- 1Division of Biomedical Sciences, Faculty of Medicine, Memorial University of Newfoundland, St. John’s, NL, Canada
- 2Department of Psychology, Faculty of Science, Memorial University of Newfoundland, St. John’s, NL, Canada
We first review our understanding of odor representations in rodent olfactory bulb (OB) and anterior piriform cortex (APC). We then consider learning-induced representation changes. Finally we describe the perspective on network representations gained from examining Arc-indexed odor networks of awake rats. Arc-indexed networks are sparse and distributed, consistent with current views. However Arc provides representations of repeated odors. Arc-indexed repeated odor representations are quite variable. Sparse representations are assumed to be compact and reliable memory codes. Arc suggests this is not necessarily the case. The variability seen is consistent with electrophysiology in awake animals and may reflect top-down cortical modulation of context. Arc-indexing shows that distinct odors share larger than predicted neuron pools. These may be low-threshold neuronal subsets. Learning’s effect on Arc-indexed representations is to increase the stable or overlapping component of rewarded odor representations. This component can decrease for similar odors when their discrimination is rewarded. The learning effects seen are supported by electrophysiology, but mechanisms remain to be elucidated.
Here we first characterize our understanding of odor representations in the olfactory bulb (OB) and anterior piriform cortex (APC). We next review learning-related modulation of those odor representations. Finally, we discuss data using Arc-indexed odor representations. These data modify our view of odor representations and their modulation by reward. How Arc expression is recruited by odor is also considered.
Odor Representations in the Olfactory Bulb and Anterior Piriform Cortex
It has long been recognized that odor representations require across-fiber coding (Chaput and Holley, 1985). This means that they are population or network representations from the beginning. Olfactory sensory neurons encode molecular features of which there is a large variety (Mombaerts et al., 1996; Mori et al., 2006; Saito et al., 2009). Natural odors activate multiple sensory neurons (Lin da et al., 2006; Mori et al., 2006).
Laurent (1997) highlighted the importance of distinguishing between maps (circuitry) and spatiotemporal codes for odors. He referred to the apparent chemotopic spatial organization of glomerular odor input which has been widely accepted (Rubin and Katz, 1999; Xu et al., 2000; Wachowiak and Cohen, 2001; Leon and Johnson, 2003; Soucy et al., 2009; see Figure 1A). But recent imaging evidence with single glomerular resolution (Ma et al., 2012) and theoretical analyses (Cleland, 2010) argue for a lack of chemotopic mapping at the glomerular level (see also Lin da et al., 2006). Thus odor representations are distributed representations even at the glomerular level as chemical characteristics do not predict odor maps. Nonetheless structurally-related odors activate similar distributed networks (Ma et al., 2012).
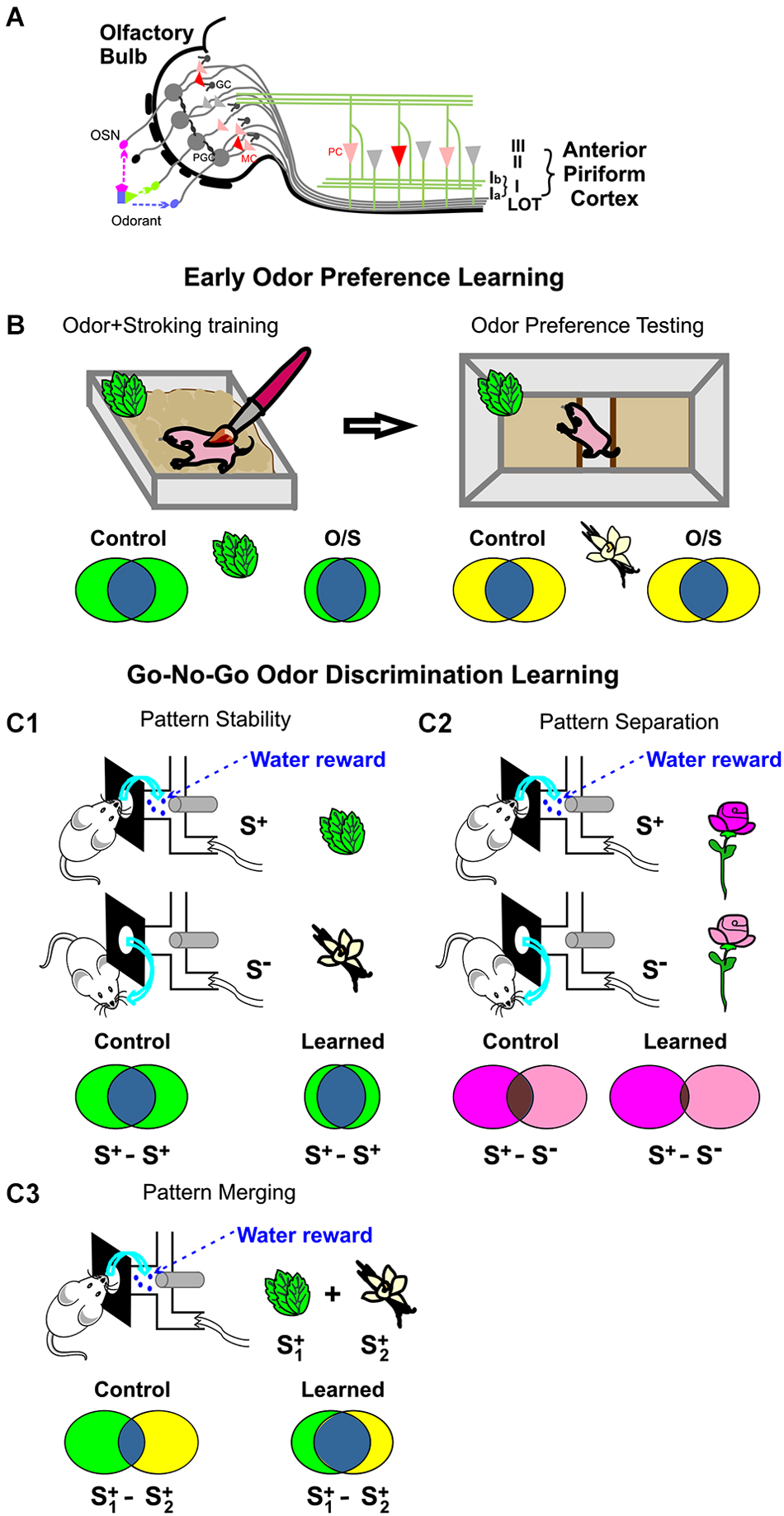
Figure 1. Odor network representations in early olfactory cortices. (A) Simplified olfactory bulb and anterior piriform cortex (APC) circuitry. Odorant molecular features are detected by olfactory sensory neurons (OSNs) in the nose and transmitted to the glomeruli of the olfactory bulb where OSNs synapse with output mitral cells (MCs). Mitral cells project to multiple pyramidal cells (PCs) in the APC via the lateral olfactory tract (LOT). Mitral cell output is regulated by interneurons at both the glomerular layer (periglomerular cells, PGCs) and the granule cell layer (granule cells, GCs). Piriform PCs receive convergent MC inputs and respond to individual odors. (B) Arc-indexed representation for early odor preference learning. A week-old rat pup undergoes odor+stroking (O/S) training with peppermint-scented bedding. This O/S training results in a preference for peppermint-scented bedding when the pup is tested 24 h later. Arc visualization to repeated peppermint following training reveals increased proportions of reliably activated neurons. Increased reliability is not seen when the pup is exposed to