- 1Department of Clinical Laboratory, Gongli Hospital of Shanghai Pudong New Area, Shanghai, China
- 2Department of Endocrinology, Rheumatology and Immunology, The Second Affiliated Hospital of Shantou University Medical College, Shantou, China
- 3Goldsite Diagnostics Inc., Shenzhen, China
- 4Department of Clinical Laboratory, Baotou Central Hospital, Baotou, China
- 5Department of Clinical Laboratory, Guilin Hospital of Integrated Traditional Chinese and Western Medicine, Guilin, China
- 6Department of Clinical Laboratory, Jinling Hospital, Affiliated Hospital of Medical School, Nanjing University, Nanjing, China
- 7Department of Clinical Laboratory, First Affiliated Hospital of Dalian Medical University, Dalian, China
Worldwide, kidney disease has grown to be an important global public health agenda that reduces longevity. Medical institutions around the globe should enhance screening efforts for kidney disease, to facilitate early kidney disease detection, diagnosis, and intervention. Common screening methods for nephropathy encompass renal tissue biopsy, urine dry chemistry tests, urine formed element analysis, and urine-specific protein assays, among others. These methodologies evaluate renal health by scrutinizing a spectrum of biomarkers. Precise classification and quantitative analysis of these biomarkers can assist in determining the site and extent of kidney injury, as well as in assessing treatment efficacy and prognosis. In this paper, we reviewed the methods and biomarkers for kidney disease and also the integration of multiple biomarkers. With the aim of reasonable applying these markers to the early detection, accurate diagnosis, and scientific management of kidney disease, thereby mitigating the threat posed by kidney disease to human health.
1 Background
Kidney disease is classified as chronic kidney disease (CKD) or acute kidney disease (AKD) depending on their duration (Figure 1). Acute kidney injury (AKI) is defined as a greater than 50% increase in serum creatinine (sCr) levels within 7 days or a rise to 0.3 mg/dL within 2 days. It is a prevalent condition that affects 10–20% of all hospitalized inpatients and is linked to high rates of morbidity, mortality, and medical expenses (1). In AKI survivors, CKD, heart disease, and other associated comorbidities are more likely to develop. Clinical practice guidelines for AKI (KDIGO) were based on changes in sCr and urine volume. sCr is easily affected by diet, muscle content and other factors; urine volume is also easily affected by urinary tract obstruction, volume status, and other factors. Due to limited sensitivity and specificity, risk stratification systems and models for AKI are rarely clinically validated and accepted (2, 3). And it cannot suggest the etiology of AKI (4). Therefore, the need for early, sensitive, stable, and reliable AKI indicators is still urgent. AKD refers to the period of acute or subacute injury and reduced kidney function that persists for 7 to 90 days following AKI. This interval is a significant phase after the occurrence of AKI, and the management during this time is crucial to the outcome of AKI (2, 5).
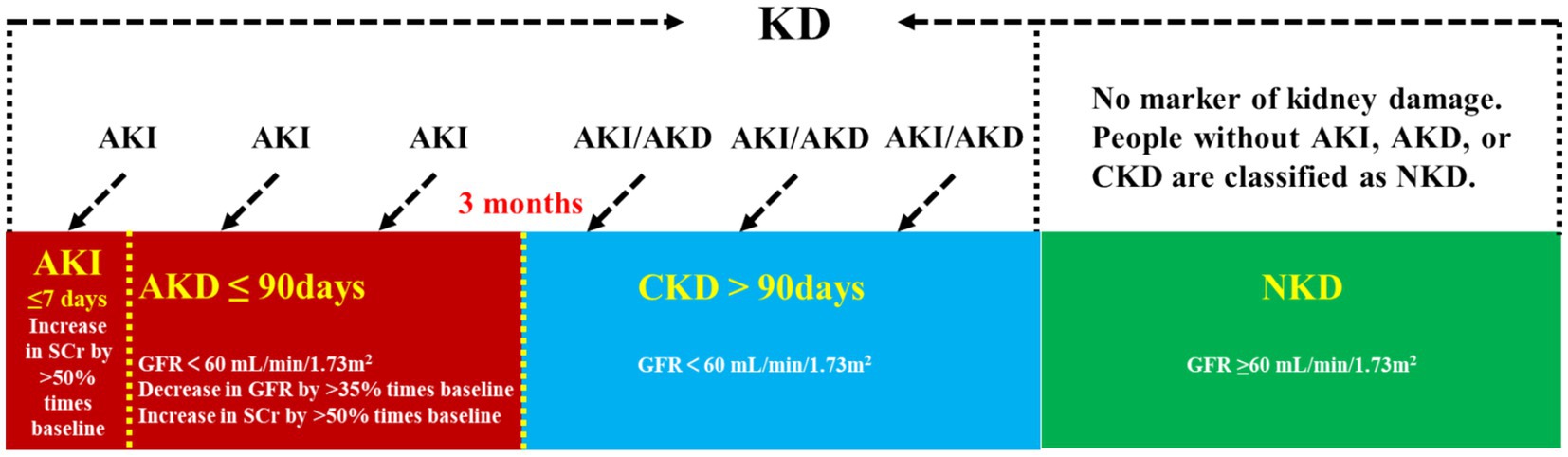
Figure 1. Kidney disease classification. KD, kidney disease; AKI, acute kidney injury; AKD, acute kidney disease; CKD, chronic kidney disease; NKD, no known kidney disease; SCr, serum creatinine; GFR, glomerular filtration rate.
CKD denotes a condition where the renal structure or function is abnormal for a period exceeding 3 months. It, characterized by its high prevalence, high disability rate, high medical expenditure, and low awareness, has become a significant global health threat. Global burden of disease studies indicated that in 2017, the prevalence of CKD worldwide was 9.1%, affecting approximately 700 million patients, with one-third of them residing in China and India (6). In the 2023 Sixth National Chronic Disease Prevalence Survey in China, the current proportion of CKD was found to be 8.2%, with a corresponding awareness rate of just 10% (7). Based on the glomerular filtration rate (GFR), kidney disease can be classified into five stages, with stages 3–5 being designated as CKD. During these stages, renal function progressively deteriorates. If left untreated, the disease can advance to stage 5, which is known as end-stage renal disease (ESRD). ESRD, if not addressed promptly, can lead to a myriad of complications and poses a significant risk to life.
In this paper, we reviewed the methods of kidney disease detection and the characteristics of different biomarkers in the diagnosis and progression of kidney disease. Concurrently, we explored the value of combining multiple markers in kidney disease. Thus, our goal is to increase people’s knowledge of kidney disease markers and to choose more sensible and sensitive detection techniques and markers when assessing kidney function.
2 The methods of kidney disease detection
There are many diagnostic methods for kidney disease, including renal biopsy and various clinical indicators. Among them, the clinical indicators include urinary formed components, urinary biochemical indicators (such as Cr and urea nitrogen), and urine specific protein markers. The comparison of each index detection method is shown in Table 1.
3 Biomarkers of kidney disease
The diverse biomarkers have different functions, and by the character and concentration of these substances, they enable precise diagnosis of the location and severity of kidney injury. This information is crucial for guiding treatment decisions, evaluating the prognosis of diagnostic and therapeutic interventions, and conducting comprehensive kidney assessments as part of routine health screenings. Broadly speaking, these markers can be categorized based on their anatomic localization and pathophysiological relevance into three main groups: (a) biomarkers that reflect glomerular and tubular (proximal tubule, medullary loop, and distal tubule) function and injury; (b) inflammatory markers; and (c) markers associated with repair and fibrosis (Figure 2).
3.1 Glomerular filtration rate
Creatinine (Cr) is the terminal catabolite of creatine and phosphocreatine, and its generation is therefore mainly dependent on muscle mass. Most Cr is eliminated by glomerular filtration; thus, it can be employed to assess kidney function as an endogenous biochemical marker. The international guideline-recommended screening marker for CKD, eGFR, can be calculated for disease staging and classification by measuring sCr and urine Cr. However, filtration indicators like Cr have inadequate sensitivity and specificity for diagnosing kidney disease; it is also raised when there is no kidney damage, but it can also stay the same if the patient has good underlying renal function and the damage is not severe (8–10). Cr is usually detected in urine biochemical analysis together with urea nitrogen and uric acid (Table 2). And it is often used in conjunction with other markers; the diagnostic detection rate of linked disorders is improved (11, 12).
Cystatin C (CysC) is a 13-kDa cysteine proteinase inhibitor protein, which is produced by all nucleated cells. It is freely filtered by the kidney with near-complete reabsorption and catabolism in the proximal tubule and no significant urinary excretion. So urinary CysC can indicate tubular damage. It is most often used as an indicator to assess glomerular filtration function, like Cr. Unlike Cr, serum CysC levels are not influenced by muscle mass, gender, age, body size and composition, or nutritional status. CysC level increases in early diabetes and pre-diabetic diabetic nephropathy (DN). Apart from other biomarkers, CysC is also an independent association with cardiovascular disease, ESRD, and all-cause mortality (13). The usage of CysC is limited by higher detection costs than Cr and standardization problems. Despite the release of an internationally certified Cyc C reference material (ERM-DA471/IFCC) by the International Federation of Clinical Chemistry (IFCC), significant differences still exist between tests from different manufacturers (14). In recent year manufacturers have markedly improved the accuracy and between-method agreement of CysC measurement procedures, which allows for greater confidence in estimated GFR relying on CysC (15).
Proenkephalin (PENK) is produced by proenkephalin A (the precursor molecule of the enkephalin family). Penk is a potential biomarker to measure glomerular function in critically ill patients since it does not bind to proteins in plasma and is exclusively filtered in the glomerulus. PENK may be a more suitable and accurate marker for determining real GFR since it has a greater correlation with measuring GFR (mGFR) than the Cr (16). According to numerous studies, plasma PENK concentrations can be used to forecast clinical outcomes and future renal deterioration in a variety of patients, including those with burns, sepsis, and acute heart failure (17).
3.2 Biomarkers of glomerular function
3.2.1 Microalbumin
mALB is a sensitive biomarker for early renal damage, particularly in diabetic and hypertensive nephropathy, and is widely used for diagnosis and monitoring of glomerular diseases (18). mALB elevation may also result from non-glomerular diseases such as urinary tract infections or heart failure, so it cannot be used alone for glomerular disease diagnosis and should be combined with other biomarkers like sCr and CysC for comprehensive evaluation (19). In the 2024 KDIGO CKD Guideline, urinary mALB-to-Cr ratio (UACR) is used to define and categorize CKD. Although albuminuria has been shown to be a crucial diagnostic and prognostic indicator of diabetic CKD, its daily fluctuations have not been sufficiently taken into account. Multiple urine collections for UACR may improve the capacity to monitor changes over time in clinical but may not be necessary for the diagnosis of albuminuria (20). mALB levels correlate with renal prognosis and cardiovascular disease risk, enabling prediction of progression to ESRD and cardiovascular events in patients with CKD (21).
3.2.2 Urinary transferrin
TRF is a 76.5 kDa glycoprotein that has been widely studied as a potential marker of glomerular injury. The negative charge of urinary TRF is less than that of urinary mALB. When the charge barrier of the glomerulus is damaged in the early stage, and urinary TRF is more likely to leak out than urinary mALB. Therefore, transferrin can more sensitively reflect the damage of the glomerular charge barrier. Urinary TRF had a sensitivity of 75% and a specificity of 100% in predicting early DN (22). Compared to mALB, TRF levels may be a more sensitive indicator for identifying glomerular damage and nephropathy in diabetic patients (23). According to reports, over 30% of type 2 diabetes mellitus patients with normal proteinuria had higher urine TRF levels (24).
