White Matter Structural Network Analysis to Differentiate Alzheimer’s Disease and Subcortical Ischemic Vascular Dementia
- 1Department of Radiology, The First Affiliated Hospital of Soochow University, Suzhou City, China
- 2Department of Geratology, The First Affiliated Hospital of Soochow University, Suzhou City, China
- 3Institute of Medical Imaging, Soochow University, Suzhou City, China
To explore the evaluation of white matter structural network analysis in the differentiation of Alzheimer’s disease (AD) and subcortical ischemic vascular dementia (SIVD), 67 participants [31 AD patients, 19 SIVD patients, and 19 normal control (NC)] were enrolled in this study. Each participant underwent 3.0T MRI scanning. Diffusion tensor imaging (DTI) data were analyzed by graph theory (GRETNA toolbox). Statistical analyses of global parameters [gamma, sigma, lambda, global shortest path length (Lp), global efficiency (Eg), and local efficiency (Eloc)] and nodal parameters [betweenness centrality (BC)] were obtained. Network-based statistical analysis (NBS) was employed to analyze the group differences of structural connections. The diagnosis efficiency of nodal BC in identifying different types of dementia was assessed by receiver operating characteristic (ROC) analysis. There were no significant differences of gender and years of education among the groups. There were no significant differences of sigma and gamma in AD vs. NC and SIVD vs. NC, whereas the Eg values of AD and SIVD were statistically decreased, and the lambda values were increased. The BC of the frontal cortex, left superior parietal gyrus, and left precuneus in AD patients were obviously reduced, while the BC of the prefrontal and subcortical regions were decreased in SIVD patients, compared with NC. SIVD patients had decreased structural connections in the frontal, prefrontal, and subcortical regions, while AD patients had decreased structural connections in the temporal and occipital regions and increased structural connections in the frontal and prefrontal regions. The highest area under curve (AUC) of BC was 0.946 in the right putamen for AD vs. SIVD. White matter structural network analysis may be a potential and promising method, and the topological changes of the network, especially the BC change in the right putamen, were valuable in differentiating AD and SIVD patients.
Introduction
According to a report from the World Health Organization in 2017, almost 50 million people have been diagnosed with dementia, and the number is said to increase to 82 million by 2030 (The Lancet Neurology, 2018). Dementia creates a heavy financial burden on society. Alzheimer’s disease (AD) is a progressive and degenerative disease resulting in cognitive impairment and behavior dysfunction. Vascular dementia (VaD) due to various vascular pathologies is the second most common cause of dementia after AD (Kang et al., 2016). AD and VaD accounts for approximately 60% and 20% of dementia, respectively (Rizzi et al., 2014). Subcortical ischemic vascular dementia (SIVD) accounts for a large part of VaD (Benjamin et al., 2016). SIVD has been focused on due to its high prevalence.
It is hard to identify SIVD and AD clinically, due to the similar neuropsychological symptoms between them. The main issues for SIVD patients are executive and semantic memory dysfunction (Palesi et al., 2018). Previous studies demonstrated that the cognitive impairment of SIVD was related to the disconnection of the frontal subcortical circuit (Seo et al., 2010). The progression of SIVD was reversible as the vascular risk factors of SIVD were controllable. However, AD patients mostly displayed diffuse cortex atrophy and the progress was irreversible (McDonald et al., 2009).
The microstructure of the human brain has been explored in vivo by neuroimaging in recent years. Many studies have proved that the cognitive impairment of SIVD resulted from a lesion in the white matter (Chen et al., 2018). Diffusion tensor imaging (DTI) was acknowledged as a precise MRI method, which is sensitive to the microstructural change of white matter. In the past couple of decades, the topological network has received great attention in neuroscience study. The network is comprised of information of relations or interconnections that link many elements in the neurobiological system. The neurons of the human brain are interconnected through synaptic connections and anatomical projections mediate the communication among brain areas, forming a highly complex network system in human beings (Bassett and Sporns, 2017; Kuang et al., 2020). Structural network analysis based on DTI is a newly-developed method to reflect the topological structural alterations and the connectivity of the brain structural network (Bassett et al., 2017). The graph theory method, a branch of mathematics, could offer important new insights into the structure and function of brain network systems including their architecture, evolution, and development (Sporns, 2018).
DTI has been applied in many studies to explore white matter changes in patients with AD and SIVD by voxel- or tract-based methods (Chen et al., 2018; Palesi et al., 2018; Liu et al., 2019). However, the human brain is a complicated and interconnected network balancing regional segregation and the specialization of function with strong integration (Zhou et al., 2020). The clinical syndromes of AD and SIVD, such as executive dysfunction and memory deficits, might result from abnormal structural connections. Due to the analogical clinical manifestation of AD and SIVD, it is hard to identify them by only relying on clinical performances. Brain network analysis may help researchers understand cerebral microstructure change, and may further elucidate the etiology of cognitive and behavioral deficits in SIVD patients. In the present study, we aim to explore brain structural network alterations in AD and SIVD patients, and to explore the value of brain structural network analysis in the differentiation of AD and SIVD.
Materials and Methods
Participants
A total of 67 right-handed Chinese Han subjects [19 SIVD, 31 AD, and 17 normal control (NC)] were enrolled in this study at the Department of Radiology in the First Affiliated Hospital of Soochow University from June 2018 to June 2020. This study was approved by the ethics committees of the First Affiliated Hospital of Soochow University, and written informed consent was obtained from each subject prior to participation. All subjects underwent a comprehensive neuropsychological test and 3.0 Tesla MRI scanning of the whole brain. The cognitive functions of all the subjects were evaluated by an experienced neuropsychologist. General cognitive function of participants was evaluated using the Beijing version of the Montreal cognitive assessment (MoCA) and the mini mental state examination (MMSE; Lu et al., 2011). Episodic memory function was assessed by the auditory verbal learning test Huashan version (AVLT) including the auditory verbal learning test immediate recall (AVLT-IR) and the auditory verbal learning test delayed recall (AVLT-DR; Zhao et al., 2012). Executive function was assessed by the Stroop color-word test. The speed (Stroop test 1) and the accuracy (Stroop test 2) of performance were measured.
