- 1Imaging Research Center, Hospital Israelita Albert Einstein, São Paulo, Brazil
- 2Electrical, Mechanical and Computer Engineering School, Federal University of Goiás, Goiânia, Brazil
- 3Studies and Researches in Science and Technology Group (GCITE), Federal Institute of Goiás, Goiânia, Brazil
Background: Skin cancer is one of the most common forms worldwide, with a significant increase in incidence over the last few decades. Early and accurate detection of this type of cancer can result in better prognoses and less invasive treatments for patients. With advances in Artificial Intelligence (AI), tools have emerged that can facilitate diagnosis and classify dermatological images, complementing traditional clinical assessments and being applicable where there is a shortage of specialists. Its adoption requires analysis of efficacy, safety, and ethical considerations, as well as considering the genetic and ethnic diversity of patients.
Objective: The systematic review aims to examine research on the detection, classification, and assessment of skin cancer images in clinical settings.
Methods: We conducted a systematic literature search on PubMed, Scopus, Embase, and Web of Science, encompassing studies published until April 4th, 2023. Study selection, data extraction, and critical appraisal were carried out by two independent reviewers. Results were subsequently presented through a narrative synthesis.
Results: Through the search, 760 studies were identified in four databases, from which only 18 studies were selected, focusing on developing, implementing, and validating systems to detect, diagnose, and classify skin cancer in clinical settings. This review covers descriptive analysis, data scenarios, data processing and techniques, study results and perspectives, and physician diversity, accessibility, and participation.
Conclusion: The application of artificial intelligence in dermatology has the potential to revolutionize early detection of skin cancer. However, it is imperative to validate and collaborate with healthcare professionals to ensure its clinical effectiveness and safety.
1 Introduction
The role of technology and artificial intelligence has gained increasing prominence in the field of dermatology. Techniques such as convolutional neural networks and image processing have been extensively examined for their capacity to identify specific features in skin lesion images, with the potential to aid in the recognition of suspicious lesions and the diagnosis of conditions like melanoma.
Skin cancer is the most common form of cancer worldwide (1). Over the past decade, there has been a concerning 27% increase in the annual diagnosis of invasive melanoma cases (2). Alarmingly, more than 5,400 people die from non-melanoma skin cancer every month (3). In the United States alone, the annual financial burden of treating skin cancer is estimated at a staggering US$8.1 billion, with approximately US$4.8 billion allocated to non-melanoma skin cancer and US$3.3 billion to melanoma (4). Among skin cancer types, basal cell carcinoma ranks as the most common, followed by squamous cell carcinoma and melanoma, which stands out as the most aggressive and lethal type of skin cancer (5, 6). Merkel cell carcinoma also stands out among aggressive tumors (7). These tumors can arise anywhere on the body but are frequently observed in regions more exposed to the sun, including the face, neck, arms, and hands. Thus, there is an imperative need for sustained efforts to promote awareness and prevention of skin cancer (8–10).
Conventional techniques for detecting these diseases include patient data analysis, as well as visual and histopathological analysis of the lesions (11). Visual assessment relies on the clinical inspection of the lesion, taking into consideration factors such as its appearance, size, shape, location, and evolution. On the other hand, histopathological analysis entails the collection of a sample of the lesion for laboratory examination, typically through techniques such as biopsy. Additionally, devices like the dermatoscope are used to facilitate the examination of the lesion and the identification of features such as pigmentation, vascularity, and regression (12). Another example is the use of confocal microscopy, a technique that allows the analysis of skin layers without the need for sample collection (13, 14).
These techniques have proven effective in the detection and diagnosis of skin diseases. However, they may present limitations, including subjectivity in visual analysis and the need for invasive sample collection procedures. Confocal microscopy incurs high financial costs and is relatively inaccessible to medical professionals, even among specialists.
It is also important to highlight that diagnosing these diseases poses a significant challenge to the healthcare system, especially in regions lacking specialized professionals or adequate equipment for skin lesion identification (15, 16). An alternative approach involves initial screening by general practitioners, who may not always possess the necessary training for early skin cancer detection (17).
The implementation of Computer-Aided Diagnosis (CAD) solutions powered by Artificial Intelligence (AI) holds the potential to address some of these limitations and offer a promising alternative for accurate and non-invasive skin disease diagnosis. Existing literature suggests that AI systems can classify skin cancers competently on par with dermatologists. Notably, the diagnostic capabilities of the dermatologist vary based on experience, i.e., it is not a uniform basis of reference. Moreover, studies highlight the feasibility of leveraging mobile devices equipped with neural networks to broaden the access of dermatological expertise, offering low-cost access to vital diagnostic care (18, 19).
While numerous solutions are being developed for skin cancer detection and classification, those are usually not evaluated and validated in real clinical settings, which limits their practical applicability. The review study conducted by Goyal et al. (20) provides an updated assessment of the performance of artificial intelligence algorithms in skin cancer classification and diagnosis. It also delves into the challenges faced by these systems and future opportunities to enhance of dermatologists' diagnostic abilities through AI support.
However, for these technologies to become effective and applicable in clinical settings, several challenges must be addressed. These challenges include the need for standardization in image acquisition and processing techniques, the requirement for extensive training datasets, and the creation of robust and representative databases (20–24). Prior studies in skin cancer classification have have demonstrated restricted generalizability due to insufficient data and an emphasis on standardized tasks (19). Furthermore, it is essential to evaluate the effectiveness and safety of these tools in diverse contexts, taking into account variables such as the ethnic and genetic diversity of the population and the specific type of skin cancer under consideration, among other factors. In this regard, it is imperative for research in this field to adhere rigorously to scientific and ethical standards. Finally, it is crucial to emphasize that automated skin disease detection should not replace clinical evaluation by medical professionals but rather complement it.
The aim of this systematic review is to investigate studies focused on the detection, classification, and evaluation of skin cancer images in a clinical setting. The main approaches and challenges encountered while implementing these techniques must be identified to do this. The importance of this systematic review lies in its ability to aggregate and thoroughly examine all pertinent research in this field, thus offering a comprehensive view of the subject. In turn, researchers can assess the quality and credibility of existing studies, identify knowledge gaps, and propose innovative research directions. Furthermore, this systematic review can provide valuable information for doctors and healthcare professionals looking to harness the potential of AI in aiding the diagnosis and treatment of skin diseases.
2 Methods
This section outlines the methodology employed for the systematic literature review, encompassing the following stages: (i) research identification, (ii) selection, (iii) eligibility, (iv) data extraction, and (v) synthesis.
