Loss of Integrity of Corpus Callosum White Matter Hyperintensity Penumbra Predicts Cognitive Decline in Patients With Subcortical Vascular Mild Cognitive Impairment
- 1Department of Radiology, Renji Hospital, School of Medicine, Shanghai Jiao Tong University, Shanghai, China
- 2Department of Neurology, Renji Hospital, School of Medicine, Shanghai Jiao Tong University, Shanghai, China
- 3Department of Health Manage Center, Renji Hospital, School of Medicine, Shanghai Jiao Tong University, Shanghai, China
Loss of white matter (WM) integrity contributes to subcortical vascular mild cognitive impairment (svMCI). Diffusion tensor imaging (DTI) has revealed damage beyond the area of WM hyperintensity (WMH) including in normal-appearing WM (NAWM); however, the functional significance of this observation is unclear. To answer this question, in this study we investigated the relationship between microstructural changes in the WMH penumbra (WMH-P) and cognitive function in patients with svMCI by regional tract-based analysis. A total of 111 patients with svMCI and 72 patients with subcortical ischemic vascular disease (SIVD) without cognitive impairment (controls) underwent DTI and neuropsychological assessment. WMH burden was determined before computing mean values of fractional anisotropy (FA) and mean diffusivity (MD) within WMHs and WMH-Ps. Pearson’s partial correlations were used to assess the relationship between measurements showing significant intergroup differences and composite Z-scores representing global cognitive function. Multiple linear regression analysis was carried out to determine the best model for predicting composite Z-scores. We found that WMH burden in the genu, body, and splenium of the corpus callosum (GCC, BCC, and SCC respectively); bilateral anterior, superior, and posterior corona radiata; left sagittal stratum was significantly higher in the svMCI group than in the control group (p < 0.05). The WMH burden of the GCC, BCC, SCC, and bilateral anterior corona radiata was negatively correlated with composite Z-scores. Among diffusion parameters showing significant differences across the 10 WM regions, mean FA values of WMH and WMH-P of the BCC were correlated with composite Z-scores in svMCI patients. The results of the multiple linear regression analysis showed that the FA of WMH-P of the BCC and WMH burden of the SCC and GCC were independent predictors of composite Z-score, with the FA of WMH-P of the BCC making the largest contribution. These findings indicate that disruption of the CC microstructure—especially the WMH-P of the BCC—may contribute to the cognitive deficits associated with SIVD.
Introduction
Age-related cognitive impairment (CI) is a significant public health concern that will become increasingly prevalent with the aging of the global population (Iadecola et al., 2019; Kaneshwaran et al., 2019). CI of vascular etiology is the second leading cause of cognitive deficits after Alzheimer’s disease (AD) worldwide, and may be the predominant cause in East Asia (Iadecola et al., 2019; Lam and Mok, 2019). Vascular cognitive impairment (VCI) is a syndrome that encompasses a wide spectrum of cognitive disorders associated with vascular disease (Gorelick et al., 2011, 2016; van der Flier et al., 2018). Subcortical VCI (SVCI), which is attributable to cerebral small vessel disease, accounts for approximately 50%–70% of VCI cases and ranges from subcortical vascular mild cognitive impairment (svMCI) to subcortical vascular dementia (SVaD; O’Brien et al., 2003; Lee et al., 2014; Shi and Wardlaw, 2016). The mechanisms of brain injury in SVCI include vessel occlusion, leakage of toxins, impaired vascular reactivity, decreased clearance of waste products, oligodendrocyte dysfunction, increased oxidation, and inflammation (Wardlaw et al., 2013). Treatment options for SVCI are limited and disease-modifying therapies are not yet available; reliable biomarkers for early diagnosis and disease monitoring are therefore urgently needed (Fu et al., 2019; Sang et al., 2020). A prodromal stage of SVaD has been recognized based on the observation that the progression from svMCI to SVaD can be prevented by managing risk factors and through drug treatments (Seo et al., 2010; Lee et al., 2014; Jung et al., 2018).
White matter hyperintensity (WMH) is the most common and critical magnetic resonance imaging (MRI) finding of SVCI (Moran et al., 2012; Wardlaw et al., 2013), and is caused by microvessel disruption, breakdown of the blood–brain barrier, small infarcts in white matter (WM), glia activation, loss of oligodendrocytes, and demyelination caused by chronic diffuse hypoperfusion or reduced cerebral blood flow (Pantoni, 2010; De Silva and Miller, 2016). The location and extent of WMH have been linked to CI (Papp et al., 2014; Altermatt et al., 2019; Lampe et al., 2019). In a previous study, tissue damage was observed to extend from WMHs to larger adjacent areas of normal-appearing WM (NAWM; Promjunyakul et al., 2016), suggesting an ischemic mechanism underlying WMH expansion. Furthermore, changes in NAWM were found to be associated with a decline in cognitive function as determined by diffusion tensor imaging (DTI; Huang et al., 2007; Papma et al., 2014; Hirsiger et al., 2017). NAWM surrounding WMHs that can only be detected on a microstructural level is referred to as the WMH-penumbra (WMH-P; Maillard et al., 2011); neuroimaging studies have revealed damage below the detection threshold in these non-lesioned WM areas (Promjunyakul et al., 2015, 2016; Wu et al., 2019). WMH does not fully explain the neuroimaging correlates of cognitive decline in SVCI, as histopathologic alterations in the WMH-P can also lead to CI (Simpson et al., 2007; Gouw et al., 2011; Promjunyakul et al., 2015); however, as these changes are subtle, it may be possible to prevent their aggravation through early intervention.
The pathology of SVCI involves structural abnormalities in WM that contribute to a disconnection syndrome and are correlated with loss of cognitive function (López-Gil et al., 2014; Tuladhar et al., 2016). Loss of WM tract integrity in SVCI has been observed by neuroimaging. For example, microstructural damage in the anterior corpus callosum (CC), internal and external capsules, and periventricular WM has been demonstrated in SVCI by tract-based spatial statistics (TBSS; Papma et al., 2014; Holland et al., 2015; Chen et al., 2018; Wang et al., 2020), which also revealed significant associations between microstructural changes in WM tracts underlying intra-and inter-hemispheric cerebral, thalamocortical, and cerebello-thalamic connections—including the CC and corona radiata—and cognitive performance (van der Holst et al., 2018; Mascalchi et al., 2019). An automated fiber quantification (AFQ) study found that changes in diffusion characteristics—especially in the right inferior fronto-occipital fasciculus and right inferior longitudinal fasciculus—may be involved in WMH-related MCI (Chen et al., 2020). However, AFQ can only analyze the central portion of WM fiber tracts, and cannot therefore exclude the contribution of other portions to CI; moreover, it does not allow tracing of all the fiber bundles in the human brain. TBSS can only be used to analyze voxels on the skeleton with the highest fractional anisotropy (FA), which also excludes a large part of the WM (Roine et al., 2015). Additionally, neither AFQ nor TBSS can be used to detect WMH or its penumbra in fibers.
In this study, we combined region-of-interest (ROI) and tract-based analyses to explore changes in WM microstructure in svMCI and their association with cognitive function. ROI and tract-based DTI analyses allow the parcellation of WM into pathways associated with specific functions, thereby allowing disease processes to be analyzed in terms of functional systems, which is an advantage over other methods (Liu et al., 2019; Chen et al., 2020). We aimed to characterize DTI changes in WMH and WMH-P of WM fiber bundles in order to explore the relationship between progressive damage to WM microstructure and decline in cognitive performance. We hypothesized that microstructural abnormalities reflected by WMH or NAWM (WMH-P) in specific WM fibers contribute to cognitive deficits in svMCI.
Materials and Methods
Participants
This research was approved by the Research Ethics Committee of Renji Hospital, School of Medicine, Shanghai Jiao Tong University. Signed, informed consent was obtained from each subject before their enrollment. All procedures were carried out in accordance with institutional guidelines.
SIVD patients (age range: 50–88 years) were recruited from the Neurology Department of Renji Hospital between January 2017 and December 2019. SIVD was defined as the presence of a subcortical WMH lesion with at least 1 lacunar infarct by neuroimaging (Erkinjuntti et al., 2000; Galluzzi et al., 2005). The patients were divided into two groups—svMCI (n = 111) and non-CI (control, n = 72)—according to previously published criteria (Petersen et al., 1999). The inclusion criteria were subjective cognitive complaints reported by the patient or caregiver; normal activities of daily living; quantifiable CI in 1 or more domains (memory, attention-executive function, language, or visuospatial function); and no dementia. The control group had neuropsychological test scores within the normal range and was matched to the svMCI group in terms of age, sex ratio, and education level. Exclusion criteria were as follows: dementia; neurodegenerative diseases (e.g., Parkinson’s disease or AD); severe brain atrophy, intracranial space-occupying lesions, microbleeds or hemorrhage revealed by susceptibility-weighted imaging; non-SVD-related WMH mimics (e.g., multiple sclerosis); psychiatric disease interfering with cognitive testing; alcoholic encephalopathy; recent or current use of certain drugs such as acetylcholine-esterase inhibitors, L-dopa, or neuroleptic agents; and MRI contraindications or known claustrophobia. Early SVCI is characterized by deficits in executive functioning or information processing speed with relatively intact retentive memory, and is less likely to produce subjective complaints; in contrast, AD or mixed CI are associated with memory problems. Thus, participants with memory complaints were excluded. All the patients were right-handed.