Included SIVD patients met the following criteria (Román et al., 2002; Liu et al., 2014; Kang et al., 2016): (1) displayed moderate and severe white matter hyperintensities (WMH). The severity of WMH was evaluated according to the modified Fazekas ischemia criteria. WMH were assessed separately as periventricular white matter hyperintensities (PWMH) and deep white matter hyperintensities (DWMH) in T2 axial or fluid-attenuated inversion recovery (FLAIR) images. DWMH were classified into D1 (<10 mm), D2 (≥10 mm <25 mm), or D3 (≥25 mm) based on the longest diameter of lesions. PWMH were split into P1 (cap and band < 5 mm), P2 (≥5 mm, <10 mm), or P3 (cap or band ≥10 mm) based on the maximum length, which were perpendicular and horizontal to the ventricle, respectively. These two ratings were combined as minimal (D1P1, D1P2), moderate (D1P3, D2P1, D2P2, D2P3, D3P1, D3P2), or severe (D3P3); (2) lacunar cases: multiple lacunas (>5) in the deep gray matter and at least moderate white matter lesions; and (3) with a MoCA score less than 26.
The inclusion criteria for AD patients referred to the National Institute on Aging-Alzheimer’s Association Criteria (McKhann et al., 2011). Besides, AD patients in our study also met following criteria: (1) absence of WMH or mild severity of WMH by T2 FLAIR image; and (2) with a MoCA score less than 26.
According to the score of MoCA, the AD patients and SIVD patients were subdivided into mild cognitive impairment (18≤MoCA< 26) and dementia (MoCA≤17).
NC were age and gender-matched healthy volunteers: (1) without clinical evidence or history of cognitive disfunction with MoCA≥26; (2) without brain abnormality detected on a routine non-contrast MRI examination; and (3) no neuropsychological disorders.
The exclusion criteria for all participants were as follows: (1) metabolic conditions, such as hypothyroidism or folic acid deficiencies; (2) a history of stroke; (3) central nervous system diseases that could cause cognitive decline, such as Parkinson’s disease, epilepsy, multiple sclerosis and so on; and (4) with MRI scanning contraindications.
Image Acquisition
All MRI examinations were performed using a 3.0 T MRI scanner (Signa HDxt, GE Healthcare, Milwaukee, WI, USA) with an eight-channel head coil. A three-dimensional fast spoiled gradient recalled (3D-FSPGR) sequence was performed with the following parameters: repetition time (TR) 6.50 ms, echo time (TE) 2.80 ms, inversion time (TI) 900 ms, flip angle 8°, field of view (FOV) 256 × 256 mm, number of slices 176, slice thickness 1 mm without slice gap, and scan time 4 min. DTI data were obtained using an echo planar imaging (EPI) sequence with the following parameters: TR 17,000 ms, TE 85.4 ms, flip angle 90°, matrix size 128 × 128, FOV 256 × 256 mm, slice thickness 2 mm without slice gap, number of signal averages (NEX) 2, with 30 non-collinear directions of diffusion encoding (b = 1,000 s/mm2 for each direction), and a scan time of 9 min. Additionally, axial T2-weighted and FLAIR sequences were obtained to detect visible white matter damage.
Data Processing
The PANDA toolbox based on FMRIB Software Library v5.0 was applied in tha DTI data process (Cui et al., 2013), containing several steps (brain extraction, DTI images format conversion, realignment, eddy current and motion artifact correction, fractional anisotropy (FA) calculation, and diffusion tensor tractography). When tracking white matter fibers, a fractional anisotropy (FA) value threshold of 0.2 and a turning angle threshold of 45° of the Fiber Assignment by Continuous Tracking (FACT) algorithm were set (Basser et al., 2000).
The Anatomical Automatic Labeling (AAL) atlas was used to parcellate each brain into 90 regions of interest (ROIs). The nodes of the structural network were defined according to the AAL template. Interconnections between brain regions were taken as the edges of the structural network. If the number of interconnected white matter fibers was more than 3, an edge in the structural network was defined. The global topological parameters including the small-world [gamma, sigma, lambda, and global shortest path length (Lp)], global efficiency (Eg), and local efficiency (Eloc) were obtained by the GRETNA toolbox (Wang et al., 2015). Eg indicates how efficiency information is exchanged over the whole network. Eloc calculates clustering and specialization within a network and the fault tolerance of the network. Lp, a measurement of the average nodal shortest path length, reflects the speed information transfer to the whole brain. Sigma, the ratio of gamma and lambda, is a measurement of the small-world property of the network. In addition, the betweenness centrality (BC) of a node was measured to describe nodal characteristics of the white matter structural network. BC is the fraction of all shortest paths in the network that pass through a given node.
