Relationship between physical activity and cerebral white matter hyperintensity volumes in older adults with depressive symptoms and mild memory impairment: a cross-sectional study
- 1Department of Laboratory and Vascular Medicine, Graduate School of Medical and Dental Sciences, Kagoshima University, Kagoshima, Japan
- 2Division of Brain Science, Department of Physiology, Kurume University School of Medicine, Kurume, Japan
- 3Department of Neurosurgery, Kurume University School of Medicine, Kurume, Japan
- 4Department of Physical Therapy, School of Health Sciences, Faculty of Medicine, Kagoshima University, Kagoshima, Japan
Introduction: Cerebral white matter hyperintensities (WMHs) are commonly found in the aging brain and have been implicated in the initiation and severity of many central nervous system diseases. Furthermore, an increased WMH volume indicates reduced brain health in older adults. This study investigated the association between WMH volume and physical activity in older adults with depressive symptoms (DS) and mild memory impairment (MMI). Factors associated with the WMH volume were also investigated.
Methods: A total of 57 individuals aged over 65 years with DS and MMI were included in this study. The participants underwent magnetic resonance imaging to quantify WMH volumes. After WMH volume was accumulated, normalized to the total intracranial volume (TIV), the percentage of WMH volume was calculated. In addition, all participants wore a triaxial accelerometer for 2 weeks, and the average daily physical activity and number of steps were measured. The levels of blood biomarkers including cortisol, interleukin-6 (IL-6), brain-derived insulin-like growth factor-1, and brain-derived neurotrophic factor were measured. Motor and cognitive functions were also assessed.
Results: Faster maximum walking speed and longer time spent engaged in moderate physical activity were associated with a smaller percent of WMH volume, whereas higher serum IL-6 levels were associated with a larger percent of WMH volume. The number of steps per day, time spent engaged in low levels of physical activity, cognitive function, and all other measured biomarkers were not significantly associated with percent of WMH volume.
Discussion: Higher blood inflammatory cytokine levels, shorter duration of moderate physical activity, and lower maximum walking speed were associated with a higher percent of WMH volume. Our results provide useful information for maintaining brain health in older adults at a high risk of developing dementia and may contribute to the development of preventive medicine for brain health.
1 Introduction
Magnetic resonance imaging (MRI) often reveals cerebral white matter hyperintensities (WMHs) in older adults. WMHs are a primary manifestation of white matter damage, including damage to small blood vessels, amyloid angiopathy, ischemic hypoxic damage, altered blood–brain barrier properties, demyelination, and axonal loss (Fernando et al., 2006; Black et al., 2009; Gouw et al., 2011; Han et al., 2019). WMHs are common in older adults and their volume increases over time (Erten-Lyons et al., 2013; Promjunyakul et al., 2015). In addition, numerous risk factors for cardiovascular diseases such as smoking and hypertension increase the prevalence of WMHs (Staals et al., 2014). WMHs can be anatomically divided into deep subcortical WMHs (DSWMHs) and periventricular hyperintensities (PVHs) based on their location and appearance. The involvement of DSWMHs in mood disorders has been reported (De Groot et al., 2000), whereas PVHs are associated with cognitive memory impairment (Bolandzadeh et al., 2012). Furthermore, larger WMH volumes are associated with a higher risk of Alzheimer’s disease (Gaubert et al., 2021), stroke (Kuller et al., 2004; Wen and Sachdev, 2004), motor dysfunction (Silbert et al., 2008), and mortality (Debette et al., 2010). However, there are currently no specific preventive measures or treatments for reducing WMH volume. Thus, to improve the early detection of WMHs, it is crucial to identify risk factors.
Several studies have documented the neuroprotective effects of physical activity against Alzheimer’s disease, stroke, lacunar infarction, and traumatic head injuries. For example, a study of 55 older adults with mild memory impairment suggested that 16 weeks of aerobic exercise was effective in increasing neurotrophic factors, decreasing certain inflammatory cytokines, and promoting neurocognitive function (Tsai et al., 2019). Moreover, animal studies have reported that treadmill training for 3 weeks before stroke onset increases neuroprotective factor expression and has neuroprotective effects, resulting in reduced infarct volume and motor sensory deficits (Otsuka et al., 2016, 2019). In addition, physical activity has beneficial effects on WMHs. A study showed that higher self-rated physical activity at baseline was linked to smaller WMH volume in the deep and periventricular regions after 3 years (Gow et al., 2012). Another study showed a relationship between increased self-rated physical activity at baseline and WMH (periventricular and deep) progression at 5 years in a cognitively intact group only (Bolandzadeh et al., 2012). However, another 5-year follow-up study of 180 people aged ≥65 years showed no association between physical activity and progression of WMH (Podewils et al., 2007). Notably, most previous studies used self-administered questionnaires to investigate physical activity. Self-reported physical activity is vulnerable to bias caused by inaccurate memory and other factors that may lead to measurement errors, preventing detailed physical activity data from being obtained (Prince et al., 2008). Direct measurement of physical activity using pedometers and triaxial accelerometers may eliminate these errors. In a previous study, physical activity was measured over a short period of 1 week to assess its association with WMH volume (Bangen et al., 2023). Nevertheless, there are limited studies on the use of triaxial accelerometers to measure physical activity and how it relates to WMH volume.
WMHs are associated with several risk factors including aging, hypertension, smoking, body mass index, and the presence of heart disease or diabetes (Johnson et al., 2019; Garfield et al., 2021). Additionally, WMH volume may be influenced by neurotrophic factors that confer neuroprotective effects and inflammatory cytokines involved in various cerebrovascular disorders. Although individual serum biomarkers and certain risk factors related to brain structural changes and WMH volumes are not well established, the identification of WMH-related serum biomarkers will aid in the development of interventions aimed at maintaining brain health in older adults.
Depressive symptoms (DS) and memory loss are common in older adults. Furthermore, older adults with both DS and memory loss are more likely to develop dementia (Paterniti et al., 2002; Barnes and Yaffe, 2011; Yaffe et al., 2011), which is likely to also be associated with increased WMH volumes. However, to date, no studies have investigated the relationship between WMH volume and daily physical activity in older adults with DS and mild memory impairment (MMI). Previous studies examining WMHs have focused on healthy older adults and those with dementia. However, no studies have investigated older adults presenting with symptoms of depressive tendencies and MMI associated with the development of dementia. Depressive tendencies and MMI may improve, and investigating the relationship between white matter lesion volume and physical activity level may provide important information for health management of older adults. Therefore, the present study focused on the association between white matter lesion volume and physical activity volume in community-dwelling older adults (aged ≥65 years) with DS and MMI, who are at a high risk of developing dementia. We also aimed to identify factors (blood biomarkers, mobility, and memory function) that may be associated with WMH and physical activity volumes.
2 Materials and methods
2.1 Study design and participants
This study analyzed the baseline data from previous community-based, single-blind, randomized controlled experiments conducted in Japan (Makizako et al., 2015a, 2019). The clinical investigation was preregistered with the clinical trial registration of the University Hospital Medical Information Network (000018547). This interventional study was conducted between February 2015 and October 2015 in Obu, Japan. In all, 2,524 older adults aged ≥65 years were enrolled in this study. From this pool, 406 patients with DS and MMI were selected (Figure 1). The inclusion criteria were as follows: (1) individuals aged ≥65 years residing in a community on their own, (2) exhibiting depressive symptoms with a Geriatric Depression Scale-15 (GDS-15) score of at least 529, and (3) memory impairment, as evidenced by an age-adjusted word list memory score > 1.0 standard deviation (SD) below the criteria threshold, which may be either subjective memory impairment or objectively measured moderate memory loss. The following were the exclusion requirements: (1) obtaining assistance or care as attested by the government-run Japanese long-term care insurance system, (2) a Mini-Mental State Examination (MMSE) score of <18 or a diagnosis of dementia, (3) a history of a severe mental illness or another neurological or musculoskeletal condition, (4) any disability that affects basic activities of daily living, (5) inability to undertake tests of cognitive performance, (6) contraindications for physical exercise, and (7) daily use of walking aids. Finally, data from 57 older adults were analyzed (see Figure 1 for details of the excluded participants). All 57 patients underwent MRI for WMH analysis. Prior to enrolment, the participants provided written informed consent. The study protocol was approved by the Ethics Committee of the National Center for Geriatrics and Gerontology in Japan (#839).
2.2 Setting
The investigation was conducted in the neighborhood of Nagoya, Japan, in Obu City. Since 2011, we have been conducting observational research in this area, including in-person interviews and evaluations of physical and mental capacity (Shimada et al., 2013). Data collection and participant screening were performed at a community center.