Neuropsychological Assessment
All subjects underwent a battery of standardized neuropsychological evaluations carried out by an experienced neurologist (QX) within 1 week after the MRI scan. None of the subjects experienced transient ischemic attack or stroke in the interval between the MRI scan and neuropsychological testing. General cognitive function was evaluated with the Mini-Mental State Examination (MMSE) and Montreal Cognitive Assessment (MoCA). Additionally, the following tests were used to evaluate four specific cognitive domains as described in a previous study (Xu et al., 2014): (1) attention and executive function: Trail-Making Tests A and B, Stroop color-word test (Stroop C-T), and verbal fluency test; (2) visuospatial function: Rey-Osterrieth Complex Figure Test (copy); (3) language function: Boston Naming Test (30 items); and (4) memory function: auditory verbal learning test (short and long delayed free recall). The reference value (norm) used for each measurement was based on the mean score, which was determined from a small-scale pilot study conducted in Shanghai, China (Guo et al., 2007). Cognitive dysfunction was defined as −1.5 standard deviation (SD) relative to the normal value for at least 1 test item. To calculate performance on each cognitive domain, the raw score was transformed to a Z-score. Global cognitive function was represented by the composite Z-score, which was calculated as the average of Z-scores of all four cognitive domains.
MRI Data Acquisition
Subjects were scanned using a 3.0T MRI scanner (Signa HDxt; GE HealthCare, Milwaukee, WI, USA) with an eight-channel phased array head coil. Head movement was restricted by placing foam padding around the head of the subject during the scan. Three-dimensional T1 high-resolution imaging, axial fluid-attenuated inversion recovery (FLAIR), and MR DTI scans were performed for each subject. Sagittal T1-weighted images covering the whole brain were acquired with a 3D-fast spoiled gradient recalled echo (SPGR) sequence with the following parameters: repetition time (TR) = 5.6 ms, echo time (TE) = 1.8 ms, inversion time (TI) = 450 ms, flip angle = 15°, matrix = 256 × 256, number of slices = 156, slice thickness = 1.0 mm, and field of view (FOV) = 256 × 256 mm2. The parameters used to obtain axial FLAIR images were as follows: TR = 9,075 ms, TE = 150 ms, TI = 2,250 ms, matrix = 256 × 256, number of slices = 66, slice thickness = 2 mm, and FOV = 256 × 256 mm2. DTI images were acquired using a spin-echo single shot echo-planar pulse sequence with the following parameters: TR = 17,000 ms, TE = 89.8 ms, matrix = 128 × 128, number of slices = 66, slice thickness = 2 mm, gap = 0, FOV = 256 × 256 mm2, number of excitations = 1, and gap = 0. Diffusion-sensitizing gradients were applied along 20 non-collinear directions with a b value of 1,000 s/mm2, a reference image with no diffusion gradients applied (b0 scan) was also acquird.
MRI Data Processing
The T1 3D-SPGR images were uniformly segmented using Statistical Parametric Mapping 81 running on MATLAB R2014a (MathWorks, Natick, MA, USA), from which tissue maps of gray matter (GM), WM and cerebrospinal fluid (CSF) were generated in the native space. WMH maps in the native space were segmented using T1 3D-SPGR and FLAIR images as raw materials with the lesion prediction algorithm in LST Toolbox v1.1.42 (Schmidt et al., 2012). Brain volumes including total intracranial, GM, WM, CSF, and WMH volumes were calculated from T1 3D-SPGR images. Normalized WMH volume (NWMHV) was calculated as the WMH volume percentage relative to brain parenchyma volume, which was the sum of GM and WM volumes (NWMHV ‰ = measured WMH volume/brain parenchyma volume × 1,000‰).
WMH-P was defined as spatially peripheral WM tissue regions forming rings around WMH lesions and was assumed to have abnormal microstructure (de Groot et al., 2013; Nasrallah et al., 2019). To more precisely measure the WMH-P, WMH clusters were further subclassified into periventricular WMHs (PVWMH) and deep WMHs (DWMH) according to the so-called “continuity to ventricle rule” (Griffanti et al., 2018). Probabilistic maps for WMHs were processed by binarization. By linearly aligning the binary WMH map to the T1 3D-SPGR image, a NAWM layer mask for each dataset was created according to previous studies (Promjunyakul et al., 2015, 2016), which comprised 15 separate layers outside of PVWMHs and DWMHs. Each parallel layer successively dilated from the WMH by 1 mm.
DTI dataset was obtained using the PANDA v1.3.1 pipeline toolbox3, which is based on the FMRIB Software Library tools (Cui et al., 2013). The original DTI images were modified using the brain extraction tool and by eddy current-induced distortion and coregistration. FA and mean diffusivity (MD) were then simultaneously calculated with a mask created from the b0 image. FA and MD maps of each subject were first coregistered to the corresponding individual T1 3D-SPGR image using the b0 image and then smoothed with a 6 mm full width at half-maximum Gaussian kernel, then resliced into a 2 × 2 × 2 mm3 voxel-size matrix. According to the previous studies and our recent work (Promjunyakul et al., 2015, 2016; Wu et al., 2019), the size of FA and MD penumbras of PVWMH and DWMH were as follows: 6 mm for both FA and MD penumbras of PVWMH, 4 mm for the FA penumbra of DWMH, and 2 mm for the MD penumbra of DWMH. The FA and MD penumbras was gained by summing the FA or MD penumbra of PVWMH and DWMH.
We used the Johns Hopkins University Inventory of Cognitive Bias in Medicine (ICBM)-DTI-81 WM label atlas (Mori et al., 2008) as an anatomic guide to parcel the WM into 68 ROI, with each ROI representing a labeled region in the atlas. We inversely coregistered the ICBM-DTI-81 WM atlas to individual space, then calculated the WMH burden in each of the 68 WM tracts for each subject. Taking into consideration differences in brain volume across subjects, WMH burden was defined and measured as the percentage of WMH voxels in all single WM fiber bundle voxels. Thus, for each subject, all relevant images—i.e., diffusion as well as brain segmentation maps, including WMH and WMH-P maps and subject-specific WM ROI mask—were uniformly co-aligned in the individual T1 3D-SPGR space. The mean values of FA and MD in WMH and WMH-P of WM ROIs were extracted. Figure 1 depicts the data processing pipeline and results from some of the intermediate steps.
