- 1Department of Biosciences, COMSATS University, Islamabad, Pakistan
- 2Water and Environment Research Group, Mazumdar-Shaw Advanced Research Centre, University of Glasgow, Glasgow, United Kingdom
- 3Moy Park, Armagh, United Kingdom
- 4Department of Infection Biology, Faculty of Infectious and Tropical Diseases, London School of Hygiene and Tropical Medicine, London, United Kingdom
- 5Department of Molecular and Clinical Cancer Medicine, University of Liverpool, Liverpool, United Kingdom
- 6College of Science and Engineering, University of Galway, Galway, Ireland
In recent years, there has been an unprecedented advancement in in situ analytical approaches that contribute to the mechanistic understanding of microbial communities by explicitly incorporating ecology and studying their assembly. In this study, we have analyzed the temporal profiles of the healthy broiler cecal microbiome from day 3 to day 35 to recover the stable and varying components of microbial communities. During this period, the broilers were fed three different diets chronologically, and therefore, we have recovered signature microbial species that dominate during each dietary regime. Since broilers were raised in multiple pens, we have also parameterized these as an environmental condition to explore microbial niches and their overlap. All of these analyses were performed in view of different parameters such as body weight (BW-mean), feed intake (FI), feed conversion ratio (FCR), and age (days) to link them to a subset of microbes that these parameters have a bearing upon. We found that gut microbial communities exhibited strong and statistically significant specificity for several environmental variables. Through regression models, genera that positively/negatively correlate with the bird’s age were identified. Some short-chain fatty acids (SCFAs)-producing bacteria, including Izemoplasmatales, Gastranaerophilales, and Roseburia, have a positive correlation with age. Certain pathogens, such as Escherichia-Shigella, Sporomusa, Campylobacter, and Enterococcus, negatively correlated with the bird’s age, which indicated a high disease risk in the initial days. Moreover, the majority of pathways involved in amino acid biosynthesis were also positively correlated with the bird’s age. Some probiotic genera associated with improved performance included Oscillospirales; UCG-010, Shuttleworthia, Bifidobacterium, and Butyricicoccaceae; UCG-009. In general, predicted antimicrobial resistance genes (piARGs) contributed at a stable level, but there was a slight increase in abundance when the diet was changed. To the best of the authors’ knowledge, this is one of the first studies looking at the stability, complexity, and ecology of natural broiler microbiota development in a temporal setting.
1. Introduction
The challenge of global food scarcity is paramount, and efforts have been directed at agro-based sectors toward high generative capacities with the minimum possible resource utilization. In this respect, the poultry industry, particularly the broiler farming sector, has been playing a pivotal role for decades, with annual growth of 5% (FAO, 2017). Broilers are an important food source, specifically due to their cheap and high-quality protein content (El-Deek et al., 2020). Bird feed conversion efficiency is actuated by gastrointestinal microbial species (Kogut, 2019; Sztandarski et al., 2022). Over 900 bacterial species have been explored so far in the chicken gut, comprising commensals and pathogens (Ranjitkar et al., 2016; Binek et al., 2017; Fathima et al., 2022).
The advantageous bacterial communities are involved in chicken feed digestion, nutrient assimilation and absorption, pathogen proscription, and immunity development, contributing to disease resistance (Stanley et al., 2012; Park et al., 2017; Borda-Molina et al., 2018). The commensal microbiota have an evident antagonistic effect on pathogenic bacteria by way of colonization conflict, immune intonation, and production of antimicrobial molecules such as organic acids, bacteriocin, and hydrogen peroxide (Yeoman et al., 2012; Kim et al., 2015). This phenomenon of competitive exclusion, first coined by Nurmi, is illustrated by the decreased relative load of Helicobacter and Campylobacter by increasing the relative load of certain other (probiotic) genera (Nurmi and Rantala, 1973; Kaakoush et al., 2014).
The chicken cecal microbial environment is dominated by a high abundance of unclassified bacteria and bacterial low abundance reads at both the genus and species levels at the early stages of development (Proszkowiec-Weglarz et al., 2022). These bacteria are mainly acquired through the passage of eggs via the mother hen’s reproductive tract (Lee et al., 2019). Newly hatched chicks are usually exposed to environmental and non-avian sources of bacteria; therefore, the gut colonization pattern is highly variable and characterized by low diversity and high instability that is governed by several environmental and host-associated factors (McKenna et al., 2020; Fathima et al., 2022; Proszkowiec-Weglarz et al., 2022). The highest microbial diversity has been observed in cecal content, holding up to 1011 organisms per gram (Huang et al., 2018). Chicken gut development in commercial production systems is relatively specific, as chicks never come into contact with adult birds (Polansky et al., 2016).
Recently, the diversity of gut bacterial communities in broiler birds was examined, and it was found that 90% of ASVs belonged to Firmicutes and Proteobacteria (Li et al., 2022). The microbial community’s complexity increased with age throughout the productive lifespan, with maximum stability during the 3rd week and instability during the 6th week (Yan et al., 2017; Huang et al., 2018; Feye et al., 2020). Previously, it has been reported that colonization and replacement of certain microbial communities with more stable taxa occurs as broiler age advances (Lu et al., 2003; Mohd Shaufi et al., 2015). In general, age is considered an important factor influencing the gut community structure, function, and diversity, with initial colonizers as facultative anaerobes followed by strict anaerobes (Zhu et al., 2002; Palmer et al., 2007; Oakley and Kogut, 2016).
In our previous study (Ijaz et al., 2018), an in-depth sampling regime was used to assess daily changes in microbiota. The study highlighted how alpha diversity increases rapidly during the initial 12 days, as well as how beta diversity converges to a stable solution. More importantly, phylogenetic alpha diversity measures such as the net relatedness index (NRI) and the nearest taxon index (NTI) showed a step response (days 12–20), hypothesizing a window of opportunity where a pathogen such as Campylobacter may appear. However, beyond diversity estimates, we did not explore the spatial (pens) and temporal dynamics and how the structure of microbial communities can reveal information about the stability of ecosystems. In the past few years, there has been a reinvigorated interest in microbial ecology, particularly in view of advancements in null modeling techniques. Therefore, to consolidate these new analytical approaches, we are revisiting them (Ijaz et al., 2018) to explore important concepts such as microbial niche differentiation (Verster and Borenstein, 2018). There are several hypotheses in circulation on how species occupy a certain niche (Granot et al., 2017), namely, the early succession hypothesis: early successional species are eventually responsible for occupying a niche; the abundance hypothesis: abundant species are more likely to occupy a niche; and the niche-breadth hypothesis: generalist species are more likely to thrive as they are capable of surviving under a wide range of conditions. In our original study, the microbial community data originated from 12 pens (each with their own environmental properties), which makes it a perfect candidate to explore concepts such as niche breadth and overlap as well as taxon-environment relationships in a recently developed null modeling-based MicroNiche framework (Finn et al., 2020).
To identify transient microbial taxa at very narrow ranges of a parameter of interest or as a result of disturbances on a temporal scale, there is an emphasis on conditionally rare taxa that have a bimodal response in terms of abundance (Lynch and Neufeld, 2015). These rare taxa provide a reservoir of functions and offer a means toward achieving resilience. Beyond this approach, we apply a null modeling-based specificity measure by Darcy et al. (2022) that is able to pick out taxa becoming active at narrow ranges. Our original study (Ijaz et al., 2018) has three diets, starter (days 0–10), grower (days 11–25), and finisher (days 26–35). We hypothesize that the introduction of individual diets should shift the microbiome by either the proliferation or disappearance of species and by making some microbes specific. This would also offer additional insights into microbial community function linked to dietary or environmental changes.
While the above approaches identify individual taxa, we are also interested in a minimal subset of taxa that form functional ecological groups based on statistical patterns. There is reasonable evidence to suggest that simplistic structure in community-level function and coarse-grained taxonomic groups arise as a result of nutrient availability (Goldford et al., 2018). These taxonomic or functional guilds assembled as a result of some outcome predictors have recently been studied as an ensemble quotient optimization problem (Shan et al., 2022), through which an “ensemble” (a minimal subset of the microbial community associated with a categorical or continuous outcome) can be extracted.
Working beyond understanding single species and their sub-composition, it is also important to understand top-level microbial community or ecosystem functioning. The recently developed method by Yonatan incorporates interacting traits such as commensalism, amensalism, competition, mutualism, and predator–prey in stability criteria (Allesina and Tang, 2012) without the need to generate co-occurrence patterns (Yonatan et al., 2022), as opposed to the traditional methods, to suggest the resilience of microbial communities against outside influences or disturbances.
The aim of this study is to explore the stability and assembly patterns of chicken cecum microbiota at diverse levels (coarse-grained to fine-grained) by revisiting the dataset of Ijaz et al. (2018) through the aforementioned novel analytical approaches. Some of these incorporate underlying ecology and environment (as discussed above); others, such as the generalized linear latent variable model (GLLVM) approach (Niku et al., 2019), were not available at the time of original publication and offer better predictive modeling for the metadata. Furthermore, with recent advancements in predictive metabolic modeling tools such as PICRUSt2 (Douglas et al., 2020), by virtue of an updated and larger database of gene families and functions, we are now better able to explore functional dynamics. Therefore, we have also explored the temporal dynamics of functional pathways and enzymes, including those related to antimicrobial resistance.
2. Materials and methods
A detailed description of the experimental design, sample collection, bird’s performance measurements, and sequencing is provided in the previous study (Ijaz et al., 2018). Briefly, the study comprises cecum microbiome samples of 396 broiler (Ross-308) that were allocated to 12 pens (33 broiler chicks/pen) and were fed with a three-phase diet, i.e., starter diet (up until day 10), a grower diet (from day 11 to day 25), and a finisher diet (from day 26 to day 35). One cecal sample was collected daily from each pen (12 samples/day), leading to 396 samples in total, out of which a total of 17 were removed from the final analysis due to poor gDNA quality, giving a final n = 379 samples, which were subjected to 16S rRNA amplicon sequencing using a V3–V4 primer set on an Illumina MiSeq, giving 300 bp paired-end reads.
2.1. Bioinformatics and statistical analysis
We have used the abundance table (p = 18,588 OTUs for n = 382 samples that include additional negative controls) and representative operational taxonomic units (OTUs) from Ijaz et al. (2018) with two modifications: (a) we re-classified the taxonomy of the OTUs using the recent SILVA SSU Ref NR database release v.138 (Quast et al., 2012), and (b) we re-generated the rooted phylogenetic tree with the QIIME2 framework (Caporaso et al., 2010). Furthermore, we used PICRUSt2 (Douglas et al., 2020) within the QIIME environment to recover KEGG enzymes and MetaCyc pathway predictions (not done in the original publication) for all the samples. For this purpose, we used the parameters --p-hsp-method pic --p-max-nsti 2 in qiime picrust2 full-pipeline.1 QIIME2 was also used to generate a final BIOM file that combined abundance information with the new taxonomy, along with the new phylogenetic tree, and the metadata were used for the downstream statistical analysis. The metadata comprised performance parameters related to each broiler including mean body weight (BW_mean), body weight gain (gain), feed intake (FI), and feed conversion ratio (FCR). These measurements were taken at time points 3–7 days, 8–14 days, 15–24 days, and 25–35 days. Statistical analysis was performed using the R software (R Core Team, 2013), with the methodology summarized in Figure 1 and the details provided in the Supplementary material.
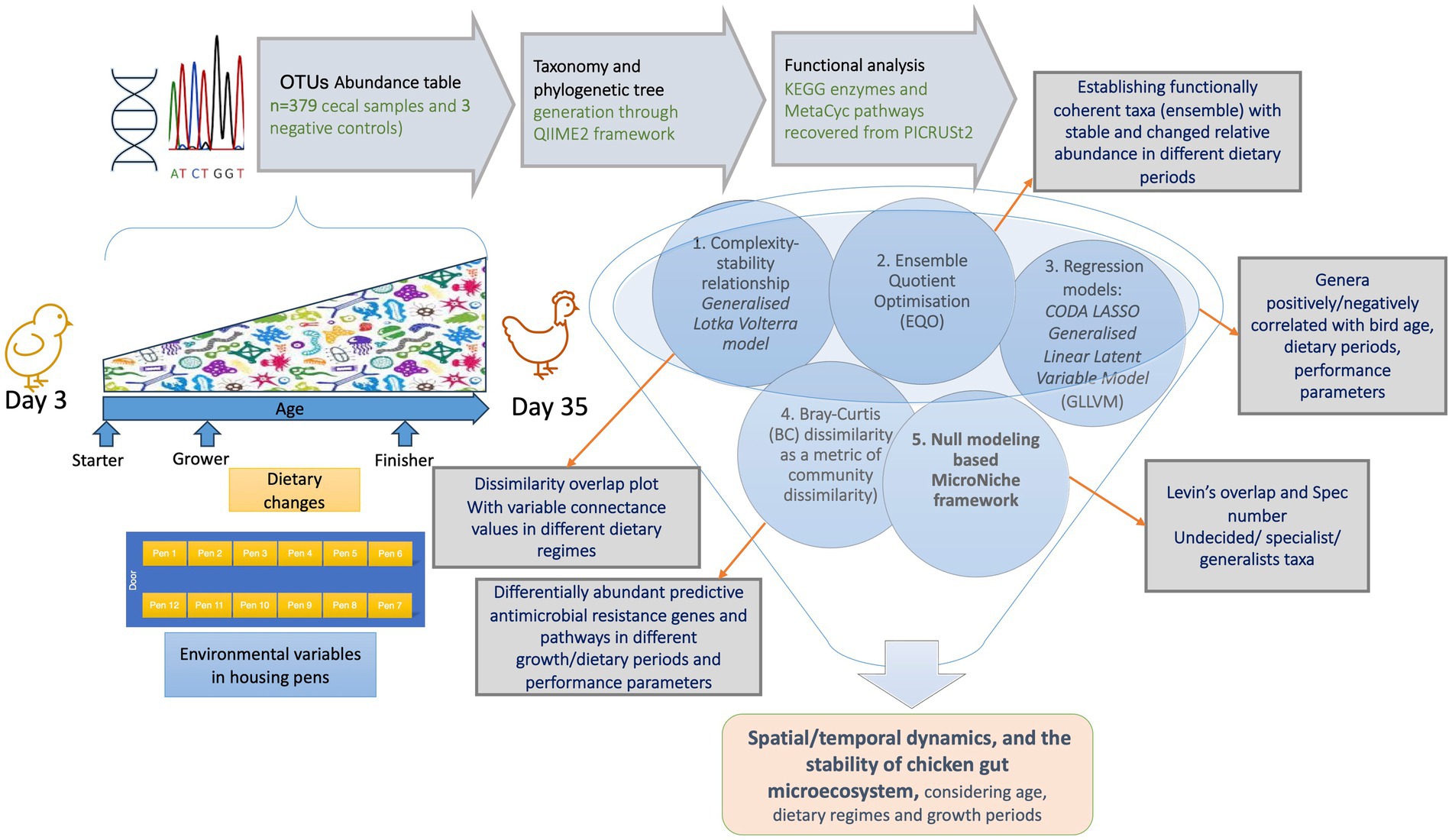
Figure 1. Schematic of the methodology used in this study with the details given in the Supplementary material.
