Designing a novel chimeric multi-epitope vaccine against Burkholderia pseudomallei, a causative agent of melioidosis
- 1Department of Biology, College of Education (Majmaah), Majmaah University, Al Majmaah, Saudi Arabia
- 2Department of Medical Biotechnology, College of Applied Medical Sciences, Qassim University, Buraydah, Saudi Arabia
Burkholderia pseudomallei, a gram-negative soil-dwelling bacterium, is primarily considered a causative agent of melioidosis infection in both animals and humans. Despite the severity of the disease, there is currently no licensed vaccine on the market. The development of an effective vaccine against B. pseudomallei could help prevent the spread of infection. The purpose of this study was to develop a multi-epitope-based vaccine against B. pseudomallei using advanced bacterial pan-genome analysis. A total of four proteins were prioritized for epitope prediction by using multiple subtractive proteomics filters. Following that, a multi-epitopes based chimeric vaccine construct was modeled and joined with an adjuvant to improve the potency of the designed vaccine construct. The structure of the construct was predicted and analyzed for flexibility. A population coverage analysis was performed to evaluate the broad-spectrum applicability of B. pseudomallei. The computed combined world population coverage was 99.74%. Molecular docking analysis was applied further to evaluate the binding efficacy of the designed vaccine construct with the human toll-like receptors-5 (TLR-5). Furthermore, the dynamic behavior and stability of the docked complexes were investigated using molecular dynamics simulation, and the binding free energy determined for Vaccine-TLR-5 was delta total −168.3588. The docking result revealed that the vaccine construct may elicit a suitable immunological response within the host body. Hence, we believe that the designed in-silico vaccine could be helpful for experimentalists in the formulation of a highly effective vaccine for B. pseudomallei.
Introduction
Burkholderia pseudomallei, a member of the family Burkholderiaceae, is a rod-shaped, gram-negative, motile, and multitrichous flagella bacterium typically of 1–5 μm long and diameter of 0.5–1.0 μm (1). Melioidosis, often known as Whitmore's infection, is caused by B. pseudomallei and is characterized by
high mortality and morbidity rates (2). The infection usually leads to abscess formation and sepsis. This pathogen infects both animals and humans when they come into contact with contaminated soil (3). The bacteria can be spread from an infected person to a non-infected person and can be acquired in the hospital environment as well. Despite the rarity of zoonotic transmission and animal-to-animal infection transfer, a wide variety of species have contracted the disease (4). The bacterium B. pseudomallei is thought to have a role in horizontal gene transfer (4). Melioidosis infection is prevalent in tropical regions while the main endemic regions include Southeast Asia and northern Australia (5, 6). Every year, around 165,000 cases of Melioidosis are reported worldwide, with 89,000 deaths (7). A high mortality rate of 42.6% was estimated in Thailand from 1997 to 2006 (6). By 2018, 30–35% of infections in Thailand's hospitals resulted in deaths (8). The bacterium is regarded as a biological weapon, and it has been linked to diabetes, chronic lung and renal diseases, malignancies, and heart diseases. Earlier diagnosis of the disease can decrease the mortality rate. Melioidosis chronic infection is frequently associated with the risk factors indicated above (9). The remarkable B. pseudomallei's adaptative characteristics allow the bacteria to survive in the host body and neutralize host immune responses (2). As this infection is associated with many clinical manifestations, its diagnosis is considered difficult (10). The incubation period also varies but usually, it is 1–21 days (11). In case of infection, the infected person's blood test generally has elevated liver enzymes, higher levels of urea and creatinine, low blood glucose levels, and fewer white blood cell counts (2). Most of the infected individuals have pneumonia. The pathogen affects internal organs like the spleen, kidney, and liver (12). Toll-like receptors (TLRs) play an important role in inducing innate immunity. Infected patients showed increased expression of TLRs (13).
Early antimicrobial therapy against the infection proved effective in lowering the mortality rate in endemic regions (11). The pathogen shows resistance to several antibiotics, including cephalosporins, imipenem, meropenem, tetracyclines, sulphonamides, etc. The effectiveness of antimicrobial therapy depends upon the duration of the treatment, which in normal cases is up to 6 months (14). A licensed vaccine for Melioidosis has not been not reported to date, but recent studies confirmed the pre-clinical trials of B. pseudomallei vaccine in animal models (15). Several strategies for vaccine development against Burkholderia have been explored, but none of them induce sterilizing immunity for a long time. Some promising live attenuated and subunit vaccines against Burkholderia have been proposed. Many of the studies revealed short-term immune protection against the pathogen in animal models (15). The experimental vaccine development can be assisted with computational vaccine approaches that use genomic information of bacterial pathogens to predict novel antigenic peptides. The peptide vaccines are easy to produce and much cheaper compared to whole or subunit-based vaccines. This study highlights several vaccine targets against the pathogen using immunoinformatic approaches. A multi-epitope peptide vaccine is constructed along with MALP-2 (macrophage activating lipoprotein 2) adjuvant. Computational analysis of the vaccine highlighted its good ability to induce protective responses against the pathogen. This strategy is considered simple and cost effective and the findings might be useful for multi-epitope peptide vaccine development against B. pseudomallei.
The multi-epitopes vaccine (MEVC) construct has many advantages over the single peptide-based vaccine. MEVC elicits both humoral and cellular immune responses because it comprises both B and B-cell derived T-cell epitopes (16, 17). Other advantages of MEVC include probable antigenicity, less toxicity, non-allergenicity, and good water-soluble properties (18). The MEVC in the present study comprises multiple epitopes which were prioritized from vaccine targets. The vaccine proteins were filtered using pan-genome analysis and reverse vaccinology techniques. Extracellular, periplasmic, and outer membrane proteins were shortlisted for epitope mapping. B-cell derived T-cell epitopes were predicted and potential epitopes were used to design a vaccine construct which further underwent different computational analyses. Toll-like receptor 5 (TLR5), shows high expression in Melioidosis (15) and is a receptor for bacterial flagellin. This receptor was used for molecular docking advanced by molecular dynamics simulation and binding free energies.
Methodology
Core proteome retrieval
Completely sequenced 91 proteomes of B. pseudomallei were obtained from the National Center for Biotechnology Information (NCBI) (19) followed by the retrieval of the core proteomes of the species by applying the bacterial pan genome analysis tool (BPGA) (19). CD-HIT was then used to extract non-redundant sequences at a 90% threshold. CD-HIT is a cluster database used to analyze the proteomes by removing similar sequences. PSORTB v 3.0 (20) was used for subcellular localization. Subcellular localization refers to the protein localization prediction in B. pseudomallei. The outer membrane, extracellular, and periplasmic proteins were chosen for epitope mapping leading to multi-epitope vaccine designing. The protein sequences obtained includes 39 extracellular, 53 periplasmic, and 55 outer membranes which were then subjected to further analysis as presented in Figure 1.
Identification of potential vaccine candidates
All the extracellular, periplasmic, and outer membrane proteins were checked for transmembrane helices using TMHMM 2.0 (https://services.healthtech.dtu.dk/service.php?TMHMM-2.0). Physiochemical properties like molecular weight, theoretical index, instability index, and GRAVY (hydrophilicity check) for proteins with transmembrane helices < 2 were checked by using the Protparam tool (https://web.expasy.org/protparam/). The molecular weight of the proteins should be <100 kDa as they can be easily purified from the cell, and their instability index should be <45. Further, antigenicity and allergenicity of the proteins were checked via Vaxijen v2.0 (21) and AllerTOP v2.0, respectively (22). The Innovagen server was used to shortlist the soluble proteins. The adhesion probability of the proteins was evaluated using Vaxign webserver (23). Finally, BLASTp was performed on shortlisted proteins against human and lactobacillus species to avoid auto-immune responses and to restrict accidental inhibition of the probiotic bacteria, respectively. The sequence identity and bit score used in this process were ≤30 % and 100, respectively.
