Changes in Cerebrospinal Fluid Tau and β-Amyloid Levels in Diabetic and Prediabetic Patients: A Meta-Analysis
- School of Nursing, Peking University Health Science Center, Beijing, China
Increased risks for Alzheimer's disease (AD) are a well-recognized consequence of diabetes, insulin resistance (IR), and hyperinsulinemia. Since cerebrospinal fluid (CSF) is surrounding the central nervous system, alterations of β-amyloid (Aβ) and tau protein in the CSF may be indicative of AD-type degenerations in the brain. Current laboratory diagnosis of AD uses three biomarkers in CSF: Aβ1-42, total tau (t-Tau), and phosphorylated tau (p-Tau). However, changes in these biomarkers in diabetic and prediabetic patients are scattered and variable in literature. Thus, we attempt to perform a systematical analysis of these available data. MEDLINE, EMBASE, the Cochrane Central database, China National Knowledge Infrastructure (CNKI), and Wanfang Data electronic databases were searched to gather published studies that have evaluated the AD-type biomarkers in the CSF of subjects with diabetes, IR, or hyperinsulinemia in comparison with respective controls. Overall analysis of the published data showed no significant differences in Aβ1-42, t-Tau, and p-Tau levels in the CSF between the (pre)diabetic subjects and controls. However, subgroup analysis suggested that (pre)diabetic conditions might accelerate decrease of Aβ1-42, but increase of t-Tau levels in the CSF of subjects with cognitive impairment, and the association with p-Tau in the CSF was stronger (P = 0.001) for diabetes than those of prediabetes (P = 0.61). Our analyses reveal that the relationship between (pre)diabetic conditions and AD-type biomarker status in the CSF was subjective to clinical characteristics.
Introduction
Diabetes represents a group of metabolic disorders caused by impaired insulin signaling and function. In addition to elevated blood glucose and insulin resistance (IR) in peripheral tissues, diabetes leads to a number of complications and comorbidities including cognitive dysfunction (American Diabetes Association, 2013). In 1950, the term “diabetic encephalopathy” was first employed to describe brain dysfunction in patients with diabetes. From then on, mounting evidences from longitudinal and cross-sectional studies as well as biological research have demonstrated a significant association between diabetes and increased risks of multiple-domain cognitive decline and even dementia (McCrimmon et al., 2012). Several excellent meta-analyses have described that diabetes results in mild-to-moderate deficits in multiple cognitive domains, especially processing speed, attention, memory, and executive function (Monette et al., 2014; Palta et al., 2014; Sadanand et al., 2016). Furthermore, other meta-analyses have found that diabetes is a significant risk factor for mild cognitive impairment (MCI) and incident dementia including Alzheimer's disease (AD), vascular dementia (VD), and any dementia (Cheng et al., 2012). Dementia is an age-related chronic and progressive disorder in late life. A characteristic feature of dementia is the deterioration of cognitive function beyond normal aging process. Approximately, 4.6 million new cases of dementia are estimated to occur globally every year (Ferri et al., 2005). The incidence of dementia and the number of individuals living with dementia are expected to be doubled in the next 20 years, which will cause enormous social and economic burdens (Tariq and Barber, 2017). The AD is the most common type of dementia, characterized by two pathological hallmarks including formation of senile plaques (SPs) by extracellular deposits of β-amyloid (Aβ) and intracellular neurofibrillary tangles (NFT) from aggregated hyperphosphorylated Tau proteins in the brain (Jack et al., 2013), which are associated with elevated levels of phosphorylated Tau (p-Tau) and decreased levels of the nonsoluble Aβ1-42 from abnormal cleavage of Aβ in the cerebrospinal fluid (CSF) (Blennow and Hampel, 2003). An updated meta-analysis of cohort studies has concluded that the risk of AD is significantly higher in diabetic patients than control subjects, especially in Eastern populations [relative risk (RR):1.62, 95% confidence interval (CI): 1.49–1.75] (Zhang et al., 2017).
While AD was not identified as one of the hallmark comorbidities of diabetes, epidemiological and biological evidences have suggested a link between these two disorders. Over the past 30 years, investigations on the potential mechanisms and pathways in this regards have grown rapidly. There have been increasing numbers of studies describing vascular, metabolic, and neuroendocrine contributions to AD (Kodl and Seaquist, 2008; Banks et al., 2012). With regards to characterizing AD pathology, phosphorylated Tau (p-Tau) and total Tau (t-Tau) are elevated, while Aβ1-42 is decreased in the CSF. Elevations of t-Tau and p-Tau in the CSF are biomarkers of tauopathy in AD and they correlated well with intracerebral AD pathology, while decreases of Aβ1-42 in the CSF are inversely proportional to amyloid in the brain (Reitz, 2012). There is also an increasing evidence that patients with diabetes shared commonality with neuropathology in AD (Matioli and Nitrini, 2015). Moreover, the risk of AD has also been shown to be increased in prediabetes individuals with high fasting glucose and/or impaired glucose tolerance due to IR and simultaneous exposure to abnormally high levels of insulin persisting for extended periods of time (hyperinsulinemia) (Roriz-Filhom et al., 2009). The IR and hyperinsulinemia are also reported to be linked with the pathological features of AD (Luchsinger et al., 2004; Westwood et al., 2017).
However, there have been reports with conflicting results (Moran et al., 2015). Apparently, the association between diabetes or prediabetes and AD-like pathology in the CSF is still a subject of controversy. Thus, we collected data from published case–control studies of diabetes, prediabetes, and CSF biomarkers, and performed a meta-analysis to help clarify the association between diabetes or prediabetes and CSF biomarkers of neurodegeneration implicated in the development of AD.