a control odor vanillin (Shakhawat et al., 2014a). (C1–C3) Arc-indexed representation of adult rat “go-no-go” odor discrimination learning in the APC. (C1) Odor reward learning increases the stability of the rewarded odor representation. S+ refers to positive rewarded odor stimulus (e.g., peppermint); S− refers to negative unrewarded odor stimulus (e.g., vanillin; Shakhawat et al., 2014b). When a trained rat is exposed to S+, the proportion of neurons recruiting Arc twice is increased. (C2) Discrimination of highly similar odors leads to pattern separation. There is less overlap between the two similar odor representations after discrimination learning than before. (C3) Reward training with an odor mixture increases representational overlap between the two component odors. and refer to the components of the rewarded odor mixture. A third odor is used as non-rewarded odor (not shown).
For mitral cells in the OB, an assumption that odor representations were dense and spatially specific has also evolved. A dramatic change in our understanding of mitral cell representations occurred when recordings were compared in anesthetized and awake mice (Rinberg et al., 2006). Under anesthesia, responses are driven by sensory input and occur against low spontaneous firing similar to antennal projection neurons in invertebrates (e.g., Krofczik et al., 2008). When awake, spontaneous activity is high (~20 Hz), and response to odor is weak and variable (Rinberg et al., 2006; Doucette and Restrepo, 2008; Zhan and Luo, 2010). Neuromodulatory input (Rinberg et al., 2006; Mandairon and Linster, 2009; Doucette et al., 2011), context (Kay and Laurent, 1999; Doucette and Restrepo, 2008; Mandairon et al., 2014), and other cortical top-down (Chapuis et al., 2013; Rothermel and Wachowiak, 2014) influences play a role in these awake representations. Odor decoding must depend on stable and/or synchronized elements within the population. Granule cells have been less studied, but evidence suggests they also have odor encoding features (Busto et al., 2009).
The distributed and sparse network representations seen in OB also occur in APC (Stettler and Axel, 2009; Isaacson, 2010; Davison and Ehlers, 2011; Wilson and Sullivan, 2011). Haberly proposes that APC is an analog of associative cortices more generally (Haberly, 2001). Mitral cell axons arrive in Layer Ia, making en passant contacts with pyramidal cell dendrites (Haberly, 2001; Isaacson, 2010; Wilson and Sullivan, 2011). Odor encoding is sustained by excitatory associational connections (Rennaker et al., 2007; Poo and Isaacson, 2011). Based on spines per dendrite in Layer Ia (Knafo et al., 2005), there is a relatively large “fan in” from mitral cells to single pyramidal cells. Such connectivity may implicate oscillations in odor decoding. In both OB and APC odor representation is best characterized as a dynamic spatiotemporal pattern (Laurent, 1996, 1997; Friedrich and Laurent, 2001; Rennaker et al., 2007; Restrepo et al., 2009).
Sparse representations as seen in OB and APC are proposed to provide a large repertoire of representations in restricted networks (Shadlen and Newsome, 1998; Olshausen and Field, 2004). Distributed representations confer the benefit of resistance to network degradation (Slotnick and Bisulco, 2003; Slotnick et al., 2004; Bracey et al., 2013).
Learning and Odor Representations
Learning-related modulations of odor representations have been examined through imaging and electrophysiology. At the glomerular level olfactory learning enlarges learned-odor-associated glomeruli in rat pups (Woo et al., 1987), increases sensory neurons (Jones et al., 2008), increases sensory neuron output (Kass et al., 2013), and strengthens odor responses in weakly-activated glomeruli (Fletcher, 2012) in adult mice.
There are also increases in cFos labeled juxtaglomerular cells in rat pups (Woo and Leon, 1991). Zif268-labeled granule cells increase in signal strength, but not in overall numbers with appetitive conditioning in adult mice (Busto et al., 2009).
Increased synchrony of mitral cell spikes to learned odors also occurs in adult mice (Doucette et al., 2011). Rewarded vs. unrewarded odors had differing mitral firing rates, which reversed with reward reversal. The firing divergences were transient (Doucette and Restrepo, 2008).
