (B).
Discussion
This is the first study in Caucasian population aiming to investigate post-COVID-19-ILD through a machine learning radiographic model. Our report presented in detail the functional and radiographic impact of this entity and validated previous evidence showing that a considerable proportion of patients is experiencing functional impairment several months following infection. Fibrotic lung disease was observed in a substantial minority of COVID-19 survivors. These patients might experience benefit from antifibrotic initiation at the time point that fibrotic-like changes are “immature.”
Our study exhibited a number of important attributes that should be presented. First, we evaluated disease extent through a machine learning model. Manual interpretation of radiographic abnormalities extent is hindered by variability, especially at centers with lack of expertise (17). Deep learning might be the key to overcome barriers for fast and massive radiological evaluation in an unbiased fashion. Deep learning could be deployed all over the world and provide homogeneous and accurate reporting (21–23). Second, we demonstrated that a short course of antifibrotics could significantly improve lung function. Third, based on the fact that the majority of our cohort was unvaccinated against COVID-19, we provided indirect evidence that vaccination against COVID-19 is not only the best way to contain the pandemic but also the best way to limit post-acute sequelae of COVID-19.
Percentage of patients with functional and radiographic impairment is in line from data of other countries (24–32). In the 3-month follow-up, almost 30% of this cohort presented with DLCO% predicted below 80. A recent, large study in China showed in the 6-month follow-up that DLCO% predicted was below 80 in 21% of patients that were not in need of oxygen supplementation and in 57% of hospitalized patients that presented with WHO ordinal scale of 5 or 6 (32). Reticulation >5% was observed in almost one out of four patients in this study. Other studies reported that fibrotic like changes ranged from 22.5 to 35% in the 6-month follow-up (26, 30). Importantly, we observed fibrotic lung disease and substantial functional impairment in 5.6% of this cohort. Previous studies reported significant functional deficit in almost 5% of COVID-19 survivors, while the prevalence of the so called post-COVID-19 interstitial lung damage was estimated between 6.5 and 8.3% (24, 25). Suggested risk factors for post-COVID-19 fibrotic lung disease have been male gender, increased age, increased body mass index, duration of hospitalization >17 days, disease severity, extent of baseline radiographic lesions, intensity of ventilatory support, persistent viremia and Epstein–Barr reactivation, diabetes mellitus, and presence of auto-antibodies (32–38).
With regards to treatment of post-COVID-19-ILD, a minority of our patients received oral corticosteroids. Percentage of treated patients in this cohort is comparable to a previously published large study reporting that corticosteroids were suggested initially in 35/837 COVID-19 survivors and finally prescribed in 3.6% (n = 30) of patients (24). Importantly, high doses of prednisolone (40 mg tapered) were not superior to lower doses (10 mg tapered) in patients with post-COVID-19-ILD (39). In the context of “fibrotic-like” changes, sequelae consistent with “fibrotic-like” parenchymal lung disease has been observed following COVID-19 infection in a minority of patients and importantly these changes seem to lack of resolution in a considerable proportion of cases (40); yet, estimates must be interpreted cautiously due to substantial heterogeneity, differences in study casemix, and baseline severity among studies (41). Antifibrotics were prescribed in a substantial minority of this cohort. Pirfenidone was administered more often, as a considerable proportion of patients had history of pulmonary embolism following COVID-19 infection or was receiving anticoagulants due to chronic heart disease. While high quality trials aiming to address their role in post-COVID-19 interstitial lung disease are greatly anticipated (42–44), observational studies have suggested that antifibrotics might confer benefit (45, 46) with regards to radiologic improvement and time to recovery in patients with sustained and extensive fibrotic changes. The concept of antifibrotics implementation in a minority of patients at the time point that fibrotic-like changes are “immature” deserves further investigation (47). It is a matter of ongoing debate if all abnormalities characterized as “fibrotic-like” can reliably indicate irreversible disease in a post-Acute Respiratory Distress Syndrome setting (48, 49). Eminent fibrotic-like changes and architectural distortion on HRCT is not necessarily synonymous with irreversible lung scarring, particularly in the context of a post-infectious syndrome (50). Similarly, bronchiectatic-like lesions during the acute phase of a respiratory infection may not necessarily represent irreversible and permanent enlargement of the airways but may largely resolve following resolution of the infection. Even if “fibrotic-like” changes on HRCT represent histologic fibrosis in a subgroup of cases, remodeling and regression of “immature” fibrosis represents an anticipated phenomenon following severe acute lung injury (49–51). In such cases, antifibrotics might be beneficial. Machine learning models might be helpful for the appropriate classification and selection of patients likely to benefit from antifibrotics as they may quantify in an unbiased and accurate fashion the extent and the type of lesions.