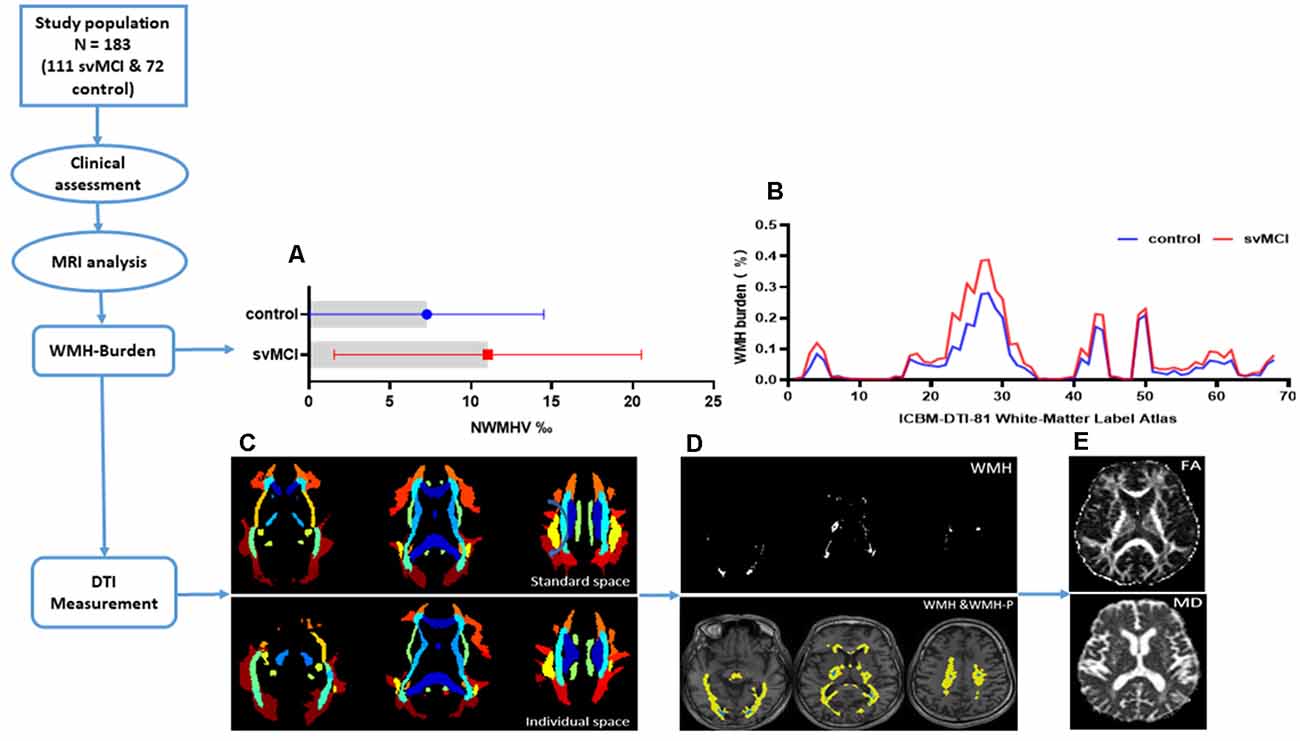
Figure 1. The flowchart showing data processing pipeline and results from some of the intermediate steps. (A) Bars showing mean and range of variation of normal-appearing white matter hyperintensity volume (NWMHV) for subcortical vascular mild cognitive impairment (svMCI) and control group. The red bar and small square stood for svMCI group while the blue bar and small circle stood for control group. (B) White matter hyperintensity (WMH) burden in each WM fiber tract based on the ICBM-DTI-81 WM label atlas. The atlas we used was a 60% probability map which included 68 WM fiber tracts in total. The red line corresponded to svMCI group and the blue line corresponded to control group. (C) ICBM-DTI-81 WM label atlas in standard (MNI) space and in individual space of one subject. (D) The WMH and WMH penumbra (WMH-P) mask image of the same subject. The white area represented for the general WMH lesion. The blue area represented for periventricular WMH (PVWMH) and deep WMH (DWMH), and the yellow area represented for penumbras surrounding either PVWMH or DWMH. (E) The fractional anisotropy (FA) and mean diffusivity (MD) map of the same subject.
Statistical Analysis
All statistical analyses were performed using SPSS v25 (IBM, Armonk, NY, USA). The Kolmogorov–Smirnov test was used to determine whether the data were normally distributed. The independent sample t-test was used to compare continuous variables and the χ2-test was used for all other variables. False discovery rate corrections were applied (q-value = 0.05). Partial correlations were used to evaluate correlations between WMH burden, DTI parameters, and composite Z-scores. A stepwise multiple linear regression model was used to predict the independent effect of each variable on composite Z-score. Variables obtained in the two steps that were strongly correlated with composite Z-score were used as predictors. Collinearity was tested using the variance inflation factor (VIF); variables with VIFs ≥5 were removed because of multicollinearity. Durbin–Watson statistics were used to detect the presence of autocorrelation. All p-value <0.05 were considered statistically significant.
Results
Demographic and Clinical Characteristics of the Study Population
There were no significant differences in age, sex ratio, or education level between svMCI and non-CI (control) groups. NWMHV was significantly higher (Figure 1A) whereas composite Z-scores and MMSE and MoCA scores were lower (Table 1) in svMCI patients than in control subjects.
WMH Burden and DTI Parameters
The svMCI group had higher WMH burden compared to control subjects in 10 fibers: the genu, body, and splenium of the CC (GCC, BCC, and SCC, respectively); bilateral anterior, superior, and posterior corona radiata (ACR, SCR, and PCR, respectively); and left sagittal stratum (all q < 0.05; Figure 2, Table 2). For the 10 WM fibers, 11 DTI parameters were found to differ significantly between groups including FA of WMH in the BCC, SCC, and right ACR; MD of WMH of right ACR; FA of WMH-P of BCC, SCC, bilateral ACR, bilateral PCR and right SCR (p < 0.05, Figure 3, Table 3). The FA values of WMH and WMH-P were lower while MD value was higher in the svMCI group compared to the control group.
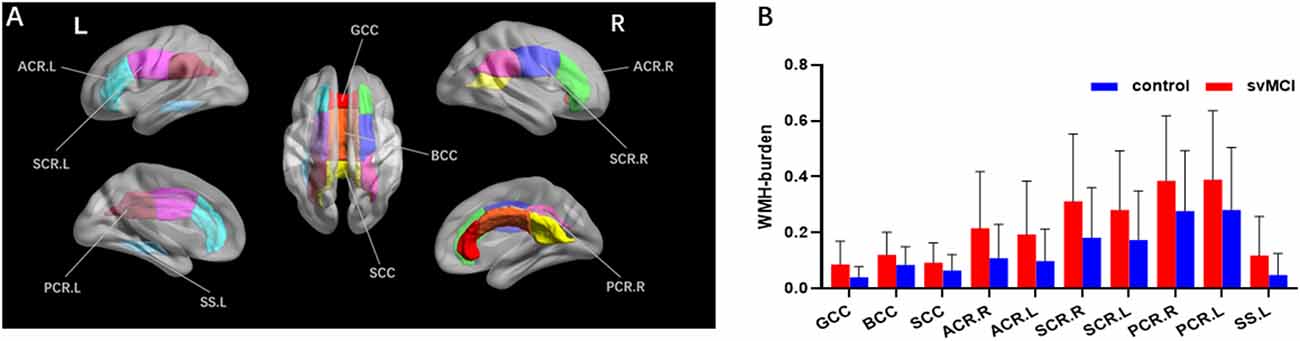
Figure 2. Results of group comparison of WMH burden. Both the visual map (A) and the column diagram (B) showed 10 WM fiber tracts altogether that had significant difference of WMH burden between svMCI and control groups. (B) The red column corresponded to svMCI group and the blue column corresponded to control group. GCC, genu of corpus callosum; BCC, body of corpus callosum; SCC, splenium of corpus callosum; ACR.R, right anterior corona radiata; ACR.L, left anterior corona radiata; SCR.R, right superior corona radiata; SCR.L, left superior corona radiata; PCR.R, right posterior corona radiata; PCR.L, left posterior corona radiata; SS.L, left sagittal stratum.
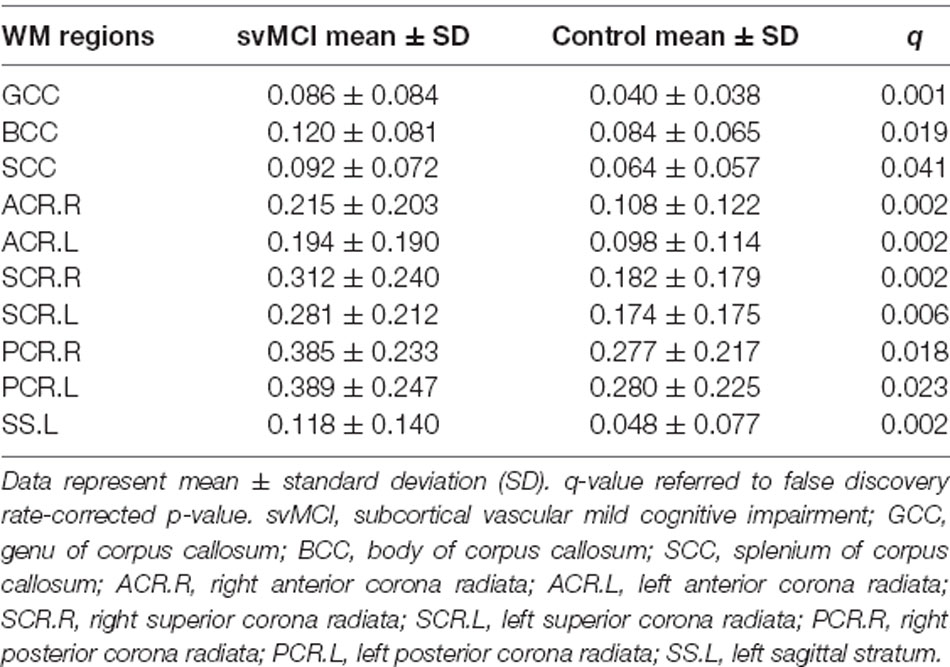
Table 2. White matter hyperintensity (WMH) burdens of WM fiber bundles that had significant differences between svMCI and control group.