Statistical Analysis
Demographics and Clinical Variables
All statistical analyses were performed using the Statistical Product and Service Software (SPSS ver. 20.0, Chicago, IL, USA). The normality of distribution of continuous variables was examined by the Kolmogorov–Smirnov test. The differences of age and years of education among groups were analyzed by the Kruskal–Wallis H test. The differences of MMSE, MoCA, AVLT-IR, AVLT-DR, and Stroop tests 1 and 2 among groups were analyzed by general linear modeling, with some effects for covariates (age, gender, and years of education); and Bonferroni correction was used to adjust p values in multiple comparisons. The distribution of cognitive severity between AD patients and SIVD patients were analyzed by the χ2 test. The group differences of categorical variables were analyzed by the Pearson test when the sample size was over 40 and the minimal expected frequency was over 5. Otherwise, the correction formula of chi-squared test would be chosen; and the R×C table was used when the dependent variable was over 2. A p value less than 0.05 was considered statistically significant and continuous variables were reported as mean ±SD.
Comparison of Topographic Network Parameters Among Groups
Statistical analyses of global and nodal parameters were performed with the gretna toolbox. Between the two groups, global network parameters and BC of each node were compared by a two-sample t-test with FDR correction and with age, gender, and years of education as covariates. For global and nodal parameters, a p-value less than 0.001 was considered statistically significant. In order to evaluate the diagnostic accuracy of BC in nodes with group differences in identifying AD patients and SIVD patients, the receiver operating characteristic (ROC) analysis was performed and the area under curve (AUC) was calculated by MedCalc (MedCalc statistical software, ver.15.8).
White Matter Structural Connectome Comparison Between Groups
Network-based statistical analysis (NBS), a methodology improving the statistical power though controlling the type I error, was applied to identify the change of structural connections in AD patients vs. NC and SIVD patients vs. NC. Statistical comparisons in NBS were conducted with age, sex, and years of education as covariates. A p-value less than 0.05 was considered statistically significant.
Results
Demographic and Neuropsychological Characteristics
The demographic information and neuropsychological performance of AD and SIVD patients are shown in Table 1. As shown in Table 1, there were no significant differences of gender and years of education among the three groups. Compared with NC, the scores of MoCA, MMSE, AVLT-IR, and AVLT-DR of AD and SIVD groups were significantly decreased. When AD patients compared with SIVD patients and NC compared with SIVD patients, Stroop tests 1 and 2 of SIVD patients were significantly reduced. However, there were no significant differences in scores of MMSE, MoCA, AVLT-IR, and AVLT-DR in AD patients vs. SIVD patients. There were no significant differences in the scores of Stroop tests 1 and 2 between AD patients and NC. There were respectively 19 AD patients and 14 SIVD patients with dementia and there was no statistical difference in the distribution of severity of cognitive dysfunction between AD and SIVD patients (p = 0.369).
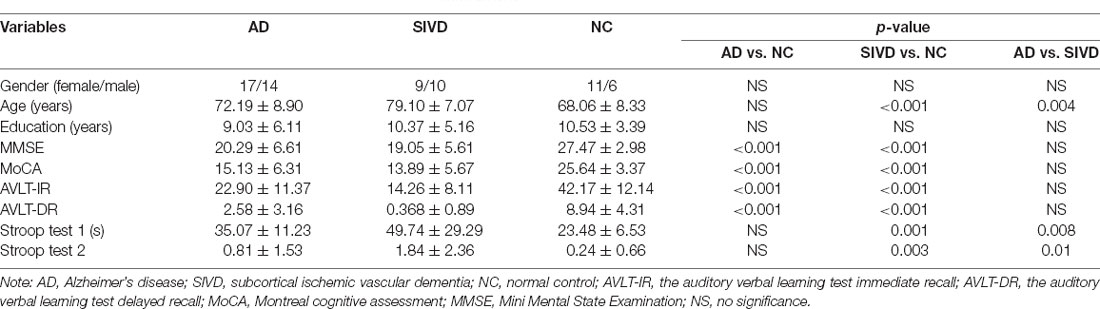
Table 1. The group difference of clinical and neuropsychological data among AD, SIVD patients, and NC.
Between-Group Difference in the Global Parameters of the Network
All the subjects performed small-world organization (sigma>1) in the DTI structural network. As shown in Table 2, there were no statistical significances in sigma and gamma when the AD group was compared with NC and the SIVD group was compared with NC. The Eg of AD patients and SIVD patients were statistically decreased and their lambda values were increased. The Eloc of the SIVD group was significantly reduced in SIVD patients vs. NC. Moreover, the lambda of SIVD patients was also increased when SIVD patients were compared with AD patients. SIVD patients showed significantly increased Lp when the SIVD group was compared with NC and the SIVD group was compared with the AD group. Compared with AD patients, the Egand Eloc in SIVD patients were reduced.
Between-Group Difference in Nodal Parameters
When AD patients were compared with NC, the BC of prefrontal cortices such as the left inferior frontal gyrus (orbital part) and left superior frontal gyrus (medial part) in the AD group were decreased significantly. Besides, AD patients had significantly decreased BC in the right rolandic operculum, right postcentral, left superior parietal gyrus, and left precuneus (Table 3; Figure 1A). Compared with NC, SIVD patients demonstrated decreased BC mainly in prefrontal regions, such as the right superior frontal gyrus, right superior frontal gyrus (orbital part), right inferior frontal gyrus (opercular part), and left anterior cingulate gyrus, as well as in the right putamen and right superior occipital gurus (Table 4 and Figure 2A).

Table 3. The group difference of nodal betweenness centrality in AD vs. NC and the diagnostic efficiency of the nodes in the differentiation between groups.