2.3 Data collection
Between February 2015 and October 2015, participants were required to participate in 2 h of baseline data collection, wherein they were assessed for general health (including blood tests), physical ability, and cognitive function. In addition, the National Center for Geriatrics and Gerontology collected MRI data to study WMHs in the brain. In the present study, we selected measures reported to be associated with WMH volume. Physical performance was assessed using a grip strength test to measure muscle strength, up-and-go test with timing to assess balance, and maximal gait speed test to measure general walking ability (Pinter et al., 2018). Grip strength, timed up-and-go, and gait speed tests are easy and simple to administer in community settings and are powerful predictors of health outcomes (Rantanen, 2003). The MMSE and word-list memory tasks (Makizako et al., 2013) were used to assess cognitive function. The MMSE is a 10-min test that evaluates general cognitive and memory capabilities. This examination includes 30 questions assessing memory, orientation, attention, math, language, and vision. The word-list memory challenge involved both delayed and quick recall of a target list of 10 words presented for 2 s. Next, 30 words (10 target words and 20 interference phrases) were proposed, and the participants were prompted to immediately select the 10 target words. This procedure was repeated thrice. The total score is determined by calculating the average number of correct responses. In addition, for a “delayed” score, the participants had to recollect 10 target phrases after around 20 min. The GDS-15 was used to assess depressive symptoms. A score of <5 on the 15-item GDS-15 was used to identify the presence of clinical depression symptoms (van Marwijk et al., 1995).
During the 2 weeks after baseline measurements, physical activity was continually measured, as detailed in a previous study (Makizako et al., 2015b) using a 3-axis accelerometer (modified HJA-350IT, Active Style Pro, Omron Healthcare Co., Ltd., Kyoto, Japan) (Oshima et al., 2010). For 2 weeks, the participants were instructed to wear an accelerometer on a waistband. Metabolic equivalents (multiples of the resting metabolic rate) were used to express the output. The non-wearing time was defined as the time at which the accelerometer data were not recorded for a period longer than a minute. We defined a low level of physical activity as 1.5–2.9 metabolic equivalents and a moderate level as 3.0–5.9 metabolic equivalents, as per a previous study (Pate et al., 1995). Every 4 s, the accelerometer counted the steps taken and calculated the amount of physical activity based on the pattern of the accelerometer signal (Oshima et al., 2010; Ohkawara et al., 2011; Moniruzzaman et al., 2020). Prior to calculating the parameters, the activity time data were examined using a computer program; if there was a continuous interval during which no body movements were recorded, it indicated a period of time during which the monitor was removed (e.g., while bathing or sleeping). Participants who did not record ≥75 percent of daytime activities (i.e., from 6 a.m. to 6 p.m.) for at least 7 days throughout the 2-week period were excluded from the study. To prevent the participants from monitoring their step counts and values to gauge their typical daily activities over the 2-week period, the accelerometer displays were blinded.
The following biomarker concentrations were measured in blood samples related to WMH volume: cortisol (Cox et al., 2015), hemoglobin A1c (HbA1c) (De Havenon et al., 2019), interleukin (IL)-6 (Satizabal et al., 2012), insulin-like growth factor-1 (IGF-1) (Wittfeld et al., 2022), highest-density lipoprotein (HDL) cholesterol (Johnson et al., 2019), total cholesterol (Williams et al., 2013), and brain-derived neurotrophic factor (BDNF) (Dalby et al., 2013). Serum IL-6 levels were measured using a validated chemiluminescent enzyme immunoassay (Shiga et al., 2016), serum IGF-1 levels were quantified using an IGF-1 immunoradiometric assay (Daiichi; TFB Inc., Tokyo, Japan) (Doi et al., 2015), and serum BDNF levels were assessed using a Quantizing Human Kit (R&D Systems, Inc., Minneapolis, MN, United States). Assays were performed at SRL Tokyo Laboratories Inc., (Tokyo, Japan) (Suzuki et al., 2013).
2.4 Measurements of WMH volumes
WMH volume was measured as previously described (Syaifullah et al., 2020, 2021). A 3 T MRI scanner (TIM Trio, Siemens, Munich, Germany) was used to scan the entire brain. Participants who could not be accurately scanned in a single scan were excluded. Prior to voxel-based morphometric analysis, the MRI images were converted into files that conformed to the Neuroimaging Informatics Technology Initiative. Scan images were edited by Brain Anatomical Analysis Using Diffeomorphic Deformation (BAAD version 4.3; accessible at http://www.shiga-med.ac.jp/hqbioph/BAAD (English)/BAAD.html). WMHs were divided into DSWHMs and PVHs, and their volumes were calculated from fluid-attenuated inversion recovery (FLAIR) images using BAAD software.
The brain MRI acquisition protocol has been described in detail in previous studies (Vellas et al., 2014; Moon et al., 2017). Briefly, three-dimensional (3D) volume acquisition of a T1-weighted gradient-echo sequence (inversion time [TI], 800 ms; repetition time [TR], 1800 ms; echo time [TE], 1.98 ms; slice thickness, 1.1 mm) was acquired to create a gapless series of thin sagittal sections using the gradient echo sequence with quick magnetization preparation acquisition (TI, 800 ms; TR, 1800 ms; TE, 1.98 ms; slice thickness, 1.1 mm). Axial FLAIR images (TI, 2500 ms; TR, 9000 ms; TE, 100 ms; slice thickness, 5.0 mm) and T2-weighted spin-echo images (TR, 4200 ms; TE, 89.0 ms; slice thickness, 5.0 mm) were acquired.
All parameters in the preprocessing steps were determined using default settings. Briefly, images were set around the anterior commissure–posterior commissure line and resampled with a 1-mm3 voxel size. Computational Anatomy Toolbox 12 (Structural Brain Mapping Group, Jena University Hospital, Jena, Germany) was used for tissue segmentation and intensity nonuniformity correction. Because WMHs exert signal intensities, such as gray matter, on T1-weighted images, we conducted automated WMH correction of the FLAIR images using the Lesion Segmentation Toolbox software (Schmidt et al., 2012). The WMH was masked using the average signal intensity of the surrounding normal white matter prior to gray and white matter segmentation. Total intracranial volume in cm3 and WMH volume in cm3 were measured using MRI. The tissue volumes provided by the SPM5 toolbox1 were used to calculate the total intracranial volume from the 3D T1-weighted data. Using software that automatically separates WMH from FLAIR and 3D T1-weighted images, WMH volumes were calculated from MRI scans using white matter hyperintensity (Samaille et al., 2012). In contrast to the intensity-based approach, this method relies on the contrast. The typical preprocessing steps were as follows: tissue information was derived from the 3D T1-weighted images and then registered to the FLAIR images to correct for intensity inhomogeneities. The WMH contrast in the FLAIR image was then improved using a nonlinear diffusion framework to produce a piecewise constant image. Finally, a pertinent region was selected based on the location of the tissue profile data collected during the preprocessing stage. Consequently, we calculated WMH volume (mL) from the MRI images (Figure 2). After WMH volume accumulation was normalized to the total intracranial volume (TIV), the percentage of WMH volume (percent of WMH) was calculated.