Our trial has some limitations. First, our report has the inherent weaknesses of a retrospective study. Second, follow-up period of patients under antifibrotics is relatively short given that post-COVID-19-ILD is a new entity; yet, even in this short period, our study provided evidence that antifibrotics might confer significant benefit if applied early during the post-COVID-19 syndrome when fibrotic-like changes appear to be “immature.” Third, a major limitation is that this cohort has not undergone HRCT prior SARS-CoV-2 infection to compare pre and post-infection radiographic abnormalities and identify a subset of patients with pre-existing ILD patterns. Nonetheless, the percentage of patients with functional and radiographic impairment is similar to other published reports and importantly our aim was to highlight the need of early detection and management of interstitial lung disease. Moreover, based on current guidelines, HRCT during infection was not performed in all cases, while PFTs could not be performed at the acute phase due to safety considerations. Thus, we could not compare radiographic and functional status between the acute phase and the 3-month follow-up. Finally, based on the fact we used a common algorithm and treated similarly all cases of same severity, we could not compare patients that receive antifibrotics with other patients of same phenotype that didn’t. Besides, this was not a randomized-controlled trial. This was a real-life study aiming to present outcomes following management with a common algorithm and using a highly novel machine learning radiographic model.
Collectively, post-COVID-19-ILD represents an emerging entity. Given that a considerable proportion of infected individuals has functional impairment several months after infection, screening 3 months following infection is encouraged, especially for severe cases that have been hospitalized for prolonged periods. On the other hand, post-COVID-19 HRCT screening may confer several other benefits, including identification of incidental lung cancer lesions, considering that the majority of patients screened for post-COVID-19 present with several risk factors for lung cancer, such as age, smoking status and comorbidities, and including chronic respiratory diseases. Current evidence does not support irrational use of corticosteroids; yet, short-term corticosteroids might confer benefit to specific patients with organizing pneumonia, functional impairment and persistent clinical symptoms without compromising patients’ immune status. Finally, a minority of these patients with persistent fibrotic lung lesions and functional disability, despite steroid treatment, could benefit from antifibrotic therapy, if applied early on during disease clinical course. Machine learning radiographic models might have a cardinal role for precise, unbiased and quantitative radiographic evaluation which may guide therapeutic decisions.
Data availability statement
The original contributions presented in this study are included in the article/supplementary material, further inquiries can be directed to the corresponding author.
Ethics statement
The studies involving human participants were reviewed and approved by the International Conference on Harmonization E6 guidelines for Good Clinical Practice, the Declaration of Helsinki and the local regulation. Written informed consent for participation was not required for this study in accordance with the national legislation and the institutional requirements.
Author contributions
TK and VS: conception and design of the work, acquisition, analysis and interpretation of data, and drafting the work and revising it critically for important intellectual content. MK, PT, VG, IP, EB, IT, IL, OP, EZ, EA, AP, EM, FS, SM, SC, GH, KD, NT, NS, and KA: contributions to the acquisition of data and critical revision for important intellectual content. AT: conception and design of the work, acquisition, analysis and interpretation of data, drafting the work and revising it critically for important intellectual content, formal analysis, and project administration and supervision. All authors final approval for publication of the content, agreement to be accountable for all aspects of the work in ensuring that questions related to the accuracy or integrity of any part of the work are appropriately investigated and resolved.
Funding
The publication fees of this manuscript have been financed by the Research Council of the University of Patras.
Conflict of interest
AT has received grants and honoraria from GlaxoSmithKline, Astra Zeneca, Chiesi, Roche, and Boehringer Ingelheim outside the submitted work.
The remaining authors declare that the research was conducted in the absence of any commercial or financial relationships that could be construed as a potential conflict of interest.