Epitope prediction and prioritization
All the protein sequences meeting the criteria of being a potential candidate and shortlisted from all the checks were then used for epitope mapping utilizing the Immune Epitope Database and Analysis Resource (IEDB) (24). The proteins subjected to epitope mapping were antigenic, non-allergen, soluble, and adhesion probability > 0.5. B-cell epitope mapping was done using the B-cell epitope prediction tool of IEDB. The predicted B-cell epitopes were then used as input sequences for MHC-II (major histocompatibility complex II) binding epitopes prediction, and the anticipated MHC-II binding epitopes were used to predict MHC-I binding epitopes. A complete reference set of alleles were selected for T-cell epitope (MHC-I & MHC-II binding) mapping. The B-cell derived T-cell epitopes were further analyzed for their binding affinity by MHCpred v2.0 (http://www.ddg-pharmfac.net/mhcpred/MHCPred/). Epitopes with IC50 <100 nM were considered good binders and further scrutinized for antigenicity, allergenicity, toxicity, and solubility. Only epitopes that were antigens, non-allergen, non-toxic, and soluble were used to design a multi-epitope vaccine construct.
Population coverage analysis
Population coverage analysis of the designed vaccine was evaluated using the Population coverage tool of IEDB. The alleles which cover most of the world population were used for the analysis.
Multi-epitopes vaccine designing and 3D modeling
Shortlisted epitopes were linked together to design a vaccine construct. GPGPG linkers were used to link epitope to epitope while EAAAK linker was used to connect epitopes peptide with an adjuvant. The adjuvant molecule was used to boost vaccine immune responses. Here, we used four different adjuvants resulting in four different constructs which were further analyzed for physiochemical properties, antigenicity, allergenicity, adhesion probability, and secondary structures. Construct with Cholera toxin-B (CTB) adjuvant met the criteria to be considered best amongst the four. MALP-2 (GNNDESNISFKEK) was added to the construct, EAAAK linker was used to link the last epitope and MALP2 sequence. MALP-2 (macrophage activating lipoprotein) is a toll-like receptor (TLR) agonist mostly used to treat certain infections and also as an adjuvant for a vaccine. Physiochemical properties like molecular weight, hydrophilicity, theoretical, and instability index of the final vaccine construct were evaluated by the ProtParam tool (25). We predicted the 3D structure modeling of the vaccine using the Scratch predictor tool (25). The vaccine model was analyzed for the presence of the loops and subjected to loop modeling via Galaxy loop followed by refinement with Galaxy refine v 2.0 tool, respectively (26).
Cabs-flex analysis
The cabs-flex analysis is a computational approach to investigating the structural flexibility of the protein. The multi-epitopes peptide vaccine was subjected to coarse-grind simulations using Cabs-flex 2.0 (27). The analysis was run for 50 cycles and an RNG seed of 8335. The temperature range, weight of global side chain restraint, and the weight of global C-alpha restraints used in the analysis were 1.40, 1.0, and 1.0, respectively. This analysis proceeded by following the identification of aggregation-prone sites in protein sequence (vaccine) by AGGRESCAN 3D server v2.0 (http://bioinf.uab.es/aggrescan/).
Disulfide engineering and in-silico cloning
Disulfide by Design 2.0 was used to perform disulfide engineering of the vaccine model. It is usually done to identify unstable residues being mutated into cysteine residues narrating the induction of disulfide bonds making the structure stable (27).
Java Codon Adaptation Tool (JCat) (28) was used for the codon optimization of the vaccine construct. Through JCat, the vaccine protein sequence is translated into nucleotide sequence. Additionally, the CAI (codon adaptation index) value and GC content were determined, enabling in-silico cloning via Snapgene (29). The in silico cloning was done in vector pET-28a (+).
Computational immune simulation
A computational immune simulation is an approach to analyzing the vaccine construct for its immunogenicity and ability to induce immunity. This analysis was done through the C-ImmSim webserver (30). The webserver uses machine learning techniques while predicting the immune cells and antigen interaction.
Molecular docking
Toll-like receptor-5 (TLR-5) and vaccine model (ligand) were subjected to molecular docking using an online docking tool ClusPro 2.0 (31). Several docked solutions were generated and ranked based on binding energy. The solution with the lowest binding energy was chosen for further investigation. The interactions between the receptor and the vaccine were visualized through UCSF Chimera v 1.15, PDBsum (http://www.ebi.ac.uk/thornton-srv/databases/pdbsum/Generate.html) and Discovery Studio v2021.
Molecular dynamics simulation
Molecular dynamics simulation was performed for the docked complex of the vaccine model and TLR5 via (Assisted Model Building with Energy Refinement tool) AMBER 20 software. The simulation was conducted for 50 ns to understand the dynamic behavior in an aqueous solution. The force field “ff14SB” was used to generate parameters for both the vaccine and the TLR5, followed by incorporation of complex into TIP3P water box by maintaining 12 Å padding distance. The Na+ ions were added to neutralize the system. The carbon alpha atoms, non-heavy atoms, hydrogen atoms, and solvation box energies were minimized for 500, 1,000, 300, and 1,000 steps, respectively. Langevin dynamics were applied to maintain the system's temperature by the execution of system heating to 300 K for 20ps. The SHAKE algorithm was applied to constraint hydrogen bonds. The production run trajectories were analyzed by using the AMBER CPPTRAJ module. Binding free energies were predicted using the MMPBSA.py module (https://ambermd.org/tutorials/advanced/tutorial3/py_script/section4.htm).
Results
Retrieval of core proteome and determining the vaccine candidates
Pan-genome analysis was conducted for 91 completely sequenced proteomes of B. pseudomallei to get core proteome (19). The total number of the core proteins obtained were 310,128, which were analyzed through the CD-HIT analysis. The non-redundant sequences obtained were 3,647 while all the duplicate sequences were removed. As only extracellular, periplasmic, and outer membrane proteins were of interest so the subcellular localization of those 3,647 sequences was done by PSORTb. Sequences obtained were 39, 56, and 53 in number for extracellular, periplasmic, and outer membrane proteins, respectively (Figure 2).
The bacterial virulence factor was determined using the virulence factor database (VFDB). The protein sequences with a sequence identity of more than 30% and a bit score >100 were considered the best vaccine targets. Virulent proteins numbered 17 in the extracellular group, 18 in the periplasmic group, and 12 in the outer membrane group. The sequences were further analyzed for the presence of transmembrane helices that should be 0 or 1. The number of transmembrane helices was 0 for extracellular proteins, except for one. In the periplasmic group, only one had transmembrane helices out of 18 proteins while the remaining were lacking the transmembrane helices. Out of the 12 outer membrane proteins, only three (core/2096/1/Org1 Gene676, core/4074/1/Org1 Gene4523, and core/4761/73/Org73 Gene5861) had transmembrane helices, while the rest had 0. In all three groups, the proteins with no transmembrane helices were subjected to physicochemical evaluation. The parameters evaluated were molecular weight, theoretical index, GRAVY (Grand average of hydropathy), and instability index. Molecular weight was <100 kDa, while the GRAVY calculated was negative and the proteins having an instability index >40 were considered unstable proteins. The instability index of 15 extracellular proteins, 13 periplasmic, and nine outer membrane proteins were stable and within the range of 40. All these stable proteins were subjected to antigenicity (threshold 0.5), allergenicity, solubility, and adhesion stability (threshold 0.5) checks. All the non-antigenic and allergen proteins were discarded including eight extracellular, four periplasmic, and two outer membrane proteins. Similarly, the poorly soluble and the proteins having adhesion probability <0.5 were discarded, and only antigenic, non-allergens, soluble, and having adhesion probability were shortlisted as potential vaccine candidates. Four proteins were filtered out of which three (core/7319/1/Org1_Gene2732, core/7521/1/Org1_Gene303, and core/8978/1/Org1_Gene1539) were from the extracellular group and only 1 (core/3042/1/Org1_Gene5247) was a periplasmic protein as listed in Table 1. The BLASTp against human and lactobacillus species was also run for the shortlisted proteins that reported no significant similarity between the proteins and human/probiotic proteomes. The core/7319/1/Org1_Gene2732 protein is a type VI secretion system involved in the transportation of effector molecules from a bacterial cell to the target cell, core/7521/1/Org1_Gene303 is an Hcp family type VI secretion system effector which is present in the outer membrane and play a role in pathogenicity. The core/8978/1/Org1_Gene1539 is a flagellar biosynthesis anti-sigma factor FlgM which acts as a negative regulator in synthesizing flagellin and core/3042/1/Org1_Gene5247 is D-alanyl-D-alanine endopeptidase (32–34).