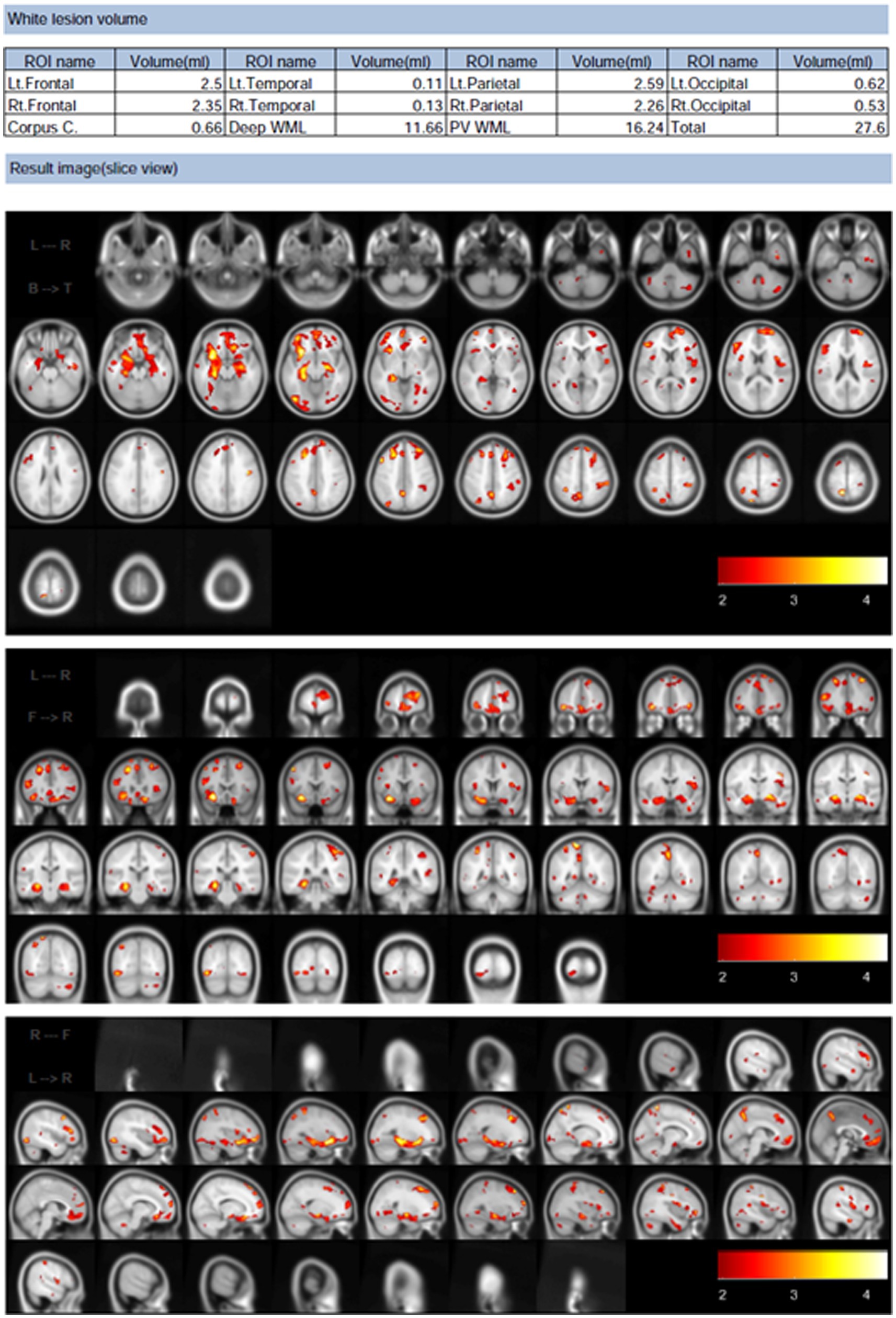
Figure 2. Example of white matter hyperintensities volume analysis results calculated using Brain Anatomical Analysis Using Diffeomorphic Deformation software. These results are of the horizontal, coronal, and sagittal planes analysis, wherein white matter lesions are brightened in red and other colors.
2.5 Statistical analysis
SPSS 27.0 for Windows (SPSS, Inc., Chicago, IL, United States) was used for the statistical analyses. The data has been presented as means ± SDs. Multivariable linear regression was used to examine (1) the correlations between percent of WMH (DSWMH and PVH) volumes and body mass index, motor function assessment (grip strength, up-and-go time, and maximum walking speed), DS, and cognitive function (i.e., GDS-15, MMSE, and word list memory [immediate and delayed]); (2) the relationship between percent of WMH volumes and blood biomarkers (i.e., cortisol, HDL and total cholesterol, IL-6, IGF-1, BDNF, and HbA1c); (3) the relationship between percent of WMH volumes and physical activity (i.e., number of steps, time spent doing a low level of activity, and time spent doing a moderate level of activity); (4) the relationship between IL-6 level and times spent doing a moderate level of activity and maximum walking speed.
All regression models were evaluated using the Pearson’s correlation coefficient (r). Furthermore, age and sex were adjusted (β). Differences were considered statistically significant at p < 0.05.
3 Results
3.1 Relationships between percent of WMH volumes and participant characteristics
In this study, a secondary examination of data from a previous study was conducted (Makizako et al., 2019). Table 1 presents information on the participant characteristics. The mean age of the participants was 73.03 years, and 42.1% (n = 24) were female. Additionally, 43.9% (n = 25) of the participants had hypertension and 24.6% (n = 14) had diabetes. The mean (SD) TIV of the participants was 1505.57 mL (133.04 mL). The DSWMH volume was 0.67% (0.12%) and PVH volume was 0.59% (0.45%). Table 2 shows the relationships between the 2 % of WMH volumes (DSWMH and PVH) and participant characteristics (body mass index, physical performance, GDS-15, and general cognitive function test), which were evaluated using the Pearson’s correlation coefficient (r). Furthermore, age and sex (β) were adjusted. Body mass index, GDS-15, and general cognitive function tests were not significantly correlated with percent of WMH volumes. Regarding physical performance, no significant correlations were found between grip strength, time up and go, and percent WMH volumes. However, a significant correlation was found between maximal walking speed and percent of DSWMH volume (β = −0.318, p = 0.018) or percent of PVH volume (β = −0.328, p = 0.015). It was clear that the higher the maximum walking speed, the lower the percent of WMH volumes.
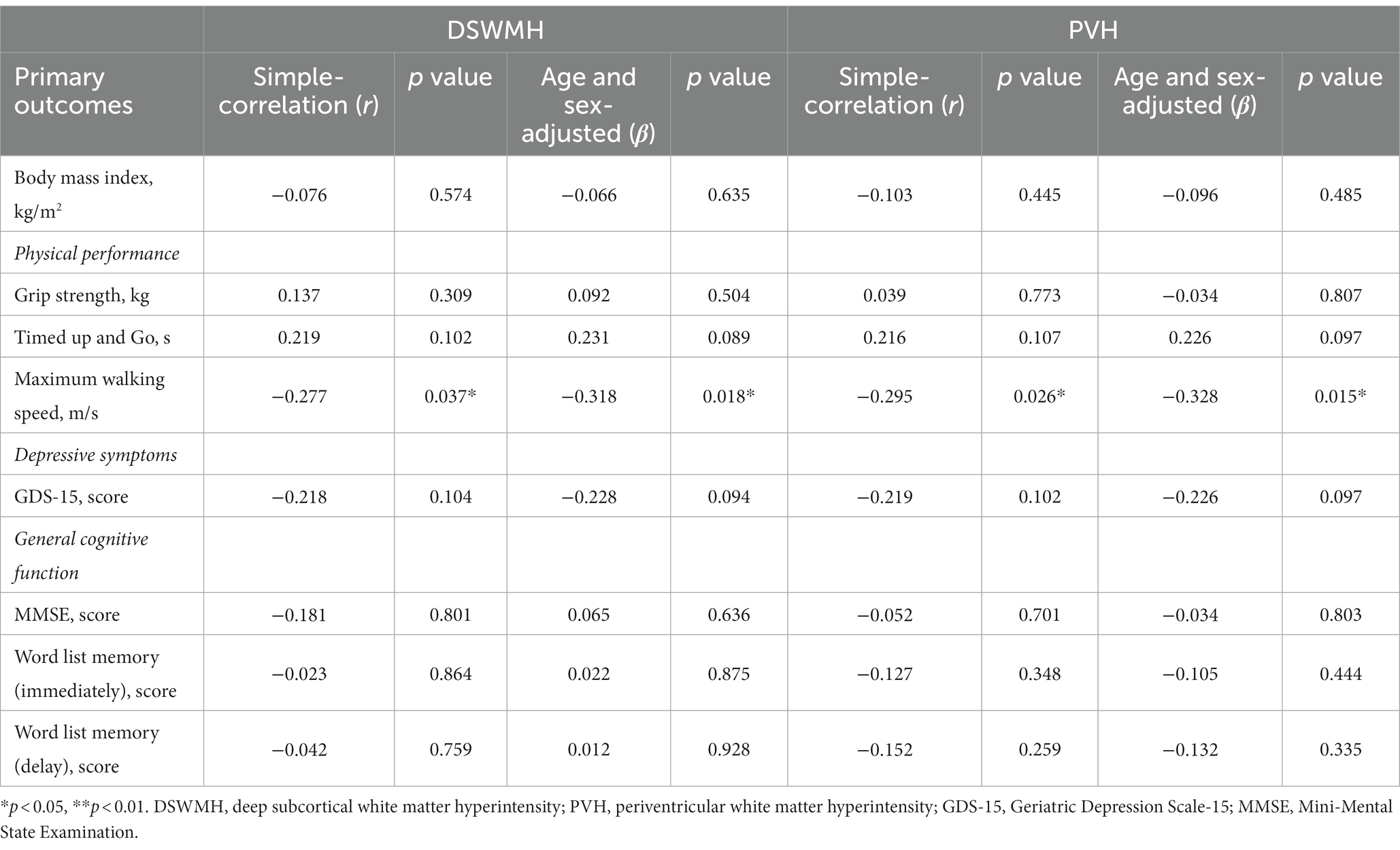
Table 2. Relationships between the percent of white matter hyperintensity volumes and participant characteristics.