2.1 Step 1: study identification
First, we established the objectives and questions that frame this literature review. The primary goal of this systematic review is to highlight research involving the implementation of AI in clinical settings. Our aim is to gain insights into the methodologies employed in previous research and the outcomes achieved when using AI in this context.
For this review, we registered a protocol with the International Prospective Register of Systematic Reviews (PROSPERO) under ID CRD42023411211 on April 4, 2023, and PRISMA guidelines were followed. PROSPERO is a global registry for systematic review protocols, where researchers publish their research methods in advance. This process promotes transparency, prevents publication bias, and improves the reproducibility of studies.
The search databases used for the literature review include PubMed, Scopus, Embase, and Web of Science, and topics are analyzed using the following search terms: (“skin cancer” OR “skin lesion” OR “dermatology” OR “dermatoscopy” OR “melanoma”) AND (“artificial intelligence” OR “neural network*” OR “deep learning” OR “convolutional neural network*” OR “transfer learning” OR “machine learning” OR “Computer aided diagnostic*” OR “CAD“ OR “image classification” OR “image processing” OR “Internet of things” OR “Data mining” OR “Iot”) AND (“real-time” or “real time” OR “real-world” OR “real world” OR “smartphone”) AND NOT (“Meta-Analysis” OR “Meta Analysis” OR “Systematic Review”).
2.2 Step 2: study selection
Secondly, we defined the search terms and established inclusion/exclusion criteria. In this literature review, we used the terms highlighted in the previous section, with the sole restriction being the inclusion of journal articles and conference proceedings only.
Our initial search yielded 760 results, of which 457 were identified as duplicates and therefore removed. This resulted in a pool of 303 distinct studies, which were subsequently evaluated for eligibility.
2.3 Step 3: study relevance and quality assessment
In the third step, we assessed the relevance and quality of the selected studies. Two authors (BCRSF and MRCR) were responsible for reading each title and abstract in order to assess the relevance and quality of each previously selected study. The criteria used to determine eligibility is as follows:
• The document's abstract presents clear objectives, methodology, and results.
• The study addresses computer-aided diagnostic solutions for skin cancer with a focus on real clinical applications.
• The study reports the accuracy, sensitivity, specificity, and/or overall accuracy of artificial intelligence systems for skin cancer.
• The study describes the development and/or validation process of the systems.
• The study provides a critical analysis of the results obtained by artificial intelligence systems and discusses their limitations and potential biases.
Based on the inclusion criteria stated above, a total of 282 studies were eliminated from consideration. Following a comprehensive review of the entire texts, three more studies were removed from consideration due to their limited content, which included only abstracts or incomplete texts. Ultimately, 18 studies have been retained. Figure 1 presents the study identification flowchart.
For the study, Mendeley and Rayyan tools were used.
2.4 Step 4: data extraction
To facilitate data extraction in our literature review, we utilized a spreadsheet to document the metadata of each selected study. The following metadata was analyzed:
1. Publication year and study objective.
2. Regarding the data used: Types of data, source, and quantity.
3. Resources used to assist in the detection and/or classification of skin lesions.
4. Technique for the detection and/or classification of skin lesions.
5. Study function.
6. Key findings and study perspectives.
7. Information regarding ethnic and genetic diversity of the population.
8. Information regarding system accessibility and availability.
9. Relationship and/or involvement of dermatologists and other medical professionals.
2.5 Step 5: data synthesis
The concluding phase of our study encompasses data synthesis, which was subdivided into two key steps. Initially, we conducted a systematic analysis of the raw data obtained through the literature review process. Subsequently, we compiled metadata pertaining to the articles chosen in our literature review.
3 Results and discussion
This section outlines the results obtained through the search strategies describes in the methodology.
3.1 Descriptive analysis
The first section of our analysis pertains to descriptive information. As part of this analysis, we examined the objectives of the selected studies.
The primary objective of all the mentioned studies is to develop, implement, and/or validate systems for the detection, diagnosis, and classification of skin cancers, particularly melanoma, using mobile devices or computers. These systems aim to improve the early detection of skin lesions and enhance diagnostic accuracy, assisting healthcare professionals and providing more accessible and efficient screening for patients. Furthermore, they explore the use of advanced techniques such as image processing, pattern recognition, and deep learning to automate the analysis process and deliver real-time results.
3.2 Data scenario
For our study, it is of utmost importance to analyze the quality and representativeness of the data, given that these factors play a critical role in developing reliable algorithms and models for skin lesion diagnosis. Diversity in data sources is key to ensure broader model generalization since different sources can provide specific and varied information about the lesions. Furthermore, the availability of large datasets containing hundreds of thousands of images can be extremely advantageous in creating more robust and accurate machine learning models. Table 1 presents important information about the data from each article. The study information is listed in chronological order based on the publication date.
