- 1Department of Respiratory Medicine, Yongjia County Traditional Chinese Medicine Hospital, Wenzhou, China
- 2Department of Neurology, Ruian People’s Hospital, The Third Affiliated Hospital of Wenzhou Medical University, Wenzhou, China
- 3Department of Intervention, Ruian People’s Hospital, The Third Affiliated Hospital of Wenzhou Medical University, Wenzhou, China
Objective: In this study, we aimed to examine if patterns of CSF inflammatory markers are correlated with global cognition, episodic memory, hippocampal volume, and CSF AD-related pathologies among non-demented older people.
Methods: We included 217 non-demented older individuals, including 87 subjects with normal cognition (NC) and 130 subjects with mild cognitive impairment (MCI) from the Alzheimer’s Disease Neuroimaging Initiative (ADNI) study. Hierarchical cluster analysis including nine inflammatory markers in CSF [Tumor necrosis factor-α(TNF-α), TNF-R1, TNF-R2, transforming growth factor-β1 (TGF-β1), TGF-β2, TGF-β3, Interleukin-21 (IL-21), IL-6, and IL-7] was conducted.
Results: We identified two clusters among non-demented older people based on nine inflammatory markers in CSF. Compared to the first cluster, the second cluster showed significantly higher levels of CSF inflammatory markers (TNF-R1, TNF-R2, TGF-β1, TGF-β3, and IL-6). Further, the second cluster was also associated with higher levels of t-tau and p-tau levels in CSF.
Conclusion: We observed a subgroup of non-demented older people characterized by increased levels of inflammatory markers in CSF. Further, this subgroup showed higher levels of t-tau and p-tau levels in CSF.
Introduction
Accumulating evidence has suggested that neuroinflammation plays a pivotal role in the pathogenesis of Alzheimer’s disease (AD) (Kinney et al., 2018; Guzman-Martinez et al., 2019; Wang et al., 2019). Several genetic studies have identified links between polymorphisms in genes associated with the immune system and the risk of developing AD (Calsolaro and Edison, 2016). In addition, previous studies have also observed changed levels of a variety of cytokines in patients with AD compared to subjects with normal cognition (NC) (Song et al., 2009; Craig-Schapiro et al., 2010; Réaux-Le Goazigo et al., 2013; Bettcher and Kramer, 2014). For instance, one study found that levels of soluble tumor necrosis factor receptor 1(TNFR1) and TNFR2 in CSF were positively associated with the BACE1 activity and tau levels in CSF among non-demented subjects, and that levels of soluble TNFR1 and TNFR2 in CSF were associated with the conversion from mild cognitive impairment (MCI) to AD dementia (Buchhave et al., 2010). Interleukin 6 (IL-6) was also found to be increased in the serum and CSF of patients with AD (Blum-Degen et al., 1995; Dursun et al., 2015). Additionally, in a 10-year longitudinal study, increased levels of peripheral IL-6 were found to be associated with steeper cognitive decline in late midlife (Singh-Manoux et al., 2014). However, given the fact that levels of these inflammatory markers are highly correlated with each other, it would be difficult to entangle the true relationships between inflammatory markers and AD-related outcomes. Alternatively, a data-driven approach could be used to identify subgroups based on several inflammatory markers. After that, differences in several AD-related outcomes can be examined between subgroups. This approach allows us to examine the relationship between patterns of inflammatory markers and AD-related outcomes.
In the present study, among non-demented subjects, cluster analysis was conducted to identify subgroups based on levels of nine inflammatory markers in CSF, including TNF-α, TNF-R1, TNF-R2, transforming growth factor-β1 (TGF-β1), TGF-β2, TGF-β3, IL-21, IL-6, and IL-7. Further, we compared several AD-related markers (global cognition, episodic memory, hippocampal volume and CSF AD pathologies) between subgroups.