3.2 Serum IL-6 and moderate physical activity are associated with percent of WMH volumes
Table 3 shows the relationships between the 2 % of WMH volume (DSWMH and PVH) and blood biomarkers. Table 4 shows the relationships between the 2% of WMH volumes (DSWMH and PVH) and physical activity levels, which were evaluated using the Pearson’s correlation coefficient (r). Age and sex were adjusted. Neither cortisol nor HbA1c were significantly correlated with DSWMH (β = 0.255, p = 0.06 and β = 0.168, p = 0.22, respectively) or PVH volume (β = 0.255, p = 0.06 and β = 0.181, p = 0.187, respectively). Additionally, HDL cholesterol, total cholesterol, IGF-1, and BDNF levels were not significantly correlated with WMH volume. In contrast, serum IL-6 levels significantly correlated with both percent of DSWMH (β = 0.305, p = 0.023) and PVH volumes (β = 0.353, p = 0.008), where higher serum IL-6 levels were associated with higher percent of WMH volumes. On average, participants completed 5732.1 ± 2829.8 steps/day (mean ± SD). There was no significant correlation between percent of DSWMH (β = −0.264, p = 0.136) or PVH (β = − 0.183, p = 0.181) volumes and the number of steps per day. In addition, time required to perform a low level of activity was not significantly correlated with percent of DSWMH (β = −0.033, p = 0.811) or PVH (β = −0.046, p = 0.736) volumes. However, there was a significant correlation between time required to perform an activity of moderate level and DSWMH (β = −0.389, p = 0.004) and PVH volumes (β = −0.362, p = 0.007), where higher time required to perform an activity of moderate level was associated with lower percent of WMH volumes.
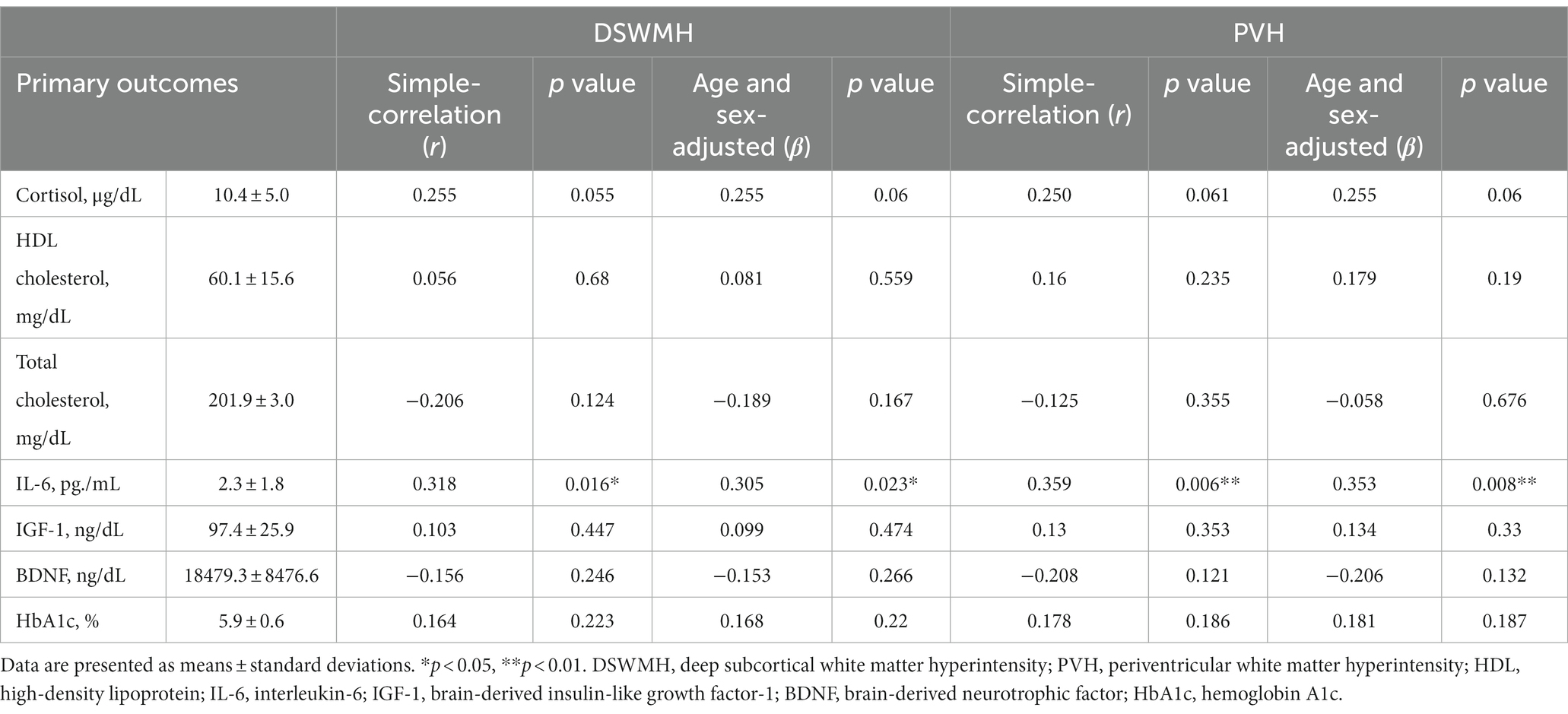
Table 3. Relationships between the percent of white matter hyperintensity volumes and blood biomarkers.
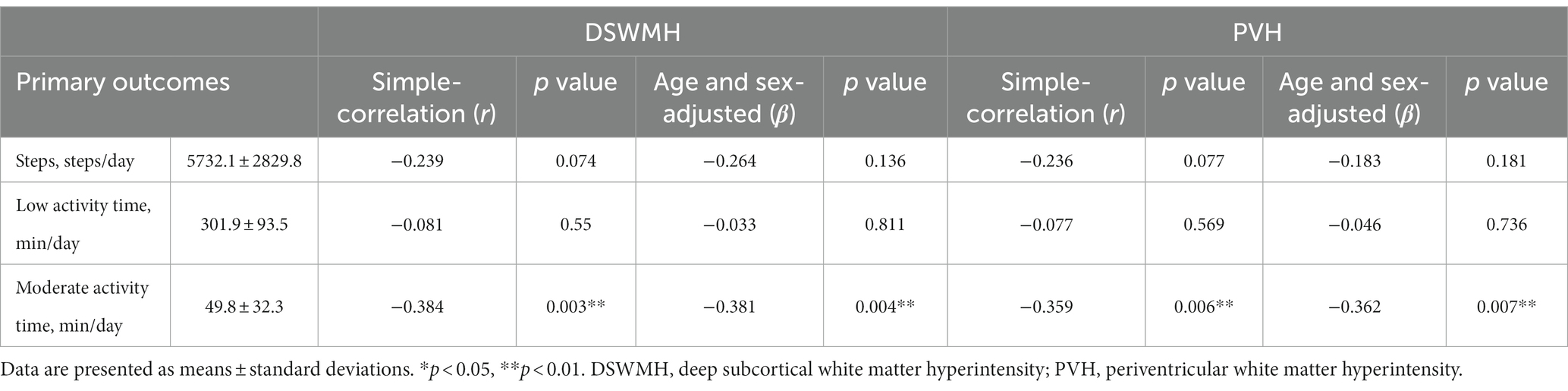
Table 4. Relationships between the percent of white matter hyperintensity volumes and physical activity.
3.3 Association between serum IL-6 level and time required to perform an activity of moderate level and maximum walking speed
We examined whether serum IL-6 levels were associated with duration of moderate activity and maximal walking speed. Moderate activity time showed a significant inverse correlation with serum IL-6 levels (r = −0.287, p = 0.03). However, when adjusted for age and sex, the correlation was not significant (β = −0.232, p = 0.088). Maximal walking speed was not correlated with serum IL-6 level (r = −0.006, p = 0.964; β = −0.018, p = 0.899).
4 Discussion
The purpose of this study was to examine the association between WMH volume and physical activity in older adults with DS and MMI and to identify factors associated with WMH volume. According to our results, longer periods of moderately intense physical activity and faster maximal walking speeds were associated with decreased percent of WMH volume. In addition, a higher percent of WMH volume were associated with higher blood IL-6 levels.