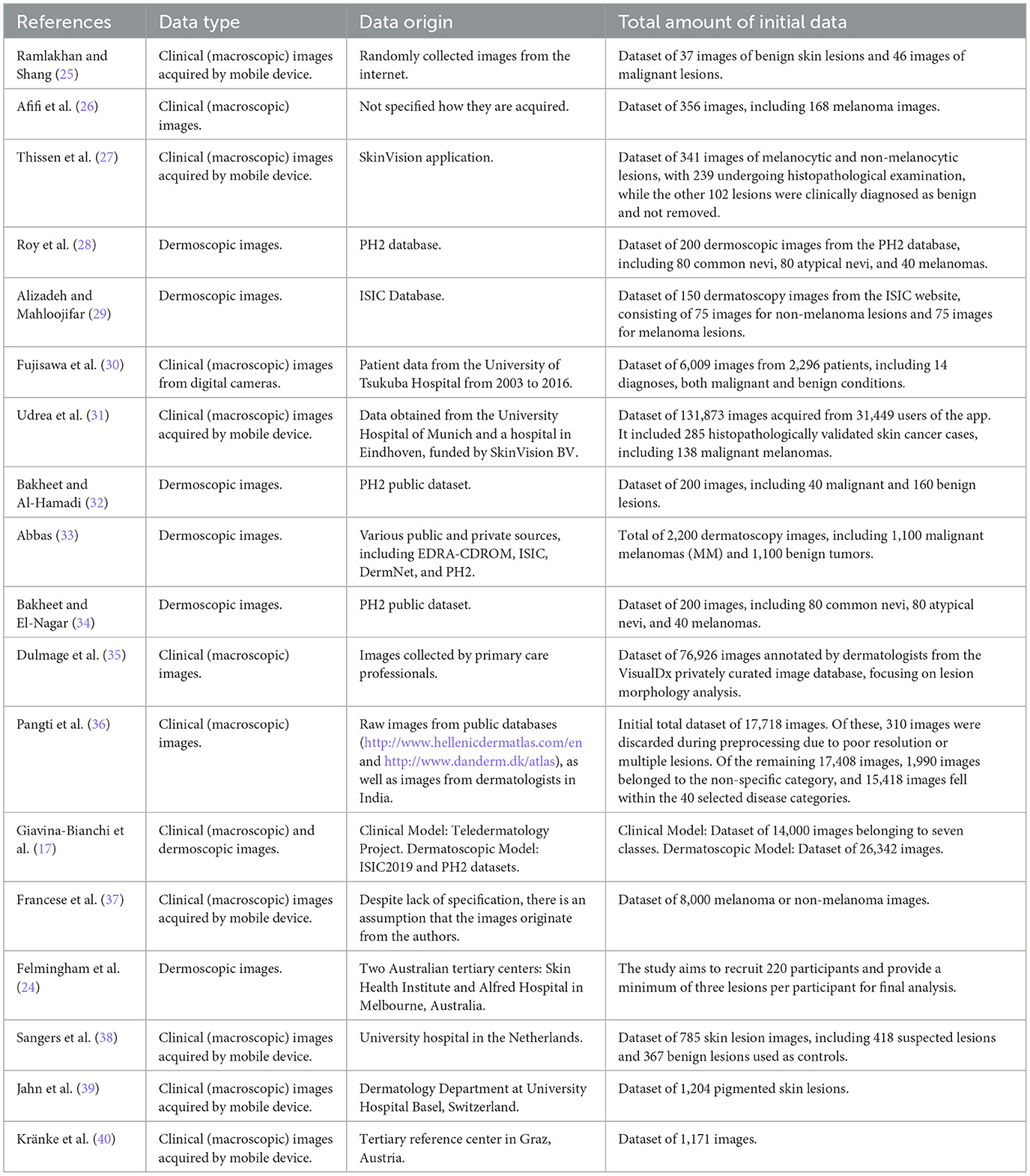
Table 1. Overview of studies on skin cancer image analysis: data type, origin, and quantity by year and author.
The analysis of the studies reveals a remarkable diversity of approaches in the diagnosis of skin tumors, with a significant emphasis on the detection of melanoma and other dermatological conditions. Among these research studies, there is a notable convergence in the preference for the use of clinical (macroscopic) images and/or dermoscopic images for analysis. The choice of these images demonstrates a consensus in the scientific community regarding the importance of this data in developing more effective and accessible diagnostic methods.
In the context of the types of data employed, Roy et al. (28) and Alizadeh and Mahloojifar (29) used dermoscopic images from established databases like PH2 and ISIC, adding to the reliability of the results. Meanwhile, Dulmage et al. (35) relies on clinical images collected by healthcare professionals, reflecting real-world conditions.
The discrepancy in the size of datasets is evident, with some studies using relatively small datasets, such as Ramlakhan and Shang (25) and Afifi et al. (26), which have 83 and 356 images, respectively. This limitation in size may restrict the models' capacity for generalization and accuracy. On the other hand, Udrea et al. (31) and Pangti et al. (36) present massive datasets containing 131,873 and 17,408 images, respectively. This provides a more solid foundation for model generalization and learning. Furthermore, Thissen et al. (27) works with a dataset of 341 images, which is still considerably limited compared to the larger datasets. This difference in dataset size directly impacts the models' ability to generalize, emphasizing the importance of carefully assessing effectiveness at different scales.
An additional disparity is observed when considering the specific focus versus the breadth of conditions addressed in studies of skin lesion diagnosis. While some studies have a narrow focus on melanoma and non-melanoma lesions (29), others adopt a broader approach, covering various categories of skin diseases (36). This distinction highlights the decision between targeting a specific condition or taking a more comprehensive approach, which directly influences the clinical applicability of the developed models.
However, there are less ideal scenarios to consider. Afifi et al. (26) and Ramlakhan and Shang (25) use clinical images without specifying their origin, which can negatively impact data quality and representativeness. Additionally, Thissen et al. (27) relies on images obtained from a commercial application, potentially resulting in limitations regarding image quality and diversity. The absence of specification of image origin in Francese et al. (37) is also a factor that can influence data quality and validity.
Finally, the study phase also presents divergences, with some studies still ongoing (24), while others already have final results. The preliminary nature of ongoing studies may limit the availability of conclusive results and the validity of analyses.
It is essential to recognize that both the quantity and type of data play crucial roles in the development of accurate and reliable cutaneous diagnostic models. Larger and more diverse datasets, coupled with high-quality images and reliable sources, tend to produce more robust and generalizable results. Therefore, the careful selection of these elements is fundamental to the effectiveness and clinical applicability of the developed models.
3.3 Techniques and processing
Next, we describe the resources employed in image processing, the classification algorithms used, and the devices on which these approaches were implemented. The resources employed in image processing are used to perform manipulation and feature extraction operations, aiming to prepare the images for analysis. Classification algorithms play the role on categorizing skin lesions based on the extracted features, enabling the precise identification of different classes. Furthermore, these algorithms can assist in clinical decision making, guiding healthcare professionals in choosing the best treatment approaches. These approaches are implemented on devices such as computers, servers, or mobile devices, providing efficient execution of algorithms and practical application of diagnostic techniques on skin lesion images.
Extracting this information from the studies presented here is crucial to guide the development of effective applications, allowing the appropriate selection of preprocessing methods, reliable classifiers, and suitable devices for achieving accurate detection and clinical assessment of skin lesions. Table 2 describes the resources used in image processing, the classification algorithms used, and the main purpose of the study.