Methods
Data Source and Search
The Cochrane Library, Medline, EMBASE, China National Knowledge Infrastructure (CNKI), and Wanfang Data electronic databases (from their inception to December 1, 2017) were searched to identify human studies published in English and Chinese. The search terms and key words included “diabetes,” “Alzheimer,” “insulin,” “cerebrospinal fluid,” “amyloid,” “tau,” and “dementia.” These key words were combined with type 2 diabetes (T2D), type 2 diabetes mellitus (T2DM), type 1 diabetes (T1D), type 1 diabetes mellitus (T2DM), IR, hyperinsulinemia, hyperglycemia, glucose, glycemia, impaired glycemia, ApoE, duration, complications, and treatment modality to locate studies on (pre)diabetes and associated variables. Reference lists from relevant original and review articles were also screened and potentially relevant papers were retrieved and assessed in accordance with the selection criteria. Citations and abstracts of all the studies have been checked to prevent duplications.
Study Selection
The protocol for selecting an eligible study was based on inclusion and exclusion criteria being screened at two levels. Firstly, the title and abstract of the paper were screened to identify whether the study fulfilled the inclusion criteria. Next, the initially selected papers were retrieved for full text by two independent reviewers to establish the final eligibility of the articles. Disagreements were adjudicated by a third reviewer based on the full text.
Studies in this meta-analysis were assessed for eligibility by fulfillment of the criteria of the population, intervention, comparison, outcomes, and setting (PICOS) question format and the details of inclusion and exclusion were as follows.
Population
We included studies with adults diagnosed with (pre)diabetes, including T1DM, T2DM, IR, hyperinsulinemia, hyperglycemia, or impaired glycemia, according to the criteria generally accepted. Studies without a non(pre)diabetic comparison group were excluded.
Intervention
We included only case–control studies; interventions were not taken into consideration.
Comparison
We included studies with comparison with (pre)diabetics or non(pre)diabetics, which allocated (pre)diabetic patients according to criteria of diagnoses. Review articles, case reports, commentaries, clinical trials, or letters were excluded.
Outcome
Studies were included if they measured at least one of the outcomes of CSF levels of AD-type biomarkers and presented original data on CSF biomarkers to permit effect size calculations (means, SD, SE, 95%CI or SEM).
Setting: Case–Control Studies
Our database searches resulted in 653 articles. Initial screening yielded 131 studies for title or abstract review, and 95 of these articles underwent full text review. After following a thorough examination for this meta-analysis, we excluded 87 of the 95 studies (without diabetic or prediabetic participants in papers, n = 17; data not applied to the research question, n = 21; nonresearch papers or review articles, n = 25; randomized control trials, n = 4; study was not in English or Chinese, n = 11; or in vivo studies, n = 10). The remaining 7 articles were included in this analysis and a flow diagram of the study selection is presented in Figure 1.
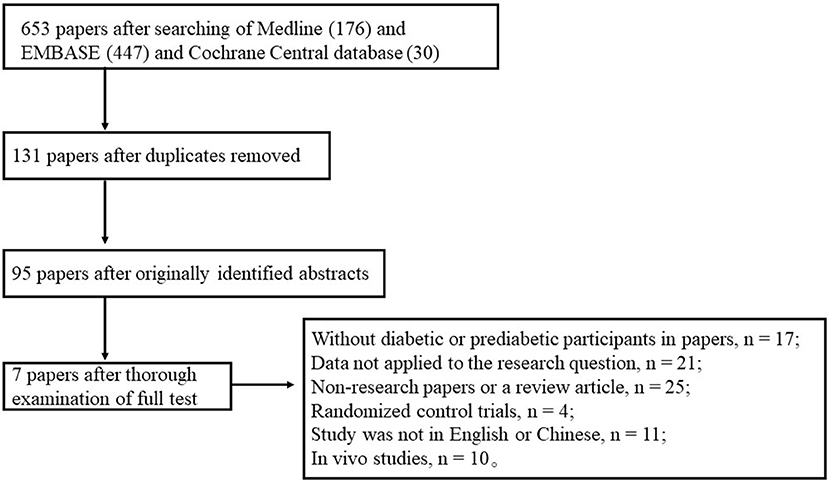
Figure 1. Flow diagram of selection of studies focusing on levels of Aβ or tau proteins in the CSF of diabetic subjects.
Quality Assessment
The quality of all the included studies was appraised using the checklists of the Newcastle–Ottawa scale (Table 1). These checklists included selection (cases definition, representativeness of cases, selection of controls and definition of controls with 4 points), comparability (age and gender and additional factors with 2 points), and exposure/outcome (ascertainment of exposure, same method for case and control and nonresponse rate with 3 points). Quality assessment was according to the guidelines for reporting meta-analyses of observational studies. Scores were awarded up to 9 scores, with the highest quality and the final score of at least 6 points indicating adequate quality that can be included in our analysis (Bashashati et al., 2017; Garcez et al., 2018; Guo et al., 2018). Two of the authors independently evaluated potentially acceptable (included) articles in accordance with these criteria, and discrepancies were dealt with by discussion.
Data Extraction and Conversion
Important details regarding the participants, methods, and measurements were extracted from the selected studies and summarized in Table 2. The elements of the checklist included (1) details of publication including first author's name and publication year; (2) characteristics of the participants including sample size, age, gender, categorization; (3) CSF levels of Aβ, p-Tau, or t-Tau; and (4) methods of measurement.