3. Results
3.1. Overall complexity-stability patterns at a temporal scale
To estimate the interactions and complexity-stability profile of microbial communities within broiler guts, we calculated the values of effective connectance and effective number of species. Results were obtained in the form of a dissimilarity overlap curve for the moving window sizes of days 3, 4, 5, 6, and 7. A sudden shift in microbial communities observed during the 3rd week is more likely due to a change in diet or age of the bird, making communities more complex, dense, and unstable. This might result in the emergence or exclusion of some genera, thereby revealing the sensitivity of their abundances toward each other. High connectance values in the figure showed maximum instability in the 4th week, specifically after introducing the finisher diet (Figure 2).
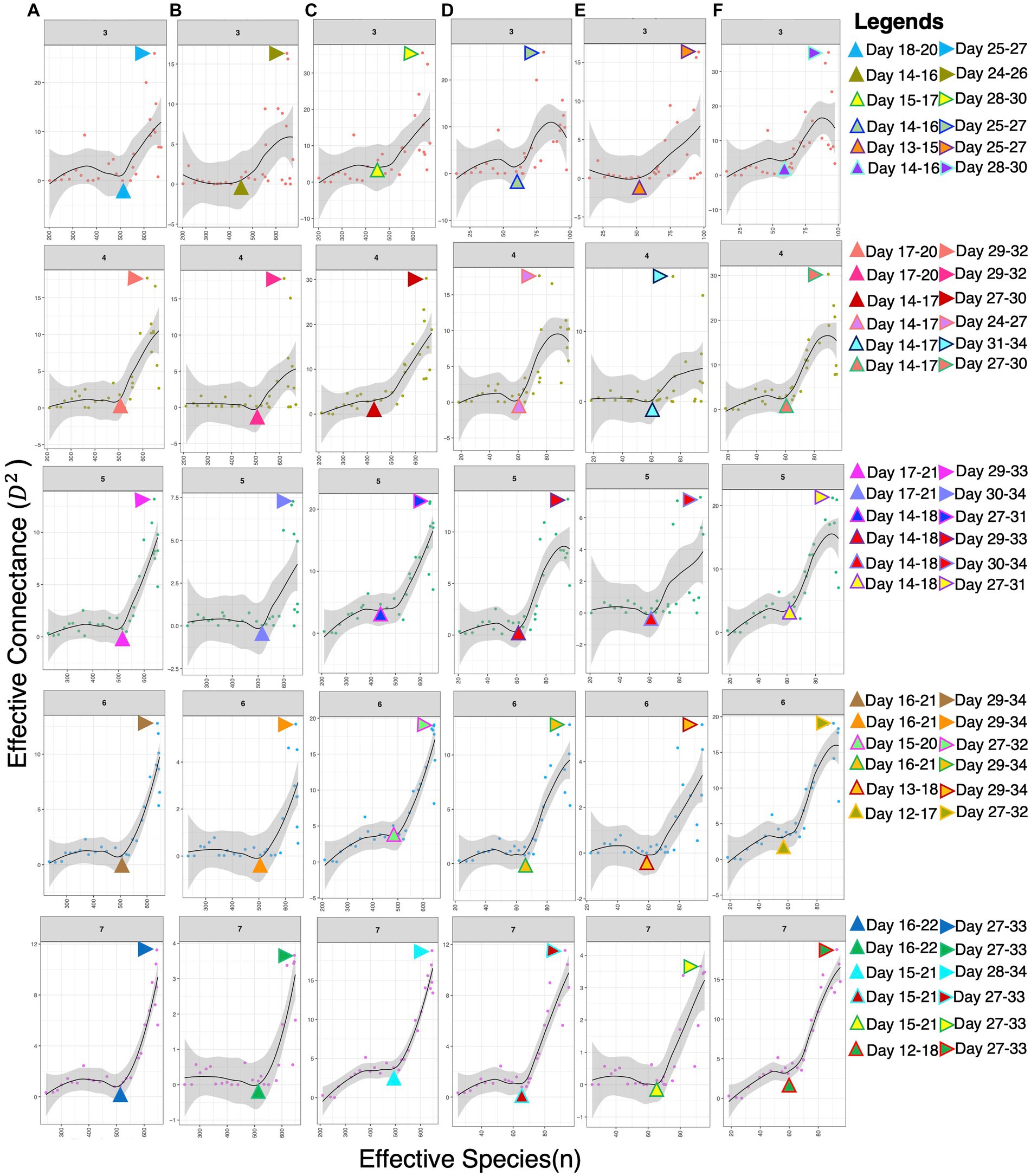
Figure 2. Stability-complexity relationship recovered for different dissimilarity measures and the ways in which the effective number of species n is calculated: (A) dissimilarity metric (DM): Jensen–Shannon divergence (RJSD); n: binary counting; (B) DM: Euclidean distance; n: binary counting; (C) DM: Spearman correlation; n: binary counting; (D) DM: RJSD; n: richness; (E) DM: Euclidean; n: richness; (F) DM: Spearman; n: richness. Since the method requires >35 samples, we have calculated the values on a moving window average for sizes 3, 4, 5, 6, and 7 days shown in the strips. For each window size and the chosen parameters, we have identified the days at which there is a drastic change in stability (represented by the triangle pointing up) with the values shooting up immediately after, and the days where there is maximum instability (represented by the triangle pointing right).
To find functionally coherent taxa (ensemble) with stable and changed relative abundance, we applied a novel approach, i.e., ensemble quotient optimization (EQO). We identified bacterial genera with stable relative abundance and minimum coefficient of variation (CV = 0.0399), including major anaerobes as commensals, e.g., Oscillibacter, Olsenella, Megamonas, Bifidobacterium, Lachnospiraceae, Shuttleworthia, Oscillospiraceae, Blautia, Lachnoclostridium, Subdoligranulum, Faecalibactrium, Butyricicoccus, and Lactobacillus (Figure 3). We also identified genera with changed relative abundance and a high correlation (R = 0.8701) based on statistical variations in age (and other environmental factors provided in the Supplementary material) and gut microbiome composition (see Figure 4). In addition to others, Colidextribacter, Oscillospiraceae; NK4A214_group, Intestinimonas, and Ruminococcus were picked out as major genera with changed relative abundance throughout the productive age of birds (Figure 4).
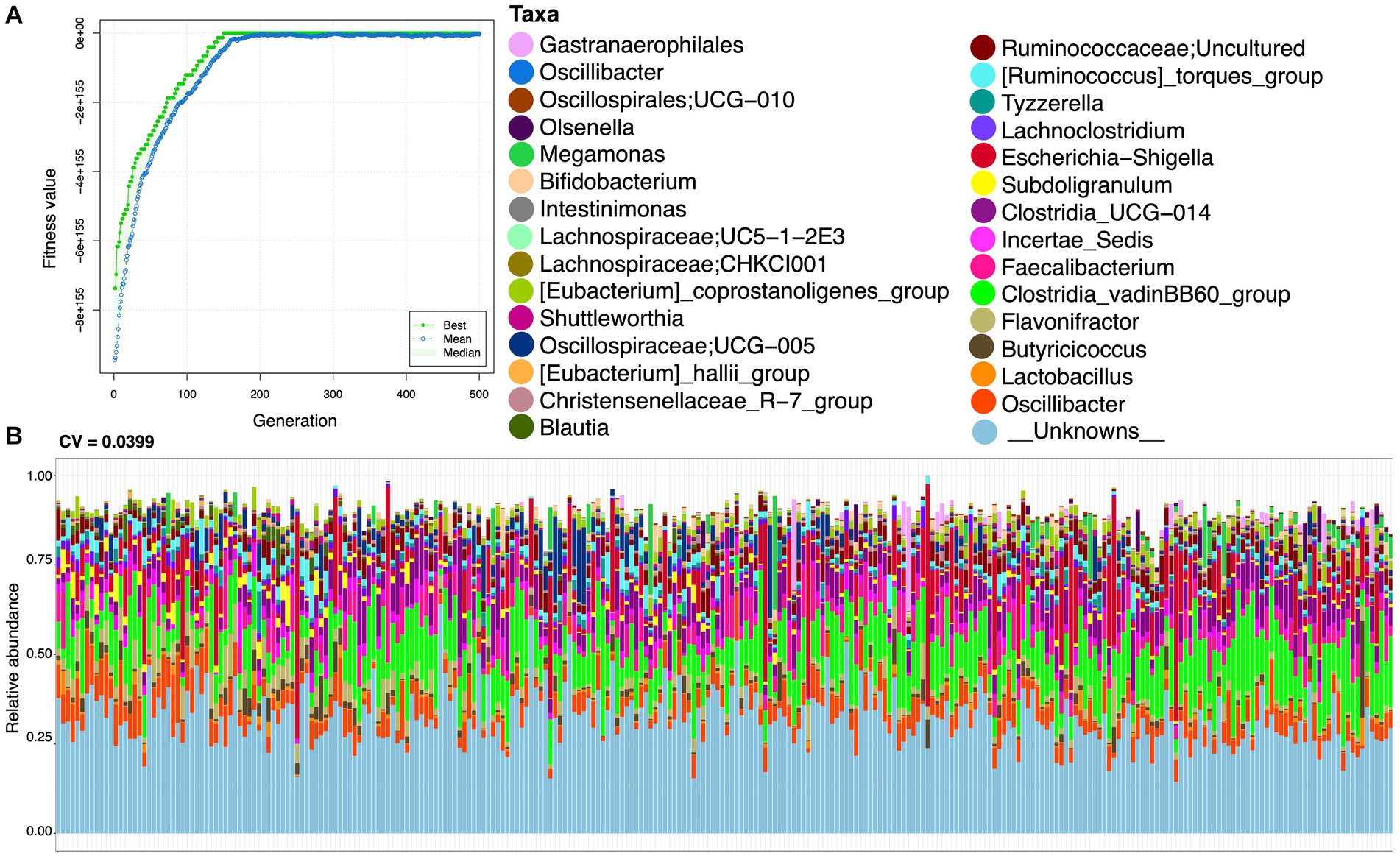
Figure 3. Stable ensemble returned after running the EQO algorithm in uniform phenotypic variable mode, with (A) showing the fitness value evolution of the genetic algorithm in finding these ensembles, highlighting the convergence to a steady state solution, and (B) showing the relative abundance profiles with coefficient of variation (CV) values given on the top of the plot. The lower CV value signifies higher stability. Here, we have only used the samples when the starter diet was given to the broiler.
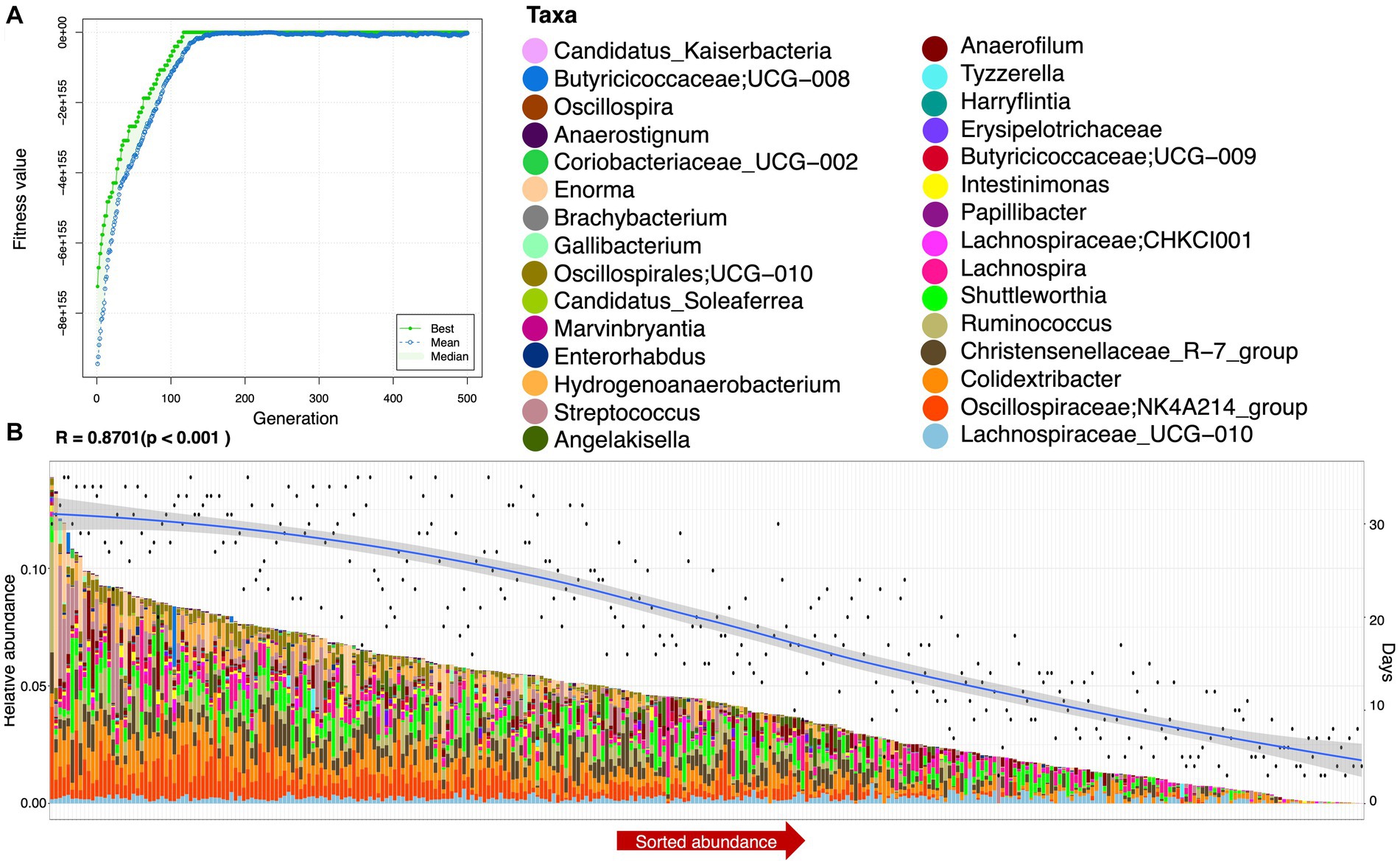
Figure 4. Ensemble (a minimal subset of genera) returned after applying the EQO technique using days as a continuous predictor. (A) Shows the fitness value evolution of the genetic algorithm in finding these ensembles, highlighting the convergence to a steady state solution, and (B) shows the sorted abundance of the ensembles with a biplot of smooth values of days (right y-axis) and shows the correlation value between the ensemble and days just above the plot. This has been performed for all samples.