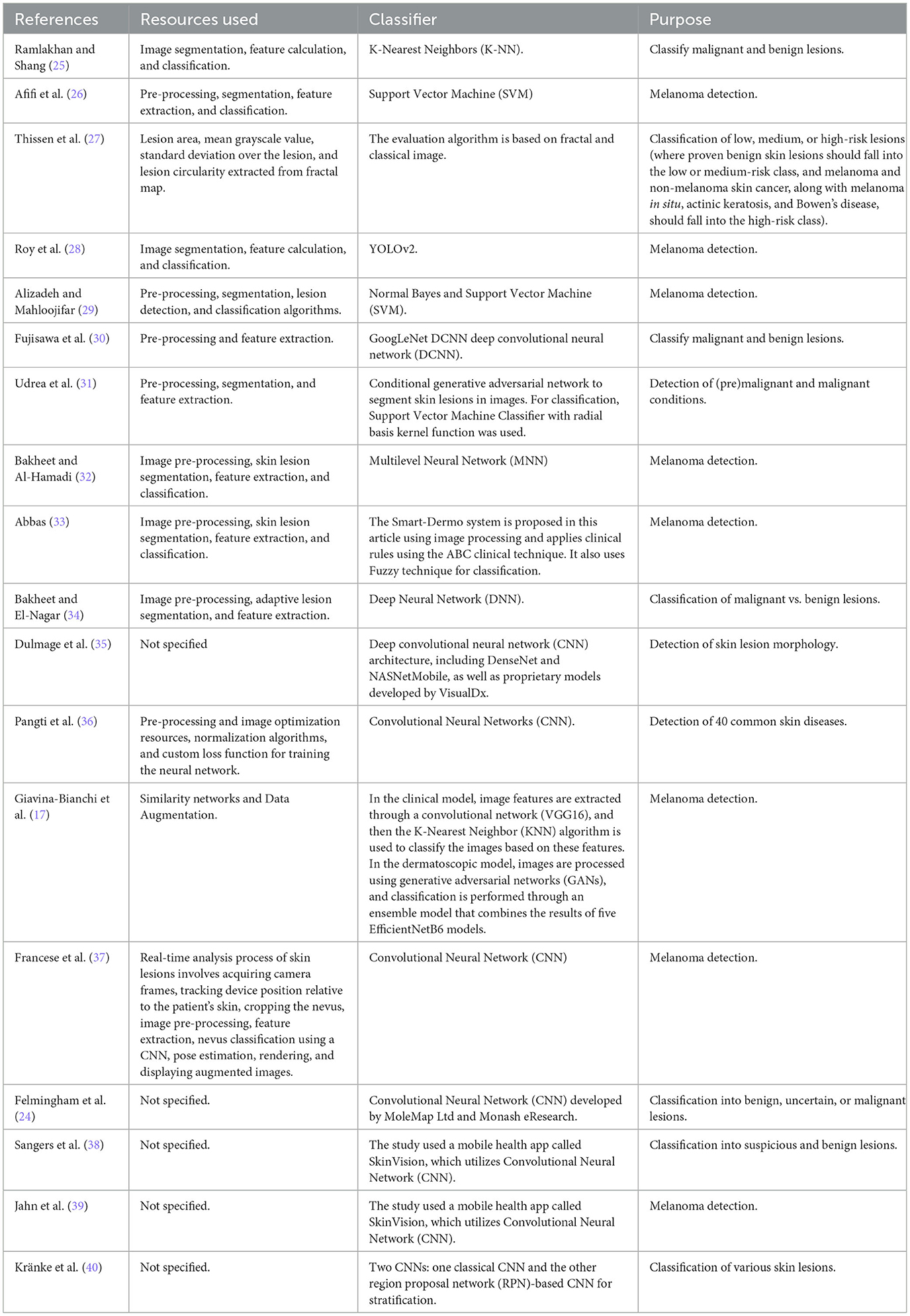
Table 2. Summary of techniques and classifiers used in skin cancer image analysis studies for clinical settings by year and author.
It is notable that several studies aim to utilize image segmentation, feature extraction, and classification techniques, as observed in Ramlakhan and Shang (25), Afifi et al. (26), Roy et al. (28), Alizadeh and Mahloojifar (29), Bakheet and Al-Hamadi (32) and Abbas (33). These steps are often fundamental for proper processing of skin lesion images and subsequent diagnostic decision-making.
On the other hand, there are differences regarding the choice of classifiers and processing devices. While some studies, such as Afifi et al. (26), employ Support Vector Machines (SVM) as classifiers, others, like Roy et al. (28), opt for more recent approaches like YOLOv2. The research by Roy et al. (28), Bakheet and Al-Hamadi (32), and Giavina-Bianchi et al. (17) presents a variety of approaches, ranging from the use of traditional machine learning algorithms to deep neural networks, such as Convolutional Neural Networks (CNNs). This diversity of techniques allows for a rich comparative analysis, enabling the identification of the most promising approaches for skin tumor detection. Additionally, the detailed description of the resources used and processing devices provides valuable insights for the development of effective applications.
Regarding processing devices, there is a distinction between approaches that perform detection and classification directly on mobile devices, such as Alizadeh and Mahloojifar (29), and approaches that send extracted features to a server for further analysis, as in the case of Giavina-Bianchi et al. (17). This difference highlights the variety of options available for implementing skin lesion detection solutions.
Finally, some studies do not provide complete information about the resources used, such as Dulmage et al. (35), which limits the understanding of the methodologies employed.
3.4 Main results and perspectives
In this section, we present the main outcomes and prospective insights derived from the various studies analyzed. The primary classification results demonstrate the accuracy, sensitivity, and specificity achieved by different approaches, allowing an assessment of how reliable these methods are in detecting malignant and benign lesions. Furthermore, the perspectives highlight the unique contributions of each study, such as the use of deep learning algorithms, real-time detection effectiveness, and the potential for screening in populations with limited access to dermatologists.
In the context of medicine and healthcare, this information assists medical professionals in choosing the most suitable approaches for early detection of malignant skin lesions, contributing to more precise and rapid diagnosis. Additionally, these results and perspectives also have significant implications for the future development of healthcare applications, guiding research and innovations in the field of artificial intelligence applied to dermatology.
Table 3 provides details related to the main results and perspectives.