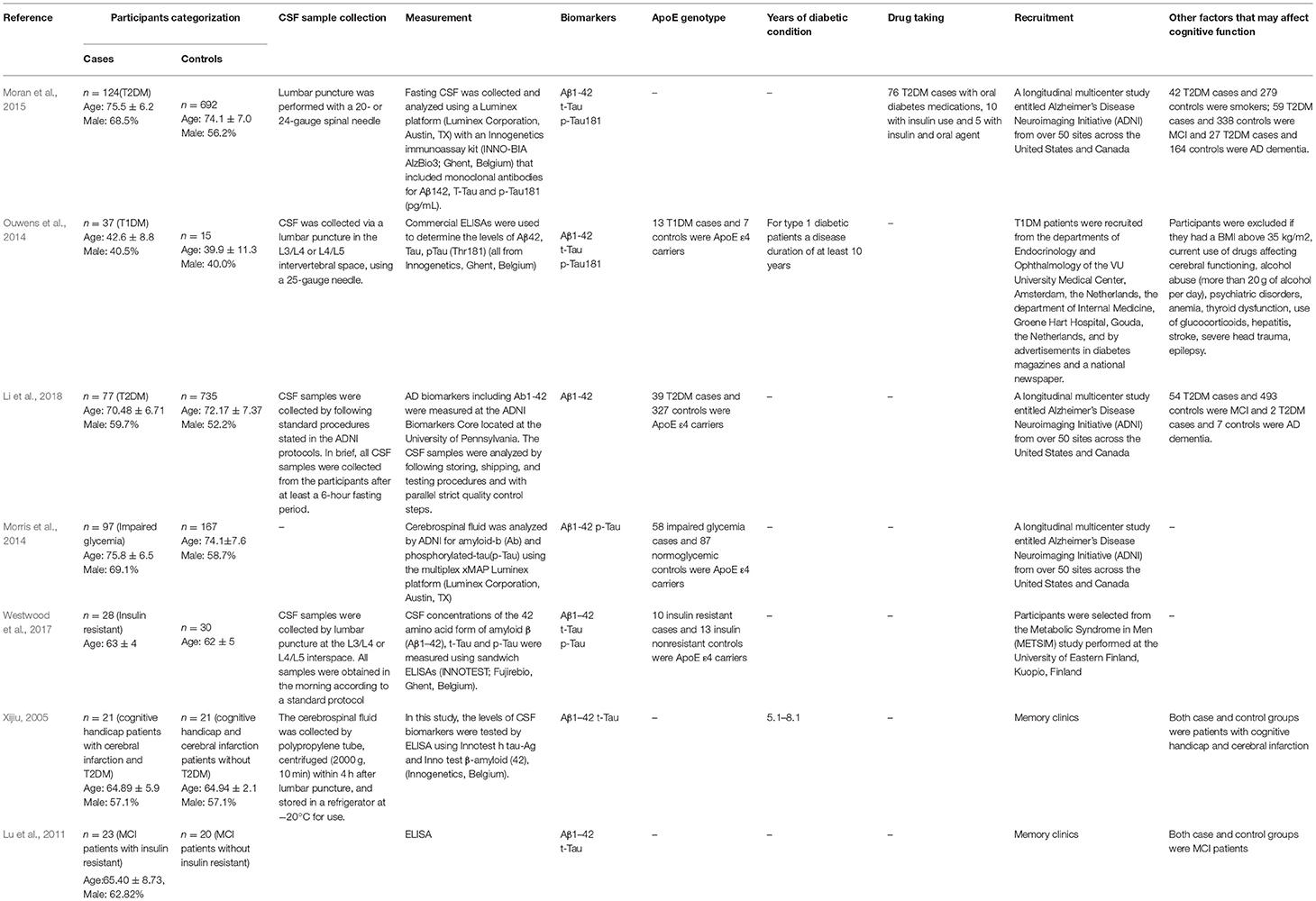
Table 2. Extracted and summarized details on subjects, methods, and measurements of the included studies.
Statistical Analysis
Data were compiled as summary statistics (N, mean, and SD) and then pooled by using an inverse-variance method. Heterogeneity among the studies was evaluated using Cochran's Chi-Squared test for homogeneity (Chi2) and estimated by calculating the I2. Random-effects meta-analyses were performed using Review Manager Version 5.3 to generate summary values, since heterogeneity was invariably high. Forest plots were presented and the results were determined to be significant when P < 0.05. Heterogeneity across studies was assessed according to I2 statistics, which was categorized as low (< 40%), moderate (40–75%), or high (>75%) to indicate the percentage of variance owing to study heterogeneity (Islam et al., 2017). Four independent subgroup analyses were conducted as follows: (i) diabetes vs. prediabetes (e.g., IR, hyperinsulinemia, hyperglycemia, or impaired glycemia); (ii) mean age of group below 65 years old vs. above 65 years old; (iii) subjects recruited through memory clinics vs. not recruited through memory clinics; (iv) studies with high quality (total score of NOS scale was at least 8) vs. studies with fair quality (total score of NOS scale was 5 to 7) (Alobaidi et al., 2018).
Results
Study Characteristics and Heterogeneity
Tables 1, 2 summarize the 7 studies included in this meta-analysis (Xijiu, 2005; Lu et al., 2011; Morris et al., 2014; Ouwens et al., 2014; Moran et al., 2015; Westwood et al., 2017; Li et al., 2018). All of these studies were identified to be of high or fair quality by using the Newcastle–Ottawa scale (Table 1). The diagnosis of diabetes or prediabetes was made according to the American Diabetes Association (ADA) guidelines and/or the reference. Heterogeneity among these studies was assessed (cases vs. controls: Figure 2, Aβ1-42, Chi2 = 1075.79, I2 = 99%; Figure 3, t-Tau, Chi2 = 25.20, I2 = 84%; Figure 4, p-Tau, Chi2 = 8.01, I2 = 63%). Since the heterogeneity ranged from moderate to high, the random-effects meta-analyses were employed.
Seven studies reporting Aβ levels were included in this meta-analysis, including 407 subjects with (pre)diabetes and 1,680 controls. The Aβ levels in the CSF were not different between the (pre)diabetic subjects and controls, with an effect size of 0.56 (95%CI: −1.37, 2.49, P = 0.57, Figure 2).
T-Tau in (pre)diabetic subjects was reported in 5 studies including 211 (pre)diabetes subjects and 800 controls. Cumulatively, t-Tau was not significantly increased in the CSF of (pre)diabetes subjects compared with that of controls, with an effect size of 0.39 (95%CI: −0.15, 0.94, P = 0.15, Figure 3).
