Sharpening Working Memory With Real-Time Electrophysiological Brain Signals: Which Neurofeedback Paradigms Work?
- 1Lexington Veteran Affairs Medical Center, Lexington, KY, United States
- 2College of Medicine, University of Kentucky, Lexington, KY, United States
- 3Department of Mechanical, Aerospace, and Biomedical Engineering, University of Tennessee, Knoxville, Knoxville, TN, United States
- 4New Mexico Veteran Affairs Medical Center, Albuquerque, NM, United States
Growing evidence supports the idea that the ultimate biofeedback is to reward sensory pleasure (e.g., enhanced visual clarity) in real-time to neural circuits that are associated with a desired performance, such as excellent memory retrieval. Neurofeedback is biofeedback that uses real-time sensory reward to brain activity associated with a certain performance (e.g., accurate and fast recall). Working memory is a key component of human intelligence. The challenges are in our current limited understanding of neurocognitive dysfunctions as well as in technical difficulties for closed-loop feedback in true real-time. Here we review recent advancements of real time neurofeedback to improve memory training in healthy young and older adults. With new advancements in neuromarkers of specific neurophysiological functions, neurofeedback training should be better targeted beyond a single frequency approach to include frequency interactions and event-related potentials. Our review confirms the positive trend that neurofeedback training mostly works to improve memory and cognition to some extent in most studies. Yet, the training typically takes multiple weeks with 2–3 sessions per week. We review various neurofeedback reward strategies and outcome measures. A well-known issue in such training is that some people simply do not respond to neurofeedback. Thus, we also review the literature of individual differences in psychological factors e.g., placebo effects and so-called “BCI illiteracy” (Brain Computer Interface illiteracy). We recommend the use of Neural modulation sensitivity or BCI insensitivity in the neurofeedback literature. Future directions include much needed research in mild cognitive impairment, in non-Alzheimer’s dementia populations, and neurofeedback using EEG features during resting and sleep for memory enhancement and as sensitive outcome measures.
Significance of Working Memory and Improvement
Most of us have experienced the “tip of the tongue” feeling of not quite recalling a name, a face, or items from memory. As part of normal brain and cognitive aging, such “senior moments” increase, especially under stress. The lure of brain training is the possibility to directly enhance neural activities associated with good memory and therefore reduce the moments of memory lapses. A central component of cognitive ability is working memory, i.e., the capacity to hold active information in one’s memory for immediate manipulation. By definition, working memory shares a large amount with other core cognitive functions (e.g., cognitive control and attention), which are strongly associated with performance in intelligence tests (Chen et al., 2019). Measurements of neural activity have become strong predictors of mild cognitive impairments in persons with various kinds of cognitive deficits. Electrophysiological changes during working memory are some of the earliest signals in preclinical risk of mild cognitive impairment (Jiang et al., 2021).
Neurofeedback (NF) is biofeedback that uses real-time sensory reward for brain activity associated with certain performance (e.g., accurate and fast recall). In contrast, traditional cognitive rehabilitation typically applies offline behavioral reward (e.g., money). Growing evidence supports the idea that the ultimate biofeedback is to reward with sensory pleasure (e.g., enhanced visual clarity) in real-time to neural circuits that are associated with a desired performance, such as excellent memory (YuLeung To et al., 2016) and attention (deBettencourt et al., 2015, 2019). Using functional neuroimaging, brain regions that have been consistently implicated in neurofeedback or real-time self-modulation include the anterior insula (cognitive control, self-awareness), and the basal ganglia (sensory motor integration, implicit learning), independent of the targeted region-of-interest (Emmert et al., 2016). There are different networks involved in neurofeedback in cognitive and affective functions (Trambaiolli et al., 2021); this is seen particularly in fMRI-based studies that allow better spatial definition of the structures involved during cognition and affective processes (Paret et al., 2019; Skottnik et al., 2019; Direito et al., 2021).
Why Focus on Real-Time Electroencephalography-Based Neurofeedback
Since fMRI-based (blood-and-oxygen-level dependent) signals are hundreds of times slower than electrophysiological signals, there is a strong case to be made for the advantages of using electrophysiological signals (Ros et al., 2013, 2014) measured by methods such as electroencephalography (EEG) or magnetoencephalography (MEG). In addition to high temporal resolution, human scalp EEG is non-invasive, affordable, with no movement restriction, and is more suitable to large-scale applications. EEG is a tool that measures the summations of neural postsynaptic potentials at the scalp. EEG-based NF has been successful in enhancing attention in older adults (Jiang et al., 2017). Using a double-blind controlled design in the older brains, seminal work by Angelakis et al. (2007) applied EEG NF in an older population and showed improved processing speed and executive functions. Additional success has been reported using EEG-based NF training in older dementia patients (Surmeli et al., 2016).
Despite exciting progress using NF in brain training to improve memory in young and older adults, most of the NF training studies thus far have been limited to traditional frequencies. NF training using event-related potentials (ERPs), i.e., averaged EEG signals associated with cognitive events, is currently lacking in the literature.
So, once the field gains new knowledge of neuromarkers of specific neurophysiological functions, then neurofeedback training should be better targeted beyond the single frequency approach. The new methods should include frequency interactions and event-related potentials. The challenges include both neurological understanding as well as technical difficulties for real-time closed-loop feedback (Sitaram et al., 2017).
Very few studies have applied memory-related potentials directly in the context of preclinical and clinical Alzheimer’s pathology. ERPs are a well-studied approach for indexing brain responses associated with memory and cognition. These electrophysiological outcome measures can predict individual risk of mild cognitive impairment 5 years before diagnosis (Jiang et al., 2021).
Neurofeedback has been used to improve cognitive and physical performance of humans (Daly and Wolpaw, 2008; Pfurtscheller et al., 2008; Machado et al., 2013; Broccard et al., 2014; Chaudhary et al., 2016). While the efficacy of these methods differs, all have been reported to enhance performance by training attention (another core function of cognition) and working memory tasks. However, evidence for improvement in everyday life utilizing cognition is limited, which provides the impetus for developing better and time-efficient methods to directly train neural processes underlying attention. A closed-loop BCI system can be designed to directly control external devices. Also, it can be utilized as a neurofeedback platform in neurorehabilitation programs to improve and enhance the cognitive abilities (Chaudhary et al., 2016).
While there is fundamental disparity between neurofeedback and BCI (Abiri et al., 2019, 2020), combining both approaches can potentially help to expand achievements of each individual approach.
For instance, the goal in motor-imagery BCIs is to control an external object by inducing and modulating the brainwaves of interest during the training session so that the BCI system can determine the user’s intention in real-time in testing sessions. Users must learn how to regulate their brainwaves which then makes the BCI analogous to neurofeedback. In both, users must learn to modulate their brain activity to acquire a certain feedback, specific output, or greater reward. The similarity may result in triggering similar cognitive and neural processes. Thus, the training process of BCI may expand our knowledge of neurofeedback.
In Search of Better Rewards in Neurofeedback Systems
Most of the neurofeedback studies have utilized a few channels of EEG signals or a fixed and narrow range of frequencies (Gruzelier, 2014a,b) while most of the BCI systems, such as motor-imagery BCIs have incorporated multiple EEG channels and individualized and adaptable ranges of frequencies determined by signal processing, machine learning, or deep learning approaches. Embracing those approaches in neurofeedback regiments may help to distinguish and extract individualized features from EEG signals with which we may obtain higher efficacy compared to having a fixed channels and fixed frequency approach (Ang et al., 2012).
There is also research about task-unspecific internal and external confounding variables characterized and studied in the BCI literature. For instance, the attentional state of the user has been quantified with the level of mu and gamma bands in different brain areas. The measure has helped to enumerate the level of attention and motivation in a motor-imagery BCI task (Grosse-Wentrup et al., 2011). Having those measures alongside the neurofeedback therapy can be beneficial to assess how likely a user is motivated to participate in neurofeedback therapy and how one neurofeedback regiment may be adapted and accepted by the users over another one.
The type and form of feedback signal is another instrumental element in neurofeedback. We have five main senses: visual, auditory, somatosensory, olfactory, and gustatory. Although vision is the most prominent sensory feedback in humans compared to other primates, other sensory feedbacks may play a significant role in learning and modulating our brainwaves in response to different phenomena.
There are yet more challenges to applying neurofeedback. One challenge is how to define the reinforcement signals. It might be easier and ethically more appropriate to define the reinforcement signal in non-human experiments. For example, withholding food from a rat and providing it later as a reward when the animal successfully modulates a neurophysiological signal may not be an ethical concern (at least now), but it would be more challenging and clearly unethical to deprive human subjects of food. Another challenge is that there is no guarantee that a human subject would interpret the feedback as a reward. So, the motivational state of the subject results in different interpretation of the feedback signal.
Clinical neurofeedback training has been shown to be an effective treatment for people with a wide range of deficits, including epilepsy (Sterman and Egner, 2006; Strehl et al., 2014), attention deficit hyperactivity disorder (ADHD) (Arns et al., 2009), stroke (Rayegani et al., 2014), autistic spectrum disorder (ASD) (Kouijzer et al., 2009), emotional disorders (Reiter et al., 2016), and tinnitus (Hartmann et al., 2014). Furthermore, neurofeedback has been evaluated as a means to enhance cognitive control in healthy people (Zoefel et al., 2011; Wang and Hsieh, 2013; Gruzelier, 2014a,b). The quality and quantity of changes (effect size) due to the neurofeedback regimen can be enumerated with behavioral responses, measured by cognitive scoring questionnaires, or demonstrated by neural measures of cognition.
Electroencephalography, Event-Related Potential Neurofeedback in Improving Cognitive Complaints and Impairment
The basic EEG components in human adults are the delta, alpha, theta, beta, and gamma frequency bands. Alpha frequency during eyes-closed resting EEG has emerged as an important biomarker in mild cognitive impairment and dementia (Babiloni et al., 2021a; Cecchetti et al., 2021; (Stoiljkovic et al., 2021). Alpha oscillations (8–12 Hz), sourced in frontal sites including the anterior cingulate cortex, are related to working memory and related performance in humans. This EEG wave is the most common wave seen during awake states (Adrian and Matthews, 1934). Global alpha power is more abnormal in younger MCI and AD patients (Babiloni et al., 2021b). Posterior alpha sources are more abnormal in male MCI/AD patients (Babiloni et al., 2021c).
The EEG theta rhythm (4–8 Hz) is also related to memory performance. Theta rhythms have been shown to play a role in encoding episodic memories and are also correlated with behavioral performance (Hasselmo and Stern, 2014). EEG delta rhythms (0.5–3.5 Hz) are somewhat different than the other three as an increase in delta band activity leads to inhibition (Harmony, 2013). This inhibition is useful however, as it blocks interference from other centers in the brain, allowing one to concentrate on the mental task at hand. Delta waves are prominent in deep stages of sleep. Finally, beta rhythms (13–35 Hz) are related to brain consciousness and motor functions. Both alpha and beta waves are common during awake states, but beta waves are also present in states of drowsiness (Nayak and Anilkumar, 2020). Finally, gamma rhythms (30–80 Hz) are higher frequency EEG rhythms that have demonstrated a role in visual and sensory processing. Due to the low amplitude of the gamma rhythms, these EEG frequencies have been historically difficult to measure but have recently been linked to other rhythms and functions. However, rapidly growing new literature on gamma rhythms and its underlying biological basis is fascinating (e.g., Milekovic et al., 2019). NF using high frequency components are a new frontier needing research.