Epitope prediction and prioritization
In epitope mapping, T-cell epitopes were predicted from B-cell epitopes for eliciting both humoral and cellular immune responses. Using the shortlisted proteins as input sequences, the B-cell derived T-cell epitopes were predicted by IEDB. The B-cell epitope prediction tool of IEDB was used to predict potential peptides acting as B-cell epitopes. Peptides having a value ≥0.5 (threshold) were considered as B-cell epitopes. Thirteen peptides were predicted as B-cell epitopes. A total of 78 T-cell MHC class II binding epitopes were predicted from the B-cell epitopes. These epitopes were then used to predict the MHC class I binding epitopes on T-cells, and 120 of these epitopes with lengths of 9 or 10 residues were selected based on their percentile rank. The compete reference set of alleles was selected as a parameter for MHC class II and I binding epitopes prediction.
The predicted epitopes were prioritized using different parameters. First, they were subjected to MHCPred to select epitopes with IC50 values <100 nM to confirm the epitopes as good binders. With the help of this analysis, 64 epitopes were chosen and further examined by VaxiJen for antigenicity; only 52 epitopes were antigenic, of which 24 were non-allergen. All the epitopes were non-toxins. Five epitopes were predicted by Innovagen to be poorly soluble and were deleted while overlapping ones were also removed. Finally, 10 epitopes listed in Table 2 were chosen for a downward analysis.
Population coverage
Population coverage analysis by IEDB was performed for the shortlisted epitopes to estimate their binding probability to MHC molecules covering the world population (35). World population coverage calculated for MHC class I was 98.55% (Figure 3A) and for MHC class II was 81.81% (Figure 3B). The combined population coverage for MHC molecules was 99.74% as in Figure 3C. MHC class combined world population coverage was also computed region-wise, as shown in Figure 4.
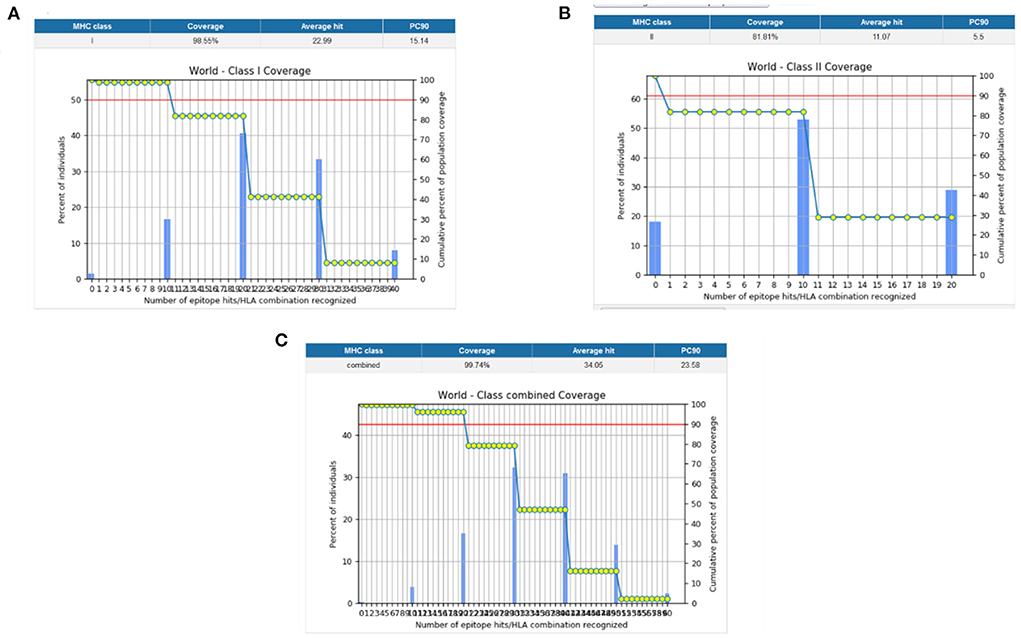
Figure 3. (A) MHC class I world population coverage (B) MHC class II world population coverage (C) MHC class I and II combined world population coverage.
Designing the vaccine constructs and 3D modeling
The 10 shortlisted epitopes that were antigenic, non-allergen, non-toxic, and soluble were considered as potential epitopes to be a part of the vaccine construct. Epitopes were chosen using GPGPG linkers. We designed four constructs using four different adjuvants like TLR-4 agonist, B-defensin, Cholera B toxin (CTB), and 50 s ribosomal adjuvant. The rationale for using different adjuvant molecules was to check which adjuvants show the best compatibility with the designed vaccine molecule and generate strong and protective immune responses. The designed constructs were then evaluated for their physiochemical properties, antigenicity, allergenicity, adhesion probability, and prediction of secondary structure on four parameters (Figure 5). The construct with the adjuvant TLR4 agonist was marked as allergen while the construct with adjuvant 50S ribosomal had an adhesion probability of 0.3 which was less than threshold 0.5 so they both were eliminated. The remaining vaccine constructs one with the adjuvant B-defensin and the other with Cholera B toxin were compared. The vaccine construct with the adjuvant CTB was shortlisted based on its secondary structure being stable. We linked MALP-2 (macrophage activating lipoprotein 2) with construct via the EAAAK linker at the end presumed to be involved in enhancing the antigenicity of the construct. The CTB vaccine construct along with MALP 2 was again evaluated for physiochemical properties thus having a molecular weight of 29.01 kDa, theoretical index of 8.92, −0.65 GRAVY, and stable with a 23.28 instability index. It was non-allergen and antigen with antigenicity of 1.0059.
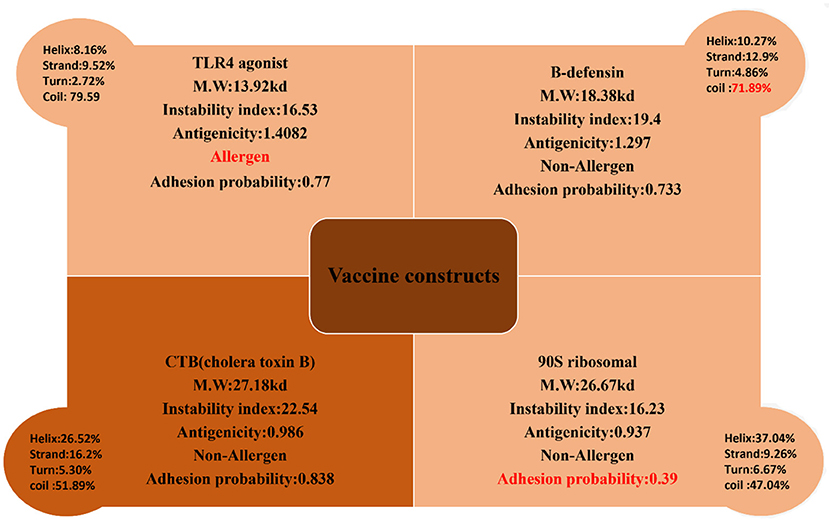
Figure 5. Four constructs with different adjuvant were analyzed for their physiochemical properties and secondary structure parameters. One construct with a TLR4 agonist was an allergen, the construct with B-defensin had a higher number of coils depicting the unstable structure, and the construct with 90S ribosomal had a very low adhesion probability; these were discarded. The construct with CTB met the criteria of a potential vaccine.
