- Istituto di Ricovero e Cura a Carattere Scientifico (IRCCS) Synlab SDN, Naples, Italy
Sleep problems are increasingly present in the general population at any age, and they are frequently concurrent with—or predictive of—memory disturbances, anxiety, and depression. In this exploratory cross-sectional study, 54 healthy participants recruited in Naples (Italy; 23 females; mean age = 37.1 years, range = 20–68) completed the Pittsburgh Sleep Quality Index (PSQI) and a neurocognitive assessment concerning both verbal and visuospatial working memory as well as subjective measures of anxiety and depression. Then, 3T fMRI images with structural and resting-state functional sequences were acquired. A whole-brain seed-to-seed functional connectivity (FC) analysis was conducted by contrasting good (PSQI score <5) vs. bad (PSQI score ≥5) sleepers. Results highlighted FC differences in limbic and fronto-temporo-parietal brain areas. Also, bad sleepers showed an anxious/depressive behavioural phenotype and performed worse than good sleepers at visuospatial working-memory tasks. These findings may help to reveal the effects of sleep quality on daily-life cognitive functioning and further elucidate pathophysiological mechanisms of sleep disorders.
Introduction
Sleep is a universal biological phenomenon among vertebrates that appear to be elemental to survival (Hartse, 2011). Individuals spend approximately a third of their lives sleeping, which testifies how crucial sleep is (Carlson, 2012). Even if we are far away from a complete understanding of the mechanisms underlying such complex behaviour, research has highlighted different functions of sleep that concern both cognition and emotion (Walker, 2009; Goldstein and Walker, 2014). Sleep is a homeostatic process that serves the brain’s vital functions, thus supporting cognitive activities (Mander et al., 2016). In fact, in a seemingly counterintuitive way, sleep does not appear to allow the body to rest, but instead, it expressly permits the brain to rest by reducing its metabolism (Reimund, 1994; Carlson, 2012). These aspects are crucial for individuals’ psychological functioning as sleep supports different cognitive processes involved in daily functioning (Waters and Bucks, 2011). For instance, sleep duration has been linked to verbal fluency and list memory, with both long and short sleepers having poorer performance in such kinds of tasks (Kronholm et al., 2009). Sleeping less than 5 h a night has been correlated with poorer global cognition and poorer performance in verbal memory, verbal fluency, and working memory (Tworoger et al., 2006). Sleep seems to modulate working memory performance (Chee and Choo, 2004; Kuriyama et al., 2008), namely a set of cognitive processes which provide a temporary buffer for maintaining and manipulating information, thus enabling daily cognitive tasks, such as language, learning and reasoning abilities (Baddeley, 1992). It seems that even short-term total sleep deprivation may produce effects on cognitive abilities mediated by the prefrontal cortex, which include attention and working memory (Lim and Dinges, 2010).
As a general practice derived from clinical neuropsychology, researchers investigated sleep from the systematic study of its absence, i.e., by considering sleep deprivation. Indeed, sleep deprivation impairs cerebral functioning, thus producing (1) increasing difficulties in performing cognitive tasks that require concentration, (2) perceptual distortions and, in rare cases, (3) mild hallucinations (e.g., Babkoff et al., 1989; Petrovsky et al., 2014; Sil’kis, 2014; Waters et al., 2018). Most importantly, the effects of sleep disturbances intervene rapidly as even momentary sleep perturbations may impact the individuals’ responsivity during the wake (e.g., Buzsáki and Watson, 2012). Alongside adverse effects on cognitive performance, disturbed sleep has been associated with poor emotional and physical health, substance use, conduct problems, anxiety, and depression (Reimer and Flemons, 2003; Holley et al., 2011; Alvaro et al., 2013; Conroy and Arnedt, 2014). Sleep disorders, particularly insomnia, are increasingly important problems nowadays, with incidences that grow with age (e.g., Foley et al., 1999; Gooneratne and Vitiello, 2014; Gulia and Kumar, 2018). In the general population, these disorders may relate to many factors, for instance, the use of caffeine, tobacco, alcohol, bad sleep habits (i.e., working late, using computers and other electronic devices, watching TV late at night), and modern society’s “24/7” rhythm (e.g., Brunborg et al., 2011; Poceta and Mitler, 2013; Garcia and Salloum, 2015). What is more, in addition to the endogenous and exogenous factors we reported above, sleep disorders may also emerge physiologically as age increases since variations in sleep patterns constitute part of the normal ageing process (e.g., Gulia and Kumar, 2018).
The evidence we briefly described above shows how sleep, on the one hand, may support cognitive and affective functioning and, on the other, may be susceptible to physiological and pathological favoured-by-ageing deterioration. Therefore, most studies focused on specific clinical populations or definite age clusters. However, a certain degree of sleep-related problems may also be found in the general population without established sleep disorders (e.g., Broman et al., 1996; Ohayon, 2011). Such problems may be concurrent with, or predictive of, memory disturbances, anxiety and depression, hence suggesting that sleep quality can be seen as a multidimensional and transdiagnostic variable in maintaining bio-psychological wellbeing (Lemola et al., 2013). Thus, studying the impact of sleep quality in healthy populations may help disentangle the relations between sleep, memory, ageing, and psychopathology, hence elucidating the effects of sleep on daily-life cognitive functioning. To examine the relations between sleep and changes in brain structure and function, over the years, multiple fMRI techniques, including resting-state fMRI, have been devised (e.g., Desseilles et al., 2008; Spiegelhalder et al., 2015; Cavaliere et al., 2020). On that basis, in this exploratory cross-sectional study, we investigated the effects of self-reported sleep quality on functional brain connectivity (FC), assessed by using 3T resting-state fMRI. We collected data from a sample of participants of varying ages (20–68 years). Behaviourally, we examined the effect of self-reported sleep quality on a neurocognitive process involved in daily-life cognitive functioning, namely working memory. We used the Italian version of the Pittsburgh Sleep Quality Index (PSQI) as a standardised self-report questionnaire to assess participants’ subjective sleep quality (Buysse et al., 1989; Curcio et al., 2013). In addition, we included subjective measures of anxiety and depression to characterise participants’ neuropsychological phenotype further.