Longer daily physical activity above a certain intensity may affect the WMH volume. Previous research has documented that lifestyle factors, such as dietary habits and physical exercise, are associated with both cortical thickness (Reiter et al., 2015; Lee et al., 2016) and WMH volume (Mosconi et al., 2014). We focused on the amount of physical activity and the number of steps taken per day and investigated how these factors relate to WMH volumes. In a 5-year follow-up study of 680 men, there was a negative correlation between the number of steps taken at baseline and WMH volume after 5 years, with a minimum of 8,150 steps per day required to reduce WMH volume (Moniruzzaman et al., 2020). The number of daily steps taken by the participants in the present study was 5732.0 ± 2829.8 (mean ± SD) and was not correlated with percent of WMH volumes. We speculate that these discrepant findings can be attributed to the relatively small number of steps taken in our investigation. A previous study on the relationship between physical activity and WMH volume in 88 healthy individuals aged 60–78 years reported that moderate or high levels of physical activity were more strongly associated with lower WMH volume than light physical activity (Burzynska et al., 2014). Several studies have found a link between increased physical activity intensity and decreased WMH volume (Gow et al., 2012; Sen et al., 2012; Tseng et al., 2013). In addition, a cross-sectional study of adults aged ≥65 years reported that a lower maximum walking speed at baseline is associated with WMH volume (Soumaré et al., 2009). Similar results were obtained in our study, suggesting that maximal walking speed is an important factor in the assessment of motor function.
The expression of BDNF and IGF-1 in blood is enhanced by physical activity (Cassilhas et al., 2010; Jeon and Ha, 2017). Based on the results showing that moderate physical activity is associated with percent of WMH volumes, we hypothesized that BDNF and IGF-1 may also be associated with WMH volume. These two neurotrophic factors have been documented to play important roles in neurogenesis, angiogenesis, and survival (McAllister et al., 1995; Horch, 2004; Åberg et al., 2006; Hung et al., 2007). According to Pikula et al. (2013), higher levels of serum BDNF are associated with lower WMH volumes. Furthermore, reduced WMHs in the prefrontal cortex are associated with higher plasma BDNF expression levels (Dalby et al., 2013). Meta-analyses have reported that the higher the IGF-1 concentration, the lower is the WMH volume (Wittfeld et al., 2022). Moreover, an earlier study that followed 757 older adults for 10 years reported that lower serum IGF-1 levels were associated with a higher incidence of stroke (Saber et al., 2017). In this study, we did not observe any correlation between blood BDNF and IGF-1 concentrations or WMH volume. Shimada et al. (2014) reported that older adults with DS have lower serum BDNF levels than cognitively normal older adults (Shimada et al., 2014). Moreover, reduced serum IGF-1 levels are associated with mild dementia (Doi et al., 2015). Our patient had symptoms of DS and MMI, which may have adversely affected the expression of neurotrophic factors (BDNF and IGF) and reduced their association with WMH volume. New insights can be gained by investigating the relationship between neurotrophic factors other than BDNF and IGF and WMH volume, which were examined in the present study.
Our study revealed that IL-6 is an important blood biomarker that may be associated with WMH volume in older adults with DS and MMI. WMHs are often observed in older adults and are associated with a higher risk of overall mortality, stroke, and cognitive and physical deterioration (Debette and Markus, 2010; Silbert et al., 2012). Inflammatory mediators are strongly associated with WMHs (Torres et al., 2015). An earlier cross-sectional study that included healthy older people aged 65–80 years found a correlation between higher concentrations of IL-6 and C-reactive protein and higher WMH volume (Satizabal et al., 2012). Conversely, among older persons without dementia, higher IL-6 levels are linked to reduced brain volume, but not WMH volume (Gu et al., 2017). Given that our participants were older adults with DS and MMI who lived in their communities, they may have had higher serum IL-6 levels than cognitively normal older adults. Indeed, previous studies have reported that patients with DS have higher expression of inflammatory cytokines and IL-6 than individuals without such symptoms (Ryan et al., 2017). Thus, IL-6 levels may not be significantly correlated with WMH volume in cognitively normal older adults. Nevertheless, multiple earlier studies have found a link between a high inflammatory state and high WMH volume (Schuitemaker et al., 2009; Lampe et al., 2019).
A longer time spent performing physical activity of moderate levels was not associated with lower serum IL-6 levels. Previous studies in primary care patients reported that moderate physical activity decreases the expression of serum IL-6 (Rethorst et al., 2011). In addition, Paolucci et al. reported that exercise of more than moderate intensity is required to exert anti-inflammatory effects (Paolucci et al., 2018). Thus, we hypothesized that the time spent performing moderate-intensity physical activity affects IL-6 expression and reduces WMH volume. However, our results did not support this hypothesis. The fact that our study participants had symptoms of depression and were less physically active than healthy adults of similar age may have contributed to this discrepancy. Future comparisons of activity levels among healthy older adults may reveal a more detailed relationship between these two factors, and how they relate to WMH volume.
In this study, we divided WMH into DSWMH and PVH and examined the associated factors at these different sites. An association between increased PVH volume and greater cognitive impairment has been observed (Kim et al., 2008), whereas in our study, percent of PVH and DSWMH volumes were not associated with memory impairments. Furthermore, DSWMHs have been reported to be involved in mood disorders (De Groot et al., 2000), which was not observed in the present study. These discrepant findings may be attributed to differences in the assessment methods and symptom severity. Although the participants in our study were older than 65 years and had DS and MMI, their mood and memory impairments were likely not associated with the percent of WMH volumes because their impairments were relatively mild. Furthermore, higher serum IL-6 levels were associated with higher percent of DSWMH and PVH volumes, whereas no other biomarkers were associated with the percent of DSWMH or PVH volumes. The longer the time spent on moderate or high levels of physical activity, the smaller the percent of DSWMH and PVH volumes. Thus, prolonged physical activity may reduce WMH volumes in all brain regions.
A limitation of this study is its cross-sectional design, as causal effects could not be explored. In addition, the sample size was relatively small (n = 57). WMH volumes could not be measured using MRI in several participants, which reduced the number of samples available for analysis. In addition, our results were based only on community-dwelling older adults with DS or MMI. Healthy older adults may have different factors that affect WMH volume. We were unable to determine a detailed relationship between physical activity and WMH volume, which may have been made possible by comparing the activity levels obtained in our study with those of healthy older adults. Furthermore, smoking history, a factor that influences the WMH volume, was not included in the survey. However, none of the participants had a history of chronic obstructive pulmonary disease. Thus, the results were unlikely to have been affected by this omission. Further studies with larger sample sizes are required to provide more detailed insight.
In summary, the present study aimed to examine the association between WMH volume and physical activity in high-risk community-dwelling older adults aged ≥65 years with DS and MMI and to identify the factors associated with WMH volume. We analyzed the MRI images, classified the WMHs into two categories (DSWMH and PVH), and calculated their volumes. Elevated levels of inflammatory cytokines in blood are associated with higher percent of DSWMH and PVH volumes. In contrast, faster maximum walking speed and more time spent performing a moderate level of physical activity correlated with smaller percent of DSWMH and PVH volumes. Given that high WMH volumes are associated with adverse effects on the brain, early detection and treatment are vital. Our results provide valuable information for maintaining brain health in older adults with DS and MMI, and may contribute to the development of basic medicine for brain health.
Data availability statement
The raw data supporting the conclusions of this article will be made available by the authors, without undue reservation.
Ethics statement
The studies involving humans were approved by the clinical investigation was pre-registered with the clinical trial registration of the University Hospital Medical Information Network (000018547). The studies were conducted in accordance with the local legislation and institutional requirements. The participants provided their written informed consent to participate in this study.
Author contributions
SO: Formal analysis, Software, Writing – original draft. KK: Methodology, Resources, Writing – review & editing. YT: Formal analysis, Writing – review & editing. ST: Formal analysis, Writing – review & editing. AT: Methodology, Writing – review & editing. HS: Project administration, Resources, Writing – review & editing. IM: Methodology, Writing – review & editing. HM: Funding acquisition, Methodology, Project administration, Resources, Writing – original draft.
Funding
The author(s) declare financial support was received for the research, authorship, and/or publication of this article. This study was supported by Research Funding for Longevity Sciences (22-16) from the National Center for Geriatrics and Gerontology (NCGG) in Japan and JSPS KAKENHI (Grant-in-Aid for Young Scientists [A]; grant number 26702033 to HM, grant number JP20H04039 to HS, and grant number JP22K17632 to SO).
Acknowledgments
We thank the study participants, and the research and healthcare staff involved in this study for their assistance with the assessments. We would like to thank Editage (www.editage.jp) for the English language editing.