The finalized vaccine construct was modeled into a 3D structure (Figure 6A) using the Scratch predictor and visualized by UCSF chimera which highlights the loop regions in the structure. The structure was loop modeled by the Galaxy loop of the Galaxy web and was refined by the Galaxy refine of the Galaxy web. The top 10 galaxy refined models along with their structural information are shown in Table 3. 2D structure analysis and structural validation were done through PDBSum Generate tool (35). Figure 6B is highlighting the secondary structure elements of the vaccine model having 13 helices, 6 helix-helix, 46 beta turns, and 3 gamma turns. Ramachandran plot as shown in Figure 6C confirmed the presence of 199 residues in the Rama favored region, 14 were in additional allowed and 1 was in disallowed region, hence validating a good model for the vaccine.
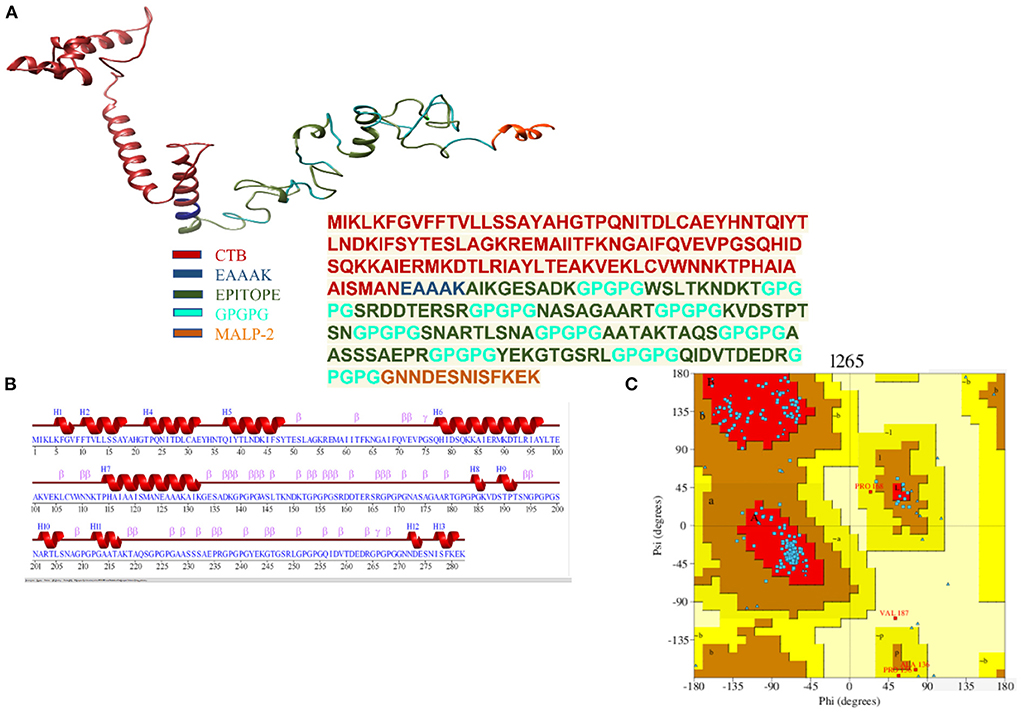
Figure 6. (A) Depiction of the details of 3D model of the multi-epitope vaccine with peptide sequence for the vaccine construct. (B) Secondary structure for the multi-epitope vaccine. (C) Ramachandran plot showing 92.6% of the residue in most favorable region.
Cabs-flex analysis
AGGRESCAN analysis was performed before a cabs-flex analysis (cabs-flex obtained model is shown in Figure 7A). The vaccine model was first subjected to AGGRESCAN for aggregation-prone regions. The residues having scores <0 are considered soluble while a positive value depicts the aggregations-prone residues as in Figure 7B. This step is followed by cabs-flex resulting in 10 models. The highest RSMF obtained was 7.117 angstrom for residue 282, and the lowest was 0.297 angstroms for residue 93 (Figure 7C).
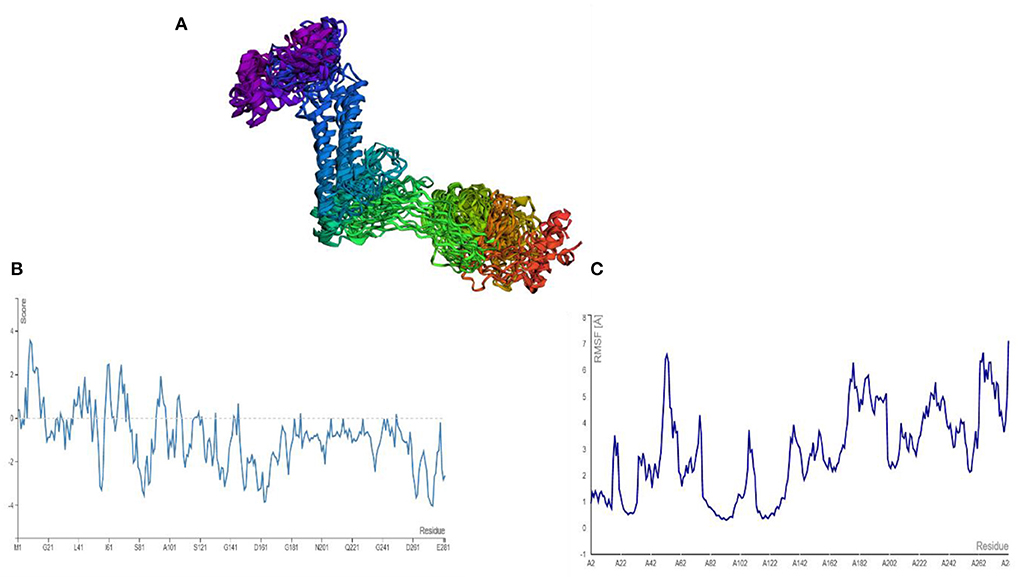
Figure 7. (A) Predicted models after a cabs-flex analysis. (B) AGGRESCAM plot which depicts that the residues with a score above zero are aggregation prone residues. (C) Cabs-flex fluctuations plot, fluctuations calculated as RSMF.
Disulfide engineering and in-silico cloning
The presence of disulfide bonds in any model confirms its structural stability. Disulfide engineering refers to marking the residues in the model as being considered unstable and mutating them as cysteine pairs. There were 18 cysteine pairs identified (Figure 8A). Mutated cysteine pairs included 3LYS-36THR (energy value 5.71 kcal/mol, X3 angle +121.45), 6PHE-12VAL (energy value 3.60 kcal/mol, X3 angle +115.46), 16SER-27THR (energy value 1.33 kcal/mol, X3 angle +96.65), 105LYS-108VAL (energy value 3.11 kcal/mol, X3 angle +95.23), 109TRP-112LYS (energy value 2.7 kcal/mol, X3 angle +119.05), 130ALA-140PRO (energy value 4.85 kcal/mol, X3 angle +88.29), 160ASP-163GLU (energy value 2.57 kcal/mol, X3 angle +107.9), 168PRO-182PRO (energy value 4.5 kcal/mol, X3 angle +103.79), 173ALA-178ALA (energy value 1.41 kcal/mol, X3 angle +85.64), 186LYS-192THR (energy value 5.21 kcal/mol, X3 angle +89.68), 195GLY-216THR (energy value 1.92 kcal/mol, X3 angle −107.05), 206SER-256GLN (energy value 3.21 kcal/mol, X3 angle +98.68), 208ALA-215ALA (energy value 6.31 kcal/mol, X3 angle −64.05), 218LYS-227GLY (energy value 2.89 kcal/mol, X3 angle −70.65), 238PRO-243GLU (energy value 2.93 kcal/mol, X3 angle −103.67), 245GLY-255GLY (energy value 5.57 kcal/mol, X3 angle −79.03), 246THR-266PRO (energy value 5.16 kcal/mol, X3 angle +73.56), and 275SER-278SER (energy value 3.07 kcal/mol, X3 angle −91.98).