Increasing evidence suggests the coupling of two different rhythms is also used to perform specific functions. Coupling of the brain’s theta-gamma rhythms (θ–γ) has demonstrated a link between the cortical regions (prefrontal areas and the cingulate cortex) which plays a major role in working memory (Schack and Klimesch, 2002). Delta-theta coupling has been shown to be important in decision making and cognitive processing (Adams et al., 2019). Interestingly, changes in theta-gamma coupling have been shown to be earlier neuromarkers than traditional AD biomarkers. AD and MCI patients demonstrate the lowest level of θ–γ coupling in a verbal working memory task in comparison with healthy participants (Goodman et al., 2018). An impairment of θ–γ coupling that increases in parallel to the progression of the MCI has been recently reported in human patients (Musaeus et al., 2020). These new findings suggest that coupling of brain oscillations is critical for proper cognitive functioning and will likely be another neuromarker for applying NF treatment.
What Neurofeedback Paradigms of Working Memory Worked and Did Not Work?
To determine the most successful way of performing a NF study, samples of previous studies of successful and unsuccessful studies were analyzed. Twelve studies involving older adults (> 60 years old) and eight involving younger adults (< 35 years old) were selected for using several different parameters with varied success reported in each study. Investigating the differences seen between different age groups, however, provided important insight into the quality of studies performed in the past. As much of human cognitive development occurs before the age of twelve, there is a rational fear that once a body reaches a certain stage, mental growth and training is impossible. Although there is increasing evidence that the brain remains plastic past its “peak years,” the physiological degeneration due to brain aging is an obstacle to training older adults with NF (Mattson and Arumugam, 2018).
After a review of successful past studies, it was demonstrated that the aging brain can in fact be trained and improved, despite the anatomic and pathological limitations faced. There were seven studies involving younger individuals investigated, five of which were successful in using NF to train the brain. Of the eleven studies involving patients over sixty, nine were successful in training the brain with NF, two of which involved patients with MCI. This demonstrates that not only can the healthy aging brain be trained successfully with NF, but a degenerating aging brain can be trained as well. Although there are many different factors that potentially change the results in these studies, it is promising to see evidence that the aging brain can still be changed and improved upon, as this provides an avenue into treating more severe diseases such as Alzheimer’s disease. The factors that potentially confound these studies are compared below.
Each study used a slightly different method in controlling for their BCI group, so it was important to investigate the different controls used (Nicolas-Alonso and Gomez-Gil, 2012; Sorger et al., 2019). Between the studies that used older and younger populations, there were three common types of control groups used: a “waitlist” control, a sham NF control, and a group that received cognitive training in place of the NF (some used a combination of these or no control at all, but these were the most common occurring groups). The waitlist control groups received no training between the first and last testing sessions. The sham NF groups received the NF training sessions but did not receive the NF protocol to change any specific EEG band. The cognitive training groups used other cognitive training methods such as brain teasers and memory games in place of the NF. Control groups will be discussed in much more detail later in this review.
Two primary modalities used in the NF studies were investigated: visual and auditory. A majority of the studies utilized a visual NF protocol. In the projects that used visual feedback (Krause et al., 2017), a visual reward such as a motivating progress bar or pleasing image was given upon activating the specific EEG band. The auditory feedback was similar except it used a pleasing tone for achieving the goal range and an unpleasant tone for missing the EEG power range. The combination study was unsuccessful at achieving its goal. The fifteen visual modality studies were successful where one out of the two auditory modality studies were successful. More research needs to be performed to determine whether auditory only NF protocols are useful in training the brain, as two studies are not a large enough sample size to draw a conclusion.
Follow up time was the major limitation found throughout each study included in this review. Only one study reported longitudinal findings (Marlats et al., 2020). This group had participants return and re-test after 1 month. They demonstrated that scores for several different memory tasks remained elevated from preliminary testing after receiving NF training. Follow up is vital to the integrity of any study like this as it demonstrates the clinical power EEG NF training can have on those with declining cognitive function. Having at least a follow up after 1 month should be an integral portion of any NF study moving forward; continuing follow ups through the year will increase the power of the studies by showing prolonged cognitive improvements.
Another component to the follow up that requires consideration is whether there is a need for re-training. Spaced repetition is a useful technique for memorizing large amounts of information and could also be a useful tool when considering the long-lasting effects of EEG based NF. Doing a certain number of sessions throughout a year could prove to have even more benefits than performing 10–20 sessions within the span of 1 month. This, as well as the vitality of the training during follow up sessions, will need to be investigated in the future.
Individual Differences in Neurofeedback
Neurofeedback did not work in some studies listed in Tables 1, 2. We investigated individual differences in brain signals for answers. Strong individual differences, including but not limited to genetic and environmental differences, have been reported in working memory and performance (Parasuraman and Jiang, 2012), and how cognitive training effects are preserved and learning is transferred (Greenwood and Parasuraman, 2015). Learning transfer means in cognitive training in one domain (working memory) can transfer in similar tasks (short-transfer) and untrained tasks (long-transfer). However, there are strong individual differences in applying learning transfer to important daily tasks (Sinotte and Coelho, 2007; Westerberg et al., 2007; Cicerone et al., 2011; Kuo et al., 2014). Attention, mood, and motivation are important factors (Kadosh and Staunton, 2019).
Placebo Effects
A placebo is a treatment with no positive or negative side effects. The placebo effect is a phenomenon where a patient’s condition improves even though they were given a treatment that has no mechanism to alleviate their symptoms. Finniss et al. (2010) provide psychological explanations for the placebo effect. First, the patient expecting their symptoms to abate can be enough to lead to the placebo effect. One example of the placebo effect is an experimenter telling the patient the medication will cause a reduction in headaches leads to an actual reduction in the patient’s headaches because they expect the medication to work. Another is classical conditioning, where if a patient’s headache gets relieved by the medication the first-time they take it, they will expect that to happen every time. The combination of expectancy and classical conditioning can cause a significant placebo effect, creating a challenge for scientists to conclude if the medication was truly helpful or if it was the placebo effect (Finniss et al., 2010). One neurofeedback study showed participants with a positive expectancy of the treatment had a decrease in symptoms while participants with a negative expectancy of the treatment had an increase in their symptoms (Lee and Suhr, 2020).
For researchers to elucidate if their treatment is helpful or if the participant is experiencing placebo effect results, they often do double blind studies. Double blind studies are structured so neither the participant nor the experimenters know who receives the real treatment and who receives the placebo. Designing an experiment this way allows adequate comparison to help draw a conclusion regarding true efficacy or a placebo effect. Neurofeedback characteristics cause difficulties in designing an effective double-blind experiment because the automatic rewards a participant receives during a double-blind study elicit a different response than the rewards manually programmed into a single-blind neurofeedback study (Lansbergen et al., 2010). The single-blind experiment leads to an increased possibility for experimenter bias. Confounding variables like a participant getting coaching or getting rewarded for focusing further complicates the experiment’s design (Eugene Arnold et al., 2020).
Bioethical Debates in Brain Training
The NF and double-blind studies have shown effectiveness in a variety of treatments e.g., psychiatric disorders (Mehler et al., 2018; Dudek and Dodell-Feder, 2021), children’s ADHD (Steiner et al., 2014; Riesco-Matías et al., 2021), chronic pain (Patel et al., 2020). They have also dealt with diseases that ranged from Parkinson’s disease to cognitive decline in stroke victims. Is it ethical to withhold treatment that could vastly improve a participant’s quality of life? Sandler and Bodfish (2008) told children diagnosed with ADHD and their parents that they were going to be given a placebo. The children’s symptoms remained constant when given the placebo and a lower dose ADHD medication but worsened when they only received the lower dose ADHD medication. This finding may mean that researchers can run more ethical placebo neurofeedback studies by making sure the participants are fully aware and agree to the possibility of receiving a placebo.
A deficiency in the studies in Tables 1, 2 was a lack of placebo and/or double-blind methodology. Table 3 consists of studies that did contain a placebo and/or double-blind methodology. Although Table 3 depicts potential benefits for older adults, only two experiments in the table used a double-blind study, meaning that it is possible there were some expectancy results in the experiments (Mottaz et al., 2018; Nicholson et al., 2020). There is hope for older adults suffering from mild cognitive impairments, strokes, and Parkinson’s disease that neurofeedback may be a safe and effective treatment for them. Further studies need to be done, particularly double-blind studies, to prove the positive outcomes of neurofeedback treatment and eliminate confounding variables. Many of the placebo tests did not have a follow up, so it is impossible to tell if the positive outcomes of the neurofeedback will be retained long term. The same limitation was found in Tables 1, 2. Mottaz et al. (2018) did have a follow up and saw an improvement in their participants’ functional connectivity (FC) over the controls’. The improvement did not last during a follow-up investigation.
Brain Computer Interface Insensitivity
Brain-Computer Interface (BCI) illiteracy describes participants in neurofeedback studies who do not adequately achieve performance goals. Due to negative connotations surrounding “illiteracy,” “BCI insensitivity” is proposed instead to describe the phenomenon of non-respondence (Sannelli et al., 2019). “BCI insensitivity” describes participants unable to train successfully as well as participants incapable of performing neurofeedback with the desired accuracy. It is estimated that BCI inefficiency can be found in 15–30% of neurofeedback participants (Blankertz et al., 2010). Researchers can divide participants into three groups—participants who can be successfully trained with appropriate accuracy, participants who can be successfully trained but did not reach the required accuracy during neurofeedback, and participants who could neither be successfully trained nor achieve the required accuracy during neurofeedback (Vidaurre and Blankertz, 2010).
Neurofeedback can be a costly and time-consuming treatment. Because up to 30% of participants may not receive the desired effects from neurofeedback, researchers look for potential predictors in participants that may have BCI inefficiency. Hammer et al. (2012), found participants who had higher fine motor skills and a better ability to self-regulate focus performed better during neurofeedback training. Lower peaks of sensory motor rhythm (SMR) may be indicative of less likelihood of a participant achieving successful neurofeedback (Sannelli et al., 2019). Participants’ initial BCI performance may also predict their overall outcome or how much training a participant will need to be successful (Neumann and Birbaumer, 2003; Kübler et al., 2004). To help save time and money in studies, Blankertz et al. (2010) designed a program to predict a participant’s performance based on a 2-min resting EEG with eyes opened.