Conflict of interest
The authors declare that the research was conducted in the absence of any commercial or financial relationships that could be construed as a potential conflict of interest.
Publisher’s note
All claims expressed in this article are solely those of the authors and do not necessarily represent those of their affiliated organizations, or those of the publisher, the editors and the reviewers. Any product that may be evaluated in this article, or claim that may be made by its manufacturer, is not guaranteed or endorsed by the publisher.
Footnotes
References
Åberg, N. D., Brywe, K. G., and Isgaard, J. (2006). Aspects of growth hormone and insulin-like growth factor-I related to neuroprotection, regeneration, and functional plasticity in the adult brain. ScientificWorldJournal 6, 53–80. doi: 10.1100/tsw.2006.22
Bangen, K. J., Calcetas, A. T., Thomas, K. R., Wierenga, C., Smith, C. N., Bordyug, M., et al. (2023). Greater accelerometer-measured physical activity is associated with better cognition and cerebrovascular health in older adults. J. Int. Neuropsychol. Soc. 29, 859–869. doi: 10.1017/S1355617723000140
Barnes, D. E., and Yaffe, K. (2011). The projected effect of risk factor reduction on Alzheimer’s disease prevalence. Lancet Neurol. 10, 819–828. doi: 10.1016/S1474-4422(11)70072-2
Black, S., Gao, F., and Bilbao, J. (2009). Understanding white matter disease: imaging-pathological correlations in vascular cognitive impairment. Stroke 40, S48–S52. doi: 10.1161/STROKEAHA.108.537704
Bolandzadeh, N., Davis, J. C., Tam, R., Handy, T. C., and Liu-Ambrose, T. (2012). The association between cognitive function and white matter lesion location in older adults: a systematic review. BMC Neurol. 12:126. doi: 10.1186/1471-2377-12-126
Burzynska, A. Z., Chaddock-Heyman, L., Voss, M. W., Wong, C. N., Gothe, N. P., Olson, E. A., et al. (2014). Physical activity and cardiorespiratory fitness are beneficial for white matter in low-fit older adults. PLoS One 9:e107413. doi: 10.1371/journal.pone.0107413
Cassilhas, R. C., Tufik, S., Antunes, H. K. M., and de Mello, M. T. (2010). Mood, anxiety, and serum IGF-1 in elderly men given 24 weeks of high resistance exercise. Percept. Mot. Skills 110, 265–276. doi: 10.2466/PMS.110.1.265-276
Cox, S. R., Bastin, M. E., Ferguson, K. J., Maniega, S. M., MacPherson, S. E., Deary, I. J., et al. (2015). Brain white matter integrity and cortisol in older men: the Lothian birth cohort 1936. Neurobiol. Aging 36, 257–264. doi: 10.1016/J.NEUROBIOLAGING.2014.06.022
Dalby, R. B., Elfving, B., Poulsen, P. H. P., Foldager, L., Frandsen, J., Videbech, P., et al. (2013). Plasma brain-derived neurotrophic factor and prefrontal white matter integrity in late-onset depression and normal aging. Acta Psychiatr. Scand. 128, 387–396. doi: 10.1111/acps.12085
De Groot, J. C., De Leeuw, F. E., Oudkerk, M., Hofman, A., Jolles, J., and Breteler, M. M. B. (2000). Cerebral white matter lesions and depressive symptoms in elderly adults. Arch. Gen. Psychiatry 57, 1071–1076. doi: 10.1001/archpsyc.57.11.1071
De Havenon, A., Majersik, J. J., Tirschwell, D. L., McNally, J. S., Stoddard, G., and Rost, N. S. (2019). Blood pressure, glycemic control, and white matter hyperintensity progression in type 2 diabetics. Neurology 92, e1168–e1175. doi: 10.1212/WNL.0000000000007093
Debette, S., Beiser, A., Decarli, C., Au, R., Himali, J. J., Kelly-Hayes, M., et al. (2010). Association of MRI markers of vascular brain injury with incident stroke, mild cognitive impairment, dementia, and mortality: the Framingham offspring study. Stroke 41, 600–606. doi: 10.1161/STROKEAHA.109.570044
Debette, S., and Markus, H. S. (2010). The clinical importance of white matter hyperintensities on brain magnetic resonance imaging: systematic review and meta-analysis. BMJ 341:c3666. doi: 10.1136/bmj.c3666
Doi, T., Shimada, H., Makizako, H., Tsutsumimoto, K., Hotta, R., Nakakubo, S., et al. (2015). Association of insulin-like growth factor-1 with mild cognitive impairment and slow gait speed. Neurobiol. Aging 36, 942–947. doi: 10.1016/j.neurobiolaging.2014.10.035
Erten-Lyons, D., Woltjer, R., Kaye, J., Mattek, N., Dodge, H. H., Green, S., et al. (2013). Neuropathologic basis of white matter hyperintensity accumulation with advanced age. Neurology 81, 977–983. doi: 10.1212/WNL.0b013e3182a43e45
Fernando, M. S., Simpson, J. E., Matthews, F., Brayne, C., Lewis, C. E., Barber, R., et al. (2006). White matter lesions in an unselected cohort of the elderly: molecular pathology suggests origin from chronic hypoperfusion injury. Stroke 37, 1391–1398. doi: 10.1161/01.STR.0000221308.94473.14
Garfield, V., Farmaki, A. E., Eastwood, S. V., Mathur, R., Rentsch, C. T., Bhaskaran, K., et al. (2021). HbA1c and brain health across the entire glycaemic spectrum. Diabetes Obes. Metab. 23, 1140–1149. doi: 10.1111/dom.14321
Gaubert, M., Lange, C., Garnier-Crussard, A., Köbe, T., Bougacha, S., Gonneaud, J., et al. (2021). Topographic patterns of white matter hyperintensities are associated with multimodal neuroimaging biomarkers of Alzheimer’s disease. Alzheimers Res. Ther. 13:29. doi: 10.1186/s13195-020-00759-3
Gouw, A. A., Seewann, A., Van Der Flier, W. M., Barkhof, F., Rozemuller, A. M., Scheltens, P., et al. (2011). Heterogeneity of small vessel disease: a systematic review of MRI and histopathology correlations. J. Neurol. Neurosurg. Psychiatry 82, 126–135. doi: 10.1136/jnnp.2009.204685
Gow, A. J., Bastin, M. E., Muñoz Maniega, S. M., Valdés Hernández, M. C., Morris, Z., Murray, C., et al. (2012). Neuroprotective lifestyles and the aging brain: activity, atrophy, and white matter integrity. Neurology 79, 1802–1808. doi: 10.1212/WNL.0b013e3182703fd2
Gu, Y., Vorburger, R., Scarmeas, N., Luchsinger, J. A., Manly, J. J., Schupf, N., et al. (2017). Circulating inflammatory biomarkers in relation to brain structural measurements in a non-demented elderly population. Brain Behav. Immun. 65, 150–160. doi: 10.1016/j.bbi.2017.04.022
Han, Q. Y., Zhang, H., Zhang, X., He, D. S., Wang, S. W., Cao, X., et al. (2019). Dl-3-n-butylphthalide preserves white matter integrity and alleviates cognitive impairment in mice with chronic cerebral hypoperfusion. CNS Neurosci. Ther. 25, 1042–1053. doi: 10.1111/cns.13189
Horch, H. W. (2004). Local effects of BDNF on dendritic growth. Rev. Neurosci. 15, 117–129. doi: 10.1515/revneuro.2004.15.2.117
Hung, K. S., Tsai, S. H., Lee, T. C., Lin, J. W., Chang, C. K., and Chiu, W. T. (2007). Gene transfer of insulin-like growth factor-I providing neuroprotection after spinal cord injury in rats. J. Neurosurg. Spine 6, 35–46. doi: 10.3171/spi.2007.6.1.35
Jeon, Y. K., and Ha, C. H. (2017). The effect of exercise intensity on brain derived neurotrophic factor and memory in adolescents. Environ. Health Prev. Med. 22:27. doi: 10.1186/S12199-017-0643-6
Johnson, N. F., Gold, B. T., Ross, D., Bailey, A. L., Clasey, J. L., Gupta, V., et al. (2019). Non-fasting high-density lipoprotein is associated with white matter microstructure in healthy older adults. Front. Aging Neurosci. 11:100. doi: 10.3389/fnagi.2019.00100
Kim, K. W., MacFall, J. R., and Payne, M. E. (2008). Classification of white matter lesions on magnetic resonance imaging in the elderly persons. Biol. Psychiatry 64, 273–280. doi: 10.1016/j.biopsych.2008.03.024