Anterior piriform cortex pyramidal cell odor representations decrease after reward pairing in cFos images in adult rats (Roullet et al., 2005). In rat pups, cFos representations increased in APC with odor/shock pairing while granule cell odor representations in OB decreased (Roth and Sullivan, 2005; Roth et al., 2006). Using electrophysiology, Chapuis and Wilson (2012) demonstrated a decrease in correlated firing when rats discriminated highly similar odors and an increase in correlated firing when two odors were associated to reward. The minimal number of active APC neurons necessary for behavioral discrimination has been estimated at 300–500 (Choi et al., 2011).
Arc-Indexed Odor Representations and Learning
In recent experiments we used two odor learning paradigms to investigate representation modifications: (1) early odor preference learning in rat pup (Figure 1B); and (2) go-no-go odor discrimination learning in adult rat (Figure 1C). We employed the cellular compartment analysis of temporal activity by fluorescence in situ hybridization (catFISH) technique to visualize Arc mRNA and characterize odor representations. Arc, as one of the immediate early genes, offers an important feature for examining representations repeatedly in the same animal (Guzowski et al., 2005). It is temporally relocated from the nucleus, where it appears 5 min after recruitment, to cytoplasm where it asymptotes ~25 min later. Thus cells where Arc transcription is recently recruited by odor can be compared to the cells that responded to the same or a different odor at an earlier time point (Shakhawat et al., 2014a,b).
Rat Pup Odor Preference Learning
In the rat pup model (Shakhawat et al., 2014a; Figure 1B), ~7–8% of the mitral cells sampled responded to peppermint odor in the dorsolateral OB. Consistent with present models of OB odor representation this is a sparse representation. When a second presentation of peppermint was given to naïve animals the network activated was again ~7–8% of sampled neurons. The overlap i.e., the proportion of neurons responding to peppermint twice was surprisingly small. Thirty percent of the odor-activated neurons participated in both representations of peppermint. This result is at odds with the theoretical benefit of sparse representations, which as mentioned, are thought to maximize memory capacity. For that idea, it is usually assumed that each sparse representation gives a reliable encoding of any given memory.
What might be the source of this representational variability? Subtle contextual changes driven by top-down cortical inputs are a likely candidate (Restrepo et al., 2009). Thus one possibility is that there is additional information in each of these odor representations. For example, visual context is encoded even at the level of the OB (Mandairon et al., 2014).
Another utility of the observed variability may be noise modulation of signal processing. Noise in neuronal circuits enhances the discriminability of representations (Ermentrout et al., 2008). Evoked variability in each odor representation might increase the likelihood of a response in the APC and enhance discriminability.
The effects of reward on the peppermint representation were to increase the proportion of repeatedly activated neurons in that representation, but not to change its overall size (Shakhawat et al., 2014a). The proportion of neurons responding to both peppermint events increased to ~50%. An increase in the stable component of the peppermint representation would increase saliency and improve discriminability. Electrophysiology suggests a similar effect occurs in adult rodent OB: synchronous firing across mitral cells is enhanced by learning (Doucette et al., 2011).
While Arc is not normally recruited in interneurons (Vazdarjanova et al., 2006; McCurry et al., 2010), it is recruited in granule cells. Granule cell Arc revealed a similar pattern of effects to that of mitral cells (Shakhawat et al., 2014a). The peppermint representation indexed by granule cells was ~5% of the population sampled. This was unchanged by training. The granule cells responding to the same odor twice was ~25% of the representation and increased to ~50% with training. The Arc pattern for an unrewarded odor did not change for mitral or granule cells (Shakhawat et al., 2014a).
While mitral cells have not been imaged in earlier learning studies, granule cell representations in rat pups decreased with odor/shock pairings using cFos (Roth and Sullivan, 2005; Roth et al., 2006). The source of the discrepancy from the Arc findings is unclear. Stronger granule cell staining by Zif268, but no change in representation size, with appetitive training in adult mice (Busto et al., 2009) is consistent with the Arc results. The odor specific granule cell representation indexed by Arc also accords with theoretical predictions for the OB (Koulakov and Rinberg, 2011).
The modulation of granule cell ensembles by training is consistent with our understanding that granule cells partner with particular odor input representations and selectively modulate or track changes in those representations (Migliore et al., 2007; Yu et al., 2014). It is assumed generally that inhibition increases in concert with excitation in neural circuits (Vogels et al., 2011). Comparable increases in the stable proportion of the mitral and granule cell representations of rewarded odor are consistent with this assumption.
In the same rat pups, APC representations paralleled what was seen in the OB (Shakhawat et al., 2014a). The pyramidal cell representation was sparser with only ~1% of neurons of those sampled participating. This contrasts with a peppermint representation size in adults of about 3–5% (Shakhawat et al., 2014b). However, only one-third of mitral cells axons are mature at this age. The smaller representation likely reflects reduced APC input (Sarma et al., 2011). The stable component of the representations for repeated odors without learning was ~20%. Given a 1% representation size, one would estimate ~1500 pyramidal neurons for the odor representation (~150,000 total in one APC, Capurso et al., 1997; Duffell et al., 2000), with a stable component of only ~300 neurons, at the limit of the predicted population needed for discrimination (Choi et al., 2011). With odor preference training, this proportion increased to ~40% with no change in representation size. Representation components were unchanged without paired reward (Shakhawat et al., 2014a). The similarity of modifications in OB and APC representations are likely supported by their mutual connectivity (Restrepo et al., 2009; Boyd et al., 2013), but studies have shown that each structure can modify connectivity when reward and odor signals are paired (Sullivan et al., 2000; Lethbridge et al., 2012; Morrison et al., 2013; see also Thum et al., 2007).
Adult Rat Go-No-Go Odor Discrimination Learning and Anterior Piriform Cortex
Adult rats were tested in three types of odor discrimination (Shakhawat et al., 2014b). The first was a simple discrimination of two dissimilar odors, peppermint (rewarded) vs. vanillin (no reward; Figure 1C1); the second involved a mixture of the two odors, which were rewarded while a third odor was not (Figure 1C2). The third discrimination employed two highly similar mixtures initially difficult to discriminate (Figure 1C3).