Methods
Participants
We enrolled a convenience sample including 54 healthy participants (23 females; mean age = 37.1 years, range = 20–68). The cohort of the study came from a more extensive study, part of a research project of the IRCCS Synlab SDN (Naples, Italy). All participants were recruited in Naples (Italy) according to the following inclusion criteria: (i) lack of current or past history of alcohol or drug abuse; (ii) lack of current or past history of major psychiatric or neurological illnesses; and (iii) lack of current or past use of psychoactive medications. Both an experienced neurologist and neuropsychologist assessed each participant before starting the experiment. Participants with incidental brain lesions to the MRI examination were excluded. No participants were excluded according to the inclusion criteria, clinical evaluations, and MRI artefacts. Each participant provided written informed consent to participate in the study. The local Ethics Committee has approved the study as all its procedures followed the ethical standards laid down in the 1964 Helsinki Declaration.
Materials
To conduct the neuropsychological assessment, several pencil-and-paper tools were used. The Italian version of the Pittsburgh Sleep Quality Index (PSQI; Buysse et al., 1989; Curcio et al., 2013) has been used to measure participants’ self-reported sleep quality. PSQI is a self-report subjective sleep-quality index that scores from 0 to 21, with a cut-off score of 5, which discriminates between good (PSQI score <5) and bad sleepers (PSQI score ≥5). The Italian version of PSQI is “a useful, valid, and reliable tool for the assessment of sleep quality, with an overall efficiency comparable to the mother language version and differentiates “good” from “bad” sleepers. The Italian version of the questionnaire provides a good and reliable differentiation between normal and pathological groups, with higher scores reported by people characterised by impaired objectively evaluated sleep quality” (Curcio et al., 2013, p. 511). Beck Depression Inventory (BDI-II; Beck et al., 1996) has been used to subjectively measure depression score (score range: 0–63; cut-off value for mild depression: 20). State-Trait Anxiety Inventory (STAI; Spielberger et al., 1983) has been used to measure trait and state anxiety. STAI consists of 20 items that assess trait anxiety (STAI-T) and 20 items that assess state anxiety (STAI-S). The Digit Span Forward and Backward tests (DSF and DSB, respectively) have been used to investigate brief-term verbal memory and working memory’s verbal component. Finally, the Corsi Block-Tapping test (CBT) has been used to assess visuospatial working memory.
Procedure
This study has been conducted at the IRCCS Synlab SDN (Naples, Italy). An expert neuropsychologist performed the neuropsychological assessment for each participant. Such an assessment included a self-report evaluation of subjective sleep quality (PSQI), an assessment of depression and anxiety, and an evaluation of verbal and visuospatial working memory performance. After the neuropsychological assessment, each participant underwent a resting-state fMRI experimental protocol. MR images were acquired using a Biograph mMR 3T scanner (Siemens Healthcare, Erlangen, Germany) and a 12-channel head coil. An ad hoc acquisition protocol was devised, which included the following structural and functional sequences: (1) 3D T1-Magnetisation Prepared Rapid Acquisition Gradient Echo (MPRAGE), voxel size 0.8 × 0.8 × 0.8 mm3, Field of View (FOV) 214 × 214 mm, TR/TE/TI = 2,400/2.25/1,000 ms, scan time 5:03; and (2) Resting-state fMRI, Echo Planar Imaging-Gradient Echo sequence (EPI-GRE), voxel-size 4 × 4 × 4 mm3, TR/TE = 1,000/21.4 ms, 350 measurements, bandwidth: 2,230 Hz, scan time 6:02.
fMRI Data Analyses
fMRI data were analysed with Functional Connectivity Toolbox (CONN v. 20b; Whitfield-Gabrieli and Nieto-Castanon, 2012; Alfano et al., 2021) and Statistical Parametric Mapping (SPM v. 12). Both CONN and SPM were executed on MATLAB (v. 2021b). With CONN, pre-processing was carried out using a pipeline that includes realignment, slice-timing, functional-image normalisation in the Montreal Neurological Institute (MNI) space, outlier detection with ART-based scrubbing smoothing, and physiological denoising. We conducted a first statistical analysis to assess participants’ resting-state brain activations. Then, we devised a second statistical data analysis to assess differences in functional connectivity (FC) between participants with higher PSQI scores (i.e., bad sleepers) and participants with lower PSQI total scores (i.e., good sleepers). We evaluated FC differences between these two sub-groups by performing CONN-based seed-to-seed analyses, which were conducted by adopting cortical and subcortical ROIs (FSL Harvard-Oxford maximum likelihood cortical and subcortical atlas, dividing bilateral areas into left/right hemisphere for a total of 106 ROIs). Finally, a third, regression-based, between-network FC analysis was conducted to investigate correlations between large-scale brain networks FC and PSQI scores (networks from CONN’s ICA analyses of HCP dataset for a total of eight networks with 32 subnetwork ROIs). For both the seed-to-seed and network-to-network comparisons, an alpha level of 0.05 was used with false discovery rate (FDR) correction for multiple comparisons (Benjamini and Hochberg, 1995).
Behavioural Data Analyses
Pearson correlations were calculated among the PSQI scores, age, and other scores at the neuropsychological tests (i.e., STAI-T, STAI-S, BDI-II, DSF, DSB, CBT). All the scores were corrected for age, gender, and education level. An alpha level of 0.05 was used for all the analyses. The jamovi package (v. 1.6.23) for R software (v. 4.0.2) was used to perform behavioural data’s statistical analyses.