As more predictors of BCI insensitivity are published, the question then becomes, is it ever possible for participants to achieve neurofeedback success with BCI insensitivity? The answer is yes. Vidaurre and Blankertz (2010) developed a coadaptation calibration program that allowed users to increase their SMR peaks, increasing BCI performance. Sannelli et al. (2016) developed an adaptive learning model using common spatial patterns and sensory motor rhythms that helped participants who failed to achieve performance goals in prior neurofeedback studies. Methods involved visual evoked potentials and tactile sensation have also been explored in BCI (Yao et al., 2018; Volosyak et al., 2020). Going forward, researchers may want to use a combination of the predictive techniques along with techniques for improving BCI efficiency to help participants achieve the best results. Furthermore, more BCI studies involving older adults are needed for researchers to make adequate conclusions for BCI training in different age groups.
The studies in Table 3 were chosen based on several different criteria. Only studies that had a control/placebo group were included. Experiments that primarily used children and young adults were excluded as the focus of this paper is on older adults. Experiments in Table 4 were chosen based on the criteria that the researchers were specifically looking at BCI with the use of neurofeedback. Unfortunately, due to the limited number of studies using controls, this table could not be limited to only experiments with control groups. The same is true for only using experiments that were mainly comprised of older adults.
The Theoretical Basis of Brain Training of Working Memory With Electroencephalography Neurofeedback Treatment
Cognitive aging is caused by synaptic, metabolic, and structural changes during brain aging which slowly lead to loss of full cognitive function. EEG measures synchronized synaptic functions and network at the scalp. Recent work has identified neurosynaptic changes as one of the earliest biomarkers of preclinical AD, appearing before onset of tau-mediated neuronal injury or brain structure changes (Jack et al., 2011; Sperling et al., 2011). Among the most common early symptoms of dementia are deficits in working memory. The exact neural mechanisms subserving working memory are under debate (Mi et al., 2017). There are three types of hypotheses: First, the Frequency model suggested that WM involves periodic reactivation of memory representations at each gamma cycle within gamma-theta nested oscillations in the hippocampus, mediated by slow after depolarizations with a time constant that should be matched to the theta period. Assuming each memory is activated exactly once during a theta burst cycle, the same memories are repeatedly reactivated over subsequent theta cycles (Lisman and Idiart, 1995; Siegel et al., 2009). Deficits in higher frequency such as gamma rhythms are associated with cognitive deficits. Therefore, brain stimulation studies (Chan et al., 2021; Mimenza-Alvarado et al., 2021), applying gamma frequency treatment in mild cognitive impairment and mild Alzheimer’s disease, set foundations for future neurofeedback applying gamma rhythm.
The question remains why manipulating lower frequencies such as delta and alpha improves working memory performance. New evidence suggests that glial astrocyte reactivity and Ca + channel related slow waves altered in the brains of persons with dementia might be the cause of the low frequency alterations (Sardinha et al., 2017). Astrocytic signaling supports hippocampal-prefrontal theta synchronization and cognitive function (Lenk et al., 2020).
Second, the persistent firing model hypothesizes that working memory is supported by persistent activity of certain neurons active in the prefrontal cortex, hippocampus, parietal, and other cortical and subcortical networks, in the absence of direct perceptual stimulations. For instance, enhanced EEG activity during memory retrieval is observed in frontal sites, which are different from visually evoked potentials during perception. The attractiveness of this model is that it explains the memory network supporting working memory functions via synchronized oscillations at the same frequency in different brain regions. Recent evidence in animal models and with neuroimaging also points to brain connectivity networks as novel neuro-markers for indexing early deficits in AD risk. Aß peptides disrupt neural activity at the synaptic level and induce aberrant activity patterns in neural network circuits within and between brain regions in animal models (Palop and Mucke, 2010). Patterns of functional brain connectivity in humans are highly predictive of cognitive performance (Hachinski et al., 2006; Finn et al., 2015). The blood-oxygen-dependent level brain signals measured by functional MRI show that brain connectivity (particularly bilateral parietal and frontal-temporal) correlates with CSF AD biomarkers (Aß and tau), particularly during working memory tasks (Jiang et al., 2016). Overall synaptic synchronization during an intrinsic spontaneous resting state measured by EEG output has also been linked to neuroinflammation (astrocytes reactivity and calcium signaling; Wang et al., 2021), which provides a potential treatment direction.
Third, Synaptic theory of working memory is a synaptic-based theory for short-term information storage in neural circuits (Mongillo et al., 2008; Lundqvist et al., 2011). In this model, memory is retained by an item-specific pattern of synaptic facilitation. This mechanism does not require neurons to fire with elevated rates for the whole duration of the memory task, resulting in a robust and metabolically more efficient scheme (Mi et al., 2017). Resting EEG and network oscillations are correlated to working memory performance (Borhani et al., 2019) and cognitive impairment (McBride et al., 2013, 2014, 2015).
Finally, the Neurofeedback to frontal and pre-frontal cortices enhance goal-directed behaviors and executive functions. The goal-directed behaviors are strongly associated with the brain activity in the prefrontal cortex (Miller and Cohen, 2001). Executive functions encompass the mental processes that allow individuals to take control over automatic responses of the brain to produce goal-oriented behaviors (Garon et al., 2008). The prefrontal cortex functions comprise planning, goal setting, decision making, voluntary attention, task switching, set shifting, behavioral and perceptual inhibitions, voluntary regulation, and error correction. In therapeutical neurofeedback, especially the ones that target cognitive control, some of the functions seem to be fundamental to set up intrinsic reward, to integrate feedback information, and to self-regulate behavior. Most of those functions interact with attention, a broad concept that can be defined as the set of processes dealing with the allocation of working memory to the different neural representations available in the brain (Knudsen, 2007). Attention plays a critical role in allocating the brain resources to working memory while high-level cognition relies on working memory to learn tasks (Cowan et al., 2005). There are studies indicating a shared neural mechanism that supports both attention and working memory (Ikkai and Curtis, 2011; Gazzaley and Nobre, 2012). The shared neural mechanisms support the theory that there is also a mutual connection between the two brain functions. Thus, event-related (memory and/or attention) brainwave patterns should be targets of NF training.
Neurofeedback Training Beyond Electroencephalography Frequency Bands: Event-Related Potential-Based Neurofeedback
Electroencephalography recordings directly measure post-synaptic potentials. Recording the averaged EEG signals, i.e., event-related potentials (ERP), during cognitive events known as cognitive ERP is a promising but less often investigated approach for indexing brain mechanisms underlying cognition and memory (Olichney et al., 2008, 2011, 2013; Li et al., 2017; Jiang et al., 2021). Memory-related neuromarkers are sensitive to general cognitive decline before conventional biomarkers of AD can be detected by CSF/PET methods and behavioral performance changes.
Using longitudinal follow-up of healthy older adults over 10 years, Jiang et al. (2021) revealed that the different brainwaves between working memory responses to Targets and Non-targets in three left frontal electrodes (F7, F5, or F3) or averaged from three left frontal sites are the best predictor for diagnosis of cognitive impairment 5 years before diagnosis. Intriguingly, memory non-targets or distractors did not predict well.
A participant wearing a wireless EEG headset performs a visual memory task (Figure 1). Recorded EEG signals are analyzed in real-time, e.g., EEG wavelets analysis (frequency, power, and time) or ERP memory-related potentials. Neurofeedback is given by rewarding brain patterns that are associated with accurate and fast memory retrieval.
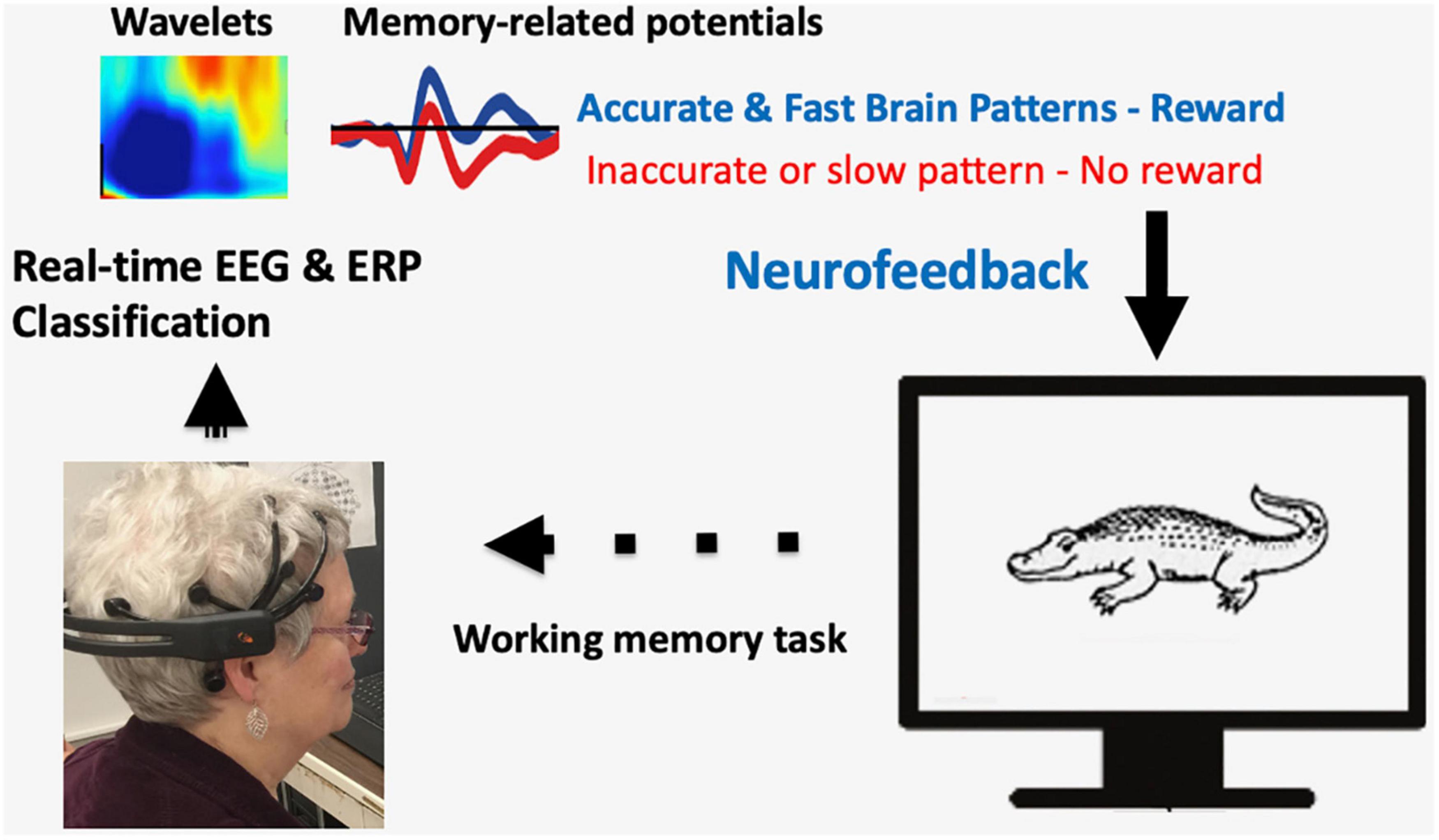
Figure 1. A sample illustration of closed-loop Neurofeedback training for memory improvement. A participant wearing a wireless EEG headset performs a visual memory task. Recorded EEG signals are analyzed in real-time, e.g., EEG wavelet analysis (frequency, power, and time) or ERP memory-related potentials. Neurofeedback is given by rewarding brain patterns that are associated with accurate and fast memory retrieval.