Electrophysiology had not revealed learning differences in APC firing patterns for simple discriminations (Chapuis and Wilson, 2012). But with Arc, it was possible to observe that representation size became significantly smaller in trained rats (2.5%) vs. untrained rats (5%), with a reduction in the variable neuronal component. The overlap component did not show an absolute increase with training, but became a larger proportion of the representation. Thus, as in the rat pups (Shakhawat et al., 2014a), the stable component of the APC odor representation increased with learning from 25% to 40% (Shakhawat et al., 2014b). A learning-induced decrease in APC odor representation size was previously seen with cFos (Roullet et al., 2005).
In the mixture discrimination, the two individual odor components were responded to as rewarded odors during behavioral probes, suggesting rats learned the components during mixture training or pattern-completed from partial cues. The degree of overlap between peppermint and vanillin in control rats was ~20% while overlap was ~45% in trained rats, consistent with the two odors becoming representationally highly similar (Shakhawat et al., 2014b).
In the difficult discrimination, the odor representation size was ~3% and did not change with learning (Shakhawat et al., 2014b). The proportion of cells responding to the similar odors was 23%, not different from the overlap seen to repeated odors (25–30%). After training to differentiate the odors, the proportion of cells responding twice to the two similar odors (overlap) was significantly less (~13%). The mechanisms by which reward-mediated decorrelation is achieved are not well understood. Cholinergic inputs have been shown to modulate odor pattern separation in the APC (Chapuis and Wilson, 2013).
General Inferences Regarding Reward Effects on Odor Representations
The mechanisms for associating odor and reward, presumably resulting in the increases in stability components seen here, have been examined earlier. In the rat pup, norepinephrine paired with odor can alter behavior and AMPA receptor-mediated connectivity in OB (Cui et al., 2011; Yuan and Harley, 2012) and APC (Fontaine et al., 2013; Morrison et al., 2013). But the common assumption that spike timing-dependent plasticity in cortical circuits underlies representational changes has not been supported when examining optimal temporal relationships between odor input and reward signals using recording of cell firing to define the two inputs (Ito et al., 2008). It has subsequently been recognized that a neuromodulator is required as a third partner to enable spike timing dependent plasticity (STDP; Pawlak et al., 2010; Cassenaer and Laurent, 2012). This appears likely to occur in rat pup odor learning. We suggest that priming of co-incident odor-activated pre- and post-synaptic elements in OB and APC of rat pups, which are then strengthened by norepinephrine, is the most probable mechanism of the representation modifications seen in pups.
Novel Representational Features Indexed by Arc
The two novel results that Arc imaging made possible was the finding of high variability in repeated odor representations and the finding of a high degree of overlap between representations of distinct odors. If cells encoding distinct odors were drawn at random from populations with replacement such that the same cell could participate in multiple representations, then the predicted overlap would be the representational size squared. In Arc studies, representational size varies from 1% to 10%, consistent with other estimates (e.g., Lin da et al., 2006, OB 3–10%; Stettler and Axel, 2009, APC 3–15% at low concentrations), and the predicted overlap would be no more than 1%. The overlap observed for the odors peppermint and vanillin, which according to the earlier spatial maps are represented differentially in OB (see Johnson and Leon’s 2-DG odor glomerular maps1), was ~20% in OB and APC. What is the source of this large overlap? The discovery of primed or widely responsive neuron subsets comprising about 20–25% of studied populations in a variety of cortical circuits including APC (Yassin et al., 2010; Zhan and Luo, 2010; Luczak and Maclean, 2012; Mizuseki and Buzsaki, 2013; Reuveni et al., 2013), may account in part for larger overlap. Another contributing factor might be that the two odors share molecular features which, despite being distributed, would still result in representations with overlapping neurons (Saito et al., 2009; Ma et al., 2012). A greater diversity of odors needs to be tested with Arc.
Arc’s Relation to Odor Evoked Activity
Finally we come to the issue of what Arc itself indicates. While it is related to neuronal activation, neural firing cannot be its sole initiator. Mitral cells fire at high spontaneous levels (Rinberg et al., 2006). This activity may be driven by muscarinic inputs in conjunction with the intrinsic variable biophysical properties of mitral cells (Padmanabhan and Urban, 2010; Angelo and Margrie, 2011) and for that reason does not recruit Arc activation. Arc recruitment is likely linked to glutamatergic excitatory synaptic driving (Cole et al., 1989), as occurs with odor input.
But even if that is the case, one must ask which driving patterns are encoded by Arc recruitment. Odor activation firing patterns in OB and APC are dynamic spatially and temporally (Friedrich and Laurent, 2001; Rennaker et al., 2007; Schaefer and Margrie, 2007), although encoding and perception of odors can occur very rapidly (Uchida and Mainen, 2003; Wesson et al., 2008). At different time points different cells fire and these responses are embedded in the high spontaneous background. Static odor representations have been extracted statistically at different points and appear to synchronize with sniffs (~250 ms). While a single sniff suffices for odor discrimination, the odor representation continues to evolve (Patterson et al., 2013). In subsequent breaths the statistical odor template changes and stabilizes (see also Broome et al., 2006). Does Arc represent the initial pattern and its variability or the combined early and later patterns and their cumulative variability? This would be another contribution to the high variability observed.
How many neurons are excited in a single synchronized odor representation? The size of odor representations appears to be normalized and varies less for concentration increases (Cleland, 2010) than one might expect given the wider glomerular activation as concentration increases (Meister and Bonhoeffer, 2001). Increased concentrations are easier to discriminate (Escanilla et al., 2008) and this may relate to stronger peak excitation (Cleland, 2010); latency for initial spikes is also reduced (Stopfer et al., 2003), suggesting an importance of early timing. From Arc patterns, we would predict a larger stable component with increasing concentrations supporting more rapid discriminations.