3.2.3 Urine immunoglobulin G
Immunoglobulin G (IgG) is a protein of high molecular weight (150 kD), which is excreted in large quantities when the permselectivity of the glomerular capillary wall is more severely disrupted. When the glomerulus is seriously damaged, UIgG is generally discharged in large quantities, forming the so-called non-selective proteinuria in clinic. The increase in UIgG reflects the serious damage to the glomerular basement membrane (25). In the 2020 KDIGO guidelines, UIgG was included in risk stratification for membranous nephropathy (UIgG < 250 mg/d is intermediate risk; UIgG > 250 mg/d is high risk). UIgG will worsen tubule interstitial damage in patients with IgA nephropathy (IgAN) whose global hard rate is higher than 25%. It can also indicate the extent of tubule atrophy and interstitial fibrosis (26).
3.2.4 Urine α2-macroglobulin
A key inhibitor of plasma proteinase, A2M is primarily produced by hepatocytes and mononuclear phagocyte cells. It is essential for controlling tissue repair, signal transduction, protease activity, and nutritional maintenance. A wide range of physiological and pathological processes are influenced by A2M, which is also essential for protecting against infections and external toxins. It also regulates hormones, cytokines, and other bioactive lipid factors (27). Studies revealed elevated levels of urinary A2M in patients with DN, indicating that urinary A2M levels rose with the aggravation of the condition (28). A2M also is a regulator of the fibrogenic process in glomerular mesangial cells. Smad3, NFAT5, and FOXP1 were identified as critical regulators of high glucose-induced A2M promoter activation in glomerular mesangial cells (29).
3.2.5 Ferritin
Ferritin is a soluble protein with a molecular weight of 450 kDa, typically made up of 12 “light” chains (Flc) and 12 “heavy” chains (Fhc) (30). Because the glomerulus typically cannot filter the higher molecular weight of ferritin and because there is no filterable free FLC or FHC in the bloodstream, ferritin in urine comes from the kidney (renal tubular epithelial cells). The average concentration of ferritin in normal human urine was 2.2 ug/L, which is only 3% of the level of serum ferritin (31). Therefore, tubulointerstitial lesions brought on by glomerular damage and other CKD—of which renal tubular iron deposition is one of the reasons—are potential causes of elevated urine ferritin levels (32). Studies have indicated that patients with lupus nephropathy (LN) had a considerable rise in urine ferritin levels, with the degree of the increase being correlated with the severity of the disease. Ferritin and urinary ferritin/Cr ratio (UFCR) are a potential biomarker for LN activity (33, 34).
3.3 Biomarkers of kidney tubular
3.3.1 Urine α-1-microglobulin, urine β-2-microglobulin, and retinol binding protein
α-1-microglobulin (A1MG) is a 27-kDa glycoprotein produced by the liver. A1MG levels are very stable in several pathological conditions: no significant changes were seen in the serum of patients with neoplastic diseases, central nervous system disorders, infections, rheumatoid arthritis and other disorders. Altered total A1MG concentrations in plasma or serum are always related to impaired liver or kidney functions (35). Urine A1MG is more crucially used in clinical applications as a sensitive indicator of tubular damage.
β-2-microglobulin (B2MG) is a 11.8-kDa polypeptide, which forms the beta chain of the human leukocyte antigen class I molecule. It is present on the surface of most nucleated cells. Most of B2MG is normally eliminated by the kidney via glomerular filtration and subsequent tubular. Extrarenal breakdown and elimination appear to be negligible (36).
Retinol binding protein (RBP), was identified in immunoelectrophoresis in 1961. Only a small portion is eliminated from the urine after being filtered via the glomeruli, with the majority being reabsorbed by the proximal renal tubular epithelial cells (37).
The tubular dysfunction biomarkers, urine A1MG, urine B2MG and RBP, are low-molecular-weight proteins that can be used to evaluate the tubular functions’ potential for reabsorption. A1MG had higher sensitivity and specificity than B2MG in identifying drug-induced kidney damage (38). RBP was a superior option because β2-MG was unstable in acid urine (39). Studies have shown that RBP could be used as reliable or good predictors of diabetic kidney disease (DKD), and the β2MG displayed a weaker diagnostic ability than RBP (40).
3.3.2 Kidney injury molecule 1
KIM-1 is a type 1 transmembrane protein that has a mucin and immunoglobulin domain. In the proximal tubule of the post-ischemic rat kidney, its expression is noticeably elevated (41). In cases of acute or chronic renal damage, KIM-1 expression increases, particularly in the proximal convoluted tubule’s apical membrane. By identifying phosphatidylserine (PS) on the surface of small extracellular vesicles (sEVs), it can also function as a receptor to enhance sEV absorption and exacerbate tubulointerstitial inflammation (TII); this is correlated with the severity of acute and chronic kidney injury (42, 43). There is no Kim-1 in the urine when the kidneys are in health (44). Therefore, KIM-1 is an ideal potential marker for renal injury detection, particularly for proximal tubular (PT) injury early assessment. It is yet unknown how KIM-1 works, but it may possibly be a potential biomarker for neutrophil antibody-associated vasculitis with glomerulonephritis AKI and tubulointerstitial injury (45).
3.3.3 N-acetyl-β-d-glucosaminidase
NAG is an enzyme found in the lysosomes of proximal renal tubular cells. Its molecular weight is large enough to prevent it from passing through the usual glomerular basement membrane. It has been shown that an elevated excretion of NAG is more specific for renal tubular disease and indicates active tubular injury (46). There is a report showing that burn patients show persistent NAG elevation during aggressive treatment, indicating that burn patients still have persistent tubular injury after transient AKI (47). The urine NAG/Cr biomarker level has a significant difference between healthy participants and people with type 2 diabetes mellitus (T2DM). It should be measured for the people with T2DM of the first-time diagnosis (48).
3.3.4 Neutrophil gelatinase-associated lipocalin
The lipocalin-2 superfamily includes the tiny protein known as neutrophil gelatinase-associated lipocalin (NGAL). In the kidneys and other human tissues, it is typically expressed at relatively low levels (49). When renal tubular epithelial cells are damaged, it is extensively expressed. As a result, it can be easily found in adult blood and urine shortly after acute renal injury. It has also been demonstrated that urinary NGAL can predict the degree of acute kidney damage following heart surgery as well as the long-term renal outcomes following therapy in an intensive care unit (50). For the diagnosis of AKI, a number of blood and urine NGAL cut-offs have been suggested; however, these cut-offs have not been standardized (51). Dialysis and chronic renal disease patients also have higher levels of NGAL.
3.3.5 Tissue inhibitor of metalloprotease-2 and insulin-like growth factor binding protein 7
TIMP-2 and IGFBP7 are involved in G1 cell cycle arrest and are released in the urine during tubular epithelial cell stress (52). In renal biopsies from nephropathy patients, their expression was significantly elevated, particularly in the renal tubular region: TIMP-2 was mainly detected in the collecting duct, whereas IGFBP7 was mainly detected in the proximal and distal tubules (53). Urine [TIMP-2]*[IGFBP7] is a biomarker for AKI risk assessment and may augment AKI staging for patients with functional criteria for AKI (54). Although it is best utilized in conjunction with other indicators or clinical risk factors, it can also be used to predict a bad prognosis in AKI. It can also be used to forecast a poor outcome in AKI, although preferably in conjunction with other clinical risk factors or markers (55).
3.3.6 Liver-type fatty acid-binding protein
FABPs are a class of low-molecular-weight cytosolic proteins that have between 14,000 and 15,000 homologies. Numerous tissues, such as the intestinal mucosa, the liver, the heart, adipose tissue, the kidney, muscle, and other tissues, contain it. In mammals, at least nine FABPs have been identified. Liver-FABP, or L-FABP, was initially discovered in the liver and is mostly expressed in the kidney’s proximal tubular epithelial cells (56). Urine L-FABP is a potential biomarker for tracking the decline of renal function and early kidney disease identification. Research has indicated that in patients with renal impairment, changes in urine L-FABP levels occur before changes in urinary albumin (57). The urine of patients with AKI, particularly acute tubular necrosis, has higher amounts of L-FABP (58). Kamijo et al. observed in their study of type 2 diabetes patients that increased urine L-FABP is a risk factor for the advancement of DKD and that these levels properly reflect the stage of DKD (59).
3.3.7 Trefoil factor 3
TFF3 is a member of the human trefoil factor family. Under healthy conditions, it’s extensive production in renal tubules. TFF3 may be an important molecule for future studies of kidney disease. Serum and urine TFF3 levels in CKD patients are typically higher than in healthy controls, and they rise significantly as the disease progresses (60). According to a study of kidney transplant recipients, TFF3 levels in their serum and urine increased the day following the surgery and then declined 6 months and a year later. TFF3 has great potential for renal transplant patient monitoring (61).
3.3.8 Activating transcription factor 3
Exosomes are the nano-vesicles released by most of the cells that contain a variety of proteins. and it is an important source of biomarkers. In urine exosomes, ATF3 is one of the key indicators (62). ATF3 plays a crucial role in how cells react to stress in their internal and external environments. Additionally, it plays a major role in physiological and pathological processes such as wound healing, tumor development, cell adhesion, and homeostasis maintenance (63). ATF3 was found earlier than sCr in the urine of patients with AKI, but not in normal persons, sepsis patients without AKI, or patients with CKD. Urinary ATF3 may therefore be a new biomarker for identifying renal tubular cell injury in early AKI and AKI in sepsis (62, 64).
3.3.9 Procollagen type III N-terminal propeptide
Collagen type I and III are the two major proteins involved in extracellular matrix (ECM) remodelling, which leads to the accumulation of fibrotic tissue in several organs, such as the heart or the kidneys. Procollagen type I carboxy-terminal propeptide (PICP) and PIIINP are peptidases that activate two types of collagen, which can indicate the rate of collagen production and be associated with fibrosis in the heart, liver, muscle, renal tubulointerstitium, and others. They can be used to gauge the severity of a number of illnesses and track the progression of fibrosis and tissue healing (65). Research shows PICP is an independent predictor of mortality in ESRD patients who are not yet on dialysis. P3NP levels in the urine may be used as a non-invasive early marker of renal fibrosis, and the ratio of P3NP to Cr can also show how renal fibrosis is progressing in recipients of renal transplants (66, 67).
3.3.10 Dickkopf-related protein 3
DKK-3 is a secreted glycoprotein that is synthesized by stressed tubular epithelia. And in vitro, it is highly expressed in mesenchymal cells and mesenchymal progenitor cells (68). DKK3 is secreted in the renal tubular epithelium under stress and leads to tubulointerstitial fibrosis via the Wnt signaling pathway (69, 70). DKK3 was elevated in patients with reduced eGFR (71). In children with CKD, urinary DKK3 can also indicate a short-term risk of renal decline. By identifying individuals who benefit from pharmacological renal protection, such as intense hypertension therapy, it may also allow for customized drug therapy (72). As a consequence, it is considered to be a valuable biomarker for assessing the different causes of renal failure.
3.3.11 Uromodulin
UMOD, or Tamm-Horsfall protein, is the most prevalent protein in healthy people’s urine and is expressed by renal tubular epithelial cells localized in the thick ascending limb of the loop of Henle. The plasma and 24 h urine UMOD are direct markers of Henle’s loop integrity (73). UMOD may indicate the distal renal tubules’ capacity for protein production. Urinary UMOD has been found to be inversely correlated with the progression of CKD, the risk of cardiovascular disease-related death, and the incidence of AKI following heart surgery. These findings may be helpful in determining the risk of death for patients with diabetes and CKD, particularly when it comes to the risk of cardiovascular disease-related death (74). Since UMOD cannot cross through the placental barrier, it can be used to identify AKI in neonates and is also regarded as a biomarker of fetal renal tubular development (75). Recent studies have demonstrated its role as a marker of tubulointerstitial fibrosis, urine UMOD is independently associated with tubulointerstitial fibrosis in both human kidney biopsies and a mouse model of fibrosis (76).