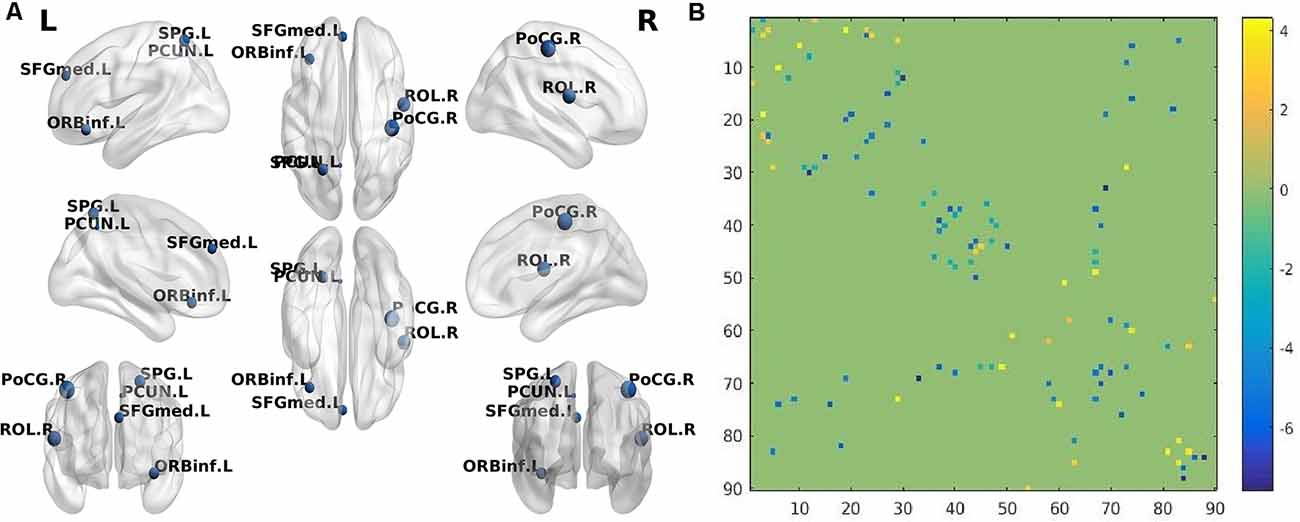
Figure 1. (A) The brain regions (nodes) visualized by BrainNet Viewer (NKLCNL, Beijing Normal University) demonstrate significantly different betweenness centrality (BC) when Alzheimer’s disease (AD) was compared with normal control (NC; p < 0.001, FDR-corrected). ORBinf.L: left frontal_inf_orb; ROL.R: right rolandic_oper; SFGmed.L: left frontal_sup_medial; PoCG.R: right postcentral; SPG.L: left parietal_sup; PCUN.L: left precuneus. (B) The structural connectivity alterations between the AD and NC groups. Horizontal axis and vertical axis respectively represent 90 brain regions in the automated anatomical labeling (AAL) template. The dots in different locations in the matrix represent the structural connectivity between the two different brain regions identified by the horizontal axis and vertical axis. The colors of the dots represent structural connection strength, quantified by the values on the right of the color bar. The dot color with a positive value represents increased structural connection between the two brain regions in the AD group, compared with the NC group, while the dot color with a negative value represents a decreased structural connection.

Table 4. The group difference of nodal betweenness centrality in SIVD vs. NC and the diagnostic efficiency of the nodes in the differentiation.
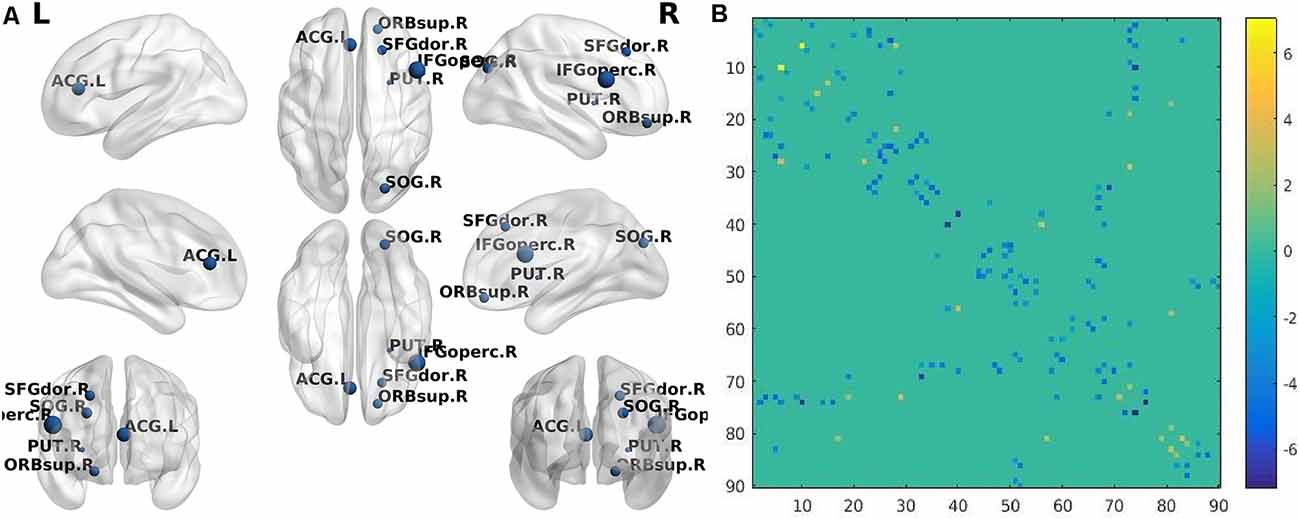
Figure 2. (A) The brain regions (nodes) visualized by BrainNet Viewer (NKLCNL, Beijing Normal University) demonstrate significantly different betweenness centrality (BC) when subcortical ischemic vascular dementia (SIVD) was compared with NC (p < 0.001, FDR-corrected). SFGdor.R: right frontal_sup; ORBsup.R: right frontal_sup_orb; IFGoperc.R: right frontal_inf_oper; ACG.L: left cingulum_ant; SOG.R: right occipital_sup; PUT.R: right putamen. (B) The structural connectivity alterations between the SIVD and NC groups. Horizontal axis and vertical axis respectively represent 90 brain regions in the automated anatomical labeling (AAL) template. The dots in different locations in the matrix represent the structural connectivity between the two different brain regions identified by horizontal axis and vertical axis. The colors of the dots represent structural connection strength, quantified by the values on the right of the color bar. The dot color with a positive value represents increased structural connection between the two brain regions in the SIVD group, compared with the NC group, while the dot color with a negative value represents a decreased structural connection.