Materials and Methods
Alzheimer’s Disease Neuroimaging Initiative
Data utilized in this work were extracted from the Alzheimer’s Disease Neuroimaging Initiative (ADNI) database1. The ADNI study was launched in 2003 as a public-private partnership, led by Principal Investigator Michael W. Weiner, MD. The ADNI study aims to examine whether clinical and neuropsychological assessment, serial magnetic resonance imaging (MRI), positron emission tomography (PET), and other biological markers can be combined to measure the progression of MCI and early AD. In the present study, we included 217 non-demented older individuals (130 males and 87 females), including 87 subjects with NC and 130 subjects with MCI. At each ADNI center across the United States and Canada, local institutional review board approved the ADNI study, and each subject provided written informed consent.
Neuropsychological Assessments
The study participants underwent clinical and neuropsychological assessments in each ADNI center. In the present study, there were two cognitive outcomes: Mini-Mental State Examination (MMSE) (Folstein et al., 1975) and Rey Auditory Verbal Learning Test (RAVLT) (Schmidt, 1996) total learning score. These two assessments were used to examine the global cognition and verbal memory of study participants.
Measurement of Hippocampal Volume
In an effort to examine neurodegeneration we utilized hippocampal volumes. The hippocampal volumes data were extracted from the ADNI file “ADNIMERGE.csv” (accessed August 2019). The neuroimaging techniques utilized by the ADNI have been detailed previously (Risacher and Saykin, 2013). Further information on neuroimaging methods could be found at the ADNI website2. In order to control the effect of sex difference in head size, adjusted hippocampal volumes were used in the present analysis. The formula is as following: Hippocampal/intracranial volumes ratio (HpVR) = hippocampal/intracranial volumes × 103.
Measurement of Aβ42, t-Tau, and p-Tau Levels in CSF
CSF AD pathologies (Aβ42, t-tau, and p-tau proteins) were analyzed by the multiplex xMAP Luminex platform and Innogenetics/Fujirebio AlzBio3 immunoassay reagents, details of which have been described previously (Shaw et al., 2009).
Measurement of Levels of CSF Inflammatory Markers
The levels of CSF inflammatory markers (TNF-α, TNF-R1, TNF-R2, TGF-β1, TGF-β2, TGF-β3, IL-21, IL-6, and IL-7) were examined by William Hu and J. Christina Howell, Department of Neurology, Emory University. All these markers were examined by commercially available multiplex immunoassays (Millipore Sigma, Burlington, MA, United States). CSF inflammatory markers of the ADNI samples were examined in duplicate. Values are given in pg/ml.
Statistical Analysis
R statistical software (R Core Team, 2013) (version 3.6.0) was utilized to conduct an agglomerative hierarchical cluster analysis using nine CSF inflammatory proteins from 217 non-demented older people. Before clustering, these nine variables were standardized [(x-mean)/sd]. Cluster analysis could categorize participants so that participants are as similar to each other as possible within a cluster. Ward’s clustering linkage method (Ward, 1963) was utilized to combine participants while minimizing the error sum of squares. Each of the 217 non-demented older people was initially placed in their own cluster and then gradually combined with other participants. The results of the agglomerative hierarchical cluster analysis were displayed as a dendrogram (Figure 1). As a result, we identified two clusters among non-demented older people by cluster analysis. We further compared differences in demographics, clinical variables, and clustering variables between two clusters using t-tests and x2 tests. To examine whether two clusters demonstrate different levels of AD-related biomarkers (MMSE, RAVLT total learning score, HpVR, and CSF AD pathologies), t-tests were used. The significant variables (p < 0.1) identified in the t-tests were then entered in linear regression models with the adjustment of the potential effects of age, gender, educational years, clinical status, and APOE4 genotype. In addition, to examine whether gender modifies the relationship between cluster status and AD-related outcomes (MMSE, RAVLT total learning score, HpVR, and CSF AD pathologies), the clusters∗gender interaction term was added in our regression models.