Results
Functional Connectivity Results
Results of the first whole-brain seed-to-seed analysis we implemented showed significant differences between participants with higher PSQI scores (i.e., 31 bad sleepers) and participants with lower PSQI (i.e., 23 good sleepers). The following pattern of seed-to-seed hypoconnectivity was found: right insular cortex (IC)—the right amygdala (T = −4.0; p = 0.024); pars triangularis of the right inferior frontal gyrus (IFGtri)—both left (T = −3.8; p = 0.035) and right IC (T = −3.2 p; = 0.05), right anterior supramarginal gyrus (aSMG; T = −0.2; p = 0.05), and left planum polare (PP; T = −3.1; p = 0.05); and left PP—right amygdala (T = −4.4; p = 0.006). The following pattern of seed-to-seed hyperconnectivity was found: right IFGtri—left posterior middle temporal gyrus (pMTG; T = 3.4; p = 0.04), left angular gyrus (AG; T = 3.3; p = 0.05), and medial frontal cortex (MedFC; T = 3.3; p = 0.05); posterior division of the right superior temporal gyrus (pSTG)—inferior division of the left lateral occipital cortex (iLOC; T = 3.7; p = 0.027); right iLOC—right pSTG (T = 3.8; p = 0.027), temporo-occipital part of the left inferior temporal gyrus (toITG; T = 3.5; p = 0.043), left IFG (T = 3.4; p = 0.043), and left superior parietal lobule (SPL; T = 3.3; p = 0.043); left SPL—right toITG (T = 3.7; p = 0.05); and left toITG—left frontal orbital cortex (OFC; T = 3.5; p = 0.05). The second analysis assessed between-network FC by considering the PSQI score as a continuous regressor. A moderate positive correlation among the PSQI score, the Salience network (peak seed: right SMG) and the Frontoparietal network [peak seed: right Posterior Parietal Cortex (PPC); T = 3.4; r = 0.42; p = 0.043] was found. Results of first FC analysis are summarised in Table 1 and Figure 1, while the results of the second FC analysis are depicted in Figure 2.
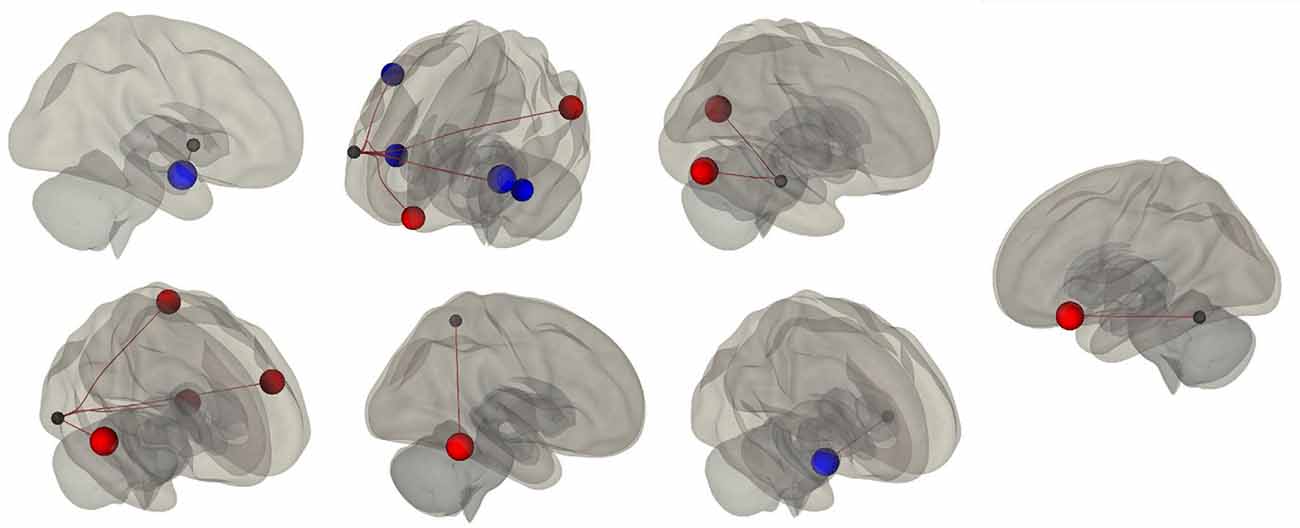
Figure 1. First-level functional connectivity (FC) analysis. 3D graphical representation of the first-level whole-brain seed-to-seed FC analysis’s results (Table 1), which shows the FC differences we found in bad sleepers (i.e., participants with a PSQI score ≥5) as compared to good sleepers (i.e., participants with a PSQI score <5). FC reductions are depicted in blue, while FC increases are illustrated in red.
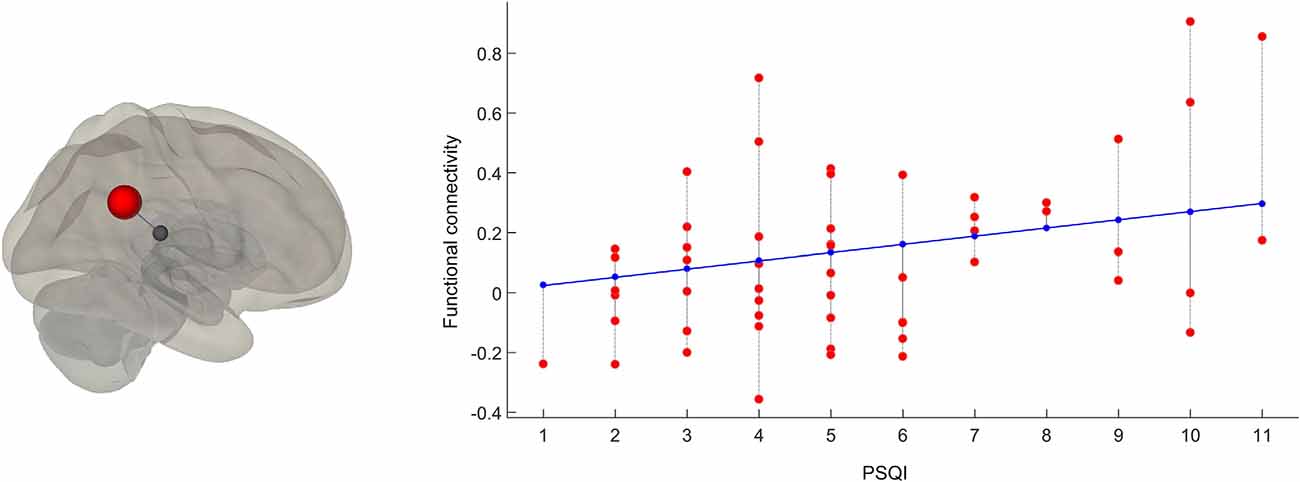
Figure 2. Between-network regression-based functional connectivity analysis. 3D graphical representation of the second-level, regression-based, between-network functional connectivity analysis’ results. FC hyperconnectivity between the Salience network (peak seed: right SMG; on the left in red) and the Frontoparietal network (peak seed: Posterior Parietal Cortex; on the left in blue) positively correlates with participants’ PSQI scores (scatter plot on the right; the x-axis represents the distribution of PSQI scores while the y-axis represents the functional connectivity value in the seeds).