3.4 Biomarkers of kidney inflammation
3.4.1 Interleukin-18
A crucial proinflammatory cytokine secreted by macrophages, IL-18 contributes to inflammation and the advancement of renal disease. Renal injury and fibrosis may be lessened by the loss or inhibition of IL-18, which offers a potential therapeutic target for the treatment of renal diseases. CKD is related to the activation of inflammasomes, the production of IL-18, and the stimulation of downstream pro-inflammatory signaling pathways (77, 78). Elevated urine IL-18 levels within 24 h of intensive care unit admission have been independently associated with poor clinical outcomes at 28 days and can offer valuable information for prognostic assessment. They also have some usefulness in predicting the development of AKI (79). In patients who have received a kidney transplant, IL-18 is also a reliable and early indicator of the requirement for dialysis and the recovery of graft function after 3 months (80).
3.4.2 Monocyte chemoattractant protein-1
MCP-1 belongs to the family of chemokines. It plays a crucial part in the pathophysiology of inflammatory nephropathy and promotes monocyte release from the bone marrow, creates chemokine gradients, and guides monocyte migration to inflammatory areas (81). Urine MCP-1 is produced by the kidney rather than as a result of serum MCP-1 filtration (lack of correlation between urinary and serum MCP-1 levels) (82). Urine MCP-1 is a more useful indicator of lupus nephritis activity than blood (83).
3.4.3 Chitinase-3-like protein 1 or YKL-40
YKL-40 is a glycoprotein. There are several cells that can express it besides monocytes, such as neutrophils, fibroblasts, chondrocytes, vascular smooth muscle, endothelial cells, hepatic stellate cells, colon, duct, and airway epithelial cells, and macrophages (84). Similar to MCP-1, urine YKL-40 has been demonstrated to be a marker of inflammation and structural kidney damage. One of the main causes of AKI and CKD development is persistent renal inflammation, and elevated levels of this inflammation have been linked to worsened renal function in hospitalized patients (85). Higher urine levels of YKL-40 and KIM-1 have been associated with an increased risk of all-cause death in people with CKD and diabetes (74). The report is suggests that urine YKL-40, as opposed to urine or plasma NGAL, be employed as a precise and trustworthy biomarker to identify patients at risk of AKI after transplantation (86).
3.4.4 Calprotectin
CLP is a calcium and zinc-binding protein with a relative molecular mass of 36 KD, which is a trimer composed of two heterologous calcium-binding proteins (S100A8 and S100A9). It belongs to the S100 protein family and is produced by neutrophils and monocytes during inflammation (87). Urinary concentration of CLP is substantially increased in intrinsic AKI. And CLP is more effective than NGAL and KIM-1 at differentiating between prerenal and intrinsic AKI, and it would avoid needless biopsies in cases of prerenal disease (88, 89). In CKD patients, CLP is involved in vascular calcification, and CLP in blood can also be used as a biomarker to predict the occurrence of cardiovascular disease. Targeting calprotectin significantly improves vascular calcification in patients with CKD (90). As an acute-phase protein, circulating CLP is significantly correlated with a higher risk of new-onset CKD in the general population (91).
3.5 Other
3.5.1 Circular RNA
CircRNA is a unique kind of long non-coding RNA that is distinguished by its circular, covalently closed structure, which resists exonucleases from breaking it down. It is very stable and exhibits tissue and cell type selectivity in its expression pattern. CircRNA is widely distributed in bodily fluids (such as blood, urine, and saliva) and exosomes, which are released by the majority of cell types. As a result, it can be applied as a novel liquid biopsy marker for numerous illnesses (92). Different forms of kidney disease also showed distinct patterns of circRNA expression. The expression of circ-0114427 increased in a mouse model of cisplatin-induced AKI and in cultured renal tubular cells exposed to cisplatin; circactr2 was upregulated in human proximal tubule epithelial cells (HK-2) exposed to high hyperglycemia. Human proximal tubular epithelial cells (HK-2) exposed to elevated glucose showed an upregulation of circACTR2. In both cultivated renal tubular cells exposed to cisplatin and a murine model of AKI caused by cisplatin, circ-0114427 expression was increased (93). CircRNA study in kidney disease is still in early stages, and further research is required to fully understand its potential as a biomarker and molecular therapeutic target.
3.5.2 Complement
The complement system is a part of innate immunity, which is crucial for immunological modulation, pathogen infection protection, and internal environment stability. According to numerous studies, activation or dysregulation of complement has been implicated in the pathophysiology of an increasing number of kidney disease. In 2024, KDIGO (Kidney Disease: Improving Global Outcomes) published the first expert consensus on the role of the complement system in kidney disease (94). Many complement inhibitors that target complement are currently being developed for utilization in treating kidney disease (Table 3).
4 Novel biomarkers
Increasing studies have shown that omics (including genomics, transcriptomics, proteomics, metabolomics, epigenomics, and more) was used for the identification of various biomarkers in renal diseases.
4.1 Genomics and metagenomes
According to the study by Nadia Ayasreh et al., MUC1, UMOD, HNF1B, REN and SEC61A1 have been found to be the pathogenic genes of autosomal dominant tubulointerstitial kidney disease, suggesting that these genes play an important role in the maintenance of renal function (95). The number of genome-wide association studies (GWAS) of kidney function has increased, from single-ethnic to cross-ethnic studies, from tens of thousands to millions of people, and the number of loci found has also increased. In a 2019 study, researchers identified 308 loci with 760,000 participants for the discovery group that explained 7.1% of the eGFR variation, validation with another group of 280,000 participants and the addition of the two groups resulted in the identification of 264 loci (96).
The human body is known to have a diverse and abundant collection of bacteria known as the microbiota (97). Furthermore, a number of diseases, including obesity, type 2 diabetes, hepatic steatosis, intestinal bowel disorders, and many cancers, are now thought to be associated with the gut microbiota (98). Mei Z et al. conducted a comprehensive study of the T2DM microbiome, analyzing 8,117 shotgun metagenomes. And they found that dysbiosis in 19 phylogenetically diverse species was associated with T2DM (false discovery rate < 0.10), for example, enriched Clostridium bolteae and depleted Butyrivibrio crossotus. These microorganism changes were potentially underlying T2DM pathogenesis (99). Linh HT et al. have identified an increase in Klebsiella oxytoca genes in the blood of DKD patients. And its copies correlated with higher sCr and BUN levels together with lower eGFR in DKD patients (100). Through microbial metabolites, microbes can communicate with their hosts. The latest publication showed that gut microbiota (like Lactobacillus species) could affect membranous nephropathy (MN) through tryptophan-produced indole derivatives that engage host receptors (101). A study by Zeng Y et al. found that both DKD patients and mice had significantly lower levels of indole-3-propionic acid (IPA), a metabolite generated from tryptophan, which was negatively correlated with impaired renal function. Supplementing with IPA improved albuminuria and strengthened the glomerular filtration barrier (102).
4.2 Proteomics and metabolomics
Proteomics uses mass spectrometry and bioinformatics analysis techniques to enable a comprehensive analysis of the proteins in a sample. Using untargeted proteomic profiling of circulating proteins, Md Dom ZI et al. identified three elevated protective proteins-fibroblast growth factor 20, angiopoietin-1, and tumor necrosis factor ligand superfamily member 12-that were associated with protection against progressive renal decline and progression to ESRD (103). Another study by Niewczas MA et al., screened 194 circulating inflammatory proteins for KRIS (kidney risk inflammatory signature), which consists of 17 proteins enriched in tumor necrosis factor-receptor superfamily members, a marker of inflammatory processes involved in the development of ESRD in diabetes (104). Catanese L et al. analyzed the proteomics data of urine samples and identified a peptidomic classifier for renal fibrosis containing 29 peptide fragments corresponding to 13 different proteins. And urinary proteomics analysis can serve as a non-invasive tool to evaluate the degree of renal fibrosis, which allows repeated measurements in contrast to kidney biopsy (105). These proteins may serve as biomarkers to stratify diabetic individuals according to risk of progression to ESRD and might also be investigated as points to new therapeutic targets of DN.
Metabolomics is an analytical study technique that shows the byproducts of biological processes in living things using high-throughput analysis. These metabolites consist of a variety of compounds, including sugars, lipids, and amino acids (106). Metabolomics has considered an efficient tool in the search for biomarkers that are critical for precision health approaches and improved diagnostics. A study by Wang et al. demonstrates that CKD is associated with phosphatidylcholine (PC) metabolism disorders by conducting ultra-high-performance liquid chromatography, and the administration of Rheum officinale can improve impaired kidney function and aberrant PC metabolism in CKD rats (107).
4.3 Integrating multi-omics
The integration of multi-omics data, including the genome, transcriptome, and metabolome, will play a key role in biomarker identification, uncovering intracellular molecular networks, and complex disease mechanisms. Wang et al.’s integrated metabolite–protein network delivers a thorough view of the “disease interactome,” offering mechanistic insights for improved clinical management (108). Si S et al.’s integrated analysis of plasma proteome and transcriptome data identifies 32 potential therapeutic targets for CKD, kidney function, and specific CKD clinical types. These proteins included 12 proteins with prior Mendelian randomization support and 20 novel causal proteins that have not been previously reported (109).
5 Biomarker combination
In most cases, detecting biomarker combinations is more efficient than using just one sign. As a result, a reasonable combination of several biomarkers can more properly estimate the degree of kidney health, injury location, and the type of disease (Table 4) (110–112).
Cr is the most often used marker in combination with others. Two crucial endogenous biomarkers for eGFR are Cr and CysC. The accuracy of eGFR is also increased by combining the two markers, particularly in cases where Cr and CysC are inconsistent (113). Urinary Cr can also be used to calculate the ratio with other markers, “correct” the quantitative results of other markers (such as mALB, NGAL, NAG, etc.), and reduce the impact of variables like urine concentration on the results (33, 46, 114, 115). The most important is the albumin-to-Cr ratio (ACR), a quick, easy, and precise indicator of the body’s albumin excretion. Its main purpose is to categorize albuminuria in cases of CKD. The cause and mechanism of AKI can also be determined by combining biomarkers of renal injury (e.g., uNGAL) with markers of renal filtration function (e.g., SCr, CysC) (116). For instance, NGAL levels may be influenced by underlying infections however if levels of NGAL were elevated along with elevations of KIM-1 and LFABP the diagnostic likelihood of AKI would be enhanced (117).
In addition to normal marker association studies, Frank Bienaim et al. use LASSO logistic regression analysis to examine 30 urine biomarkers. They find multiple possible biomarker combinations that indicate the rapid advancement of CKD (118).
Table 4 displays the evolution of a few kidney disease biomarker combinations.
6 Discussion and future directions
A prevalent issue all around the world is kidney disease. Therefore, there are many markers for the diagnosis of kidney disease. Under specific conditions, these markers have a strong relationship with the diagnosis, management, and prognosis of kidney disease (such as AKI, CKD, lupus nephritis, nephrotoxicity, renal transplantation, DN, hypertensive nephropathy, etc.). The results of them may differ due to variations in the environment, interfering factors, and detecting methods. Many new biomarkers have been discovered and validated, but not all of them are specific for a specific kidney disease. At the same time, the specificity of these biomarkers may vary depending on the clinical situation of the patient. A broad understanding of the unique characteristics of biomarkers, as well as their sensitivity and specificity in different types of kidney disease, is needed. Finally, there is a lack of uniform testing standards and cutoff values for these markers, although some have been approved by the FDA (e.g., Kim-1, TIMP-2*Igfbp-7) (119). Therefore, the introduction of these biomarkers into medical practice remains challenging. On the other hand, the combination of biomarkers often leads to better detection performance, so exploring the combination of more markers is also an important research direction to obtain more accurate diagnostic results (like Frank Bienaim et al.).