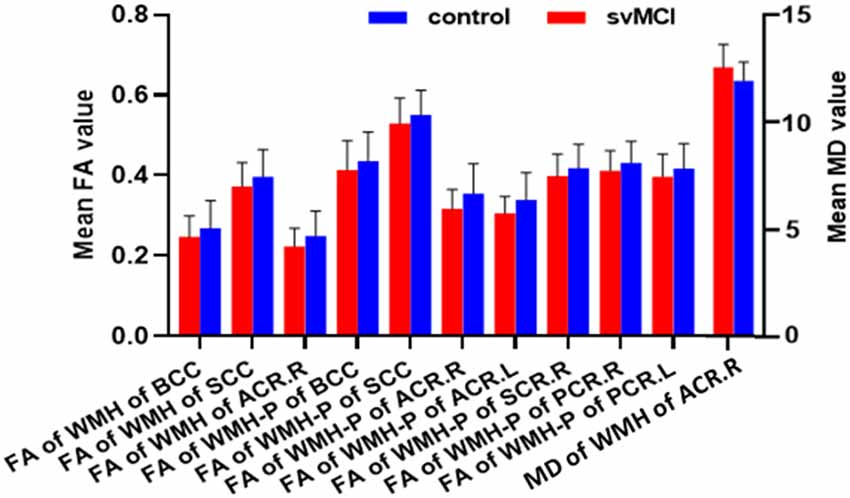
Figure 3. Results of group comparison of diffusion tensor imaging (DTI) parameters. Column diagram showing diffusion parameters based on the 10 WM fiber tracts which had significant between-group difference of WMH burden. The red column corresponded to svMCI group and the blue column corresponded to control group. The left Y-axis indicated mean FA value and the right Y-axis indicated mean MD value.
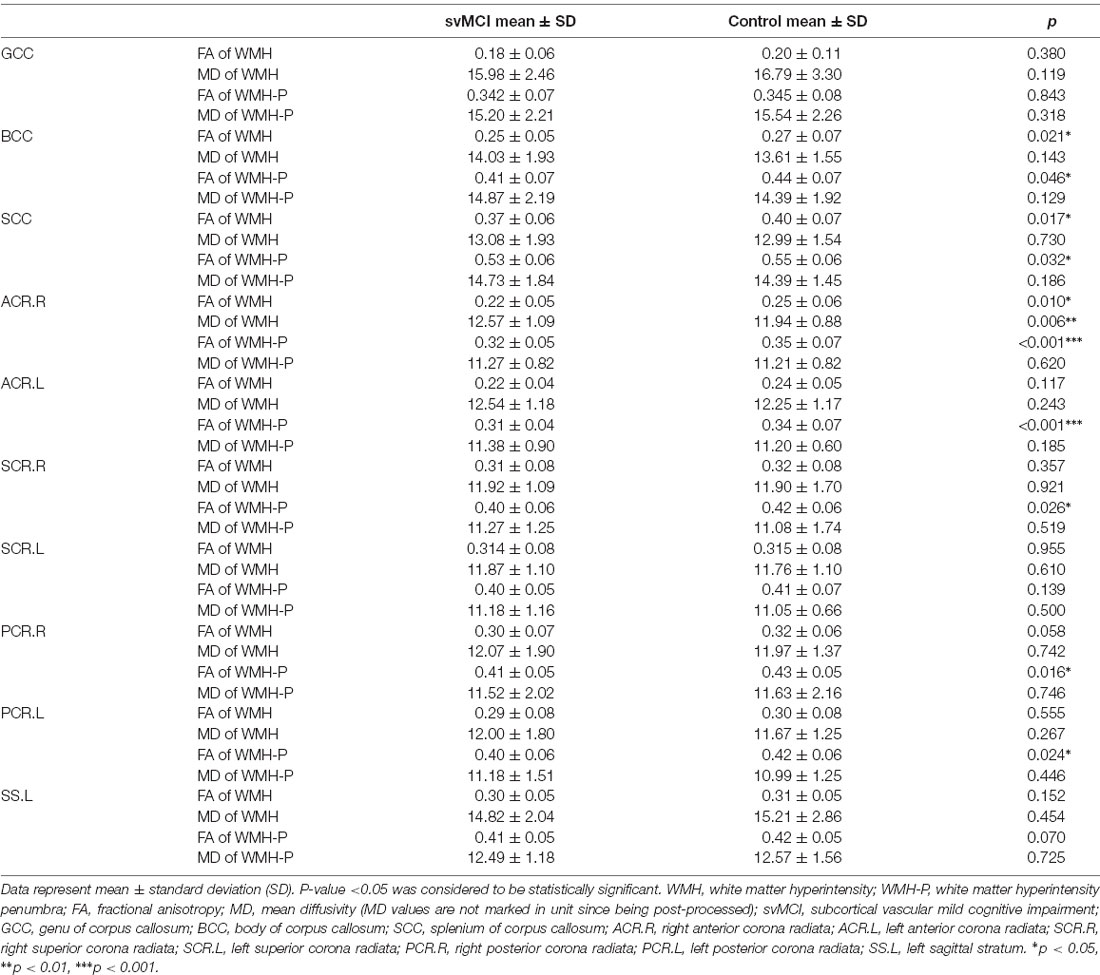
Table 3. Diffusion tensor imaging (DTI) parameter values of the WMH and WMH-P of WM fiber bundles that had significant WMH-burden differences between svMCI and control group.
Associations Between Influential Factors and General Cognitive Function
In svMCI patients, the WMH burden of the GCC, BCC, SCC, bilateral ACR, and SCR was negatively correlated with composite Z-scores (Table 4), with age, sex ratio, and education as covariates. In the svMCI group, the FA values of WMH and WMH-P in the BCC were positively correlated with composite Z-scores (Figure 4, Table 5), with age, sex ratio, education level, and corresponding WMH burden as covariates. In the control group, only the FA value of WMH in the BCC had significantly negative correlation with composite Z-score (Table 6), with age, sex ratio and education level as covariates. A regression equation was developed based on the relationship between observed and predicted values for composite Z-scores. The composite Z-score was significantly and independently associated with FA of WMH-P in the BCC, WMH burden of the SCC, and WMH burden of the GCC, with the FA of WMH-P in the BCC making the most significant contribution (Table 7).
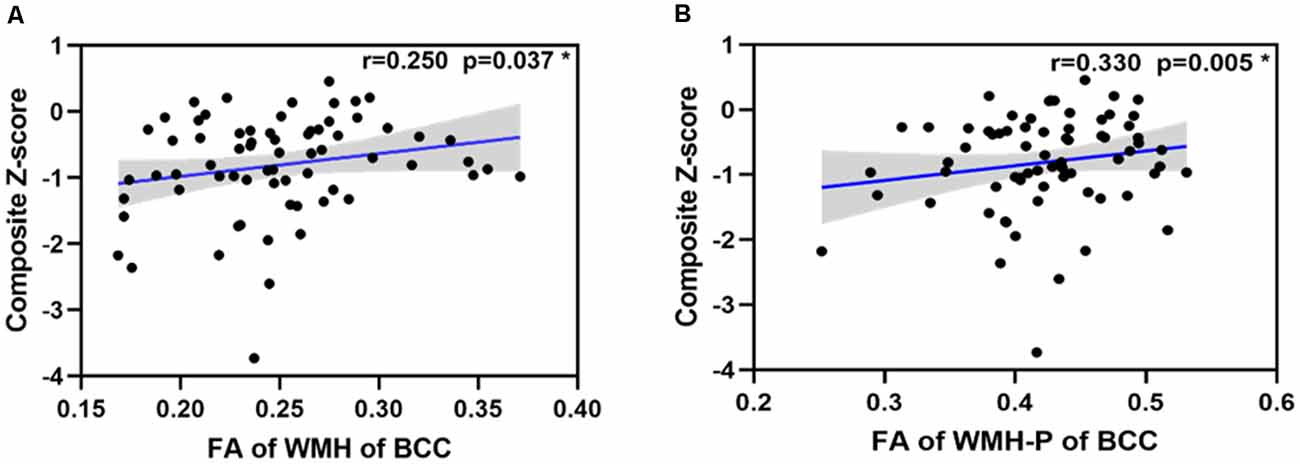
Figure 4. Scatterplot of the partial correlations of composite Z-score with DTI parameters on WM tracts (A,B). For panels (A,B), lines and shaded areas represented the correlation coefficient and each 95% confidence interval (CI), respectively.