Conclusion
Arc-indexed cell networks portray odor representations in both OB and APC as sparse and distributed consistent with current understanding. Arc-indexed networks also reveal a considerable variability in the awake mammalian odor representation consistent with the electrophysiological evidence. This methodology further reveals a larger component of common neuronal activation for distinct odors than predicted by theory. Appetitive learning modifies odor representations to increase the proportion of stable neurons. Network representations can also decrease the proportion of stable neurons when increased behavioral discrimination is required.
Conflict of Interest Statement
The authors declare that the research was conducted in the absence of any commercial or financial relationships that could be construed as a potential conflict of interest.
Acknowledgments
This work was supported by Canadian Institutes of Health Research Operating Grant (MOP-102624) and Natural Sciences and Engineering Research Council of Canada Discovery Grant (418451-2013) to Qi Yuan.
Footnotes
References
Angelo, K., and Margrie, T. W. (2011). Population diversity and function of hyperpolarization-activated current in olfactory bulb mitral cells. Sci. Rep. 1:50. doi: 10.1038/srep00050
Pubmed Abstract | Pubmed Full Text | CrossRef Full Text | Google Scholar
Boyd, A. M., Sturgill, J. F., Poo, C., and Isaacson, J. S. (2013). Cortical feedback control of olfactory bulb circuits. Neuron 76, 1161–1174. doi: 10.1016/j.neuron.2012.10.020
Pubmed Abstract | Pubmed Full Text | CrossRef Full Text | Google Scholar
Bracey, E. F., Pichler, B., Schaefer, A. T., Wallace, D. J., and Margrie, T. W. (2013). Perceptual judgements and chronic imaging of altered odour maps indicate comprehensive stimulus template matching in olfaction. Nat. Commun. 4:2100. doi: 10.1038/ncomms3100
Pubmed Abstract | Pubmed Full Text | CrossRef Full Text | Google Scholar
Broome, B. M., Jayaraman, V., and Laurent, G. (2006). Encoding and decoding of overlapping odor sequences. Neuron 51, 467–482. doi: 10.1016/j.neuron.2006.07.018
Pubmed Abstract | Pubmed Full Text | CrossRef Full Text | Google Scholar
Busto, G. U., Elie, J. E., Kermen, F., Garcia, S., Sacquet, J., Jourdan, F., et al. (2009). Expression of Zif268 in the granule cell layer of the adult mouse olfactory bulb is modulated by experience. Eur. J. Neurosci. 29, 1431–1439. doi: 10.1111/j.1460-9568.2009.06689.x
Pubmed Abstract | Pubmed Full Text | CrossRef Full Text | Google Scholar
Capurso, S. A., Calhoun, M. E., Sukhov, R. R., Mouton, P. R., Price, D. L., and Koliatsos, V. E. (1997). Deafferentation causes apoptosis in cortical sensory neurons in the adult rat. J. Neurosci. 17, 7372–7384.
Cassenaer, S., and Laurent, G. (2012). Conditional modulation of spike-timing-dependent plasticity for olfactory learning. Nature 482, 47–52. doi: 10.1038/nature10776
Pubmed Abstract | Pubmed Full Text | CrossRef Full Text | Google Scholar
Chapuis, J., Cohen, Y., He, X., Zhang, Z., Jin, S., Xu, F., et al. (2013). Lateral entorhinal modulation of piriform cortical activity and fine odor discrimination. J. Neurosci. 33, 13449–13459. doi: 10.1523/jneurosci.1387-13.2013
Pubmed Abstract | Pubmed Full Text | CrossRef Full Text | Google Scholar
Chapuis, J., and Wilson, D. A. (2012). Bidirectional plasticity of cortical pattern recognition and behavioral sensory acuity. Nat. Neurosci. 15, 155–161. doi: 10.1038/nn.2966
Pubmed Abstract | Pubmed Full Text | CrossRef Full Text | Google Scholar
Chapuis, J., and Wilson, D. A. (2013). Cholinergic modulation of olfactory pattern separation. Neurosci. Lett. 545, 50–53. doi: 10.1016/j.neulet.2013.04.015
Pubmed Abstract | Pubmed Full Text | CrossRef Full Text | Google Scholar
Chaput, M. A., and Holley, A. (1985). Responses of olfactory bulb neurons to repeated odor stimulations in awake freely-breathing rabbits. Physiol. Behav. 34, 249–258. doi: 10.1016/0031-9384(85)90113-1
Pubmed Abstract | Pubmed Full Text | CrossRef Full Text | Google Scholar
Choi, G. B., Stettler, D. D., Kallman, B. R., Bhaskar, S. T., Fleischmann, A., and Axel, R. (2011). Driving opposing behaviors with ensembles of piriform neurons. Cell 146, 1004–1015. doi: 10.1016/j.cell.2011.07.041
Pubmed Abstract | Pubmed Full Text | CrossRef Full Text | Google Scholar
Cleland, T. A. (2010). Early transformations in odor representation. Trends Neurosci. 33, 130–139. doi: 10.1016/j.tins.2009.12.004
Pubmed Abstract | Pubmed Full Text | CrossRef Full Text | Google Scholar