The reviewer CB declared a shared affiliation, with no collaboration, with the authors to the handling editor at the time of the review.
Publisher’s note
All claims expressed in this article are solely those of the authors and do not necessarily represent those of their affiliated organizations, or those of the publisher, the editors and the reviewers. Any product that may be evaluated in this article, or claim that may be made by its manufacturer, is not guaranteed or endorsed by the publisher.
References
1. Guan W, Ni Z, Hu Y, Liang W, Ou C, He J, et al. Clinical characteristics of coronavirus disease 2019 in China. N Engl J Med. (2020) 382:1708–20. doi: 10.1056/NEJMoa2002032
2. Karampitsakos, T, Papaioannou O, Tsiri P, Katsaras M, Katsimpris A, Kalogeropoulos A, et al. Tocilizumab versus baricitinib in hospitalized patients with severe COVID-19: an open label, randomized controlled trial. Clin Microbiol Infect. (2022). doi: 10.1101/2022.06.13.22276211 [Epub ahead of print].
3. Karampitsakos T, Malakounidou E, Papaioannou O, Dimakopoulou V, Zarkadi E, Katsaras M, et al. Tocilizumab improves 28-day survival in hospitalized patients with severe COVID-19: an open label, prospective study. Respir Res. (2021) 22:317. doi: 10.1186/s12931-021-01914-6
4. Vehar S, Boushra M, Ntiamoah P, Biehl M. Post-acute sequelae of SARS-CoV-2 infection: caring for the ‘long-haulers’. Cleve Clin J Med. (2021) 88:267–72. doi: 10.3949/ccjm.88a.21010
5. Chen C, Haupert S, Zimmermann L, Shi X, Fritsche L, Mukherjee B. Global prevalence of post COVID-19 condition or long COVID: a meta-analysis and systematic review. J Infect Dis. (2022) 226:1593–607. doi: 10.1093/infdis/jiac136
6. Brodin P, Casari G, Townsend L, O’Farrelly C, Tancevski I, Löffler-Ragg J, et al. Studying severe long COVID to understand post-infectious disorders beyond COVID-19. Nat Med. (2022) 28:879–82. doi: 10.1038/s41591-022-01766-7
7. Datta S, Talwar A, Lee JTA. Proposed framework and timeline of the spectrum of disease due to SARS-CoV-2 infection: illness beyond acute infection and public health implications. JAMA. (2020) 324:2251–2. doi: 10.1001/jama.2020.22717
8. Venkatesan P. NICE guideline on long COVID. Lancet Respir Med. (2021) 9:129. doi: 10.1016/S2213-2600(21)00031-X
9. Nalbandian A, Sehgal K, Gupta A, Madhavan M, McGroder C, Stevens J, et al. Post-acute COVID-19 syndrome. Nat Med. (2021) 27:601–15. doi: 10.1038/s41591-021-01283-z
10. Culebras M, Loor K, Sansano I, Persiva Ó, Clofent D, Polverino E, et al. Histological findings in transbronchial cryobiopsies obtained from patients after COVID-19. Chest. (2022) 161:647–50. doi: 10.1016/j.chest.2021.09.016
11. Tourki B, Vera I, Perrot C, Farsaei F, Arsenault A, Juan-Guardela B, et al. Peripheral blood gene expression changes predict mortality and treatment response in acute Covid-19 and associate with lung fibrosis. C32 interrogation of genetic variation, gene expression, and other biological layers with omics techniques to understand respiratory traits. Am J Respir Crit Care Med. (2022) 205:A3929. doi: 10.1164/ajrccm-conference.2022.205.1_MeetingAbstracts.A3929
12. Boutou A, Asimakos A, Kortianou E, Vogiatzis I, Tzouvelekis A. Long COVID-19 pulmonary sequelae and management considerations. J Pers Med. (2021) 11:838. doi: 10.3390/jpm11090838