The field of genomics has advanced quickly since 1986, propelling the growth of fields such as transcriptomics, proteomics, metabolomics, epigenomics, and more. Using omics technology to analyze the body fluids of patients with kidney disease, followed by machine learning tools and pathway analysis, differs from traditional research methods in that it allows us to rapidly screen a large number of disease-related candidate markers and potential therapeutic targets (120). However, the application of omics in kidney disease faces two significant challenges: (a) Due to the limited detection technology, few omics-screened markers can be comprehensively and in-depth verified, so they are still difficult to apply to clinical practice; (b) The transformation of biomarkers from basic research to clinical application is a complex process. It requires technological breakthroughs and large-scale population studies; (c) In the application of clinical practice, it is necessary to strengthen the education and training of clinicians, and develop user-friendly tools, so that clinicians can interpret the results easily and conveniently.
With the increase of detection methods, markers and OMICS technology to generate more and more large data sets, artificial intelligence (AI) has become an important tool to analyze these complex data. The application of AI in kidney diseases is gradually increasing and shows great potential. AI technologies, especially machine learning and deep learning, can be used to analyze complex clinical data, including biochemical indicators, imaging data, and electronic medical records to help clinicians better diagnose kidney diseases. AI technology can also use patients’ genetic information, biomarkers and drug response data to customize treatment plans and achieve precision diagnosis and treatment. However, the application and promotion of AI face many problems such as data privacy and security issues, transparency and interpretability of algorithms, complex regulatory environment, standardization and quality control. Therefore, the economic factors and practical difficulties involved in integrating these technologies into existing health care systems may be greater than we think.
However, it will be essential to clinical diagnosis and treatment, including focused therapy and exact diagnosis, to improve patient diagnosis, treatment, and prognosis as technology develops and research becomes more comprehensive.
7 Conclusion
Kidney function can be examined using a variety of methods and markers, each with pros and cons of its own. Numerous putative biomarkers for renal health have demonstrated promise in enhancing the clinical management of kidney disease and are implicated in the pathophysiological process of kidney injury. It has been demonstrated that these biomarkers can identify and detect kidney injury at an early stage, as well as forecast the course, severity, and long-term risk of death associated with the disease. With the study of the combination of markers and the discovery of new markers, the detection of kidney disease will be more rapid and accurate.
Author contributions
ZL: Data curation, Writing – original draft. WN: Data curation, Writing – original draft. YW: Data curation, Writing – original draft. BZ: Data curation, Writing – original draft. QZ: Data curation, Writing – original draft. TZ: Data curation, Writing – review & editing. QL: Supervision, Writing – review & editing. DD: Supervision, Writing – review & editing.
Funding
The author(s) declare that financial support was received for the research and/or publication of this article. This study was supported by the Key Disciplines Group Construction Project of Pudong Health Bureau of Shanghai (Grant No. PWZxq2022-08) and Shanghai Pudong New Area Health Commission joint research project (PW2023D-01).
Conflict of interest
YW was employed by Goldsite Diagnostics Inc.
The remaining authors declare that the research was conducted in the absence of any commercial or financial relationships that could be construed as a potential conflict of interest.
Generative AI statement
The authors declare that no Gen AI was used in the creation of this manuscript.
Publisher’s note
All claims expressed in this article are solely those of the authors and do not necessarily represent those of their affiliated organizations, or those of the publisher, the editors and the reviewers. Any product that may be evaluated in this article, or claim that may be made by its manufacturer, is not guaranteed or endorsed by the publisher.
References
1. Susantitaphong, P, Cruz, DN, Cerda, J, Abulfaraj, M, Alqahtani, F, Koulouridis, I, et al. Acute kidney injury advisory Group of the American Society of nephrology world incidence of AKI: a meta-analysis. Clin J Am Soc Nephrol. (2013) 8:1482–93. doi: 10.2215/CJN.00710113
2. Levey, AS. Defining AKD: the Spectrum of AKI, AKD, and CKD. Nephron. (2022) 146:302–5. doi: 10.1159/000516647
3. Yoon, SY, Kim, JS, Jeong, KH, and Kim, SK. Acute kidney injury: biomarker-guided diagnosis and management. Medicina (Kaunas). (2022) 58:340. doi: 10.3390/medicina58030340
4. Kellum, JA, and Lameire, NKDIGO AKI Guideline Work Group. Diagnosis, evaluation, and management of acute kidney injury: a KDIGO summary (part 1). Crit Care. (2013) 17:204. doi: 10.1186/cc11454
5. Lameire, NH, Levin, A, Kellum, JA, Cheung, M, Jadoul, M, Winkelmayer, WC, et al. Harmonizing acute and chronic kidney disease definition and classification: report of a kidney disease: improving global outcomes (KDIGO) consensus conference. Kidney Int. (2021) 100:516–26. doi: 10.1016/j.kint.2021.06.028
6. GBD Chronic Kidney Disease Collaboration. Global, regional, and national burden of chronic kidney disease, 1990–2017: a systematic analysis for the global burden of disease study 2017. Lancet. (2020) 395:709–33. doi: 10.1016/S0140-6736(20)30045-3
7. Wang, L, Xu, X, Zhang, M, Hu, C, Zhang, X, Li, C, et al. Prevalence of chronic kidney disease in China: results from the sixth China chronic disease and risk factor surveillance. JAMA Intern Med. (2023) 183:298–310. doi: 10.1001/jamainternmed.2022.6817
8. Koyner, JL, Garg, AX, Coca, SG, Sint, K, Thiessen-Philbrook, H, Patel, UD, et al. Biomarkers predict progression of acute kidney injury after cardiac surgery. J Am Soc Nephrol. (2012) 23:905–14. doi: 10.1681/ASN.2011090907
9. Nickolas, TL, Barasch, J, and Devarajan, P. Biomarkers in acute and chronic kidney disease. Curr Opin Nephrol Hypertens. (2008) 17:127–32. doi: 10.1097/MNH.0b013e3282f4e525
10. Wu, I, and Parikh, CR. Screening for kidney diseases: older measures versus novel biomarkers. Clin J Am Soc Nephrol. (2008) 3:1895–901. doi: 10.2215/CJN.02030408
11. Shatat, IF, Qanungo, S, Hudson, S, Laken, MA, and Hailpern, SM. Changes in urine microalbumin-to-creatinine ratio in children with sickle cell disease over time. Front Pediatr. (2016) 4, 106. doi: 10.3389/fped.2016.00106
12. Nakamura, T, Ito, M, Yoshimura, T, Mabe, K, and Okamura, H. Usefulness of the urinary microalbumin/creatinine ratio in predicting pregnancy-induced hypertension. Int J Gynaecol Obstet. (1992) 37:99–103. doi: 10.1016/0020-7292(92)90489-6
13. Benoit, SW, Ciccia, EA, and Devarajan, P. Cystatin C as a biomarker of chronic kidney disease: latest developments. Expert Rev Mol Diagn. (2020) 20:1019–26. doi: 10.1080/14737159.2020.1768849
14. Eckfeldt, JH, Karger, AB, Miller, WG, Rynders, GP, and Inker, LA. Performance in measurement of serum cystatin C by laboratories participating in the College of American Pathologists 2014 CYS survey. Arch Pathol Lab Med. (2015) 139:888–93. doi: 10.5858/arpa.2014-0427-CP
15. Karger, AB, Long, T, Inker, LA, and Eckfeldt, JH. College of American Pathologists Accuracy Based Committee and Chemistry Resource Committee. Improved performance in measurement of serum cystatin C by laboratories participating in the College of American Pathologists 2019 CYS survey. Arch Pathol Lab Med. (2022) 146:1218–23. doi: 10.5858/arpa.2021-0306-CP
16. Khorashadi, M, Beunders, R, Pickkers, P, and Legrand, M. Proenkephalin: a new biomarker for glomerular filtration rate and acute kidney injury. Nephron. (2020) 144:655–61. doi: 10.1159/000509352
17. Ng, LL, Squire, IB, Jones, DJL, Cao, TH, Chan, DCS, Sandhu, JK, et al. Proenkephalin, renal dysfunction, and prognosis in patients with acute heart failure: a GREAT network study. J Am Coll Cardiol. (2017) 69:56–69. doi: 10.1016/j.jacc.2016.10.038
18. KDOQI. KDOQI clinical practice guidelines and clinical practice recommendations for diabetes and chronic kidney disease. Am J Kidney Dis. (2007) 49:S12–S154. doi: 10.1053/j.ajkd.2006.12.005
19. Bakris, GL, Williams, M, Dworkin, L, Elliott, WJ, Epstein, M, Toto, R, et al. Preserving renal function in adults with hypertension and diabetes: a consensus approach. National Kidney Foundation hypertension and diabetes executive committees working group. Am J Kidney Dis. (2000) 36:646–61. doi: 10.1053/ajkd.2000.16225
20. Rasaratnam, N, Salim, A, Blackberry, I, Cooper, ME, Magliano, DJ, Van Wijngaarden, P, et al. Urine albumin-creatinine ratio variability in people with type 2 diabetes: clinical and research implications. Am J Kidney Dis. (2024) 84:8–17.e1. doi: 10.1053/j.ajkd.2023.12.018
21. Xie, J, Wang, X, Zhang, Y, Li, H, Xu, Y, and Zheng, D. The longitudinal effect of subclinical hypothyroidism on urine microalbumin-to-urine creatinine ratio in patients with type 2 diabetes mellitus. BMC Endocr Disord. (2019) 19:84. doi: 10.1186/s12902-019-0405-0
22. Ahmed, SA, Aziz, WM, Shaker, SE, Fayed, DB, and Shawky, H. Urinary transferrin and proinflammatory markers predict the earliest diabetic nephropathy onset. Biomarkers. (2022) 27:178–87. doi: 10.1080/1354750X.2021.2023639
23. Li, B, Wang, J, and Ye, W. A meta-analysis of urinary transferrin for early diagnosis of diabetic nephropathy. Lab Med. (2024) 55:413–9. doi: 10.1093/labmed/lmad115
24. Kanauchi, M, Nishioka, H, Hashimoto, T, and Dohi, K. Diagnostic significance of urinary transferrin in diabetic nephropathy. Nihon Jinzo Gakkai Shi. (1995) 37:649–54.