Between-Group Difference in Structural Connectivity
Compared with NC, the AD group demonstrated 41 significantly decreased structural connections and 18 increased structural connections. The decreased structural connections of AD patients mainly involved temporal and occipital regions, while approximately 50% of increased structural connections involved frontal and prefrontal regions (Figure 1B). When SIVD patients were compared with NC, SIVD patients manifested 80 significantly decreased structural connections and 13 increased structural connections. Structural connections between frontal and prefrontal regions were significantly decreased in SIVD patients. Additionally, the structural connections between frontal-subcortical regions and prefrontal-subcortical regions were also decreased (Figure 2B).
Discriminative Power of BC Among AD, SIVD, and NC Groups
The AUC of BC in significantly different cortices between AD patients and NC ranged from 0.766 to 0.935. As shown in Table 3; the AUC of BC in the left precuneus was 0.935 which was the highest. When SIVD was compared with NC, the AUC of BC in right putamen was 0.974 which was the highest (Table 4). As shown in Table 5; the AUC of BC in significantly different nodes (from the SIVD group vs. the NC group and the AD group vs. the NC group) in identifying AD patients and SIVD patients ranged from 0.599 to 0.946; and the AUC of BC in the right putamen was 0.946 which was the highest (Table 5).
Discussion
According to the present study, executive dysfunction was significantly different between the SIVD and AD groups. SIVD patients performed worse in the Stroop color-word tests than AD patients. SIVD patients spent more time on Stroop test 1 (lower speed) and naming/reading errors (lower accuracy) occurred more frequently than in AD patients, as shown in Table 1. It was indicated that the executive function of SIVD patients was more seriously damaged. Subcortical vascular pathology which interrupted frontal-striatal circuits was frequently present in SIVD patients. Executive dysfunction in SIVD patients may result from the disruption of cortical and subcortical connections (O’Brien and Thomas, 2015; Tuladhar et al., 2015). Many studies suggested that memory deficit was more obvious in AD patients. However, the SIVD group performed worse than the AD group in the present study (Graham et al., 2004; Reed et al., 2007). Liu et al. (2019) found that memory loss existed in the deteriorated process of SIVD patients and memory decreased with cognitive deterioration. Moreover, there were studies that proved that memory deterioration was correlated with the overall severity of dementia (Kang et al., 2016). Thus, the discrepancy supposedly resulted from the impartial distribution of global cognitive severity.
DTI and the graph theory method were applied to explore the structural network alteration of AD and SIVD patients. In the present study, AD and SIVD patients and NC performed small-world properties. Small-world organization, reflecting an optimal balance of integration and segregation, appears to be a ubiquitous organization of anatomical connectivity (Bassett and Bullmore, 2006; Zhou et al., 2020). Sigma is a measurement of small-world property in the structural network (Sun et al., 2017). The decreased sigma in the structural network indicates a less optimal topological structure. Although there were no significant differences in sigma between AD and NC and SIVD and NC, it did not indicate that the small-world topological properties of AD and SIVD were normal. Because sigma was calculated by dividing gamma by lambda, the sigma value could be affected both by the gamma value and lambda value. Lambda is the ratio of the characteristic path length between real and 100 random networks, which quantifies the overall routing efficiency of a network (Zhang et al., 2019). The lambda values of SIVD and AD patients were obviously increased in the present study. It was suggested that the network integration in SIVD and AD patients was disrupted. Eg and Eloc respectively measures the ability of parallel information transmission over the network and the fault tolerance of the network (Zhang et al., 2019). The Eg and Elocin SIVD patients and Eg in AD patients were significantly reduced compared with NC, which indicated that global integration of the structural network was disrupted and information processing was impaired in the AD and SIVD groups (Bai et al., 2012). Lp, a measurement of the average of the nodal shortest path length, reflects the speed of information transfer to the whole brain (Li et al., 2017). Significantly increased Lp in SIVD indicated the disrupted global integration of the structural network. When SIVD patients compared with AD patients, SIVD patients performed lower Eg, Eloc and higher Lp, lambda, suggesting that the destruction of white matter structure in the SIVD group was worse than that in the AD group.
BC is the fraction of all shortest paths in the network that pass through a given node (Fortanier et al., 2019). Decreased nodal BC means reduced nodal importance in the network. Compared with NC, AD patients demonstrated significantly reduced BC in the left precuneus. A previous study found that inappropriate atrophy existed in the precuneus in AD patients and the precuneus played an important role in the default mode network (Abu-Akel and Shamay-Tsoory, 2011; Hojjati et al., 2018). Besides the left precuneus, AD patients showed decreased BC in parietal and prefrontal cortices, such as the right postcentral, left superior parietal gyrus, right rolandic operculum, left inferior frontal gyrus (orbital part), and left superior frontal gyrus (medial part). Some studies suggested that atrophy of the AD brain may initiate in the inferior parietal cortices and spread to the prefrontal cortices (McDonald et al., 2009). These structural alterations may be linked to the corresponding BC changes in these regions and illuminate one of the underlying reasons of cognitive dysfunction in AD patients.