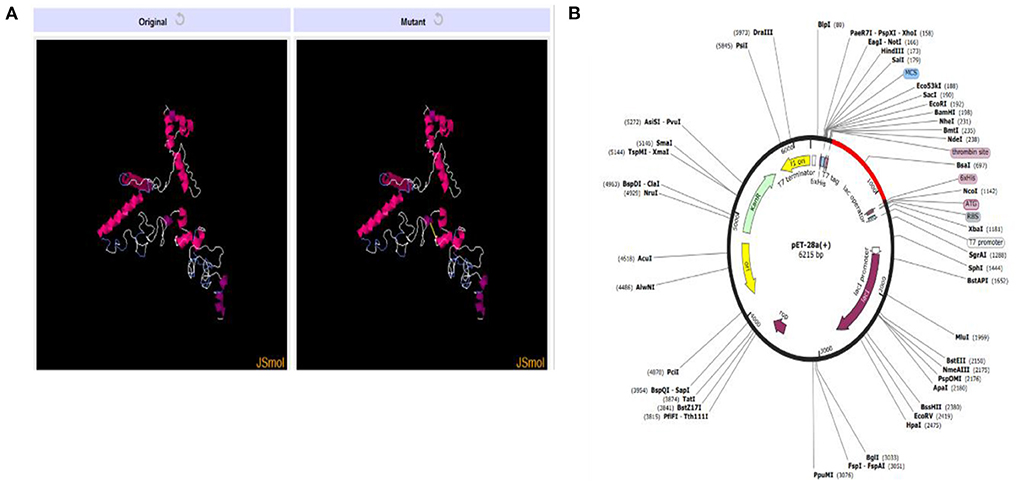
Figure 8. (A) Original and mutant vaccine model obtained by disulfide engineering. (B) Vaccine (in red) cloned in pET28a (+) vector.
In-silico cloning for the vaccine construct was also performed following the codon adaptation in which the sequence for the vaccine construct was reverse-translated. The CAI value obtained for the sequence was 1.0 and the calculated GC content was 53.66%. The expression system was E. coli K12 and the vaccine sequence was expressed in expression vector pET-28a (+) colored red in Figure 8B. The reverse translated sequence being cloned in the vector was: ATGATCAAACTGAAATTCGGTGTTTTCTTCACCGTTCTGCTGTCTTCTGCTTACGCTCACGGTACCCC
GCAGAACATCACCGACCTGTGCGCTGAATACCACAACACCCAGATCTACACCCTGAACGACAAAAT
CTTCTCTTACACCGAATCTCTGGCTGGTAAACGTGAAATGGCTATCATCACCTTCAAAAACGGTGCT
ATCTTCCAGGTTGAAGTTCCGGGTTCTCAGCACATCGACTCTCAGAAAAAAGCTATCGAACGTATGA
AAGACACCCTGCGTATCGCTTACCTGACCGAAGCTAAAGTTGAAAAACTGTGCGTTTGGAACAACA
AAACCCCGCACGCTATCGCTGCTATCTCTATGGCTAACGAAGCTGCTGCTAAAGCTATCAAAGGTGA
ATCTGCTGACAAAGGTCCGGGTCCGGGTTGGTCTCTGACCAAAAACGACAAAACCGGTCCGGGTCC
GGGTTCTCGTGACGACACCGAACGTTCTCGTGGTCCGGGTCCGGGTAACGCTTCTGCTGGTGCTGCT
CGTACCGGTCCGGGTCCGGGTAAAGTTGACTCTACCCCGACCTCTAACGGTCCGGGTCCGGGTTCTA
ACGCTCGTACCCTGTCTAACGCTGGTCCGGGTCCGGGTGCTGCTACCGCTAAAACCGCTCAGTCTGG
TCCGGGTCCGGGTGCTGCTTCTTCTTCTGCTGAACCGCGTGGTCCGGGTCCGGGTTACGAAAAAGG
TACCGGTTCTCGTCTGGGTCCGGGTCCGGGTCAGATCGACGTTACCGACGAAGACCGTGGTCCGGGT
CCGGGTGGTAACAACGACGAATCTAACATCTCTTTCAAAGAAAAA.
Computational immune simulation
We used a C-ImmSim online webserver for the computational immune simulation demonstrating the immune responses expected against the designed multi-epitopes vaccine. Production of immunoglobulins and interleukins proved the effectiveness of the vaccine. Figure 9A shows that IgM production is 700,000 and IgM + IgG production is 10,000 in the first 15 days. Figure 9B is depicting the interleukins induction in response to the vaccine. The amount of IFN-g generated was >400,000 ng/ml. IFN-g levels are higher, indicating that the human immune system is activated (36).
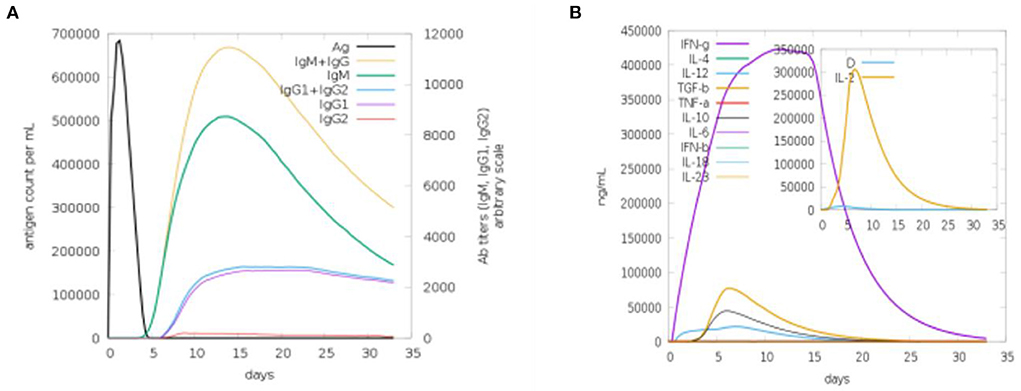
Figure 9. Computational immune simulation against the vaccine model. (A) Induction of immunoglobulins. (B) Induction of interleukins.
Molecular docking of vaccine
The designed vaccine was docked with the receptor TLR 5. TLR5 recognizes the flagellin of the bacterium and is highly expressed in patients infected with B. pseudomallei (37). TLRs are involved in inducing immunity and cytokine induction. Docking refers to the prediction of interactions between ligand (vaccine) and the receptor (TLR 5) (38). Here, the docked models were obtained from the ClusPro server and the top 10 are shown in Table 4. These models are arranged based on their cluster size. The clustering step was done using the pairwise IRMSD (39). The best docked complex obtained is shown in Figure 10A. The interactions between the vaccine (chain A) and TLR-5 (chain B) in a docked complex are presented in Figure 10B. The interactions in red color are salt bridges, while those in yellow are disulfide, and hydrogen bonds are in blue color. The non-bonded interactions are in orange.
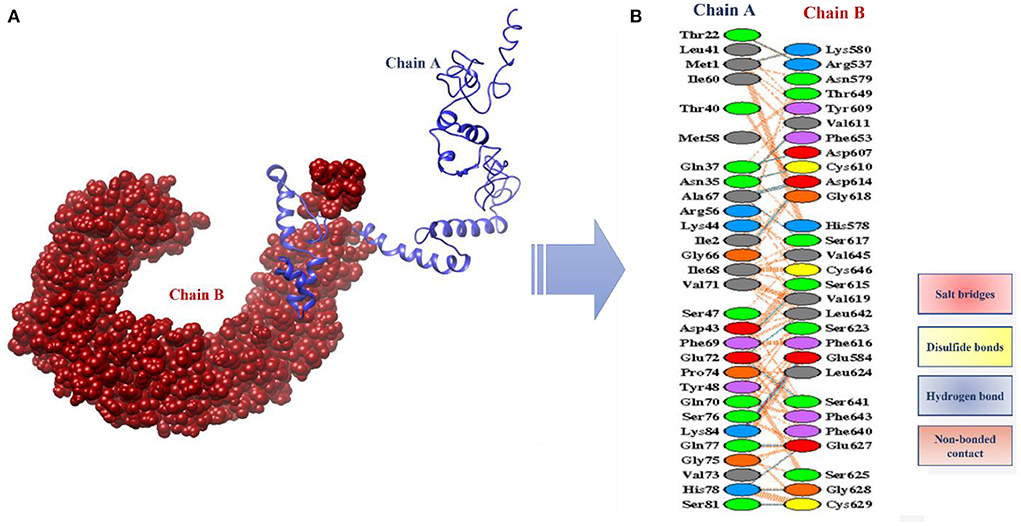
Figure 10. (A) Docked complex of vaccine model and TLR5 (B) Interactions between the chain A (vaccine) and the chain B (TLR5).