Results
Cluster Analysis
In the present analysis, we identified two different clusters among non-demented older people (Figure 1). Compared to the first cluster (n = 161), the second cluster (n = 56) showed significantly higher levels of CSF TNF-R1, TNF-R2, TGF-β1, TGF-β3, and IL-6 (Table 1). However, no significant differences in CSF TNF-α, TGF-β2, IL-21, or IL-7 levels were found between two clusters (Table 1).
Demographics and Clinical Variables Between Clusters
As shown in Table 1, the first cluster were younger and more likely to be women than the second cluster. However, no differences in educational years, percentage of APOE4 carriers, or percentage of MCI individuals were found between two clusters (all p > 0.05).
AD-Related Markers Between Two Clusters
To examine whether two clusters demonstrate different levels of AD-related markers, several t tests were performed. As shown in Table 1 and Figure 2A, compared to the first cluster, the second cluster showed significantly higher levels of CSF t-tau (p = 0.03). Further, the differences in CSF p-tau levels between two clusters were marginally significant (p = 0.05; Table 1 and Figure 2B). However, no differences in MMSE, RAVLT total learning score, HpVR or CSF Aβ42 were found between two clusters (Table 1).
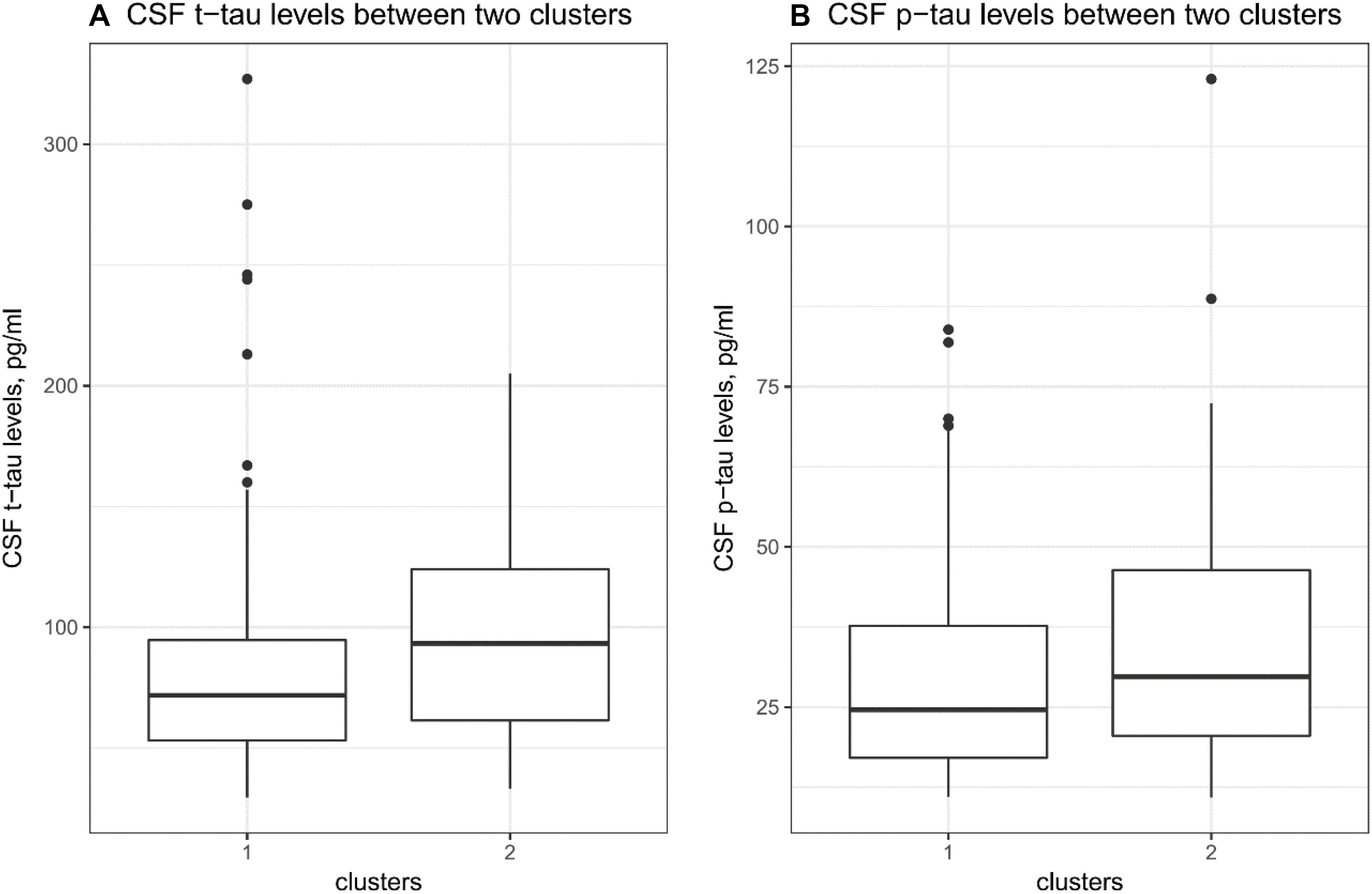
Figure 2. CSF tau pathologies between clusters. (A) There was a significant difference in CSF t-tau levels between two clusters (p = 0.03). (B) There was a marginally significant difference in CSF p-tau levels between two clusters (p = 0.05).
To further investigate the relationship between cluster status and CSF tau pathologies, several linear regression models were performed with the adjustment of several potential covariates. As shown in Table 2, compared to the first cluster, the second cluster showed significantly higher levels of CSF tau pathologies (t-tau and p-tau) after controlling for other potential covariates.
Supplementary Analysis