Behavioural Results
Significant positive Pearson’s correlations were found among PSQI scores and: STAI-S scores (r = 0.34; p < 0.05); STAI-T scores (r = 0.64; p < 0.001); BDI-II scores (r = 0.58; p < 0.001); and age (r = 0.34; p < 0.05). A significant negative correlation between PSQI scores and CBT scores was found (r = −0.36; p < 0.01). Two non-significant negative correlations were found among PSQI scores and: DSF (r = −0.14, p = 0.31); and DSB (r = −0.13, p = 0.37). Descriptive statistics concerning all the measures considered in the study are summarised in Table 2, while a correlation matrix of them is presented in Table 3.

Table 2. Descriptive data concerning all the neuropsychological tests involved in the study (i.e., STAI-T, STAI-S, BDI-II, DSF, DSB, CBT).
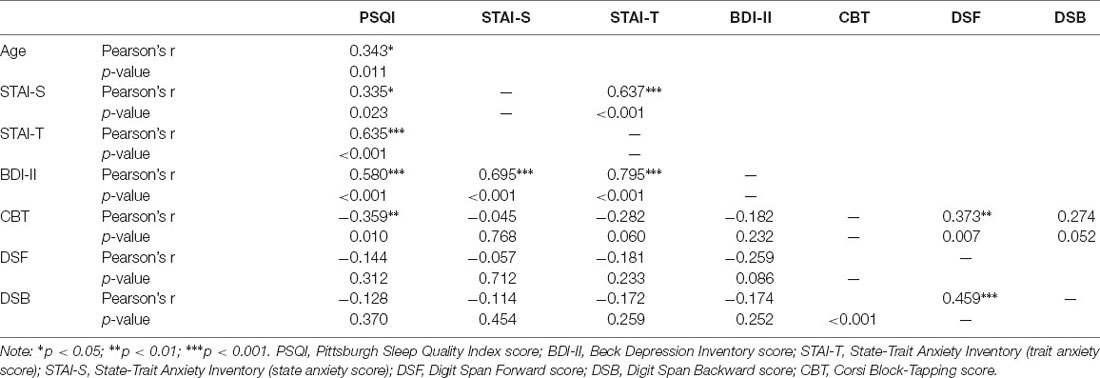
Table 3. Pearson’s correlation matrix concerning all scores at the neuropsychological tests involved in the study (i.e., STAI-T, STAI-S, BDI-II, DSF, DSB, CBT).
Discussion
We analysed the brain functional connectivity (FC) of a large sample of healthy participants of varying ages, taking into account the self-reported quality of their sleep according to the Pittsburgh Sleep Quality Index (PSQI; Buysse et al., 1989) and other neuropsychological measures related to anxiety (i.e., State-Trait anxiety inventory, STAI; Spielberger et al., 1983), depression (i.e., Beck Depression Inventory, BDI-II; Beck et al., 1996), verbal working memory (i.e., Forward and Backward Digit Span), and visuospatial working memory (i.e., Corsi Block-Tapping tasks). For each participant, we first carried out the brief neuropsychological assessment and then, on the same day, acquired 3T resting-state fMRI images. Given its interoperability with other high-level cognitive processes (e.g., reasoning, language comprehension, and learning), we considered participants’ working-memory-related scores as a general measure of cognitive functioning involved in daily tasks (Baddeley, 1992). Thus, on the one hand, we analysed some of the neuropsychological sequelae recurrently associated with sleep problems. On the other hand, we assessed whether, and if so how, sleep quality may modulate whole-brain FC. We did not involve a specific clinical sample, focusing on sleep problems possibly occurring in large-scale healthy populations across different ages. Our results showed that sleep quality might modulate the FC of a large set of limbic and fronto-temporo-parietal brain areas that are crucially involved in everyday cognitive functioning. Consistent with the neuroimage data, behavioural results show a neuropsychological profile characterised by higher anxiety, depression and worse cognitive performance among bad sleepers.
The main indication of this study concerns the modulation of resting-state FC as a function of participants’ self-reported sleep quality. We investigated FC by making distinct analyses at several levels of detail. As a first-level analysis, we considered the effects of self-reported sleep quality on FC by contrasting good sleepers (i.e., participants with PSQI score <5) with bad sleepers (i.e., participants with PSQI score >5). Such an analysis highlighted a diversified seed-to-seed FC modulation pattern involving multiple cortical and subcortical areas. In particular, bad sleepers exhibited FC reductions between right IC and right amygdala; between right IFGtri and both left and right IC, right aSMG, and left PP; between left PP and right amygdala. In bad sleepers, we also found FC increases between right IFG and left pMTG, left AG, and MedFC; between right pSTG and left iLOC; between right iLOC and right pSTG, left toITG, left IFG, and left SPL; between left SPL and right toITG; between left toITG and left OFC. The interplay among large-scale brain networks is critical for supporting cognitive functions since information processing in the cerebral cortex involves functional interactions among distributed brain areas (e.g., Yeo et al., 2011). Therefore, sleep-quality-related cognitive effects might reverberate on large-scale brain networks connectivity. Based on that, as a second-level analysis, we assessed between-network FC by considering PSQI scores as a distinct source of variance. Consistent with the first-level seed-to-seed analysis, we found a moderate positive correlation among the PSQI score, the Salience network (with the peak seed located in right SMG) and the Frontoparietal network (with the peak seed located in the right PPC).