Cole, A. J., Saffen, D. W., Baraban, J. M., and Worley, P. F. (1989). Rapid increase of an immediate early gene messenger RNA in hippocampal neurons by synaptic NMDA receptor activation. Nature 340, 474–476. doi: 10.1038/340474a0
Pubmed Abstract | Pubmed Full Text | CrossRef Full Text | Google Scholar
Cui, W., Darby-King, A., Grimes, M. T., Howland, J. G., Wang, Y. T., Mclean, J. H., et al. (2011). Odor preference learning and memory modify GluA1phosphorylation and GluA1distribution in the neonate rat olfactory bulb: testing the AMPA receptor hypothesis in an appetitive learning model. Learn. Mem. 18, 283–291. doi: 10.1101/lm.1987711
Pubmed Abstract | Pubmed Full Text | CrossRef Full Text | Google Scholar
Davison, I. G., and Ehlers, M. D. (2011). Neural circuit mechanisms for pattern detection and feature combination in olfactory cortex. Neuron 70, 82–94. doi: 10.1016/j.neuron.2011.02.047
Pubmed Abstract | Pubmed Full Text | CrossRef Full Text | Google Scholar
Doucette, W., Gire, D. H., Whitesell, J., Carmean, V., Lucero, M. T., and Restrepo, D. (2011). Associative cortex features in the first olfactory brain relay station. Neuron 69, 1176–1187. doi: 10.1016/j.neuron.2011.02.024
Pubmed Abstract | Pubmed Full Text | CrossRef Full Text | Google Scholar
Doucette, W., and Restrepo, D. (2008). Profound context-dependent plasticity of mitral cell responses in olfactory bulb. PLoS Biol. 6:e258. doi: 10.1371/journal.pbio.0060258
Pubmed Abstract | Pubmed Full Text | CrossRef Full Text | Google Scholar
Duffell, S. J., Soames, A. R., and Gunby, S. (2000). Morphometric analysis of the developing rat brain. Toxicol. Pathol. 28, 157–163. doi: 10.1177/019262330002800120
Pubmed Abstract | Pubmed Full Text | CrossRef Full Text | Google Scholar
Ermentrout, G. B., Galán, R. F., and Urban, N. N. (2008). Reliability, synchrony and noise. Trends Neurosci. 31, 428–434. doi: 10.1016/j.tins.2008.06.002
Pubmed Abstract | Pubmed Full Text | CrossRef Full Text | Google Scholar
Escanilla, O., Mandairon, N., and Linster, C. (2008). Odor-reward learning and enrichment have similar effects on odor perception. Physiol. Behav. 94, 621–626. doi: 10.1016/j.physbeh.2008.03.008
Pubmed Abstract | Pubmed Full Text | CrossRef Full Text | Google Scholar
Fletcher, M. L. (2012). Olfactory aversive conditioning alters olfactory bulb mitral/tufted cell glomerular odor responses. Front. Syst. Neurosci. 6:16. doi: 10.3389/fnsys.2012.00016
Pubmed Abstract | Pubmed Full Text | CrossRef Full Text | Google Scholar
Fontaine, C. J., Harley, C. W., and Yuan, Q. (2013). Lateralized odor preference training in rat pups reveals an enhanced network response in anterior piriform cortex to olfactory input that parallels extended memory. J. Neurosci. 33, 15126–15131. doi: 10.1523/jneurosci.2503-13.2013
Pubmed Abstract | Pubmed Full Text | CrossRef Full Text | Google Scholar
Friedrich, R. W., and Laurent, G. (2001). Dynamic optimization of odor representations by slow temporal patterning of mitral cell activity. Science 291, 889–894. doi: 10.1126/science.291.5505.889
Pubmed Abstract | Pubmed Full Text | CrossRef Full Text | Google Scholar
Guzowski, J. F., Timlin, J. A., Roysam, B., Mcnaughton, B. L., Worley, P. F., and Barnes, C. A. (2005). Mapping behaviorally relevant neural circuits with immediate-early gene expression. Curr. Opin. Neurobiol. 15, 599–606. doi: 10.1016/j.conb.2005.08.018
Pubmed Abstract | Pubmed Full Text | CrossRef Full Text | Google Scholar
Haberly, L. B. (2001). Parallel-distributed processing in olfactory cortex: new insights from morphological and physiological analysis of neuronal circuitry. Chem. Senses 26, 551–576. doi: 10.1093/chemse/26.5.551
Pubmed Abstract | Pubmed Full Text | CrossRef Full Text | Google Scholar
Isaacson, J. S. (2010). Odor representations in mammalian cortical circuits. Curr. Opin. Neurobiol. 20, 328–331. doi: 10.1016/j.conb.2010.02.004
Pubmed Abstract | Pubmed Full Text | CrossRef Full Text | Google Scholar
Ito, I., Ong, R. C., Raman, B., and Stopfer, M. (2008). Olfactory learning and spike timing dependent plasticity. Commun. Integr. Biol. 1, 170–171. doi: 10.4161/cib.1.2.7140
Pubmed Abstract | Pubmed Full Text | CrossRef Full Text | Google Scholar
Jones, S. V., Choi, D. C., Davis, M., and Ressler, K. J. (2008). Learning-dependent structural plasticity in the adult olfactory pathway. J. Neurosci. 28, 13106–13111. doi: 10.1523/jneurosci.4465-08.2008
Pubmed Abstract | Pubmed Full Text | CrossRef Full Text | Google Scholar
Kass, M. D., Rosenthal, M. C., Pottackal, J., and Mcgann, J. P. (2013). Fear learning enhances neural responses to threat-predictive sensory stimuli. Science 342, 1389–1392. doi: 10.1126/science.1244916