Kuller, L. H., Longstreth, W. T., Arnold, A. M., Bernick, C., Bryan, R. N., Beauchamp, N. J., et al. (2004). White matter hyperintensity on cranial magnetic resonance imaging: a predictor of stroke. Stroke 35, 1821–1825. doi: 10.1161/01.STR.0000132193.35955.69
Lampe, L., Zhang, R., Beyer, F., Huhn, S., Kharabian Masouleh, S., Preusser, S., et al. (2019). Visceral obesity relates to deep white matter hyperintensities via inflammation. Ann. Neurol. 85, 194–203. doi: 10.1002/ana.25396
Lee, J. S., Shin, H. Y., Kim, H. J., Jang, Y. K., Jung, N. Y., Lee, J., et al. (2016). Combined effects of physical exercise and education on age-related cortical thinning in cognitively normal individuals. Sci. Rep. 6:24284. doi: 10.1038/srep24284
Makizako, H., Liu-Ambrose, T., Shimada, H., Doi, T., Park, H., Tsutsumimoto, K., et al. (2015a). Moderate-intensity physical activity, hippocampal volume, and memory in older adults with mild cognitive impairment. J. Gerontol. A Biol. Sci. Med. Sci. 70, 480–486. doi: 10.1093/gerona/glu136
Makizako, H., Shimada, H., Park, H., Doi, T., Yoshida, D., Uemura, K., et al. (2013). Evaluation of multidimensional neurocognitive function using a tablet personal computer: test-retest reliability and validity in community-dwelling older adults. Geriatr Gerontol Int 13, 860–866. doi: 10.1111/ggi.12014
Makizako, H., Tsutsumimoto, K., Doi, T., Hotta, R., Nakakubo, S., Liu-Ambrose, T., et al. (2015b). Effects of exercise and horticultural intervention on the brain and mental health in older adults with depressive symptoms and memory problems: study protocol for a randomized controlled trial [UMIN000018547]. Trials 16:499. doi: 10.1186/s13063-015-1032-3
Makizako, H., Tsutsumimoto, K., Doi, T., Makino, K., Nakakubo, S., Liu-Ambrose, T., et al. (2019). Exercise and horticultural programs for older adults with depressive symptoms and memory problems: a randomized controlled trial. J. Clin. Med. 9:99. doi: 10.3390/jcm9010099
McAllister, A. K., Lo, D. C., and Katz, L. C. (1995). Neurotrophins regulate dendritic growth in developing visual cortex. Neuron 15, 791–803. doi: 10.1016/0896-6273(95)90171-x
Moniruzzaman, M., Kadota, A., Segawa, H., Kondo, K., Torii, S., Miyagawa, N., et al. (2020). Relationship between step counts and cerebral small vessel disease in Japanese men. Stroke 51, 3584–3591. doi: 10.1161/STROKEAHA.120.030141
Moon, S. Y., de Souto Barreto, P., Chupin, M., Mangin, J. F., Bouyahia, A., Fillon, L., et al. (2017). Associations between white matter hyperintensities and cognitive decline over three years in non-dementia older adults with memory complaints. J. Neurol. Sci. 379, 266–270. doi: 10.1016/j.jns.2017.06.031
Mosconi, L., Murray, J., Tsui, W. H., Li, Y., Davies, M., Williams, S., et al. (2014). Mediterranean diet and magnetic resonance imaging-assessed brain atrophy in cognitively normal individuals at risk for Alzheimer’s disease. J. Prev Alzheimers Dis. 1, 23–32. doi: 10.14283/jpad.2014.17
Ohkawara, K., Oshima, Y., Hikihara, Y., Ishikawa-Takata, K., Tabata, I., and Tanaka, S. (2011). Real-time estimation of daily physical activity intensity by a triaxial accelerometer and a gravity-removal classification algorithm. Br. J. Nutr. 105, 1681–1691. doi: 10.1017/S0007114510005441
Oshima, Y., Kawaguchi, K., Tanaka, S., Ohkawara, K., Hikihara, Y., Ishikawa-Takata, K., et al. (2010). Classifying household and locomotive activities using a triaxial accelerometer. Gait Posture 31, 370–374. doi: 10.1016/j.gaitpost.2010.01.005
Otsuka, S., Sakakima, H., Sumizono, M., Takada, S., Terashi, T., and Yoshida, Y. (2016). The neuroprotective effects of preconditioning exercise on brain damage and neurotrophic factors after focal brain ischemia in rats. Behav. Brain Res. 303, 9–18. doi: 10.1016/j.bbr.2016.01.049
Otsuka, S., Sakakima, H., Terashi, T., Takada, S., Nakanishi, K., and Kikuchi, K. (2019). Preconditioning exercise reduces brain damage and neuronal apoptosis through enhanced endogenous 14–3-3r after focal brain ischemia in rats. Brain Struct. Funct. 224, 727–738. doi: 10.1007/s00429-018-1800-4
Paolucci, E. M., Loukov, D., Bowdish, D. M. E., and Heisz, J. J. (2018). Exercise reduces depression and inflammation but intensity matters. Biol. Psychol. 133, 79–84. doi: 10.1016/j.biopsycho.2018.01.015
Pate, R. R., Pratt, M., Blair, S. N., Haskell, W. L., Macera, C. A., Bouchard, C., et al. (1995). Physical activity and public health. A recommendation from the Centers for Disease Control and Prevention and the American College of Sports Medicine. JAMA 273, 402–407. doi: 10.1001/jama.273.5.402
Paterniti, S., Verdier-Taillefer, M. H., Dufouil, C., and Alpérovitch, A. (2002). Depressive symptoms and cognitive decline in elderly people. Longitudinal study. Br. J. Psychiatry. 181, 406–410. doi: 10.1192/bjp.181.5.406
Pikula, A., Beiser, A. S., Chen, T. C., Preis, S. R., Vorgias, D., DeCarli, C., et al. (2013). Serum brain-derived neurotrophic factor and vascular endothelial growth factor levels are associated with risk of stroke and vascular brain injury: Framingham study. Stroke 44, 2768–2775. doi: 10.1161/STROKEAHA.113.001447
Pinter, D., Ritchie, S. J., Gattringer, T., Bastin, M. E., Hernández, M. C. V., Corley, J., et al. (2018). Predictors of gait speed and its change over three years in community-dwelling older people. Aging 10, 144–153. doi: 10.18632/AGING.101365
Podewils, L. J., Guallar, E., Beauchamp, N., Lyketsos, C. G., Kuller, L. H., and Scheltens, P. (2007). Physical activity and white matter lesion progression: assessment using MRI. Neurology 68, 1223–1226. doi: 10.1212/01.wnl.0000259063.50219.3e
Prince, S. A., Adamo, K. B., Hamel, M. E., Hardt, J., Connor Gorber, S., and Tremblay, M. (2008). A comparison of direct versus self-report measures for assessing physical activity in adults: a systematic review. Int. J. Behav. Nutr. Phys. Act. 5:56. doi: 10.1186/1479-5868-5-56
Promjunyakul, N., Lahna, D., Kaye, J. A., Dodge, H. H., Erten-Lyons, D., Rooney, W. D., et al. (2015). Characterizing the white matter hyperintensity penumbra with cerebral blood flow measures. Neuro Image Clin. 8, 224–229. doi: 10.1016/j.nicl.2015.04.012
Rantanen, T. (2003). Muscle strength, disability and mortality. Scand. J. Med. Sci. Sports 13, 3–8. doi: 10.1034/j.1600-0838.2003.00298.x
Reiter, K., Nielson, K. A., Smith, T. J., Weiss, L. R., Alfini, A. J., and Smith, J. C. (2015). Improved cardiorespiratory fitness is associated with increased cortical thickness in mild cognitive impairment. J. Int. Neuropsychol. Soc. 21, 757–767. doi: 10.1017/S135561771500079X
Rethorst, C. D., Moynihan, J., Lyness, J. M., Heffner, K. L., and Chapman, B. P. (2011). Moderating effects of moderate-intensity physical activity in the relationship between depressive symptoms and interleukin-6 in primary care patients. Psychosom. Med. 73, 265–269. doi: 10.1097/PSY.0b013e3182108412
Ryan, J., Pilkington, L., Neuhaus, K., Ritchie, K., Ancelin, M. L., and Saffery, R. (2017). Investigating the epigenetic profile of the inflammatory gene IL-6 in late-life depression. BMC Psychiatry 17:354. doi: 10.1186/s12888-017-1515-8