In addition, we further tested whether gender modifies the relationship between cluster status between each AD-related outcome (MMSE, RAVLT total learning score, HpVR, and CSF AD pathologies) in our regression models. The cluster status∗gender interaction term was added in our regression models with adjustment of other covariates (age, education, APOE4 genotype and clinical diagnosis). However, this interaction term was not significant for any AD-related outcome (all p > 0.05, MMSE, RAVLT total scores, HpVR or CSF AD biomarkers). This indicated that gender did not modify the relationship between cluster status and AD-related outcomes.
Discussion
In the present study, we observed a subgroup of non-demented older people characterized by increased levels of inflammatory markers in CSF (TNF-R1, TNF-R2, TGF-β1, TGF-β3, and IL-6). To our knowledge, this is the first study to show a subgroup of non-demented older people based on levels of nine inflammatory markers in CSF using cluster analysis. In addition, this cluster showed higher levels of t-tau and p-tau levels in CSF.
Some data-driven methods, such as cluster analysis, can categorize participants into several subgroups based on heterogeneity within a group of biomarkers. Previously, several studies have been conducted using this approach among healthy controls and patients with cognitive impairment (Iqbal et al., 2005; van der Vlies et al., 2009; Nettiksimmons et al., 2010, 2013, 2014; Wallin et al., 2010; Escudero et al., 2011; Skillbäck et al., 2013; Noh et al., 2014; Racine et al., 2016). However, no previous studies using cluster analysis have attempted to examine levels of these inflammatory markers in CSF to identify potential subgroups among non-demented older people. In this study, we observed two subgroups of non-demented older people. Cluster 1 was characterized by low levels of several inflammatory markers in CSF (TNF-R1, TNF-R2, TGF-β1, TGF-β3, and IL-6) while Cluster 2 by increased levels of these markers in CSF (Table 1). However, no significant differences in CSF TNF-α, TGF-β2, IL-21, or IL-7 levels were found between two clusters (Table 1 and Figure 1). Compared to participants in Cluster 1, those in Cluster 2 were older and had a higher percentage of men (Table 1).
