- 1School of Pharmacy and Biomedical Sciences, Faculty of Health Sciences, Curtin University, Bentley, WA, Australia
- 2Division of Pharmacy, Faculty of Medicine, University of Pristina, Prishtina, Kosovo, Albania
Background: Pain in dementia is predominant particularly in the advanced stages or in those who are unable to verbalize. Uncontrolled pain alters the course of behaviors in patients with dementia making them perturbed, unsettled, and devitalized. Current measures of assessing pain in this population group are inadequate and underutilized in clinical practice because they lack systematic evaluation and innovative design.
Objective: To describe a novel method and system of pain assessment using a combination of technologies: automated facial recognition and analysis (AFRA), smart computing, affective computing, and cloud computing (Internet of Things) for people with advanced dementia.
Methods and Results: Cognification and affective computing were used to conceptualize the system. A computerized clinical system was developed to address the challenging problem of identifying pain in non-verbal patients with dementia. The system is composed of a smart device enabled app (App) linked to a web admin portal (WAP). The App “PainChek™” uses AFRA to identify facial action units indicative of pain presence, and user-fed clinical information to calculate a pain intensity score. The App has various functionalities including: pain assessment, pain monitoring, patient profiling, and data synchronization (into the WAP). The WAP serves as a database that collects the data obtained through the App in the clinical setting. These technologies can assist in addressing the various characteristics of pain (e.g., subjectivity, multidimensionality, and dynamicity). With over 750 paired assessments conducted, the App has been validated in two clinical studies (n = 74, age: 60–98 y), which showed sound psychometric properties: excellent concurrent validity (r = 0.882–0.911), interrater reliability (Kw = 0.74–0.86), internal consistency (α = 0.925–0.950), and excellent test-retest reliability (ICC = 0.904), while it possesses good predictive validity and discriminant validity. Clinimetric data revealed high accuracy (95.0%), sensitivity (96.1%), and specificity (91.4%) as well as excellent clinical utility (0.95).
Conclusions: PainChek™ is a comprehensive and evidence-based pain management system. This novel approach has the potential to transform pain assessment in people who are unable to verbalize because it can be used by clinicians and carers in everyday clinical practice.
Introduction
In 2017, there are an estimated 962 million people aged 60 or over in the world, comprising 13% of the global population (United Nations Department of Economic Social Affairs Population Division, 2017). This number is projected to increase to 1.4 billion in 2030 and 2.1 billion in 2050, and could rise to 3.1 billion in 2100 (United Nations Department of Economic Social Affairs Population Division, 2017). The incidence of dementia doubles beyond the age of 65 and becomes prevalent by up to 50% over the age of 85 (Duthey, 2013). Currently, there are 50 million people living with the condition worldwide (ADI, 2017; WHO, 2017). Dementia is a clinical neurodegenerative syndrome characterized by progressively impaired cognition, communication (including pain self-reporting), and comprehension as well as the lack of ability to execute simple daily activities (Mitchell et al., 2009; WHO, 2017). Pain is common (up to 80%) in people with dementia but it often goes undetected and untreated, particularly in those who cannot verbalize or express their needs (Hadjistavropoulos et al., 2014). Uncontrolled pain alters the course of behaviors in patients with dementia making them perturbed, unsettled, and devitalized (Hadjistavropoulos et al., 2014).
Despite the availability of more than 35 observational-behavioral pain assessment tools for adults with communication difficulties (Hadjistavropoulos et al., 2014), including those with advanced dementia, none are currently approved by any regulatory agency, such as the United States' Food and Drug Administration (FDA), Australia's Therapeutic Goods Administration (TGA), and European Conformity (CE) mark. As of July 2017, the electronic Pain Assessment Tool (ePAT) [now known as PainChek™] received regulatory clearance as a Class 1 medical device for pain assessment and monitoring in adults who cannot verbalize (e.g., those with dementia) from the TGA and CE marking (ARTG, 2017). The mobile application (App) as a medical device has also been approved for use in other non-verbal adult populations such as those with other neurodegenerative disorders, intellectual disability, traumatic brain injury, aphasia, those receiving palliative care, and post stroke patients (ARTG, 2017). Further, none of the existing tools has their own electronic database, which collects data in real time. This is an important feature of clinical tools because it identifies the need for therapeutic intervention(s) in a timely fashion, which if successful lead(s) to improvement in patient outcomes. To achieve this goal we have developed an online secure portal linked to the tool App that can be accessed through various computing devices (e.g., PC, smart tablet, smartphone).
Current pain assessment tools in dementia also lack the innovative design and advanced technological characteristics. In a large meta-review of pain assessment tools in dementia Lichtner et al. (2014), argue the need for new tools that contain innovative characteristics to be able to transform the process of pain assessment in non-verbal older adults with dementia.
This note aims to describe a novel system called PainChek™ focusing on its conceptual foundation, clinical and technical contents, clinical use, and practical tips for use in clinical settings.
Methods and Results
Conceptual Foundation of PainChek™ System (Figure 1)
In designing the PainChek™ system, the following conceptualizations were considered:
1. The subjective nature of pain i.e. individualized experience of pain as per its definition by the International Association for the Study of Pain (IASP) (Merskey and Bogduk, 1994).
2. The multi-dimensionality, complexity, and dynamicity of pain as a construct (Merskey and Bogduk, 1994).
3. The American Geriatric Society (AGS) Indicators of Persistent Pain were selected as a basic framework to enrich comprehensiveness and to meet the objectives of the tool (AGS Panel on Persistent Pain in Older Persons, 2002).
4. The temporality of pain and related behaviors, so that trends and patterns of pain scores provide a comprehensive clinical picture of the patient under assessment.
5. Objective description of key pain behaviors, such as Facial Action Coding System (FACS)—pain relevant expressions (Ekman et al., 1978).
6. Items sensitive to the presence and intensity of pain were selected on the basis of current evidence (clinical guidelines, previous studies, and other pain assessment tools in dementia).