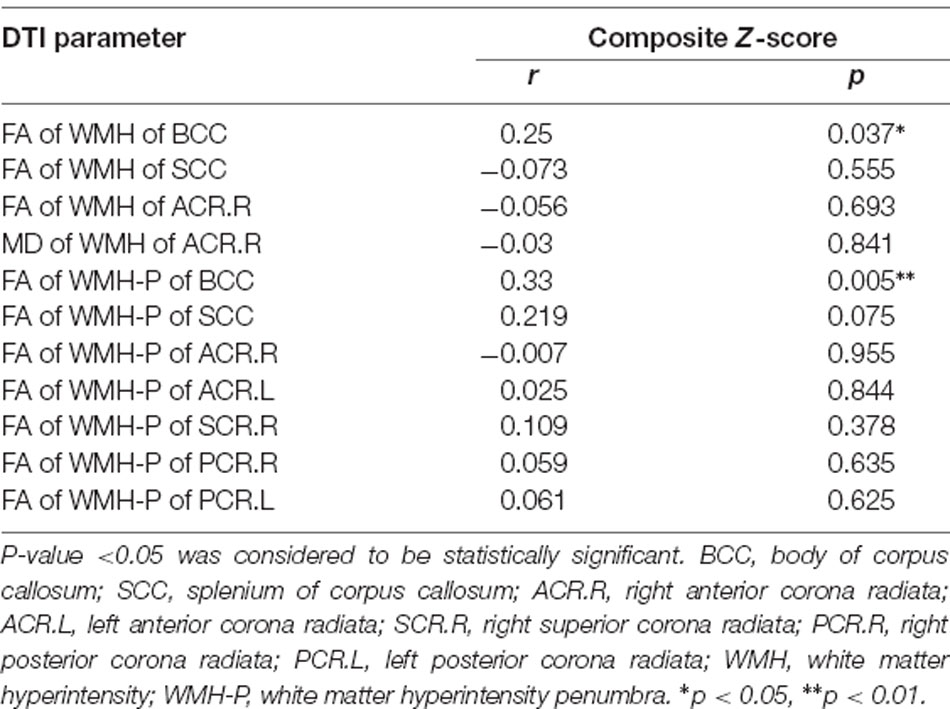
Table 5. Partial correlations between DTI parameters of WMH and WMH-P of significant WM fiber bundles and composite Z-scores in svMCI group adjusted for age, sex ratio, education level, and WMH burden.
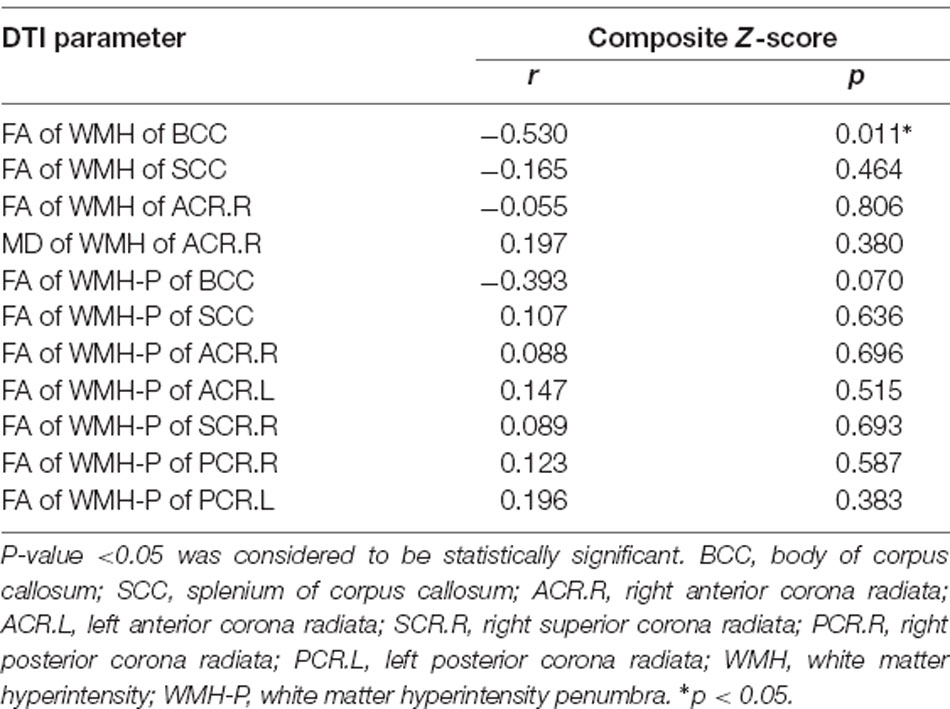
Table 6. Partial correlations between DTI parameters of WMH and WMH-P in significant WM fiber bundles and composite Z-scores in control group adjusted for age, sex ratio and education level.
Discussion
The main objective of this study was to investigate the relationship between microstructural abnormalities in WMH and NAWM (WMH-P) in specific fiber tracts and the development of CI in svMCI, which is an early stage of SVCI that has been overlooked in most previous work. Thus, our results provide prospective information that can guide early therapeutic decision-making.
We found that the WMH burden in some parts of the CC and corona radiata was significantly higher in patients with svMCI than in control subjects. FA values of WMH in the BCC, SCC, and right ACR and those of WMH-P in the BCC, SCC, bilateral ACR, right SCR, and bilateral PCR were lower whereas the MD value of WMH in the right ACR was higher in svMCI patients. Furthermore, a significant correlation between FA of WMH-P in the BCC and general cognitive function was found in svMCI patients but not in control subjects.
Consistent with previous studies (Lin et al., 2015; Tu et al., 2017; Chen et al., 2018; Liu et al., 2019; Wang et al., 2020), the strongest associations between abnormal DTI parameters and cognitive decline were found in the CC and corona radiata. As one of the most extensively myelinated brain regions, the CC is composed of fibers arising from large pyramidal neurons, with the anterior portion containing axons of corticocortical communicating fibers from corresponding posterior lobar regions (Matsunami et al., 1994; Abe et al., 2004). Fibers from these regions converge in the corona radiata, which contains projection fibers that are involved in information transmission. The critical roles of these ascending/descending pathways and inter-hemispheric connections in cognitive function can explain the CI associated with microvascular damage in the CC and corona radiata. WM tracts in the CC harboring commissural fibers connecting the frontal lobe and other cortical areas were found to be damaged at the early stage of SVCI (Reginold et al., 2016; Moscufo et al., 2018), indicating that SVCI is associated with the loss of integrity of the CC microstructure. The association between WM tract abnormalities and CI was also evidenced by the fact that SVCI lesions were present not only in certain areas but along the entire fiber structure.
The correlation between FA of WMH in the BCC and cognitive function was positive in svMCI patients and negative in control subjects. This discrepancy may be explained by the heterogeneity of pathologic changes that give rise to WMHs, which include incomplete ischemia mainly related to cerebral small vessel arteriolosclerosis; disruption of the blood-brain barrier; vasomotor reactivity, autoregulation, and endothelial dysfunction; systemic oxidative stress; inflammation; and chronic edema (Bakker et al., 1999; Pantoni, 2002; Hassan et al., 2003; Chung and Hu, 2010; Xu et al., 2010), which may coexist with tissue damage and repair. SIVD has a relatively long disease course. Although WMH progression was traditionally thought to be continuousand uniform, it is now known to be a dynamic and highly variable process that sometimes regresses (Schmidt et al., 2002; van Leijsen et al., 2017). The attenuation of WMHs is often incomplete because repair processes can be disrupted by the inhibitory responses of glial cells (Hayakawa and Lo, 2016). Thus, changes in FA values are complex and can be difficult to interpret.
Previous studies have shown that ischemic changes in the WMH-P reflect those in WMHs, with the only difference being that the former are more subtle and prodromal (Maillard et al., 2011; Jiaerken et al., 2019). We found a significant association between FA of WMH-P in the BCC and composite Z-scores in svMCI subjects but not in control subjects, underscoring the contribution of the penumbra to the cognitive deficits observed in svMCI, although the mechanistic details remain unclear.
We found that diffusion features of the WMH-P distinguished svMCI patients from control subjects, indicating that the degree of abnormality in WMH-P microstructure plays an important role in cognitive decline, possibly through a decreased connectivity caused by demyelination and axonal damage (Moscufo et al., 2018). The WMH-P represents a region of milder injury surrounding WMH lesions and has a higher probability of progressing to WMH than NAWM outside the penumbra (Maillard et al., 2011); moreover, while it is not in itself destructive, the WMH-P is a risk factor for future brain injury that presumably contributes to CI. The histopathologic origins of age-related WM degeneration include gliosis, degeneration in myelinated axons, and importantly, small vessel changes whose direct effects on WM integrity may induce the transition of WMH-P to WMH. Hypertension, diabetes, and other SVCI risk factors at their earliest stages are accompanied by progressive and subtle WM degeneration that manifests as WMH (de Leeuw et al., 2002; van Dijk et al., 2004). This is relevant to the clinical interpretation of WMH-P, which is considered as a more diffuse and incipient brain injury, and may represent a novel treatment target that, if salvaged, might modify the time course of progressive WMH and its cognitive consequences. Additionally, early treatment of reversible cardiovascular risk factors may slow the progression of WM damage in SVCI. Previous longitudinal studies found that some WMHs regress after minor stroke, with potential improvement of neurologic outcomes. Thus, WMH is reversible, which may be attributable to the transience of blood-brain barrier disruption and resultant changes in interstitial fluid (Cho et al., 2015; Ding et al., 2015; Wardlaw et al., 2017).