Next, we applied a regression model, i.e., the generalized linear latent variable model (GLLVM), in order to identify genera that positively or negatively correlate with bird’s age (Figure 5A). Some SCFA-producing bacteria, including Izemoplasmatales, Gastranaerophilales, (Eubacterium)_ventriosum_group, and Roseburia, are found as top genera in positive correlation with age. Certain taxa, such as Sporomusa, Campylobacter, Clostridium_sensu_stricto_1, Enterococcus, and the phytopathogen Paenibacillus, negatively correlated with bird’s age, indicating a greater risk of disease in the initial days.
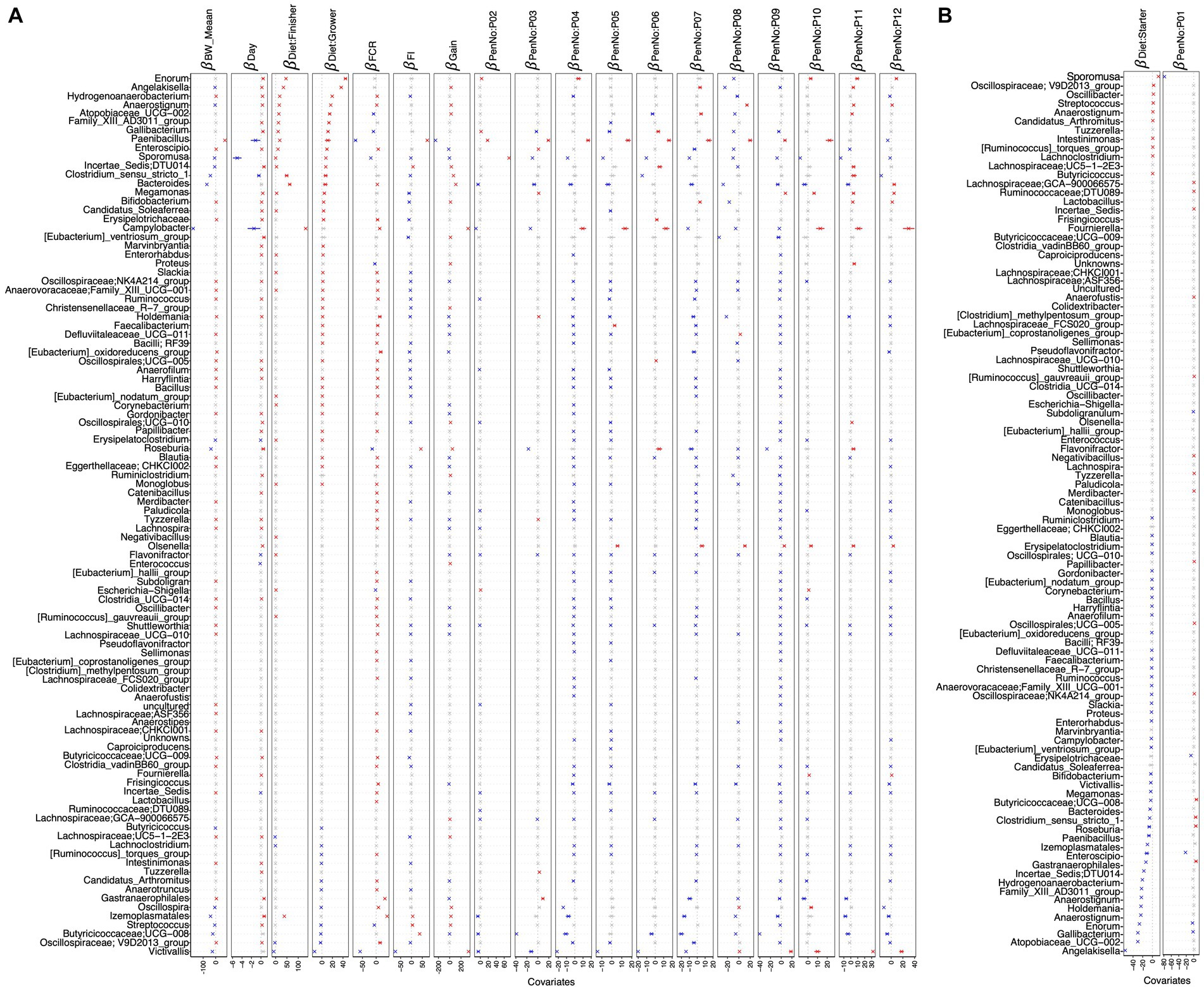
Figure 5. -coefficients returned from the GLLVM procedure for covariates considered in this study. The top 100 most abundant genera were considered, incorporating both continuous data (BW_mean, day, FCR, FI, and gain) as well as categorical labeling of the samples (pen and diet). Those coefficients that are positively associated with the microbial abundance of a particular genus are represented in red color, while those that are negatively associated are represented in blue color, respectively. Where the 95% confidence interval of the -coefficients crosses the 0 boundary, the coefficients are insignificant and are represented by a gray color. Since the collation of OTUs was performed at the genus level, all those OTUs that cannot be categorized based on taxonomy are collated under “__unknowns__” category. Incorporating a categorical variable in a regression model involves dropping one level as a reference when using one-hot encoding, and therefore, the algorithm was run twice: (A) with diet: starter and PenNo: P01 as references and (B) with diet: grower and PenNo: P02 as references. For (B), the remaining coefficients are shown in Supplementary Figure S12.
3.2. Taxonomic and functional structure (stable and changing) for different dietary regimes (starter, grower, and finisher)
After applying EQO to different dietary regimes, i.e., starter, grower, and finisher, genera with stable and changed relative abundances were identified. The genera with stable relative abundance in the initial 10 days when the starter diet was offered to the birds mostly include genera involved in feed digestion, such as Oscillibacter, Clostridia_vadinBB60_group, Flavonifractor, Lachnoclostridium, and Faecalibacterium. Broilers were also found to be at higher risk of some pathogenic genera, e.g., Escherichia-Shigella, during that time (Supplementary Figure S6). As the diet shifted from starter to grower, a change in stable genera was noticed, including Tyzzerella, Clostridia_UCG-014, (Eubacterium)_coprostanoligenes_group, and Bifidobacterium (Supplementary Figure S8). In addition to the previously mentioned genera, Ruminococcus and (Ruminococcus)_torques_group also stabilized their abundance when a finisher diet was offered (Supplementary Figure S10).
The genera identified with changed abundance in the starter diet period were Subdoligranulum, Tyzzerella, Ruminococcaceae; DTU089, Oscillospiraceae; UCG-005, and (Eubacterium)_coprostanoligenes_group (Supplementary Figure S7). As a grower diet was provided, Angelakisella, Hydrogenonanaerobacterium, Oscillospirales; UCG-010, Butyricicoccaceae; UCG-009, Streptococcus, and Anaerofilum were found in varied abundances (Supplementary Figure S9). It is suggested that the relative abundance of Megamonas and Enorma decreased when a finisher diet was provided (Supplementary Figure S11).
After applying the generalized linear latent variable model (GLVVM), Oscillospiraceae; V9D2013_group, and Streptococcus were found to be positively associated with the starter diet period but negatively associated with the grower and finisher diet periods. Angelakisella and Coriobacteriaceae_UCG-002 are found as top genera associated positively with grower diet periods but negatively with starter diet periods. Izemoplasmatales are associated positively with the finisher diet but negatively with the grower diet. Enorma and Victivallis showed positive and negative associations, respectively, with grower/finisher diet periods. Moreover, Sporomusa, Oscillospira, and Anaerotruncus positively correlated with the starter diet. Similarly, Hydrogenoanaerobacterium showed a positive correlation with the grower diet. In addition, Campylobacter, Clostridium_sensu_stricto_1, and Bacteroides are among the top genera with a positive association with finisher diet. Gallibacterium is negatively correlated with the starter diet. Butyricicoccaceae; UCG-008, Lachnospiraceae; UC5-1-2E3, and Lachnoclostridium are negatively associated with grower/finisher diets (Figures 5A,B).
3.3. Performance parameters and their association with key microbes and functions
To identify the significant genera in terms of their increased or decreased abundance in association with performance parameters including BW_mean, FI, FCR, and gain, we applied two regression models, including CODA LASSO and GLLVM (Supplementary Table S1). From both of the analyses, we found key probiotic genera positively associated with BW_mean, including Paenibacillus, Oscillospiraceae; V9D2013_group, Oscillospirales; UCG-010, NK4A214_group, UCG-005, Holdemania, Shuttleworthia, Bifidobacterium, Colidextribacter, and Butyricicoccaceae; UCG-009. In contrast, some pathogenic anaerobes are found to be in negative association with BW_mean, such as Victivallis, Campylobacter, Sporomusa, Clostridium_sensu_stricto_1, Anaerofustis, and Anaerovoracaceae; Family_XIII_AD3011_group (Figure 4A; Supplementary Figure S20).
Some major commensals, including Oscillospiraceae; V9D2013_group, Oscillospirales; UCG-010, NK4A214_group, UCG-005, Butyricicoccaceae; UCG-008, Roseburia, Shuttleworthia, Hydrogenoanaerobacterium, Fournierella, and Colidextribacter are related to improved gut health and increased FI. We also found some probiotic genera negatively associated with FI (improved FCR), including Holdemania, Eubacterium_ventriosum_group, nodatum_group, hallii_group, Bifidobacterium, Lachnospiraceae; GCA-900066575, ASF356, Eubacterium_oxidoreducens_group, Ruminiclostridium, Allisonella, Lachnospiraceae; UC5-1-2E3, FCS020_group, Erysipelotrichaceae, Anaerofustis, Butyricicoccaceae; UCG-009, and Subdoligranulum (Figure 5A; Supplementary Figure S22).
Increased abundance of some beneficial genera such as Oscillospiraceae; V9D2013_group, Oscillospirales; UCG-010, NK4A214_group, UCG-005, Roseburia, Shuttleworthia, Oscillospira, Bifidobacterium, Anaerovoracaceae; Family_XIII_UCG-001, Fournierella, and Colidextribacter are noticed, resulting in more gain. Some commensals are also found to be negatively related to gain (Figure 5A; Supplementary Figure S21).
In terms of FCR, major commensals are found to be in negative association, including Paenibacillus, Sporomusa, Roseburia, Lachnospiraceae; GCA-900066575, ASF356, (Clostridium)_methylpentosum_group, and Clostridiaceae; Candidatus_Arthromitus, which may result in improved feed efficiency and more weight gain (Figure 5A; Supplementary Figure S23).
3.4. Predictive antimicrobial resistance evolution and key relationships with predictors
To find the contribution of predictive antimicrobial resistance genes (piARGs) and other genes for the whole experimental period, we applied Bray–Curtis contribution analysis, doing pairwise comparisons (Figures 6A,B). Overall, piARGs contribute at a stable level, but there is a slight increase in abundance, particularly when the diet is changed. An increase in piARGs abundance can be seen on day 11 when the grower diet was introduced. In the initial 10 days until the starter diet was offered, it was observed using EQO analysis that genera like Oscillibacter, Clostridia_vadinBB60_group, Flavonifractor, Lachnoclostridium, and Faecalibacterium were found in most abundance, and here, we can predict that these genera are involved in contributing the piARGs. Similarly, the percentage of piARGs increased in the finisher diet period, and during this period, Ruminococcus and (Ruminococcus)_torques_group also stabilized their abundance, so we can predict that these genera are contributing more piARGs. In general, piARG’s contribution increased in the initial couple of weeks and then stabilized for the rest of the life span.
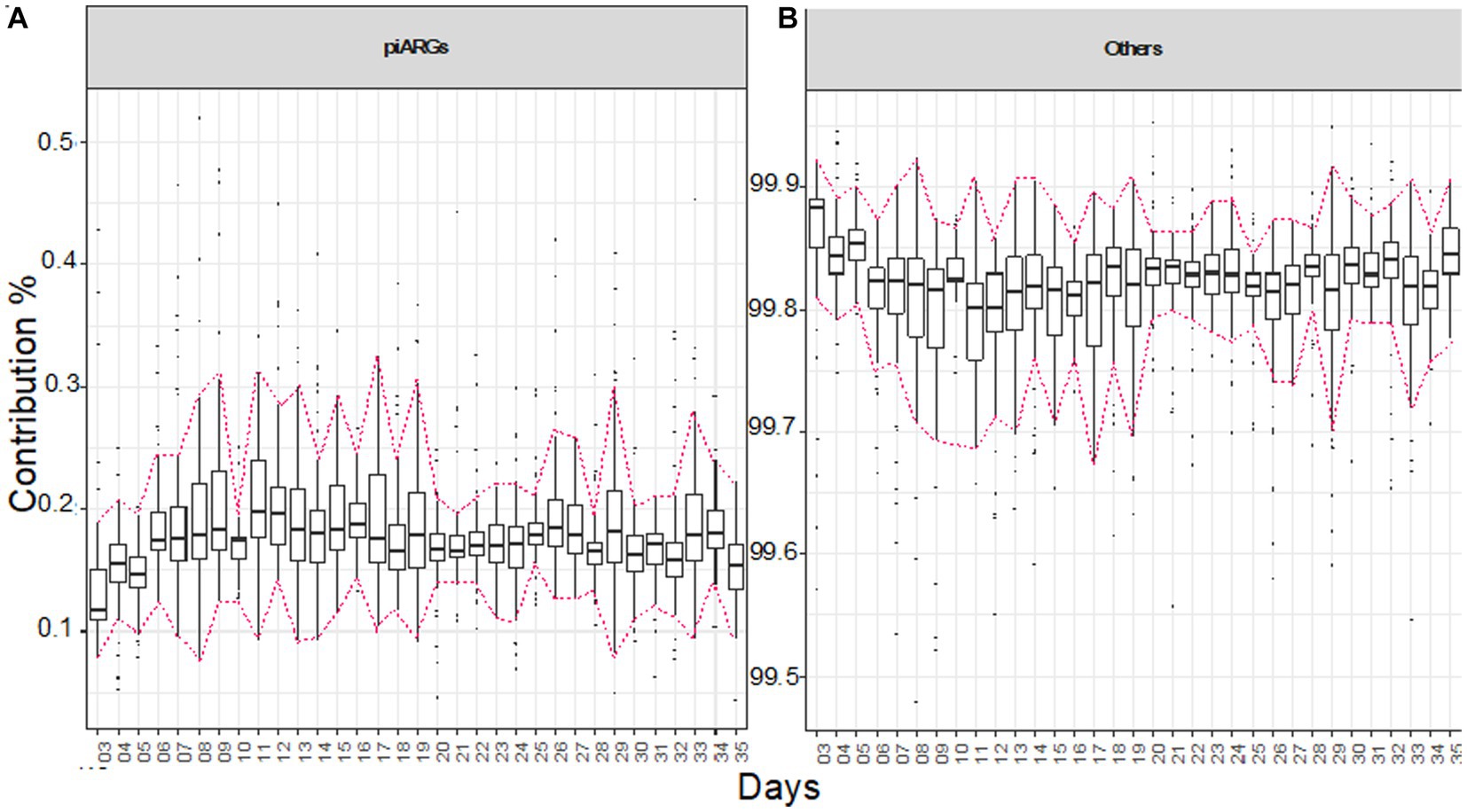
Figure 6. Bray–Curtis contribution split of KEGG genes to piARGs genes (A) and the rest of the genes (B). For each day, the plot shows pair-wise comparison of samples (N ≅ 12 pens/day). The highlighted area shows when the diets were administered, in chronological order as starter, grower, and finisher diets. Tukey’s honest significant difference (HSD) test as a post-hoc test was used to assess if the contribution by piARG KOs was significantly different between the days, with the details given in Supplementary Table S5.