Existing studies on ERP modulation using NF have revealed insights into designing reward functions. These studies mainly investigate the decoding method of single-trial responses (Brandmeyer et al., 2013) as well as the neural mechanism underling reward stimuli (Moser et al., 2014; Zioga et al., 2019; Wang et al., 2021). Research in the literature demonstrates broad applications of ERP-based NF training in cognitive deficits, including Attention-deficit/hyperactivity disorder (ADHD), Post-traumatic stress disorder (PTSD), and Subjective cognitive decline (SCD) (Askovic et al., 2020; Pei et al., 2020; Deiber et al., 2021). The main challenge of developing NF training using ERP is designing reward functions associated with components of ERP as the adjustment target in single-trial responses. In a recent study, Wang et al. (2021) compared the change of event-related desynchronization (ERD) power attenuation using ERP-based NF vs. motor imagery (MI)-based NF and showed that only ERP based study presents significant differences in the frequency bands of EEG signals between the neurofeedback and non-feedback groups.
The Summary of Neurofeedback Clinical Trials to Improve Mild Cognitive Impairment
Important for clinical applications of neurofeedback, we reviewed clinical trials related to mild cognitive impairment and dementia using NF training around the world. Table 5 includes studies using only neurofeedback to improve cognitive ability in older adults, in Alzheimer’s disease, and related dementia (AD/ADRD). Importantly, there are different EEG and ERP variation in MCI induced by subtypes of ADRD (Güntekin et al., 2021). Note three times more neurofeedback trials are being conducted to improve mood, depression, and attention at the clinicaltrials.gov site that are not included in the current review.
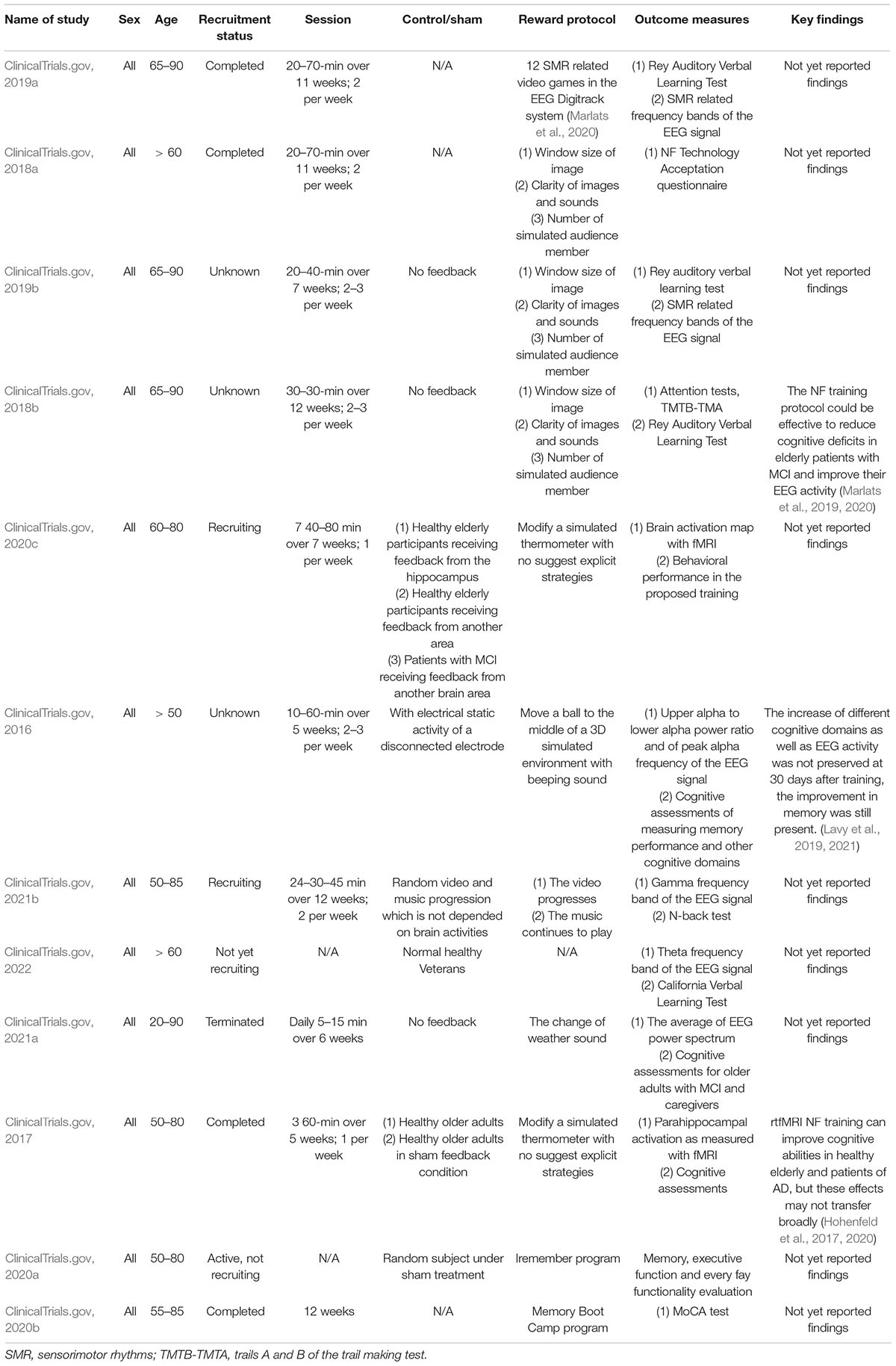
Table 5. Summary of clinical trials at clinicaltrials.gov of applying NF training for older adults with MCI.
The Take-Home Message of Electroencephalography Neurofeedback Studies and Future Directions
Most current Neurofeedback training improves memory and cognition to a certain extent. Large scale and optimal clinical applications of neurofeedback are limited by several challenges. Debate remains about the frequency and length of optimal NF effects, outcome measures, and long-term effects.
A well-known issue in such training is that some people simply do not respond to neurofeedback. Thus, we also reviewed the literature of individual differences in placebo effects and non-responses. Future work needs to focus on an individual based approach.
Sleep EEG has also emerged as a very important biomarker (Whitehurst et al., 2020), especially for outcome measures in neurofeedback studies in the future (Alfini et al., 2020). D’Atri et al. (2021) reported the importance of time of day showing differences in Delta and alpha signals during PM and AM wake studies (lack of difference in patients with cognitive impairment and AD). Rapid Eye Movement (REM) EEG slowing during sleep showed the strongest correlation with cognitive decline than other wake EEG in their study. Sleep EEG also has stable signals. If daytime nap EEG can be managed (Alger et al., 2012), it should be an ideal outcome measure for effectiveness in NF training.
The range of EEG features for NF training are as follows:
– EEG (Various Alpha, frontal theta, sensory-motor rhythm, connectivity during resting-state)
– ERP (Memory-related potentials; event-related connectivity)
– Cognitive impairment due to different pathologies warrants different neurofeedback, e.g., AD vs. Non-AD (LB dementia)
– Higher EEG rhythm such as gamma frequency is gaining attention.
– EEG features during sleep for memory enhancement during sleep and as outcome measures.
Conclusion
Our review brings overall good news of the potential effectiveness of neurofeedback brain training. Most training studies typically take multiple weeks (e.g., 5–20 weeks) with 2–3 sessions per week. We review various neurofeedback reward strategies (visual and auditory methods) and outcome measures. Our review recognizes that individuals’ neural responses are on a continuous spectrum. We recommend that “neural modulation sensitivity” instead of “BCI illiteracy” is to be considered as the preferred terminology in brain training. Future directions include much needed research in mild cognitive impairment, in non-Alzheimer’s dementia populations, and neurofeedback using EEG features during resting and sleep for memory enhancement and as sensitive outcome measures.
Author Contributions
YJ wrote the first draft and oversaw all aspects of the revisions. WJ wrote the reviews summarized in Tables 1, 2. SH wrote the reviews summarized in Tables 3, 4. SB wrote the closed-loop real-time NF section. ZL wrote the related review summarized in Table 5. XZ conceptualized and wrote the BCI portion. LP and WH contributed to conceptualization and interpretation of EEG-based NF training. JS and SC-S contributed to conceptualization of clinical implications, critical revision, overall edits, and to Figure 1.
Funding
Part of this work was supported by United States Department of Veterans Affairs grant RX003173-01, National Institute of Health AG060608, a pilot grant from Behavioral Science Department, a PSMRF research award, University of Kentucky College of Medicine, and Alzheimer’s Tennessee.
Conflict of Interest
The authors declare that the research was conducted in the absence of any commercial or financial relationships that could be construed as a potential conflict of interest.
Publisher’s Note
All claims expressed in this article are solely those of the authors and do not necessarily represent those of their affiliated organizations, or those of the publisher, the editors and the reviewers. Any product that may be evaluated in this article, or claim that may be made by its manufacturer, is not guaranteed or endorsed by the publisher.
Acknowledgments
We would like to thank Timothy Carbary at Lexington VAMC for helpful discussions and Grace Markowski for editing assistance.
References
Abiri, R., Borhani, S., Kilmarx, J., Esterwood, C., Jiang, Y., and Zhao, X. (2020). A usability study of low-cost wireless brain-computer interface for cursor control using online linear model. IEEE Trans. Hum. Machine Syst. 50, 287–297. doi: 10.1109/THMS.2020.2983848
Abiri, R., Borhani, S., Sellers, E. W., Jiang, Y., and Zhao, X. (2019). A comprehensive review of EEG-based brain-computer interface paradigms. J. Neural Eng. 16:011001. doi: 10.1088/1741-2552/aaf12e
Adams, N. E., Teige, C., Mollo, G., Karapanagiotidis, T., Cornelissen, P. L., Smallwood, J., et al. (2019). Theta/delta coupling across cortical laminae contributes to semantic cognition. J. Neurophysiol. 121, 1150–1161. doi: 10.1152/jn.00686.2018
Adrian, E. D., and Matthews, B. H. C. (1934). The berger rhythm: potential changes from the occipital lobes in man. Brain 57, 355–385. doi: 10.1093/brain/57.4.355
Alfini, A. J., Tzuang, M., Owusu, J. T., and Spira, A. P. (2020). Later-life sleep, cognition, and neuroimaging research: an update for 2020. Curr. Opin. Behav. Sci. 33, 72–77.