7. Simple scoring mechanism. For clinicians and carers, it is difficult and highly subjective to make a distinction between whether a patient has mild, moderate or severe pain-related behaviors (Flaherty, 1996). We adopted binary scoring in the PainChek™ pain scale, because such mathematical basis is more predictive of event outcomes and less prone to error than ordinal rating (Ridley, 2002). These criteria are also linked to improved accuracy (Ridley, 2002).
8. Innovative technologies were considered in developing the system. Cognification and affective computing were conceptualized as a model in designing the App to provide a synergistic effect on the use of the tool (Kelly, 2016). Cognification integrates artificial (emotional) intelligence (AI) or affective computing e.g., automated facial recognition and analysis (AFRA), smart computing, and “Internet of Things” (IoT). Automation was integrated because the FACS requires lengthy training, and a certified skilled observer (coder), which render its use in clinical settings impractical (Craig et al., 2011). Smart device technology was selected because they are mobile, miniaturized, cost-efficient, easy to use, have high processing power, and they allow interoperability. IoT and cloud computing allow data management in real time, and transfer of data among different networks. Further, the App does not need to be connected to the internet while in use. A glossary of terminology used in the technical characteristics of the PainChek™ is presented in Table 1.
Rationale for PainChek™ Development
There are various perspectives as to why we have an urgent need to develop a valid, reliable, and more objective pain assessment system for people with dementia. From the patient's perspective, dementia limits their verbal and cognitive abilities to report the presence, nature, location, and/or intensity of pain. These impairments combined with subjective assessment of pain by health professionals and carers are primarily responsible for the failure to identify pain in this group. This then leads to adverse health outcomes, such as behavioral disturbances, use of inappropriate medications, poor quality of life, and premature death (Schneider et al., 2005; Holmes et al., 2008). From the carer's perspective, care is less burdensome when pain related-behavioral problems are well managed. There are also many potential benefits from proper pain management to the organization, which include staff efficiency and productivity, staff retention, and better quality of care for their patients.
The PainChek™ System
The PainChek™ system is a software system which is comprised of the following components:
a) Mobile Application (App)
b) Web Admin Portal (WAP)
PainChek™ is intended to be used to assess and monitor pain in people who cannot verbalize such as people with dementia or communication difficulties (ARTG, 2017).
A glossary of terminology used to describe the psychometric and clinimetric properties of pain assessment tools is displayed in Table 2. A comprehensive account of the clinical and technical characteristics of the PainChek™ system is described in Table 3.
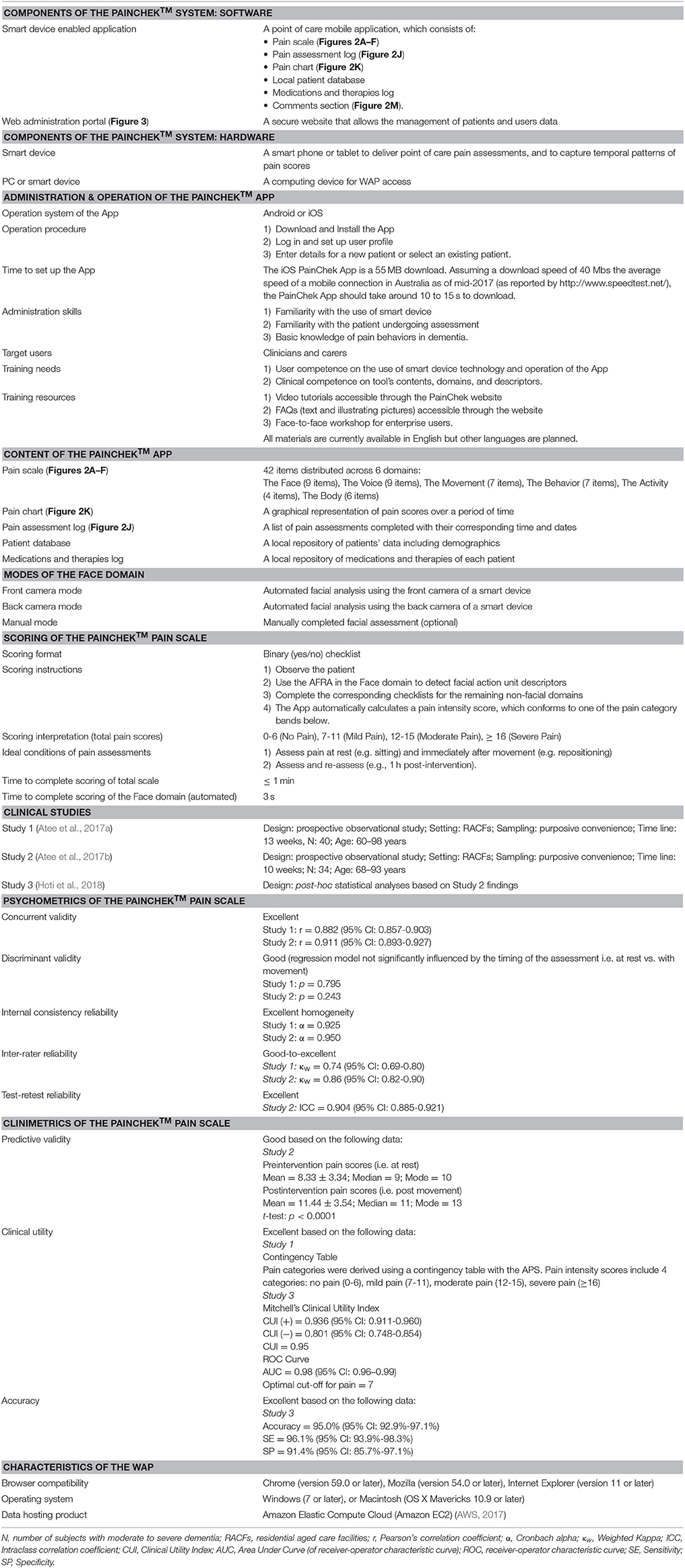
Table 2. Glossary of terms used to describe psychometric and clinimetric properties of pain assessment tools.