Executive dysfunction is the main clinical symptom of SIVD patients which may be correlated with the integrity of prefrontal-subcortical circuits (Román et al., 2002). Compared with NC, the BC of the left anterior cingulate gyrus in SIVD patients was significantly decreased in the present study. The cingulum, a complex structure that interconnects the frontal, parietal, and medial temporal regions (Bubb et al., 2018), plays an important role in executive function and episodic memory. The impairment of the cingulum may result in an executive dysfunction and memory deficit in SIVD patients. Additionally, the BC was decreased in some prefrontal cortices such as the right superior frontal gyrus, right superior frontal gyrus (orbital part), and right inferior frontal gyrus (opercular part), as well as in the right putamen and right superior occipital gyrus in SIVD patients. Some researchers suggested that the volume of the putamen was smaller and that the occipital gyrus was thinner in SIVD patients (Seo et al., 2010; Thong et al., 2014). The occipital gyrus is the main origin and destination of long-association fibers, among which the inferior fronto-occipital fasciculus plays an important role in attention and visual processing. Therefore, alterations in the structural occipital gyrus may lead to function deficits in attention and visual processing (Catani and Thiebaut De Schotten, 2008).
There were some differing main brain regions involved in the structural network in AD and SIVD patients. It was suggested that the left precuneus was vulnerable to damage in AD patients while the right putamen was vulnerable to damage in SIVD patients in the present study. The prefrontal and frontal cortices and subcortical regions were the main regions affected in the SIVD group which was consistent with a previous study (Yi et al., 2012; Thong et al., 2014). As to the diagnostic efficiency of nodal BC in differentiating SIVD and AD patients, the AUC of the right putamen was 0.946 which was the highest. Therefore, the change of BC in the right putamen could identify and distinguish SIVD patients and AD patients.
Structural connections in frontal-prefrontal regions, frontal-subcortical regions, and prefrontal-subcortical regions were reduced in SIVD patients in the present study. Previous studies demonstrated decreased frontal-subcortical connections in SIVD patients (Sang et al., 2020), which was in line with the present study. Besides, the structural connections between prefrontal and frontal regions were reduced which supposedly resulted in their damage in SIVD patients. AD patients mostly manifested decreased structural connections in the temporal and occipital regions and increased connections in the frontal and prefrontal regions in the present study. In the study of McDonald et al. (2009), AD patients with mild cognitive impairment suffered from atrophy of the temporal and occipital cortices. Structural alterations in the temporal and occipital cortices may explain the decreased structural connections in these regions which are related to function deficits. However, increased structural connections in the frontal and prefrontal regions could potentially compensate for function deficits in AD patients.
As discussed above, although most of the conclusions from the present study were similar with those from previous studies, there were still many new findings. First, the structure of the precuneus was deconstructed in AD patients, which illuminated part of underlying reasons for cognitive dysfunction, while AD patients had increased prefrontal-frontal structural connections, which were supposed to compensate for function deficits. Second, the structure of occipital cortices were impaired in SIVD patients, which pertained to the function deficits of attention and visual processes. Finally, the BC change of the right putamen had the highest AUC in the ROC analysis, suggesting that it was suitable to differentiate between SIVD patients and AD patients.
There were several limitations in the present study. First, the sample size of the SIVD group was quite small, which needs to be enlarged in future studies. Second, the intra-individual and intra-group differences in global cognition were not considered sufficiently. Although there were no statistical differences in MMSE and MoCA scores between AD and SIVD patients, and there was no statistical difference in the distribution of severity of cognitive dysfunction between AD and SIVD patients, the confounding factor of the severity of cognitive impairment existed. AD and SIVD patients need to be further subdivided in a future study. Third, the AD patients were recruited by clinical criteria which had not been proved by pathology.
Conclusion
White matter structural network analysis including the topological changes of the network, especially the BC change in the right putamen may be a potential and promising method for differentiating AD and SIVD patients.
Data Availability Statement
The raw data supporting the conclusions of this article will be made available by the authors, without undue reservation.
Ethics Statement
The studies involving human participants were reviewed and approved by the ethics committees of the First Affiliated Hospital of Soochow University. The patients/participants provided their written informed consent to participate in this study.
Author Contributions
HD and YW designed this study. MF, YL, ZW, ZS, and MM collected the patients’ data. The cognitive functions of all the subjects were evaluated by YZ. Each author participated in writing the article. Each author gave final agreement to be accountable for all aspects of the work in ensuring that questions related to the accuracy or integrity of any part of the work are appropriately investigated and resolved. All authors contributed to the article and approved the submitted version.
Funding
This work was partially supported by the National Natural Science Foundation of China (grant number 81971573), the Suzhou Gusu Medical Youth Talent (grant number GSWS2020019), the Project of Invigorating Health Care through Science, Technology and Education, Jiangsu Provincial Medical Youth Talent (grant number QNRC2016709), and Jiangsu Province Cadre Health Project (grant number BJ19008).
Conflict of Interest
The authors declare that the research was conducted in the absence of any commercial or financial relationships that could be construed as a potential conflict of interest.
References
Abu-Akel, A., and Shamay-Tsoory, S. (2011). Neuroanatomical and neurochemical bases of theory of mind. Neuropsychologia 49, 2971–2984. doi: 10.1016/j.neuropsychologia.2011.07.012
Bai, F., Shu, N., Yuan, Y., Shi, Y., Yu, H., and Wu, D. (2012). Topologically convergent and divergent structural connectivity patterns between patients with remitted geriatric depression and amnestic mild cognitive impairment. J. Neurosci. 32, 4307–4318. doi: 10.1523/JNEUROSCI.5061-11.2012
Basser, P. J., Pajevic, S., Pierpaoli, C., Duda, J., and Aldroubi, A. (2000). in vivo fiber tractography using DT-MRI data. Magn. Reson. Med. 44, 625–632. doi: 10.1002/1522-2594(200010)44:4<625::aid-mrm17>3.0.co;2-o
Bassett, D. S., Khambhati, A. N., and Grafton, S. T. (2017). “Emerging frontiers of neuroengineering: a network science of brain connectivity,” in Annual Review of Biomedical Engineering, ed M. L. Yarmush (Palo Alto, CA: Annual Reviews), 327–352.