We found peculiar FC modulation patterns concerning cortical and subcortical brain areas critically associated with distinct neurocognitive functions. For instance, the IC is part of the salience network, which permits the identification of behaviourally relevant stimuli in the environment, thus coordinating the allocation of attention and neural resources to them (e.g., Uddin, 2014). Extensive literature underlines how sleep may directly modulate IC activity (Flynn, 1999), and many fMRI studies highlighted the impact of sleep disturbances in altering the neural activity of IC alongside frontoparietal networks (e.g., Chee et al., 2006; Chuah et al., 2006; Venkatraman et al., 2011). Chronic sleep deprivation may negatively impact attention and alertness due to prefrontal cortex dysfunctions (Killgore, 2010). Such a “sleep-related hypofrontality” may produce waking-state instability, which may reverberate in several cognitive processes, such as working memory, visuomotor abilities and reasoning skills (Alhola and Polo-Kantola, 2007). Consistent with our behavioural results, impairments in working memory after sleep deprivation seem to be associated with reductions in prefrontal and parietal cortex activations (Mu et al., 2005). The functional hypoconnectivity we found in bad sleepers between right IFG and right SMG and the reduced performance at the working memory task seems to be rather suggestive in this respect. Activations of frontoparietal regions after normal sleep have been recently negatively correlated with working-memory performance decline from normal sleep to 24 h of sleep deprivation, differentiating individuals who maintained such performance following sleep deprivation from those who were more vulnerable to its effects (Chee et al., 2006). Thus, FC increases in different fronto-temporo-parietal regions might signal compensatory mechanisms which intervene as an effect of poor sleep quality, thereby differentiating individual tolerance to sleep deprivation. Whereas frontal lobes are particularly vulnerable to sleep disturbances, more posterior brain mechanisms might compensate for the relative deficits produced by the sleeping debt (e.g., Gosselin et al., 2005). Our results show both hyper- and hypo-activations in FC among areas of opposite hemispheres, possibly indicating how such a compensatory mechanism may involve both hemispheres. Concerning the subcortical involvement, recent evidence indicated that self-reported sleep quality might moderate the relationships among the amygdala reactivity, negative affectivity, and perceived stress (Prather et al., 2013). Therefore, limbic FC modulation and the anxious/depressive behavioural pattern we found in bad sleepers seem to support the strong connections highlighted in the literature among sleep disorders, anxiety/depressive disorders and negative affectivity (e.g., Alvaro et al., 2013; Prather et al., 2013).
By considering the behavioural data, we found multiple associations between self-reported sleep quality and the neuropsychological variables we analysed. In particular, we found positive correlations among the self-reported sleep quality score and both the anxiety scores we used (i.e., trait anxiety and state anxiety). We also found positive correlations between the self-reported sleep quality score and the depression score. In this respect, our findings are consistent with the literature, as sleep disorders have been often associated with behavioural disorders such as anxiety and depression (Alvaro et al., 2013). Indeed, about 90% of depressed individuals may complain of sleep disorders, while insomnia patients may have twice the risk of developing depression during their lifetime (Baglioni et al., 2011). It should be noted that a general dysfunctional emotional reactivity’s mechanism, which mediates the association between insomnia, depression and anxiety, has been recently proposed (Baglioni et al., 2010). Interestingly for our purposes, both anxiety and depression have been associated with attention, working memory and executive function impairments, which are neurocognitive functions mediated by the frontoparietal network (e.g., Kane and Engle, 2002; Castaneda et al., 2008; Rossi et al., 2009; Friedman and Robbins, 2022). Congruently, we found differences in the functional connectivity of these brain areas as a function of self-report sleep quality. Behaviourally, we found negative correlations between self-reported sleep quality and the visuospatial working memory’s task. Intriguingly, we found no correlations among self-reported sleep quality and verbal working memory tasks, thus suggesting sleep quality may impact working memory’s visuospatial component preferentially. It is worth noticing that right-brain networks are more involved in the visuospatial working memory than left ones, while left-brain structures are involved more than right ones in verbal working memory (Hennecke et al., 2021). Thus, spatial abilities are more related to right hemisphere activations (van Asselen et al., 2006). Consistent with that, we found a primarily right-lateralised FC hypoconnectivity in bad sleepers, which may have contributed to degrading visuospatial working memory performance.
The present study has some limitations concerning its cross-sectional nature (i.e., the inability to trace direct causal mechanisms between participants’ sleep quality and their behavioural phenotype) as well as the absence of a direct comparison between objective and subjective sleep quality’s measures (e.g., Landry et al., 2015; Hsiao et al., 2018). A third limitation concerns the convenience sample. However, post hoc evaluation of the literature indicated such a sample size as adequate for an exploratory fMRI study. Indeed, similar neuroimaging studies on the effects of poor sleep quality, sleep deprivation, and insomnia used even smaller samples (e.g., Bu et al., 2018; Kong et al., 2018; Li et al., 2019). Therefore, while the results we reported appear to be quite suggestive in indicating effects in the functional brain connectivity that might refer to the behavioural phenotype we found, further studies involving larger samples as well as objective measures of sleep quality should corroborate and extend the preliminary findings we presented here.
Conclusions
The preliminary findings we report here may help reveal the effects of sleep quality on daily-life cognitive functioning and further elucidate pathophysiological mechanisms of sleep disorders. Indeed, taken as a whole, our results support a consistent trend in literature that underlines how bad sleepers may expose a neurocognitive profile characterised by higher levels of anxiety and depression and lower visuospatial working memory functioning. Furthermore, we found differences in the functional brain connectivity that may reasonably refer to such a behavioural phenotype. Alongside drawing connections between sleep quality and subsequent cognitive and psychological effects, we speculated about a supplementary mechanism that may support the cognitive function in the case of qualitative and quantitative sleep deprivation. Although the idea we present may appear plausible and recent evidence seems to support it, further experimental evidence is required.
Data Availability Statement
The raw data supporting the conclusions of this article will be made available by the authors, without undue reservation.