Alger, S. E., Lau, H., and Fishbein, W. (2012). Slow wave sleep during a daytime nap is necessary for protection from subsequent interference and long-term retention. Neurobiol. Learn. Mem. 98, 188–196. doi: 10.1016/j.nlm.2012.06.003
Ang, K. K., Chin, Z. Y., Zhang, H., and Guan, C. (2012). Mutual information-based selection of optimal spatial–temporal patterns for single-trial EEG-based BCIs. Pattern Recognit. Lett. 45, 2137–2144. doi: 10.1016/j.patcog.2011.04.018
Angelakis, E., Stathopoulou, S., Frymiare, J. L., Green, D. L., Lubar, J. F., and Kounios, J. (2007). EEG neurofeedback: a brief overview and an example of peak alpha frequency training for cognitive enhancement in the elderly. Clin. Neuropsychol. 21, 110–129. doi: 10.1080/13854040600744839
Arns, M., De Ridder, S., Strehl, U., Breteler, M., and Coenen, A. (2009). Efficacy of neurofeedback treatment in ADHD: the effects on inattention, impulsivity and hyperactivity: a meta-analysis. Clin. EEG Neurosci. Biobehav. Rev. 40, 180–189. doi: 10.1177/155005940904000311
Askovic, M., Watters, A. J., Coello, M., Aroche, J., Harris, A. W., and Kropotov, J. (2020). Evaluation of neurofeedback for posttraumatic stress disorder related to refugee experiences using self-report and cognitive ERP measures. Clin. EEG Neurosci. 51, 79–86.
Babiloni, C., Arakaki, X., Azami, H., Bennys, K., Blinowska, K., Bonanni, L., et al. (2021a). Measures of resting state EEG rhythms for clinical trials in Alzheimer’s disease: Recommendations of an expert panel. Alzheimers Dement. 17, 1528–1553. doi: 10.1002/alz.12311
Babiloni, C., Ferri, R., Noce, G., Lizio, R., Lopez, S., Lorenzo, I., et al. (2021b). Resting-state alpha electroencephalographic rhythms are differently related to aging in cognitively unimpaired seniors and patients with alzheimer’s disease and amnesic mild cognitive impairment. J. Alzheimers Dis. 82, 1085–1114. doi: 10.3233/JAD-201271
Babiloni, C., Noce, G., Ferri, R., Lizio, R., Lopez, S., Lorenzo, I., et al. (2021c). Resting state alpha electroencephalographic rhythms are affected by sex in cognitively unimpaired seniors and patients with alzheimer’s disease and amnesic mild cognitive impairment: a retrospective and exploratory study. Cereb. Cortex. 6:bhab348. doi: 10.1093/cercor/bhab348
Bagherzadeh, Y., Baldauf, D., Pantazis, D., and Desimone, R. (2019). Alpha synchrony and the neurofeedback control of spatial attention. Neuron. 105, 577–587.e5. doi: 10.1016/j.neuron.2019.11.001
Becerra, J., Fernández, T., Roca-Stappung, M., Díaz-Comas, L., Galán, L., Bosch, J., et al. (2011). Neurofeedback in healthy elderly human subjects with electroencephalographic risk for cognitive disorder. J. Alzheimers Dis. 28, 357–367. doi: 10.3233/JAD-2011-111055
Bielas, J., and Michalczyk, Ł (2021). Beta neurofeedback training improves attentional control in the elderly. Psychol. Rep. 124, 54–69. doi: 10.1177/0033294119900348
Blankertz, B., Sannelli, C., Halder, S., Hammer, E. M., Kübler, A., Müller, K.-R., et al. (2010). Neurophysiological predictor of SMR-based BCI performance. Neuroimage 51, 1303–1309. doi: 10.1016/j.neuroimage.2010.03.022
Borhani, S., Abiri, R., Jiang, Y., Burger, T., and Zhao, X. (2019). Brain connectivity evaluation during selective attention using EEG-based brain-computer interface. Brain Comput. Interfaces 6, 1–11. doi: 10.1080/2326263X.2019.1651186
Brandmeyer, A., Sadakata, M., Spyrou, L., McQueen, J., and Desain, P. (2013). Decoding of single-trial auditory mismatch responses for online perceptual monitoring and neurofeedback. Front. Neurosci. 7:265. doi: 10.3389/fnins.2013.00265
Broccard, F. D., Mullen, T., Chi, Y. M., Peterson, D., Iversen, J. R., and Arnold, M. (2014). Closed-loop brain–machine–body interfaces for noninvasive rehabilitation of movement disorders. Ann. Biomed. Eng. 42, 1573–1593. doi: 10.1007/s10439-014-1032-6
Campos da Paz, V. K., Garcia, A., Campos, da Paz, Neto, A., and Tomaz, C. (2018). SMR neurofeedback training facilitates working memory performance in healthy older adults: a behavioral and EEG study. Front. Behav. Neurosci. 12:321. doi: 10.3389/fnbeh.2018.00321
Cecchetti, G., Agosta, F., Basaia, S., Cividini, C., Cursi, M., Santangelo, R., et al. (2021). Resting-state electroencephalographic biomarkers of Alzheimer’s disease. Neuroimage Clin. 31:102711. doi: 10.1016/j.nicl.2021.102711
Chan, D., Suk, H. J., Jackson, B. L., Milman, N. P., Stark, D., Klerman, E. B., et al. (2021). Gamma frequency sensory stimulation in probable mild alzheimer’s dementia patients: results of a preliminary clinical trial. metRxiv [Preprint] doi: 10.1101/2021.03.01.21252717
Chaudhary, U., Birbaumer, N., and Ramos-Murguialday, A. (2016). Brain-computer interfaces for communication and rehabilitation. Nat. Rev. Neurol. 12, 513–525. doi: 10.1038/nrneurol.2016.113
Chen, Y., Spagna, A., Wu, T., Kim, T. H., Wu, Q., Chen, C., et al. (2019). Testing a Cognitive Control Model of Human Intelligence. Sci. Rep. 9:2898. doi: 10.1038/s41598-019-39685-2
Cicerone, K. D., Langenbahn, D. M., Braden, C., Malec, J. F., Kalmar, K., Fraas, M., et al. (2011). Evidence-based cognitive rehabilitation: updated review of the literature from 2003 through 2008. Arch. Phys. Med. Rehabil. 92, 519–530. doi: 10.1016/j.apmr.2010.11.015
ClinicalTrials.gov (2016). Neurofeedback as a Novel Treatment for Mild Cognitive Impairment & Early Alzheimer’s Disease. Available online at: https://ClinicalTrials.gov/show/NCT02987842 [accessed on December 13, 2016].
ClinicalTrials.gov (2017). Therapy of Alzheimer’s Disease With Neurofeedback. Available online at: https://ClinicalTrials.gov/show/NCT03070821 [accessed on March 6, 2017].
ClinicalTrials.gov (2018a). Feasibility Study of Neurofeedback Training for Older Adults With Mild Cognitive Impairment. Available online at: https://ClinicalTrials.gov/show/NCT03686839 [accessed on September 27, 2018].
ClinicalTrials.gov (2018b). Neurofeedback Training for Older Adults With Mild Cognitive Impairment: a Protocol Study. Available online at: https://ClinicalTrials.gov/show/NCT03526692 [accessed on May 18, 2018].
ClinicalTrials.gov (2019a). Neurofeedback Improves Cognitive Performance and EEG Activity in Elderly With Mild Cognitive Impairment. Available online at: https://ClinicalTrials.gov/show/NCT03855319 [accessed on February 28, 2019].
ClinicalTrials.gov (2019b). Neurofeedback Training for Older Adults with Mild Cognitive Impairment: A Pilot Study. Available online at: https://ClinicalTrials.gov/show/NCT04027725 [accessed on October 21, 2019].
ClinicalTrials.gov (2020a). BestBrain Evaluation of Cognitive Memory & Executive-Function. Available online at: https://ClinicalTrials.gov/show/NCT03954340 [accessed on October 22, 2020].
ClinicalTrials.gov (2020b). Effects of Memory Boot Camp on Brain Function in Adults with Symptoms of Mild Cognitive Impairment (MCI). Available online at: https://ClinicalTrials.gov/show/NCT04426162 [accessed on June 11, 2020].
ClinicalTrials.gov (2020c). Real-time fMRI Neurofeedback in Patients With MCI. Available online at: https://ClinicalTrials.gov/show/NCT04020744 [accessed on December 23, 2020].
ClinicalTrials.gov (2021a). iMeditate at Home for Older Adults With Mild Cognitive Impairment and Caregivers. Available online at: https://ClinicalTrials.gov/show/NCT04184037 [accessed on January 22, 2021].
ClinicalTrials.gov (2021b). Neurofeedback to Improve Working Memory in Mild Cognitive Impairment. Available online at: https://ClinicalTrials.gov/show/NCT04566900 [accessed on March 2, 2021].
ClinicalTrials.gov (2022). Neurofeedback to Aid Vets’ Memory. Available online at: https://ClinicalTrials.gov/show/NCT04446481 [accessed on January 10, 2022].
Cowan, N., Elliott, E., Saults, J., Morey, C., Mattox, S., Hismjatullina, A., et al. (2005). On the capacity of attention: Its estimation and its role in working memory and cognitive aptitudes. Cogn. Psychol. 51, 42–100. doi: 10.1016/j.cogpsych.2004.12.001
Daly, J. J., and Wolpaw, J. R. (2008). Brain-computer interfaces in neurological rehabilitation. Lancet Neurol. 7, 1032–1043. doi: 10.1016/s1474-4422(08)70223-0
D’Atri, A., Scarpelli, S., Gorgoni, M., Truglia, I., Lauri, G., Cordone, S., et al. (2021). EEG alterations during wake and sleep in mild cognitive impairment and Alzheimer’s disease. Iscience 24:102386. doi: 10.1016/j.isci.2021.102386
deBettencourt, M. T., Turk-Browne, N. B., and Norman, K. A. (2019). Neurofeedback helps to reveal a relationship between context reinstatement and memory retrieval. Neuroimage 200, 292–301. doi: 10.1016/j.neuroimage.2019.06.001
deBettencourt, M., Cohen, J. D., Lee, R. F., Norman, K. A., and Turk-Browne, N. B. (2015). Closed-loop training of memory with real-time brain imaging. Nat. Neurosci. 18, 470–475. doi: 10.1038/nn.3940
Deiber, M.-P., Ammann, C., Hasler, R., Colin, J., Perroud, N., and Ros, T. (2021). Electrophysiological correlates of improved executive function following EEG neurofeedback in adult attention deficit hyperactivity disorder. Clin. Neurophysiol. 132, 1937–1946.