Considering performance parameters, i.e., BW_mean, FI, FCR, gain, and different pens in which birds were allocated, as environmental covariates, the abundance of piARGs considering KEGG orthologs (KOs) was analyzed using GLLVM (Figures 7A,B and Supplementary Table S2). A total of 34 piARGs were found to have a positive correlation with the defined variables, out of which 20 belong to the drug group of beta-lactams, including carbapenems, extended-spectrum cephalosporins, extended-spectrum penicillin, and monobactams; 12 belong to aminoglycoside drug groups; and 2 belong to trimethoprim.
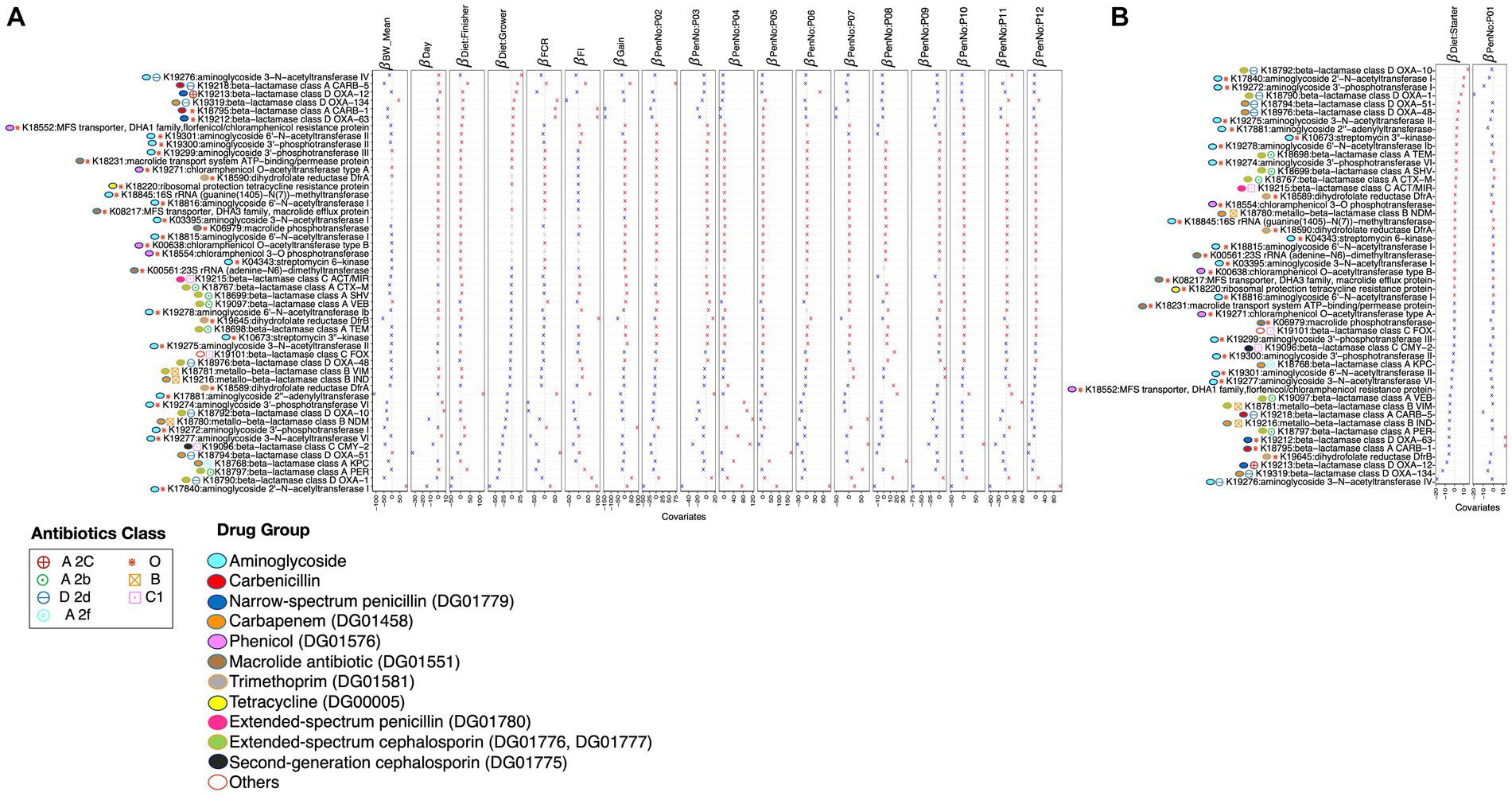
Figure 7. -coefficients returned from the GLLVM procedure for covariates considered in this study by considering 50 out of 90 reference piARGs KEGG orthologs (KOs) detected in this study using the PICRUSt2 procedure. Those coefficients that are positively associated with the abundance of a particular piARG KO are represented in red color, while those that are negatively associated are represented in blue color. Where the 95% confidence interval of the -coefficients crosses the 0 boundary, the coefficients are insignificant and are represented by a gray color. Similar to Figure 4, the algorithm was run twice: (A) with diet: starter and PenNo: P01 as references and (B) with diet: grower and PenNo: P02 as references. For (B), the remaining coefficients are shown in Supplementary Figure S13. Additional annotations of threat levels, classes, and drug groups; these piARGs are categorized.
We also identified 24 piARGs with a negative correlation to defined variables, out of which 15 belong to the beta-lactam drug group, including carbapenem, extended-spectrum cephalosporins, extended-spectrum penicillin, and monobactams; 7 belong to the aminoglycoside drug group; 1 belongs to trimethoprim; and 1 belongs to phenicol-resistant protein.
The piARGs are positively associated with the species that are categorized in a group with serious threats by the CDC, which includes multidrug-resistant Acinetobacter, multidrug-resistant Pseudomonas aeruginosa, drug-resistant non-typhoidal Salmonella, drug-resistant Salmonella enterica serovar Typhi, drug-resistant Shigella, and drug-resistant Streptococcus pneumoniae.
In general, the majority of the piARGs belonging to the drug group of aminoglycosides (DG01447) are upregulated in the finisher diet period and are also positively correlated with most performance parameters. In contrast, piARGs belonging to the drug group of carbapenems (DG01458) are found to be downregulated in grower diet/finisher diet periods and negatively associated with most performance parameters. piARGs belonging to extended-spectrum cephalosporin (DG01776 and DG01777) and penicillin (DG01780) are found to be more abundant in the starter diet period but less abundant in the finisher diet period, and they also show a negative correlation with the age of the birds. It has been made evident that the drug groups of second- and third-generation cephalosporin (DG01775 and DG01776) are positively correlated with BW_mean of birds. Extended-spectrum cephalosporin (DG01776 and DG01777) and monobactam (DG01454) are negatively associated with the grower and finisher diet periods. Carbenicillin (DG00519) showed a positive association with the grower and finisher diet periods but a negative association with the starter diet period. Some KOs belonging to trimethoprim (DG01581), extended-spectrum penicillin (DG01780), second-generation cephalosporin (DG01775), phenicol (DG01576), and cephalosporins (no DG number) are positively or negatively associated with some of the pens (e.g., P08).
To determine how age (days) and different performance parameters (BW_mean, FCR, FI, and gain) contribute to the functional profiles of bacterial communities, we applied the CODA-LASSO regression model to recovered metacyc pathways (Supplementary Tables S3, S4). The majority of pathways involved in amino acid biosynthesis are found to be positively correlated with bird’s age such as L-histidine biosynthesis, L-arginine biosynthesis I (via L-ornithine), and the superpathway of taurine degradation. Some nucleotide biosynthesis/repair pathways, like guanosine ribonucleotides de novo biosynthesis and pyrimidine deoxyribonucleotides de novo biosynthesis II, were also found to have a positive correlation with the age of birds. Additionally, a peptidoglycan biosynthesis pathway and a coumarin biosynthesis pathway, most likely conferring some antibiotic resistance, were also found among the top positively correlated pathways to age. In contrast, pathways related to cofactors/coenzymes biosynthesis like tetrapyrrole biosynthesis I (from glutamate), foliate transformations III (E. coli), and nicotinamide adenine dinucleotide (NAD) biosynthesis are found to be negatively associated with age (Supplementary Figure S19). Age progression was positively associated with pathways involved in protein, DNA, and peptidoglycan biosynthesis, while pathways related to cofactors/coenzymes biosynthesis were negatively associated with most of the performance parameters (Supplementary Figures S20–23).
3.5. Environmental niche breadth and overlap
From the GLLVM, we estimated the effect of different environments, considering the 12 pens in which birds were allocated (Supplementary Figure S1), on the abundance of major bacterial genera. Some commensals are found in positive association with most of the pens, including Paenibacillus, Enorma, Roseburia, Olsenella, and Megamonas. Similarly, some other commensals such as Oscillospiraceae; V9D2013_group, Oscillospirales; UCG-010, Bacteroides, Butyricicoccaceae; UCG-008, and Shuttleworthia were detected in negative relation to some pens. Some pathogenic genera, such as Victivallis and Campylobacter, were also found to be either positively or negatively associated with certain pens (Figures 5A,B and Supplementary Table S1).
We applied Levin’s BN to find bacterial genera that are generalists or specialists, considering pens as different sets of environments. We did not find any generalists, but all the above-mentioned genera were found as specialists in addition to others. Then, we applied Hulbert’s BN to find positive and negative associations between specialist genera and environmental properties, i.e., BW_mean, FI, FCR, gain, and days (Supplementary Figures S17, S18), within different environments. Furthermore, genera were selected from Levin’s BN to calculate Levin’s overlap, highlighting some specialists including Gallibacterium, Enorma, Coriobacteriaceeae_UCG-002, and Proteus (Figures 8A,B).
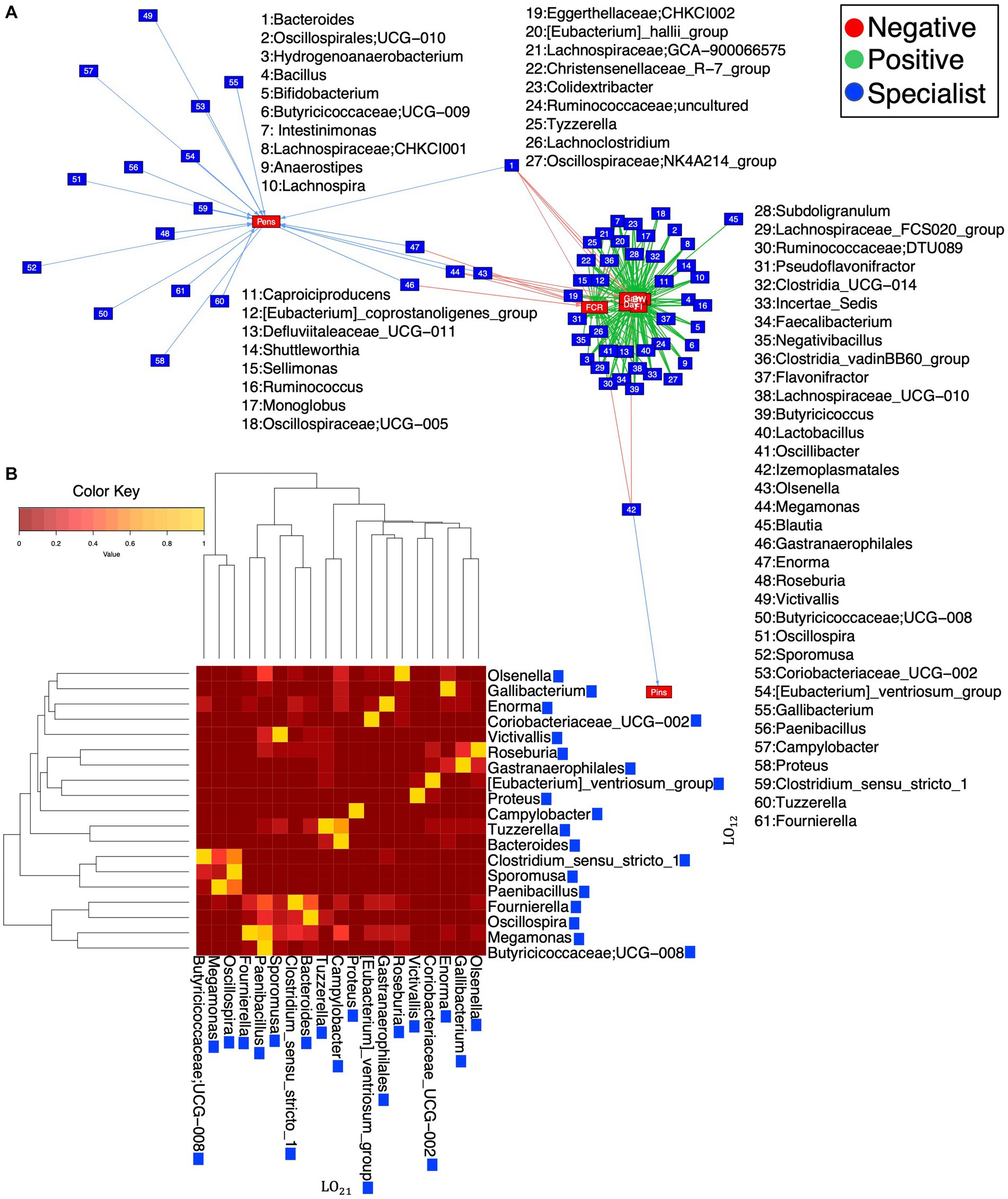
Figure 8. Network of relationships (A) recovered after applying Levin’s BN to find generalists and specialists and Hurlbert’s BN to find positive and negative associations with respect to environmental properties. These properties are mean body weight (BW_mean), feed intake (FI), feed conversion ratio (FCR), gain, and days calculated on different sets of environments (R ≅ 12 pens). The genera selected from Levin’s BN are further used to calculate Levin’s overlap (B), with blue boxes signifying that they are all specialists. See further details in Supplementary Figures S17, S18.