Ethics Statement
The studies involving human participants were reviewed and approved by Ethics Committee of IRCCS Pascale. The patients/participants provided their written informed consent to participate in this study.
Author Contributions
GF, CC and VA conceived the study. GF, VA, FG and GM acquired behavioural and fMRI data and conducted the study. GF and VA analysed the data. GF wrote the manuscript’s first draft. VA, FG, GM, MA, MS and CC revised the manuscript and provided critical comments and theoretical contributions. All authors contributed to the article and approved the submitted version.
Funding
Ricerca Corrente projects of the Italian Ministry of Health supported this work. The funders had no role in study design, data collection and analysis, decision to publish, or manuscript preparation.
Conflict of Interest
The authors declare that the research was conducted in the absence of any commercial or financial relationships that could be construed as a potential conflict of interest.
Publisher’s Note
All claims expressed in this article are solely those of the authors and do not necessarily represent those of their affiliated organizations, or those of the publisher, the editors and the reviewers. Any product that may be evaluated in this article, or claim that may be made by its manufacturer, is not guaranteed or endorsed by the publisher.
Acknowledgements
We would like to thank Angelica Di Cecca, a trainee at IRCCS Synlab SDN (Naples, Italy), for her support during the manuscript’s first draft.
References
Alfano, V., Longarzo, M., Mele, G., Esposito, M., Aiello, M., Salvatore, M., et al. (2021). Identifying a common functional framework for apathy large-scale brain network. J. Pers. Med. 11:679. doi: 10.3390/jpm11070679
Alhola, P., and Polo-Kantola, P. (2007). Sleep deprivation: impact on cognitive performance. J. Neuropsychiatr. Dis. Treat. 3, 553–567.
Alvaro, P. K., Roberts, R. M., and Harris, J. K. (2013). A systematic review assessing bidirectionality between sleep disturbances, anxiety and depression. Sleep 36, 1059–1068. doi: 10.5665/sleep.2810
Babkoff, H., Sing, H. C., Thorne, D. R., Genser, S. G., and Hegge, F. W. (1989). Perceptual distortions and hallucinations reported during the course of sleep deprivation. Percept. Mot. Skills 68, 787–798. doi: 10.2466/pms.1989.68.3.787
Baglioni, C., Spiegelhalder, K., Lombardo, C., and Riemann, D. (2010). Sleep and emotions: a focus on insomnia. Sleep Med. Rev. 14, 227–238. doi: 10.1016/j.smrv.2009.10.007
Baglioni, C., Spiegelhalder, K., Nissen, C., and Riemann, D. (2011). Clinical implications of the causal relationship between insomnia and depression: how individually tailored treatment of sleeping difficulties could prevent the onset of depression. EPMA J. 2, 287–293. doi: 10.1007/s13167-011-0079-9
Beck, A. T., Steer, R. A., and Brown, G. K. (1996). Manual for the Beck Depression Inventory-II. San Antonio, TX: Psychological Corporation.
Benjamini, Y., and Hochberg, Y. (1995). Controlling the false discovery rate: a practical and powerful approach to multiple testing author(s). J. R. Stat. Soc. Ser. B 57, 289–300. doi: 10.2307/2346101
Broman, J. E., Lundh, L. G., and Hetta, J. (1996). Insufficient sleep in the general population. Neurophysiol. Clin. 26, 30–39. doi: 10.1016/0987-7053(96)81532-2
Brunborg, G. S., Mentzoni, R. A., Molde, H., Myrseth, H., Skouverøe, K. J. M., Bjorvatn, B., et al. (2011). The relationship between media use in the bedroom, sleep habits and symptoms of insomnia. J. Sleep Res. 20, 569–575. doi: 10.1111/j.1365-2869.2011.00913.x
Bu, L., Wang, D., Huo, C., Xu, G., Li, Z., and Li, J. (2018). Effects of poor sleep quality on brain functional connectivity revealed by wavelet-based coherence analysis using NIRS methods in elderly subjects. Neurosci. Lett. 668, 108–114. doi: 10.1016/j.neulet.2018.01.026
Buysse, D. J., Reynolds III, C. F., Monk, T. H., Berman, S. R., and Kupfer, D. J. (1989). The pittsburgh sleep quality index: a new instrument for psychiatric practice and research. Psychiatry Res. 28, 193–213. doi: 10.1016/0165-1781(89)90047-4
Buzsáki, G., and Watson, B. O. (2012). Brain rhythms and neural syntax: implications for efficient coding of cognitive content and neuropsychiatric disease. Dialogues Clin. Neurosci. 14, 345–367. doi: 10.31887/DCNS.2012.14.4/gbuzsaki
Castaneda, A. E., Tuulio-Henriksson, A., Marttunen, M., Suvisaari, J., and Lönnqvist, J. (2008). A review on cognitive impairments in depressive and anxiety disorders with a focus on young adults. J. Affect. Disord. 106, 1–27. doi: 10.1016/j.jad.2007.06.006
Cavaliere, C., Longarzo, M., Fogel, S., Engström, M., and Soddu, A. (2020). Neuroimaging of narcolepsy and primary hypersomnias. Neuroscientist 26, 310–327. doi: 10.1177/1073858420905829
Chee, M. W., and Choo, W. C. (2004). Functional imaging of working memory after 24 hr of total sleep deprivation. J. Neurosci. 24, 4560–4567. doi: 10.1523/JNEUROSCI.0007-04.2004
Chee, M. W. L., Chuah, L. Y. M., Venkatraman, V., Chan, W. Y., Philip, P., and Dinges, D. F. (2006). Functional imaging of working memory following normal sleep and after 24 and 35 h of sleep deprivation: correlations of fronto-parietal activation with performance. Neuroimage 31, 419–428. doi: 10.1016/j.neuroimage.2005.12.001
Chuah, Y. L., Venkatraman, V., Dinges, D. F., and Chee, M. W. (2006). The neural basis of interindividual variability in inhibitory efficiency after sleep deprivation. J. Neurosci. 26, 7156–7162. doi: 10.1523/JNEUROSCI.0906-06.2006
Conroy, D. A., and Arnedt, J. T. (2014). Sleep and substance use disorders: an update. Curr. Psychiatry Rep. 16:487. doi: 10.1007/s11920-014-0487-3
