- 1School of Computer Science and Mathematics, Liverpool John Moores University, Liverpool, United Kingdom
- 2Liverpool Centre for Cardiovascular Science, Liverpool, United Kingdom
- 3Institute of Life Course and Medical Sciences, University of Liverpool, Liverpool, United Kingdom
- 4Liverpool University Hospitals NHS Foundation Trust, Liverpool, United Kingdom
Background: Sepsis is a life-threatening disease commonly complicated by activation of coagulation and immune pathways. Sepsis-induced coagulopathy (SIC) is associated with micro- and macrothrombosis, but its relation to other cardiovascular complications remains less clear. In this study we explored associations between SIC and the occurrence of atrial fibrillation (AF) in patients admitted to the Intensive Care Unit (ICU) in sinus rhythm. We also aimed to identify predictive factors for the development of AF in patients with and without SIC.
Methods: Data were extracted from the publicly available AmsterdamUMCdb database. Patients with sepsis and documented sinus rhythm on admission to ICU were included. Patients were stratified into those who fulfilled the criteria for SIC and those who did not. Following univariate analysis, logistic regression models were developed to describe the association between routinely documented demographics and blood results and the development of at least one episode of AF. Machine learning methods (gradient boosting machines and random forest) were applied to define the predictive importance of factors contributing to the development of AF.
Results: Age was the strongest predictor for the development of AF in patients with and without SIC. Routine coagulation tests activated Partial Thromboplastin Time (aPTT) and International Normalized Ratio (INR) and C-reactive protein (CRP) as a marker of inflammation were also associated with AF occurrence in SIC-positive and SIC-negative patients. Cardiorespiratory parameters (oxygen requirements and heart rate) showed predictive potential.
Conclusion: Higher INR, elevated CRP, increased heart rate and more severe respiratory failure are risk factors for occurrence of AF in critical illness, suggesting an association between cardiac, respiratory and immune and coagulation pathways. However, age was the most dominant factor to predict the first episodes of AF in patients admitted in sinus rhythm with and without SIC.
1. Introduction
Sepsis is a potentially life-threatening condition which is complicated by organ dysfunction due to a dysregulated host response to infection (1). During sepsis, invading pathogens are recognized by the innate immune system leading to the release of proinflammatory mediators into the circulation. This in turn triggers endothelial activation and recruitment of immune cells to infection sites. The interaction of innate immune cells, in particular neutrophils and monocytes with extrinsic and contact-dependent coagulation pathways, forms a major part of the body’s response to infection (2). This orchestrated activation of immune, endothelial and coagulation systems, oftendescribed as immunothrombosis, leads to macro- and microthrombotic complications and together with mitochondrial damage, energy depletion andtissue hypoxia results in cardiovascular dysfunction, including, altered myocardial contractility and arrhythmias (3). Atrial Fibrillation (AF) is the most common arrhythmia in critically ill patients with an incidence of 10–15% (4–6) in the general critical care population. In septic shock, the incidence of AF increases to over 40% (4). During sepsis, but also in patients suffering from AF, hypercoagulability is commonly observed. It is therefore warranted to investigate if the occurrence of AF in patients with sepsis is linked to an abnormal coagulation profile.
In sepsis, increased tissue factor expression, down-regulation of natural anticoagulant pathways, and hypofibrinolysis result in increased thrombin generation and clot formation in the macro- and microvasculature. Microvascular thrombosis contributes to tissue hypoxia and organ dysfunction. A recent review reported that Disseminated intravascular coagulation (DIC) is observed in 30–60% of critically ill patients with sepsis (7), depending on the score used for diagnosis. DIC is characterized by the activation of procoagulant and fibrinolytic pathways and results in intravascular micro- and macrothrombosis, which is associated with the consumption of platelets and coagulation factors (7). In later stages, bleeding is a common complication. Specifically for DIC observed in sepsis, the Sepsis-induced Coagulopathy (SIC) score (8) has been developed based on routine coagulation tests including platelet count and prothrombin time ratio together with components of the Sequential Organ Failure Assessment (SOFA) score (9).
In the general population, AF is associated with a prothrombotic state (10). The earlier stages of SIC are also characterized by hypercoagulability, which, however, differs in origin and pathophysiology. Key mechanisms of SIC include release of tissue factor into the circulation by activated monocytes and endothelial cells, impaired fibrinolysis, amplification of procoagulant pathways by cytokines and suppression of anticoagulant pathways. Together with the complement system, platelets trigger the formation of neutrophil extracellular traps (NETs) by activated neutrophils (11). NETs are structures consisting of histones, nuclear DNA and granule proteins secreted by neutrophils. NETs are highly prothrombotic and contribute to the procoagulant state of sepsis.
In critical illness, it remains unclear if the prothrombotic state observed in SIC predisposes patients to episodes of AF. Both sepsis and AF are associated with thrombotic complications including microvascular thrombosis, venous thromboembolic disease and stroke. Formation of microthrombi in small vessels is regarded as a key factor for multiorgan failure in sepsis. The pathophysiological mechanisms that link arrythmias and immunothrombosis have not been fully elucidated.
Given that coagulopathy and arrythmias are commonly observed during sepsis, we hypothesized that the incidence of AF episodes increases in patients with SIC compared to those without. Based on SIC scores we stratified patients in a large European intensive care database to investigate this hypothesis. We also aimed to describe factors associated with and predictive of the occurrence of AF episodes in septic patients admitted to ICU in sinus rhythm, discriminating between patients with SIC and those without.
2. Materials and methods
2.1. Data extraction
Data was extracted from the AmsterdamUMCdb, the first freely accessible European intensive care database and endorsed by the European Society of Intensive Care Medicine (ESICM) (12). Extracted variables included demographic data (e.g., age, gender, weight and height groups), vital signs (e.g., heart rate, respiratory rate, temperature, systolic blood pressure, and oxygen saturation), blood results and variables reflecting respiratory support (e.g., ventilation status, O2 concentration, Positive Endexspiratory Pressure).
Missing values were treated as described before (13), i.e., excluding admissions with more than 35% missing data, and imputing the missing data with the median (numeric variables) or the mode (categorical variables). Variables with dynamic features were converted into tabular representations by extracting their means.
The SIC (8), SOFA (9) and the Acute Physiology And Chronic Health Evaluation II (APACHE II) (14) scores were calculated based on data points for admission to ICU after extracting the respective variables from the database.
2.2. Diagnosis of sepsis
As information regarding the diagnosis of sepsis is not readily available in the AmsterdamUMCdb, we used a previously described method based on the Sepsis-3 definition (15). This enabled us to include patients with a diagnosis of sepsis before admission to ICU. We included patients in whom a diagnosis of sepsis had been coded for a time window reaching from 3 days before ICU admission until day 1 (first 24 h) of ICU admission. This time window was chosen because sepsis-induced coagulopathy is often present before ICU admission and in some cases may resolve early in the ICU stay after treatment of the underlying cause of sepsis (16).