Further, we found that the second cluster demonstrated higher levels of t-tau and p-tau in CSF compared to the first cluster, indicating that neuroinflammation may play a crucial role in the pathogenesis of AD. A recent meta-analysis involving 170 studies showed that levels of multiple inflammatory markers were substantially altered in comparison between AD, MCI and healthy control, also suggesting an important role of inflammation in the development of AD (Shen et al., 2019). In addition, alterations in several inflammatory markers, such as TNF-α-related cytokines levels in plasma, have been reported to be associated with cognitive decline in AD (Bettcher and Kramer, 2014; Hye et al., 2014). In line with our findings, a previous study also showed that six inflammatory markers were positively correlated with levels of t-tau and p-tau in CSF among subjects with NC and patients with cognitive impairment (Popp et al., 2017). In addition, Buchhave and colleagues found that levels of soluble TNFRs in CSF were positively correlated with CSF tau levels in healthy controls and MCI subjects (Buchhave et al., 2010). A previous animal study suggested that induction of inflammation could contribute to tau hyperphosphorylation (Kitazawa et al., 2005). On the contrary, in tau transgenic model, the early immunosuppression could lead to the reduction of tau pathology and increased lifespan (Yoshiyama et al., 2007). Taken together, previous findings and ours suggested that inflammation may play a critical role in the development of AD.
However, we did not find a significant difference in CSF Aβ42 between two clusters. This finding is consistent with a previous study showing that inflammatory markers were not correlated with CSF Aβ42 (Popp et al., 2017). In AD models, microglial inhibition can decrease neuronal loss, while it does not affect levels of amyloid-β and plague load (Spangenberg et al., 2016), indicating that the production of amyloid in AD may rely on other mechanisms.
Several limitations should be noted. First, given the cross-sectional nature of this study, we cannot clarify the temporal relationship between clusters and tau pathology in CSF among non-demented older people. Therefore, further longitudinal studies would be important to examine the association between inflammatory patterns and change in tau pathology in CSF over time. Second, given that participants of the ADNI study are dominantly white and well-educated, this may limit our findings to be generalized to other populations. Therefore, population-based studies should be conducted. Third, it would be interesting to examine the relationship between cluster status and the conversion rate from control to MCI or from MCI to AD dementia, future longitudinal studies should be conducted to examine these research questions.
In conclusion, we observed a subgroup of non-demented older people characterized by increased levels of inflammatory markers in CSF (TNF-R1, TNF-R2, TGF-β1, TGF-β3, and IL-6). Further, this subgroup showed higher levels of t-tau and p-tau levels in CSF.
Data Availability Statement
The datasets presented in this article are not readily available because we downloaded data from the ADNI study, which is open to the public (adni.loni.usc.edu). Requests to access the datasets should be directed to adni.loni.usc.edu.
Ethics Statement
The studies involving human participants were reviewed and approved by at each ADNI center across the United States and Canada, local institutional review board approved the ADNI study, and each subject provided written informed consent. The patients/participants provided their written informed consent to participate in this study.
Author Contributions
ZS and YP conceived and designed the study. YP, BC, LC, and QZ performed the research and analyzed the data. YP wrote the manuscript. All authors approved the final version of the manuscript.
Conflict of Interest
The authors declare that the research was conducted in the absence of any commercial or financial relationships that could be construed as a potential conflict of interest.
Acknowledgments
The data collection and sharing for this project was funded by the Alzheimer’s Disease Neuroimaging Initiative (ADNI) (National Institutes of Health Grant U01 AG024904) and DOD ADNI (Department of Defense award number W81XWH-12-2-0012). ADNI is funded by the National Institute on Aging, the National Institute of Biomedical Imaging and Bioengineering, and through generous contributions from the following: AbbVie, Alzheimer’s Association; Alzheimer’s Drug Discovery Foundation; Araclon Biotech; BioClinica, Inc.; Biogen; Bristol-Myers Squibb Company; CereSpir, Inc.; Cogstate; Eisai Inc.; Elan Pharmaceuticals, Inc.; Eli Lilly and Company; EuroImmun; F. Hoffmann-La Roche Ltd.; and its affiliated company Genentech, Inc.; Fujirebio; GE Healthcare; IXICO Ltd.; Janssen Alzheimer Immunotherapy Research and Development, LLC.; Johnson and Johnson Pharmaceutical Research and Development LLC.; Lumosity; Lundbeck; Merck and Co., Inc.; Meso Scale Diagnostics, LLC.; NeuroRx Research; Neurotrack Technologies; Novartis Pharmaceuticals Corporation; Pfizer Inc.; Piramal Imaging; Servier; Takeda Pharmaceutical Company; and Transition Therapeutics. The Canadian Institutes of Health Research is providing funds to support ADNI clinical sites in Canada. Private sector contributions are facilitated by the Foundation for the National Institutes of Health (www.fnih.org). The grantee organization is the Northern California Institute for Research and Education, and the study is coordinated by the Alzheimer’s Therapeutic Research Institute at the University of Southern California. ADNI data are disseminated by the Laboratory for Neuro Imaging at the University of Southern California.