We also found that the MD of WMH in the right ACR was higher in svMCI patients than in control subjects, which was the only positive result for MD value. This suggests that MD is less sensitive than FA in detecting the degree of WM damage—in disagreement with previous studies on AD (Jin et al., 2017) or CSVD (Chen et al., 2020)—and that the magnitude of diffusion is a more sensitive index than the direction, possibly because microstructural changes in the chronic stage of injury reflects a combination of quantitative and directional injury caused by Wallerian degeneration.
There were several limitations to our study. First, the study design was cross-sectional and the sample size was small; longitudinal studies with a larger sample are needed in the future. Second, as with all MRI studies of svMCI we were limited by the lack of pathologically confirmed cases, although we used both neuropsychological assessment and MRI for diagnosis. Third, we excluded patients with serious brain atrophy and microbleeds but did not control for the number of lacunar infarcts, which may have confounded our analysis of the association between WMH and its penumbra and cognitive performance. In our future work, we will quantify and model this confounder to clarify the role of the WMH-P in cognitive function. Fourth, the cognitive data were combined into a composite Z-score; however, analyses of individual cognitive domains are warranted as they involve different brain regions. Finally, the field strength and resolution of the MRI could be improved by including a larger number of gradient directions for a more sensitive measure of changes in WM microstructure.
Conclusions
The significant fiber damage observed in svMCI suggests that loss of microstructural integrity of specific WM fibers—especially those of WMH-P in the BCC—contributes to cognitive deficits in patients with svMCI. These findings can aid the early diagnosis of svMCI so that timely and appropriate interventions can be initiated to slow cognitive decline in patients and reduce the progression from svMCI to SVaD.
Data Availability Statement
The data analyzed in this study is subject to the following licenses/restrictions: de-identified data are available from the corresponding author upon reasonable request subject to a material transfer agreement. Requests to access these datasets should be directed to YQ, jacobqiu1@163.com.
Ethics Statement
The studies involving human participants were reviewed and approved by Research Ethics Committee of Renji Hospital, School of Medicine, Shanghai Jiao Tong University. The patients/participants provided their written informed consent to participate in this study.
Author Contributions
YZ and YS designed the study, provided the resources, wrote, reviewed, and edited the manuscript. YQ, LY, and XG contributed to the methodology, wrote the original draft, and curated data. YQ, YW, and XW processed the data. YZ, QX, and JX reviewed the draft and manuscript. All authors contributed to the article and approved the submitted version.
Funding
This work was supported by the Shanghai Science and Technology Committee Project (Natural Science Funding; grant Nos. 20ZR1433200 and 19ZR1430500), National Natural Science Foundation of China (grant Nos. 81901693 and 82001457), the Project of Collaborative Innovation Center of Translational Medicine (TM201808), Incubating Program for Clinical Research and Innovation of Renji Hospital, School of Medicine, Shanghai Jiao Tong University (grant Nos. PYIV-17-003, PYIII-18-03, and PYIII-17-027), and Shanghai “Rising Stars of Medical Talent” Youth Development Program, Youth Medical Talents-Medical Imaging Practitioner Program [grant No. SHWRS(2020)_087].
Conflict of Interest
The authors declare that the research was conducted in the absence of any commercial or financial relationships that could be construed as a potential conflict of interest.
Footnotes
- ^ www.fil.ion.ucl.ac.uk/spm/software/spm8/
- ^ www.statistical-modeling.de/lst.html
- ^ www.nitrc.org/projects/panda
References
Abe, O., Masutani, Y., Aoki, S., Yamasue, H., Yamada, H., Kasai, K., et al. (2004). Topography of the human corpus callosum using diffusion tensor tractography. J. Comput. Assist. Tomogr. 28, 533–539. doi: 10.1097/00004728-200407000-00016
Altermatt, A., Gaetano, L., Magon, S., Bauer, L., Feurer, R., Gnahn, H., et al. (2019). Clinical associations of T2-weighted lesion load and lesion location in small vessel disease: insights from a large prospective cohort study. NeuroImage 189, 727–733. doi: 10.1016/j.neuroimage.2019.01.052
Bakker, S. L., de Leeuw, F. E., de Groot, J. C., Hofman, A., Koudstaal, P. J., and Breteler, M. M. (1999). Cerebral vasomotor reactivity and cerebral white matter lesions in the elderly. Neurology 52, 578–583. doi: 10.1212/wnl.52.3.578
Chen, H.-F., Huang, L.-L., Li, H.-Y., Qian, Y., Yang, D., Qing, Z., et al. (2020). Microstructural disruption of the right inferior fronto-occipital and inferior longitudinal fasciculus contributes to WMH-related cognitive impairment. CNS Neurosci. Ther. 26, 576–588. doi: 10.1111/cns.13283
Chen, H.-J., Gao, Y.-Q., Che, C.-H., Lin, H., and Ruan, X.-L. (2018). Diffusion tensor imaging with tract-based spatial statistics reveals white matter abnormalities in patients with vascular cognitive impairment. Front. Neuroanat. 12:53. doi: 10.3389/fnana.2018.00053
Cho, A.-H., Kim, H.-R., Kim, W., and Yang, D. W. (2015). White matter hyperintensity in ischemic stroke patients: it may regress over time. J. Stroke 17, 60–66. doi: 10.5853/jos.2015.17.1.60
Chung, C.-P., and Hu, H.-H. (2010). Pathogenesis of leukoaraiosis: role of jugular venous reflux. Med. Hypotheses 75, 85–90. doi: 10.1016/j.mehy.2010.01.042
Cui, Z., Zhong, S., Xu, P., He, Y., and Gong, G. (2013). PANDA: a pipeline toolbox for analyzing brain diffusion images. Front. Hum. Neurosci. 7:42. doi: 10.3389/fnhum.2013.00042
de Groot, M., Verhaaren, B. F., de Boer, R., Klein, S., Hofman, A., van der Lugt, A., et al. (2013). Changes in normal-appearing white matter precede development of white matter lesions. Stroke 44, 1037–1042. doi: 10.1161/STROKEAHA.112.680223
de Leeuw, F.-E., de Groot, J. C., Oudkerk, M., Witteman, J. C. M., Hofman, A., van Gijn, J., et al. (2002). Hypertension and cerebral white matter lesions in a prospective cohort study. Brain 125, 765–772. doi: 10.1093/brain/awf077
De Silva, T. M., and Miller, A. A. (2016). Cerebral small vessel disease: targeting oxidative stress as a novel therapeutic strategy? Front. Pharmacol. 7:61. doi: 10.3389/fphar.2016.00061
Ding, X., Wu, J., Zhou, Z., and Zheng, J. (2015). Specific locations within the white matter and cortex are involved in the cognitive impairments associated with periventricular white matter lesions (PWMLs). Behav. Brain Res. 289, 9–18. doi: 10.1016/j.bbr.2015.04.021
Erkinjuntti, T., Inzitari, D., Pantoni, L., Wallin, A., Scheltens, P., Rockwood, K., et al. (2000). Research criteria for subcortical vascular dementia in clinical trials. J. Neural Transm. Suppl. 59, 23–30. doi: 10.1007/978-3-7091-6781-6_4
Fu, Z., Caprihan, A., Chen, J., Du, Y., Adair, J. C., Sui, J., et al. (2019). Altered static and dynamic functional network connectivity in Alzheimer’s disease and subcortical ischemic vascular disease: shared and specific brain connectivity abnormalities. Hum. Brain Mapp. 40, 3203–3221. doi: 10.1002/hbm.24591
Galluzzi, S., Sheu, C.-F., Zanetti, O., and Frisoni, G. B. (2005). Distinctive clinical features of mild cognitive impairment with subcortical cerebrovascular disease. Dement. Geriatr. Cogn. Disord. 19, 196–203. doi: 10.1159/000083499