The PainChek™ App
PainChek™ is a point of care software application (App) that is compatible with Android and iOS smart devices. The tool uses automated facial recognition technology in real time to identify nine facial micro-expressions called action units (AUs), which are derived from the FACS (Atee et al., 2017a). These facial AUs are validated indicators of the presence of pain (Prkachin, 1992, 2009; Prkachin and Solomon, 2008; Craig et al., 2011; Kunz, 2014; Kunz and Lautenbacher, 2014). These data are then combined with other non-facial pain cues (also known as communicative and protective pain behaviors) such as vocalizations, movements, and behaviors inputted by the user to automatically calculate a pain severity score (Atee et al., 2017a). The App includes a number of components, which are outlined in Table 3.
The PainChek™ pain scale is composed of 42 items distributed across six domains (Table 3; Atee et al., 2017a). Using the smart-device camera to capture a short video of a person's face, the App automatically identifies the face in real time, then maps the face to analyze facial expressions (using a built-in AI algorithm) indicative of the presence of pain. This step provides a score for Domain 1. The user of the App then completes the checklists in Domains 2–6, to give rise a numerical pain score which fits one of the following categories: no pain (0–6), mild pain, (7–11), moderate pain (12–15), or severe pain (≥16) (Atee et al., 2017a).
The PainChek™ App (Figures 2A–M) is commercially available through PainChek Ltd, and is demonstrated in this animated video: https://www.youtube.com/watch?v=rGhX91ZEX9w.
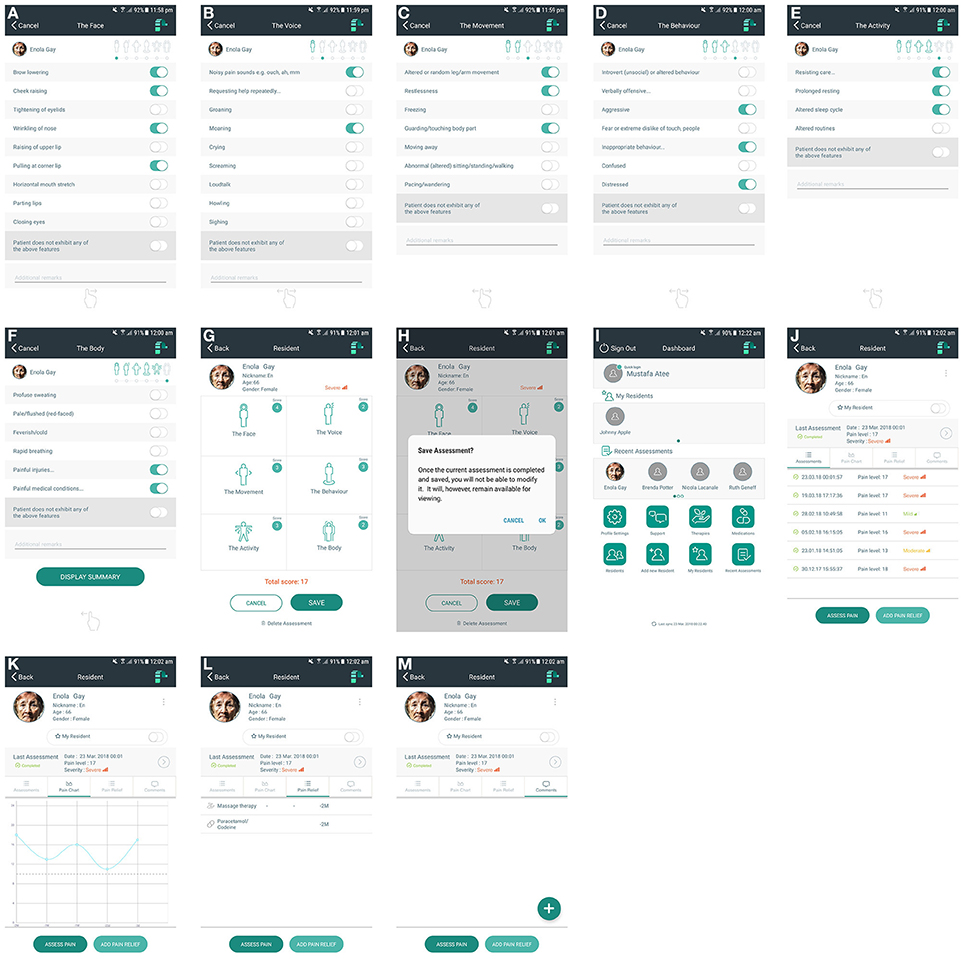
Figure 2. (A) PainChek™ pain assessment tool-The Face (Domain 1). (B) PainChek™ pain assessment tool-The Voice (Domain 2). (C) PainChek™ pain assessment tool-The Movement (Domain 3). (D) PainChek™ pain assessment tool-The Behavior (Domain 4). (E) PainChek™ pain assessment tool-The Activity (Domain 5). (F) PainChek™ pain assessment tool-The Body (Domain 6). (G) PainChek™ pain assessment tool-Summary screen. (H) PainChek™ pain assessment tool–Saving assessment. (I) PainChek™ App—“Dashboard” screen. (J) PainChek™ App—“Assessments” log. (K) PainChek™ App—“Pain Chart.” (L) PainChek™ App—“Pain Relief” list. (M) PainChek™ App—“Comments” section.
The Web Administration Portal (WAP)
The PainChek™ Web Administration Portal is a secure website that allows administrators to manage patient data, and activate new users. The WAP is a cloud hosted web application that can be accessed via a dedicated URL using any of the following internet browsers: Chrome (version 59.0 or later), Mozilla (version 54.0 or later), Internet Explorer (version 11 or later). The WAP of PainChek™ is supported through the operating systems of Windows (7 or later), or Macintosh (OS X Mavericks 10.9 or later). The portal is currently hosted on Amazon Webs Services using the Amazon Elastic Compute Cloud (Amazon EC2) (AWS, 2017). Figure 3 illustrates a screenshot of the WAP. Characteristics of the WAP are also summarized in Table 3.