Bassett, D. S., and Bullmore, E. T. (2006). Small-world brain networks. Neuroscientist 12, 512–523. doi: 10.1177/1073858406293182
Bassett, D. S., and Sporns, O. (2017). Network neuroscience. Nat. Neurosci. 20, 353–364. doi: 10.1038/nn.4502
Benjamin, P., Zeestraten, E., Lambert, C., Ster, I. C., Williams, O. A., and Lawrence, A. J. (2016). Progression of MRI markers in cerebral small vessel disease: sample size considerations for clinical trials. J. Cereb. Blood Flow. Meteb. 36, 228–240. doi: 10.1038/jcbfm.2015.113
Bubb, E. J., Metzler-Baddeley, C., and Aggleton, J. P. (2018). The cingulum bundle: anatomy, function, and dysfunction. Neurosci. Biobehav. Rev. 92, 104–127. doi: 10.1016/j.neubiorev.2018.05.008
Catani, M., and Thiebaut De Schotten, M. (2008). A diffusion tensor imaging tractography atlas for virtual in vivo dissections. Cortex 44, 1105–1132. doi: 10.1016/j.cortex.2008.05.004
Chen, H.-J., Gao, Y.-Q., Che, C.-H., Lin, H., and Ruan, X.-L. (2018). Diffusion tensor imaging with tract-based spatial statistics reveals white matter abnormalities in patients with vascular cognitive impairment. Front. Neuroanat. 12:53. doi: 10.3389/fnana.2018.00053
Cui, Z., Zhong, S., Xu, P., He, Y., and Gong, G. (2013). PANDA: a pipeline toolbox for analyzing brain diffusion images. Front. Hum. Neurosci. 7:42. doi: 10.3389/fnhum.2013.00042
Fortanier, E., Grapperon, A., Le Troter, A., Verschueren, A., Ridley, B., and Guye, M. (2019). Structural connectivity alterations in amyotrophic lateral sclerosis: a graph theory based imaging study. Front. Neurosci. 13:1044. doi: 10.3389/fnins.2019.01044
Graham, N. L., Emery, T., and Hodges, J. R. (2004). Distinctive cognitive profiles in Alzheimer’s disease and subcortical vascular dementia. J. Neurol. Neurosurg. Psychiatry 75, 61–71. doi: 10.1016/s0084-3970(08)70286-x
Hojjati, S. H., Ebrahimzadeh, A., Khazaee, A., and Babajani-Feremi, A. (2018). Predicting conversion from MCI to AD by integrating rs-fMRI and structural MRI. Comput. Biol. Med. 102, 30–39. doi: 10.1016/j.compbiomed.2018.09.004
Kang, H. S., Kwon, J. H., Kim, S., Na, D. L., Kim, S. Y., and Lee, J. (2016). Comparison of neuropsychological profiles in patients with Alzheimer’s disease and mixed dementia. J. Neurol. Sci. 369, 134–138. doi: 10.1016/j.jns.2016.08.022
Kuang, L., Gao, Y., Chen, Z., Xing, J., Xiong, F., and Han, X. (2020). White matter brain network research in Alzheimer’s disease using persistent features. Molecules 25:2472. doi: 10.3390/molecules25112472
Li, X., Steffens, D. C., Potter, G. G., Guo, H., Song, S., and Wang, L. (2017). Decreased between-hemisphere connectivity strength and network efficiency in geriatric depression. Hum. Brain Mapp. 38, 53–67. doi: 10.1002/hbm.23343
Liu, X., Cheng, R., Chen, L., Luo, T., Lv, F., and Gong, J. (2019). Alterations of white matter integrity in subcortical ischemic vascular disease with and without cognitive impairment: a TBSS study. J. Mol. Neurosci. 67, 595–603. doi: 10.1007/s12031-019-01266-3
Liu, C., Li, C., Yin, X., Yang, J., Zhou, D., and Gui, L. (2014). Abnormal intrinsic brain activity patterns in patients with subcortical ischemic vascular dementia. PLoS One 9:e87880. doi: 10.1371/journal.pone.0087880
Lu, J., Li, D., Li, F., Zhou, A., Wang, F., and Zuo, X. (2011). Montreal cognitive assessment in detecting cognitive impairment in chinese elderly individuals: a population-based study. J. Geriatr. Psychiatry Neurol. 24, 184–190. doi: 10.1177/0891988711422528
McDonald, C. R., McEvoy, L. K., Gharapetian, L., Fennema-Notestine, C. Jr., Hagler, D. J., and Holland, D. (2009). Regional rates of neocortical atrophy from normal aging to early Alzheimer disease. Neurology 73, 457–465. doi: 10.1212/WNL.0b013e3181b16431
McKhann, G. M., Knopman, D. S., Chertkow, H., Hyman, B. T. Jr., Jack, C. R., and Kawas, C. H. (2011). The diagnosis of dementia due to Alzheimer’s disease: Recommendations from the National Institute on Aging-Alzheimer’s Association workgroups on diagnostic guidelines for Alzheimer’s disease. Alzheimers Dement. 7, 263–269. doi: 10.1016/j.jalz.2011.03.005
O’Brien, J. T., and Thomas, A. (2015). Vascular dementia. Lancet 386, 1698–1706. doi: 10.1016/S0140-6736(15)00463-8
Palesi, F., De Rinaldis, A., Vitali, P., Castellazzi, G., Casiraghi, L., and Germani, G. (2018). Specific patterns of white matter alterations help distinguishing Alzheimer’s and vascular dementia. Front. Neurosci. 12:274. doi: 10.3389/fnins.2018.00274