Pubmed Abstract | Pubmed Full Text | CrossRef Full Text | Google Scholar
Kay, L. M., and Laurent, G. (1999). Odor- and context-dependent modulation of mitral cell activity in behaving rats. Nat. Neurosci. 2, 1003–1009. doi: 10.1038/14801
Pubmed Abstract | Pubmed Full Text | CrossRef Full Text | Google Scholar
Knafo, S., Libersat, F., and Barkai, E. (2005). Dynamics of learning-induced spine redistribution along dendrites of pyramidal neurons in rats. Eur. J. Neurosci. 21, 927–935. doi: 10.1111/j.1460-9568.2005.03936.x
Pubmed Abstract | Pubmed Full Text | CrossRef Full Text | Google Scholar
Koulakov, A. A., and Rinberg, D. (2011). Sparse incomplete representations: a potential role of olfactory granule cells. Neuron 72, 124–136. doi: 10.1016/j.neuron.2011.07.031
Pubmed Abstract | Pubmed Full Text | CrossRef Full Text | Google Scholar
Krofczik, S., Menzel, R., and Nawrot, M. P. (2008). Rapid odor processing in the honeybee antennal lobe network. Front. Comput. Neurosci. 2:9. doi: 10.3389/neuro.10.009.2008
Pubmed Abstract | Pubmed Full Text | CrossRef Full Text | Google Scholar
Laurent, G. (1996). Dynamical representation of odors by oscillating and evolving neural assemblies. Trends Neurosci. 19, 489–496. doi: 10.1016/s0166-2236(96)10054-0
Pubmed Abstract | Pubmed Full Text | CrossRef Full Text | Google Scholar
Laurent, G. (1997). Olfactory processing: maps, time and codes. Curr. Opin. Neurobiol. 7, 547–553. doi: 10.1016/s0959-4388(97)80035-9
Pubmed Abstract | Pubmed Full Text | CrossRef Full Text | Google Scholar
Leon, M., and Johnson, B. A. (2003). Olfactory coding in the mammalian olfactory bulb. Brain Res. Brain Res. Rev. 42, 23–32. doi: 10.1016/s0165-0173(03)00142-5
Pubmed Abstract | Pubmed Full Text | CrossRef Full Text | Google Scholar
Lethbridge, R., Hou, Q., Harley, C. W., and Yuan, Q. (2012). Olfactory bulb glomerular NMDA receptors mediate olfactory nerve potentiation and odor preference learning in the neonate rat. PLoS One 7:e35024. doi: 10.1371/journal.pone.0035024
Pubmed Abstract | Pubmed Full Text | CrossRef Full Text | Google Scholar
Lin da, Y., Shea, S. D., and Katz, L. C. (2006). Representation of natural stimuli in the rodent main olfactory bulb. Neuron 50, 937–949. doi: 10.1016/j.neuron.2006.03.021
Pubmed Abstract | Pubmed Full Text | CrossRef Full Text | Google Scholar
Luczak, A., and Maclean, J. N. (2012). Default activity patterns at the neocortical microcircuit level. Front. Integr. Neurosci. 6:30. doi: 10.3389/fnint.2012.00030
Pubmed Abstract | Pubmed Full Text | CrossRef Full Text | Google Scholar
Ma, L., Qiu, Q., Gradwohl, S., Scott, A., Yu, E. Q., Alexander, R., et al. (2012). Distributed representation of chemical features and tunotopic organization of glomeruli in the mouse olfactory bulb. Proc. Natl. Acad. Sci. U S A 109, 5481–5486. doi: 10.1073/pnas.1117491109
Pubmed Abstract | Pubmed Full Text | CrossRef Full Text | Google Scholar
Mandairon, N., Kermen, F., Charpentier, C., Sacquet, J., Linster, C., and Didier, A. (2014). Context-driven activation of odor representations in the absence of olfactory stimuli in the olfactory bulb and piriform cortex. Front. Behav. Neurosci. 8:138. doi: 10.3389/fnbeh.2014.00138
Pubmed Abstract | Pubmed Full Text | CrossRef Full Text | Google Scholar
Mandairon, N., and Linster, C. (2009). Odor perception and olfactory bulb plasticity in adult mammals. J. Neurophysiol. 101, 2204–2209. doi: 10.1152/jn.00076.2009
Pubmed Abstract | Pubmed Full Text | CrossRef Full Text | Google Scholar
McCurry, C. L., Shepherd, J. D., Tropea, D., Wang, K. H., Bear, M. F., and Sur, M. (2010). Loss of Arc renders the visual cortex impervious to the effects of sensory experience or deprivation. Nat. Neurosci. 13, 450–457. doi: 10.1038/nn.2508
Pubmed Abstract | Pubmed Full Text | CrossRef Full Text | Google Scholar
Meister, M., and Bonhoeffer, T. (2001). Tuning and topography in an odor map on the rat olfactory bulb. J. Neurosci. 21, 1351–1360.
Migliore, M., Inzirillo, C., and Shepherd, G. M. (2007). Learning mechanism for column formation in the olfactory bulb. Front. Integr. Neurosci. 1:12. doi: 10.3389/neuro.07.012.2007
Pubmed Abstract | Pubmed Full Text | CrossRef Full Text | Google Scholar
Mizuseki, K., and Buzsaki, G. (2013). Preconfigured, skewed distribution of firing rates in the hippocampus and entorhinal cortex. Cell Rep. 4, 1010–1021. doi: 10.1016/j.celrep.2013.07.039
Pubmed Abstract | Pubmed Full Text | CrossRef Full Text | Google Scholar
Mombaerts, P., Wang, F., Dulac, C., Chao, S. K., Nemes, A., Mendelsohn, M., et al. (1996). Visualizing an olfactory sensory map. Cell 87, 675–686.