Clinical Studies
To date, three studies about the PainChek™ App have been published (Table 3; Atee et al., 2017a,b; Hoti et al., 2018). In blind comparisons with the Abbey Pain Scale, PainChek™ has been clinically evaluated in aged care residents with moderate to severe dementia in two prospective observational studies (Atee et al., 2017a,b). The third study provided a comprehensive clinimetric analysis on the performance of the App (Hoti et al., 2018).
Study 1: Pain Assessment in Dementia: Evaluation of a Point-of-Care Technological Solution (Atee et al., 2017a).
In this study, Atee el al. provided an account of the description, content, and conceptual synthesis as well as the psychometric properties of the PainChek™. The App was tested in 40 residents, who underwent 353 paired assessments during rest (n = 209) and movement (n = 144). PainChek™ was demonstrated to have excellent concurrent validity and internal consistency, together with good interrater reliability and discriminant validity (Table 3; Atee et al., 2017a).
Study 2: Psychometric evaluation of the Electronic Pain Assessment Tool (ePAT): An Innovative Instrument for Individuals With Moderate-to-Severe Dementia (Atee et al., 2017b).
Based on 400 paired assessments, the psychometric properties of the tool were further examined in 34 geriatric residents. Again, the App demonstrated strong psychometric properties: excellent concurrent validity, interrater reliability, internal consistency, and excellent test-retest reliability. Discriminant validity and predictive validity were both good (Table 3) (Atee et al., 2017b).
Study 3: Clinimetric Properties of the Electronic Pain Assessment Tool (ePAT) for Aged-Care Residents With Moderate to Severe Dementia (Hoti et al., 2018).
Hoti et al. further analyzed Study 2 data to confirm the cut-off scores, and predictive validity of the tool, whilst also reporting on its clinical utility. Using the ROC curve methodology, the cut-off points for presence of pain was confirmed to be ≥ 7. The study demonstrated the high accuracy, sensitivity and specificity of the App in detecting pain in individuals with dementia. It also demonstrated the excellent clinical utility of the App for pain screening and case finding, as indicated by Mitchell's Index (Table 3) (Hoti et al., 2018).
Clinical Guide and Training on the Use of PainChek™
A wide variety of training resources have been developed to assist users with the operation of PainChek™. Resources include face-to-face training, and web-based materials.
The face-to-face training is available for institutional users. The training is a 2.5 h program which comprises of four sessions: (1) pain associated behaviors in people with dementia, (2) pain assessment in people with dementia, (3) PainChek™ (e.g., contents, scoring, and administration), and (4) practical training and clinical shadowing. The program consists of video and written materials, which have been prepared or collated by clinical researchers (who developed the PainChek™ system, and experienced in the area of pain and dementia). The video clips cover a wide range of pain relevant materials including AUs typically displayed during pain (simulated). Similarly, a number of facial images of people in pain and clinical cases are also used during the training. A two-page clinical guide handout (Figure 4), explaining the content, domains, corresponding descriptors and recommended time frame of observation is also available to the trained users. A clinical facilitator experienced on the use of the system is responsible for running the training program.
For each patient, it is essential that pain assessments are carried out during rest first. This is in order to determine baseline scores for benchmarking purposes, which then followed by post movement assessment to capture the nociceptive experience. Kinesthetic activities such as bending and walking are part of daily living. These activities involve joint activity, which generates nociceptive signals, encoding the sensory aspect of pain phenomenon (Breivik et al., 2008).
The web-based resources (https://www.painchek.com/) include user guides, video materials, and frequently asked questions. All training resources are currently available in English language, although translation to other languages is planned.
Discussion
This article reports on an innovative pain assessment system that includes a point of care App and WAP. The PainChek™ App (Figure 2) is a newly TGA-approved and CE marked medical device for pain assessment in non-verbal adult populations (ARTG, 2017). The App is linked to WAP (Figure 3) to allow capturing of data collected in the clinical setting.
The conceptual model around which the PainChek™ was developed is multifaceted (Figure 1). This model entails subjectivity, multi-dimensionality, and dynamicity of pain as a construct, objectivity, and comprehensiveness of pain behaviors included in the tool, simple scoring, and administration procedure of the pain scale, as well as technological advancements and innovative characteristics of the platform used. Of particular importance, AFRA were used for the first time in a smart device enabled tool for pain assessment targeted at people with dementia. This novel design (including the AI-assisted scoring) facilitates the process of pain assessment and allows pain scores to be obtained in a less subjective way. The system has password-enabled authentication to protect and secure the data. Further, the binary scoring of pain scale reduces the likelihood of user judgement bias when recording non-facial items (Ridley, 2002). It also allows easier quantification of patient's characteristics in terms of pain which in turn facilitates the prognostication of pain severity. This allows for conversion of probability (present or absent) into a binary outcome which informs the tool's ordinal scale of pain severity (mild, moderate, severe; Ridley, 2002). The latter is arrived through automated summation of the total pain score (Figure 2G). Because PainChek™ is a digital tool linked to the WAP, the technology overcomes the limitations of guessing practices and poor identification of the presence of pain by nurses and geriatricians in people with severe cognitive impairment (Kovach et al., 2000; Cohen-Mansfield and Lipson, 2002). Through systemizing and structuring the process of assessment, PainChek™ will overcome these challenges and improve multidisciplinary communication among health care professionals. For its use in clinical practice, the App does not require Internet connection. Synchronizing the clinical data (collected through the App) with the WAP even at a later time still preserves the actual time of pain assessment as the App saves each assessment in real time (Figures 2H,J). Electronic documentation of the system has the capacity to allow patient profiling using their corresponding pain assessment (Figure 2J) and management logs (Figure 2L). The ability of the system to collect a large amount of pain data over a period of time helps in identifying temporal patterns (Figure 2K), which can offer useful clinical insights in terms of patients' responsiveness to interventions provided during a specified period. PainChek™ also facilitates the interdisciplinary communication among health care practitioners through providing a more comprehensive and up-to-date picture of patient's pain. This approach was also suggested by Lichtner et al. for decision support systems for pain management in patients with dementia (Lichtner et al., 2015, 2016).