Curcio, G., Tempesta, D., Scarlata, S., Marzano, C., Moroni, F., Rossini, P. M., et al. (2013). Validity of the Italian version of the Pittsburgh sleep quality index (PSQI). Neurol. Sci. 34, 511–519. doi: 10.1007/s10072-012-1085-y
Desseilles, M., Dang-Vu, T., Schabus, M., Sterpenich, V., Maquet, P., and Schwartz, S. (2008). Neuroimaging insights into the pathophysiology of sleep disorders. Sleep 31, 777–794. doi: 10.1093/sleep/31.6.777
Flynn, F. G. (1999). Anatomy of the insula functional and clinical correlates. Aphasiology 13, 55–78. doi: 10.1080/026870399402325
Foley, D. J., Monjan, A. A., Masaki, K. H., Enright, P. L., Quan, S. F., and White, L. R. (1999). Associations of symptoms of sleep apnea with cardiovascular disease, cognitive impairment and mortality among older Japanese-American men. J. Am. Geriatr. Soc. 47, 524–528. doi: 10.1111/j.1532-5415.1999.tb02564.x
Friedman, N. P., and Robbins, T. W. (2022). The role of prefrontal cortex in cognitive control and executive function. Neuropsychopharmacology 47, 72–89. doi: 10.1038/s41386-021-01132-0
Garcia, A. N., and Salloum, I. M. (2015). Polysomnographic sleep disturbances in nicotine, caffeine, alcohol, cocaine, opioid and cannabis use: a focused review. Am. J. Addict. 24, 590–598. doi: 10.1111/ajad.12291
Goldstein, A. N., and Walker, M. P. (2014). The role of sleep in emotional brain function. Annu. Rev. Clin. Psychol. 10, 679–708. doi: 10.1146/annurev-clinpsy-032813-153716
Gooneratne, N. S., and Vitiello, M. V. (2014). Sleep in older adults: normative changes, sleep disorders and treatment options. Clin. Geriatr. Med. 30, 591–627. doi: 10.1016/j.cger.2014.04.007
Gosselin, A., De Koninck, J., and Campbell, K. B. (2005). Total sleep deprivation and novelty processing: implications for frontal lobe functioning. Clin. Neurophysiol. 116, 211–222. doi: 10.1016/j.clinph.2004.07.033
Gulia, K. K., and Kumar, V. M. (2018). Sleep disorders in the elderly: a growing challenge. Psychogeriatrics 18, 155–165. doi: 10.1111/psyg.12319
Hartse, K. M. (2011). The phylogeny of sleep. Handb. Clin. Neurol. 98, 97–109. doi: 10.1016/B978-0-444-52006-7.00007-1
Hennecke, E., Lange, D., Steenbergen, F., Fronczek-Poncelet, J., Elmenhorst, D., Bauer, A., et al. (2021). Adverse interaction effects of chronic and acute sleep deficits on spatial working memory but not on verbal working memory or declarative memory. J. Sleep Res. 30:e13225. doi: 10.1111/jsr.13225
Holley, S., Hill, C. M., and Stevenson, J. (2011). An hour less sleep is a risk factor for childhood conduct problems. Child Care Health Dev. 37, 563–570. doi: 10.1111/j.1365-2214.2010.01203.x
Hsiao, F. C., Tsai, P. J., Wu, C. W., Yang, C. M., Lane, T. J., Lee, H. C., et al. (2018). The neurophysiological basis of the discrepancy between objective and subjective sleep during the sleep onset period: an EEG-fMRI study. Sleep 41:zsy056. doi: 10.1093/sleep/zsy056
Kane, M. J., and Engle, R. W. (2002). The role of prefrontal cortex in working-memory capacity, executive attention and general fluid intelligence: an individual-differences perspective. Psychon. Bull. Rev. 9, 637–671. doi: 10.3758/bf03196323
Killgore, W. D. (2010). Effects of sleep deprivation on cognition. Prog. Brain Res. 185, 105–129. doi: 10.1016/B978-0-444-53702-7.00007-5
Kong, D., Liu, R., Song, L., Zheng, J., Zhang, J., and Chen, W. (2018). Altered long-and short-range functional connectivity density in healthy subjects after sleep deprivations. Front. Neurol. 9:546. doi: 10.3389/fneur.2018.00546
Kronholm, E., Sallinen, M., Suutama, T., Sulkava, R., Era, P., and Partonen, T. (2009). Self-reported sleep duration and cognitive functioning in the general population. J. Sleep Res. 18, 436–446. doi: 10.1111/j.1365-2869.2009.00765.x
Kuriyama, K., Mishima, K., Suzuki, H., Aritake, S., and Uchiyama, M. (2008). Sleep accelerates the improvement in working memory performance. J. Neurosci. 28, 10145–10150. doi: 10.1523/JNEUROSCI.2039-08.2008
Landry, G. J., Best, J. R., and Liu-Ambrose, T. (2015). Measuring sleep quality in older adults: a comparison using subjective and objective methods. Front. Aging Neurosci. 7:166. doi: 10.3389/fnagi.2015.00166
Lemola, S., Ledermann, T., and Friedman, E. M. (2013). Variability of sleep duration is related to subjective sleep quality and subjective wellbeing: an actigraphy study. PLoS One 8:e71292. doi: 10.1371/journal.pone.0071292
Li, M., Wang, R., Zhao, M., Zhai, J., Liu, B., Yu, D., et al. (2019). Abnormalities of thalamus volume and resting state functional connectivity in primary insomnia patients. Brain Imaging Behav. 13, 1193–1201. doi: 10.1007/s11682-018-9932-y
Lim, J., and Dinges, D. F. (2010). A meta-analysis of the impact of short-term sleep deprivation on cognitive variables. Psychol. Bull. 136, 375–389. doi: 10.1037/a0018883
Mander, B. A., Winer, J. R., Jagust, W. J., and Walker, M. P. (2016). Sleep: a novel mechanistic pathway, biomarker and treatment target in the pathology of Alzheimer’s disease? Trends Neurosci. 39, 552–566. doi: 10.1016/j.tins.2016.05.002
Mu, Q., Nahas, Z., Johnson, K. A., Yamanaka, K., Mishory, A., Koola, J., et al. (2005). Decreased cortical response to verbal working memory following sleep deprivation. Sleep. 28, 55–67. doi: 10.1093/sleep/28.1.55
Ohayon, M. M. (2011). Epidemiological overview of sleep disorders in the general population. Sleep Med. Res. 2, 1–9. doi: 10.17241/SMR.2011.2.1.1
Petrovsky, N., Ettinger, U., Hill, A., Frenzel, L., Meyhöfer, I., Wagner, M., et al. (2014). Sleep deprivation disrupts prepulse inhibition and induces psychosis-like symptoms in healthy humans. J. Neurosci. 34, 9134–9140. doi: 10.1523/JNEUROSCI.0904-14.2014
Poceta, J. S., and Mitler, M. M. (2013). Sleep Disorders: Diagnosis and Treatment. New Delhi, India: Springer Science and Business Media.