25. Bazzi, C, Petrini, C, Rizza, V, Arrigo, G, Beltrame, A, Pisano, L, et al. Urinary excretion of IgG and alpha(1)-microglobulin predicts clinical course better than extent of proteinuria in membranous nephropathy. Am J Kidney Dis. (2001) 38:240–8. doi: 10.1053/ajkd.2001.26080
26. Xu, X, Huang, X, Chen, Y, Li, J, Shen, M, Hou, Y, et al. The role of urine IgG in the progression of IgA nephropathy with a high proportion of global glomerulosclerosis. Int Urol Nephrol. (2022) 54:323–30. doi: 10.1007/s11255-021-02858-y
27. Garcia-Ferrer, I, Marrero, A, Gomis-Rüth, FX, and Goulas, T. α2-macroglobulins: structure and function. Subcell Biochem. (2017) 83:149–83. doi: 10.1007/978-3-319-46503-6_6
28. Li, JJ, Sa, RL, Zhang, Y, and Yan, ZL. Evaluating new biomarkers for diabetic nephropathy: role of α2-macroglobulin, podocalyxin, α-L-fucosidase, retinol-binding protein-4, and cystatin C. World J Diabetes. (2024) 15:1212–25. doi: 10.4239/wjd.v15.i6.1212
29. Trink, J, Li, R, Gao, B, Lu, C, and Krepinsky, JC. Modulators of Alpha-2 macroglobulin upregulation by high glucose in glomerular mesangial cells. Biomol Ther. (2024) 14:1444. doi: 10.3390/biom14111444
30. Johnson, AC, Gooley, T, Guillem, A, Keyser, J, Rasmussen, H, Singh, B, et al. Parenterial iron sucrose-induced renal preconditioning: differential ferritin heavy and light chain expression in plasma, urine, and internal organs. Am J Physiol Renal Physiol. (2019) 317:F1563–71. doi: 10.1152/ajprenal.00307.2019
31. Lipschitz, DA, Allegre, A, and Cook, JD. The clinical significance of ferritinuria. Blood. (1980) 55:260–4. doi: 10.1182/blood.V55.2.260.260
32. van Raaij, S, van Swelm, R, Bouman, K, Cliteur, M, van den Heuvel, MC, Pertijs, J, et al. Tubular iron deposition and iron handling proteins in human healthy kidney and chronic kidney disease. Sci Rep. (2018) 8:9353. doi: 10.1038/s41598-018-27107-8
33. Qi, L, Xu, J, Yang, C, Hou, X, and Yang, P. Urinary ferritin creatinine ratio, a potential biomarker for lupus nephritis activity. Clin Rheumatol. (2021) 40:143–9. doi: 10.1007/s10067-020-05214-0
34. García-Martínez, JD, Martinez-Subiela, S, Tvarijonaviciute, A, Caldin, M, and Ceron, JJ. Urinary ferritin and cystatin C concentrations at different stages of kidney disease in leishmaniotic dogs. Res Vet Sci. (2015) 99:204–7. doi: 10.1016/j.rvsc.2015.01.002
35. Penders, J, and Delanghe, JR. Alpha 1-microglobulin: clinical laboratory aspects and applications. Clin Chim Acta. (2004) 346:107–18. doi: 10.1016/j.cccn.2004.03.037
36. Drüeke, TB, and Massy, ZA. Beta2-microglobulin. Semin Dial. (2009) 22:378–80. doi: 10.1111/j.1525-139X.2009.00584.x
37. Zhou, Y, Zhang, Y, Chen, J, Wang, T, Li, H, Wu, F, et al. Diagnostic value of α1-MG and URBP in early diabetic renal impairment. Front Physiol. (2023) 14:1173982. doi: 10.3389/fphys.2023.1173982
38. Yu, XL, Sun, W, Liu, L, Hong, K, and Song, H. Urinary α 1-microglobulin and β 2-microglobulin as markers of early kidney injury in HIV-positive male patients on tenofovir-based antiretroviral therapy. PLoS One. (2024) 19:e0303442. doi: 10.1371/journal.pone.0303442
39. Cai, F, Zhang, L, Zhao, P, Qiu, F, Mukhtar, AM, Ma, Y, et al. Urinary RBP as an independent predictor of renal outcome in diabetic nephropathy. Dis Markers. (2022) 2022:1–13. doi: 10.1155/2022/9687868
40. Qin, Y, Zhang, S, Shen, X, Zhang, S, Wang, J, Zuo, M, et al. Evaluation of urinary biomarkers for prediction of diabetic kidney disease: a propensity score matching analysis. Ther Adv Endocrinol Metab. (2019) 10:2042018819891110. doi: 10.1177/2042018819891110
41. Han, WK, Bailly, V, Abichandani, R, Thadhani, R, and Bonventre, JV. Kidney injury Molecule-1 (KIM-1): a novel biomarker for human renal proximal tubule injury. Kidney Int. (2002) 62:237–44. doi: 10.1046/j.1523-1755.2002.00433.x
42. Chen, J, Tang, TT, Cao, JY, Li, ZL, Zhong, X, Wen, Y, et al. KIM-1 augments hypoxia-induced tubulointerstitial inflammation through uptake of small extracellular vesicles by tubular epithelial cells. Mol Ther. (2023) 31:1437–50. doi: 10.1016/j.ymthe.2022.08.013
43. Carter, JL, Parker, CT, Stevens, PE, Eaglestone, G, Knight, S, Farmer, CKT, et al. Biological variation of plasma and urinary markers of acute kidney injury in patients with chronic kidney disease. Clin Chem. (2016) 6:876–83. doi: 10.1373/clinchem.2015.250993
44. Bonventre, JV. Kidney injury molecule-1 (KIM-1): a urinary biomarker and much more. Nephrol Dial Transplant. (2009) 24:3265–8. doi: 10.1093/ndt/gfp010
45. Brilland, B, Boudhors, C, Wacrenier, S, Blanchard, S, Cayon, J, Blanchet, O, et al. Kidney injury molecule 1 (KIM-1): a potential biomarker of acute kidney injury and tubulointerstitial injury in patients with ANCA-glomerulonephritis. Clin Kidney J. (2023) 16:1521–33. doi: 10.1093/ckj/sfad071
46. Huang, Q, Fei, X, Zhan, H, Gong, J, Zhou, J, Zhang, Y, et al. Urinary N-acetyl-β-d-glucosaminidase-creatine ratio is a valuable predictor for advanced diabetic kidney disease. J Clin Lab Anal. (2023) 37:e24769. doi: 10.1002/jcla.24769
47. Kang, HK, Kim, DK, Lee, BH, Om, AS, Hong, JH, Koh, HC, et al. Urinary N-acetyl-beta-D-glucosaminidase and malondialdehyde as a markers of renal damage in burned patients. J Korean Med Sci. (2001) 16:598–602. doi: 10.3346/jkms.2001.16.5.598
48. Zhang, C, Liu, T, Wang, X, Yang, J, Qin, D, Liang, Y, et al. Urine biomarkers in type 2 diabetes mellitus with or without microvascular complications. Nutr Diabetes. (2024) 14:51. doi: 10.1038/s41387-024-00310-5
49. Mishra, J, Ma, Q, Prada, A, Mitsnefes, M, Zahedi, K, Yang, J, et al. Identification of neutrophil gelatinase-associated lipocalin as a novel early urinary biomarker for ischemic renal injury. J Am Soc Nephrol. (2003) 14:2534–43. doi: 10.1097/01.ASN.0000088027.54400.C6
50. Isshiki, R, Asada, T, Sato, D, Sumida, M, Hamasaki, Y, Inokuchi, R, et al. Association of Urinary Neutrophil Gelatinase-Associated Lipocalin with Long-Term Renal Outcomes in ICU survivors: a retrospective observational cohort study. Shock. (2016) 46:44–51. doi: 10.1097/SHK.0000000000000580
51. Cho, SY, and Hur, M. New issues with neutrophil gelatinase-associated Lipocalin in acute kidney injury. Ann Lab Med. (2023) 43:529–30. doi: 10.3343/alm.2023.43.6.529
52. Li, Z, Tie, H, Shi, R, Rossaint, J, and Zarbock, A. Urinary [TIMP-2]·[IGFBP7]-guided implementation of the KDIGO bundle to prevent acute kidney injury: a meta-analysis. Br J Anaesth. (2022) 128:e24–6. doi: 10.1016/j.bja.2021.10.015
53. Schanz, M, Kimmel, M, Alscher, MD, Amann, K, and Daniel, C. TIMP-2 and IGFBP7 in human kidney biopsies in renal disease. Clin Kidney J. (2023) 16:1434–46. doi: 10.1093/ckj/sfad010
54. Molinari, L, Del Rio-Pertuz, G, Smith, A, Landsittel, DP, Singbartl, K, Palevsky, PM, et al. Utility of biomarkers for Sepsis-associated acute kidney injury staging. JAMA Netw Open. (2022) 5:e2212709. doi: 10.1001/jamanetworkopen.2022.12709
55. Wang, W, Shen, Q, and Zhou, X. The predictive value of [TIMP-2]*[IGFBP7] in adverse outcomes for acute kidney injury: a systematic review and meta-analysis. Ren Fail. (2023) 45:2253933. doi: 10.1080/0886022X.2023.2253933
56. Kamijo-Ikemori, A, Sugaya, T, Matsui, K, Yokoyama, T, and Kimura, K. Roles of human liver type fatty acid binding protein in kidney disease clarified using hL-FABP chromosomal transgenic mice. Nephrology (Carlton). (2011) 16:539–44. doi: 10.1111/j.1440-1797.2011.01469.x
57. Thi, TND, Gia, BN, Thi, HLL, Thi, TNC, and Thanh, HP. Evaluation of urinary L-FABP as an early marker for diabetic nephropathy in type 2 diabetic patients. J Med Biochem. (2020) 39:224–30. doi: 10.2478/jomb-2019-0037
58. Juanola, A, Graupera, I, Elia, C, Piano, S, Solé, C, Carol, M, et al. Urinary L-FABP is a promising prognostic biomarker of ACLF and mortality in patients with decompensated cirrhosis. J Hepatol. (2022) 76:107–14. doi: 10.1016/j.jhep.2021.08.031
59. Kamijo-Ikemori, A, Sugaya, T, Yasuda, T, Kawata, T, Ota, A, Tatsunami, S, et al. Clinical significance of urinary liver-type fatty acid-binding protein in diabetic nephropathy of type 2 diabetic patients. Diabetes Care. (2011) 34:691–6. doi: 10.2337/dc10-1392
60. Lin, Z, Wan, X, Zhang, T, Huo, H, Zhang, X, Li, K, et al. Trefoil factor 3: new highlights in chronic kidney disease research. Cell Signal. (2022) 100:110470. doi: 10.1016/j.cellsig.2022.110470
61. Rogulska, K, Wojciechowska-Koszko, I, Krasnodębska-Szponder, B, Kwiatkowski, P, Roszkowska, P, Dołęgowska, B, et al. TFF3 as a diagnostic biomarker in kidney transplant patients. Int J Mol Sci. (2023) 24:11925. doi: 10.3390/ijms241511925
62. Panich, T, Chancharoenthana, W, Somparn, P, Issara-Amphorn, J, Hirankarn, N, and Leelahavanichkul, A. Urinary exosomal activating transcriptional factor 3 as the early diagnostic biomarker for sepsis-induced acute kidney injury. BMC Nephrol. (2017) 18:10. doi: 10.1186/s12882-016-0415-3
63. Wu, X, Qiu, F, Jin, X, Zhou, J, and Zang, W. ATF3: a novel biomarker for the diagnosis of acute kidney injury after cardiac surgery. Ann Transl Med. (2021) 9:1655. doi: 10.21037/atm-21-5231
64. Zhou, H, Cheruvanky, A, Hu, X, Matsumoto, T, Hiramatsu, N, Cho, ME, et al. Urinary exosomal transcription factors, a new class of biomarkers for renal disease. Kidney Int. (2008) 74:613–21. doi: 10.1038/ki.2008.206