Direito, B., Ramos, M., Pereira, J., Campos, A. S., Sousa, T., and de Sá e Sousa Castelo-Branco, M. (2021). Directly exploring the neural correlates of feedback-related reward saliency during fMRI- based Neurofeedback. Front. Hum. Neurosci. 14:578119. doi: 10.3389/fnhum.2020.578119
Dudek, E., and Dodell-Feder, D. (2021). The efficacy of real-time functional magnetic resonance imaging neurofeedback for psychiatric illness: a meta-analysis of brain and behavioral outcomes. Neurosci. Biobehav. Rev. 121, 291–306. doi: 10.1016/j.neubiorev.2020.12.020
Emmert, K., Kopel, R., Sulzer, J., Brühl, A. B., Berman, B. D., and Linden, D. E. (2016). Meta-analysis of real-time fMRI neurofeedback studies using individual participant data: How is brain regulation mediated? NeuroImage 124(Pt A), 806–812. doi: 10.1016/j.neuroimage.2015.09.042
Escolano, C., Aguilar, M., and Minguez, J. (2011). “EEG-based upper alpha neurofeedback training improves working memory performance,” in Proceedings of the Annual International Conference of the IEEE Engineering in Medicine and Biology Society, (Berlin: IEEE), 2327–2330. doi: 10.1109/IEMBS.2011.6090651
Eugene Arnold, L., Pan, J., Arns, M., Tan, Y., Barterian, J., Cottrell, A. A., et al. (2020). A 25-month follow-up of a double-blind randomized controlled trial of neurofeedback for ADHD. J. Am. Acad. Child Adolesc. Psychiatry 59, S325–S326.
Finn, E. S., Shen, X., Scheinost, D., Rosenberg, M. D., Huang, J., Chun, M. M., et al. (2015). Functional connectome fingerprinting: identifying individuals using patterns of brain connectivity. Nat. Neurosci. 18, 1664–1671. doi: 10.1038/nn.4135
Finniss, D. G., Kaptchuk, T. J., Miller, F., and Benedetti, F. (2010). Biological, clinical, and ethical advances of placebo effects. Lancet 375, 686–695. doi: 10.1016/s0140-6736(09)61706-2
Garon, N., Bryson, S. E., and Smith, I. M. (2008). Executive function in preschoolers: a review using an integrative framework. Psychol. Bull. 134, 31–60. doi: 10.1037/0033-2909.134.1.31
Gazzaley, A., and Nobre, A. C. (2012). Top-down modulation: bridging selective attention and working memory. Trends Cognit. Sci. 16, 129–135. doi: 10.1016/j.tics.2011.11.014
Gomez-Pilar, J., Corralejo, R., Nicolas-Alonso, L. F., Álvarez, D., and Hornero, R. (2016). Neurofeedback training with a motor imagery-based BCI: neurocognitive improvements and EEG changes in the elderly. Med. Biol. Eng. Comput. 54, 1655–1666. doi: 10.1007/s11517-016-1454-4
Goodman, M. S., Kumar, S., Zomorrodi, R., Ghazala, Z., Cheam, A. S. M., Barr, M. S., et al. (2018). Theta-Gamma coupling and working memory in Alzheimer’s dementia and mild cognitive impairment. Front. Aging Neurosci. 10:101. doi: 10.3389/fnagi.2018.00101
Gordon, S., Todder, D., Deutsch, I., Garbi, D., Alkobi, O., Shriki, O., et al. (2019). Effects of neurofeedback and working memory-combined training on executive functions in healthy young adults. Psychol. Res. 84, 1586–1609. doi: 10.1007/s00426-019-01170-w
Greenwood, P. M., and Parasuraman, R. (2015). The Mechanisms of Far Transfer From Cognitive Training: Review and Hypothesis. Neuropsychology 30, 742–755. doi: 10.1037/neu0000235
Grosse-Wentrup, M., Schölkopf, B., and Hill, J. (2011). Causal influence of gamma oscillations on the sensorimotor rhythm. NeuroImage 56, 837–842. doi: 10.1016/j.neuroimage.2010.04.265
Gruzelier, J. H. (2014a). EEG-neurofeedback for optimising performance. I: a review of cognitive and affective outcome in healthy participants. Neurosci. Biobehav. Rev. 44, 124–141. doi: 10.1016/j.neubiorev.2013.09.015
Gruzelier, J. H. (2014b). EEG-neurofeedback for optimising performance. III: a review of methodological and theoretical considerations. Neurosci. Biobehav. Rev. 44, 159–182. doi: 10.1016/j.neubiorev.2014.03.015
Güntekin, B., Aktürk, T., Arakaki, X., Bonanni, L., Del Percio, C., Edelmayer, R., et al. (2021). Are there consistent abnormalities in event-related EEG oscillations in patients with Alzheimer’s disease compared to other diseases belonging to dementia? Psychophysiology 30:e13934. doi: 10.1111/psyp.13934
Hachinski, V., Iadecola, C., Petersen, R. C., Breteler, M. M., Nyenhuis, D. L., and Black, S. E. (2006). National Institute of Neurological Disorders and Stroke-Canadian Stroke Network vascular cognitive impairment harmonization standards. Stroke 37, 2220–2241. doi: 10.1161/01.STR.0000237236.88823.47
Hammer, E. M., Halder, S., Blankertz, B., Sannelli, C., Dickhaus, T., Kleih, S., et al. (2012). Psychological predictors of SMR-BCI performance. Biol. Psychol. 89, 80–86. doi: 10.1016/j.biopsycho.2011.09.006
Harmony, T. (2013). The functional significance of delta oscillations in cognitive processing. Front. Integr. Neurosci. 7:83. doi: 10.3389/fnint.2013.00083
Hartmann, T., Lorenz, I., Müller, N., Langguth, B., and Weisz, N. (2014). The effects of neurofeedback on oscillatory processes related to tinnitus. Brain Topogr. 27, 149–157. doi: 10.1007/s10548-013-0295-9
Hasselmo, M. E., and Stern, C. E. (2014). Theta rhythm and the encoding and retrieval of space and time. NeuroImage 85, 656–666. doi: 10.1016/j.neuroimage.2013.06.022
Hohenfeld, C., Kuhn, H., Müller, C., Nellessen, N., Ketteler, S., Heinecke, A., et al. (2020). Changes in brain activation related to visuo-spatial memory after real-time fMRI neurofeedback training in healthy elderly and Alzheimer’s disease. Behav. Brain Res. 381:112435.
Hohenfeld, C., Nellessen, N., Dogan, I., Kuhn, H., Müller, C., Papa, F., et al. (2017). Cognitive improvement and brain changes after real-time functional mri neurofeedback training in healthy elderly and prodromal Alzheimer’s Disease. Front. Neurol. 8:384. doi: 10.3389/fneur.2017.00384
Hsueh, J.-J., Chen, T.-S., Chen, J.-J., and Shaw, F.-Z. (2016). Neurofeedback training of EEG alpha rhythm enhances episodic and working memory. Hum. Brain Mapp. 37, 2662–2675. doi: 10.1002/hbm.23201
Ikkai, A., and Curtis, C. E. (2011). Common neural mechanisms supporting spatial working memory, attention and motor intention. Neuropsychologia 49, 1428–1434. doi: 10.1016/j.neuropsychologia.2010.12.020
Jack, C. R. Jr., Albert, M. S., Knopman, D. S., McKhann, G. M., Sperling, R. A., Carrillo, M. C., et al. (2011). Introduction to the recommendations from the National Institute on Aging-Alzheimer’s Association workgroups on diagnostic guidelines for Alzheimer’s disease. Alzheimers Dement. 7, 257–262. doi: 10.1016/j.jalz.2011.03.004
Jiang, Y., Abiri, R., and Zhao, X. (2017). Tuning up the old brain with new tricks: attention training via neurofeedback. Front. Aging Neurosci. 13:52. doi: 10.3389/fnagi.2017.00052
Jiang, Y., Huang, H., Abner, E., Broster, L. S., Jicha, G. A., and Schmitt, F. A. (2016). Alzheimer’s Biomarkers are correlated with Brain connectivity in Older adults Differentially during resting and Task states. Front. Aging Neurosci. 8:15. doi: 10.3389/fnagi.2016.00015
Jiang, Y., Li, J., Schmitt, F. A., Jicha, G. A., Munro, N. B., Zhao, X., et al. (2021). Memory-related frontal brainwaves predict transition to mild cognitive impairment in healthy older individuals five years before diagnosis. J. Alzheimers Dis. 79, 1–11. doi: 10.3233/JAD-200931
Jirayucharoensak, S., Israsena, P., Pan-ngum, S., Hemrunrojn, S., and Maes, M. (2019). A game-based neurofeedback training system to enhance cognitive performance in healthy elderly subjects and in patients with amnestic mild cognitive impairment. Clin. Interv. Aging 14, 347–360. doi: 10.2147/CIA.S189047
Jurewicz, K., Paluch, K., Kublik, E., Rogala, J., Mikicin, M., and Wróbel, A. (2017). EEG-neurofeedback training of beta band (12–22 Hz) affects alpha and beta frequencies – A controlled study of a healthy population. Neuropsychologia 108, 13–24. doi: 10.1016/j.neuropsychologia.2017.11.021
Kadosh, K. C., and Staunton, G. (2019). A systematic review of the psychological factors that influence neurofeedback learning outcomes. Neuroimage 185, 545–555. doi: 10.1016/j.neuroimage.2018.10.021
Knudsen, E. I. (2007). Fundamental components of attention. Annu. Rev. Neurosci. 30, 57–78. doi: 10.1146/annurev.neuro.30.051606.094256
Kouijzer, M. E., de Moor, J. M., Gerrits, B. J., Buitelaar, J. K., and van Schie, H. T. (2009). Long-term effects of neurofeedback treatment in autism. Res. Autism Spectr. Disord. 3, 496–501. doi: 10.1016/j.rasd.2008.10.003
Krause, F., Benjamins, C., Lührs, M., Eck, J., Noirhomme, Q., Rosenke, M., et al. (2017). Real-time fMRI-based self-regulation of brain activation across different visual feedback presentations. Brain Comput. Interfaces 4, 87–101. doi: 10.1080/2326263X.2017.1307096
Kübler, A., Neumann, N., Wilhelm, B., Hinterberger, T., and Birbaumer, N. (2004). Predictability of brain-computer communication. J. Psychophysiol. 18, 121–129. doi: 10.1027/0269-8803.18.23.121
Kuo, C.-C., Zhang, C., Rissman, R. A., and Chiu, A. W. (2014). Long-term electrophysiological and behavioral analysis on the improvement of visual working memory load, training gains, and transfer benefits. J. Behav. Brain Sci. 4, 234–246. doi: 10.4236/jbbs.2014.45025
Lansbergen, M. M., Van Dongen-Boomsma, M., Buitelaar, J. K., and Slaats-Willemse, D. (2010). ADHD and EEG-neurofeedback: a double-blind randomized placebo-controlled feasibility study. J. Neural Transm. 118, 275–284. doi: 10.1007/s00702-010-0524-2
Lavy, Y., Dwolatzky, T., Kaplan, Z., Guez, J., and Todder, D. (2019). Neurofeedback improves memory and peak alpha frequency in individuals with mild cognitive impairment. Appl. Psychophysiol. Biofeedback 44, 41–49.