Footnotes
References
Bettcher, B. M., and Kramer, J. H. (2014). Longitudinal inflammation, cognitive decline, and Alzheimer’s disease: a mini-review. Clin. Pharmacol. Ther. 96, 464–469. doi: 10.1038/clpt.2014.147
Blum-Degen, D., Müller, T., Kuhn, W., Gerlach, M., Przuntek, H., and Riederer, P. (1995). Interleukin-1 beta and interleukin-6 are elevated in the cerebrospinal fluid of Alzheimer’s and de novo Parkinson’s disease patients. Neurosci. Lett. 202, 17–20. doi: 10.1016/0304-3940(95)12192-7
Buchhave, P., Zetterberg, H., Blennow, K., Minthon, L., Janciauskiene, S., and Hansson, O. (2010). Soluble TNF receptors are associated with AÎ?? metabolism and conversion to dementia in subjects with mild cognitive impairment. Neurobiol. Aging 31, 1877–1884.
Calsolaro, V., and Edison, P. (2016). Neuroinflammation in Alzheimer’s disease: current evidence and future directions. Alzheimers Dement. 12, 719–732. doi: 10.1016/j.jalz.2016.02.010
Craig-Schapiro, R., Perrin, R. J., Roe, C. M., Xiong, C., Carter, D., Cairns, N. J., et al. (2010). YKL-40: a novel prognostic fluid biomarker for preclinical Alzheimer’s disease. Biol. Psychiatry 68, 903–912. doi: 10.1016/j.biopsych.2010.08.025
Dursun, E., Gezen-Ak, D., Hanaðası, H., Bilgiç, B., Lohmann, E., Ertan, S., et al. (2015). The interleukin 1 alpha, interleukin 1 beta, interleukin 6 and alpha-2-macroglobulin serum levels in patients with early or late onset Alzheimer’s disease, mild cognitive impairment or Parkinson’s disease. J. Neuroimmunol. 283, 50–57. doi: 10.1016/j.jneuroim.2015.04.014
Escudero, J., Zajicek, J. P., and Ifeachor, E. (2011). Early detection and characterization of Alzheimer’s disease in clinical scenarios using Bioprofile concepts and K-means. Conf. Proc. IEEE Eng. Med. Biol. Soc. 2011, 6470–6473.
Folstein, M. F., Folstein, S. E., and Mchugh, P. R. (1975). Mini-mental state”. A practical method for grading the cognitive state of patients for the clinician. J. Psychiatr. Res. 12, 189–198.
Guzman-Martinez, L., Maccioni, R. B., Andrade, V., Navarrete, L. P., Pastor, M. G., and Ramos-Escobar, N. (2019). Neuroinflammation as a common feature of neurodegenerative disorders. Front. Pharmacol. 10:1008. doi: 10.3389/fphar.2019.01008
Hye, A., Riddoch-Contreras, J., Baird, A. L., Ashton, N. J., Bazenet, C., Leung, R., et al. (2014). Plasma proteins predict conversion to dementia from prodromal disease. Alzheimers Dement. 10, 799–807.e792.