65. Ureche, C, Dodi, G, Șerban, AM, Covic, AS, Voroneanu, L, Hogaș, S, et al. Predictive value of collagen biomarkers in advanced chronic kidney disease patients. Biomol Ther. (2023) 13:389. doi: 10.3390/biom13020389
66. Ghoul, BE, Squalli, T, Servais, A, Elie, C, Meas-Yedid, V, Trivint, C, et al. Urinary procollagen III aminoterminal propeptide (PIIINP): a fibrotest for the nephrologist. Clin J Am Soc Nephrol. (2010) 5:205–10. doi: 10.2215/CJN.06610909
67. Teppo, AM, Törnroth, T, Honkanen, E, and Grönhagen-Riska, C. Urinary amino-terminal propeptide of type III procollagen (PIIINP) as a marker of interstitial fibrosis in renal transplant recipients. Transplantation. (2003) 75:2113–9. doi: 10.1097/01.TP.0000066809.60389.48
68. Fang, X, Hu, J, Chen, Y, Shen, W, and Ke, B. Dickkopf-3: current knowledge in kidney diseases. Front Physiol. (2020) 11:533344. doi: 10.3389/fphys.2020.533344
69. Liu, M, Shi, S, Senthilnathan, S, Yu, J, Wu, E, Bergmann, C, et al. Genetic variation of DKK3 may modify renal disease severity in ADPKD. J Am Soc Nephrol. (2010) 21:1510–20. doi: 10.1681/ASN.2010030237
70. Schunk, SJ, Floege, J, Fliser, D, and Speer, T. WNT-β-catenin signalling – a versatile player in kidney injury and repair. Nat Rev Nephrol. (2021) 17:172–84. doi: 10.1038/s41581-020-00343-w
71. Canki, E, Kho, E, and Hoenderop, JGJ. Urinary biomarkers in kidney disease. Clin Chim Acta. (2024) 555:117798. doi: 10.1016/j.cca.2024.117798
72. Speer, T, Schunk, SJ, Sarakpi, T, Schmit, D, Wagner, M, Arnold, L, et al. Urinary DKK3 as a biomarker for short-term kidney function decline in children with chronic kidney disease: an observational cohort study. Lancet Child Adolesc Health. (2023) 7:405–14. doi: 10.1016/S2352-4642(23)00049-4
73. Wen, Y, and Parikh, CR. Current concepts and advances in biomarkers of acute kidney injury. Crit Rev Clin Lab Sci. (2021) 58:354–68. doi: 10.1080/10408363.2021.1879000
74. Vasquez-Rios, G, Katz, R, Levitan, EB, Cushman, M, Parikh, CR, Kimmel, PL, et al. Urinary biomarkers of kidney tubule health and mortality in persons with CKD and diabetes mellitus. Kidney360. (2023) 4:e1257–64. doi: 10.34067/KID.0000000000000226
75. Devuyst, O, Olinger, E, and Rampoldi, L. Uromodulin: from physiology to rare and complex kidney disorders. Nat Rev Nephrol. (2017) 13:525–44. doi: 10.1038/nrneph.2017.101
76. Melchinger, H, Calderon-Gutierrez, F, Obeid, W, Xu, L, Shaw, MM, Luciano, RL, et al. Urine uromodulin as a biomarker of kidney Tubulointerstitial fibrosis. Clin J Am Soc Nephrol. (2022) 17:1284–92. doi: 10.2215/CJN.04360422
77. Thomas, JM, Huuskes, BM, Sobey, CG, Drummond, GR, and Vinh, A. The IL-18/IL-18R1 signalling axis: diagnostic and therapeutic potential in hypertension and chronic kidney disease. Pharmacol Ther. (2022) 239:108191. doi: 10.1016/j.pharmthera.2022.108191
78. Luan, J, Fu, J, Jiao, C, Hao, X, Feng, Z, Zhu, L, et al. IL-18 deficiency ameliorates the progression from AKI to CKD. Cell Death Dis. (2022) 13:957. doi: 10.1038/s41419-022-05394-4
79. Siew, ED, Ikizler, TA, Gebretsadik, T, Shintani, A, Wickersham, N, Bossert, F, et al. Elevated urinary IL-18 levels at the time of ICU admission predict adverse clinical outcomes. Clin J Am Soc Nephrol. (2010) 5:1497–505. doi: 10.2215/CJN.09061209
80. Hall, IE, Yarlagadda, SG, Coca, SG, Wang, Z, Doshi, M, Devarajan, P, et al. IL-18 and urinary NGAL predict dialysis and graft recovery after kidney transplantation. J Am Soc Nephrol. (2010) 21:189–97. doi: 10.1681/ASN.2009030264
81. Haller, H, Bertram, A, Nadrowitz, F, and Menne, J. Monocyte chemoattractant protein-1 and the kidney. Curr Opin Nephrol Hypertens. (2016) 25:42–9. doi: 10.1097/MNH.0000000000000186
82. Zhang, WR, and Parikh, CR. Biomarkers of acute and chronic kidney disease. Annu Rev Physiol. (2019) 81:309–33. doi: 10.1146/annurev-physiol-020518-114605
83. Lee, YH, and Song, GG. Urinary MCP-1 as a biomarker for lupus nephritis: a meta-analysis. Z Rheumatol. (2017) 76:357–63. doi: 10.1007/s00393-016-0109-z
84. Lee, CG, Da Silva, CA, Dela Cruz, CS, Ahangari, F, Ma, B, Kang, MJ, et al. Role of chitin and chitinase/chitinase-like proteins in inflammation, tissue remodeling, and injury. Annu Rev Physiol. (2011) 73:479–501. doi: 10.1146/annurev-physiol-012110-142250
85. Puthumana, J, Thiessen-Philbrook, H, Xu, L, Coca, SG, Garg, AX, Himmelfarb, J, et al. Biomarkers of inflammation and repair in kidney disease progression. J Clin Invest. (2021) 131:139927. doi: 10.1172/JCI139927
86. Hall, IE, Stern, EP, Cantley, LG, Elias, JA, and Parikh, CR. Urine YKL-40 is associated with progressive acute kidney injury or death in hospitalized patients. BMC Nephrol. (2014) 15:133. doi: 10.1186/1471-2369-15-133
87. Carnazzo, V, Redi, S, Basile, V, Natali, P, Gulli, F, Equitani, F, et al. Calprotectin: two sides of the same coin. Rheumatology. (2024) 63:26–33. doi: 10.1093/rheumatology/kead405
88. Heller, F, Frischmann, S, Grünbaum, M, Zidek, W, and Westhoff, TH. Urinary calprotectin and the distinction between prerenal and intrinsic acute kidney injury. Clin J Am Soc Nephrol. (2011) 6:2347–55. doi: 10.2215/CJN.02490311
89. Chang, CH, Yang, CH, Yang, HY, Chen, TH, Lin, CY, Chang, SW, et al. Urinary biomarkers improve the diagnosis of intrinsic acute kidney injury in coronary care units. Medicine (Baltimore). (2015) 94:e1703. doi: 10.1097/MD.0000000000001703
90. Amaya-Garrido, A, Brunet, M, Buffin-Meyer, B, Piedrafita, A, Grzesiak, L, Agbegbo, E, et al. Calprotectin is a contributor to and potential therapeutic target for vascular calcification in chronic kidney disease. Sci Transl Med. (2023) 15:eabn5939. doi: 10.1126/scitranslmed.abn5939
91. Bourgonje, AR, Bourgonje, MF, La Bastide-van, GS, Nilsen, T, Hidden, C, Gansevoort, RT, et al. A prospective study of the association between plasma calprotectin levels and new-onset CKD in the general population. Kidney Int Rep. (2024) 9:1265–75. doi: 10.1016/j.ekir.2024.02.1392
92. Yu, J, Xie, D, Huang, N, and Zhou, Q. Circular RNAs as novel diagnostic biomarkers and therapeutic targets in kidney disease. Front Med. (2021) 8:714958. doi: 10.3389/fmed.2021.714958
93. van Zonneveld, AJ, Kölling, M, Bijkerk, R, and Lorenzen, JM. Circular RNAs in kidney disease and cancer. Nat Rev Nephrol. (2021) 17:814–26. doi: 10.1038/s41581-021-00465-9
94. Vivarelli, M, Barratt, J, Beck, LH, Fakhouri, F, Gale, DP, Goicoechea De Jorge, E, et al. The role of complement in kidney disease: conclusions from a kidney disease: improving global outcomes (KDIGO) controversies conference. Kidney Int. (2024) 106:369–91. doi: 10.1016/j.kint.2024.05.015
95. Ayasreh, N, Bullich, G, Miquel, R, Furlano, M, Ruiz, P, Lorente, L, et al. Autosomal dominant Tubulointerstitial kidney disease: clinical presentation of patients with ADTKD-UMOD and ADTKD-MUC1. Am J Kidney Dis. (2018) 72:411–8. doi: 10.1053/j.ajkd.2018.03.019
96. Wuttke, M, Li, Y, Li, M, Sieber, KB, Feitosa, MF, Gorski, M, et al. A catalog of genetic loci associated with kidney function from analyses of a million individuals. Nat Genet. (2019) 51:957–72. doi: 10.1038/s41588-019-0407-x
97. Hashimoto, K. Emerging role of the host microbiome in neuropsychiatric disorders: overview and future directions. Mol Psychiatry. (2023) 28:3625–37. doi: 10.1038/s41380-023-02287-6
98. de Vos, WM, Tilg, H, Van Hul, M, and Cani, PD. Gut microbiome and health: mechanistic insights. Gut. (2022) 71:1020–32. doi: 10.1136/gutjnl-2021-326789
99. Mei, Z, Wang, F, Bhosle, A, Dong, D, Mehta, R, Ghazi, A, et al. Strain-specific gut microbial signatures in type 2 diabetes identified in a cross-cohort analysis of 8,117 metagenomes. Nat Med. (2024) 30:2265–76. doi: 10.1038/s41591-024-03067-7
100. Linh, HT, Oshima, M, Sako, K, Konishi, M, Hayashi, D, Sanada, H, et al. Identification of circulating microbial DNA and its association with kidney function in patients with diabetic kidney disease. Nephrology. (2024) 29:909–16. doi: 10.1111/nep.14408
101. Miao, H, Wang, Y, Yu, X, Zou, L, Guo, Y, Su, W, et al. Lactobacillus species ameliorate membranous nephropathy through inhibiting the aryl hydrocarbon receptor pathway via tryptophan-produced indole metabolites. Br J Pharmacol. (2024) 181:162–79. doi: 10.1111/bph.16219
102. Zeng, Y, Guo, M, Wu, Q, Tan, X, Jiang, C, Teng, F, et al. Gut microbiota-derived indole-3-propionic acid alleviates diabetic kidney disease through its mitochondrial protective effect via reducing ubiquitination mediated-degradation of SIRT1. J Adv Res. (2024) S2090-1232:00361–8. doi: 10.1016/j.jare.2024.08.018
103. Md Dom, ZI, Satake, E, Skupien, J, Krolewski, B, O’Neil, K, Willency, JA, et al. Circulating proteins protect against renal decline and progression to end-stage renal disease in patients with diabetes. Sci Transl Med. (2021) 13:eabd2699. doi: 10.1126/scitranslmed.abd2699
104. Niewczas, MA, Pavkov, ME, Skupien, J, Smiles, A, Md Dom, ZI, Wilson, JM, et al. A signature of circulating inflammatory proteins and development of end-stage renal disease in diabetes. Nat Med. (2019) 25:805–13. doi: 10.1038/s41591-019-0415-5
105. Catanese, L, Siwy, J, Mavrogeorgis, E, Amann, K, Mischak, H, Beige, J, et al. A novel urinary proteomics classifier for non-invasive evaluation of interstitial fibrosis and tubular atrophy in chronic kidney disease. Proteomes. (2021) 9:32. doi: 10.3390/proteomes9030032