To explore if certain genera exist within a narrow range of covariates (days, BW_mean, FI, FCR, and gain) and different dietary regimes (starter, grower, and finisher), we used a null modeling approach to calculate the “spec” number. This number offers a threshold to decide between cosmopolitan (>0) and specific (<0) in Figure 9A. Pairwise comparisons of all covariates were done to calculate correlation coefficients, indicating a high correlation between covariates (Figures 8A,B; Supplementary Figures 24–31). We found that gut microbial communities exhibited strong and statistically significant specificity to several environmental variables, in particular days (bird’s age), which implicitly capture the underlying dietary regimen. Genera found specific to the starter diet period were Brebundimonas, Brevibacillus, Sphingopyxis, Allorhizobium-Neorhizobium-Paraihizobium-Rhizobium, Paenibacillus, Pediococcus, Trachelomonas, and Cupriavidus. Specific genera in the grower diet period include Microbacterium, Allisonella, Campylobacter, Rhodococcus, Lysinibacillus, and Mesorhizobium. In the finisher diet period, Oscillospiraceae; UCG-007, Bacteroides, Gallibacterium, Izemoplasmatales, Incertae_Sedis; DTU014, DTU014, DTU014, Romboutsia, Gastranaerophilales, Victivallis, Butyricicoccaceae; UCG-008, Oscillospira, Coriobacteriaceae_UCG-002, and Enteroscipio found as specific genera (Supplementary Figures S24, S25).
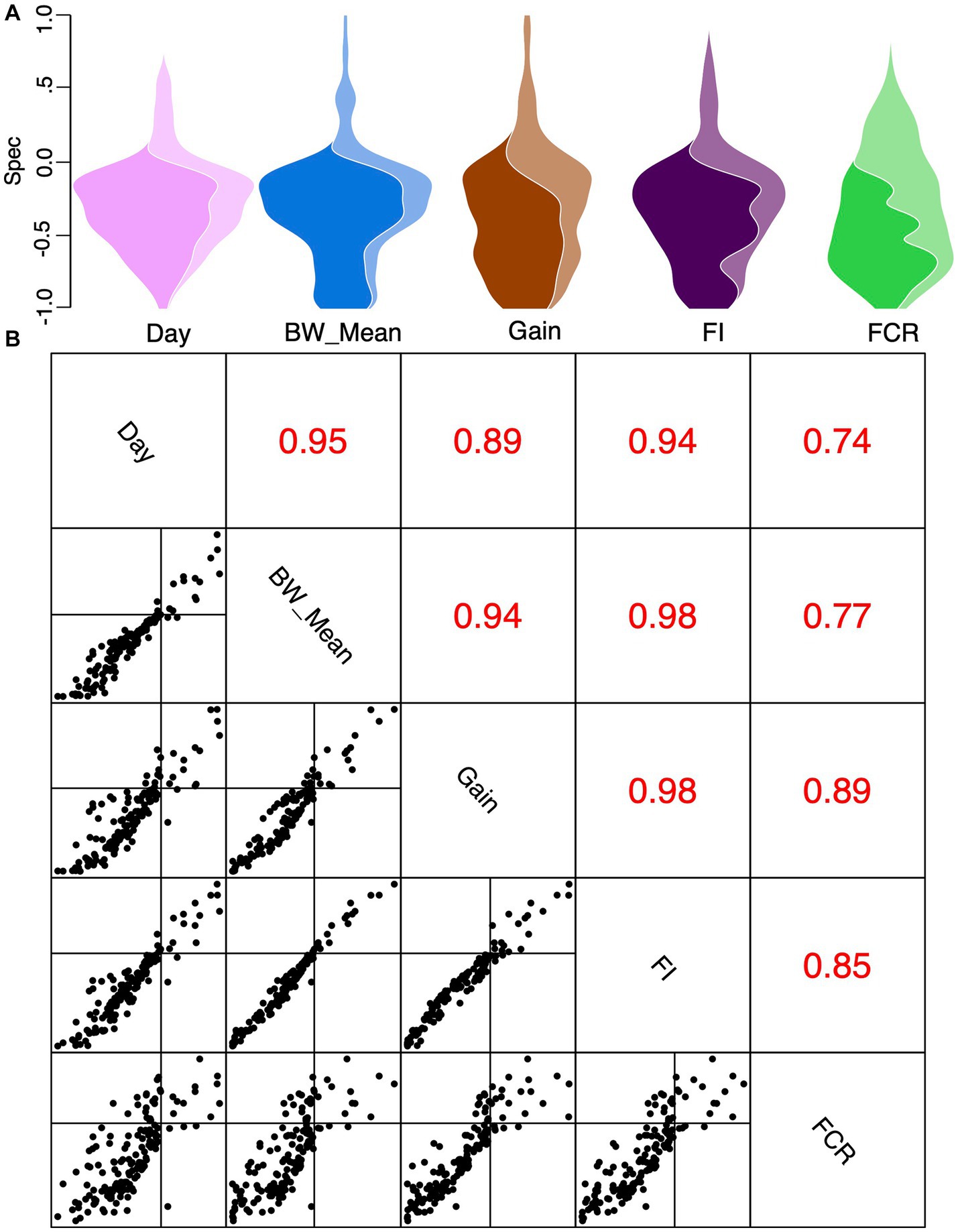
Figure 9. Specificity values Spec shown as a violin plot with the area divided between genera with statistically significant specificity (dark) versus genera without (light) for different covariates considered in this study (A). Pairwise Spec correlations (B) with correlation coefficients (R) for each pairwise comparison of covariates are shown in this subplot’s upper triangles for the data plotted in the lower triangle of this subplot.
4. Discussion
At the global level, chicken, being the dominant livestock, has the optimal calorie retention when considering feed conversions (Fry et al., 2018). Productivity in terms of weight gain and disease resistance is highly influenced by gut bacterial communities. Here, in this manuscript, we elucidate the spatial temporal dynamics of broiler cecal microbiota development by emphasizing on stability and complexity of bacterial species as different diets are introduced in their development cycle (from day 3 to day 35). In doing so, we are also able to ascertain the niches some of the species occupy and establish links between gut bacterial profiles and environmental (performance) covariates.
Our results demonstrate that gut communities are majorly influenced by the age of birds, highlighting the significance of different growth periods in microbial community assembly mechanisms, which is also supported by previous studies (Crhanova et al., 2011; Danzeisen et al., 2011; Oakley et al., 2014; Mohd Shaufi et al., 2015; Pourabedin et al., 2015; Joat et al., 2021; Xiao et al., 2021; Zou et al., 2022). The age of birds is found to be the most important factor that may affect the stability of microbial communities. Our results suggest that based on the interaction of bacterial species, the microbiota starts to become unstable at the onset of the 3rd week, with instability gradually increasing. Instability is also previously reported beyond the 5th week (Lu et al., 2003; Feye et al., 2020; Li et al., 2022), which affects the growth performance of the bird. We explored some ecological relationships between microbial communities and found functionally coherent taxa with respect to their relative abundances (uniform or varied). In the majority of cases, anaerobic bacteria dominated as commensals and were identified as members of a stable or uniform ensemble, including Oscillibacter, Megamonas, Shuttleworthia, Blautia, Lachnoclostridium, Subdoligranulum, Faecalibactrium, Butyricicoccus, Lactobacillus, Olsenella, and Bifidobacterium. While some anaerobes, such as Oscillospiraceae; NK4A214_group, Intestinimonas, and Ruminococcus were found to be part of a changing ensemble with varied relative abundances. Most of the genera are involved in saccharolytic fermentation and belong to Firmicutes, the dominant phylum, which has also been braced by many microbiome studies in the preceding decade (Danzeisen et al., 2011; Choi et al., 2014; Xiao et al., 2017; Clavijo and Flórez, 2018; Venegas et al., 2019; Mandal et al., 2020; Han et al., 2022). Furthermore, genera having a positive or negative correlation with age were also highlighted through regression models, i.e., GLLVM. Some genera, predominantly short-chain fatty acids (SCFAs) producers, e.g., Izemoplasmatales, Gastranaerophilales, (Eubacterium)_ventriosum_group, and Roseburia were found to have increasing species abundance as birds matured, and vice versa. Certain taxa, such as Sporomusa, Clostridium_sensu_stricto_1, and Enterococcus, negatively correlated with the bird’s age. These findings indicate that birds are potentially at higher risk of disease and death (especially under poor management practices) in their initial days, as supported by some studies (Hafez and Attia, 2020; Yerpes et al., 2020). Although there are disparities in the reported literature, the major factors often implicated in microbial compositional changes include rearing periods, genetics, sampling sections of gastrointestinal tracts, age, feed, and management practices (Lu et al., 2003; Ballou et al., 2016; Ranjitkar et al., 2016; Wang et al., 2016; Ngunjiri et al., 2019).
In addition to applying EQO to the whole 33 days time window, we wanted to know what remained stable during different diet periods. We found the diet to drive changes in microbial communities, particularly when they changed. Studies have demonstrated the effect of dietary composition along with food particle size on chicken gut microbial populations (Apajalahti et al., 2001, 2004). The genera with stable relative abundance in the initial 10 days (starter diet period) mostly included anaerobic Firmicutes and Bacteroidetes such as Oscillibacter, Clostridia_vadinBB60_group, Flavonifractor, Lachnoclostridium, and Faecalibacterium. The majority of them were previously found to be involved in proteolytic fermentation, producing SCFAs, and branched chain fatty acids (BCFAs) (Macfarlane et al., 1992; Smith and Macfarlane, 1998; Aguirre et al., 2016). We hypothesize that these findings are a consequence of common dietary practices at poultry farms where the starter feed is typically rich in protein composition (>20%) (Esmail, 2016). Note that, in the initial days, birds are found to be at higher risk of being exposed to pathogenic genera such as Escherichia-Shigella. When birds are shifted to a grower diet, a change in stable genera is noticed, with the major inclusion of commensals such as Tyzzerella, Clostridia_UCG-014, (Eubacterium)_coprostanoligenes_group, and Bifidobacterium. Likewise, some cellulolytic genera, including Ruminococcus and (Ruminococcus)_torques_group also stabilized their abundance when the finisher diet was introduced. The genera with varied abundance in the starter diet period are predominantly SCFA producers, including Subdoligranulum, Tyzzerella, Ruminococcaceae; DTU089, Oscillospiraceae; UCG-005, and (Eubacterium)_coprostanoligenes_group. Some commensals as well as pathogens, including Angelakisella, Hydrogenonanaerobacterium, Oscillospirales; UCG-010, Butyricicoccaceae; UCG-009, Streptococcus, and Anaerofilum, are found to have changed abundance during the grower diet period. It was also found that the abundances of some commensals, such as Megamonas and Enorma, went down when the finisher diet was introduced.
To further our understanding of different genera in the context of sources of variation, a regression model (GLVVM) was applied to fit the abundance of each genus against all sources of variability that we observed, primarily focusing on different diets. Strict anaerobes like Oscillospiraceae; V9D2013_group were found to be positively associated with the starter diet but negatively associated with the grower and the finisher diets. This is in line with studies revealing that initial chicken gut colonizers are mostly facultative anaerobes, followed by strict anaerobes specifically after day 3 (Rehman et al., 2007; Stanley et al., 2014). Some anaerobes most likely involved in energy production, such as Angelakisella and Coriobacteriaceae_UCG-002, are found to be the top genera associating positively with the grower diet but negatively with the starter diet. This is supported by the fact that grower diets are high in energy content as compared to the starter diet (Yücel and Taşkin, 2018). Enorma has shown a positive association with grower/finisher diets, which was previously found to be involved in the production of micronutrients (Khan and Chousalkar, 2020; Gong et al., 2021). Moreover, some probiotic anaerobes, including Sporomusa, Oscillospira, and Anaerotruncus, were positively associated with the starter diet. A positive association of Hydrogenoanaerobacterium with the grower diet was also observed in (Clavijo et al., 2022), where they showed a strong association with the diet and age of broilers. In addition to some opportunistic pathogens like Campylobacter and Clostridium_sensu_stricto_1, saccharolytic bacterial genera such as Bacteroides are among the top genera with a positive association with the finisher diet. Gamma proteobacteria, i.e., Gallibacterium, was found to be positively associated with the grower diet and was previously implicated in many avian species (El-Adawy et al., 2018). Additionally, some anaerobic genera like Butyricicoccaceae; UCG-008, Lachnospiraceae; UC5-1-2E3, and Lachnoclostridium were negatively associated with both grower and finisher diets.
Genera that had increased or decreased abundances in association with the performance parameters were identified through two regression models, i.e., CODA LASSO and GLLVM. We found key probiotic genera positively associated with BW_mean, FI, gain, and FCR, including Paenibacillus, Oscillospiraceae; V9D2013_group, Oscillospirales; UCG-010, NK4A214_group, UCG-005, Lachnospiraceae; GCA-900,066,575, ASF356, Holdemania, Roseburia, Fournierella, Shuttleworthia, Bifidobacterium, Colidextribacter, and Butyricicoccaceae; UCG-009, UCG-008. The majority of these were previously associated with improved growth performance in chickens as they increased resistance to infections and balanced the gut ecosystem (Perić et al., 2010; Amara and Shibl, 2015; Sarangi et al., 2016; Wang et al., 2017). In parallel, we have also found some understudied but most likely pathogenic genera in a negative association with the growth parameters, such as Victivallis, Sporomusa, Clostridium_sensu_stricto_1, Anaerofustis, and Anaerovoracaceae; Family_XIII_AD3011_group.
In addition to finding positive and negative associations between microbial species and the continuous predictor variables, a specificity analysis was performed. This unraveled proliferation of microbes within a narrow “specific” range, e.g., bird’s age, coincided with time periods when the diets were introduced and are in line with the literature (van der Wielen et al., 2002; Hiett et al., 2013; Cui et al., 2017). Note that some of the variables had a very strong correlation, and thus species that were found to be specific to mean body weight are likely to be similar to those found to be specific to days. Indeed, the same is true for other parameters, albeit to a lesser extent. Genera that were found to be specific to the starter diet, albeit when birds were of low weight, were non-fermenting aerobic autotrophs such as Brebundimonas, Brevibacillus, Sphingopyxis, and Cupriavidus (García-Romero et al., 2016; Ryan and Pembroke, 2018; Amaresan et al., 2020; Jahn et al., 2021). Other genera were either obligate aerobes or anaerobes and were found to be specific to the grower diet when the birds had already gained weight. These are Microbacterium, Allisonella, Campylobacter, Rhodococcus, Lysinibacillus, and Mesorhizobium. Furthermore, Oscillospiraceae; UCG-007, Bacteroides, Gallibacterium, Izemoplasmatales, Incertae_Sedis; DTU014, DTU014, DTU014, Romboutsia, Gastranaerophilales, Victivallis, Butyricicoccaceae; UCG-008, Coriobacteriaceae_UCG-002, and Enteroscipio were found to be specific to the finisher diet.