Iqbal, K., Flory, M., Khatoon, S., Soininen, H., Pirttila, T., Lehtovirta, M., et al. (2005). Subgroups of Alzheimer’s disease based on cerebrospinal fluid molecular markers. Ann. Neurol. 58, 748–757.
Kinney, J. W., Bemiller, S. M., Murtishaw, A. S., Leisgang, A. M., Salazar, A. M., and Lamb, B. T. (2018). Inflammation as a central mechanism in Alzheimer’s disease. Alzheimer’s Dement. 4, 575–590.
Kitazawa, M., Oddo, S., Yamasaki, T. R., Green, K. N., and Laferla, F. M. (2005). Lipopolysaccharide-induced inflammation exacerbates tau pathology by a cyclin-dependent kinase 5-mediated pathway in a transgenic model of Alzheimer’s disease. J. Neurosci. 25, 8843–8853.
Nettiksimmons, J., Beckett, L., Schwarz, C., Carmichael, O., Fletcher, E., and Decarli, C. (2013). Subgroup of ADNI normal controls characterized by atrophy and cognitive decline associated with vascular damage. Psychol. Aging 28, 191–201. doi: 10.1037/a0031063
Nettiksimmons, J., Decarli, C., Landau, S., and Beckett, L. (2014). Biological heterogeneity in ADNI amnestic mild cognitive impairment. Alzheimers Dement. 10, 511–521.e511.
Nettiksimmons, J., Harvey, D., Brewer, J., Carmichael, O., Decarli, C., Jack, C. R., et al. (2010). Subtypes based on cerebrospinal fluid and magnetic resonance imaging markers in normal elderly predict cognitive decline. Neurobiol. Aging 31, 1419–1428. doi: 10.1016/j.neurobiolaging.2010.04.025
Noh, Y., Jeon, S., Lee, J. M., Seo, S. W., Kim, G. H., Cho, H., et al. (2014). Anatomical heterogeneity of Alzheimer disease: based on cortical thickness on MRIs. Neurology 83, 1936–1944. doi: 10.1212/wnl.0000000000001003
Popp, J., Oikonomidi, A., Tautvydait, D., Dayon, L., Bacher, M., Migliavacca, E., et al. (2017). Markers of neuroinflammation associated with Alzheimer’s disease pathology in older adults. Brain Behav. Immun. 62, 203–211.
Racine, A. M., Koscik, R. L., Berman, S. E., Nicholas, C. R., Clark, L. R., Okonkwo, O. C., et al. (2016). Biomarker clusters are differentially associated with longitudinal cognitive decline in late midlife. Brain 139, 2261–2274. doi: 10.1093/brain/aww142
Réaux-Le Goazigo, A., Van Steenwinckel, J., Rostène, W., and Parsadaniantz, S. M. (2013). Current status of chemokines in the adult CNS. Progr. Neurobiol. 104, 67–92. doi: 10.1016/j.pneurobio.2013.02.001
Risacher, S. L., and Saykin, A. J. (2013). Neuroimaging and other biomarkers for Alzheimer’s disease: the changing landscape of early detection. Annu. Rev. Clin. Psychol. 9, 621–648. doi: 10.1146/annurev-clinpsy-050212-185535
Schmidt, M. (1996). Rey Auditory Verbal Learning Test: A Handbook. Los Angeles, CA: Western Psychological Services.