13. Boutou A, Georgopoulou A, Pitsiou G, Stanopoulos I, Kontakiotis T, Kioumis I. Changes in the respiratory function of COVID-19 survivors during follow-up: a novel respiratory disorder on the rise? Int J Clin Pract. (2021) 75:e14301. doi: 10.1111/ijcp.14301
14. Karampitsakos T, Kalogeropoulou C, Tzilas V, Papaioannou O, Kazantzi A, Koukaki E, et al. Safety and effectiveness of mycophenolate mofetil in interstitial lung diseases: insights from a machine learning radiographic model. Respiration. (2022) 101:262–71. doi: 10.1159/000519215
15. Levin D. Deep learning and the evaluation of pulmonary fibrosis. Lancet Respir Med. (2018) 6:803–5. doi: 10.1016/S2213-2600(18)30371-0
16. González G, Ash S. Disease staging and prognosis in smokers using deep learning in chest computed tomography. Am J Respir Crit Care Med. (2018) 197:193–203. doi: 10.1164/rccm.201705-0860OC
17. Walsh S, Calandriello L, Silva M, Sverzellati N. Deep learning for classifying fibrotic lung disease on high-resolution computed tomography: a case-cohort study. Lancet Respir Med. (2018) 6:837–45. doi: 10.1016/S2213-2600(18)30286-8
18. Salisbury M, Lynch D, van Beek E, Kazerooni E, Guo J, Xia M, et al. Idiopathic pulmonary fibrosis: the association between the adaptive multiple features method and fibrosis outcomes. Am J Respir Crit Care Med. (2017) 195:921–9. doi: 10.1164/rccm.201607-1385OC
19. Maldonado F, Moua T, Rajagopalan S, Karwoski R, Raghunath S, Decker P, et al. Automated quantification of radiological patterns predicts survival in idiopathic pulmonary fibrosis. Eur Respir J. (2014) 43:204–12. doi: 10.1183/09031936.00071812
20. Trusculescu A, Manolescu D, Tudorache E, Oancea C. Deep learning in interstitial lung disease—how long until daily practice. Eur Radiol. (2020) 30:6285–92. doi: 10.1007/s00330-020-06986-4
21. Lynch D, Sverzellati N, Travis W, Brown K, Colby T, Galvin J, et al. Diagnostic criteria for idiopathic pulmonary fibrosis: a Fleischner society white paper. Lancet Respir Med. (2018) 6:138–53. doi: 10.1016/S2213-2600(17)30433-2
22. Raghu GR-JM, Myers J, Richeldi L, Ryerson C, Lederer D, Behr J, et al. Diagnosis of idiopathic pulmonary fibrosis. An official ATS/ERS/JRS/ALAT clinical practice guideline. Am J Respir Crit Care Med. (2018) 198:e44–68. doi: 10.1164/rccm.201807-1255ST
23. Raghu G, Remy-Jardin M, Ryerson C, Myers J, Kreuter M, Vasakova M, et al. Diagnosis of hypersensitivity pneumonitis in adults. An official ATS/JRS/ALAT clinical practice guideline. Am J Respir Crit Care Med. (2020) 202:e36–69.
24. Myall K, Mukherjee B, Castanheira A, Lam J, Benedetti G, Mak S, et al. Persistent post-COVID-19 interstitial lung disease. An observational study of corticosteroid treatment. Ann Am Thorac Soc. (2021) 18:799–806. doi: 10.1513/AnnalsATS.202008-1002OC
25. Stewart I, Jacob J, George P, Molyneaux P, Porter J, Allen R, et al. Interstitial lung damage following COVID-19 hospitalisation: an interim analysis of the UKILD Post-COVID study. medRxiv [Preprint]. (2022). doi: 10.1101/2022.03.10.22272081
26. Tomassetti S, Oggionni T, Barisione E, Bargagli E, Bonifazi M, Confalonieri M, et al. A multidisciplinary multicenter study evaluating risk factors, prevalence and characteristics of post-Covid-19 interstitial lung syndrome (PCOILS). Eur Respir J. (2021) 58(Suppl. 65):OA1567. doi: 10.1183/13993003.congress-2021.OA1567