Lavy, Y., Dwolatzky, T., Kaplan, Z., Guez, J., and Todder, D. (2021). Mild cognitive impairment and neurofeedback: a randomized controlled trial. Front. Aging Neurosci. 13:657646. doi: 10.3389/fnagi.2021.657646
Lecomte, G., and Juhel, J. (2011). The effects of neurofeedback training on memory performance in Elderly subjects. Psychology 2, 846–852. doi: 10.4236/psych.2011.28129
Lee, G. J., and Suhr, J. A. (2020). Expectancy effects of placebo neurofeedback in adhd treatment seekers: a neuropsychological investigation. Neuropsychology 34, 774–782. doi: 10.1037/neu0000689
Lee, Y.-S., Bae, S. H., Lee, S. H., and Kim, K. Y. (2015). Neurofeedback training improves the dual-task performance ability in stroke patients. Tohoku J. Exp. Med. 236, 81–88. doi: 10.1620/tjem.236.81
Lenk, K., Satuvuori, E., Lallouette, J., Ladrón-de-Guevara, A., Berry, H., and Hyttinen, J. A. K. (2020). A computational model of interactions between neuronal and astrocytic networks: the role of astrocytes in the stability of the neuronal firing rate. Front. Computat. Neurosci. 13:92. doi: 10.3389/fncom.2019.00092
Li, J., Broster, L., Jicha, G., Munro, N., Schmitt, F., Abner, E., et al. (2017). A cognitive electrophysiological signature differentiates amnestic mild cognitive impairment from normal aging. Alzheimers Res. Ther. 9:3. doi: 10.1186/s13195-016-0229-3
Lisman, J. E., and Idiart, M. A. P. (1995). Storage of 7 ± 2 short-term memories in oscillatory subcycles. Science 267, 1512–1515. doi: 10.1126/science.7878473
Lundqvist, M., Herman, P., and Lansner, A. (2011). Theta and gamma power increases and alpha/beta power decreases with memory load in an attractor network model. J. Cogn. Neurosci. 23, 3008–3020. doi: 10.1162/jocn_a_00029
Machado, S., Almada, L. F., and Annavarapu, R. N. (2013). Progress and prospects in EEG-based brain-computer interface: clinical applications in neurorehabilitation. J. Rehabil. Robot. 1, 28–41. doi: 10.1515/REVNEURO.2010.21.6.451
Marlats, F., Bao, G., Chevallier, S., Boubaya, M., Djabelkhir-Jemmi, L., Wu, Y. H., et al. (2020). SMR/theta neurofeedback training improves cognitive performance and EEG activity in elderly with mild cognitive impairment: a pilot study. Front. Aging Neurosci. 12:147. doi: 10.3389/fnagi.2020.00147
Marlats, F., Djabelkhir-Jemmi, L., Azabou, E., Boubaya, M., Pouwels, S., and Rigaud, A. S. (2019). Comparison of effects between SMR/delta-ratio and beta1/theta-ratio neurofeedback training for older adults with Mild Cognitive Impairment: a protocol for a randomized controlled trial. Trials 20:88.
Mattson, M. P., and Arumugam, T. V. (2018). Hallmarks of brain aging: adaptive and pathological modification by metabolic states. Cell Metab. 27, 1176–1199. doi: 10.1016/j.cmet.2018.05.011
McBride, J. C., Zhao, X., Munro, N. B., Jicha, G. A., Schmitt, F. A., Kryscio, R. J., et al. (2015). Sugihara causality analysis of scalp EEG for detection of early Alzheimer’s disease. NeuroImage 7, 258–265. doi: 10.1016/j.nicl.2014.12.005
McBride, J. C., Zhao, X., Munro, N. B., Smith, C. D., Jicha, G. A., Hively, L., et al. (2014). Spectral and complexity analysis of scalp EEG characteristics for mild cognitive impairment and early Alzheimer’s disease. Comput. Methods Programs Biomed. 114, 153–163. doi: 10.1016/j.cmpb.2014.01.019
McBride, J., Zhao, X., Munro, N., Smith, C., Jicha, G., and Jiang, Y. (2013). Resting EEG discrimination of early stage Alzheimer’s disease from normal aging using inter-channel coherence network graphs. Ann. Biomed. Eng. 41, 1233–1242. doi: 10.1007/s10439-013-0788-4
Mehler, D. M. A., Sokunbi, M. O., Habes, I., Barawi, K., Subramanian, L., Range, M., et al. (2018). Targeting the affective brain—a randomized controlled trial of real-time fMRI neurofeedback in patients with depression. Neuropsychopharmacology 43, 2578–2585. doi: 10.1038/s41386-018-0126-5
Mi, Y., Katkov, M., and Tsodyks, M. (2017). Synaptic correlates of working memory capacity. Neuron 93, 323–330. doi: 10.1016/j.neuron.2016.12.004
Milekovic, T., Bacher, D., Sarma, A. A., Simeral, J. D., Saab, J., Pandarinath, C., et al. (2019). Volitional control of single-electrode high gamma local field potentials by people with paralysis. J. Neurophysiol. 121, 1428–1450. doi: 10.1152/jn.00131.2018
Miller, E. K., and Cohen, J. D. (2001). An integrative theory of prefrontal cortex function. Annu. Rev. Neurosci. 24, 167–202. doi: 10.1152/jn.00131.2018
Mimenza-Alvarado, A. J., Aguilar-Navarro, S. G., Martinez-Carrillo, F. M., Ríos-Ponce, A. E., and Villafuerte, G. (2021). Use of fast gamma magnetic stimulation over the left prefrontal dorsolateral cortex for the treatment of MCI and mild Alzheimer’s Disease: a double-blind, randomized, sham-controlled, pilot study. Front. Neurol. 12:729872. doi: 10.3389/fneur.2021.729872
Mongillo, G., Barak, O., and Tsodyks, M. (2008). Synaptic theory of working memory. Science 319, 1543–1546. doi: 10.1126/science.1150769
Moser, J. S., Hartwig, R., Moran, T. P., Jendrusina, A. A., and Kross, E. (2014). Neural markers of positive reappraisal and their associations with trait reappraisal and worry. J. Abnorm. Psychol. 123, 91–105.
Mottaz, A., Corbet, T., Doganci, N., Magnin, C., Nicolo, P., Schnider, A., et al. (2018). Modulating functional connectivity after stroke with neurofeedback: Effect on motor deficits in a controlled cross-over study. NeuroImage Clin. 20, 336–346. doi: 10.1016/j.nicl.2018.07.029
Musaeus, C. S., Nielsen, M. S., Musaeus, J. S., and Høgh, P. (2020). Electroencephalographic cross-frequency coupling as a sign of disease progression in patients with mild cognitive impairment: a pilot study. Front. Neurosci. 14:790. doi: 10.3389/fnins.2020.00790
Neumann, N., and Birbaumer, N. (2003). Predictors of successful self control during brain-computer communication. J. Neurol. Neurosurg. Psychiatry 74, 1117–1121. doi: 10.1136/jnnp.74.8.1117
Nicholson, A. A., Ros, T., Densmore, M., Frewen, P. A., Neufeld, R. W. J., Théberge, J., et al. (2020). A randomized, controlled trial of alpha-rhythm EEG neurofeedback in posttraumatic stress disorder: a preliminary investigation showing evidence of decreased PTSD symptoms and restored default mode and salience network connectivity using fMRI. NeuroImage Clin. 28:102490. doi: 10.1016/j.nicl.2020.102490
Nicolas-Alonso, L. F., and Gomez-Gil, J. (2012). Brain computer interfaces, a review. Sensors 12, 1211–1279. doi: 10.3390/s120201211
Olichney, J. M., Pak, J., Salmon, D. P., Yang, J.-C., Gahagan, T., Nowacki, R., et al. (2013). Abnormal P600 word repetition effect in elderly persons with preclinical Alzheimer’s disease. Cognit. Neurosci. 4, 143–151. doi: 10.1080/17588928.2013.838945
Olichney, J. M., Taylor, J., Gatherwright, J., Salmon, D., Bressler, A., Kutas, M., et al. (2008). Patients with MCI and N400 or P600 abnormalities are at very high risk for conversion to dementia. Neurology 70(19 Part 2), 1763–1770. doi: 10.1212/01.wnl.0000281689.28759.ab
Olichney, J. M., Yang, J.-C., Taylor, J., and Kutas, M. (2011). Cognitive event-related potentials: biomarkers of synaptic dysfunction across the stages of Alzheimer’s disease. J. Alzheimers Dis. 26, 215–228. doi: 10.3233/JAD-2011-0047
Palop, J. J., and Mucke, L. (2010). Amyloid-[beta]-induced neuronal dysfunction in Alzheimer’s disease: from synapses toward neural networks. Nat. Neurosci. 13, 812–818. doi: 10.1038/nn.2583
Parasuraman, R., and Jiang, Y. (2012). Individual differences in cognition, affect, and performance: behavioral, neuroimaging, and molecular genetic approaches. NeuroImage 59, 70–82. doi: 10.1016/j.neuroimage.2011.04.040
Paret, C., Goldway, N., Zich, C., Keynan, J. N., Hendler, T., Linden, D., et al. (2019). Current progress in real-time functional magnetic resonance-based neurofeedback: Methodological challenges and achievements. Neuroimage 202:116107. doi: 10.1016/j.neuroimage.2019.116107
Patel, K., Sutherland, H., Henshaw, J., Taylor, J. R., Brown, C. A., Casson, A. J., et al. (2020). Effects of neurofeedback in the management of chronic pain: a systematic review and meta-analysis of clinical trials. Eur. J. Pain 24, 1440–1457. doi: 10.1002/ejp.1612
Pei, G., Yang, R., Shi, Z., Guo, G., Wang, S., Liu, M., et al. (2020). Enhancing working memory based on mismatch negativity neurofeedback in subjective cognitive decline patients: a preliminary study. Front. Aging Neurosci. 12:263. doi: 10.3389/fnagi.2020.00263
Pfurtscheller, G., Müller-Putz, G. R., Scherer, R., and Neuper, C. (2008). Rehabilitation with brain-computer interface systems. Computer 41, 58–65. doi: 10.1109/MC.2008.432
Rayegani, S., Raeissadat, S. A., Sedighipour, L., Rezazadeh, I. M., Bahrami, M. H., Eliaspour, D., et al. (2014). Effect of neurofeedback and electromyographic-biofeedback therapy on improving hand function in stroke patients. Top. Stroke Rehabil. 21, 137–151. doi: 10.1310/tsr2102-137
Reis, J., Portugal, A. M., Fernandes, L., Afonso, N., Pereira, M., Sousa, N., et al. (2016). An alpha and theta intensive and short neurofeedback protocol for healthy aging working-memory training. Front. Aging Neurosci. 8:157. doi: 10.3389/fnagi.2016.00157