Saber, H., Himali, J. J., Beiser, A. S., Shoamanesh, A., Pikula, A., Roubenoff, R., et al. (2017). Serum insulin-like growth factor 1 and the risk of ischemic stroke: the Framingham Study. Stroke 48, 1760–1765. doi: 10.1161/STROKEAHA.116.016563
Samaille, T., Fillon, L., Cuingnet, R., Jouvent, E., Chabriat, H., Dormont, D., et al. (2012). Contrast-based fully automatic segmentation of white matter hyperintensities: method and validation. PLoS One 7:e48953. doi: 10.1371/journal.pone.0048953
Satizabal, C. L., Zhu, Y. C., Mazoyer, B., Dufouil, C., and Tzourio, C. (2012). Circulating IL-6 and CRP are associated with MRI findings in the elderly: the 3C-Dijon study. Neurology 78, 720–727. doi: 10.1212/WNL.0b013e318248e50f
Schmidt, P., Gaser, C., Arsic, M., Buck, D., Förschler, A., Berthele, A., et al. (2012). An automated tool for detection of FLAIR-hyperintense white-matter lesions in multiple sclerosis. NeuroImage 59, 3774–3783. doi: 10.1016/j.neuroimage.2011.11.032
Schuitemaker, A., Dik, M. G., Veerhuis, R., Scheltens, P., Schoonenboom, N. S., Hack, C. E., et al. (2009). Inflammatory markers in AD and MCI patients with different biomarker profiles. Neurobiol. Aging 30, 1885–1889. doi: 10.1016/j.neurobiolaging.2008.01.014
Sen, A., Gider, P., Cavalieri, M., Freudenberger, P., Farzi, A., Schallert, M., et al. (2012). Association of cardiorespiratory fitness and morphological brain changes in the elderly: results of the Austrian stroke prevention study. Neurodegener Dis 10, 135–137. doi: 10.1159/000334760
Shiga, K., Hara, M., Nagasaki, T., Sato, T., Takahashi, H., Sato, M., et al. (2016). Preoperative serum interleukin-6 is a potential prognostic factor for colorectal cancer, including stage II patients. Gastroenterol. Res. Pract. 2016:9701574. doi: 10.1155/2016/9701574
Shimada, H., Makizako, H., Doi, T., Yoshida, D., Tsutsumimoto, K., Anan, Y., et al. (2013). Combined prevalence of frailty and mild cognitive impairment in a population of elderly Japanese people. J. Am. Med. Dir. Assoc. 14, 518–524. doi: 10.1016/j.jamda.2013.03.010
Shimada, H., Park, H., Makizako, H., Doi, T., Lee, S., and Suzuki, T. (2014). Depressive symptoms and cognitive performance in older adults. J. Psychiatr. Res. 57, 149–156. doi: 10.1016/j.jpsychires.2014.06.004
Silbert, L. C., Dodge, H. H., Perkins, L. G., Sherbakov, L., Lahna, D., Erten-Lyons, D., et al. (2012). Trajectory of white matter hyperintensity burden preceding mild cognitive impairment. Neurology 79, 741–747. doi: 10.1212/WNL.0b013e3182661f2b
Silbert, L. C., Nelson, C., Howieson, D. B., Moore, M. M., and Kaye, J. A. (2008). Impact of white matter hyperintensity volume progression on rate of cognitive and motor decline. Neurology 71, 108–113. doi: 10.1212/01.wnl.0000316799.86917.37
Soumaré, A., Elbaz, A., Zhu, Y., Maillard, P., Crivello, F., Tavernier, B., et al. (2009). White matter lesions volume and motor performances in the elderly. Ann. Neurol. 65, 706–715. doi: 10.1002/ana.21674
Staals, J., Makin, S. D. J., Doubal, F. N., Dennis, M. S., and Wardlaw, J. M. (2014). Stroke subtype, vascular risk factors, and total MRI brain small-vessel disease burden. Neurology 83, 1228–1234. doi: 10.1212/WNL.0000000000000837
Suzuki, T., Shimada, H., Makizako, H., Doi, T., Yoshida, D., Ito, K., et al. (2013). A randomized controlled trial of multicomponent exercise in older adults with mild cognitive impairment. PLoS One 8:e61483. doi: 10.1371/journal.pone.0061483
Syaifullah, A. H., Shiino, A., Fujiyoshi, A., Kadota, A., Kondo, K., Ito, T., et al. (2021). Alcohol drinking and brain morphometry in apparently healthy community-dwelling Japanese men. Alcohol 90, 57–65. doi: 10.1016/j.alcohol.2020.11.006
Syaifullah, A. H., Shiino, A., Kitahara, H., Ito, R., Ishida, M., and Tanigaki, K. (2020). Machine learning for diagnosis of AD and prediction of MCI progression from brain MRI using brain anatomical analysis using diffeomorphic deformation. Front. Neurol. 11:576029. doi: 10.3389/fneur.2020.576029
Torres, E. R., Strack, E. F., Fernandez, C. E., Tumey, T. A., and Hitchcock, M. E. (2015). Physical activity and white matter hyperintensities: a systematic review of quantitative studies. Prev. Med. Rep. 2, 319–325. doi: 10.1016/j.pmedr.2015.04.013
Tsai, C. L., Pai, M. C., Ukropec, J., and Ukropcová, B. (2019). Distinctive effects of aerobic and resistance exercise modes on neurocognitive and biochemical changes in individuals with mild cognitive impairment. Curr. Alzheimer Res. 16, 316–332. doi: 10.2174/1567205016666190228125429
Tseng, B. Y., Gundapuneedi, T., Khan, M. A., Diaz-Arrastia, R., Levine, B. D., Lu, H., et al. (2013). White matter integrity in physically fit older adults. NeuroImage 82, 510–516. doi: 10.1016/j.neuroimage.2013.06.011
van Marwijk, H. W., Wallace, P., de Bock, G. H., Hermans, J., Kaptein, A. A., and Mulder, J. D. (1995). Evaluation of the feasibility, reliability and diagnostic value of shortened versions of the geriatric depression scale. Br. J. Gen. Pract. 45, 195–199.
Vellas, B., Carrie, I., Gillette-Guyonnet, S., Touchon, J., Dantoine, T., Dartigues, J. F., et al. (2014). MAPT study: a multidomain approach for preventing Alzheimer’s disease: design and baseline data. J. Prev Alzheimers Dis. 1, 13–22. doi: 10.14283/jpad.2014.34
Wen, W., and Sachdev, P. S. (2004). Extent and distribution of white matter hyperintensities in stroke patients: the Sydney stroke study. Stroke 35, 2813–2819. doi: 10.1161/01.STR.0000147034.25760.3d
Williams, V. J., Leritz, E. C., Shepel, J., Mcglinchey, R. E., Milberg, W. P., Rudolph, J. L., et al. (2013). Interindividual variation in serum cholesterol is associated with regional white matter tissue integrity in older adults. Hum. Brain Mapp. 34, 1826–1841. doi: 10.1002/HBM.22030
Wittfeld, K., Raman, M. R., Conner, S. C., Aslam, A., Teumer, A., Nauck, M., et al. (2022). Insulin-like growth factor, inflammation, and MRI markers of Alzheimer’s disease in predominantly middle-aged adults. J. Alzheimers Dis. 88, 311–322. doi: 10.3233/JAD-220356
Keywords: aging, depressive symptoms, memory problems, physical activity, interleukin 6 (IL-6)
Citation: Otsuka S, Kikuchi K, Takeshita Y, Takada S, Tani A, Sakakima H, Maruyama I and Makizako H (2024) Relationship between physical activity and cerebral white matter hyperintensity volumes in older adults with depressive symptoms and mild memory impairment: a cross-sectional study. Front. Aging Neurosci. 16:1337397. doi: 10.3389/fnagi.2024.1337397
Edited by:
Philip P. Foster, Baylor College of Medicine, United StatesReviewed by:
Ryota Sakurai, Tokyo Metropolitan Institute of Gerontology, JapanValentin Ourry, McGill University, Canada
Copyright © 2024 Otsuka, Kikuchi, Takeshita, Takada, Tani, Sakakima, Maruyama and Makizako. This is an open-access article distributed under the terms of the Creative Commons Attribution License (CC BY). The use, distribution or reproduction in other forums is permitted, provided the original author(s) and the copyright owner(s) are credited and that the original publication in this journal is cited, in accordance with accepted academic practice. No use, distribution or reproduction is permitted which does not comply with these terms.
*Correspondence: Shotaro Otsuka, k3360022@kadai.jp; Hyuma Makizako, makizako@health.nop.kagoshima-u.ac.jp