Molecular dynamics simulation analysis and binding free energies estimation
The stability and dynamic behavior of the docked Vaccine-TLR-5 complex was further assessed through molecular dynamics simulation analysis. The root-mean-square deviation (RMSD), root-mean-square fluctuation (RMSF), and radius of gyration (RoG) were computed for the system. RMSD analysis during simulation revealed that the complex reaches 7Å at 10.5 ns, however, this is due to the presence of loops. The graph became stable and showed maximum stability toward the end of simulation time as presented in Figure 11A. Next, in RMSF analysis the docked complex was analyzed to check the residue level fluctuations. In RMSF analysis, very low-level fluctuations were observed throughout the simulation time. The few fluctuations were due to the reason for vaccine adjustment at the proper docked site. However, these fluctuations did not affect the overall stability and binding mode of the vaccine to the receptor as shown in Figure 11B. Moreover, the intermolecular stability of the vaccine-TLR-5 can be also witnessed by the radius of gyration analysis, which predicted the maximum compactness of the complex as mentioned in Figure 11C. Additionally, the MM-GBSA analysis was utilized to estimate vaccine-TLR-5 complex free binding energies. The overall binding affinity of the vaccine-TLR-5 complex was a total of −168.35 kcal/mol. The net van der Waals and electrostatic energies are the most favorable in the docked complex formation. The gas phase energy of the complex dominates the overall energy of the system while polar energy is non-favorable in complex formation. The terms for different binding free energies are tabulated in Table 5.
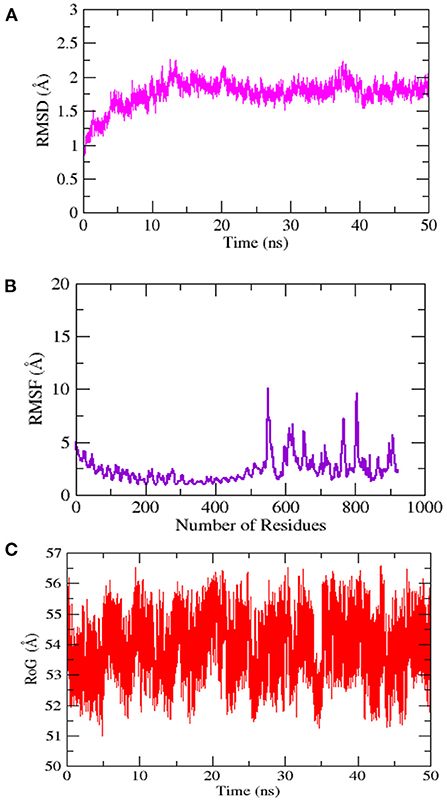
Figure 11. Simulation trajectories analysis (A) Root-mean-square deviation RMSD (B) Root-mean-square fluctuation (RMSF) and (C) Radius of gyration (RoG).
Discussion
B. pseudomallei is a gram-negative bacterium that causes melioidosis and can be lethal if not adequately treated. It can sometimes act as a facultative intracellular pathogen, emphasizing the pathogenesis of the infection (40). Long medications can be used to treat the infection; however, reports have shown that the bacterium has developed resistance to several antibiotics. Many vaccines against this infection were designed and some of them are in preclinical trials as well. Here, we applied cost-effective immunoinformatic and reverse vaccinology approaches to design a multi-epitope vaccine against B. pseudomallei. Immunoinformatic approaches for vaccine designing can develop effective vaccines in less time and can provoke both innate and humoral immune responses (40). The previous vaccine development techniques are considered less capable as compared to multi-epitope vaccines (41). Core proteome was retrieved from 91 B. pseudomallei strains and subjected to various filters resulting in potential vaccine candidates. This was done via a subtractive proteomics approach in which desired proteins were filtered (42, 43). The extracellular, periplasmic, and outer membrane protein sequences were used to design a multi-epitope vaccine. These are the surface proteins involved in bacterial pathogenesis and are very crucial in the development of vaccines (42, 43). These proteins also play a role in pathogen attachment, cell entrance, and disease prognosis (44). The virulence of a protein is very important for disease development (45). These proteins were checked for their capability to be a part of the vaccine and went through various filters like allergenicity, antigenicity, adhesion probability, and solubility. The shortlisted vaccine proteins were used in epitope mapping. B-cell derived T-cell epitopes were used in vaccine construct as B- and T-cells play vital roles in inducing cell-mediated immunity against the pathogen (46). Predicted epitopes were prioritized and shortlisted for vaccine designing. Similar studies were also conducted against Acinetobacter baumannii (18), Enterobacter hormaechei (16), and Enterobacter xiangfangensis (17) etc. These studies prioritized several vaccine targets against the pathogens and proposed chimeric epitope peptides that can better induce humoral and cellular immunity.
The vaccine construct was designed by linking the epitopes with GPGPG linkers and joining the epitope to the adjuvant via the EAAAK linker. The purpose of utilizing linkers is to facilitate the separation of epitopes and adjuvants while avoiding overlaps (46, 47). Four different adjuvants were utilized, and the final construct with adjuvant CTB was 3D modeled and subjected to further analysis. CTB is a non-toxic part of Cholera toxin which, when linked to antigen, results in boosting the immunity and immune responses (48). CTB is associated with the induction of CD4+ T-cell immune activation (49). The vaccine model was visualized through Chimera to identify the loop regions followed by loop modeling and refinement. Loop modeling is involved in predicting secondary structure elements for the loop. Loop regions play a crucial role in several biochemical functions (49, 50). The structure of the vaccine model was improved by refinement because some structures are not predicted accurately, hindering the protein from function properly, and causing difficulty in structure-based research (49, 50). Structural validation of the 3D model was done, and the Ramachandran plot (51) explained the stability of the structure having 92.6% of its residues in the most favored regions. Disulfide engineering and codon optimization were important steps performed to improve structural stability (52). The main aim of the codon optimization was to increase the vaccine's expression level resulting in its higher efficiency for experimental research (18). To generate proper immune responses, the designed vaccine construct should have the binding capability with immune cell receptors. Among immune cells receptors, the TLRs family plays a vital role in the generation of proper immune responses.
In this study, we performed a docking analysis to assess the vaccine's binding potency with TLR-5. The server generates 10 complexes with different binding energy scores. From the docking analysis, the designed vaccine can interact with TLR-5 molecules and can provoke proper immune reactions. For long-term immunity, the docking stability of the docked complexes must be maintained. To evaluate the dynamic movement of the docked complex, a molecular dynamics simulation approach was performed. The results of in-silico host immune simulation revealed that the chimeric vaccine can induce a proper immune response in the host body. The immune response was observed in the form of different antibodies and cytokines. According to our findings, the proposed vaccine is promising and capable of eliciting a proper immune response against B. pseudomallei.