Four studies reported p-Tau in the CSF of 286 (pre)diabetic and 904 control subjects. There was no significant difference in the level between the two groups (effect size 0.13, 95% CI: −0.14, 0.41, P = 0.35, Figure 4).
Results of subgroup analyses which explored potential sources of heterogeneity were summarized in Table 3. Pooled effects of Aβ1-42 and t-Tau in the CSF between cases and controls were significant in studies recruited through memory clinics (Aβ1-42: SMD: −1.76; 95% CI: −2.27, −1.25; I2 = 0%, P < 0.001; t-Tau: SMD: 1.15; 95% CI: 0.25, 2.05; I2 = 73%, P = 0.01) compared with nonsignificant correlations found from studies not recruited through memory clinics (Aβ1-42: SMD: 1.48; 95% CI: −0.85, 3.81; I2 = 100%, P = 0.21; t-Tau: SMD: −0.01; 95% CI: −0.47, 0.46; I2 = 71%, P = 0.98; see Figures 5, 6). Pooled effects of p-Tau in the CSF across studies that only included diabetic cases were significant (SMD: 0.30; 95% CI: 0.12, 0.49; I2 = 0%, P < 0.001) compared with nonsignificant correlations found from studies that only included pre-diabetic cases (SMD: −0.08; 95% CI: −0.39, 0.23; I2 = 30%, P = 0.21; see Figure 7). Both of which were affected by a substantial degree of heterogeneity. Neither mean age of groups nor quality score was significant moderators of the association between (pre)diabetes and AD-type CSF biomarkers (all subgroup analyses, P > 0.05; subgroup I2 range from 30 to 100%; see Table 3).
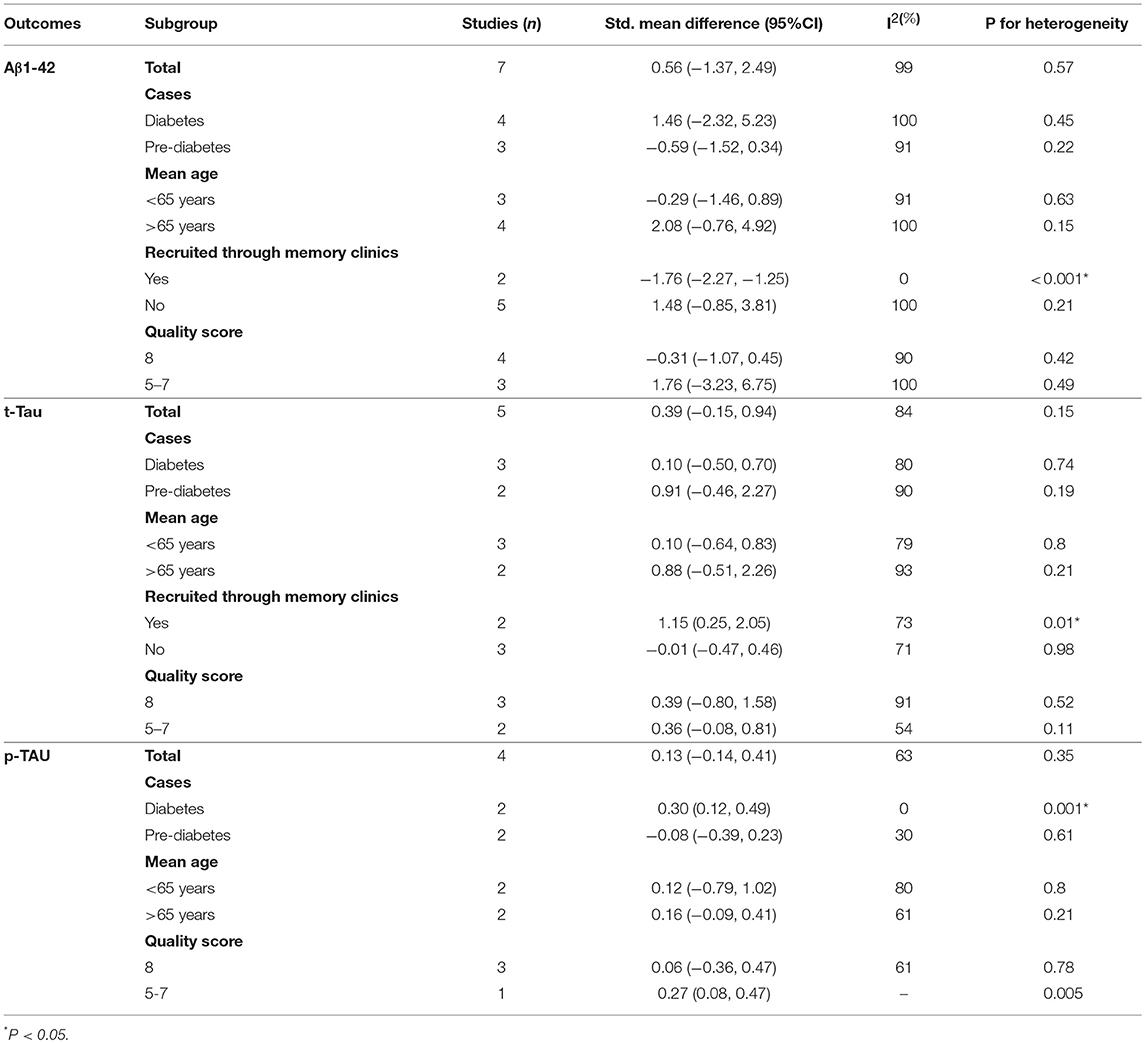
Table 3. Subgroup analyses of Aβ1-42, t-Tau, and p-Tau in the CSF between (pre)diabetic cases and controls.
Discussion
Our analysis showed no differences in Aβ1-42, t-Tau, or p-Tau levels in the CSF between (pre)diabetic and control subjects. Seemingly, neurodegenerations induced by diabetes may not be readily associated with changes in the biomarkers of Alzheimer's type pathology in the CSF.