A primary deficiency in the development of majority of the health related apps is the fact that they are designed by non-health care practitioners (Lalloo et al., 2015). In contrast, our system was developed by clinical researchers in collaboration with AI and web interface engineers (Atee et al., 2017a). In addition, most existing health apps including those related to pain, lack evidence in their development and testing (Rosser and Eccleston, 2011), whereas the PainChek™ App has been conceptualized, and clinically tested around the best available evidence in gerontology, pain, and dementia (Atee et al., 2017a,b). Further, the PainChek™ App is to our knowledge the only pain assessment tool in dementia that has regulatory clearance in Australia (TGA) and Europe (CE mark), as a medical device (ARTG, 2017). Through clinical studies, we have also demonstrated that our approach in developing a hybrid model (automated FACS pain relevant items and other clinical indicators related to older adults with dementia) is a valid and reliable method in evaluating pain (Atee et al., 2017a,b).
Our conceptualized model design was also supported by the literature (Herr and Garand, 2001; AGS Panel on Persistent Pain in Older Persons, 2002; Herr, 2002; Herr et al., 2006, 2011; Hadjistavropoulos et al., 2007, 2014; Beach et al., 2016). Lints-Martindale et al. (2012) found that the AGS-recommended pain behavioral domains are comprehensive and useful indicators in recognizing painful episodes. Beach et al. (2016) reported that integrating objective behavioral descriptors into observational tools improve pain assessment in people with Alzheimer's dementia (AD). Defined as “Actions or postural displays that are enacted during the experience of pain,” pain behaviors are important manifestations that convey rich information about patient's pain severity (Hadjistavropoulos and Craig, 2002; Herr et al., 2017). Of these behaviors, non-verbal expressions of pain such as facial expressions, vocalizations, and body movements are generally difficult to suppress (Martel et al., 2012). Of note, facial expressions of pain have been widely researched because they are “readily accessible, highly plastic, and are believed to be the most specific, encodable form of pain behavior” (Williams, 2002). Facial expressions are also one of the strongest indicators of pain particularly in people with cognitive impairment or dementia (Kunz et al., 2007, 2009). There is a significant increase of pain behaviors in AD compared to healthy control (Lautenbacher et al., 2013; Beach et al., 2017). Horgas et al. (2009) indicated that the resultant numerical scores from summating pain behaviors are closely linked to the self-report of pain. In their meta-analysis Labus et al. (2003), also found that the use of multiple behavioral domains has a synergistic effect on pain assessment because the obtained scores are more representative of subjective pain experience. McCahon et al. (2005) noted that observation of pain behaviors is a valid and reliable assessment method for use with patients with chronic pain. Although these findings were drawn from samples of cognitively intact individuals with chronic pain, similar trends were also observed in people with cognitive impairments. A recent review of pain behaviors in people with dementia by Herr et al. (2011, 2017) revealed that these behaviors are strong indicators of the presence and intensity of pain. Further, these pain behaviors configure the item descriptors list of observational pain assessment tools in verbal and non-verbal geriatric populations (Herr et al., 2011, 2017; Lichtner et al., 2014). In older adults with dementia, pain behavior tools improved binary pain recognition (i.e., presence or absence of pain) by up to 25.4%, and ordinal level of pain intensity by up to 42.5% above chance (Lukas et al., 2013). It is thus evident from the above that there is a consensus in the literature about the predictability of pain related behaviors in informing the assessment of clinical pain.
The App has been designed for administration by a wide range of users including clinicians and carers (Atee et al., 2017a). The training resources are comprehensive and diversified. The tool is available as a smart device App compatible with various mobile operating systems such as Android and iOS (Atee et al., 2017a,b). Thus, these useful and unique characteristics cover multiple aspects of clinical utility, such as scoring, administration time and skills, and supporting materials which most of the other tools are currently missing (Lichtner et al., 2014; Herr et al., 2017).
In conclusion, evidence to date suggests that the PainChek™ system offers a novel method that should make the process of pain assessment and monitoring simpler and more objective for clinicians and carers of patients who cannot verbalize their pain.
Availability of Materials
The PainChek™ system and materials reported in this manuscript are commercially available from PainChek Ltd, Sydney, NSW, Australia.
Data Availability Statement
All relevant data is contained within the manuscript. No additional datasets were generated for this manuscript because the submitted work is a technology report.
Ethics Statement
All clinical studies of PainChek™ that involved human subjects reported in this manuscript were conducted in accordance with the World Medical Association's Declaration of Helsinki, the recommendations and policy statements of the Alzheimer's Association and the World Health Organization on assistive technologies for people with dementia. These studies were approved by the Human Research Ethics Committee of Curtin University, Western Australia (HREC: HR10/2014), and the participating aged care groups. Study 2 was also registered with Therapeutic Goods Administration (TGA) under the Clinical Trial Notification (CTN) Scheme (CT-2016-CTN-04886-1 v1). Due to cognitive impairment of the involved subjects, proxies gave authorized written informed consent on their behalf. All data were de-identified to protect confidentiality.
Author Contributions
MA, KH, and JH: conceived the idea; MA: drafted and organized the manuscript, and conducted the literature search. All authors reviewed, contributed to and approved the final version of the manuscript.