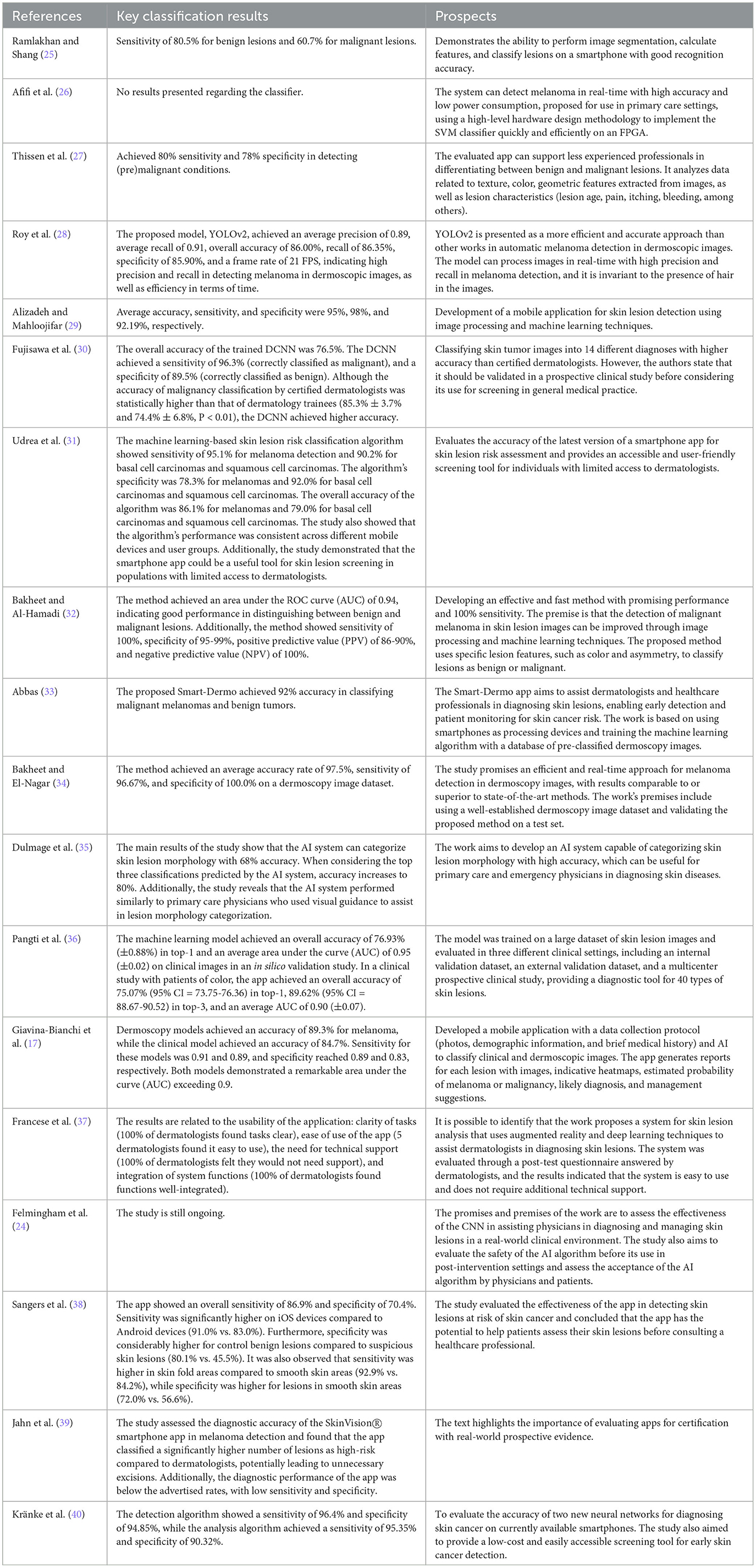
Table 3. Overview of classification results and potential implications of skin cancer studies for clinical settings by year and author.
The analysis of Table 3 highlights the positive aspects of recent advances in the detection, classification, and evaluation of skin cancer applications using machine learning and image processing, achieving high sensitivity and specificity in identifying malignant lesions. Furthermore, mobile applications offer an accessible approach to screening in populations with limited access to dermatologists.
However, more robust clinical validation is needed, considering the testing stage and comparison with traditional diagnosis. Performance variation between devices and the possibility of unnecessary excisions are also issues to be addressed. These advancements represent significant potential, but it is essential to balance opportunities with challenges, prioritizing ongoing research and validations for effective implementations in medical practice.
Among the studies presented, the YOLOv2 model, proposed by Roy et al. (28), stands out by demonstrating high precision and sensitivity in the detection of melanoma in dermoscopic images, processing in real-time efficiently. Additionally, Udrea et al. (31) present a machine learning-based method that achieves significant results in sensitivity and specificity for the detection of melanomas and basal cell carcinomas and squamous cell carcinomas. In turn, Giavina-Bianchi et al. (17) develop dermatoscopy models to assist dermatologists, offering positive perspectives for improving the detection and management of skin lesions. Furthermore, an innovative approach by Francese et al. (37) uses augmented reality and deep learning in a lesion analysis system, with the potential to facilitate dermatological diagnosis.
It is important to note that, although all the approaches highlighted in Table 3 show promising results, many of them are still undergoing testing and clinical validation phases. Therefore, it is crucial to continue rigorous research and in-depth evaluations, as emphasized by various researchers, before considering the widespread and effective implementation of these approaches in medical practice. These innovations have the potential to revolutionize early detection and diagnosis of skin cancer, but ensuring their reliability and clinical utility through robust studies is fundamental.
3.5 Diversity, accessibility, and medical collaboration
Ethnic diversity, the involvement of medical professionals, and ethical considerations play a pivotal and indispensable role in the development of applications designed for the detection and classification of skin lesions. These factors significantly contribute to the efficacy, validity, and accessibility of these technological solutions, thereby ensuring their widespread acceptance and adoption within the medical community, characterized by both confidence and equity. The continuous advancement within this scientific domain necessitates a multidisciplinary approach that seamlessly amalgamates the expertise of dermatologists, data scientists, and healthcare practitioners with the overarching objective of further enhancing the precision and impact of these pioneering applications.
Within this context, the systematic incorporation of a comprehensive array of ethnicities and genotypes into the training and evaluation datasets assumes fundamental importance. This strategic inclusion is essential to ensure the capability of such applications to meticulously identify and classify lesions across diverse skin types. This strategic approach contributes profoundly to the reduction of potential biases and affirms the technology's reliability for a broad and variegated spectrum of end-users.
Additionally, the active involvement of seasoned healthcare professionals plays a critical role in the formulation of the training parameters for AI models and the meticulous review of the decisions emanating from these applications. This collaborative synergy serves as an anchor to guarantee diagnostic precision while also facilitating the identification of intricate cases that may pose challenges to the technology. Furthermore, the validation of these applications by dermatologists is of paramount importance in the comprehensive evaluation of their effectiveness in comparison to conventional diagnostic methodologies.
In this manner, Table 4 presents a repository of pertinent information pertaining to the ethnic and genetic diversity of the study population, in conjunction with a meticulous assessment of the participation levels of dermatologists and other healthcare professionals in each research study.