Domain-specific cognitive impairment and cognitive dysfunction such as MCI, AD, and other types of dementia have recently been recognized to be common complications of diabetes (Neth and Craft, 2017). In addition, several studies have suggested that metabolic disorders associated with alterations of insulin homeostasis are risk factors for developing cognitive decline and even dementia. These disorders include IR, hyperinsulinemia, and diabetes, which are also attributed to impaired performance in several neuropsychological functions (Bitra et al., 2015). The molecular and cellular mechanisms underlying defective brain in (pre)diabetes have been investigated, and protein glycation and increased oxidative stress may probably be etiologic factors of AD (De Felice, 2013). In addition, there are evidences to suggest that AD and diabetes may share common signs of cerebral alterations (van Duinkerken et al., 2012a,b). Hyperinsulinemia and hyperglycemia seem to accelerate brain aging by inducing amyloid oligomerization and tau hyperphosphorylation, but the results are conflicting.
Amyloid plaque is a classical pathological AD biomarker. As the major protein component of amyloid plaque, Aβ is generated by a sequential cleavage of β- and γ-secretase from amyloid precursor protein (APP) (Faull et al., 2014). Consequently, being less soluble and more likely to aggregate than other forms, Aβ1-42 exists as the predominant form of amyloid deposited in neuritic plaques (Jovanovic et al., 2014). Plenty of evidence has demonstrated that CSF Aβ1-42 level is significantly lower in mild AD or MCI patients than normal aging individuals, thus becoming a useful pathological biomarker for AD (Frisoni et al., 2009). Although we did not find significant differences in CSF Aβ1-42 levels between (pre)diabetic and nondiabetic subjects from the overall analysis, the subgroup analysis revealed that the CSF level of Aβ1-42 was significantly lower in (pre)diabetic subjects than the controls when they were recruited from memory clinics with cognitive dysfunction, which matched the profile characteristics in patients with MCI or AD. It has been known that diabetes is associated with microvascular lesions, which can contribute to an increased permeability of blood brain barrier (BBB) and thus change the distribution of Aβ1-42 (Serlin et al., 2011; Xu et al., 2017). However, some included studies found no difference in the CSF Aβ1-42 levels between IR and non-IR or impaired glycemia (IG) and non-IG subjects. In contrast, some studies found increased CSF Aβ1-42 level in both types of diabetic patients. The subgroup analysis suggested that the cognitive dysfunction in (pre)diabetic subjects may not be due to disturbed CSF Aβ1-42 biomarker load, but diabetes or IR may accelerate the decrease of CSF Aβ1-42 level in subjects with cognitive impairment.
Hyperphosphorylation of tau protein is associated with increased intracellular NFT formation in AD (Blennow and Hampel, 2003). Recent studies have found that patients with T1DM showed increased CSF p-Tau (Ouwens et al., 2014). Meanwhile, T2DM was also associated with greater CSF t-Tau and p-Tau (Moran et al., 2015). Consequently, subgroup analysis found that CSF levels of t-Tau only significantly increased in (pre)diabetic patients with cognitive dysfunction and p-Tau in diabetic patients were significantly higher than controls. These observations fit with animal studies on streptozotocin-induced diabetic mouse model in which hyperphosphorylation of tau protein has been found in the cortex and hippocampus by histopathologic measures (Kim et al., 2009; Jung et al., 2013). Given that, scholars have proposed several pathways through which diabetes may contribute to increased p-Tau in the brain. An increased p-Tau in AD brain samples may be attributed to impaired neuronal glucose metabolism and a consequent reduced β-O-linkage of N-acetylglucosamine to tau (Liu et al., 2009). In addition, chronic hyperglycemia can increase levels of advanced glycation end products (AGEs), which may lead to protein cross-linking and promote stabilization of the paired helical filament tau (Münch et al., 2012). However, the significant differences of t-Tau were not replicated in subjects not recruited from memory clinics. In addition, the nonsignificant results of p-Tau in prediabetic cases and controls suggested that the association between prediabetes and CSF levels of p-Tau was attenuated.
Contrary to the subgroup analysis, postmortem human studies on diabetes with AD pathology have shown that the cerebral load of tau-related NFTs are either lower (Ahtiluoto et al., 2010) or similar (Thambisetty et al., 2013) between diabetic individuals and nondiabetic ones. This is consistent with the nonsignificant results of our overall analysis of p-Tau. Discrepancies among difference studies may largely be attributed to limited sample sizes with heterogeneity. Only four eligible studies with 286 cases and 904 controls measuring CSF p-Tau levels were included, and subgroup analysis of diabetes and age just included two studies in each group. However, the subgroup of age below 65 years old was presented with even higher heterogeneity, which indicated that age among the recruited prediabetes and diabetes should be considered as a potential contributing factor, but the limited studies may obscure some difference in those CSF biomarkers among young prediabetes versus aged ones. Subjects with cognitive dysfunction (e.g., MCI, AD) were also included even if these four studies were not recruited from memory clinics. In addition, the Honolulu-Asia Aging Study using rigorous phenotyping of diabetes has found greater risk of AD pathology in T2DM patients, but only among those carriers of APOE ε4 allele (Peila et al., 2002). Geijselaers et al. (2018) have also found that diabetes is associated with higher levels of insulin in the CSF, and this association is related to cognitive impairment and AD-type biomarkers in noncarriers of the APOE ε4 allele. Besides, diabetic patients usually manifest comorbidities, such as kidney disease. Similar to AD, the prevalence of diabetes and comorbidities is also higher in the elderly. As a result, the roles of comorbidities correlated with dementia in diabetic patients were also evaluated. Kuo et al. have found the hazard ratio (HR) for dementia in diabetic subjects rose from 1.45 in those without comorbidities to 1.50 in those with kidney comorbidities (Kuo et al., 2015). Sasaki et al. also reported the incidence of dementia was strongly related with kidney diseases independent of other vascular factors (Sasaki et al., 2011). Another meta-analysis including 54,779 subjects suggested that kidney disease was an independent risk factor for cognitive decline (Etgen et al., 2012). Therefore, the comorbidities of diabetic patients may also contribute to the heterogeneity of studies. However, the lack of corresponding database makes it impossible to exclude subjects with cognitive dysfunction. Stratifying these clinical studies into groups according to APOE ε4 genotype and taking comorbidities into consideration may partly explain some of the insignificant associations from our analyses.