Funding
The authors would like to acknowledge the contribution of an Australian Government Research Training Program Scholarship in supporting this research. The original research that led to the development of the PainChek™ tool is part of a Ph.D. project which was also supported by the Dementia Australia Research Foundation (DARF) through grant funding and a stipend scholarship. The content of the article is solely the responsibility of the authors and does not necessarily represent the official views of DARF. The project has been commercialized into a spin-off start-up company (ePAT Pty Ltd), which has been publicly listed as PainChek Ltd in the Australian Share Securities (ASX) since October 2016. The current manuscript is also sponsored by PainChek Ltd. The funding bodies had no role in writing the manuscript.
Conflict of Interest Statement
All authors are shareholders in PainChek Ltd, which is marketing the PainChek™ instrument. They also have a patent application titled “A pain assessment method and system” (PCT/AU2015/000501), which is currently under national phase examination since February 2, 2017. MA is a Research Scientist for PainChek Ltd while serving as a Research Fellow and Ph.D. Candidate with the School of Pharmacy and Biomedical Sciences, Curtin University. KH is employed as a consultant by PainChek Ltd while serving as an Assistant Professor at University of Pristina, and an Adjunct Senior Lecturer at the School of Pharmacy and Biomedical Sciences, Curtin University. JH holds the position of Chief Scientific Officer of PainChek Ltd while serving as a Professor at the School of Pharmacy and Biomedical Sciences, Curtin University.
Acknowledgments
Sincere thanks goes to Scott Robertson from PainChek Ltd for providing some technical details about the PainChek™ system, and gratitude to Mustafa Al Abbasi for assisting with placing the images on the manuscript.
References
AAAI (1995-2013). Association for the Advancement of Artificial Intelligence (AAAI). AI Overview. AAAI. Available online at: http://aitopics.org/topic/ai-overview
ADI (2017). Alzheimer's Disease International (ADI) (2017). About Dementia. London: ADI[10/01/2018]. Available online at: https://www.alz.co.uk/about-dementia.
American Geriatrics Society (AGS) Panel on Persistent Pain in Older Persons. (2002). The management of persistent pain in older persons. J. Am. Geriatr. Soc. 50, S205–S224. doi: 10.1046/j.1532-5415.50.6s.1.x
ARTG (2017). Australian Government, Department of Health, Therapeutic Goods Administration, eBS Australian Register of Therapeutic Goods (ARTG) Medicines. Public Summary: ePAT Technologies Ltd- Information system software, application program. Available online at: https://www.ebs.tga.gov.au/servlet/xmlmillr6?dbid=ebs/PublicHTML/pdfStore.nsf&docid=CD49D725FBC4131ECA2581610042344A&agid=(PrintDetailsPublic)&actionid=1
AWS (2017). Amazon Web Services (AWS). Amazon EC2: Secure and Resizable Compute Capacity in the Cloud. AWS. Available online at: https://aws.amazon.com/ec2/
Altman, D. G., Machin, D., Bryant, T. N., and Gardner, M. J. (eds.). (2000). Statistics with Confidence, 2nd Edn. London: BMJ Books.
Lints-Martindale, A. C., Hadjistavropoulos, T., Lix, L. M., and Thorpe, L. (2012). a comparative investigation of observational pain assessment tools for older adults with dementia. Clin. J. Pain 28, 226–237. doi: 10.1097/AJP.0b013e3182290d90
Atee, M., Hoti, K., and Hughes, J. D. (2017b). Psychometric evaluation of the electronic Pain Assessment Tool (ePAT): an innovative instrument for individuals with moderate to severe dementia. Dement. Geriatr. Cogn. Disord. 44, 256–267. doi: 10.1159/000485377
Atee, M., Hoti, K., Parsons, R., and Hughes, J. D. (2017a). Pain assessment in dementia: evaluation of a point-of-care technological solution. J. Alzheimers Dis. 60, 137–150. doi: 10.3233/JAD-170375
Bamidis, P., Tarnanas, I., Hadjileontiadis, L., and Tsolaki, M. (2015). Handbook of Research on Innovations in the Diagnosis and Treatment of Dementia. Hershy, PA: Medical Information Science Reference (an imprint of IGI Global).
Bartels, A. H., Daley, E., Parker, A., Evelson, B., and Muteba, C. (2009). Smart Computing Drives the New Era of IT Growth. Cambridge: UK Forrester Research.
Beach, P. A., Huck, J. T., Miranda, M. M., Foley, K. T., and Bozoki, A. C. (2016). Effects of Alzheimer Disease on the facial expression of pain. Clin. J. Pain 32, 478–487. doi: 10.1097/AJP.0000000000000302
Beach, P. A., Huck, J. T., Zhu, D. C., and Bozoki, A. C. (2017). Altered behavioral and autonomic pain responses in Alzheimer's Disease are associated with dysfunctional affective, self-reflective and salience network resting-state connectivity. Front. Aging Neurosci. 9:297. doi: 10.3389/fnagi.2017.00297
Breivik, H., Borchgrevink, P. C., Allen, S. M., Rosseland, L. A., Romundstad, L., Breivik Hals, E. K., et al. (2008). Assessment of pain. BJA 101, 17–24. doi: 10.1093/bja/aen103
Cohen-Mansfield, J., and Lipson, S. (2002). Pain in cognitively impaired nursing home residents: how well are physicians diagnosing it? J. Am. Geriatr. Soc. 50, 1039–1044. doi: 10.1046/j.1532-5415.2002.50258.x
Craig, K. D., Prkachin, K. M., and Grunau, R. V. E. (2011). “The facial expression of pain,” in Handbook of Pain Assessment, 3rd Edn, eds D. C. Turk and R. Melzack (New York, NY: The Guilford Press), 117–133.
Duthey, B. (2013). Background Paper 6.11: Alzheimer Disease and Other Dementias. A Public Health Approach to Innovation, Update on 2004 Background Paper. Available online at: http://www.who.int/medicines/areas/priority_medicines/BP6_11Alzheimer.pdf
Ekman, P., Friesen, W. V., and Hager, J. (1978). The Facial Action Coding System (FACS): A Technique for the Measurement of Facial Action. Palo Alto, CA: Consulting Psychologists Press.