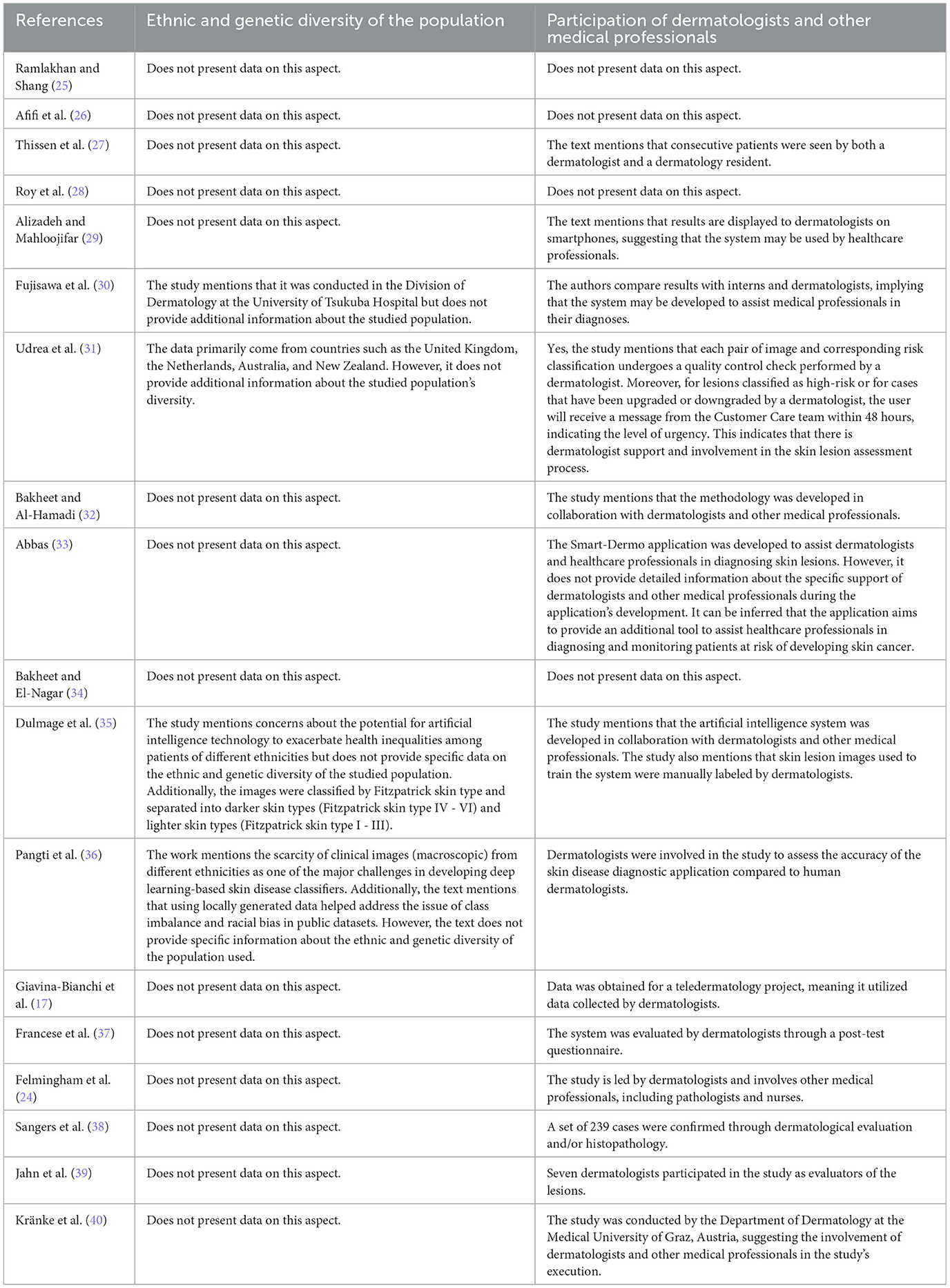
Table 4. Diversity considerations and medical professional involvement in skin cancer studies for clinical settings by year and author.
The studies present diverse approaches in their research endeavors. For instance, Udrea et al. (31) emphasizes the inclusion of data origin information, indicating that the data predominantly comes from countries such as the United Kingdom, the Netherlands, Australia, and New Zealand. On the other hand, Pangti et al. (36) mentions the scarcity of clinical images from different ethnicities as a challenge but addresses this issue by using locally generated data to mitigate class imbalance and racial bias in public datasets.
Another notable difference lies in the validation approach. While Fujisawa et al. (30) and Pangti et al. (36) highlight comparative validation with diagnoses performed by healthcare professionals, Francese et al. (37) focuses on evaluation by dermatologists through post-test questionnaires. Each of these studies adopts a unique strategy to verify the effectiveness and accuracy of the applications.
Moreover, Dulmage et al. (35) draws attention to image classification based on the Fitzpatrick skin type, emphasizing specific considerations for variations in skin tone in their assessments. Conversely, Bianchi et al. (17) utilizes data collected through teledermatology for their project, highlighting a different data acquisition approach.
In summary, the studies exhibit differences in terms of data origin, validation strategies, considerations regarding ethnic diversity, and specific data collection approaches, showcasing the diversity and innovation in the approaches taken to create skin lesion detection applications. However, a central characteristic is the close collaboration with dermatologists and medical professionals, as evidenced in multiple studies. This direct interaction ensures the clinical validity of the applications by aligning the AI decisions with specialized medical knowledge.
Furthermore, comparing results with assessments by dermatologists reinforces the diagnostic accuracy of these technologies. Notably, the explicit consideration of ethnic and genetic diversity within the population, as discussed in Fujisawa et al. (30) and Pangti et al. (36), also stands out as a signigficant strength. By encompassing various skin types and demographic characteristics, such applications become more comprehensive and reliable in real-world scenarios. Taken together, these aspects underscore the relevance of these applications in medical practice and their potential to significantly contribute to early and accurate skin lesion detection.
When analyzing the studies, a consensus becomes evident regarding the importance of accessibility and availability of systems and applications for skin lesion detection and classification. However, many systems still fail fully meet these requirements due to resource limitations, technical complexity, or the absence of clear guidelines. To address this issue, broader collaboration among companies, accessibility experts, programmers, and users is crucial in translating intentions into practical actions. Such collaborative effort will result in significant benefits for all parties involved.
Finally, it is essential to ensure that AI applications are developed and tested ethically and responsibly. This includes safeguarding patient data privacy and security, as well as ensuring transparency in the of development and training processes of algorithms.
4 Conclusion
The application of artificial intelligence in dermatology has the potential to revolutionize the detection and diagnosis of skin lesions, especially in the case of melanoma, a severe and potentially fatal disease.
This review highlights that several studies are making significant advancements in improving image processing capabilities, pattern recognition, and deep learning. These advancements enable rapid and accurate analyses that can lead to real-time diagnoses. This evolution contributes to early detection of skin cancer, expanding the prospects for cure and minimizing the reliance on invasive procedures.
However, it is important to note that the vast majority of the solutions presented have not yet been validated in clinical settings or developed in collaboration with dermatologists and other healthcare professionals to ensure they meet patients' needs and are effective in clinical practice.
In summary, the solutions presented can help enhance the efficiency of healthcare services, reducing the time required for examinations and diagnoses. This can be especially important in areas with a shortage of healthcare professionals or in emergency situations where time is critical. However, they should be used with caution and responsibility, in collaboration with dermatologists and other healthcare professionals, to ensure they meet patients' needs and are effective in clinical practice.