Limitations of this meta-analysis should be considered. The included studies exploring the relationship between AD-type CSF biomarkers and (pre)diabetes had relatively small numbers of subjects. Besides, the diabetic individuals with collected CSF also included subjects with objective cognitive disturbances, MCI, AD, or other types of cognitive dysfunction (Exalto et al., 2010). That might hamper proper conclusions on the effects of (pre)diabetes on the levels of the biomarkers examined. Thus, caution should be given to generalize our findings for the population at large. Furthermore, details of duration of (pre)diabetes and effectiveness of glucose control were unavailable in most studies, which would have provided valuable clues for us to explore our hypotheses.
Conclusion
In conclusion, the associations between CSF levels of AD-type biomarkers and (pre)diabetic conditions may be affected by cognitive function, phenotypes of diabetes, and other clinical characteristics. The relatively higher heterogeneity and limited sample size contributed to the nonsignificant differences of AD-type biomarkers between (pre)diabetic cases and controls. Thus, it remains to be investigated whether the CSF levels of AD-type biomarkers change over time as well as to what extent these biomarkers relate to or can predict cerebral compromise in (pre)diabetic patients.
Author Contributions
ML conceived and designed the study. YL and XJ conducted the systematic search, screened articles, and selected eligible articles. SL and XJ extracted information from eligible studies. YL performed the analyses and interpreted the results. All authors read and approved the final manuscript.
Conflict of Interest Statement
The authors declare that the research was conducted in the absence of any commercial or financial relationships that could be construed as a potential conflict of interest.
The reviewer YL and handling Editor declared their shared affiliation at the time of the review.
References
Ahtiluoto, S., Polvikoski, T., Peltonen, M., Solomon, A., Tuomilehto, J., Winblad, B., et al. (2010). Diabetes, Alzheimer disease, and vascular dementia: a population-based neuropathologic study. Neurology 75, 1195–1202. doi: 10.1212/WNL.0b013e3181f4d7f8
Alobaidi, R., Morgan, C., Basu, R. K., Stenson, E., Featherstone, R., and Majumdar, S. R. (2018). Association between fluid balance and outcomes in critically ill children: a systematic review and meta-analysis. JAMA Pediatr. 172, 257–268. doi: 10.1001/jamapediatrics.2017.4540
American Diabetes Association (2013). Standards of medical care in diabetes−2013. Diabetes Care 36(Suppl 1), S11–S66. doi: 10.2337/dc13-S011
Banks, W. A., Owen, J. B., and Erickson, M. A. (2012). Insulin in the brain: there and back again. Pharmacol Ther. 136, 82–93. doi: 10.1016/j.pharmthera.2012.07.006
Bashashati, M., Moradi, M., and Sarosiek, I. (2017). Interleukin-6 in irritable bowel syndrome: a systematic review and meta-analysis of IL-6 (-G174C) and circulating IL-6 levels. Cytokine 99, 132–138. doi: 10.1016/j.cyto.2017.08.017
Bitra, V. R., Rapaka, D., and Akula, A. (2015). Prediabetes and Alzheimer's disease. Indian J. Pharm. Sci. 77, 511–514.
Blennow, K., and Hampel, H. (2003). CSF markers for incipient Alzheimer's disease. Lancet Neurol. 2, 605–613. doi: 10.1016/S1474-4422(03)00530-1
Cheng, G., Huang, C., Deng, H., and Wang, H. (2012). Diabetes as a risk factor for dementia and mild cognitive impairment: a meta-analysis of longitudinal studies. Intern. Med. J. 42, 484–491. doi: 10.1111/j.1445-5994.2012.02758.x
De Felice, F. G. (2013). Alzheimer's disease and insulin resistance: translating basic science into clinical applications. J. Clin. Invest. 123, 531–539. doi: 10.1172/JCI64595
Etgen, T., Chonchol, M., Förstl, H., and Sander, D. (2012). Chronic kidney disease and cognitive impairment: a systematic review and meta-analysis. Am. J. Nephrol. 35, 474–482. doi: 10.1159/000338135
Exalto, L. G., van der Flier, W. M., Scheltens, P., and Biessels, G. J. (2010). Glycemia and levels of cerebrospinal fluid amyloid and tau in patients attending a memory clinic. J. Am. Geriatr. Soc. 58, 1318–1321. doi: 10.1111/j.1532-5415.2010.02854.x
Faull, M., Ching, S. Y., Jarmolowicz, A. I., Beilby, J., and Panegyres, P. K. (2014). Comparison of two methods for the analysis of CSF Abeta and tau in the diagnosis of Alzheimer's disease. Am. J. Neurodegener. Dis. 3, 143–151.