2.3. Diagnosis of Sepsis-induced coagulopathy
The SIC score was calculated using ICU admission data points to classify patients as SIC-positive (SIC score ≥ 4) and SIC-negative (SIC score 0–3) as described previously (8). Patients with incomplete information for the calculation of the SIC score were excluded from this analysis.
2.4. Identification of AF patients
The AmsterdamUMCdb allows the identification of the heart rhythm by hourly recordings. We discriminated AF versus sinus rhythm using this variable. We only included septic patients admitted to ICU in sinus rhythm who later in their ICU stay developed an episode of AF. Variables were extracted until 1 h before the first recorded AF episode for AF patients, whereas for non-AF patients, data were analyzed for the whole ICU stay. We deliberately chose a cut-off of 1 h before the onset of AF to factor in a time window which would be required in clinical practice to conduct preventative measures (e.g., electrolyte supplementation, fluid administration). Different models were built to describe associations of physiological variables with AF episodes and to determine the importance of factors predictive of the occurrence of AF in patients admitted to ICU in sinus rhythm. We developed separate models for the following patient cohorts: all septic patients regardless of SIC status, patients with a diagnosis of SIC (SIC-positive) and patients without a diagnosis of SIC (SIC-negative).
2.5. Disease severity scores
We classified patients according to their APACHE II score into three groups: <25, 25–34, >34. An APACHE II score of 25 represents a predicted hospital mortality of 50% and a score of over 35 represents a predicted hospital mortality of 80%. SOFA classification was chosen to match the mortality estimates described before (17, 18) with scores of 0–6 representing <10% risk, 7–9 representing 15–20% risk, 10–12 representing 40–50%, 13–14 representing 50–60%, and 15 or over representing >80% risk of dying during the ICU stay.
2.6. Univariate and multivariate analysis
Medians and interquartile ranges were calculated for continuous variables, and frequencies and proportions were used for categorical variables. Differences between SIC-positive vs. SIC-negative septic patients were assessed using Kruskal-Wallis rank sum and Chi-square tests.
Multiple logistic regression (LR) was used to describe associations, displayed as odds ratios (OR), between physiological variables and the occurrence of AF (18). Relevant input variables were automatically selected using a sequential forward search algorithm with 3-fold cross-validation.
2.7. Machine learning analysis
The Machine learning (ML) algorithms random forest (RF) and gradient boosting machines (GBM) were used for prediction modeling. Both, RF and GBM are ensemble learning techniques that make their predictions by aggregating the outputs from multiple individual trees. RF repeatedly fits induction trees to many subsets of random samples with replacements extracted from the training set. In classification tasks, RF predicts a new outcome by taking the majority vote (19). Many decision trees are also used by GBM to make predictions, but unlike RF, it implements an iterative learning algorithm such that a new tree model is fitted using the instances where a previous tree performed inadequately (20). Both the RF and the GBM algorithms used the Gini impurity index to determine the ideal split at each node of their decision trees. Since they show how frequently variables were used for the splits, aggregated Gini index values offer a variable importance score (i.e., how relevant they are to the model predictions), which provides a relative rank of relevance/importance of the input variables (19).
Several RF and GBM hyperparameters were tuned using the same validation splits as for LR. For RF models, we tested a range of several variables randomly sampled between 5 and 30 and a range of minimum node size (which controls the depth of the trees) between 3 and 18. For GBM models, a range of shrinkage values (which controls the impact of each additional fitted tree) from 10−3 to 101 and a range of minimum number of observations in a node from 3 to 18 was tested. The RF and GBM variable importance of the models with the best hyperparameter set for each cross-validation cycle was also estimated.
2.8. Model performance
Nested cross-validation was implemented, with the inner iterations to evaluate the variable selection, and the outer iterations to evaluate the training with the selected set of variables. Model performances were measured using the area under the receiver operator characteristic (AUC) curve. We report AUC means and confidence intervals (CI) separately for the overall cohort of septic patients, and for patients with and without SIC.
3. Results
From a total of 23,106 admissions, patients <18 years of age, multiple admissions and cases with >35% missing data were excluded, resulting in 18,518 analyzable cases, of which 5,822 had sepsis recorded within day −3 to day 1 of admission (Figure 1). Of these septic patients, information to calculate the SIC score was available for 1,970 patients, with 922 patients fulfilling SIC criteria and 1,048 patients without a diagnosis of SIC (Figure 1). Of the 922 SIC-positive patients, 286 had an episode of AF, while only 164 of 1,048 patients without SIC developed this complication. A total of 286 septic patients with AF and 636 non-AF septic patients were diagnosed with SIC (SIC score of 4 or more), while 164 patients with AF and 884 non-AF patients were not diagnosed with SIC.
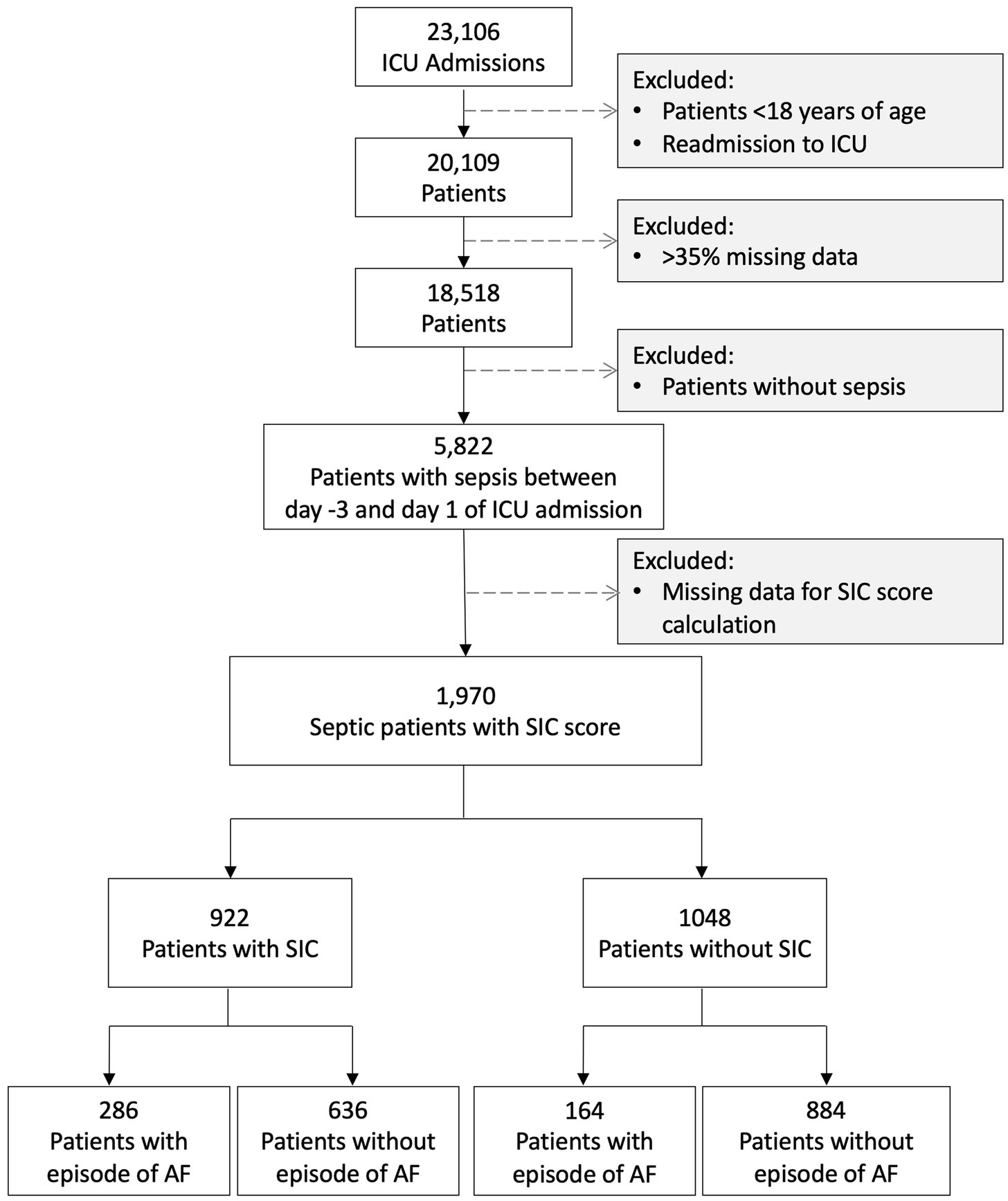
Figure 1. Flowchart describing the cohorts included and excluded from the analysis. ICU, intensive care unit; AF, atrial fibrillation, SIC, Sepsis-induced coagulopathy.