27. Ravaglia C, Doglioni C, Chilosi M, Piciucchi S, Dubini A, Rossi G, et al. Clinical, radiological and pathological findings in patients with persistent lung disease following SARS-CoV-2 infection. Eur Respir J. (2022) 60:2102411. doi: 10.1183/13993003.02411-2021
28. Cau R, Faa G, Nardi V, Balestrieri A, Puig J, Suri J, et al. Long-COVID diagnosis: from diagnostic to advanced AI-driven models. Eur J Radiol. (2022) 148:110164. doi: 10.1016/j.ejrad.2022.110164
29. Tarraso J, Safont B, Carbonell-Asins J, Fernandez-Fabrellas E, Sancho-Chust J, Naval E, et al. Lung function and radiological findings 1 year after COVID-19: a prospective follow-up. Respir Res. (2022) 23:242. doi: 10.1186/s12931-022-02166-8
30. Han X, Fan Y, Alwalid O, Li N, Jia X, Yuan M, et al. Six-month follow-up chest CT findings after severe COVID-19 pneumonia. Radiology. (2021) 299:E177–86. doi: 10.1148/radiol.2021203153
31. Caruso D, Guido G, Zerunian M, Polidori T, Lucertini E, Pucciarelli F, et al. Post-acute sequelae of COVID-19 pneumonia: six-month chest CT follow-up. Radiology. (2021) 301:E396–405. doi: 10.1148/radiol.2021210834
32. Huang L, Yao Q, Gu X, Wang Q, Ren L, Wang Y, et al. 1-year outcomes in hospital survivors with COVID-19: a longitudinal cohort study. Lancet. (2021) 398:747–58. doi: 10.1016/S0140-6736(21)01755-4
33. Wallis T, Heiden E, Horno J, Welham B, Burke H, Freeman A, et al. Risk factors for persistent abnormality on chest radiographs at 12-weeks post hospitalisation with PCR confirmed COVID-19. Respir Res. (2021) 22:157. doi: 10.1186/s12931-021-01750-8
34. Ntatsoulis K, Karampitsakos T, Tsitoura E, Stylianaki E, Matralis A, Tzouvelekis A, et al. Commonalities between ARDS. pulmonary fibrosis and COVID-19: the potential of autotaxin as a therapeutic target. Front Immunol. (2021) 12:687397. doi: 10.3389/fimmu.2021.687397
35. Lee J, Yim J, Park J. Pulmonary function and chest computed tomography abnormalities 6–12 months after recovery from COVID-19: a systematic review and meta-analysis. Respir Res. (2022) 23:233. doi: 10.1186/s12931-022-02163-x
36. Valenzuela C, Waterer G, Raghu G. Interstitial lung disease before and after COVID-19: a double threat? Eur Respir J. (2021) 58:2101956. doi: 10.1183/13993003.01956-2021
37. Cocconcelli E, Bernardinello N, Giraudo C, Castelli G, Giorgino A, Leoni D, et al. Characteristics and prognostic factors of pulmonary fibrosis after COVID-19 pneumonia. Front Med. (2022) 8:823600. doi: 10.3389/fmed.2021.823600
38. Su Y, Yuan D, Chen D, Ng R, Wang K, Choi J, et al. Multiple early factors anticipate post-acute COVID-19 sequelae. Cell. (2022) 185:881–95.e20.
39. Dhooria S, Chaudhary S, Sehgal I, Agarwal R, Arora S, Garg M, et al. High-dose versus low-dose prednisolone in symptomatic patients with post-COVID-19 diffuse parenchymal lung abnormalities: an open-label, randomised trial (the COLDSTER trial). Eur Respir J. (2022) 59:2102930. doi: 10.1183/13993003.02930-2021
40. Han X, Fan Y, Alwalid O, Zhang X, Jia X, Zheng Y, et al. Fibrotic interstitial lung abnormalities at 1-year follow-up CT after severe COVID-19. Radiology. (2021) 301:E438–40. doi: 10.1148/radiol.2021210972
41. Fabbri L, Moss S, Khan F, Chi W, Xia J, Robinson K, et al. Parenchymal lung abnormalities following hospitalisation for COVID-19 and viral pneumonitis: a systematic review and meta-analysis. Thorax. (2022). doi: 10.1101/2021.03.15.21253593 [Epub ahead of print].