Ferri, C. P., Prince, M., Brayne, C., Brodaty, H., Fratiglioni, L., Ganguli, M., et al. (2005). Global prevalence of dementia: a Delphi consensus study. Lancet 366, 2112–2117. doi: 10.1016/S0140-6736(05)67889-0
Frisoni, G. B., Prestia, A., Zanetti, O., Galluzzi, S., Romano, M., Cotelli, M., et al. (2009). Markers of Alzheimer's disease in a population attending a memory clinic. Alzheimers Dement. 5, 307–317. doi: 10.1016/j.jalz.2009.04.1235
Garcez, A., Leite, H. M., Weiderpass, E., Paniz, V. M. V., Watte, G., Canuto, R., et al. (2018). Basal cortisol levels and metabolic syndrome: a systematic review and meta-analysis of observational studies. Psychoneuroendocrinology 95, 50–62. doi: 10.1016/j.psyneuen.2018.05.023
Geijselaers, S., Aalten, P., Ramakers, I. H. G. B., De Deyn, P. P., Heijboer, A. C., Koek, H. L., et al. (2018). Association of cerebrospinal fluid (CSF) insulin with cognitive performance and CSF biomarkers of Alzheimer's disease. J. Alzheimers Dis. 61, 309–320. doi: 10.3233/JAD-170522
Guo, V. Y., Cao, B., Cai, C., Cheng, K. K., and Cheung, B. M. Y. (2018). Fetuin-A levels and risk of type 2 diabetes mellitus: a systematic review and meta-analysis. Acta Diabetol. 55, 87–98. doi: 10.1007/s00592-017-1068-9
Islam, M. A., Alam, F., Kamal, M. A., Gan, S. H., Sasongko, T. H., and Wong, K. K. (2017). Presence of anticardiolipin antibodies in patients with dementia: a systematic review and meta-analysis. Front. Aging Neurosci. 9:250. doi: 10.3389/fnagi.2017.00250
Jack, C. R., Knopman, D. S., Jagust, W. J., Petersen, R. C., Weiner, M. W., Aisen, P. S., et al. (2013). Tracking pathophysiological processes in Alzheimer's disease: an updated hypothetical model of dynamic biomarkers. Lancet Neurol. 12, 207–216. doi: 10.1016/S1474-4422(12)70291-0
Jovanovic, K., Loos, B., Da, Costa Dias, B., Penny, C., and Weiss, S. F. (2014). High resolution imaging study of interactions between the 37 kDa/67 kDa laminin receptor and APP, beta-secretase and gamma-secretase in Alzheimer's disease. PLoS ONE 9:e100373. doi: 10.1371/journal.pone.0100373
Jung, H. J., Kim, Y. J., Eggert, S., Chung, K. C., Choi, K. S., and Park, S. A. (2013). Age-dependent increases in tau phosphorylation in the brains of type 2 diabetic rats correlate with a reduced expression of p62. Exp. Neurol. 248, 441–450. doi: 10.1016/j.expneurol.2013.07.013
Kim, B., Backus, C., Oh, S., Hayes, J. M., and Feldman, E. L. (2009). Increased tau phosphorylation and cleavage in mouse models of type 1 and type 2 diabetes. Endocrinology 150, 5294–5301. doi: 10.1210/en.2009-0695
Kodl, C. T., and Seaquist, E. R. (2008). Cognitive dysfunction and diabetes mellitus. Endocr. Rev. 29, 494–511. doi: 10.1210/er.2007-0034
Kuo, S. C., Lai, S. W., Hung, H. C., Muo, C. H., Hung, S. C., Liu, L. L., et al. (2015). Association between comorbidities and dementia in diabetes mellitus patients: population-based retrospective cohort study. J. Diabetes Complications 29, 1071–1076. doi: 10.1016/j.jdiacomp.2015.06.010
Li, W., Risacher, S. L., Gao, S., Boehm, S. L., Elmendorf, J. S., and Saykin, A. J. (2018). Type 2 diabetes mellitus and cerebrospinal fluid Alzheimer's disease biomarker amyloid β1−42 in Alzheimer's Disease Neuroimaging Initiative participants. Alzheimers Dement (Amst) 10, 94–98. doi: 10.1016/j.dadm.2017.11.002
Liu, F., Shi, J., Tanimukai, H., Gu, J., Gu, J., Grundke-Iqbal, I., et al. (2009). Reduced O-GlcNAcylation links lower brain glucose metabolism and tau pathology in Alzheimer's disease. Brain 132, 1820–1832. doi: 10.1093/brain/awp099
Lu, Z., Yu, F., Yajun, L., Jianke, W., Yake, Z., and Zhiyi, L. (2011). Study on relationship between the insulin resistance with the concentration of tau protein and β-amyloid protein in cerebrospinal fluid of patients with mild cognitive impairment. Modern Prevent. Med. 38, 1774–1775.
Luchsinger, J. A., Tang, M. X., Shea, S., and Mayeux, R. (2004). Hyperinsulinemia and risk of Alzheimer disease. Neurology 63, 1187–1192. doi: 10.1212/01.WNL.0000140292.04932.87
Matioli, M., and Nitrini, R. (2015). Mechanisms linking brain insulin resistance to Alzheimer's disease. Dement. Neuropsychol. 9, 96–102. doi: 10.1590/1980-57642015D.N.92000003
McCrimmon, R. J., Ryan, C. M., and Frier, B. M. (2012). Diabetes and cognitive dysfunction. Lancet 379, 2291–2299. doi: 10.1016/S0140-6736(12)60360-2
Monette, M. C., Baird, A., and Jackson, D. L. (2014). A meta-analysis of cognitive functioning in nondemented adults with type 2 diabetes mellitus. Can. J. Diabetes 38, 401–408. doi: 10.1016/j.jcjd.2014.01.014
Moran, C., Beare, R., Phan, T. G., Bruce, D. G., Callisaya, M. L., and Srikanth, V. (2015). Type 2 diabetes mellitus and biomarkers of neurodegeneration. Neurology 85, 1123–1130. doi: 10.1212/WNL.0000000000001982
Morris, J. K., Vidoni, E. D., Honea, R. A., and Burns, J. M. (2014). Impaired glycemia increases disease progression in mild cognitive impairment. Neurobiol. Aging 35, 585–589. doi: 10.1016/j.neurobiolaging.2013.09.033
Münch, G., Westcott, B., Menini, T., and Gugliucci, A. (2012). Advanced glycation endproducts and their pathogenic roles in neurological disorders. Amino Acids 42, 1221–1236. doi: 10.1007/s00726-010-0777-y