3.1. Univariate comparison between SIC positive and SIC negative patients
ICU mortality was more than twice as high in SIC-positive patients compared to SIC-negative patients (28.5% versus 12.2%, p < 0.001). ICU length of stay was longer in SIC-positive patients, however, this result did not reach statistical significance. We found statistically significant differences in occurrence of AF, blood results, vital signs and blood gas analysis results between SIC-positive and SIC-negative patients (Table 1). SIC-positive patients developed significantly more frequent episodes of AF (286/922 patients, 31%) than patients without SIC (164/1048 patients, 15.6%, p < 0.001). Figure 2 shows the percentage of AF and non-AF patients depending on disease severity as determined by SIC, SOFA and APACHE II. The occurrence of AF increased with higher disease severity.
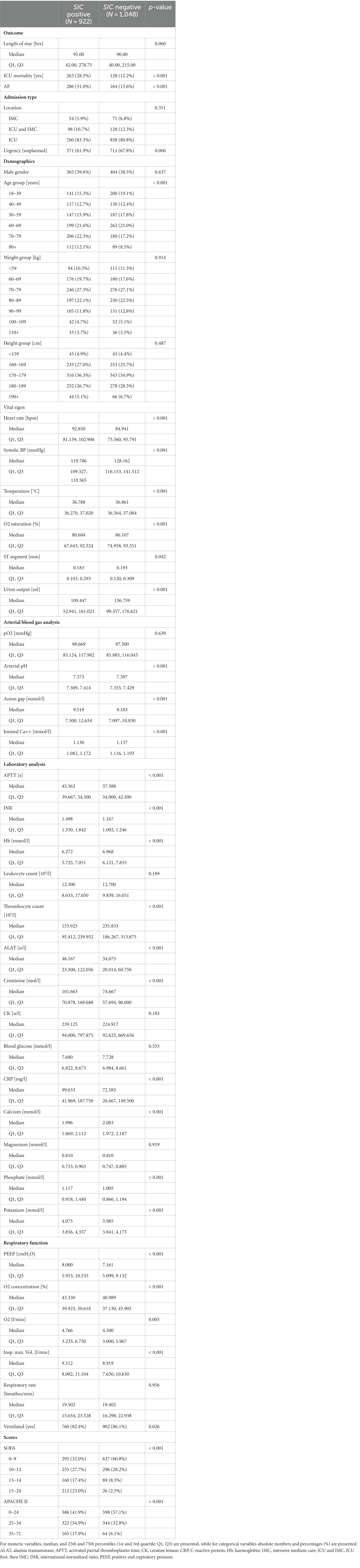
Table 1. Demographics, vital signs, lab results, etc. used for modeling, and routine prognostic scores.
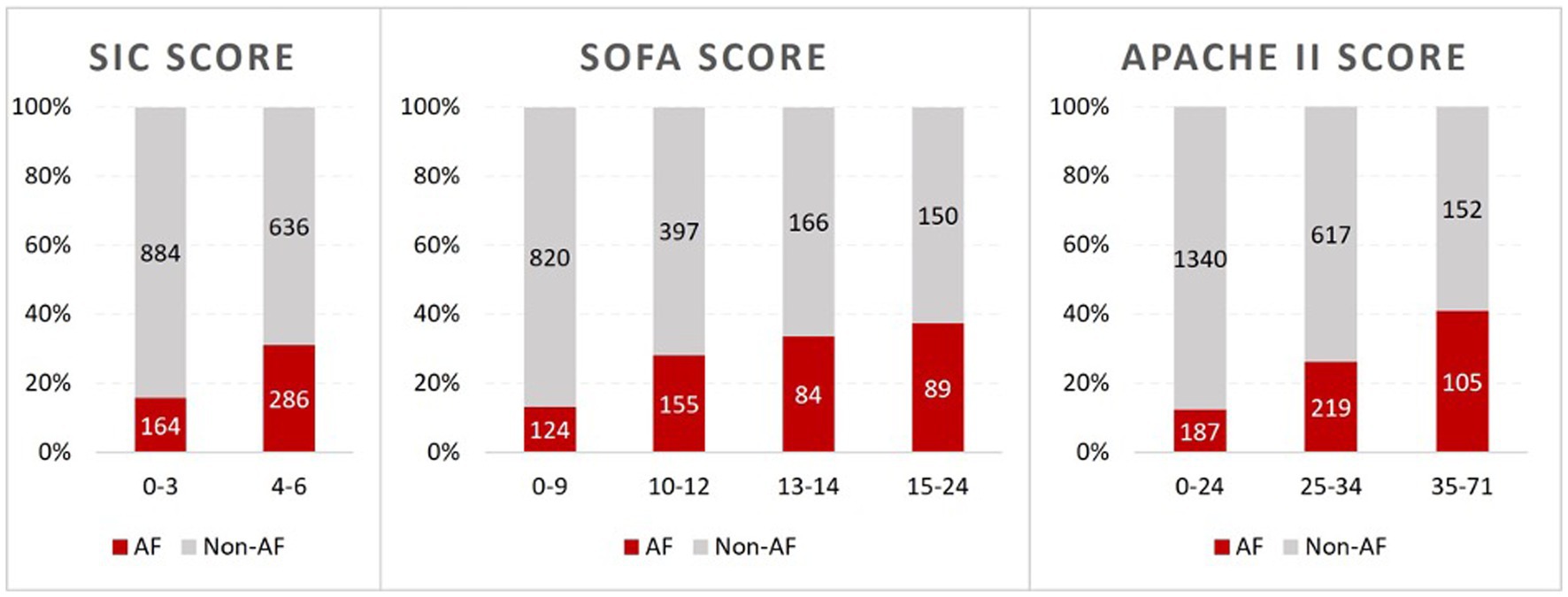
Figure 2. SIC, SOFA and APACHE II scores of AF and non-AF septic patients grouped in ranges of values. AF patients are represented in red and non-AF patients in grey. The top row shows the scores in counts and the bottom row shows them as a proportion (percentage) of AF and non-AF cases within each range of value. The bottom row shows how the proportion of AF cases increases as each of these severity scores increases.