Data availability statement
The original contributions presented in the study are included in the article/supplementary material, further inquiries can be directed to the corresponding author.
Author contributions
BF: Writing—original draft, Writing—review & editing. BO: Writing—review & editing. RP: Writing—review & editing. JP: Writing—review & editing. RL: Writing—original draft, Writing—review & editing. WC: Writing—review & editing. MR: Writing—review & editing. MG-B: Writing—original draft, Writing—review & editing.
Funding
The author(s) declare financial support was received for the research, authorship, and/or publication of this article. This work was supported by the Program of Support for the Institutional Development of the Unified Health System (PROADI-SUS,01/2020; NUP: 25000.161106/2020-61) and Hospital Israelita Albert Einstein.
Conflict of interest
The authors declare that the research was conducted in the absence of any commercial or financial relationships that could be construed as a potential conflict of interest.
Publisher's note
All claims expressed in this article are solely those of the authors and do not necessarily represent those of their affiliated organizations, or those of the publisher, the editors and the reviewers. Any product that may be evaluated in this article, or claim that may be made by its manufacturer, is not guaranteed or endorsed by the publisher.
References
1. Balaha HM, Hassan AE-S. Skin cancer diagnosis based on deep transfer learning and sparrow search algorithm. Neural Comp Appl. (2023) 35:815–53.
2. In T. Facts & Figures 2019: US Cancer Death rate has Dropped 27% in 25 Years. Washington, DC: American CancerSociety. (2019).
3. Fitzmaurice C, Akinyemiju TF, Al Lami FH, Alam T, Alizadeh-Navaei R, Allen C, et al. Global, regional, and national cancer incidence, mortality, years of life lost, years lived with disability, and disability-adjusted life-years for 29 cancer groups, 1990 to 2016: a systematic analysis for the global burden of disease study. JAMA Oncol. (2018) 4:1553–68. doi: 10.1200/JCO.2018.36.15_suppl.1568
4. Guy Jr GP, Machlin SR, Ekwueme DU, Yabroff KR. Prevalence and costs of skin cancer treatment in the US, 2002- 2006 and 2007–2011. Am J Prev Med. (2015) 48:183–7. doi: 10.1016/j.amepre.2014.08.036
5. Gordon R. Skin cancer: an overview of epidemiology and risk factors. Semi Oncol Nurs. (2013) 29:160–9. doi: 10.1016/j.soncn.2013.06.002
6. Leiter U, Keim U, Garbe C. Epidemiology of skin cancer: update 2019. Sunlight Vitamin D Skin Cancer. (2020) 123–39.
7. di Meo N, Vernoni S, Longone M, Trevisan G. Image gallery: Merkel cell carcinoma under the rainbow. Br J Dermatol. (2017) 177:e166–e166. doi: 10.1111/bjd.15815
8. Molina DAC, Rios-Duarte JA, Mu noz-Ordo nez S, Fierro-Lozada JD, Celorio-Murillo WJ, Hernandez-Amaris MF, et al. 33734 Patient education as the main target in skin cancer prevention: knowledge, attitudes, and practices toward sun exposure and use of sun protection. J Am Acad Dermat. (2022) 87:AB189. doi: 10.1016/j.jaad.2022.06.789
9. Hammad SSAEH, Gaber MA. Knowledge, attitudes and practices of the general puplic toward the harmful effects of sun exposure and protection. J Health Sci. 6:14007–20. doi: 10.53730/ijhs.v6nS2.8675
10. Besch-Stokes J, Brumfiel CM, Patel MH, Harvey J, Montoya J, Severson KJ, et al. Skin cancer knowledge, attitudes and sun protection practices in the hispanic population: a cross-sectional survey. J Racial Ethn Health Disparitie. (2022) 10:1293–303. doi: 10.1007/s40615-022-01314-6
11. Jiang S, Li H, Jin Z. A.visually interpretable deep learning framework for histopathological image-based skin cancer diagnosis. IEEE J Biomed Health Inform. (2021) 25:1483–94. doi: 10.1109/JBHI.2021.3052044
12. Parsons SK, Chan JA, Winifred WY, Obadan N, Ratichek SJ, Lee J, et al. Noninvasive Diagnostic Techniques for the Detection of Skin Cancers. (2011).
13. Malvehy J, Pellacani G. Dermoscopy, confocal microscopy and other non-invasive tools for the diagnosis of non-melanoma skin cancers and other skin conditions. Acta Derm Venereol. (2017) 97:22–30. doi: 10.2340/00015555-2720
14. Narayanamurthy V, Padmapriya P, Noorasafrin A, Pooja B, Hema K, Nithyakalyani K, et al. Skin cancer detection using non-invasive techniques. RSC Adv. (2018) 8:28095–130. doi: 10.1039/C8RA04164D
15. Garbe C, Peris K, Soura E, Forsea AM, Hauschild A, Arenbergerova M, et al. The evolving field of Dermato-oncology and the role of dermatologists: position paper of the EADO, EADV and task forces, EDF, IDS, EBDV-UEMS and EORTC cutaneous lymphoma task force. J Eur Acad Dermatol Venereol. (2020) 34:2183–97. doi: 10.1111/jdv.16849
16. Frisinger A, Papachristou P. The voice of healthcare: introducing digital decision support systems into clinical practice-a qualitative study. BMC Primary Care. (2023) 24:67. doi: 10.1186/s12875-023-02024-6
17. Giavina-Bianchi M, de Sousa RM, Paciello VZdA, Vitor WG, Okita AL, Prôa R, et al. Implementation of artificial intelligence algorithms for melanoma screening in a primary care setting. PLoS ONE. (2021) 16:e0257006. doi: 10.1371/journal.pone.0257006
18. Esteva A, Kuprel B, Novoa RA, Ko J, Swetter SM, Blau HM, et al. Dermatologist-level classification of skin cancer with deep neural networks. Nature. (2017) 542:115–8. doi: 10.1038/nature21056
19. Kwiatkowska D, Kluska P, Reich A. Convolutional neural networks for the detection of malignant melanoma in dermoscopy images. Adv Dermatol Allergol. (2021) 38:412. doi: 10.5114/ada.2021.107927
20. Goyal M, Knackstedt T, Yan S, Hassanpour S. Artificial intelligence-based image classification methods for diagnosis of skin cancer: Challenges and opportunities. Comput Biol Med. (2020) 127:104065. doi: 10.1016/j.compbiomed.2020.104065
21. Hosny KM, Kassem MA, Foaud MM. Classification of skin lesions using transfer learning and augmentation with Alex-net. PLoS ONE. (2019) 14:e0217293. doi: 10.1371/journal.pone.0217293
22. Barros WK, Morais DS, Lopes FF, Torquato MF, Barbosa RdM, Fernandes MA. Proposal of the CAD system for melanoma detection using reconfigurable computing. Sensors. (2020) 20:3168. doi: 10.3390/s20113168
23. Pyun SH, Min W, Goo B, Seit S, Azzi A, Wong DYS, et al. Real-time, in vivo skin cancer triage by laser-induced plasma spectroscopy combined with a deep learning-based diagnostic algorithm. J Am Acad Dermatol. (2022). doi: 10.1016/j.jaad.2022.06.1166
24. Felmingham C, MacNamara S, Cranwell W, Williams N, Wada M, Adler NR, et al. Improving Skin cancer Management with ARTificial Intelligence (SMARTI): protocol for a preintervention/postintervention trial of an artificial intelligence system used as a diagnostic aid for skin cancer management in a specialist dermatology setting. BMJ Open. (2022) 12:e050203. doi: 10.1136/bmjopen-2021-050203
25. Ramlakhan K, Shang Y. A mobile automated skin lesion classification system. In: 2011 IEEE 23rd International Conference on Tools with Artificial Intelligence. Boca Raton: IEEE. (2011) p. 138–41.