42. U.S. National Library of Medicine. Pirfenidone vs. Nintedanib for Fibrotic Lung Disease After Coronavirus Disease-19 Pneumonia (PINCER). Available online at: https://clinicaltrials.gov/ct2/show/NCT04856111
43. U.S. National Library of Medicine. Nintedanib for the Treatment of SARS-Cov-2 Induced Pulmonary Fibrosis (NINTECOR). Available online at: https://clinicaltrials.gov/ct2/show/NCT04541680
44. U.S. National Library of Medicine. Pirfenidone Compared to Placebo in Post-COVID19 Pulmonary Fibrosis COVID-19 (FIBRO-COVID). Available online at: https://clinicaltrials.gov/ct2/show/NCT04607928
45. Singh P, Behera D, Gupta S, Deep A, Priyadarshini S, Padhan P. Nintedanib vs pirfenidone in the management of COVID-19 lung fibrosis: a single-centre study. J R Coll Physicians Edinb. (2022) 52:100–4. doi: 10.1177/14782715221103402
46. Umemura Y, Mitsuyama Y, Minami K, Nishida T, Watanabe A, Okada N, et al. Efficacy and safety of nintedanib for pulmonary fibrosis in severe pneumonia induced by COVID-19: an interventional study. Int J Infect Dis. (2021) 108:454–60. doi: 10.1016/j.ijid.2021.05.055
47. Hashemian, S, Farhadi T, Varahram M, Velayati A. Nintedanib: a review of the properties, function, and usefulness to minimize COVID-19 induced lung injury. Expert Rev Anti Infect Ther. (2022) 1–8. doi: 10.1080/14787210.2023.2153116 [Epub ahead of print].
48. Spagnolo P, Balestro E, Aliberti S, Cocconcelli E, Biondini D, Casa G, et al. Pulmonary fibrosis secondary to COVID-19: a call to arms? Lancet Respir Med. (2020) 8:750–2. doi: 10.1016/S2213-2600(20)30222-8
49. Wells A, Devaraj A, Desai S. Interstitial lung disease after COVID-19 infection: a catalog of uncertainties. Radiology. (2021) 299:E216–8. doi: 10.1148/radiol.2021204482
50. Wells A, Devaraj A. Residual lung disease at six-month follow-up CT after COVID-19: clinical significance is a key issue. Radiology. (2021) 301:E406–8. doi: 10.1148/radiol.2021211284
Keywords: post-COVID-19, long COVID, interstitial lung disease, antifibrotics, machine learning
Citation: Karampitsakos T, Sotiropoulou V, Katsaras M, Tsiri P, Georgakopoulou VE, Papanikolaou IC, Bibaki E, Tomos I, Lambiri I, Papaioannou O, Zarkadi E, Antonakis E, Pandi A, Malakounidou E, Sampsonas F, Makrodimitri S, Chrysikos S, Hillas G, Dimakou K, Tzanakis N, Sipsas NV, Antoniou K and Tzouvelekis A (2023) Post-COVID-19 interstitial lung disease: Insights from a machine learning radiographic model. Front. Med. 9:1083264. doi: 10.3389/fmed.2022.1083264
Received: 28 October 2022; Accepted: 30 December 2022;
Published: 17 January 2023.
Edited by:
Rifat Hamoudi, University of Sharjah, United Arab EmiratesReviewed by:
Constantina A. Bounia, General University Hospital of Patras, GreeceLudovica Capitelli, University of Naples Federico II, Italy
Athanasios Voulgaris, Democritus University of Thrace, Greece
Copyright © 2023 Karampitsakos, Sotiropoulou, Katsaras, Tsiri, Georgakopoulou, Papanikolaou, Bibaki, Tomos, Lambiri, Papaioannou, Zarkadi, Antonakis, Pandi, Malakounidou, Sampsonas, Makrodimitri, Chrysikos, Hillas, Dimakou, Tzanakis, Sipsas, Antoniou and Tzouvelekis. This is an open-access article distributed under the terms of the Creative Commons Attribution License (CC BY). The use, distribution or reproduction in other forums is permitted, provided the original author(s) and the copyright owner(s) are credited and that the original publication in this journal is cited, in accordance with accepted academic practice. No use, distribution or reproduction is permitted which does not comply with these terms.
*Correspondence: Argyris Tzouvelekis, YXR6b3V2ZWxla2lzQHVwYXRyYXMuZ3I=, YXJneXJpcy50em91dmVsZWtpc0BnbWFpbC5jb20=
†These authors have contributed equally to this work and share first authorship