We also observed significant differences in blood results for SIC-negative versus SIC-positive patients (Table 1). Median ALAT serum concentrations were higher in SIC-positive than in SIC-negative patients. Kidney function, measured as higher creatinine serum concentrations, was significantly worse in SIC-positive patients compared to SIC-negative patients. The cardiovascular impact of SIC is evidenced by significantly higher average heart rates in SIC-positive versus SIC-negative patients. In parallel, SIC-positive patients displayed an average systolic blood pressure that was significantly lower than in SIC-negative patients.
3.2. Multivariate logistic regression
The odds ratios (OR) for the occurence of AF in all septic patients, SIC positive and SIC negative patients are presented in Figure 3. We identified input variables (factors) that are either positively or negatively associated with the risk of AF. The most significant factor is age, with patients over 80 years having the greatest risk of developing AF (age group 80+ OR: all = 13.168, SIC-positive = 29.935, SIC-negative = 40.656).
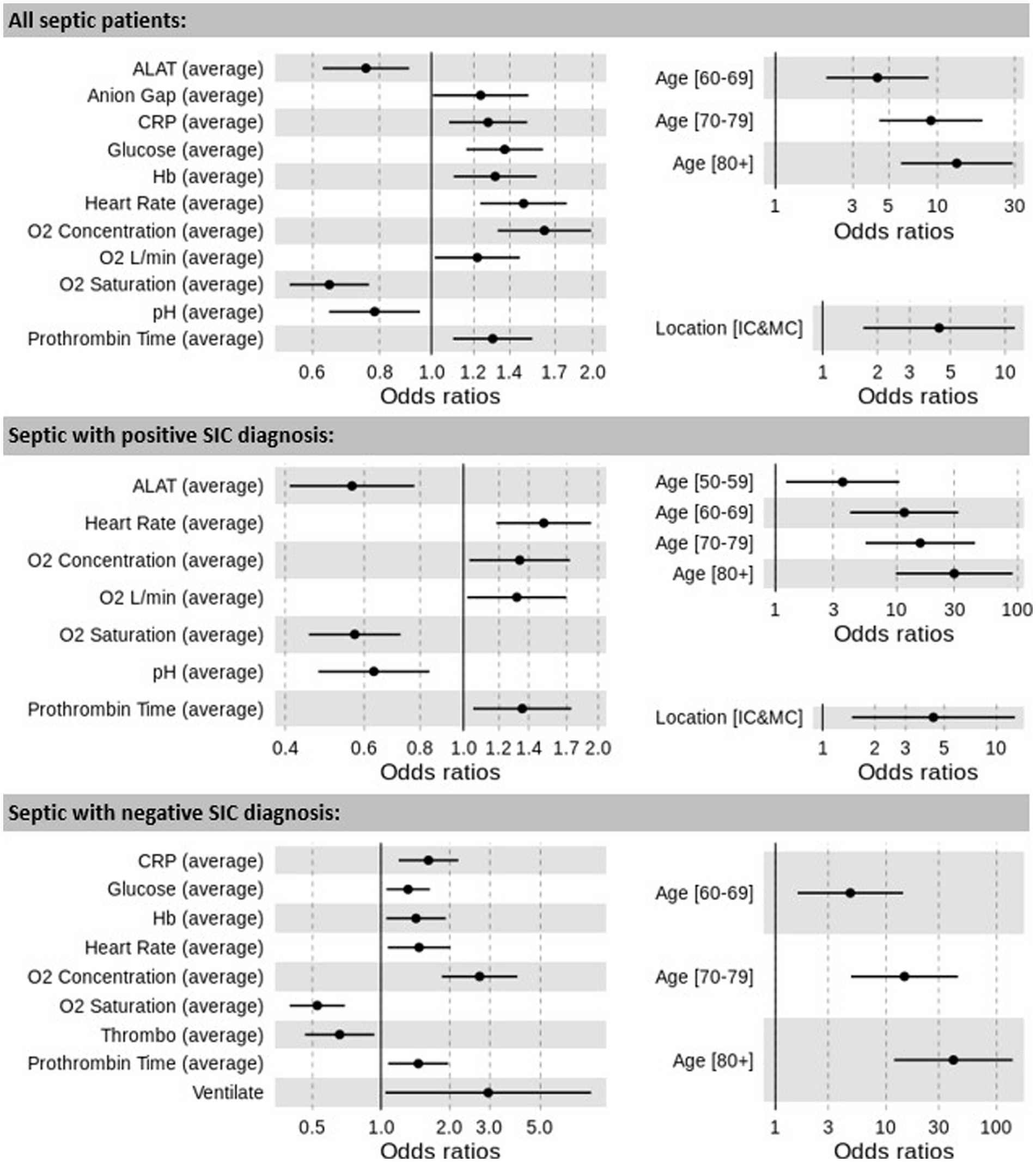
Figure 3. Odds ratios between significant results and prediction of AF for the overall cohort of septic patients, SIC-positive and SIC-negative patients. ALAT, alanine transaminase; CRP, C-reactive protein; Hb, haemoglobin; Ventilate, ventilated. Prothrombin time reflects international normalized prothrombin time ratio (INR).
Other factors that are positively associated with the development of AF in the three models (all septic patients, SIC-positive and SIC-negative) include heart rate (OR: all = 1.486, SIC-positive = 1.511, SIC-negative = 1.469), and INR (OR: all = 1.301, SIC-positive = 1.353, SIC-negative = 1.458). Increased oxygen requirements (O2 concentration, OR: all = 1.625, SIC-positive = 1.336, SIC-negative = 2.708, and O2 L/min, OR: all = 1.218, SIC-positive = 1.316), as well as reduced oxygen saturations (O2 saturation, OR: all = 0.645, SIC-positive = 0.572, SIC-negative = 0.526), were also associated with the development of AF.