Shaw, L. M., Vanderstichele, H., Knapik-Czajka, M., Clark, C. M., Aisen, P. S., Petersen, R. C., et al. (2009). Cerebrospinal fluid biomarker signature in Alzheimer’s disease neuroimaging initiative subjects. Ann. Neurol. 65, 403–413. doi: 10.1002/ana.21610
Shen, X. N., Niu, L. D., Wang, Y. J., Cao, X. P., Liu, Q., Tan, L., et al. (2019). Inflammatory markers in Alzheimer’s disease and mild cognitive impairment: a meta-analysis and systematic review of 170 studies. J. Neurol. Neurosurg. Psychiatry 90, 590–598. doi: 10.1136/jnnp-2018-319148
Singh-Manoux, A., Dugravot, A., Brunner, E., Kumari, M., Shipley, M., Elbaz, A., et al. (2014). Interleukin-6 and C-reactive protein as predictors of cognitive decline in late midlife. Neurology 83, 486–493. doi: 10.1212/wnl.0000000000000665
Skillbäck, T., Zetterberg, H., Blennow, K., and Mattsson, N. (2013). Cerebrospinal fluid biomarkers for Alzheimer disease and subcortical axonal damage in 5,542 clinical samples. Alzheimers Res. Ther. 5:47. doi: 10.1186/alzrt212
Song, F., Poljak, A., Smythe, G. A., and Sachdev, P. (2009). Plasma biomarkers for mild cognitive impairment and Alzheimer’s disease. Brain Res. Rev. 61, 69–80.
Spangenberg, E. E., Lee, R. J., Najafi, A. R., Rice, R. A., Elmore, M. R., Blurton-Jones, M., et al. (2016). Eliminating microglia in Alzheimer’s mice prevents neuronal loss without modulating amyloid-Î?? pathology. Brain 139, 1265–1281. doi: 10.1093/brain/aww016
van der Vlies, A. E., Verwey, N. A., Bouwman, F. H., Blankenstein, M. A., Klein, M., Scheltens, P., et al. (2009). CSF biomarkers in relationship to cognitive profiles in Alzheimer disease. Neurology 72, 1056–1061. doi: 10.1212/01.wnl.0000345014.48839.71
Wallin, A. K., Blennow, K., Zetterberg, H., Londos, E., Minthon, L., and Hansson, O. (2010). CSF biomarkers predict a more malignant outcome in Alzheimer disease. Neurology 74, 1531–1537. doi: 10.1212/wnl.0b013e3181dd4dd8
Wang, H., Shen, Y., Chuang, H., Chiu, C., Ye, Y., and Zhao, L. (2019). Neuroinflammation in alzheimer’s disease: microglia, molecular participants and therapeutic choices. Curr. Alzheimer Res. 16, 659–674. doi: 10.2174/1567205016666190503151648
Ward, J. H. Jr. (1963). Hierarchical grouping to optimize an objective function. J. Am. Stat. Assoc. 58, 236–244. doi: 10.1080/01621459.1963.10500845
Keywords: Alzheimer’s disease, inflammation, cytokines, tau, cluster analysis
Citation: Peng Y, Chen B, Chi L, Zhou Q, Shi Z and The Alzheimer’s Disease Neuroimaging Initiative (2020) Patterns of CSF Inflammatory Markers in Non-demented Older People: A Cluster Analysis. Front. Aging Neurosci. 12:577685. doi: 10.3389/fnagi.2020.577685
Received: 29 June 2020; Accepted: 15 September 2020;
Published: 06 October 2020.
Edited by:
Yi Li, Weill Cornell Medicine, Cornell University, United StatesReviewed by:
Sachchida Nand Rai, University of Allahabad, IndiaScott Edward Counts, Michigan State University, United States
Copyright © 2020 Peng, Chen, Chi, Zhou, Shi and The Alzheimer’s Disease Neuroimaging Initiative. This is an open-access article distributed under the terms of the Creative Commons Attribution License (CC BY). The use, distribution or reproduction in other forums is permitted, provided the original author(s) and the copyright owner(s) are credited and that the original publication in this journal is cited, in accordance with accepted academic practice. No use, distribution or reproduction is permitted which does not comply with these terms.
*Correspondence: Zhenjing Shi, empyYXN6akAxNjMuY29t
†Data used in preparation of this article were obtained from the Alzheimer’s Disease Neuroimaging Initiative (ADNI) database (adni.loni.usc.edu). As such, the investigators within the ADNI contributed to the design and implementation of ADNI and/or provided data but did not participate in analysis or writing of this report. A complete listing of ADNI investigators can be found at: http://adni.loni.usc.edu/wp-content/uploads/how_to_apply/ADNI_Acknowledgement_List.pdf