106. Luo, Y, Zhang, W, and Qin, G. Metabolomics in diabetic nephropathy: unveiling novel biomarkers for diagnosis (review). Mol Med Rep. (2024) 30:156. doi: 10.3892/mmr.2024.13280
107. Wang, YN, Zhang, ZH, Liu, HJ, Guo, ZY, Zou, L, Zhang, YM, et al. Integrative phosphatidylcholine metabolism through phospholipase A2 in rats with chronic kidney disease. Acta Pharmacol Sin. (2023) 44:393–405. doi: 10.1038/s41401-022-00947-x
108. Lin, W, Mousavi, F, Blum, BC, Heckendorf, CF, Moore, J, Lampl, N, et al. Integrated metabolomics and proteomics reveal biomarkers associated with hemodialysis in end-stage kidney disease. Front Pharmacol. (2023) 14:1243505. doi: 10.3389/fphar.2023.1243505
109. Si, S, Liu, H, Xu, L, and Zhan, S. Identification of novel therapeutic targets for chronic kidney disease and kidney function by integrating multi-omics proteome with transcriptome. Genome Med. (2024) 16:84. doi: 10.1186/s13073-024-01356-x
110. McCullough, PA, Shaw, AD, Haase, M, Bouchard, J, Waikar, SS, Siew, ED, et al. Diagnosis of acute kidney injury using functional and injury biomarkers: workgroup statements from the tenth acute Dialysis quality initiative consensus conference. Contrib Nephrol. (2013) 182:13–29. doi: 10.1159/000349963
111. Padhy, M, Kaushik, S, Girish, MP, Mohapatra, S, Shah, S, and Koner, BC. Serum neutrophil gelatinase associated lipocalin (NGAL) and cystatin C as early predictors of contrast-induced acute kidney injury in patients undergoing percutaneous coronary intervention. Clin Chim Acta. (2014) 435:48–52. doi: 10.1016/j.cca.2014.04.016
112. Mishra, J, Dent, C, Tarabishi, R, Mitsnefes, MM, Ma, Q, Kelly, C, et al. Neutrophil gelatinase-associated lipocalin (NGAL) as a biomarker for acute renal injury after cardiac surgery. Lancet. (2005) 365:1231–8. doi: 10.1016/S0140-6736(05)74811-X
113. Stehlé, T, and Delanaye, P. Which is the best glomerular filtration marker: creatinine, cystatin C or both? Eur J Clin Investig. (2024) 54:e14278. doi: 10.1111/eci.14278
114. Smith, ER, Lee, D, Cai, MM, Tomlinson, LA, Ford, ML, McMahon, LP, et al. Urinary neutrophil gelatinase-associated lipocalin may aid prediction of renal decline in patients with non-proteinuric stages 3 and 4 chronic kidney disease (CKD). Nephrol Dial Transplant. (2013) 28:1569–79. doi: 10.1093/ndt/gfs586
115. Newman, DJ, Pugia, MJ, Lott, JA, Wallace, JF, and Hiar, AM. Urinary protein and albumin excretion corrected by creatinine and specific gravity. Clin Chim Acta. (2000) 294:139–55. doi: 10.1016/S0009-8981(00)00181-9
116. Basu, RK, Wong, HR, Krawczeski, CD, Wheeler, DS, Manning, PB, Chawla, LS, et al. Combining functional and tubular damage biomarkers improves diagnostic precision for acute kidney injury after cardiac surgery. J Am Coll Cardiol. (2014) 64:2753–62. doi: 10.1016/j.jacc.2014.09.066
117. Murray, PT, Mehta, RL, Shaw, A, Ronco, C, Endre, Z, Kellum, JA, et al. Potential use of biomarkers in acute kidney injury: report and summary of recommendations from the 10th acute Dialysis quality initiative consensus conference. Kidney Int. (2014) 85:513–21. doi: 10.1038/ki.2013.374
118. Bienaimé, F, Muorah, M, Metzger, M, Broeuilh, M, Houiller, P, Flamant, M, et al. Combining robust urine biomarkers to assess chronic kidney disease progression. EBioMedicine. (2023) 93:104635. doi: 10.1016/j.ebiom.2023.104635
119. Bufkin, KB, Karim, ZA, and Silva, J. Review of the limitations of current biomarkers in acute kidney injury clinical practices. SAGE Open Med. (2024) 12:20503121241228446. doi: 10.1177/20503121241228446
120. Pejchinovski, I, Turkkan, S, and Pejchinovski, M. Recent advances of proteomics in Management of Acute Kidney Injury. Diagnostics (Basel). (2023) 13:2648. doi: 10.3390/diagnostics13162648
121. Luciano, RL, and Moeckel, GW. Update on the native kidney biopsy: Core curriculum 2019. Am J Kidney Dis. (2019) 73:404–15. doi: 10.1053/j.ajkd.2018.10.011
122. Bonani, M, Seeger, H, Weber, N, Lorenzen, JM, Wüthrich, RP, and Kistler, AD. Safety of kidney biopsy when performed as an outpatient procedure. Kidney Blood Press Res. (2021) 46:310–22. doi: 10.1159/000515439
123. Cavanaugh, C, and Perazella, MA. Urine sediment examination in the diagnosis and Management of Kidney Disease: Core curriculum 2019. Am J Kidney Dis. (2019) 73:258–72. doi: 10.1053/j.ajkd.2018.07.012
124. Magiorkinis, E, and Diamantis, A. The fascinating story of urine examination: from uroscopy to the era of microscopy and beyond. Diagn Cytopathol. (2015) 43:1020–36. doi: 10.1002/dc.23370
125. Kavuru, V, Vu, T, Karageorge, L, Choudhury, D, Senger, R, and Robertson, J. Dipstick analysis of urine chemistry: benefits and limitations of dry chemistry-based assays. Postgrad Med. (2020) 132:225–33. doi: 10.1080/00325481.2019.1679540
126. Kutter, D. Dry chemistry urinalysis of pathological proteinuria. Clin Chem Lab Med. (1998) 36:929–33. doi: 10.1515/CCLM.1998.161
127. Beier, K, Eppanapally, S, Bazick, HS, Chang, D, Mahadevappa, K, Gibbons, FK, et al. Elevation of blood urea nitrogen is predictive of long-term mortality in critically ill patients independent of “normal” creatinine. Crit Care Med. (2011) 39:305–13. doi: 10.1097/CCM.0b013e3181ffe22a
128. Giordano, C, Karasik, O, King-Morris, K, and Asmar, A. Uric acid as a marker of kidney disease: review of the current literature. Dis Markers. (2015) 2015:382918:1–6. doi: 10.1155/2015/382918
129. Feig, DI. Uric acid: a novel mediator and marker of risk in chronic kidney disease? Curr Opin Nephrol Hypertens. (2009) 18:526–30. doi: 10.1097/MNH.0b013e328330d9d0
130. Min, B, Yun, SR, Yoon, SH, Kim, JD, Hwang, WJ, Hwang, WM, et al. Comparison of the association intensity of creatinine and cystatin C with hyperphosphatemia and hyperparathyroidism in patients with chronic kidney disease. Sci Rep. (2023) 13:3855. doi: 10.1038/s41598-023-31048-2
131. Lassus, J, and Harjola, VP. Cystatin C: a step forward in assessing kidney function and cardiovascular risk. Heart Fail Rev. (2012) 17:251–61. doi: 10.1007/s10741-011-9242-6
132. Togashi, Y, Sakaguchi, Y, Miyamoto, M, and Miyamoto, Y. Urinary cystatin C as a biomarker for acute kidney injury and its immunohistochemical localization in kidney in the CDDP-treated rats. Exp Toxicol Pathol. (2012) 64:797–805. doi: 10.1016/j.etp.2011.01.018
133. Martin, L, Martin, C, Peine, A, Imöhl, M, Kersten, A, Kramann, R, et al. Implementation and one-year evaluation of Proenkephalin a in critical care. Int J Mol Sci. (2025) 26:2602. doi: 10.3390/ijms26062602
134. McQuarrie, EP, Shakerdi, L, Jardine, AG, Fox, JG, and Mackinnon, B. Fractional excretions of albumin and IgG are the best predictors of progression in primary glomerulonephritis. Nephrol Dial Transplant. (2011) 26:1563–9. doi: 10.1093/ndt/gfq605
135. Narita, T, Hosoba, M, Kakei, M, and Ito, S. Increased urinary excretions of immunoglobulin g, ceruloplasmin, and transferrin predict development of microalbuminuria in patients with type 2 diabetes. Diabetes Care. (2006) 29:142–4. doi: 10.2337/diacare.29.01.06.dc05-1063
136. Cohen-Bucay, A, and Viswanathan, G. Urinary markers of glomerular injury in diabetic nephropathy. Int J Nephrol. (2012) 2012:146987:1–11. doi: 10.1155/2012/146987
137. Trink, J, Li, R, Palarasah, Y, Troyanov, S, Andersen, TE, Sidelmann, JJ, et al. Activated alpha 2-macroglobulin is a novel mediator of mesangial cell Profibrotic signaling in diabetic kidney disease. Biomedicines. (2021) 9:1112. doi: 10.3390/biomedicines9091112
138. McCullough, K, and Bolisetty, S. Ferritins in kidney disease. Semin Nephrol. (2020) 40:160–72. doi: 10.1016/j.semnephrol.2020.01.007
139. Wan, X, Zhang, L, Gu, H, Wang, S, and Liu, X. The Association of Serum hsCRP and urinary Alpha1-microglobulin in patients with type 2 diabetes mellitus. Biomed Res Int. (2019) 2019:1–10. doi: 10.1155/2019/6364390
140. Rebholz, CM, Grams, ME, Matsushita, K, Selvin, E, and Coresh, J. Change in novel filtration markers and risk of ESRD. Am J Kidney Dis. (2015) 66:47–54. doi: 10.1053/j.ajkd.2014.11.009
141. Zhou, XH, Liu, SY, Yang, B, Li, YF, and Hou, BH. Urinary orosomucoid and retinol binding protein levels as early diagnostic markers for diabetic kidney disease. BMC Nephrol. (2023) 24:357. doi: 10.1186/s12882-023-03363-3
142. Kim, HK, Lee, M, Lee, YH, Kang, ES, Cha, BS, and Lee, BW. Renal tubular damage marker, urinary N-acetyl-β-D-Glucosaminidase, as a predictive marker of hepatic fibrosis in type 2 diabetes mellitus. Diabetes Metab J. (2022) 46:104–16. doi: 10.4093/dmj.2020.0273
143. Obermüller, N, Geiger, H, Weipert, C, and Urbschat, A. Current developments in early diagnosis of acute kidney injury. Int Urol Nephrol. (2014) 46:1–7. doi: 10.1007/s11255-013-0448-5
144. Rico-Fontalvo, J, Aroca-Martínez, G, Daza-Arnedo, R, Cabrales, J, Rodríguez-Yanez, T, Cardona-Blanco, M, et al. Novel biomarkers of diabetic kidney disease. Biomol Ther. (2023) 13:633. doi: 10.3390/biom13040633
145. Moresco, RN, Bochi, GV, Stein, CS, De Carvalho, JAM, Cembranel, BM, and Bollick, YS. Urinary kidney injury molecule-1 in renal disease. Clin Chim Acta. (2018) 487:15–21. doi: 10.1016/j.cca.2018.09.011
146. Colhoun, HM, and Marcovecchio, ML. Biomarkers of diabetic kidney disease. Diabetologia. (2018) 61:996–1011. doi: 10.1007/s00125-018-4567-5
147. Seibert, FS, Sitz, M, Passfall, J, Haesner, M, Laschinski, P, Buhl, M, et al. Prognostic value of urinary calprotectin, NGAL and KIM-1 in chronic kidney disease. Kidney Blood Press Res. (2018) 43:1255–62. doi: 10.1159/000492407