Flaherty, S. A. (1996). Pain measurement tools for clinical practice and research. J. Am. Assoc. Nurse Anesth. 64, 133–140.
Hadjistavropoulos, T., and Craig, K. D. (2002). A theoretical framework for understanding self-report and observational measures of pain: a communications model. Behav. Res. Ther. 40, 551–570. doi: 10.1016/S0005-7967(01)00072-9
Hadjistavropoulos, T., Herr, K., Prkachin, K. M., Craig, K. D., Gibson, S. J., Lukas, A., et al. (2014). Pain assessment in elderly adults with dementia. Lancet. Neurol. 13, 1216–1227. doi: 10.1016/S1474-4422(14)70103-6
Hadjistavropoulos, T., Herr, K., Turk, D. C., Fine, P. G., Dworkin, R. H., Helme, R., et al. (2007). An interdisciplinary expert consensus statement on assessment of pain in older persons. Clin. J. Pain 23, S1–S43. doi: 10.1097/AJP.0b013e31802be869
Herr, K. (2002). Pain assessment in cognitively impaired older adults. Am. J. Nurs. 102, 65–67. doi: 10.1097/00000446-200212000-00020
Herr, K. A., and Garand, L. (2001). Assessment and measurement of pain in older adults. Clin. Geriatr. Med. 17, 457–478. doi: 10.1016/S0749-0690(05)70080-X
Herr, K., Bjoro, K., and Decker, S. (2006). Tools for assessment of pain in nonverbal older adults with dementia: a state-of-the-science review. J. Pain Symptom. Manage. 31, 170–192. doi: 10.1016/j.jpainsymman.2005.07.001
Herr, K., Coyne, P. J., McCaffery, M., Manworren, R., and Merkel, S. (2011). Pain assessment in the patient unable to self-report: position statement with clinical practice recommendations. Pain Manage. Nurs. 12, 230–250. doi: 10.1016/j.pmn.2011.10.002
Herr, K., Zwakhalen, S., and Swafford, K. (2017). Observation of pain in dementia. Curr. Alzheimer Res. 14, 486–500. doi: 10.2174/1567205013666160602234526
Holmes, H. M., Sachs, G. A., Shega, J. W., Hougham, G. W., Hayley, D., and Dale, W. (2008). Integrating palliative medicine into the care of persons with advanced dementia: identifying appropriate medication use. J. Am. Geriatr. Soc. 56, 1306–1311. doi: 10.1111/j.1532-5415.2008.01741.x
Horgas, A. L., Elliott, A. F., and Marsiske, M. (2009). Pain assessment in persons with dementia: relationship between self-report and behavioral observation. J. Am. Geriatr. Soc. 57, 126–132. doi: 10.1111/j.1532-5415.2008.02071.x
Hoti, K., Atee, M., and Hughes, J. D. (2018). Clinimetric properties of the electronic Pain Assessment Tool (ePAT) for aged-care residents with moderate to severe dementia. J. Pain Res. 11, 1037–1044. doi: 10.2147/JPR.S158793
Kelly, K. (2016). The Inevitable: Understanding the 12 Technological Forces that Will Shape Our Future. New York, NY: Viking.
Kovach, C. R., Griffie, J., Muchka, S., Noonan, P. E., and Weissman, D. E. (2000). Nurses' perceptions of pain assessment and treatment in the cognitively impaired elderly. It's not a guessing game. Clin. Nurse Spec. 14, 215–220. doi: 10.1097/00002800-200009000-00011
Kunz, M. (2014). “Behavioural/facial markers of pain, emotion, cognition,” in Pain, Emotion and Cognition: A Complex Nexus, eds G. Pickering, and S. Gibson (Clermont-Ferrand: Springer), 123–33.
Kunz, M., and Lautenbacher, S. (2014). The faces of pain: a cluster analysis of individual differences in facial activity patterns of pain. Eur. J. Pain 18, 813–823. doi: 10.1002/j.1532-2149.2013.00421.x
Kunz, M., Mylius, V., Schepelmann, K., and Lautenbacher, S. (2009). Effects of age and mild cognitive impairment on the pain response system. Gerontology 55, 674–682. doi: 10.1159/000235719
Kunz, M., Scharmann, S., Hemmeter, U., Schepelmann, K., and Lautenbacher, S. (2007). The facial expression of pain in patients with dementia. Pain 133, 221–228. doi: 10.1016/j.pain.2007.09.007
Labus, J. S., Keefe, F. J., and Jensen, M. P. (2003). Self-reports of pain intensity and direct observations of pain behavior: when are they correlated? Pain 102, 109–124. doi: 10.1016/s0304-3959(02)00354-8
Lalloo, C., Jibb, L. A., Rivera, J., Agarwal, A., and Stinson, J. N. (2015). “There's a Pain App for That” review of patient-targeted smartphone applications for pain management. Clin. J. Pain 31, 557–563. doi: 10.1097/AJP.0000000000000171
Lautenbacher, S., Niewelt, B. G., and Kunz, M. (2013). Decoding pain from the facial display of patients with dementia: a comparison of professional and nonprofessional observers. Pain Med. 14, 469–477. doi: 10.1111/pme.12050
LeCun, Y., Bengio, Y., and Hinton, G. (2015). Deep learning. Nature 521, 436–444. doi: 10.1038/nature14539
Lichtner, V., Dowding, D., Allcock, N., Keady, J., Sampson, E. L., Briggs, M., et al. (2016). The assessment and management of pain in patients with dementia in hospital settings: a multi-case exploratory study from a decision making perspective. BMC Health Serv. Res. 16:427. doi: 10.1186/s12913-016-1690-1
Lichtner, V., Dowding, D., and Closs, S. J. (2015). The relative meaning of absolute numbers: the case of pain intensity scores as decision support systems for pain management of patients with dementia. BMC Med. Inform. Decis. Mak. 15:111. doi: 10.1186/s12911-015-0233-8
Lichtner, V., Dowding, D., Esterhuizen, P., Closs, S. J., Long, A. F., Corbett, A., et al. (2014). Pain assessment for people with dementia: a systematic review of systematic reviews of pain assessment tools. BMC Geriatr. 14:138. doi: 10.1186/1471-2318-14-138
Lukas, A., Barber, J. B., Johnson, P., and Gibson, S. J. (2013). Observer-rated pain assessment instruments improve both the detection of pain and the evaluation of pain intensity in people with dementia. Eur. J. Pain 17, 1558–1568. doi: 10.1002/j.1532-2149.2013.00336.x
Martel, M. O., Wideman, T. H., and Sullivan, M. J. (2012). Patients who display protective pain behaviors are viewed as less likable, less dependable, and less likely to return to work. Pain 153, 843–849. doi: 10.1016/j.pain.2012.01.007
McCahon, S., Strong, J., Sharrj, R., and Cramond, T. (2005). Self-report and pain behavior among patients with chronic pain. Clin. J. Pain 21, 223–231. doi: 10.1097/00002508-200505000-00005
Mell, P., and Grance, T. (2011). The NIST Definition of Cloud Computing: Recommendations of the National Institute of Standards and Technology. National Institute of Standards and Technology (NIST), Gaithersburg, MD: US Department of Commerce, Contract No. NIST Special Publication, 80–145.