Reiter, K., Andersen, S. B., and Carlsson, J. (2016). Neurofeedback treatment and posttraumatic stress disorder: effectiveness of neurofeedback on posttraumatic stress disorder and the optimal choice of protocol. J. Nervous Mental Dis. 204, 69–77. doi: 10.1097/NMD.0000000000000418
Riesco-Matías, P., Yela-Bernabé, J. R., Crego, A., and Sánchez-Zaballos, E. (2021). What do meta-analyses have to say about the efficacy of neurofeedback applied to children with adHD? Review of previous meta-analyses and a new meta-analysis. J. Atten. Disord. 25, 473–485. doi: 10.1177/1087054718821731
Ros, T. J., Baars, B., Lanius, R. A., and Vuilleumier, P. (2014). Tuning pathological brain oscillations with neurofeedback: a systems neuroscience framework. Front. Hum. Neurosci. 8:1008. doi: 10.3389/fnhum.2014.01008
Ros, T., Théberge, J., Frewen, P. A., Kluetsch, R., Densmore, M., Calhoun, V. D., et al. (2013). Mind over chatter: Plastic up-regulation of the fMRI salience network directly after EEG neurofeedback. Neuroimage 65, 324–335. doi: 10.1016/j.neuroimage.2012.09.046
Sandler, A. D., and Bodfish, J. W. (2008). Open-label use of placebos in the treatment of ADHD: A pilot study. Child 34, 104–110. doi: 10.1111/j.1365-2214.2007.00797.x
Sannelli, C., Vidaurre, C., Müller, K. R., and Blankertz, B. (2016). Ensembles of adaptive spatial filters increase BCI performance: an online evaluation. J. Neural Eng. 13:046003. doi: 10.1088/1741-2560/13/4/046003
Sannelli, C., Vidaurre, C., Müller, K.-R., and Blankertz, B. (2019). A large scale screening study with a SMR-based BCI: categorization of BCI users and differences in their SMR activity. PLoS One 14:e0207351. doi: 10.1371/journal.pone.0207351
Sardinha, V. M., Guerra-Gomes, S., Caetano, I., Tavares, G., Martins, M., Reis, J. S., et al. (2017). Astrocytic signaling supports hippocampal-prefrontal theta synchronization and cognitive function. Glia 65, 1944–1960. doi: 10.1002/glia.23205
Schack, B., and Klimesch, W. (2002). Frequency characteristics of evoked and oscillatory electroencephalic activity in a human memory scanning task. Neurosci. Lett. 331, 107–110. doi: 10.1016/S0304-3940(02)00846-7
Siegel, M., Warden, M. R., and Miller, E. K. (2009). Phase-dependent neuronal coding of objects in short-term memory. PNAS 106, 21341–21346. doi: 10.1073/pnas.0908193106
Sinotte, M. P., and Coelho, C. A. (2007). Memory training for reading impairment in mild aphasia: a follow-up study. NeuroRehabilitation 22, 303–310. doi: 10.3233/NRE-2007-22408
Sitaram, R., Ros, T., Stoeckel, L., Haller, S., Scharnowski, F., Lewis-Peacock, J., et al. (2017). Closed-loop brain training: the science of neurofeedback. Nat. Rev. Neurosci. 18, 86–100. doi: 10.1038/nrn.2016.164
Skottnik, L., Sorger, B., Kamp, T., Linden, D. E. J., and Goebel, R. (2019). Success and failure of controlling the fMRI-neurofeedback signal are reflected in the striatum. Brain Behav. 9:e01240. doi: 10.1002/brb3.1240
Sorger, B., Scharnowski, F., Linden, D. E. J., Hampson, M., and Young, K. D. (2019). Control freaks: Towards optimal selection of control conditions for fMRI neurofeedback studies. Neuroimage 186, 256–265. doi: 10.1016/j.neuroimage.2018.11.004
Sperling, R. A., Aisen, P. S., Beckett, L. A., Bennett, D. A., Craft, S., Fagan, A. M., et al. (2011). Toward defining the preclinical stages of Alzheimer’s disease: recommendations from the National Institute on Aging-Alzheimer’s Association workgroups on diagnostic guidelines for Alzheimer’s disease. Alzheimers Dement. 7, 280–292. doi: 10.1016/j.jalz.2011.03.003
Staufenbiel, S. M., Brouwer, A. W., Keizer, A. W., and van Wouwe, N. C. (2013). Effect of beta and gamma neurofeedback on memory and intelligence in the elderly. Biol. Psychol. 95, 74–85. doi: 10.1016/j.biopsycho.2013.05.020
Steiner, N. J., Frenette, E. C., Rene, K. M., Brennan, R. T., and Perrin, E. C. (2014). Neurofeedback and cognitive memory training for children with attention-deficit hyperactivity disorder in schools. J. Dev. Behav. Pediatr. 35, 18–27. doi: 10.1097/DBP.0000000000000009
Sterman, M. B., and Egner, T. (2006). Foundation and practice of neurofeedback for the treatment of epilepsy. Appl. Psychophysiol. Biofeedback Self Regulat. 31:21. doi: 10.1007/s10484-006-9002-x
Stoiljkovic, M., Horvath, T. L., and Hajós, M. (2021). Therapy for Alzheimer’s disease: missing targets and functional markers? Ageing Res. Rev. 68:101318. doi: 10.1016/j.arr.2021.101318
Strehl, U., Birkle, S. M., Wörz, S., and Kotchoubey, B. (2014). Sustained reduction of seizures in patients with intractable epilepsy after self-regulation training of slow cortical potentials–10 years after. Front. Hum. Neurosci. 8:604. doi: 10.3389/fnhum.2014.00604
Subramanian, L., Hindle, J. V., Johnston, S., Roberts, M. V., Husain, M., Goebel, R., et al. (2011). Real-time functional magnetic resonance imaging neurofeedback for treatment of Parkinson’s disease. J. Neurosci. 31, 16309–16317. doi: 10.1523/JNEUROSCI.3498-11.2011
Surmeli, T., Eralp, E., Mustafazade, I., Kos, H., Özer, G. E., and Surmeli, O. H. (2016). Quantitative EEG neurometric analysis–guided neurofeedback treatment in dementia. Clin. EEG Neurosci. 47, 118–133. doi: 10.1177/1550059415590750
Trambaiolli, L. R. Jr., Biazoli, C. E., Cravo, A. M., and Sato, J. R. (2021). “Feedback congruence affects real and perceived performance of an affective neurofeedback task,” in Proceedings of the International IEEE/EMBS Conference on Neural Engineering (NER), (Italy: IEEE), 14–16. doi: 10.1109/NER49283.2021.9441389
Vidaurre, C., and Blankertz, B. (2010). Towards a cure for BCI illiteracy. Brain Topogr. 23, 194–198. doi: 10.1007/s10548-009-0121-6
Volosyak, I., Rezeika, A., Benda, M., Gembler, F., and Stawicki, P. (2020). Towards solving of the Illiteracy phenomenon for VEP-based brain-computer interfaces. Biomed. Phys. Eng. Exp. 6, 035034–035034. doi: 10.1088/2057-1976/ab87e6
Wang, J.-R., and Hsieh, S. (2013). Neurofeedback training improves attention and working memory performance. Clin. Neurophysiol. 124, 2406–2420. doi: 10.1016/j.clinph.2013.05.020
Wang, Y., Luo, J., Guo, Y., Du, Q., Cheng, Q., and Wang, H. (2021). Changes in EEG brain connectivity caused by short-term bci neurofeedback-rehabilitation training: a case study. Front. Hum. Neurosci. 15:627100. doi: 10.3389/fnhum.2021.627100
Wei, T. Y., Chang, D. W., Liu, Y. D., Liu, C. W., Young, C. P., Liang, S. F., et al. (2017). Portable wireless neurofeedback system of EEG alpha rhythm enhances memory. BioMed. Eng. OnLine 16:128. doi: 10.1186/s12938-017-0418-8
Westerberg, H. J., Hirvikoski, T., Clevberger, P., Östensson, M.-L., Bartfai, A., and Klingberg, T. (2007). Computerized working memory training after stroke–A pilot study. Brain Injury 21, 21–29. doi: 10.1080/02699050601148726
Whitehurst, L., Chen, P. C., Naji, M., and Mednick, S. (2020). New directions in sleep and memory research: the role of autonomic activity. Curr. Opin. Behav. Sci. 33, 17–24. doi: 10.1016/j.cobeha.2019.11.001
Xiong, S., Cheng, C., Wu, X., Guo, X., Yao, L., and Zhang, J. (2014). Working memory training using EEG neurofeedback in normal young adults. Biomed. Mater. Eng. 24, 3637–3644. doi: 10.3233/BME-141191
Yao, L., Sheng, X., Mrachacz-Kersting, N., Zhu, X., Farina, D., and Jiang, N. (2018). Performance of brain-computer interfacing based on tactile selective sensation and motor imagery. IEEE Trans. Neural Syst. Rehabil. Eng. 26, 60–68. doi: 10.1109/TNSRE.2017.2769686
YuLeung To, E., Abbott, K., Foster, D. S., and Helmer, D. A. (2016). Working memory and neurofeedback. Appl. Neuropsychol. 5, 214–222. doi: 10.1080/21622965.2016.1167500
Zioga, I., Hassan, R., and Di Bernardi Luft, C. (2019). Success, but not failure feedback guides learning during neurofeedback: an ERP study. NeuroImage 200, 26–37. doi: 10.1016/j.neuroimage.2019.06.002
Keywords: closed-loop feedback, brain computer interface (BCI), working memory, EEG-ERPs, BCI illiteracy, biofeedback
Citation: Jiang Y, Jessee W, Hoyng S, Borhani S, Liu Z, Zhao X, Price LK, High W, Suhl J and Cerel-Suhl S (2022) Sharpening Working Memory With Real-Time Electrophysiological Brain Signals: Which Neurofeedback Paradigms Work? Front. Aging Neurosci. 14:780817. doi: 10.3389/fnagi.2022.780817
Received: 21 September 2021; Accepted: 08 February 2022;
Published: 28 March 2022.
Edited by:
Boon-Seng Wong, Singapore Institute of Technology, SingaporeReviewed by:
Dongrui Wu, Huazhong University of Science and Technology, ChinaVassiliy Tsytsarev, University of Maryland, College Park, United States
Tianyi Yan, Beijing Institute of Technology, China
Copyright © 2022 Jiang, Jessee, Hoyng, Borhani, Liu, Zhao, Price, High, Suhl and Cerel-Suhl. This is an open-access article distributed under the terms of the Creative Commons Attribution License (CC BY). The use, distribution or reproduction in other forums is permitted, provided the original author(s) and the copyright owner(s) are credited and that the original publication in this journal is cited, in accordance with accepted academic practice. No use, distribution or reproduction is permitted which does not comply with these terms.
*Correspondence: Yang Jiang, yjiang@uky.edu