Reed, B. R., Mungas, D. M., Kramer, J. H., Ellis, W., Vinters, H. V., and Zarow, C. (2007). Profiles of neuropsychological impairment in autopsy-defined Alzheimer’s disease and cerebrovascular disease. Brain 130, 731–739. doi: 10.1093/brain/awl385
Rizzi, L., Rosset, I., and Roriz-Cruz, M. (2014). Global epidemiology of dementia: Alzheimer’s and vascular types. Biomed Res. Int. 2014:908915. doi: 10.1155/2014/908915
Román, G. C., Erkinjuntti, T., Wallin, A., Pantoni, L., and Chui, H. C. (2002). Subcortical ischaemic vascular dementia. Lancet Neurol. 1, 426–436. doi: 10.1016/s1474-4422(02)00190-4
Sang, L., Liu, C., Wang, L., Zhang, J., Zhang, Y., and Li, P. (2020). Disrupted brain structural connectivity network in subcortical ischemic vascular cognitive impairment with no dementia. Front. Aging Neurosci. 12:6. doi: 10.3389/fnagi.2020.00006
Seo, S. W., Ahn, J., Yoon, U., Im, K., Lee, J. M., and Tae, K. S. (2010). Cortical thinning in vascular mild cognitive impairment and vascular dementia of subcortical type. J. Neuroimaging 20, 37–45. doi: 10.1111/j.1552-6569.2008.00293.x
Sporns, O. (2018). Graph theory methods: applications in brain networks. Dialogues Clin. Neurosci. 20, 111–120. doi: 10.31887/DCNS.2018.20.2/osporns
Sun, Y., Chen, Y., Collinson, S. L., Bezerianos, A., and Sim, K. (2017). Reduced hemispheric asymmetry of brain anatomical networks is linked to schizophrenia: a connectome study. Cereb. Cortex 27, 602–615. doi: 10.1093/cercor/bhv255
The Lancet Neurology. (2018). Response to the growing dementia burden must be faster. Lancet Neurol. 17:651. doi: 10.1016/S1474-4422(18)30256-4
Thong, J. Y. J., Du, J., Ratnarajah, N., Dong, Y., Soon, H. W., and Saini, M. (2014). Abnormalities of cortical thickness, subcortical shapes and white matter integrity in subcortical vascular cognitive impairment. Hum. Brain Mapp. 35, 2320–2332. doi: 10.1002/hbm.22330
Tuladhar, A. M., van Norden, A. G. W., de Laat, K. F., Zwiers, M. P., van Dijk, E. J., and Norris, D. G. (2015). White matter integrity in small vessel disease is related to cognition. Neuroimage Clin. 7, 518–524. doi: 10.1016/j.nicl.2015.02.003
Wang, J., Wang, X., Xia, M., Liao, X., Evans, A., and He, Y. (2015). GRETNA: a graph theoretical network analysis toolbox for imaging connectomics (vol 9, 386, 2015). Front. Hum. Neurosci. 9:458. doi: 10.3389/fnhum.2015.00458
Yi, L., Wang, J., Jia, L., Zhao, Z., Lu, J., and Li, K. (2012). Structural and functional changes in subcortical vascular mild cognitive impairment: a combined voxel-based morphometry and resting-State fMRI study. PLoS One 7:e44758. doi: 10.1371/journal.pone.0044758
Zhang, Y., Cao, Y., Xie, Y., Liu, L., Qin, W., and Lu, S. (2019). Altered brain structural topological properties in type 2 diabetes mellitus patients without complications. J. Diabetes 11, 129–138. doi: 10.1111/1753-0407.12826
Zhao, Q., Lv, Y., Zhou, Y., Hong, Z., and Guo, Q. (2012). Short-term delayed recall of auditory verbal learning test is equivalent to long-term delayed recall for identifying amnestic mild cognitive impairment. PLoS One 7:e51157. doi: 10.1371/journal.pone.0051157
Keywords: Alzheimer’s disease, subcortical ischemic vascular dementia, diffusion tensor imaging, structural network analysis, graph theory method
Citation: Feng M, Zhang Y, Liu Y, Wu Z, Song Z, Ma M, Wang Y and Dai H (2021) White Matter Structural Network Analysis to Differentiate Alzheimer’s Disease and Subcortical Ischemic Vascular Dementia. Front. Aging Neurosci. 13:650377. doi: 10.3389/fnagi.2021.650377
Received: 07 January 2021; Accepted: 25 February 2021;
Published: 31 March 2021.
Edited by:
Mingxia Liu, University of North Carolina at Chapel Hill, United StatesReviewed by:
Erkun Yang, University of North Carolina at Chapel Hill, United StatesMingliang Wang, Nanjing University of Information Science and Technology, China
Yongsheng Pan, Northwestern Polytechnical University, China
Copyright © 2021 Feng, Zhang, Liu, Wu, Song, Ma, Wang and Dai. This is an open-access article distributed under the terms of the Creative Commons Attribution License (CC BY). The use, distribution or reproduction in other forums is permitted, provided the original author(s) and the copyright owner(s) are credited and that the original publication in this journal is cited, in accordance with accepted academic practice. No use, distribution or reproduction is permitted which does not comply with these terms.
*Correspondence: Hui Dai, huizi198208@126.com; Yueju Wang, wangyueju@suda.edu.cn