Reduced levels of alanine transaminase (ALAT, OR: all = 0.755, SIC-positive = 0.564) and arterial pH (OR: all = 0.783, SIC-positive = 0.631) were associated with AF episodes in the overall sepsis and the SIC-positive cohorts, whilst reduced values of thrombocyte count (OR: SIC-negative = 0.659) increased the AF risk in the SIC-negative cohort. Increased values of CRP (OR: all = 1.276, SIC-negative = 1.616), blood glucose (OR: all = 1.370, SIC-negative = 1.315) and Hb (OR: all = 1.316, SIC-negative = 1.425) are positively associated with the development of AF in the overall sepsis and the SIC-negative cohorts. Anion gap was also found to be a risk factor in the overall cohort (OR: all = 1.236).
3.3. Variable importance analysis
We used RF and GBM to analyse the variable importance in each cohort (Figure 4). The variation in Gini variable importance between all septic patients, septic patients with a positive SIC diagnosis, and septic patients with a negative SIC diagnosis is shown in Figure 4. Although a group of variables (e.g., age, O2 concentration, and O2 saturation) were found to be important across all models, overall, the variable rankings were different. Also, LR and the non-linear models RF and GBM identified different predictive factors depending on the patient cohort investigated (Figure 4).
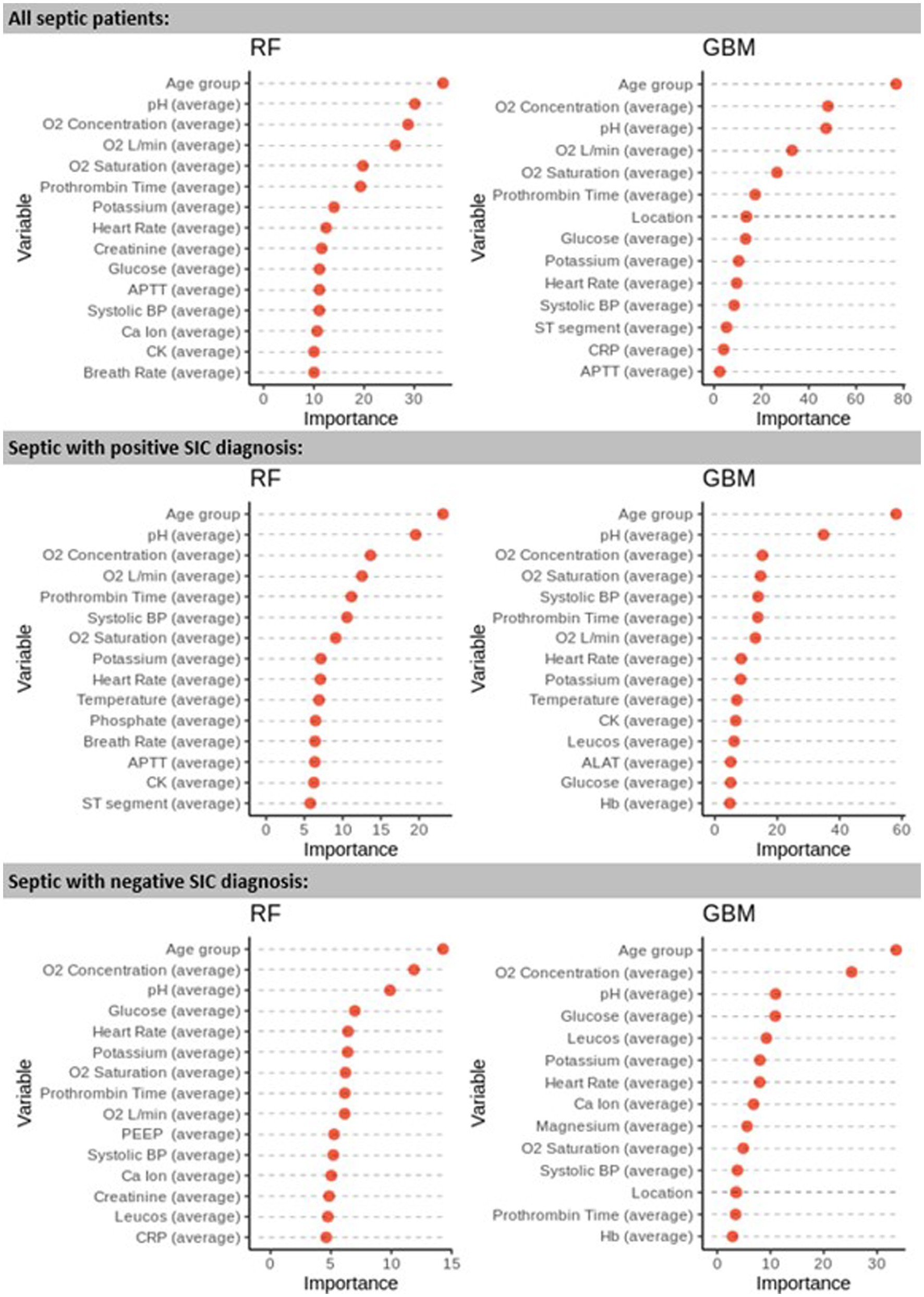
Figure 4. Gini variable importance as calculated by the RF and GBM models for the 3 cohorts studied: all septic patients, septic patients with SIC diagnosis, and non-SIC septic patients. Prothrombin time reflects international normalized prothrombin time ratio (INR). Abbreviations as in Table 1.
3.4. Performance of ML and multivariate statistical models
In the three cohorts investigated (overall cohort of septic patients, SIC-positive and SIC-negative patients) LR, RF, and GBM models all demonstrated excellent performance (AUC >0.8) for prediction of the first occurrence of AF in septic patients admitted to critical care in sinus rhythm. GBM and RF outperformed LR in both SIC strata, but had comparable AUC characteristics for the overall cohort of septic patients (Table 2).

Table 2. Model performance comparisons as measured using the area under the ROC curve (AUC) for the different models developed.
4. Discussion
We demonstrate significant differences in vital signs, respiratory support, blood results and organ function in patients with SIC compared to those without. Our results confirm previous reports that patients who develop SIC when critically ill, have higher ICU mortality and longer ICU stay compared to those without SIC. In the subgroup analysis stratified by SIC diagnosis, the occurrence of AF was also associated with worse outcomes, suggesting an interaction between a first episode of AF in critically ill patients and SIC on mortality for patients with SIC.
A main finding of our work is that patients with SIC who are admitted to ICU in sinus rhythm, develop episodes of AF more often than those without coagulopathy. We used a SIC score of ≥4 to stratify septic patients into those with a diagnosis of SIC and those without. Several reports have demonstrated that in the general population the occurrence of AF is associated with platelet and coagulation activation (21, 22) and hypofibrinolysis (23, 24). Experimental data suggest that hypercoagulability in itself with enhanced thrombin activity as the main feature, can promote the onset of AF in mice (25). Fibrotic atrial changes and altered atrial function especially in elderly patients are established risk factors for AF in the general population but also in critically ill patients. It is therefore tempting to speculate that similar mechanisms contribute to AF observed in acutely and critically ill patients. Long et al. defined an “early coagulation disorder” within 24 h after ICU admission as a risk factor for AF in individuals with sepsis (26).