Gorelick, P. B., Counts, S. E., and Nyenhuis, D. (2016). Vascular cognitive impairment and dementia. Biochim. Biophys. Acta 1862, 860–868. doi: 10.1016/j.bbadis.2015.12.015
Gorelick, P. B., Scuteri, A., Black, S. E., Decarli, C., Greenberg, S. M., Iadecola, C., et al. (2011). Vascular contributions to cognitive impairment and dementia: a statement for healthcare professionals from the american heart association/american stroke association. Stroke 42, 2672–2713. doi: 10.1161/STR.0b013e3182299496
Gouw, A. A., Seewann, A., van der Flier, W. M., Barkhof, F., Rozemuller, A. M., Scheltens, P., et al. (2011). Heterogeneity of small vessel disease: a systematic review of MRI and histopathology correlations. J. Neurol. Neurosurg. Psychiatry 82, 126–135. doi: 10.1136/jnnp.2009.204685
Griffanti, L., Jenkinson, M., Suri, S., Zsoldos, E., Mahmood, A., Filippini, N., et al. (2018). Classification and characterization of periventricular and deep white matter hyperintensities on MRI: a study in older adults. NeuroImage 170, 174–181. doi: 10.1016/j.neuroimage.2017.03.024
Guo, Q., Sun, Y., and Yuan, J. (2007). Application of eight executive tests in participants at shanghai communities. Chin. J. Behav. Med. Sci. 16, 628–631. doi: 10.3760/CMA.J.ISSN.1005-8559.2007.07.022
Hassan, A., Hunt, B. J., O’Sullivan, M., Parmar, K., Bamford, J. M., Briley, D., et al. (2003). Markers of endothelial dysfunction in lacunar infarction and ischaemic leukoaraiosis. Brain 126, 424–432. doi: 10.1093/brain/awg040
Hayakawa, K., and Lo, E. H. (2016). Brain-peripheral cell crosstalk in white matter damage and repair. Biochim. Biophys. Acta 1862, 901–908. doi: 10.1016/j.bbadis.2015.08.006
Hirsiger, S., Koppelmans, V., Mérillat, S., Erdin, C., Narkhede, A., Brickman, A. M., et al. (2017). Executive functions in healthy older adults are differentially related to macro- and microstructural white matter characteristics of the cerebral lobes. Front. Aging Neurosci. 9:373. doi: 10.3389/fnagi.2017.00373
Holland, P. R., Searcy, J. L., Salvadores, N., Scullion, G., Chen, G. Q., Lawson, G., et al. (2015). Gliovascular disruption and cognitive deficits in a mouse model with features of small vessel disease. J. Cereb. Blood Flow Metab. 35, 1005–1014. doi: 10.1038/jcbfm.2015.12
Huang, J., Friedland, R. P., and Auchus, A. P. (2007). Diffusion tensor imaging of normal-appearing white matter in mild cognitive impairment and early Alzheimer disease: preliminary evidence of axonal degeneration in the temporal lobe. Am. J. Neuroradiol. 28, 1943–1948. doi: 10.3174/ajnr.A0700
Iadecola, C., Duering, M., Hachinski, V., Joutel, A., Pendlebury, S. T., Schneider, J. A., et al. (2019). Vascular cognitive impairment and dementia: JACC scientific expert panel. J. Am. Coll. Cardiol. 73, 3326–3344. doi: 10.1016/j.jacc.2019.04.034
Jiaerken, Y., Luo, X., Yu, X., Huang, P., Xu, X., Zhang, M., et al. (2019). Microstructural and metabolic changes in the longitudinal progression of white matter hyperintensities. J. Cereb. Blood Flow Metab. 39, 1613–1622. doi: 10.1177/0271678X18761438
Jin, Y., Huang, C., Daianu, M., Zhan, L., Dennis, E. L., Reid, R. I., et al. (2017). 3D tract-specific local and global analysis of white matter integrity in Alzheimer’s disease. Hum. Brain Mapp. 38, 1191–1207. doi: 10.1002/hbm.23448
Jung, N.-Y., Cho, H., Kim, Y. J., Kim, H. J., Lee, J. M., Park, S., et al. (2018). The impact of education on cortical thickness in amyloid-negative subcortical vascular dementia: cognitive reserve hypothesis. Alzheimers Res. Ther. 10:103. doi: 10.1186/s13195-018-0432-5
Kaneshwaran, K., Olah, M., Tasaki, S., Yu, L., Bradshaw, E. M., Schneider, J. A., et al. (2019). Sleep fragmentation, microglial aging and cognitive impairment in adults with and without Alzheimer’s dementia. Sci. Adv. 5:eaax7331. doi: 10.1126/sciadv.aax7331
Lam, B. Y. K., and Mok, V. C. T. (2019). Is vascular cognitive impairment more common in asia? Asian J. Psychiatr. 43, 202–204. doi: 10.1016/j.ajp.2017.03.043
Lampe, L., Kharabian-Masouleh, S., Kynast, J., Arelin, K., Steele, C. J., Löffler, M., et al. (2019). Lesion location matters: the relationships between white matter hyperintensities on cognition in the healthy elderly. J. Cereb. Blood Flow Metab. 39, 36–43. doi: 10.1177/0271678X17740501
Lee, M. J., Seo, S. W., Na, D. L., Kim, C., Park, J. H., Kim, G. H., et al. (2014). Synergistic effects of ischemia and β-amyloid burden on cognitive decline in patients with subcortical vascular mild cognitive impairment. JAMA Psychiatry 71, 412–422. doi: 10.1001/jamapsychiatry.2013.4506
Lin, L., Xue, Y., Duan, Q., Sun, B., Lin, H., Chen, X., et al. (2015). Microstructural white matter abnormalities and cognitive dysfunction in subcortical ischemic vascular disease: an atlas-based diffusion tensor analysis study. J. Mol. Neurosci. 56, 363–370. doi: 10.1007/s12031-015-0550-5
Liu, X., Cheng, R., Chen, L., Luo, T., Lv, F., Gong, J., et al. (2019). Alterations of white matter integrity in subcortical ischemic vascular disease with and without cognitive impairment: a TBSS study. J. Mol. Neurosci. 67, 595–603. doi: 10.1007/s12031-019-01266-3
López-Gil, X., Amat-Roldan, I., Tudela, R., Castañé, A., Prats-Galino, A., Planas, A. M., et al. (2014). DWI and complex brain network analysis predicts vascular cognitive impairment in spontaneous hypertensive rats undergoing executive function tests. Front. Aging Neurosci. 6:167. doi: 10.3760/cma.j.cn112138-20200421-00404
Maillard, P., Fletcher, E., Harvey, D., Carmichael, O., Reed, B., Mungas, D., et al. (2011). White matter hyperintensity penumbra. Stroke 42, 1917–1922. doi: 10.1161/STROKEAHA.110.609768
Mascalchi, M., Salvadori, E., Toschi, N., Giannelli, M., Orsolini, S., Ciulli, S., et al. (2019). DTI-derived indexes of brain WM correlate with cognitive performance in vascular MCI and small-vessel disease. A TBSS study. Brain Imaging Behav. 13, 594–602. doi: 10.1007/s11682-018-9873-5
Matsunami, K., Kawashima, T., Ueki, S., Fujita, M., and Konishi, T. (1994). Topography of commissural fibers in the corpus callosum of the cat: a study using WGA-HRP method. Neurosci. Res. 20, 137–148. doi: 10.1016/0168-0102(94)90031-0
Moran, C., Phan, T. G., and Srikanth, V. K. (2012). Cerebral small vessel disease: a review of clinical, radiological and histopathological phenotypes. Int. J. Stroke 7, 36–46. doi: 10.1111/j.1747-4949.2011.00725.x
Mori, S., Oishi, K., Jiang, H., Jiang, L., Li, X., Akhter, K., et al. (2008). Stereotaxic white matter atlas based on diffusion tensor imaging in an ICBM template. NeuroImage 40, 570–582. doi: 10.1016/j.neuroimage.2007.12.035
Moscufo, N., Wakefield, D. B., Meier, D. S., Cavallari, M., Guttmann, C. R. G., White, W. B., et al. (2018). Longitudinal microstructural changes of cerebral white matter and their association with mobility performance in older persons. PLoS One 13:e0194051. doi: 10.1371/journal.pone.0194051
Nasrallah, I. M., Hsieh, M.-K., Erus, G., Battapady, H., Dolui, S., Detre, J. A., et al. (2019). White matter lesion penumbra shows abnormalities on structural and physiologic MRIs in the coronary artery risk development in young adults cohort. Am. J. Neuroradiol. 40, 1291–1298. doi: 10.3174/ajnr.A6119
O’Brien, J. T., Erkinjuntti, T., Reisberg, B., Roman, G., Sawada, T., Pantoni, L., et al. (2003). Vascular cognitive impairment. Lancet Neurol. 2, 89–98. doi: 10.1016/s1474-4422(03)00305-3
Pantoni, L. (2002). Pathophysiology of age-related cerebral white matter changes. Cerebrovasc. Dis. 13, 7–10. doi: 10.1159/000049143
Pantoni, L. (2010). Cerebral small vessel disease: from pathogenesis and clinical characteristics to therapeutic challenges. Lancet Neurol. 9, 689–701. doi: 10.1016/S1474-4422(10)70104-6