Next, we wanted to see if there was a selection of predictive antimicrobial genes (piARGs) in the observed range. We found that bacterial community structure predicts the growth performance of the broiler, but in parallel, these communities are also contributing to antimicrobial resistance. As we have reported above, Firmicutes are the major phyla found in the gut microbial communities, and we hypothesize that they may be the major contributor of piARGs as depicted earlier (Juricova et al., 2021; Li et al., 2022; Xu et al., 2022). It has been observed in our results that most of the piARGs belonging to aminoglycosides (DG01447) were positively associated with the finisher diet period and also with the majority of the performance parameters, such as body weight. In a similar manner, we also observed that piARGs belonging to carbapenems (DG01458) were negatively associated with the grower/finisher diets and also with performance parameters. When the diet changed from starter to grower on day 11, a slight increase in piARGs was observed, indicating the effect of the feed change. A similar trend was observed on day 26 when the diet was switched to finisher, followed by a slight decrease in the 5th week. In general, the abundance of piAGRs remained stable throughout the experimental period. This is in line with recent studies that piARGs contribution is a continuous process effectuated by microbial communities themselves but is invariably influenced by the use of specific antimicrobials during the growth period (Thomas et al., 2017; Skarżyńska et al., 2020; Li et al., 2022). Since the diets were proprietary and we had no information about which antibiotics were used, with these results, we can only hypothesize that the predicted piARGs give sufficient evidence to suggest the above antibiotics were used. Our results indicate that the abundance of piARGs is influenced by the change in bacterial community structure, which we witnessed on days 11 and 26. It has been reported that the use of antimicrobials during the growth of broilers changes the gut microbial communities and enhances their resistance (Allen and Stanton, 2014; Xiong et al., 2018).
Overall, piARGs (specifically belonging to ß-lactams and aminoglycosides) abundance increased with the age of broilers, which may be linked with the increased microbial richness, as supported by previous studies (Butaye et al., 1999; Ozaki et al., 2011; Temmerman et al., 2022). piARGs belonging to carbapenems (DG01458) are found to decrease with the age of birds and are also negatively associated with the majority of the performance parameters. Our results are in agreement with the studies performed in the broiler in which it has been observed that ARGs decrease with age (Linton et al., 1982; Gaire et al., 2021). Many studies suggest that using antimicrobials as growth promoters or prophylaxis during the growth and production periods ultimately contributes to the resistance against the same antimicrobial class (Luiken et al., 2019; Gupta et al., 2021; De Cesare et al., 2022). As the diet used in this study was proprietary and we were not able to obtain information regarding antibiotic supplementation within the feed, it is not possible to determine whether the ARG enrichment observed with the diet was due to a change in bacterial communities because of protein content variation or due to antibiotic supplementation. However, it has been observed that AGP discontinuation in feed leads to decreased antibiotic selection pressure, and the resultant antibiotic resistance profiles of intestinal bacteria change rapidly (Wang et al., 2020). Antibiotic alternatives in broiler production include phytogenics, organic acids, prebiotics, probiotics, enzymes, and their derivatives. Antibiotic alternatives have been reported to increase feed intake, stimulate digestion, improve feed efficiency, increase growth performance, and reduce the incidence of diseases by modulating the intestinal microbiota and immune system, inhibiting pathogens, and improving intestinal integrity (Ayalew et al., 2022). However, how these interventions modulate the resistance within the chicken gut needs to be explored further.
We also explored how age and different performance parameters contribute to the functional profiles of bacterial communities through the CODA-LASSO regression model on the recovered metacyc pathways. Amino acid biosynthesis pathways such as L-histidine biosynthesis, L-arginine biosynthesis I (via L-ornithine), and the super-pathway of taurine degradation are found to be positively associated with a bird’s age. It has been reported that these amino acids are involved in the growth of the bird, specifically during the early days, and also play an important role in managing stress responses (Chowdhury et al., 2021). Histidine has an effect on the metabolites of various metabolic pathways. L-arginine has a vital role in reducing fat deposition by moderating lipid metabolism (Fouad et al., 2013; Lackner et al., 2022). Taurine affects meat quality by enhancing the anti-oxidative capacity and lipid metabolism, particularly under heat stress (Lu et al., 2019; Xu et al., 2019; Han et al., 2020). Peptidoglycan, coumarins, and some nucleotide biosynthesis/repair pathways are found to be positively associated with the age and growth of the birds, as supported by a few other studies (McFarland and Coon, 2016). As evident from previous studies, peptidoglycan biosynthesis, which is more perfectly enabled by gram-positive than gram-negative bacteria, may also be one cause of the proliferation of piARGs. If a gut has more probiotic bacterial species producing SCFAs such as lactic acid, this will then positively affect the growth by hindering the peptidoglycan biosynthesis in pathogenic bacteria (Lovering et al., 2012; Hidayat et al., 2018; Lee et al., 2022). Furthermore, the coumarins biosynthesis pathway (which increases with age) plays an important role in gut homeostasis. These have antibacterial, antiviral, antifungal, antioxidant, anticancer, and anti-inflammatory activity (El-Far et al., 2016; Duskaev et al., 2021; Sahoo et al., 2021; Cheke et al., 2022). Another important pathway, the tetrapyrrole biosynthesis pathway, that was negatively associated with the age and weight of the broilers was also implicated in earlier studies (Zappa et al., 2010; Plata et al., 2022). On a similar note, foliate transformations and nicotinamide adenine dinucleotide (NAD) biosynthesis are negatively associated with the bird’s age. Similar results regarding the anti-aging effects of NAD are found in other recent studies as well (Johnson and Imai, 2018; Zhang et al., 2020). We have used a predictive functional modeling approach, and there is often a degree of skepticism associated with applying such approaches. However, the majority of our findings were corroborated by published literature, increasing our confidence in the utility of PICRUSt2 to supplement microbial community analyses.
5. Conclusion
Through the use of new in situ analytical tools, we have demonstrated how certain species persisted as stable components of microbial communities by emphasizing periods where diets remained unchanged. In parallel, we have explored the dynamic components of microbial communities, whether they were positively or negatively associated with the performance parameters of interest, or whether they shot up within a specific narrow range. The microbial community dataset, which originated from 12 pens, each with their own idiosyncrasy, was explicitly modeled in the MicroNiche algorithm to mask out any biases associated with the environments and reveal patterns that lead us to harness their true potential. Overall, our findings suggest a route toward improving the performance of the birds by modulating their microbiome and improving their health by highlighting specific parameters such as diet that are associated with microbial communities’ flux. Considering the content estimation of the different diets and looking at what microbes were promoted by the diet (specificity analyses), we should be able to come up with a dietary intervention to modulate the microbiome to optimize some sort of fitness function. A strong association of microbes with FCR, FI, body weight, and weight gain then enables us to design future intervention strategies where the findings of this study can serve as a reference, as well as help us establish a dietary plan that can help us forgo the use of antibiotic growth promoters for enhancing feed conversion.
Data availability statement
The raw sequence files supporting the results of this article are available in the European Nucleotide Archive under the project accession number PRJEB25776.
Ethics statement
The animal study was approved by Agri-Food and Biosciences Institute (AFBI) Establishment License 5002 for AFBI Veterinary Science Division. The study was conducted in accordance with the local legislation and institutional requirements.
Author contributions
AA: data curation, investigation, methodology, writing—original draft, and writing—review and editing. YC: data curation, investigation, methodology, visualisation, validation, and writing—review and editing. FS: data curation, investigation, methodology, writing—original draft, and writing—review and editing. Uzma: data curation, methodology, visualisation, validation, writing—review and editing, and software. AM: investigation, resources, and funding acquisition. AR: resources, funding acquisition, and project administration. OG and WS: writing—review and editing and funding acquisition. SJ: writing—original draft, writing—review and editing, project administration, and supervision. UI: methodology, software, writing—original draft, writing—review and editing, project administration, supervision, and funding acquisition. All authors contributed to the article and approved the submitted version.
Funding
AA acknowledges support from the International Research Support Initiative Program of the Higher Education Commission, Pakistan Project No. 1-8/HEC/HRD/2023/12790. UI is funded by the NERC Independent Research Fellowship (NE/L011956/1). UI and WS are further supported by EPSRC (EP/P029329/1 and EP/V030515/1).
Acknowledgments
The authors would like to thank all the field and laboratory teams of OG and NC who generated the data for this manuscript.
Conflict of interest
AM and AR were employed by Moy Park.
The remaining authors declare that the research was conducted in the absence of any commercial or financial relationships that could be construed as a potential conflict of interest.
Publisher’s note
All claims expressed in this article are solely those of the authors and do not necessarily represent those of their affiliated organizations, or those of the publisher, the editors and the reviewers. Any product that may be evaluated in this article, or claim that may be made by its manufacturer, is not guaranteed or endorsed by the publisher.
Supplementary material
The Supplementary material for this article can be found online at: https://www.frontiersin.org/articles/10.3389/fmicb.2023.1197838/full#supplementary-material
Footnotes
References
Aguirre, M., Eck, A., Koenen, M. E., Savelkoul, P. H. M., Budding, A. E., and Venema, K. (2016). Diet drives quick changes in the metabolic activity and composition of human gut microbiota in a validated in vitro gut model. Res. Microbiol. 167, 114–125. doi: 10.1016/J.RESMIC.2015.09.006
Allen, H. K., and Stanton, T. B. (2014). Altered egos: antibiotic effects on food animal microbiomes. Annu. Rev. Microbiol. 68, 297–315. doi: 10.1146/ANNUREV-MICRO-091213-113052
Allesina, S., and Tang, S. (2012). Stability criteria for complex ecosystems. Nature 483, 205–208. doi: 10.1038/nature10832
Amara, A. A., and Shibl, A. (2015). Role of probiotics in health improvement, infection control and disease treatment and management. Saudi Pharm. J. 23, 107–114. doi: 10.1016/J.JSPS.2013.07.001
Amaresan, N., Kumar, M. S., Annapurna, K., Kumar, K., and Sankaranarayanan, A. (Eds.) (2020). Beneficial microbes in agro-ecology: bacteria and fungi. Academic Press.