Merskey, H., and Bogduk, N. (eds.) (1994). IASP Task Force on Taxonomy: Classification of Chronic Pain: Description of Chronic Pain Syndromes and Definition of Pain Terms. Seattle, WA: IASP Press.
Mitchell, A. J. (2010). “How do we know when a screening test is clinically useful?,” in Coyne Screening for Depression in Clinical Practice : an Evidence-based Guide eds A. J. Mitchell and J. C. Coyne (Oxford; New York, NY: Oxford University Press), 395.
Mitchell, S. L., Teno, J. M., Kiely, D. K., Shaffer, M. L., Jones, R. N., Prigerson, H. G., et al. (2009). The clinical course of advanced dementia. New Engl. J Med. 361, 1529–1538. doi: 10.1056/NEJMoa0902234
Polit, D. F., and Hungler, B. P. (1991). Nursing Research: Principals and Methods. Philadelphia, PA: JB Lippincott.
Portney, L., and Mary, P. (2009). Foundations of Clinical Research: Applications to Practice. Upper Saddle River, NJ: Pearson/Prentice Hall.
Prkachin, K. M. (1992). The consistency of facial expressions of pain: a comparison across modalities. Pain 51, 297–306. doi: 10.1016/0304-3959(92)90213-U
Prkachin, K. M. (2009). Assessing pain by facial expression: facial expression as nexus. Pain Res. Manage. 14, 53–58. doi: 10.1155/2009/542964
Prkachin, K. M., and Solomon, P. E. (2008). The structure, reliability and validity of pain expression: evidence from patients with shoulder pain. Pain 139, 267–274. doi: 10.1016/j.pain.2008.04.010
Ridley, S. A. (2002). Uncertainty and scoring systems. Anaesthesia 57, 761–767. doi: 10.1046/j.1365-2044.2002.02619.x
Rosser, B. A., and Eccleston, C. (2011). Smartphone applications for pain management. J. Telemed. Telecare 17, 308–312. doi: 10.1258/jtt.2011.101102
Schneider, L. S., Dagerman, K. S., and Insel, P. (2005). Risk of death with atypical antipsychotic drug treatment for dementia - meta-analysis of randomized placebo-controlled trials. JAMA 294, 1934–1943. doi: 10.1001/jama.294.15.1934
United Nations Department of Economic and Social Affairs Population Division (2017). World Population Prospects: The 2017 Revision, Key Findings and Advance Tables. New York, NY: United Nations.
van Herk, R., van Dijk, M., Baar, F. P. M., Tibboel, D., and de Wit, R. (2007). Observation scales for pain assessment in older adults with cognitive impairments or communication difficulties. Nurs. Res. 56, 34–43. doi: 10.1097/00006199-200701000-00005
Waltz, C. F., Strickland, O. L., and Lenz, E. R. (1991). Measurement in Nursing Research. Philadelphia, PA: F.A Davis.
Williams, A. C. (2002). Facial expression of pain: an evolutionary account. Behav. Brain Sci. 25, 439–455. doi: 10.1017/S0140525X02000080
World Health Organisation (WHO) (2017). Dementia: Fact sheet. Geneva: WHO Media Centre. Available online at: http://www.who.int/mediacentre/factsheets/fs362/en/ (Accessed January 10, 2018).
Keywords: PainChek™, pain assessment system, artificial intelligence, automated facial recognition, dementia, smart device application, technology
Citation: Atee M, Hoti K and Hughes JD (2018) A Technical Note on the PainChek™ System: A Web Portal and Mobile Medical Device for Assessing Pain in People With Dementia. Front. Aging Neurosci. 10:117. doi: 10.3389/fnagi.2018.00117
Received: 17 January 2018; Accepted: 04 April 2018;
Published: 12 June 2018.
Edited by:
Donna M. Wilcock, University of Kentucky, United StatesReviewed by:
Paola Sandroni, Mayo Clinic, United StatesFilippo Brighina, Università degli Studi di Palermo, Italy
Copyright © 2018 Atee, Hoti and Hughes. This is an open-access article distributed under the terms of the Creative Commons Attribution License (CC BY). The use, distribution or reproduction in other forums is permitted, provided the original author(s) and the copyright owner are credited and that the original publication in this journal is cited, in accordance with accepted academic practice. No use, distribution or reproduction is permitted which does not comply with these terms.
*Correspondence: Mustafa Atee, bXVzdGFmYS5hdGVlQGN1cnRpbi5lZHUuYXU=