Conclusions
Antibiotic abuse in animals, humans, and agriculture has resulted in the emergence of antibiotic-resistant bacterial infections, which has significantly raised hospitalization, community mobility, and mortality rates. Melioidosis, a condition that can be fatal, is caused by B. pseudomallei. There is no licensed vaccination to protect against B. pseudomallei infection. Immunoinformatics, bioinformatics, and reverse vaccinology were employed to speed up vaccine target discovery while also lowering costs and saving time. In the current research, a multi-epitopes-based vaccine was designed that may produce innate and adaptive immunity against B. pseudomallei using a variety of bioinformatics, immunoinformatic, and reverse vaccinology methodologies. For the above-said purpose, the first proteins were shortlisted from pathogen core proteins and utilized for probable antigenic, non-allergic, non-toxic, and good water-soluble epitopes prediction. Several analyses demonstrate that the developed vaccine model is capable of inducing a proper innate and adaptive immune response against the target pathogen. However, wet laboratory confirmation and validations are strongly advised to validate in-silico findings. Although the in-silico results are encouraging, additional efforts are required, such as the use of more accurate servers/tools to validate the results.
Data availability statement
Publicly available datasets were analyzed in this study. This data can be found here: NCBI GenBank.
Author contributions
All authors listed have made a substantial, direct, and intellectual contribution to the work and approved it for publication.
Acknowledgments
The researcher would like to thank the Deanship of Scientific Research, Qassim University for funding the publication of this project.
Conflict of interest
The authors declare that the research was conducted in the absence of any commercial or financial relationships that could be construed as a potential conflict of interest.
Publisher's note
All claims expressed in this article are solely those of the authors and do not necessarily represent those of their affiliated organizations, or those of the publisher, the editors and the reviewers. Any product that may be evaluated in this article, or claim that may be made by its manufacturer, is not guaranteed or endorsed by the publisher.
References
1. Choh LC, Ong GH, Vellasamy KM, Kalaiselvam K, Kang WT, Al-Maleki AR, et al. Burkholderia vaccines: are we moving forward? Front Cell Infect Microbiol. (2013) 4:5. doi: 10.3389/fcimb.2013.00005
2. Wiersinga WJ, Virk HS, Torres AG, Currie BJ, Peacock SJ, Dance DAB, et al. Melioidosis. Nat Rev Dis Primers. (2018) 4:1–22. doi: 10.1038/nrdp.2017.107
3. Kaestli M, Schmid, M, Mayo M, Rothballer M, Harrington G, et al. Out of the Ground: Aerial and Exotic Habitats of the Melioidosis Bacterium Burkholderia Pseudomallei in Grasses in Australia. Environ Microbiol. (2012) 14:2058–70. doi: 10.1111/j.1462-2920.2011.02671.x
4. Pearson T, Giffard P, Beckstrom-Sternberg S, Auerbach R, Hornstra H, et al. Phylogeographic Reconstruction of a Bacterial Species with High Levels of Lateral Gene Transfer. BMC Biol. (2009) 7:1–14. doi: 10.1186/1741-7007-7-78
5. Limmathurotsakul D, Golding N, Dance DAB, Messina JP, Pigott DM, Moyes CL, et al. Predicted global distribution of burkholderia pseudomallei and burden of melioidosis. Nat Microbiol. (2016) 1:1–5. doi: 10.1038/nmicrobiol.2015.8
6. Limmathurotsakul D, Wongratanacheewin S, Teerawattanasook N, Wongsuvan G, Chaisuksant S, Chetchotisakd P, et al. Increasing incidence of human melioidosis in northeast Thailand. Am J Trop Med Hyg. (2010) 82:1113. doi: 10.4269/ajtmh.2010.10-0038
7. Currie BJ, Dance DAB, Cheng AC. The global distribution of burkholderia pseudomallei and melioidosis: an update. Trans R Soc Trop Med Hyg. (2008) 102:S1–4. doi: 10.1016/S0035-9203(08)70002-6
8. Currie BJ, Ward L, Cheng AC. The epidemiology and clinical spectrum of melioidosis: 540 cases from the 20 year darwin prospective study. PLoS Negl Trop Dis. (2010) 4:e900. doi: 10.1371/journal.pntd.0000900
9. Birnie E, Virk HS, Savelkoel J, Spijker R, Bertherat E, Dance DAB, et al. Global burden of melioidosis in 2015: a systematic review and data synthesis. Lancet Infect Dis. (2019) 19:892–902. doi: 10.1016/S1473-3099(19)30157-4
10. Limmathurotsakul D, Peacock SJ. Melioidosis: a clinical overview. Br Med Bull. (2011) 99:125–39. doi: 10.1093/bmb/ldr007
11. Cheng AC, Currie BJ. Melioidosis: epidemiology, pathophysiology, and management. Clin Microbiol Rev. (2005) 18:383. doi: 10.1128/CMR.18.2.383-416.2005
12. Wiersinga WJ, Wieland CW, Dessing MC, Chantratita N, Cheng AC, Limmathurotsakul D, et al. Toll-like receptor 2 impairs host defense in gram-negative sepsis caused by burkholderia (Melioidosis). PLoS Med. (2007) 4:1268–80. doi: 10.1371/journal.pmed.0040248
13. Hamad MA, Austin CR, Stewart AL, Higgins M, Vázquez-Torres A, Voskuil MI. Adaptation and antibiotic tolerance of anaerobic burkholderia pseudomallei. Antimicrob Agents Chemother. (2011) 55:3313–23. doi: 10.1128/AAC.00953-10
14. Morici L, Torres AG, Titball RW. Novel multi-component vaccine approaches for Burkholderia Pseudomallei. Clin Exp Immunol. (2019) 196:178–88. doi: 10.1111/cei.13286
15. Chantratita N, Tandhavanant S, Myers ND, Chierakul W, Robertson JD, Mahavanakul W, et al. Screen of whole blood responses to flagellin identifies TLR5 variation associated with outcome in melioidosis. Genes Immunity. (2013) 15:63–71. doi: 10.1038/gene.2013.60
16. Albekairi TH, Alshammari A, Alharbi M, Alshammary AF, Tahir ul Qamar M, Ullah A, et al. Designing of a novel multi-antigenic epitope-based vaccine against e hormaechei: an intergraded reverse vaccinology and immunoinformatics approach. Vaccines (Basel). (2022) 10:665. doi: 10.3390/vaccines10050665
17. Alshammari A, Alharbi M, Alghamdi A, Alharbi SA, Ashfaq UA, Tahir ul Qamar M, et al. Computer-aided multi-epitope vaccine design against enterobacter xiangfangensis. Int J Environ Res Public Health. (2022) 19:7723. doi: 10.3390/ijerph19137723
18. ud-din M, Albutti A, Ullah A, Ismail S, Ahmad S, Naz A, et al. Vaccinomics to design a multi-epitopes vaccine for acinetobacter baumannii. Int J Environ Res Public Health. (2022) 19:5568. doi: 10.3390/ijerph19095568
19. Chaudhari NM, Gupta VK, Dutta C. BPGA- an ultra-fast pan-genome analysis pipeline. Sci Rep. (2016) 6:1–10. doi: 10.1038/srep24373
20. Gardy JL, Spencer C, Wang K, Ester M, Tusnády GE, Simon I, et al. PSORT-B: Improving protein subcellular localization prediction for gram-negative bacteria. Nucleic Acids Res. (3613) 2003:31. doi: 10.1093/nar/gkg602
21. He Y, Xiang Z, Mobley HLT. Vaxign: The first web-based vaccine design program for reverse vaccinology and applications for vaccine development. J Biomed Biotechnol. (2010) 2010:297505. doi: 10.1155/2010/297505
22. Dimitrov I, Flower DR, Doytchinova I. AllerTOP - a server for in silico prediction of allergens. BMC Bioinform. (2013) 14:1–9. doi: 10.1186/1471-2105-14-S6-S4
23. Fleri W, Paul S, Dhanda SK, Mahajan S, Xu X, Peters B, et al. The immune epitope database and analysis resource in epitope discovery and synthetic vaccine design. Front Immunol. (2017) 8:278. doi: 10.3389/fimmu.2017.00278
24. ProtParam - SIB Swiss Institute of Bioinformatics | Expasy. Available online at: https://www.expasy.org/resources/protparam (accessed 24 February, 2022).