Apajalahti, J. H. A., Kettunen, A., Bedford, M. R., and Holben, W. E. (2001). Percent G+C profiling accurately reveals diet-related differences in the gastrointestinal microbial community of broiler chickens. Appl. Environ. Microbiol. 67, 5656–5667. doi: 10.1128/AEM.67.12.5656-5667.2001
Apajalahti, J., Kettunen, A., and Graham, H. (2004). Characteristics of the gastrointestinal microbial communities, with special reference to the chicken. Worlds Poult. Sci. J. 60, 223–232. doi: 10.1079/WPS200415
Ayalew, H., Zhang, H., Wang, J., Wu, S., Qiu, K., Qi, G., et al. (2022). Potential feed additives as antibiotic alternatives in broiler production. Front. Vet. Sci. 9:916473. doi: 10.3389/FVETS.2022.916473
Ballou, A. L., Ali, R. A., Mendoza, M. A., Ellis, J. C., Hassan, H. M., Croom, W. J., et al. (2016). Development of the chick microbiome: how early exposure influences future microbial diversity. Front. Vet. Sci. 3:2. doi: 10.3389/FVETS.2016.00002
Binek, M., Cisek, A. A., Chrobak-Chmiel, M. R. D., Stefańska, I., and Kizerwetter-Świda, M. (2017). Mikrobiom jelitowy kury domowej—Rozwój i funkcja. Med. Weter. 73, 618–625. doi: 10.21521/MW.5790
Borda-Molina, D., Seifert, J., and Camarinha-Silva, A. (2018). Current perspectives of the chicken gastrointestinal tract and its microbiome. Comput. Struct. Biotechnol. J. 16, 131–139. doi: 10.1016/J.CSBJ.2018.03.002
Butaye, P., Devriese, L. A., Goossens, H., Ieven, M., and Haesebrouck, F. (1999). Enterococci with acquired vancomycin resistance in pigs and chickens of different age groups. Antimicrob. Agents Chemother. 43, 365–366. doi: 10.1128/AAC.43.2.365
Caporaso, J. G., Kuczynski, J., Stombaugh, J., Bittinger, K., Bushman, F. D., Costello, E. K., et al. (2010). QIIME allows analysis of high-throughput community sequencing data. Nat. Methods 7, 335–336. doi: 10.1038/NMETH.F.303
Cheke, R. S., Patel, H. M., Patil, V. M., Ansari, I. A., Ambhore, J. P., Shinde, S. D., et al. (2022). Molecular insights into coumarin analogues as antimicrobial agents: recent developments in drug discovery. Antibiotics 11:566. doi: 10.3390/ANTIBIOTICS11050566
Choi, J. H., Kim, G. B., and Cha, C. J. (2014). Spatial heterogeneity and stability of bacterial community in the gastrointestinal tracts of broiler chickens. Poult. Sci. 93, 1942–1950. doi: 10.3382/PS.2014-03974
Chowdhury, V. S., Han, G., Eltahan, H. M., Haraguchi, S., Gilbert, E. R., Cline, M. A., et al. (2021). Potential role of amino acids in the adaptation of chicks and market-age broilers to heat stress. Front. Vet. Sci. 7:1109. doi: 10.3389/FVETS.2020.610541
Clavijo, V., and Flórez, M. J. V. (2018). The gastrointestinal microbiome and its association with the control of pathogens in broiler chicken production: a review. Poult. Sci. 97, 1006–1021. doi: 10.3382/PS/PEX359
Clavijo, V., Morales, T., Vives-Flores, M. J., and Reyes Muñoz, A. (2022). The gut microbiota of chickens in a commercial farm treated with a Salmonella phage cocktail. Sci. Rep. 12, 1–16. doi: 10.1038/s41598-021-04679-6
Crhanova, M., Hradecka, H., Faldynova, M., Matulova, M., Havlickova, H., Sisak, F., et al. (2011). Immune response of chicken gut to natural colonization by gut microflora and to Salmonella enterica serovar enteritidis infection. Infect. Immun. 79, 2755–2763. doi: 10.1128/IAI.01375-10
Cui, Y., Wang, Q., Liu, S., Sun, R., Zhou, Y., and Li, Y. (2017). Age-related variations in intestinal microflora of free-range and caged hens. Front. Microbiol. 8:1310. doi: 10.3389/FMICB.2017.01310
Danzeisen, J. L., Kim, H. B., Isaacson, R. E., Tu, Z. J., and Johnson, T. J. (2011). Modulations of the chicken cecal microbiome and metagenome in response to anticoccidial and growth promoter treatment. PLoS One 6:e27949. doi: 10.1371/JOURNAL.PONE.0027949
Darcy, J. L., Amend, A. S., Swift, S. O. I., Sommers, P. S., and Lozupone, C. A. (2022). Specificity: an R package for analysis of feature specificity to environmental and higher dimensional variables, applied to microbiome species data. Environ. Microbiome 17:34. doi: 10.1186/s40793-022-00426-0
De Cesare, A., Oliveri, C., Lucchi, A., Savini, F., Manfreda, G., and Sala, C. (2022). Pilot study on poultry meat from antibiotic free and conventional farms: can metagenomics detect any difference? Foods 11:249. doi: 10.3390/FOODS11030249/S1
Douglas, G. M., Maffei, V. J., Zaneveld, J. R., Yurgel, S. N., Brown, J. R., Taylor, C. M., et al. (2020). PICRUSt2 for prediction of metagenome functions. Nat. Biotechnol. 38, 685–688. doi: 10.1038/S41587-020-0548-6
Duskaev, G., Kvan, O., Kosyan, D., Rakhmatullin, S., and Levakhin, G. (2021). Coumarin derivative and Bacillus cereus change live weight and cecal ecology in broilers. AIMS Agric. Food 6, 360–380. doi: 10.3934/agrfood.2021022
El-Adawy, H., Bocklisch, H., Neubauer, H., Hafez, H. M., and Hotzel, H. (2018). Identification, differentiation and antibiotic susceptibility of Gallibacterium isolates from diseased poultry. Ir. Vet. J. 71, 1–10. doi: 10.1186/s13620-018-0116-2
El-Deek, A. A., Abdel-Wareth, A. A. A., Osman, M., El-Shafey, M., Khalifah, A. M., Elkomy, A. E., et al. (2020). Alternative feed ingredients in the finisher diets for sustainable broiler production. Sci. Rep. 10, 17743–17749. doi: 10.1038/s41598-020-74950-9
El-Far, A. H., Ahmed, H. A., and Shaheen, H. M. (2016). Dietary supplementation of Phoenix dactylifera seeds enhances performance, immune response, and antioxidant status in broilers. Oxidative Med. Cell. Longev. 2016, 1–9. doi: 10.1155/2016/5454963
FAO (2017). Prospects for food, nutrition, agriculture and major commodity groups. World Agriculture: towards 2030/2050. London: Routledge. Available at: https://www.taylorfrancis.com/books/mono/10.4324/9781315083858/world-agriculture-towards-2015-2030-jelle-bruinsma
Fathima, S., Shanmugasundaram, R., Adams, D., and Selvaraj, R. K. (2022). Gastrointestinal microbiota and their manipulation for improved growth and performance in chickens. Foods 11, 11:1401. doi: 10.3390/FOODS11101401
Feye, K. M., Baxter, M. F. A., Tellez-Isaias, G., Kogut, M. H., and Ricke, S. C. (2020). Influential factors on the composition of the conventionally raised broiler gastrointestinal microbiomes. Poult. Sci. 99, 653–659. doi: 10.1016/J.PSJ.2019.12.013
Finn, D. R., Yu, J., Ilhan, Z. E., Fernandes, V. M. C., Penton, C. R., Krajmalnik-Brown, R., et al. (2020). MicroNiche: an R package for assessing microbial niche breadth and overlap from amplicon sequencing data. FEMS Microbiol. Ecol. 96:131. doi: 10.1093/FEMSEC/FIAA131
Fouad, A. M., El-Senousey, H. K., Yang, X. J., and Yao, J. H. (2013). Dietary L-arginine supplementation reduces abdominal fat content by modulating lipid metabolism in broiler chickens. Animal 7, 1239–1245. doi: 10.1017/S1751731113000347
Fry, J. P., Mailloux, N. A., Love, D. C., Milli, M. C., and Cao, L. (2018). Feed conversion efficiency in aquaculture: do we measure it correctly? Environ. Res. Lett. 13:024017. doi: 10.1088/1748-9326/AAA273
Gaire, T. N., Scott, H. M., Sellers, L., Nagaraja, T. G., and Volkova, V. V. (2021). Age dependence of antimicrobial resistance among fecal bacteria in animals: a scoping review. Front. Vet. Sci. 7:1174. doi: 10.3389/FVETS.2020.622495
García-Romero, I., Pérez-Pulido, A. J., González-Flores, Y. E., Reyes-Ramírez, F., Santero, E., and Floriano, B. (2016). Genomic analysis of the nitrate-respiring Sphingopyxis granuli (formerly Sphingomonas macrogoltabida) strain TFA. BMC Genomics 17, 1–15. doi: 10.1186/S12864-016-2411-1
Goldford, J. E., Lu, N., Bajić, D., Estrela, S., Tikhonov, M., Sanchez-Gorostiaga, A., et al. (2018). Emergent simplicity in microbial community assembly. Science 361, 469–474. doi: 10.1126/science.aat1168
Gong, H., Yang, Z., Celi, P., Yan, L., Ding, X., Bai, S., et al. (2021). Effect of benzoic acid on production performance, egg quality, intestinal morphology, and cecal microbial community of laying hens. Poult. Sci. 100, 196–205. doi: 10.1016/J.PSJ.2020.09.065
Granot, I., Shenkar, N., and Belmaker, J. (2017). Habitat niche breadth predicts invasiveness in solitary ascidians. Ecol. Evol. 7, 7838–7847. doi: 10.1002/ECE3.3351
Gupta, C. L., Blum, S. E., Kattusamy, K., Daniel, T., Druyan, S., Shapira, R., et al. (2021). Longitudinal study on the effects of growth-promoting and therapeutic antibiotics on the dynamics of chicken cloacal and litter microbiomes and resistomes. Microbiome 9, 1–19. doi: 10.1186/S40168-021-01136-4
Hafez, H. M., and Attia, Y. A. (2020). Challenges to the poultry industry: current perspectives and strategic future after the COVID-19 outbreak. Front. Vet. Sci. 7:516. doi: 10.3389/FVETS.2020.00516
Han, H., Sun, Y., Fan, Y., Zhang, H., Yang, J., Chi, R., et al. (2022). Microbial diversity and community composition of duodenum microbiota of high and low egg-yielding Taihang chickens identified using 16S rRNA amplicon sequencing. Life 12:1262. doi: 10.3390/LIFE12081262/S1
Han, H. L., Zhang, J. F., Yan, E. F., Shen, M. M., Wu, J. M., Gan, Z. D., et al. (2020). Effects of taurine on growth performance, antioxidant capacity, and lipid metabolism in broiler chickens. Poult. Sci. 99, 5707–5717. doi: 10.1016/J.PSJ.2020.07.020
Hidayat, M. N., Malaka, R., Agustina, L., and Pakiding, W. (2018). Characteristics isolate bacteria lactic acid of origin digestive tract of broiler as probiotic candidate for poultry. Int. J. Sci. Eng. Res. 9, 1787–1794.
Hiett, K. L., Cox, N. A., and Rothrock, M. J. (2013). Polymerase chain reaction detection of naturally occurring Campylobacter in commercial broiler chicken embryos. Poult. Sci. 92, 1134–1137. doi: 10.3382/PS.2012-02812
Huang, P., Zhang, Y., Xiao, K., Jiang, F., Wang, H., Tang, D., et al. (2018). The chicken gut metagenome and the modulatory effects of plant-derived benzylisoquinoline alkaloids. Microbiome 6:211. doi: 10.1186/s40168-018-0590-5
Ijaz, U. Z., Sivaloganathan, L., McKenna, A., Richmond, A., Kelly, C., Linton, M., et al. (2018). Comprehensive longitudinal microbiome analysis of the chicken cecum reveals a shift from competitive to environmental drivers and a window of opportunity for Campylobacter. Front. Microbiol. 9:2452. doi: 10.3389/FMICB.2018.02452
Jahn, M., Crang, N., Janasch, M., Hober, A., Forsström, B., Kimler, K., et al. (2021). Protein allocation and utilization in the versatile chemolithoautotroph Cupriavidus necator. eLife 10:e69019. doi: 10.7554/ELIFE.69019
Joat, N., Van, T. T. H., Stanley, D., Moore, R. J., and Chousalkar, K. (2021). Temporal dynamics of gut microbiota in caged laying hens: a field observation from hatching to end of lay. Appl. Microbiol. Biotechnol. 105, 4719–4730. doi: 10.1007/s00253-021-11333-8
Johnson, S., and Imai, S. I. (2018). NAD+ biosynthesis, aging, and disease. F1000Res. 7:132. doi: 10.12688/F1000RESEARCH.12120.1
Juricova, H., Matiasovicova, J., Kubasova, T., Cejkova, D., and Rychlik, I. (2021). The distribution of antibiotic resistance genes in chicken gut microbiota commensals. Sci. Rep. 11, 3290–3210. doi: 10.1038/s41598-021-82640-3
Kaakoush, N. O., Sodhi, N., Chenu, J. W., Cox, J. M., Riordan, S. M., and Mitchell, H. M. (2014). The interplay between Campylobacter and Helicobacter species and other gastrointestinal microbiota of commercial broiler chickens. Gut Pathog. 6, 1–10. doi: 10.1186/1757-4749-6-18
Khan, S., and Chousalkar, K. K. (2020). Short-term feeding of probiotics and synbiotics modulates caecal microbiota during Salmonella typhimurium infection but does not reduce shedding and invasion in chickens. Appl. Microbiol. Biotechnol. 104, 319–334. doi: 10.1007/S00253-019-10220-7
Kim, J. Y., Young, J. A., Gunther, N. W., and Lee, J. L. (2015). Inhibition of Salmonella by bacteriocin-producing lactic acid bacteria derived from U.S. kimchi and broiler chicken. J. Food Saf. 35, 1–12. doi: 10.1111/JFS.12141
Kogut, M. H. (2019). The effect of microbiome modulation on the intestinal health of poultry. Anim. Feed Sci. Technol. 250, 32–40. doi: 10.1016/J.ANIFEEDSCI.2018.10.008
Lackner, J., Hess, V., Marx, A., Hosseini-Ghaffari, M., and Sauerwein, H. (2022). Effects of dietary supplementation with histidine and β-alanine on blood plasma metabolome of broiler chickens at different ages. PLoS One 17:e0277476. doi: 10.1371/JOURNAL.PONE.0277476
Lee, T. T., Chou, C. H., Wang, C., Lu, H. Y., and Yang, W. Y. (2022). Bacillus amyloliquefaciens and Saccharomyces cerevisiae feed supplements improve growth performance and gut mucosal architecture with modulations on cecal microbiota in red-feathered native chickens. Anim. Biosci. 35, 869–883. doi: 10.5713/AB.21.0318
Lee, S. W., La, T. M., Lee, H. J., Choi, I. S., Song, C. S., Park, S. Y., et al. (2019). Characterization of microbial communities in the chicken oviduct and the origin of chicken embryo gut microbiota. Sci. Rep. 9, 6838–6811. doi: 10.1038/s41598-019-43280-w
Li, M. H., Meng, J. X., Wang, W., He, M., Zhao, Z. Y., Ma, N., et al. (2022). Dynamic description of temporal changes of gut microbiota in broilers. Poult. Sci. 101:102037. doi: 10.1016/J.PSJ.2022.102037
Linton, A. H., Chalaby, Z. A. M., and Allen, V. (1982). The persistence of drug resistant Escherichia coli in the intestinal flora of healthy broiler chicks. J. Hyg. 89, 269–278. doi: 10.1017/S0022172400070807
Lovering, A. L., Safadi, S. S., and Strynadka, N. C. J. (2012). Structural perspective of peptidoglycan biosynthesis and assembly. Annu. Rev. Biochem. 81, 451–478. doi: 10.1146/ANNUREV-BIOCHEM-061809-112742
Lu, Z., He, X. F., Ma, B. B., Zhang, L., Li, J. L., Jiang, Y., et al. (2019). The alleviative effects and related mechanisms of taurine supplementation on growth performance and carcass characteristics in broilers exposed to chronic heat stress. Poult. Sci. 98, 878–886. doi: 10.3382/PS/PEY433
Lu, J., Idris, U., Harmon, B., Hofacre, C., Maurer, J. J., and Lee, M. D. (2003). Diversity and succession of the intestinal bacterial community of the maturing broiler chicken. Appl. Environ. Microbiol. 69, 6816–6824. doi: 10.1128/AEM.69.11.6816-6824.2003
Luiken, R. E. C., Van Gompel, L., Munk, P., Sarrazin, S., Joosten, P., Dorado-García, A., et al. (2019). Associations between antimicrobial use and the faecal resistome on broiler farms from nine European countries. J. Antimicrob. Chemother. 74, 2596–2604. doi: 10.1093/JAC/DKZ235
Lynch, M. D. J., and Neufeld, J. D. (2015). Ecology and exploration of the rare biosphere. Nat. Rev. Microbiol. 13, 217–229. doi: 10.1038/NRMICRO3400
Macfarlane, G. T., Gibson, G. R., Beatty, E., and Cummings, J. H. (1992). Estimation of short-chain fatty acid production from protein by human intestinal bacteria based on branched-chain fatty acid measurements. FEMS Microbiol. Ecol. 10, 81–88. doi: 10.1111/J.1574-6941.1992.TB00002.X
Mandal, R. K., Jiang, T., Wideman, R. F., Lohrmann, T., and Kwon, Y. M. (2020). Microbiota analysis of chickens raised under stressed conditions. Front. Vet. Sci. 7:696. doi: 10.3389/FVETS.2020.482637
McFarland, D. C., and Coon, C. N. (2016). Purine metabolism in high- and low-uric acid lines of chickens: hypoxanthine/guanine phosphoribosyltransferase activities. Proc. Soc. Exp. Biol. Med. 173, 41–47. doi: 10.3181/00379727-173-41607
McKenna, A., Ijaz, U. Z., Kelly, C., Linton, M., Sloan, W. T., Green, B. D., et al. (2020). Impact of industrial production system parameters on chicken microbiomes: mechanisms to improve performance and reduce Campylobacter. Microbiome 8:128. doi: 10.1186/S40168-020-00908-8
Mohd Shaufi, M. A., Sieo, C. C., Chong, C. W., Gan, H. M., and Ho, Y. W. (2015). Deciphering chicken gut microbial dynamics based on high-throughput 16S rRNA metagenomics analyses. Gut Pathog. 7, 1–12. doi: 10.1186/S13099-015-0051-7
Ngunjiri, J. M., Taylor, K. J. M., Abundo, M. C., Jang, H., Elaish, M., Mahesh, K. C., et al. (2019). Farm stage, bird age, and body site dominantly affect the quantity, taxonomic composition, and dynamics of respiratory and gut microbiota of commercial layer chickens. Appl. Environ. Microbiol. 85:e03137. doi: 10.1128/AEM.03137-18
Niku, J., Hui, F. K. C., Taskinen, S., and Warton, D. I. (2019). gllvm: fast analysis of multivariate abundance data with generalized linear latent variable models in R. Methods Ecol. Evol. 10, 2173–2182. doi: 10.1111/2041-210X.13303
Nurmi, E., and Rantala, M. (1973). New aspects of Salmonella infection in broiler production. Nature 241, 210–211. doi: 10.1038/241210a0
Oakley, B. B., Buhr, R. J., Ritz, C. W., Kiepper, B. H., Berrang, M. E., Seal, B. S., et al. (2014). Successional changes in the chicken cecal microbiome during 42 days of growth are independent of organic acid feed additives. BMC Vet. Res. 10, 1–8. doi: 10.1186/S12917-014-0282-8
Oakley, B. B., and Kogut, M. H. (2016). Spatial and temporal changes in the broiler chicken cecal and fecal microbiomes and correlations of bacterial taxa with cytokine gene expression. Front. Vet. Sci. 3:11. doi: 10.3389/FVETS.2016.00011
Ozaki, H., Esaki, H., Takemoto, K., Ikeda, A., Nakatani, Y., Someya, A., et al. (2011). Antimicrobial resistance in fecal Escherichia coli isolated from growing chickens on commercial broiler farms. Vet. Microbiol. 150, 132–139. doi: 10.1016/J.VETMIC.2010.12.020
Palmer, C., Bik, E. M., DiGiulio, D. B., Relman, D. A., and Brown, P. O. (2007). Development of the human infant intestinal microbiota. PLoS Biol. 5:e177. doi: 10.1371/JOURNAL.PBIO.0050177
Park, S. H., Perrotta, A., Hanning, I., Diaz-Sanchez, S., Pendleton, S., Alm, E., et al. (2017). Pasture flock chicken cecal microbiome responses to prebiotics and plum fiber feed amendments. Poult. Sci. 96, 1820–1830. doi: 10.3382/PS/PEW441
Perić, L., Milošević, N., Žikić, D., Bjedov, S., Cvetković, D., Markov, S., et al. (2010). Effects of probiotic and phytogenic products on performance, gut morphology and cecal microflora of broiler chickens. Arch. Anim. Breed. 53, 350–359. doi: 10.5194/AAB-53-350-2010
Plata, G., Baxter, N. T., Susanti, D., Volland-Munson, A., Gangaiah, D., Nagireddy, A., et al. (2022). Growth promotion and antibiotic induced metabolic shifts in the chicken gut microbiome. Commun. Biol. 5, 293–214. doi: 10.1038/s42003-022-03239-6
Polansky, O., Sekelova, Z., Faldynova, M., Sebkova, A., Sisak, F., and Rychlik, I. (2016). Important metabolic pathways and biological processes expressed by chicken cecal microbiota. Appl. Environ. Microbiol. 82, 1569–1576. doi: 10.1128/AEM.03473-15
Pourabedin, M., Guan, L., and Zhao, X. (2015). Xylo-oligosaccharides and virginiamycin differentially modulate gut microbial composition in chickens. Microbiome 3, 1–12. doi: 10.1186/S40168-015-0079-4
Proszkowiec-Weglarz, M., Miska, K. B., Ellestad, L. E., Schreier, L. L., Kahl, S., Darwish, N., et al. (2022). Delayed access to feed early post-hatch affects the development and maturation of gastrointestinal tract microbiota in broiler chickens. BMC Microbiol. 22, 1–20. doi: 10.1186/S12866-022-02619-6
Quast, C., Pruesse, E., Yilmaz, P., Gerken, J., Schweer, T., Yarza, P., et al. (2012). The SILVA ribosomal RNA gene database project: improved data processing and web-based tools. Nucleic Acids Res. 41, D590–D596. doi: 10.1093/NAR/GKS1219
R Core Team (2013). R: a language and environment for statistical computing (2014). R Foundation for Statistical Computing. Vienna, Austria.