Increased fibrin formation, reduced fibrinolytic activity, platelet activation and consumption characterize coagulopathy in sepsis (27, 28). Similar changes are also observed with increasing age (29) but to a lower extent. Fibrinogen serum concentrations increase by 10 mg/dL per each decade even in healthy subjects and may contribute to the higher cardiovascular risk observed in elderly people. As acute phase reactant elevated fibrinogen levels also reflect a proinflammatory state. In sepsis high fibrinogen levels can be observed, however, the degree to which they contribute to proinflammation and hypercoagulability as dynamic processes, which are time and disease burden specific, is not fully understood. Nevertheless, during sepsis as the most severe state of acute inflammation, the cross-talk between endothelium, coagulation cascades and the immune system is now regarded as an essential process of the body’s defence (27). The association of ageing with chronic inflammation together with the increased risk of AF indicates that the cardiac-endothelial-immune axis is already activated subclinically in older individuals. When faced with severe infection, older patients are more likely to develop cardiac complications compared to younger patients due to the structural cardiovascular changes associated with ageing (30). Episodes of AF in critical illness can therefore be interpreted as the cardiac manifestation of an acute-on-chronic condition, while the occurrence of SIC is dominated by the severity of sepsis and the acute activation of immune and coagulation systems. This concept is supported by our observation that age is the main risk factor for AF episodes in all models developed (13).
Long et al. categorized APTT, INR and platelet count into three different severity classes to construct a 6-point score for “early coagulation disorder” and identified the presence of early coagulation disorder as a risk factor for the occurrence of AF in septic patients (26). In line with this report, our work highlights the association of septic coagulopathy with AF. Long et al. used a novel score which is not widely used in clinical practice to define “early coagulation disorder.” This limits transferability into clinical practice. Our work demonstrates that a diagnosis of SIC predisposes septic patients to the development of arrhythmias such as AF. In addition to demonstrating the association between coagulopathy and AF we provide comprehensive models based on machine learning and logistic regression to predict the onset of AF. We expect that such models will be integrated into modern monitoring devices and electronic patient records in the future.
Even subclinical changes in coagulation likely increase the risk of AF in septic patients. This suggests that more specific markers of coagulation may be required to better understand the role of endothelial, immune cell and coagulation cascades in the pathogenesis of AF during acute illness. However, differentiated coagulation profiles come at a cost and are currently not available as part of the routine blood panels recorded in large databases. Measurement of the coagulation disturbances in acute sepsis is complex, time-sensitive and requires serial measurements (16). aPTT and PT as measures of coagulation disregard the contribution of platelets in thrombin generation, clot formation and (immuno)thrombosis. Whole blood viscoelastic testing is becoming more widely available and further studies should include global clotting tests such as rotational thromboelastometry to fully understand the role of clinical and subclinical alterations in coagulation for the development of AF during sepsis.
The pathomechanisms how SIC may trigger episodes of AF remain unclear. Several of the pathways involved in the development of SIC have also been associated with the occurrence of arrhythmias, and in particular AF. Recently NETosis, the formation of Neutrophil Extracellular Traps (NETs), and histone release have been shown to be major contributors to thrombosis in bacterial infection (11, 31). A study linking histone levels and arrhythmias found that circulating histone levels in these patients were significantly higher in patients with new onset arrhythmias than in those without (3). Patients who developed paroxysmal AF had particularly high histone levels (3). These findings make it tempting to speculate that histones and NETs can directly affect the cardiac conduction system to trigger arrhythmias. Our finding that increased heart rates are a predictor of AF episodes could also be explained by direct effects of histones on the cardiac conduction system. Further research is required to specify such cross talk between the immune system, the coagulation cascades and the heart in more detail.
Both pathologies, sepsis and coagulopathy occur as a consequence of complex and multi-layered activation of immune, adrenergic, epigenetic, inflammatory, anti-inflammatory, endothelial and other pathways. Although we could clearly demonstrate an association between the occurrence of atrial fibrillation and sepsis-induced coagulopathy, a causal relationship between both conditions remains speculative and further research is required to explore this hypothesis.
In this study, we used conventional multivariate analysis to identify possible associations between SIC and a first episode of AF in patients admitted to ICU with sepsis. For many years, LR has been successfully used for predictive modeling in healthcare, showing to be a competitive algorithm when compared to other ML algorithms (32). However, as a linear model, LR has limitations when presented with non-linear data or variables with complex relationships. ML algorithms such as RF and GBM are usually better at modeling complex relationships between variables and can make more accurate predictions (33–35), contributing significantly to the development of our understanding of AF (36). ML algorithms also tend to be more robust to outliers and can handle larger datasets better. In our dataset, the ML-based models improved the performance of the LR models in SIC-positive and SIC-negative patients as evidenced by higher AUCs.
Our study has several limitations. Major limitations lie in the data set and the data that are recorded in the database. Data about a previous history of AF are not available in the AmsterdamUMC database, hence it was not possible to discriminate between new onset and pre-existing AF. However, since the recurrence of AF after its first occurrence in critical illness is frequently observed (37) it is likely that acute triggers unmask underlying chronic structural changes and a chronically altered cardiovascular function. Therefore any episode of AF may be regarded as the clinical manifestation of a chronic underlying pathological process. The coagulation results available in the AmsterdamUMCdb are limited to routinely performed tests such as aPTT, platelet count and prothrombin time ratios. Hence more specific markers of SIC, including D-Dimer and fibrinogen, which are likely to improve the performance of our models further, are not available in a sufficient number of patients to allow statistical analysis. Changes in aPTT, platelet count and INR are complex and vary depending and underlying cause of infection, premorbid condition and treatment. Impaired synthetic capacity, but also consumption of coagulation factors, Vitamin K deficiency or anticoagulants may prolong aPTT or increase INR. The lack of data for specific coagulation tests in the Amsterdam database make a more detailed analysis of the underlying subtype of coagulopathy impossible. Lastly, the presence of SIC may reflect mainly the severity of disease instead of representing a separate distinct risk factor for the development of AF. Investigating different non-septic patient cohorts with disseminated intravascular coagulation regarding their risk profile for AF may help to clarify the role of the endothelial-immune-coagulation axis for the development of AF in acute illness.
For patients that were invasively ventilated, O2 concentration was documented, while O2 in L/min was recorded for patients on non-invasive ventilatory support. We refrained from converting Oxygen supply in l/min into FiO2 due to the lack of universally accepted conversion formulas.