25. Cheng J, Randall AZ, Sweredoski MJ, Baldi P. SCRATCH A protein structure and structural feature prediction server. Nucleic Acids Res. (2005) 33:W72. doi: 10.1093/nar/gki396
26. Kuriata A, Gierut AM, Oleniecki T, Ciemny MP, Kolinski A, Kurcinski M, et al. CABS-flex 20: a web server for fast simulations of flexibility of protein structures. Nucleic Acids Res. (2018) 46:W338–43. doi: 10.1093/nar/gky356
27. Craig DB, Dombkowski AA. Disulfide by design 20: a web-based tool for disulfide engineering in proteins. BMC Bioinform. (2013) 14:1–7. doi: 10.1186/1471-2105-14-346
28. Grote A, Hiller K, Scheer M, Münch R, Nörtemann B, Hempel DC, et al. JCat: a novel tool to adapt codon usage of a target gene to its potential expression host. Nucleic Acids Res. (2005) 33:W526. doi: 10.1093/nar/gki376
29. SnapGene | Software for Everyday Molecular Biology. Available online at: https://www.snapgene.com/ (accessed 24 February 2022).
30. Rapin N, Lund O, Bernaschi M, Castiglione F. Computational immunology meets bioinformatics: the use of prediction tools for molecular binding in the simulation of the immune system. PLoS ONE. (2010) 5:e9862. doi: 10.1371/journal.pone.0009862
31. ClusPro 2.0: Protein-Protein Docking. Available online at: https://cluspro.bu.edu/login.php?redir=/queue.php (accessed 24 February 2022).
32. Zhou Y, Tao J, Yu H, Ni J, Zeng L, Teng Q, et al. Hcp family proteins secreted via the type VI secretion system coordinately regulate escherichia coli K1 interaction with human brain microvascular endothelial cells. Infect Immun. (2012) 80:1243. doi: 10.1128/IAI.05994-11
33. Wang J, Yang Y, Cao Z, Li Z, Zhao H, Zhou Y. The role of semidisorder in temperature adaptation of bacterial FlgM proteins. Biophys J. (2013) 105:2598–605. doi: 10.1016/j.bpj.2013.10.026
34. Hung WC, Jane WN, Wong HC. Association of a D-Alanyl-D-Alanine carboxypeptidase gene with the formation of aberrantly shaped cells during the induction of viable but nonculturable vibrio parahaemolyticus. Appl Environ Microbiol. (2013) 79:7305–12. doi: 10.1128/AEM.01723-13
35. Laskowski RA, Jabłońska J, Pravda L, Vareková RS, Thornton JM. PDBsum: structural summaries of PDB entries. Protein Sci. (2018) 27:129. doi: 10.1002/pro.3289
36. Birnie E, Weehuizen TAF, Lankelma JM, de Jong HK, Koh GCKW, van Lieshout MHP, et al. Role of Toll-like Receptor 5 (TLR5) in experimental melioidosis. Infect Immun. (2019) 87:e00409–18. doi: 10.1128/IAI.00409-18
37. Desta IT, Porter KA, Xia B, Kozakov D, Vajda S. Performance and its limits in rigid body protein-protein docking. Structure. (2020) 28:1071. doi: 10.1016/j.str.2020.06.006
38. Kozakov D, Hall DR, Xia B, Porter KA, Padhorny D, Yueh C, et al. The ClusPro web server for protein–protein docking. Nat Protoc. (2017) 12:255–78. doi: 10.1038/nprot.2016.169
39. Harley VS, Dance DAB, Drasar BS, Tovey G. Effects of burkholderia pseudomallei and other burkholderia species on eukaryotic cells in tissue culture. Microbios. (1998) 96:71–93.
40. Tahir Ul, Qamar M, Ismail S, Ahmad S, Mirza MU, Abbasi SW, et al. Development of a Novel multi-epitope vaccine against crimean-congo hemorrhagic fever virus: an integrated reverse vaccinology, vaccine informatics and biophysics approach. Front Immunol. (2021) 12:1. doi: 10.3389/fimmu.2021.669812
41. Ismail S, Shahid F, Khan A, Bhatti S, Ahmad S, Naz A, et al. Pan-vaccinomics approach towards a universal vaccine candidate against WHO priority pathogens to address growing global antibiotic resistance. Comput Biol Med. (2021) 136:104705. doi: 10.1016/j.compbiomed.2021.104705
42. Moxon R, Reche PA, Rappuoli R. Editorial: reverse vaccinology. Front Immunol. (2019) 10:2776. doi: 10.3389/fimmu.2019.02776
43. Grandi G. Bacterial surface proteins and vaccines. F1000 Biol Rep. (2010) 2:36. doi: 10.3410/B2-36
44. Naz A, Awan FM, Obaid A, Muhammad SA, Paracha RZ, Ahmad J, et al. Identification of putative vaccine candidates against helicobacter pylori exploiting exoproteome and secretome: a reverse vaccinology based approach. Infect Genet Evol. (2015) 32:280–91. doi: 10.1016/j.meegid.2015.03.027
45. Bonilla FA, Oettgen HC. Adaptive immunity. J Allergy Clin Immunol. (2010) 125:S33–40. doi: 10.1016/j.jaci.2009.09.017
46. Multiepitope Subunit Vaccine Design against COVID-19 Based on the Spike Protein of SARS-CoV-2: An in silico analysis Available online at: https://www.hindawi.com/journals/jir/2020/8893483/#discussion (accessed 27 April 2022).
47. Meza B, Ascencio F, Sierra-Beltrán AP, Torres J, Angulo CA. Novel design of a multi-antigenic, multistage and multi-epitope vaccine against helicobacter pylori: an in silico approach infection. Genet Evol. (2017) 49:309–17. doi: 10.1016/j.meegid.2017.02.007
48. Stratmann T. Cholera toxin subunit b as adjuvant—-an accelerator in protective immunity and a break in autoimmunity. Vaccines. (2015) 3:579–96. doi: 10.3390/vaccines3030579
49. Sun JB, Czerkinsky C, Holmgren J. Mucosally induced immunological tolerance, regulatory T cells and the adjuvant effect by cholera toxin B subunit. Scand J Immunol. (2010) 71:1–11. doi: 10.1111/j.1365-3083.2009.02321.x
50. Heo L, Park H, Seok C. GalaxyRefine: protein structure refinement driven by side-chain repacking. Nucleic Acids Res. (2013) 41:W384. doi: 10.1093/nar/gkt458
51. The Ramachandran Plot and Protein Structure Validation | Biomolecular Forms and Functions. Available online at: https://www.worldscientific.com/doi/abs/10.1142/9789814449144_0005 (accessed 27 April 2022).
Keywords: Burkholderia pseudomallei, melioidosis, immunoinformatics, molecular dynamics simulation, vaccine
Citation: Alsowayeh N and Albutti A (2022) Designing a novel chimeric multi-epitope vaccine against Burkholderia pseudomallei, a causative agent of melioidosis. Front. Med. 9:945938. doi: 10.3389/fmed.2022.945938
Received: 17 May 2022; Accepted: 20 September 2022;
Published: 18 October 2022.
Edited by:
Adriana Harbuzariu, Emory University, United StatesReviewed by:
Ahmed A. Al-Karmalawy, Horus University, EgyptMuhammad Alaa Eldeen, Zagazig University, Egypt
Hamidreza Majidiani, Neyshabur University of Medical Sciences, Iran
Copyright © 2022 Alsowayeh and Albutti. This is an open-access article distributed under the terms of the Creative Commons Attribution License (CC BY). The use, distribution or reproduction in other forums is permitted, provided the original author(s) and the copyright owner(s) are credited and that the original publication in this journal is cited, in accordance with accepted academic practice. No use, distribution or reproduction is permitted which does not comply with these terms.
*Correspondence: Noorah Alsowayeh, n.alsowayeh@mu.edu.sa; Aqel Albutti, as.albutti@qu.edu.sa
†These authors have contributed equally to this work