148. Rossiter, A, La, A, Koyner, JL, and Forni, LG. New biomarkers in acute kidney injury. Crit Rev Clin Lab Sci. (2024) 61:23–44. doi: 10.1080/10408363.2023.2242481
149. Pan, HC, Yang, SY, Chiou, TTY, Shiao, CC, Wu, CH, Huang, CT, et al. Comparative accuracy of biomarkers for the prediction of hospital-acquired acute kidney injury: a systematic review and meta-analysis. Crit Care. (2022) 26:349. doi: 10.1186/s13054-022-04223-6
150. Delrue, C, and Speeckaert, MM. Tissue inhibitor of Metalloproteinases-2 (TIMP-2) as a prognostic biomarker in acute kidney injury: a narrative review. Diagnostics. (2024) 14:1350. doi: 10.3390/diagnostics14131350
151. Vandenberghe, W, De Loor, J, Francois, K, Vandekerckhove, K, Herck, I, Vande Walle, J, et al. Potential of urine biomarkers CHI3L1, NGAL, TIMP-2, IGFBP7, and combinations as complementary diagnostic tools for acute kidney injury after pediatric cardiac surgery: a prospective cohort study. Diagnostics (Basel). (2023) 13:1047. doi: 10.3390/diagnostics13061047
152. Guzzi, LM, Bergler, T, Binnall, B, Engelman, DT, Forni, L, Germain, MJ, et al. Clinical use of [TIMP-2]•[IGFBP7] biomarker testing to assess risk of acute kidney injury in critical care: guidance from an expert panel. Crit Care. (2019) 23:225. doi: 10.1186/s13054-019-2504-8
153. Xu, Y, Xie, Y, Shao, X, Ni, Z, and Mou, S. L-FABP: a novel biomarker of kidney disease. Clin Chim Acta. (2015) 445:85–90. doi: 10.1016/j.cca.2015.03.017
154. Ku, CY, Liu, YH, Lin, HY, Lu, SC, and Lin, JY. Liver fatty acid-binding protein (L-FABP) promotes cellular angiogenesis and migration in hepatocellular carcinoma. Oncotarget. (2016) 7:18229–46. doi: 10.18632/oncotarget.7571
155. Panduru, NM, Forsblom, C, Saraheimo, M, Thorn, L, Bierhaus, A, Humpert, PM, et al. Urinary liver-type fatty acid-binding protein and progression of diabetic nephropathy in type 1 diabetes. Diabetes Care. (2013) 36:2077–83. doi: 10.2337/dc12-1868
156. Kamianowska, M, Rybi-Szumińska, A, Kamianowska, A, Maciejczyk, M, Sołomianko, K, Koput, A, et al. The urinary concentration of trefoil factor 3 (TFF3) in the term and preterm neonates. J Clin Med. (2023) 12:4936. doi: 10.3390/jcm12154936
157. Dobre, MA, Ahlawat, S, and Schelling, JR. Chronic kidney disease associated cardiomyopathy: recent advances and future perspectives. Curr Opin Nephrol Hypertens. (2024) 33:203–11. doi: 10.1097/MNH.0000000000000952
158. Taranta-Janusz, K, Moczulska, A, Nosek, H, Michaluk-Skutnik, J, Klukowski, M, and Wasilewska, A. Urinary procollagen III aminoterminal propeptide and β-catenin – new diagnostic biomarkers in solitary functioning kidney? Adv Med Sci. (2019) 64:189–94. doi: 10.1016/j.advms.2018.10.002
159. Lieb, W, Song, RJ, Xanthakis, V, and Vasan, RS. Association of Circulating Tissue Inhibitor of Metalloproteinases-1 and procollagen type III Aminoterminal peptide levels with incident heart failure and chronic kidney disease. J Am Heart Assoc. (2019) 8:e011426. doi: 10.1161/JAHA.118.011426
160. Park, M, Katz, R, Shlipak, MG, Weiner, D, Tracy, R, Jotwani, V, et al. Urinary markers of fibrosis and risk of cardiovascular events and death in kidney transplant recipients: the FAVORIT trial. Am J Transplant. (2017) 17:2640–9. doi: 10.1111/ajt.14284
161. Caffo, M, Casili, G, Caruso, G, Barresi, V, Campolo, M, Paterniti, I, et al. DKK3 expression in glioblastoma: correlations with biomolecular markers. Int J Mol Sci. (2024) 25:4091. doi: 10.3390/ijms25074091
162. Arjune, S, Späth, MR, Oehm, S, Todorova, P, Schunk, SJ, Lettenmeier, K, et al. DKK3 as a potential novel biomarker in patients with autosomal polycystic kidney disease. Clin Kidney J. (2024) 17:sfad262. doi: 10.1093/ckj/sfad262
163. Carbayo, J, Verdalles, Ú, Díaz-Crespo, F, Lázaro, A, González-Nicolás, M, Arroyo, D, et al. Tubular biomarkers in proteinuric kidney disease: histology correlation and kidney prognosis of tubular biomarkers. Clin Kidney J. (2024) 17:sfae146. doi: 10.1093/ckj/sfae146
164. Olinger, E, Schaeffer, C, Kidd, K, Elhassan, EAE, Cheng, Y, Dufour, I, et al. An intermediate-effect size variant in UMOD confers risk for chronic kidney disease. Proc Natl Acad Sci USA. (2022) 119:e2114734119. doi: 10.1073/pnas.2114734119
165. Nanamatsu, A, De Araújo, L, LaFavers, KA, and El-Achkar, TM. Advances in uromodulin biology and potential clinical applications. Nat Rev Nephrol. (2024) 20:806–21. doi: 10.1038/s41581-024-00881-7
166. Hirooka, Y, and Nozaki, Y. Interleukin-18 in inflammatory kidney disease. Front Med. (2021) 8:639103. doi: 10.3389/fmed.2021.639103
167. Mosa, O, Skitek, M, and Jerin, A. Validity of klotho, CYR61 and YKL-40 as ideal predictive biomarkers for acute kidney injury: review study. Sao Paulo Med J. (2017) 135:57–65. doi: 10.1590/1516-3180.2016.0099220516
168. Seibert, FS, Sitz, M, Passfall, J, Haesner, M, Laschinski, P, Buhl, M, et al. Urinary calprotectin, NGAL, and KIM-1 in the differentiation of primarily inflammatory vs. non-inflammatory stable chronic kidney diseases. Ren Fail. (2021) 43:417–24. doi: 10.1080/0886022X.2021.1885442
169. Jin, J, Sun, H, Shi, C, Yang, H, Wu, Y, Li, W, et al. Circular RNA in renal diseases. J Cell Mol Med. (2020) 24:6523–33. doi: 10.1111/jcmm.15295
170. Petr, V, and Thurman, JM. The role of complement in kidney disease. Nat Rev Nephrol. (2023) 19:771–87. doi: 10.1038/s41581-023-00766-1
171. Lee, AK, Katz, R, Jotwani, V, Garimella, PS, Ambrosius, WT, Cheung, AK, et al. Distinct dimensions of kidney health and risk of cardiovascular disease, heart failure, and mortality. Hypertension. (1979) 74:872–9. doi: 10.1161/HYPERTENSIONAHA.119.13339
172. Fernando, BNTW, Alli-Shaik, A, Hemage, RKD, Badurdeen, Z, Hettiarachchi, TW, Abeysundara, HTK, et al. Pilot study of renal urinary biomarkers for diagnosis of CKD of uncertain etiology. Kidney Int Rep. (2019) 4:1401–11. doi: 10.1016/j.ekir.2019.07.009
173. Bazzi, C, Rizza, V, Raimondi, S, Casellato, D, Napodano, P, and D’Amico, G. In crescentic IgA nephropathy, fractional excretion of IgG in combination with nephron loss is the best predictor of progression and responsiveness to immunosuppression. Clin J Am Soc Nephrol. (2009) 4:929–35. doi: 10.2215/CJN.05711108
174. Bazzi, C, Rizza, V, Casellato, D, Stivali, G, Rachele, G, Napodano, P, et al. Urinary IgG and α2-macroglobulin are powerful predictors of outcome and responsiveness to steroids and cyclophosphamide in idiopathic focal segmental glomerulosclerosis with nephrotic syndrome. Biomed Res Int. (2013) 2013:941831:1–8. doi: 10.1155/2013/941831
175. Zhang, D, Ye, S, and Pan, T. The role of serum and urinary biomarkers in the diagnosis of early diabetic nephropathy in patients with type 2 diabetes. PeerJ. (2019) 7:e7079. doi: 10.7717/peerj.7079
176. Musiał, K, Stojanowski, J, Miśkiewicz-Bujna, J, Kałwak, K, and Ussowicz, M. KIM-1, IL-18, and NGAL, in the machine learning prediction of kidney injury among children undergoing hematopoietic stem cell transplantation-a pilot study. Int J Mol Sci. (2023) 24:15791. doi: 10.3390/ijms242115791
177. Zhang, Y, Zhang, B, Wang, D, Shi, W, and Zheng, A. Evaluation of novel biomarkers for early diagnosis of acute kidney injury in asphyxiated full-term newborns: a case-control study. Med Princ Pract. (2020) 29:285–91. doi: 10.1159/000503555
178. Ma, J, Deng, Y, Lao, H, Ouyang, X, Liang, S, Wang, Y, et al. A nomogram incorporating functional and tubular damage biomarkers to predict the risk of acute kidney injury for septic patients. BMC Nephrol. (2021) 22:176. doi: 10.1186/s12882-021-02388-w
179. Lei, L, Li, LP, Zeng, Z, Mu, JX, Yang, X, Zhou, C, et al. Value of urinary KIM-1 and NGAL combined with serum Cys C for predicting acute kidney injury secondary to decompensated cirrhosis. Sci Rep. (2018) 8:7962. doi: 10.1038/s41598-018-26226-6
180. Katagiri, D, Doi, K, Honda, K, Negishi, K, Fujita, T, Hisagi, M, et al. Combination of two urinary biomarkers predicts acute kidney injury after adult cardiac surgery. Ann Thorac Surg. (2012) 93:577–83. doi: 10.1016/j.athoracsur.2011.10.048
Keywords: biomarker, kidney disease, chronic kidney disease, CKD, acute kidney disease, AKD, kidney injury, biomarker combination
Citation: Lu Z, Ni W, Wu Y, Zhai B, Zhao Q, Zheng T, Liu Q and Ding D (2025) Application of biomarkers in the diagnosis of kidney disease. Front. Med. 12:1560222. doi: 10.3389/fmed.2025.1560222
Edited by:
Ying-Yong Zhao, Northwest University, ChinaReviewed by:
Sahadeb Jana, Midnapore City College, IndiaFabrizio Cristiano, University of Studies G. d’Annunzio Chieti and Pescara, Italy
Rinini Dastidar, Nirnayan Health Care Private Limited, India
Copyright © 2025 Lu, Ni, Wu, Zhai, Zhao, Zheng, Liu and Ding. This is an open-access article distributed under the terms of the Creative Commons Attribution License (CC BY). The use, distribution or reproduction in other forums is permitted, provided the original author(s) and the copyright owner(s) are credited and that the original publication in this journal is cited, in accordance with accepted academic practice. No use, distribution or reproduction is permitted which does not comply with these terms.
*Correspondence: Qianqian Liu, cWlhbnFpYW5saXUyNUAxNjMuY29t; Dapeng Ding, MTgwOTg4NzcyNjlAMTYzLmNvbQ==
†These authors have contributed equally to this work and share first authorship