Prather, A. A., Bogdan, R., and Hariri, P. A. R. (2013). Impact of sleep quality on amygdala reactivity, negative affect and perceived stress. Psychosom. Med. 75, 350–358. doi: 10.1097/PSY.0b013e31828ef15b
Reimer, M. A., and Flemons, W. W. (2003). Quality of life in sleep disorders. Sleep Med. Rev. 7, 335–349. doi: 10.1053/smrv.2001.0220
Reimund, E. (1994). The free radical flux theory of sleep. Med. Hypotheses 43, 231–233. doi: 10.1016/0306-9877(94)90071-x
Rossi, A. F., Pessoa, L., Desimone, R., and Ungerleider, L. G. (2009). The prefrontal cortex and the executive control of attention. Exp. Brain Res. 192, 489–497. doi: 10.1007/s00221-008-1642-z
Sil’kis, I. G. (2014). Possible mechanisms for impairments to learning, memory and attention due to sleep deprivation. Neurosci. Behav. Physiol. 44, 576–583. doi: 10.1007/s11055-014-9954-x
Spiegelhalder, K., Regen, W., Baglioni, C., Nissen, C., Riemann, D., and Kyle, S. D. (2015). Neuroimaging insights into insomnia. Curr. Neurol. Neurosci. Rep. 15:9. doi: 10.1007/s11910-015-0527-3
Spielberger, C. D., Gorsuch, R. L., Lushene, R., Vagg, P. R., and Jacobs, G. A. (1983). Manual for the State-Trait Anxiety Inventory. Palo Alto, CA: Consulting Psychologists Press.
Tworoger, S. S., Lee, S., Schernhammer, E. S., and Grodstein, F. (2006). The association of self-reported sleep duration, difficulty sleeping and snoring with cognitive function in older women. Alzheimer Dis. Assoc. Disord. 20, 41–48. doi: 10.1097/01.wad.0000201850.52707.80
Uddin, L. Q. (2014). Insula: Neuroanatomy, Functions and Clinical Disorders. Hauppauge, NY: Nova Science Publishers, Inc.
van Asselen, M., Kessels, R. P., Neggers, S. F., Kappelle, L. J., Frijns, C. J., and Postma, A. (2006). Brain areas involved in spatial working memory. Neuropsychologia 44, 1185–1194. doi: 10.1016/j.neuropsychologia.2005.10.005
Venkatraman, V., Huettel, S. A., Chuah, L. Y., Payne, J. W., and Chee, M. W. (2011). Sleep deprivation biases the neural mechanisms underlying economic preferences. J. Neurosci. 31, 3712–3718. doi: 10.1523/JNEUROSCI.4407-10.2011
Walker, M. P. (2009). The role of sleep in cognition and emotion. Ann. N Y Acad. Sci. 1156, 168–197. doi: 10.1111/j.1749-6632.2009.04416.x
Waters, F., and Bucks, R. S. (2011). Neuropsychological effects of sleep loss: implication for neuropsychologists. J. Int. Neuropsychol. Soc. 17, 571–586. doi: 10.1017/S1355617711000610
Waters, F., Chiu, V., Atkinson, A., and Blom, J. D. (2018). Severe sleep deprivation causes hallucinations and a gradual progression toward psychosis with increasing time awake. Front. Psychiatry 9:303. doi: 10.3389/fpsyt.2018.00303
Whitfield-Gabrieli, S., and Nieto-Castanon, A. (2012). Conn: a functional connectivity toolbox for correlated and anticorrelated brain networks. Brain Connect 2, 125–141. doi: 10.1089/brain.2012.0073
Keywords: subjective sleep quality, functional connectivity, anxiety, depression, working memory
Citation: Federico G, Alfano V, Garramone F, Mele G, Salvatore M, Aiello M and Cavaliere C (2022) Self-Reported Sleep Quality Across Age Modulates Resting-State Functional Connectivity in Limbic and Fronto-Temporo-Parietal Networks: An Exploratory Cross-Sectional fMRI Study. Front. Aging Neurosci. 14:806374. doi: 10.3389/fnagi.2022.806374
Received: 31 October 2021; Accepted: 12 January 2022;
Published: 07 February 2022.
Edited by:
Alison Mary, Université libre de Bruxelles, BelgiumReviewed by:
Arun Sasidharan, National Institute of Mental Health and Neurosciences, IndiaNadine Correia Santos, University of Minho, Portugal
Copyright © 2022 Federico, Alfano, Garramone, Mele, Salvatore, Aiello and Cavaliere. This is an open-access article distributed under the terms of the Creative Commons Attribution License (CC BY). The use, distribution or reproduction in other forums is permitted, provided the original author(s) and the copyright owner(s) are credited and that the original publication in this journal is cited, in accordance with accepted academic practice. No use, distribution or reproduction is permitted which does not comply with these terms.
*Correspondence: Vincenzo Alfano, dmluY2Vuem8uYWxmYW5vOTFAZ21haWwuY29t