Ranjitkar, S., Lawley, B., Tannock, G., and Engberg, R. M. (2016). Bacterial succession in the broiler gastrointestinal tract. Appl. Environ. Microbiol. 82, 2399–2410. doi: 10.1128/AEM.02549-15
Rehman, H. U., Vahjen, W., Awad, W. A., and Zentek, J. (2007). Indigenous bacteria and bacterial metabolic products in the gastrointestinal tract of broiler chickens. Arch. Anim. Nutr. 61, 319–335. doi: 10.1080/17450390701556817
Ryan, M. P., and Pembroke, J. T. (2018). Brevundimonas spp: emerging global opportunistic pathogens. Virulence 9, 480–493. doi: 10.1080/21505594.2017.1419116
Sahoo, C. R., Sahoo, J., Mahapatra, M., Lenka, D., Kumar Sahu, P., Dehury, B., et al. (2021). Coumarin derivatives as promising antibacterial agent(s). Arab. J. Chem. 14:102922. doi: 10.1016/J.ARABJC.2020.102922
Sarangi, N. R., Babu, L. K., Kumar, A., Pradhan, C. R., Pati, P. K., and Mishra, J. P. (2016). Effect of dietary supplementation of prebiotic, probiotic, and synbiotic on growth performance and carcass characteristics of broiler chickens. Vet. World 9, 313–319. doi: 10.14202/VETWORLD.2016.313-319
Shan, X., Goyal, A., Gregor, R., and Cordero, O. X. (2022). Annotation-free discovery of functional groups in microbial communities. Nat. Ecol. Evol. 7:716. doi: 10.1038/s41559-023-02021-z
Skarżyńska, M., Leekitcharoenphon, P., Hendriksen, R. S., Aarestrup, F. M., and Wasyl, D. (2020). A metagenomic glimpse into the gut of wild and domestic animals: quantification of antimicrobial resistance and more. PLoS One 15:e0242987. doi: 10.1371/journal.pone.0242987
Smith, E. A., and Macfarlane, G. T. (1998). Enumeration of amino acid fermenting bacteria in the human large intestine: effects of pH and starch on peptide metabolism and dissimilation of amino acids. FEMS Microbiol. Ecol. 25, 355–368. doi: 10.1016/S0168-6496(98)00004-X
Stanley, D., Denman, S. E., Hughes, R. J., Geier, M. S., Crowley, T. M., Chen, H., et al. (2012). Intestinal microbiota associated with differential feed conversion efficiency in chickens. Appl. Microbiol. Biotechnol. 96, 1361–1369. doi: 10.1007/S00253-011-3847-5
Stanley, D., Hughes, R. J., and Moore, R. J. (2014). Microbiota of the chicken gastrointestinal tract: influence on health, productivity and disease. Appl. Microbiol. Biotechnol. 98, 4301–4310. doi: 10.1007/S00253-014-5646-2
Sztandarski, P., Marchewka, J., Konieczka, P., Zdanowska-Sąsiadek, Ż., Damaziak, K., Riber, A. B., et al. (2022). Gut microbiota activity in chickens from two genetic lines and with outdoor-preferring, moderate-preferring, and indoor-preferring ranging profiles. Poult. Sci. 101:102039. doi: 10.1016/J.PSJ.2022.102039
Temmerman, R., Ghanbari, M., Antonissen, G., Schatzmayr, G., Duchateau, L., Haesebrouck, F., et al. (2022). Dose-dependent impact of enrofloxacin on broiler chicken gut resistome is mitigated by synbiotic application. Front. Microbiol. 13:2862. doi: 10.3389/FMICB.2022.869538
Thomas, M., Webb, M., Ghimire, S., Blair, A., Olson, K., Fenske, G. J., et al. (2017). Metagenomic characterization of the effect of feed additives on the gut microbiome and antibiotic resistome of feedlot cattle. Sci. Rep. 7, 12257–12213. doi: 10.1038/s41598-017-12481-6
van der Wielen, P. W. J. J., Keuzenkamp, D. A., Lipman, L. J. A., Van Knapen, F., and Biesterveld, S. (2002). Spatial and temporal variation of the intestinal bacterial community in commercially raised broiler chickens during growth. Microb. Ecol. 44, 286–293. doi: 10.1007/S00248-002-2015-Y
Venegas, D. P., De La Fuente, M. K., Landskron, G., González, M. J., Quera, R., Dijkstra, G., et al. (2019). Short chain fatty acids (SCFAs)mediated gut epithelial and immune regulation and its relevance for inflammatory bowel diseases. Front. Immunol. 10:277. doi: 10.3389/FIMMU.2019.00277
Verster, A. J., and Borenstein, E. (2018). Competitive lottery-based assembly of selected clades in the human gut microbiome. Microbiome 6, 1–17. doi: 10.1186/S40168-018-0571-8
Wang, L., Lilburn, M., and Yu, Z. (2016). Intestinal microbiota of broiler chickens as affected by litter management regimens. Front. Microbiol. 7:593. doi: 10.3389/FMICB.2016.00593
Wang, Y., Sun, J., Zhong, H., Li, N., Xu, H., Zhu, Q., et al. (2017). Effect of probiotics on the meat flavour and gut microbiota of chicken. Sci. Rep. 7, 6400–6413. doi: 10.1038/s41598-017-06677-z
Wang, Y., Xu, C., Zhang, R., Chen, Y., Shen, Y., Hu, F., et al. (2020). Changes in colistin resistance and mcr-1 abundance in Escherichia coli of animal and human origins following the ban of colistin-positive additives in China: an epidemiological comparative study. Lancet Infect. Dis. 20, 1161–1171. doi: 10.1016/S1473-3099(20)30149-3
Xiao, S. S., Mi, J. D., Mei, L., Liang, J., Feng, K. X., Wu, Y. B., et al. (2021). Microbial diversity and community variation in the intestines of layer chickens. Animals 11, 1–17. doi: 10.3390/ANI11030840
Xiao, Y., Xiang, Y., Zhou, W., Chen, J., Li, K., and Yang, H. (2017). Microbial community mapping in intestinal tract of broiler chicken. Poult. Sci. 96, 1387–1393. doi: 10.3382/PS/PEW372
Xiong, W., Wang, Y., Sun, Y., Ma, L., Zeng, Q., Jiang, X., et al. (2018). Antibiotic-mediated changes in the fecal microbiome of broiler chickens define the incidence of antibiotic resistance genes. Microbiome 6:34. doi: 10.1186/S40168-018-0419-2
Xu, Y., Huang, Y., Guo, L., Zhang, S., Wu, R., Fang, X., et al. (2022). Metagenomic analysis reveals the microbiome and antibiotic resistance genes in indigenous Chinese yellow-feathered chickens. Front. Microbiol. 13:3501. doi: 10.3389/FMICB.2022.930289
Xu, S. W., Lu, Z., Ma, B. B., Xing, T., Li, J. L., Zhang, L., et al. (2019). Dietary taurine supplementation enhances antioxidative capacity and improves breast meat quality of broiler chickens. Br. Poult. Sci. 61, 140–145. doi: 10.1080/00071668.2019.1691147
Yan, W., Sun, C., Yuan, J., and Yang, N. (2017). Gut metagenomic analysis reveals prominent roles of Lactobacillus and cecal microbiota in chicken feed efficiency. Sci. Rep. 7, 1–11. doi: 10.1038/srep45308
Yeoman, C. J., Chia, N., Jeraldo, P., Sipos, M., Goldenfeld, N. D., and White, B. A. (2012). The microbiome of the chicken gastrointestinal tract. Anim. Health Res. Rev. 13, 89–99. doi: 10.1017/S1466252312000138
Yerpes, M., Llonch, P., and Manteca, X. (2020). Factors associated with cumulative first-week mortality in broiler chicks. Animals 10, 10:310. doi: 10.3390/ANI10020310
Yonatan, Y., Amit, G., Friedman, J., and Bashan, A. (2022). Complexity-stability trade-off in empirical microbial ecosystems. Nat. Ecol. Evol. 6, 693–700. doi: 10.1038/s41559-022-01745-8
Zappa, S., Li, K., and Bauer, C. E. (2010). The tetrapyrrole biosynthetic pathway and its regulation in Rhodobacter capsulatus. Adv. Exp. Med. Biol. 675:229. doi: 10.1007/978-1-4419-1528-3_13
Zhang, Y., Jing, W., Zhang, N., Hao, J., and Xing, J. (2020). Effect of maternal folate deficiency on growth performance, slaughter performance, and serum parameters of broiler offspring. J. Poult. Sci. 57, 270–276. doi: 10.2141/JPSA.0190125
Zhu, X. Y., Zhong, T., Pandya, Y., and Joerger, R. D. (2002). 16S rRNA-based analysis of microbiota from the cecum of broiler chickens. Appl. Environ. Microbiol. 68, 124–137. doi: 10.1128/AEM.68.1.124-137.2002
Zou, A., Nadeau, K., Xiong, X., Wang, P. W., Copeland, J. K., Lee, J. Y., et al. (2022). Systematic profiling of the chicken gut microbiome reveals dietary supplementation with antibiotics alters expression of multiple microbial pathways with minimal impact on community structure. Microbiome 10:127. doi: 10.1186/S40168-022-01319-7
Keywords: 16SrRNA gene sequencing, microbiota, stability, assembly, broiler
Citation: Ameer A, Cheng Y, Saleem F, Uzma, McKenna A, Richmond A, Gundogdu O, Sloan WT, Javed S and Ijaz UZ (2023) Temporal stability and community assembly mechanisms in healthy broiler cecum. Front. Microbiol. 14:1197838. doi: 10.3389/fmicb.2023.1197838
Edited by:
Leonardo Erijman, National Scientific and Technical Research Council (CONICET), ArgentinaReviewed by:
Tarique Hussain, Nuclear Institute for Agriculture and Biology, PakistanSarah C. Pearce, United States Department of Agriculture, United States
Copyright © 2023 Ameer, Cheng, Saleem, Uzma, McKenna, Richmond, Gundogdu, Sloan, Javed and Ijaz. This is an open-access article distributed under the terms of the Creative Commons Attribution License (CC BY). The use, distribution or reproduction in other forums is permitted, provided the original author(s) and the copyright owner(s) are credited and that the original publication in this journal is cited, in accordance with accepted academic practice. No use, distribution or reproduction is permitted which does not comply with these terms.
*Correspondence: Sundus Javed, sundus.javed@comsats.edu.pk; Umer Zeeshan Ijaz, umer.ijaz@glasgow.ac.uk
†These authors have contributed equally to this work and share first authorship