We have not yet externally validated the models developed which limits the generalisability and transferability to other settings. Further research is also required to evaluate how the implementation of such models can support clinical decision-making at the bedside.
5. Conclusion
Our results confirm previous studies that the occurrence of AF in patients during critical illness and SIC is associated with higher mortality. Higher INR, increased heart rate and more severe respiratory failure were also identified as risk factors, suggesting an association between cardiac, respiratory and coagulation systems. Despite the influence of routine coagulation markers and cardiorespiratory parameters, age was the most dominant factor to predict the first episodes of AF in patients admitted in sinus rhythm with and without SIC.
Data availability statement
Publicly available datasets were analyzed in this study. This data can be found at: https://amsterdammedicaldatascience.nl/amsterdamumcdb/.
Ethics statement
Ethical approval was not required for the study involving humans in accordance with the local legislation and institutional requirements. Written informed consent to participate in this study was not required from the participants or the participants’ legal guardians/next of kin in accordance with the national legislation and the institutional requirements.
Author contributions
SO-M extracted and prepared the data. SO-M and IO conducted the statistical and machine learning analysis, and evaluated the results. IW and BJ provided the clinical expertise. IW and SOM conceptualised the study. All authors were involved in the study design and the selection of relevant variables from the dataset and contributed to the writing, reviewing and editing, and approved the final manuscript.
Funding
IW was an employee of the University of Liverpool. BJ was an employee of Liverpool University Hospitals NHS Foundation Trust. SO-M and IO were employed by the Liverpool John Moores University.
Acknowledgments
We acknowledge the 3rd Critical Care Datathon organised by the European Society of Intensive Care Medicine (ESICM), as well as their organisers (particularly Ari Ercole, Patrick Thoral and Paul Elbers), who provided an opportunity for the understanding and exploitation of the AmsterdamUMCdb database.
Conflict of interest
The authors declare that the research was conducted in the absence of any commercial or financial relationships that could be construed as a potential conflict of interest.
Publisher’s note
All claims expressed in this article are solely those of the authors and do not necessarily represent those of their affiliated organizations, or those of the publisher, the editors and the reviewers. Any product that may be evaluated in this article, or claim that may be made by its manufacturer, is not guaranteed or endorsed by the publisher.
References
1. Singer, M, Deutschman, CS, Seymour, CW, Shankar-Hari, M, Annane, D, Bauer, M, et al. The third international consensus definitions for Sepsis and septic shock (Sepsis-3). JAMA. (2016) 315:801. doi: 10.1001/jama.2016.0287
2. Fiusa, MML, Carvalho-Filho, MA, Annichino-Bizzacchi, JM, and de Paula, EV. Causes and consequences of coagulation activation in sepsis: an evolutionary medicine perspective. BMC Med. (2015) 13:1–9. doi: 10.1186/s12916-015-0327-2
3. Alhamdi, Y, Abrams, ST, Cheng, Z, Jing, S, Su, D, Liu, Z, et al. Circulating histones are major mediators of cardiac injury in patients with sepsis. Crit Care Med. (2015) 43:2094–103. doi: 10.1097/CCM.0000000000001162
4. Klein Klouwenberg, PMC, Frencken, JF, Kuipers, S, Ong, DSY, Peelen, LM, van Vught, LA, et al. Incidence, predictors, and outcomes of new-onset atrial fibrillation in critically ill patients with Sepsis. A cohort study. Am J Respir Crit Care Med. (2017) 195:205–11. doi: 10.1164/rccm.201603-0618OC
5. Wetterslev, M, Haase, N, Hassager, C, Belley-Cote, EP, McIntyre, WF, An, Y, et al. New-onset atrial fibrillation in adult critically ill patients: a scoping review. Intensive Care Med. (2019) 45:928–38. doi: 10.1007/s00134-019-05633-x
6. Johnston, BW, Chean, CS, Duarte, R, Hill, R, Blackwood, B, McAuley, DF, et al. Management of new onset atrial fibrillation in critically unwell adult patients: a systematic review and narrative synthesis. Br J Anaesth. (2022) 128:759–71. doi: 10.1016/j.bja.2021.11.016
7. Adelborg, K, Larsen, JB, and Hvas, AM. Disseminated intravascular coagulation: epidemiology, biomarkers, and management. Br J Haematol. (2021) 192:803–18. doi: 10.1111/bjh.17172
8. Iba, T, di Nisio, M, Levy, JH, Kitamura, N, and Thachil, J. New criteria for sepsis-induced coagulopathy (SIC) following the revised sepsis definition: a retrospective analysis of a nationwide survey. BMJ Open. (2017) 7:e017046. doi: 10.1136/bmjopen-2017-017046
9. Vincent, JL, Moreno, R, Takala, J, Willatts, S, de Mendonça, A, Bruining, H, et al. The SOFA (Sepsis-related organ failure assessment) score to describe organ dysfunction/failure. On behalf of the working group on Sepsis-related problems of the European Society of Intensive Care Medicine. Intensive Care Med. (1996) 22:707–10. doi: 10.1007/BF01709751
10. Ding, WY, Gupta, D, and Lip, GYH. Atrial fibrillation and the prothrombotic state: revisiting Virchow’s triad in 2020. Heart. (2020) 106:1463–8. doi: 10.1136/heartjnl-2020-316977
11. Stark, K, and Massberg, S. Interplay between inflammation and thrombosis in cardiovascular pathology. Nat Rev Cardiol. (2021) 18:666–82. doi: 10.1038/s41569-021-00552-1
12. Thoral, PJ, Fornasa, M, de Bruin, DP, Tonutti, M, Hovenkamp, H, Driessen, RH, et al. Explainable machine learning on AmsterdamUMCdb for ICU discharge decision support: uniting intensivists and data scientists. Crit Care Explor. (2021) 3:e0529. doi: 10.1097/CCE.0000000000000529
13. Ortega-Martorell, S, Pieroni, M, Johnston, BW, Olier, I, and Welters, ID. Development of a risk prediction model for new episodes of atrial fibrillation in medical-surgical critically ill patients using the AmsterdamUMCdb. Front Cardiovasc Med. (2022) 9:7709. doi: 10.3389/FCVM.2022.897709
14. Knaus, WA, Draper, EA, Wagner, DP, and Zimmerman, JE. APACHE II: a severity of disease classification system. Crit Care Med. (1985) 13:818–29. doi: 10.1097/00003246-198510000-00009