Neth, B. J., and Craft, S. (2017). Insulin resistance and Alzheimer's disease: bioenergetic linkages. Front. Aging Neurosci. 9:345. doi: 10.3389/fnagi.2017.00345
Ouwens, D. M., van Duinkerken, E., Schoonenboom, S. N., Herzfeld, deWiza, D., Klein, M., van Golen, L., et al. (2014). Cerebrospinal fluid levels of Alzheimer's disease biomarkers in middle-aged patients with type 1 diabetes. Diabetologia 57, 2208–2214. doi: 10.1007/s00125-014-3333-6
Palta, P., Schneider, A. L., Biessels, G. J., Touradji, P., and Hill-Briggs, F. (2014). Magnitude of cognitive dysfunction in adults with type 2 diabetes: a meta-analysis of six cognitive domains and the most frequently reported neuropsychological tests within domains. J. Int. Neuropsychol. Soc. 20, 278–291. doi: 10.1017/S1355617713001483
Peila, R., Rodriguez, B. L., and Launer, L. J. (2002). Type 2 diabetes, APOE gene, and the risk for dementia and related pathologies: the Honolulu-Asia aging study. Diabetes 51, 1256–1262. doi: 10.2337/diabetes.51.4.1256
Reitz, C. (2012). Alzheimer's disease and the amyloid cascade hypothesis: a critical review. Int. J. Alzheimers Dis. 2012:369808. doi: 10.1155/2012/369808
Sa-Roriz, T. M., Rosset, I., Camozzato, A. L., Santos, A. C., Chaves, M. L., et al. (2009). (Pre)diabetes, brain aging, and cognition. Biochim. Biophys. Acta 1792, 432–443. doi: 10.1016/j.bbadis.2008.12.003
Sadanand, S., Balachandar, R., and Bharath, S. (2016). Memory and executive functions in persons with type 2 diabetes: a meta-analysis. Diabetes Metab. Res. Rev. 32, 132–142. doi: 10.1002/dmrr.2664
Sasaki, Y., Marioni, R., Kasai, M., Ishii, H., Yamaguchi, S., and Meguro, K. (2011). Chronic kidney disease: a risk factor for dementia onset: a population-based study. The Osaki-Tajiri Project. J. Am. Geriatr. Soc. 59, 1175–1181. doi: 10.1111/j.1532-5415.2011.03477.x
Serlin, Y., Levy, J., and Shalev, H. (2011). Vascular pathology and blood-brain barrier disruption in cognitive and psychiatric complications of type 2 diabetes mellitus. Cardiovasc. Psychiatry Neurol. 2011:609202. doi: 10.1155/2011/609202
Tariq, S., and Barber, P. A. (2017). Dementia risk and prevention by targeting modifiable vascular risk factors. J. Neurochem. 144, 565–581. doi: 10.1111/jnc.14132
Thambisetty, M., Jeffrey Metter, E., Yang, A., Dolan, H., Marano, C., Zonderman, A. B., et al. (2013). Glucose intolerance, insulin resistance, and pathological features of Alzheimer disease in the Baltimore longitudinal study of aging. JAMA Neurol. 70, 1167–1172. doi: 10.1001/jamaneurol.2013.284
van Duinkerken, E., Schoonheim, M. M., Ijzerman, R. G., Klein, M., Ryan, C. M., Moll, A. C., et al. (2012a). Diffusion tensor imaging in type 1 diabetes: decreased white matter integrity relates to cognitive functions. Diabetologia 55, 1218–1220. doi: 10.1007/s00125-012-2488-2
van Duinkerken, E., Schoonheim, M. M., Sanz-Arigita, E. J., IJzerman, R. G., Moll, A. C., Snoek, F. J., et al. (2012b). Resting-state brain networks in type 1 diabetic patients with and without microangiopathy and their relation to cognitive functions and disease variables. Diabetes 61, 1814–1821. doi: 10.2337/db11-1358
Westwood, S., Liu, B., Baird, A. L., Anand, S., Nevado-Holgado, A. J., Newby, D., et al. (2017). The influence of insulin resistance on cerebrospinal fluid and plasma biomarkers of Alzheimer's pathology. Alzheimers Res. Ther. 9:31. doi: 10.1186/s13195-017-0258-6
Xijiu, F. (2005). Determination of tau protein and beta-amyloid 42 in cerebrospinal fluid of cognitive handicap patients with cerebral infarction and diabetes mellitus. Chin. J. Clin. Rehabilitation 9, 92–94.
Xu, Z., Zeng, W., Sun, J., Chen, W., Zhang, R., Yang, Z., et al. (2017). The quantification of blood-brain barrier disruption using dynamic contrast-enhanced magnetic resonance imaging in aging rhesus monkeys with spontaneous type 2 diabetes mellitus. Neuroimage 158, 480–487. doi: 10.1016/j.neuroimage.2016.07.017
Keywords: Alzheimer's disease, diabetes, meta-analysis, β-amyloid, tau protein
Citation: Lu Y, Jiang X, Liu S and Li M (2018) Changes in Cerebrospinal Fluid Tau and β-Amyloid Levels in Diabetic and Prediabetic Patients: A Meta-Analysis. Front. Aging Neurosci. 10:271. doi: 10.3389/fnagi.2018.00271
Received: 23 May 2018; Accepted: 23 August 2018;
Published: 11 October 2018.
Edited by:
Eminy Hsiao-Yuan Lee, Academia Sinica, TaiwanReviewed by:
Irene HanJuo Cheng, National Yang-Ming University, TaiwanYung-Feng Liao, Academia Sinica, Taiwan
Copyright © 2018 Lu, Jiang, Liu and Li. This is an open-access article distributed under the terms of the Creative Commons Attribution License (CC BY). The use, distribution or reproduction in other forums is permitted, provided the original author(s) and the copyright owner(s) are credited and that the original publication in this journal is cited, in accordance with accepted academic practice. No use, distribution or reproduction is permitted which does not comply with these terms.
*Correspondence: Mingzi Li, limingzi@bjmu.edu.cn