Papma, J. M., de Groot, M., de Koning, I., Mattace-Raso, F. U., van der Lugt, A., Vernooij, M. W., et al. (2014). Cerebral small vessel disease affects white matter microstructure in mild cognitive impairment. Hum. Brain Mapp. 35, 2836–2851. doi: 10.1002/hbm.22370
Papp, K. V., Kaplan, R. F., Springate, B., Moscufo, N., Wakefield, D. B., Guttmann, C. R., et al. (2014). Processing speed in normal aging: effects of white matter hyperintensities and hippocampal volume loss. Neuropsychol. Dev. Cogn. B Aging Neuropsychol. Cogn. 21, 197–213. doi: 10.1080/13825585.2013.795513
Petersen, R. C., Smith, G. E., Waring, S. C., Ivnik, R. J., Tangalos, E. G., and Kokmen, E. (1999). Mild cognitive impairment: clinical characterization and outcome. Arch. Neurol. 56, 303–308. doi: 10.1001/archneur.56.3.303
Promjunyakul, N.-O., Lahna, D. L., Kaye, J. A., Dodge, H. H., Erten-Lyons, D., Rooney, W. D., et al. (2016). Comparison of cerebral blood flow and structural penumbras in relation to white matter hyperintensities: a multi-modal magnetic resonance imaging study. J. Cereb. Blood Flow Metab. 36, 1528–1536. doi: 10.1177/0271678X16651268
Promjunyakul, N., Lahna, D., Kaye, J. A., Dodge, H. H., Erten-Lyons, D., Rooney, W. D., et al. (2015). Characterizing the white matter hyperintensity penumbra with cerebral blood flow measures. Neuroimage Clin. 8, 224–229. doi: 10.1016/j.nicl.2015.04.012
Reginold, W., Itorralba, J., Luedke, A. C., Fernandez-Ruiz, J., Reginold, J., Islam, O., et al. (2016). Tractography at 3T MRI of corpus callosum tracts crossing white matter hyperintensities. Am. J. Neuroradiol. 37, 1617–1622. doi: 10.3174/ajnr.A4788
Roine, U., Salmi, J., Roine, T., Wendt, T. N., Leppämäki, S., Rintahaka, P., et al. (2015). Constrained spherical deconvolution-based tractography and tract-based spatial statistics show abnormal microstructural organization in asperger syndrome. Mol. Autism 6:4. doi: 10.1186/2040-2392-6-4
Sang, L., Liu, C., Wang, L., Zhang, J., Zhang, Y., Li, P., et al. (2020). Disrupted brain structural connectivity network in subcortical ischemic vascular cognitive impairment with no dementia. Front. Aging Neurosci. 12:6. doi: 10.3389/fnagi.2020.00006
Schmidt, R., Schmidt, H., Kapeller, P., Enzinger, C., Ropele, S., Saurugg, R., et al. (2002). The natural course of MRI white matter hyperintensities. J. Neurol. Sci. 203-204, 253–257. doi: 10.1016/s0022-510x(02)00300-3
Schmidt, P., Gaser, C., Arsic, M., Buck, D., Förschler, A., Berthele, A., et al. (2012). An automated tool for detection of FLAIR-hyperintense white matter lesions in multiple sclerosis. NeuroImage 59, 3774–3783. doi: 10.1016/j.neuroimage.2011.11.032
Seo, S. W., Ahn, J., Yoon, U., Im, K., Lee, J.-M., Tae Kim, S., et al. (2010). Cortical thinning in vascular mild cognitive impairment and vascular dementia of subcortical type. J. Neuroimaging 20, 37–45. doi: 10.1111/j.1552-6569.2008.00293.x
Shi, Y., and Wardlaw, J. M. (2016). Update on cerebral small vessel disease: a dynamic whole-brain disease. Stroke Vasc. Neurol. 1, 83–92. doi: 10.1136/svn-2016-000035
Simpson, J. E., Ince, P. G., Higham, C. E., Gelsthorpe, C. H., Fernando, M. S., Matthews, F., et al. (2007). Microglial activation in white matter lesions and nonlesional white matter of ageing brains. Neuropathol. Appl. Neurobiol. 33, 670–683. doi: 10.1111/j.1365-2990.2007.00890.x
Tu, M. C., Huang, W. H., Hsu, Y. H., Lo, C. P., Deng, J. F., and Huang, C. F. (2017). Comparison of neuropsychiatric symptoms and diffusion tensor imaging correlates among patients with subcortical ischemic vascular disease and Alzheimer’s disease. BMC Neurol. 17:144. doi: 10.1186/s12883-017-0911-5
Tuladhar, A. M., van Dijk, E., Zwiers, M. P., van Norden, A. G., de Laat, K. F., Shumskaya, E., et al. (2016). Structural network connectivity and cognition in cerebral small vessel disease. Hum. Brain Mapp. 37, 300–310. doi: 10.1002/hbm.23032
van der Flier, W. M., Skoog, I., Schneider, J. A., Pantoni, L., Mok, V., Chen, C. L. H., et al. (2018). Vascular cognitive impairment. Nat. Rev. Dis. Primers 4:18003. doi: 10.1038/nrdp.2018.3
van der Holst, H. M., Tuladhar, A. M., Zerbi, V., van Uden, I. W. M., de Laat, K. F., and van Leijsen, E. M. C. (2018). White matter changes and gait decline in cerebral small vessel disease. Neuroimage Clin. 17, 731–738. doi: 10.1016/j.nicl.2017.12.007
van Dijk, E. J., Breteler, M. M., Schmidt, R., Berger, K., Nilsson, L.-G., Oudkerk, M., et al. (2004). The association between blood pressure, hypertension and cerebral white matter lesions: cardiovascular determinants of dementia study. Hypertension 44, 625–630. doi: 10.1161/01.HYP.0000145857.98904.20
van Leijsen, E. M. C., de Leeuw, F.-E., and Tuladhar, A. M. (2017). Disease progression and regression in sporadic small vessel disease-insights from neuroimaging. Clin. Sci. 131, 1191–1206. doi: 10.1042/CS20160384
Wang, Z., Bai, L., Liu, Q., Wang, S., Sun, C., Zhang, M., et al. (2020). Corpus callosum integrity loss predicts cognitive impairment in leukoaraiosis. Ann. Clin. Transl. Neurol. 7, 2409–2420. doi: 10.1002/acn3.51231
Wardlaw, J. M., Chappell, F. M., Valdés, H. M. D. C., Makin, S. D. J., Staals, J., Shuler, K., et al. (2017). White matter hyperintensity reduction and outcomes after minor stroke. Neurology 89, 1003–1010. doi: 10.1212/WNL.0000000000004328
Wardlaw, J. M., Smith, C., and Dichgans, M. (2013). Mechanisms of sporadic cerebral small vessel disease: insights from neuroimaging. Lancet Neurol. 12, 483–497. doi: 10.1016/S1474-4422(13)70060-7
Wu, X., Ge, X., Du, J., Wang, Y., Sun, Y., Han, X., et al. (2019). Characterizing the penumbras of white matter hyperintensities and their associations with cognitive function in patients with subcortical vascular mild cognitive impairment. Front. Neurol. 10:348. doi: 10.3389/fneur.2019.00348
Xu, H., Stamova, B., Jickling, G., Tian, Y., Zhan, X., Ander, B. P., et al. (2010). Distinctive RNA expression profiles in blood associated with white matter hyperintensities in brain. Stroke 41, 2744–2749. doi: 10.1161/STROKEAHA.110.591875
Xu, Q., Cao, W.-W., Mi, J.-H., Yu, L., Lin, Y., and Li, Y.-S. (2014). Brief screening for mild cognitive impairment in subcortical ischemic vascular disease: a comparison study of the montreal cognitive assessment with the mini-mental state examination. Eur. Neurol. 71, 106–114. doi: 10.1159/000353988
Keywords: subcortical vascular mild cognitive impairment, white matter hyperintensities, normal-appearing white matter, penumbra, diffusion tensor imaging
Citation: Qiu Y, Yu L, Ge X, Sun Y, Wang Y, Wu X, Xu Q, Zhou Y and Xu J (2021) Loss of Integrity of Corpus Callosum White Matter Hyperintensity Penumbra Predicts Cognitive Decline in Patients With Subcortical Vascular Mild Cognitive Impairment. Front. Aging Neurosci. 13:605900. doi: 10.3389/fnagi.2021.605900
Received: 13 September 2020; Accepted: 25 January 2021;
Published: 18 February 2021.
Edited by:
Boon-Seng Wong, Singapore Institute of Technology, SingaporeReviewed by:
Matteo De Marco, The University of Sheffield, United KingdomEvan Fletcher, University of California, Davis, United States
Copyright © 2021 Qiu, Yu, Ge, Sun, Wang, Wu, Xu, Zhou and Xu. This is an open-access article distributed under the terms of the Creative Commons Attribution License (CC BY). The use, distribution or reproduction in other forums is permitted, provided the original author(s) and the copyright owner(s) are credited and that the original publication in this journal is cited, in accordance with accepted academic practice. No use, distribution or reproduction is permitted which does not comply with these terms.
*Correspondence: Yan Zhou, clare1475@hotmail.com; Qun Xu, xuqun628@hotmail.com
† These authors have contributed equally to this work