15. Edinburgh, T, Eglen, SJ, Thoral, P, Elbers, P, and Ercole, A. Sepsis-3 criteria in AmsterdamUMCdb: Open-source code implementation. (2022) 2022:gigabyte45. doi: 10.46471/gigabyte.45
16. Simmons, J, and Pittet, JF. The coagulopathy of acute sepsis. Curr Opin Anaesthesiol. (2015) 28:227–36. doi: 10.1097/ACO.0000000000000163
17. Vincent, JL, de Mendonça, A, Cantraine, F, Moreno, R, Takala, J, Suter, PM, et al. Use of the SOFA score to assess the incidence of organ dysfunction/failure in intensive care units: results of a multicenter, prospective study. Working group on “sepsis-related problems” of the European Society of Intensive Care Medicine. Crit Care Med. (1998) 26:1793–800. doi: 10.1097/00003246-199811000-00016
18. Lopes Ferreira, F, Peres Bota, D, Bross, A, Mélot, C, and Vincent, JL. Serial evaluation of the SOFA score to predict outcome in critically Ill patients. JAMA. (2001) 286:1754–8. doi: 10.1001/JAMA.286.14.1754
20. Friedman, JH. Stochastic gradient boosting. Comput Stat Data Anal. (2002) 38:367–78. doi: 10.1016/S0167-9473(01)00065-2
21. Lip, GYH, Lip, PL, Zarifis, J, Watson, RDS, Bareford, D, Lowe, GDO, et al. Fibrin D-dimer and beta-thromboglobulin as markers of thrombogenesis and platelet activation in atrial fibrillation. Effects of introducing ultra-low-dose warfarin and aspirin. Circulation. (1996) 94:425–31. doi: 10.1161/01.CIR.94.3.425
22. Colkesen, Y, Acil, T, Abayli, B, Yigit, F, Katircibasi, T, Kocum, T, et al. Mean platelet volume is elevated during paroxysmal atrial fibrillation: a marker of increased platelet activation? Blood Coagul Fibrinolysis. (2008) 19:411–4. doi: 10.1097/MBC.0b013e3283049697
23. Marín, F, Roldán, V, and Lip, GYH. Fibrinolytic function and atrial fibrillation. Thromb Res. (2003) 109:233–40. doi: 10.1016/S0049-3848(03)00259-7
24. Wang, TD, Chen, WJ, Su, SSYI, Su, TAC, Chen, MF, Liau, CS, et al. Increased levels of tissue plasminogen activator antigen and factor VIII activity in nonvalvular atrial fibrillation: relation to predictors of thromboembolism. J Cardiovasc Electrophysiol. (2001) 12:877–84. doi: 10.1046/j.1540-8167.2001.00877.x
25. Spronk, HMH, de Jong, AM, Verheule, S, de Boer, HC, Maass, AH, Lau, DH, et al. Hypercoagulability causes atrial fibrosis and promotes atrial fibrillation. Eur Heart J. (2017) 38:38–50. doi: 10.1093/eurheartj/ehw119
26. Long, Y, Tong, Y, Miao, R, Fan, R, Cao, X, Wang, J, et al. Early coagulation disorder is associated with an increased risk of atrial fibrillation in septic patients. Front Cardiovasc Med. (2021) 8:724942. doi: 10.3389/fcvm.2021.724942
27. Iba, T, and Levy, JH. Sepsis-induced coagulopathy and disseminated intravascular coagulation. Anesthesiology. (2020) 132:1238–45. doi: 10.1097/ALN.0000000000003122
28. Tsantes, AG, Parastatidou, S, Tsantes, EA, Bonova, E, Tsante, KA, Mantzios, PG, et al. Sepsis-induced coagulopathy: an update on pathophysiology, biomarkers, and current guidelines. Life. (2023) 13:350. doi: 10.3390/life13020350
29. Mari, D, Ogliari, G, Castaldi, D, Vitale, G, Bollini, EM, and Lio, D. Hemost ageing. Immun Ageing. (2008) 5:1–4. doi: 10.1186/1742-4933-5-12/METRICS
30. Ho, S, Phua, HP, Lim, WY, Mahalingam, N, Tan, GHC, Puah, SH, et al. Sepsis, cardiovascular events and short-term mortality risk in critically ill patients. Ann Acad Med Singap. (2022) 51:272–82. doi: 10.47102/annals-acadmedsg.202220
31. Yokoyama, Y, Ito, T, Yasuda, T, Furubeppu, H, Kamikokuryo, C, Yamada, S, et al. Circulating histone H3 levels in septic patients are associated with coagulopathy, multiple organ failure, and death: a single-center observational study. Thromb J. (2019) 17:1. doi: 10.1186/s12959-018-0190-4
32. Christodoulou, E, Ma, J, Collins, GS, Steyerberg, EW, Verbakel, JY, and van Calster, B. A systematic review shows no performance benefit of machine learning over logistic regression for clinical prediction models. J Clin Epidemiol. (2019) 110:12–22. doi: 10.1016/j.jclinepi.2019.02.004
33. Couronné, R, Probst, P, and Boulesteix, AL. Random forest versus logistic regression: a large-scale benchmark experiment. BMC Bioinform. (2018) 19:270. doi: 10.1186/s12859-018-2264-5
34. Watanabe, E, Noyama, S, Kiyono, K, Inoue, H, Atarashi, H, Okumura, K, et al. Comparison among random forest, logistic regression, and existing clinical risk scores for predicting outcomes in patients with atrial fibrillation: a report from the J-RHYTHM registry. Clin Cardiol. (2021) 44:1305–15. doi: 10.1002/clc.23688
35. Weng, SF, Reps, J, Kai, J, Garibaldi, JM, and Qureshi, N. Can machine-learning improve cardiovascular risk prediction using routine clinical data? PLoS One. (2017) 12:e0174944. doi: 10.1371/journal.pone.0174944
36. Olier, I, Ortega-Martorell, S, Pieroni, M, and Lip, GYH. How machine learning is impacting research in atrial fibrillation: implications for risk prediction and future management. Cardiovasc Res. (2021) 117:1700–17. doi: 10.1093/cvr/cvab169
Keywords: atrial fibrillation, sepsis, machine learning, coagulopathy, database analysis, Sepsis-induced coagulopathy
Citation: Ortega-Martorell S, Olier I, Johnston BW and Welters ID (2023) Sepsis-induced coagulopathy is associated with new episodes of atrial fibrillation in patients admitted to critical care in sinus rhythm. Front. Med. 10:1230854. doi: 10.3389/fmed.2023.1230854
Edited by:
W. Conrad Liles, University of Washington, United StatesReviewed by:
Zhigang Chang, National Center of Gerontology, ChinaMasayuki Ozaki, Aichi Medical University, Japan
Copyright © 2023 Ortega-Martorell, Olier, Johnston and Welters. This is an open-access article distributed under the terms of the Creative Commons Attribution License (CC BY). The use, distribution or reproduction in other forums is permitted, provided the original author(s) and the copyright owner(s) are credited and that the original publication in this journal is cited, in accordance with accepted academic practice. No use, distribution or reproduction is permitted which does not comply with these terms.
*Correspondence: Sandra Ortega-Martorell, Uy5PcnRlZ2FNYXJ0b3JlbGxAbGptdS5hYy51aw==; Ingeborg D. Welters, SS5XZWx0ZXJzQGxpdmVycG9vbC5hYy51aw==