26. Afifi S, GholamHosseini H, Sinha R. A low-cost FPGA-based SVM classifier for melanoma detection. In: 2016 IEEE EMBS Conference on Biomedical Engineering and Sciences (IECBES). Kuala Lumpur: IEEE. (2016) p. 631–6.
27. Thissen M, Udrea A, Hacking M, von Braunmuehl T, Ruzicka T. mHealth app for risk assessment of pigmented and nonpigmented skin lesions–a study on sensitivity and specificity in detecting malignancy. Telemed e-Health. (2017) 23:948–54. doi: 10.1089/tmj.2016.0259
28. Roy SS, Haque AU, Neubert J. Automatic diagnosis of melanoma from dermoscopic image using real-time object detection. In: 2018 52nd Annual Conference on Information Sciences and Systems (CISS). Princeton, NJ: IEEE. (2018) p. 1–5.
29. Alizadeh SM, Mahloojifar A. A mobile application for early detection of melanoma by image processing algorithms. In: 2018 25th National and 3rd International Iranian Conference on Biomedical Engineering (ICBME). Qom: IEEE. (2018) p. 1–5.
30. Fujisawa Y, Otomo Y, Ogata Y, Nakamura Y, Fujita R, Ishitsuka Y, et al. Deep-learning-based, computer-aided classifier developed with a small dataset of clinical images surpasses board-certified dermatologists in skin tumour diagnosis. Br J Dermatol. (2019) 180:373–81. doi: 10.1111/bjd.16924
31. Udrea A, Mitra G, Costea D, Noels E, Wakkee M, Siegel D, et al. Accuracy of a smartphone application for triage of skin lesions based on machine learning algorithms. J Eur Acad Dermatol Venereol. (2020) 34:648–55. doi: 10.1111/jdv.15935
32. Bakheet S, Al-Hamadi A. Computer-aided diagnosis of malignant melanoma using gabor-based entropic features and multilevel neural networks. Diagnostics. (2020) 10:822. doi: 10.3390/diagnostics10100822
33. Abbas Q. Smart-Dermo: a computerize tool for classification of skin cancer using smartphone through image processing and fuzzy logic. Int J Comp Sci Netw Secur. (2020).
34. Bakheet S, El-Nagar A. A deep neural approach for real-time malignant melanoma detection. Appl Math. (2021) 15:89–96. doi: 10.18576/amis/150111
35. Dulmage B, Tegtmeyer K, Zhang MZ, Colavincenzo M, Xu S, A. point-of-care, real-time artificial intelligence system to support clinician diagnosis of a wide range of skin diseases. J Investigat Dermatol. (2021) 141:1230–5. doi: 10.1016/j.jid.2020.08.027
36. Pangti R, Mathur J, Chouhan V, Kumar S, Rajput L, Shah S, et al. A machine learning-based, decision support, mobile phone application for diagnosis of common dermatological diseases. J Eur Acad Dermatol Venereol. (2021) 35:536–45. doi: 10.1111/jdv.16967
37. Francese R, Frasca M, Risi M, Tortora G, A. mobile augmented reality application for supporting real-time skin lesion analysis based on deep learning. J Real-Time Image Proc. (2021) 18:1247–59. doi: 10.1007/s11554-021-01109-8
38. Sangers T, Reeder S, van der Vet S, Jhingoer S, Mooyaart A, Siegel DM, et al. Validation of a market-approved artificial intelligence mobile health app for skin cancer screening: a prospective multicenter diagnostic accuracy study. Dermatology. (2022) 238:649–56. doi: 10.1159/000520474
39. Jahn AS, Navarini AA, Cerminara SE, Kostner L, Huber SM, Kunz M, et al. Over-detection of melanoma-suspect lesions by a CE-certified smartphone app: performance in comparison to dermatologists, 2D and 3D convolutional neural networks in a prospective data set of 1204 pigmented skin lesions involving patients' perception. Cancers. (2022) 14:3829. doi: 10.3390/cancers14153829
Keywords: Skin cancer, artificial intelligence, melanoma, detection, classification, feature extraction
Citation: Furriel BCRS, Oliveira BD, Prôa R, Paiva JQ, Loureiro RM, Calixto WP, Reis MRC and Giavina-Bianchi M (2024) Artificial intelligence for skin cancer detection and classification for clinical environment: a systematic review. Front. Med. 10:1305954. doi: 10.3389/fmed.2023.1305954
Received: 02 October 2023; Accepted: 12 December 2023;
Published: 08 January 2024.
Edited by:
Zubair Shah, Hamad Bin Khalifa University, QatarReviewed by:
Ionela Manole, Colentina Clinical Hospital, RomaniaGiusto Trevisan, University of Trieste, Italy
Copyright © 2024 Furriel, Oliveira, Prôa, Paiva, Loureiro, Calixto, Reis and Giavina-Bianchi. This is an open-access article distributed under the terms of the Creative Commons Attribution License (CC BY). The use, distribution or reproduction in other forums is permitted, provided the original author(s) and the copyright owner(s) are credited and that the original publication in this journal is cited, in accordance with accepted academic practice. No use, distribution or reproduction is permitted which does not comply with these terms.
*Correspondence: Brunna C. R. S. Furriel, YnJ1bm5hLnNpbHZhQGlmZy5lZHUuYnI=
†ORCID: Brunna C. R. S. Furriel orcid.org/0000-0003-1654-5980