Mori, K., Takahashi, Y. K., Igarashi, K. M., and Yamaguchi, M. (2006). Maps of odorant molecular features in the Mammalian olfactory bulb. Physiol. Rev. 86, 409–433. doi: 10.1152/physrev.00021.2005
Pubmed Abstract | Pubmed Full Text | CrossRef Full Text | Google Scholar
Morrison, G. L., Fontaine, C. J., Harley, C. W., and Yuan, Q. (2013). A role for the anterior piriform cortex in early odor preference learning: evidence for multiple olfactory learning structures in the rat pup. J. Neurophysiol. 110, 141–152. doi: 10.1152/jn.00072.2013
Pubmed Abstract | Pubmed Full Text | CrossRef Full Text | Google Scholar
Olshausen, B. A., and Field, D. J. (2004). Sparse coding of sensory inputs. Curr. Opin. Neurobiol. 14, 481–487. doi: 10.1016/j.conb.2004.07.007
Pubmed Abstract | Pubmed Full Text | CrossRef Full Text | Google Scholar
Padmanabhan, K., and Urban, N. N. (2010). Intrinsic biophysical diversity decorrelates neuronal firing while increasing information content. Nat. Neurosci. 13, 1276–1282. doi: 10.1038/nn.2630
Pubmed Abstract | Pubmed Full Text | CrossRef Full Text | Google Scholar
Patterson, M. A., Lagier, S., and Carleton, A. (2013). Odor representations in the olfactory bulb evolve after the first breath and persist as an odor afterimage. Proc. Natl. Acad. Sci. U S A 110, E3340–E3349. doi: 10.1073/pnas.1303873110
Pubmed Abstract | Pubmed Full Text | CrossRef Full Text | Google Scholar
Pawlak, V., Wickens, J. R., Kirkwood, A., and Kerr, J. N. (2010). Timing is not everything: neuromodulation opens the STDP gate. Front. Synaptic Neurosci. 2:146. doi: 10.3389/fnsyn.2010.00146
Pubmed Abstract | Pubmed Full Text | CrossRef Full Text | Google Scholar
Poo, C., and Isaacson, J. S. (2011). A major role for intracortical circuits in the strength and tuning of odor-evoked excitation in olfactory cortex. Neuron 72, 41–48. doi: 10.1016/j.neuron.2011.08.015
Pubmed Abstract | Pubmed Full Text | CrossRef Full Text | Google Scholar
Rennaker, R. L., Chen, C. F., Ruyle, A. M., Sloan, A. M., and Wilson, D. A. (2007). Spatial and temporal distribution of odorant-evoked activity in the piriform cortex. J. Neurosci. 27, 1534–1542. doi: 10.1523/jneurosci.4072-06.2007
Pubmed Abstract | Pubmed Full Text | CrossRef Full Text | Google Scholar
Restrepo, D., Doucette, W., Whitesell, J. D., Mctavish, T. S., and Salcedo, E. (2009). From the top down: flexible reading of a fragmented odor map. Trends Neurosci. 32, 525–531. doi: 10.1016/j.tins.2009.06.001
Pubmed Abstract | Pubmed Full Text | CrossRef Full Text | Google Scholar
Reuveni, I., Saar, D., and Barkai, E. (2013). A novel whole-cell mechanism for long-term memory enhancement. PLoS One 8:e68131. doi: 10.1371/journal.pone.0068131
Pubmed Abstract | Pubmed Full Text | CrossRef Full Text | Google Scholar
Rinberg, D., Koulakov, A., and Gelperin, A. (2006). Sparse odor coding in awake behaving mice. J. Neurosci. 26, 8857–8865. doi: 10.1523/jneurosci.0884-06.2006
Pubmed Abstract | Pubmed Full Text | CrossRef Full Text | Google Scholar
Roth, T. L., Moriceau, S., and Sullivan, R. M. (2006). Opioid modulation of Fos protein expression and olfactory circuitry plays a pivotal role in what neonates remember. Learn. Mem. 13, 590–598. doi: 10.1101/lm.301206
Pubmed Abstract | Pubmed Full Text | CrossRef Full Text | Google Scholar
Roth, T. L., and Sullivan, R. M. (2005). Memory of early maltreatment: neonatal behavioral and neural correlates of maternal maltreatment within the context of classical conditioning. Biol. Psychiatry 57, 823–831. doi: 10.1016/j.biopsych.2005.01.032
Pubmed Abstract | Pubmed Full Text | CrossRef Full Text | Google Scholar
Rothermel, M., and Wachowiak, M. (2014). Functional imaging of cortical feedback projections to the olfactory bulb. Front. Neural Circuits 8:73. doi: 10.3389/fncir.2014.00073
Pubmed Abstract | Pubmed Full Text | CrossRef Full Text | Google Scholar
Roullet, F., Datiche, F., Liénard, F., and Cattarelli, M. (2005). Learning-stage dependent Fos expression in the rat brain during acquisition of an olfactory discrimination task. Behav. Brain Res. 157, 127–137. doi: 10.1016/j.bbr.2004.06.017
Pubmed Abstract | Pubmed Full Text | CrossRef Full Text | Google Scholar
Rubin, B. D., and Katz, L. C. (1999). Optical imaging of odorant representations in the mammalian olfactory bulb. Neuron 23, 499–511. doi: 10.1016/s0896-6273(00)80803-x
Pubmed Abstract | Pubmed Full Text | CrossRef Full Text | Google Scholar
Saito, H., Chi, Q., Zhuang, H., Matsunami, H., and Mainland, J. D. (2009). Odor coding by a Mammalian receptor repertoire. Sci. Signal 2:ra9. doi: 10.1126/scisignal.2000016
Pubmed Abstract | Pubmed Full Text | CrossRef Full Text | Google Scholar
Sarma, A. A., Richard, M. B., and Greer, C. A. (2011). Developmental dynamics of piriform cortex. Cereb. Cortex 21, 1231–1245. doi: 10.1093/cercor/bhq199
Pubmed Abstract | Pubmed Full Text | CrossRef Full Text | Google Scholar
Schaefer, A. T., and Margrie, T. W. (2007). Spatiotemporal representations in the olfactory system. Trends Neurosci. 30, 92–100. doi: 10.1016/j.tins.2007.01.001
Pubmed Abstract | Pubmed Full Text | CrossRef Full Text | Google Scholar
Shadlen, M. N., and Newsome, W. T. (1998). The variable discharge of cortical neurons: implications for connectivity, computation and information coding. J. Neurosci. 18, 3870–3896.
Shakhawat, A. M., Gheidi, A., Hou, Q., Dhillon, S. K., Marrone, D. F., Harley, C. W., et al. (2014a). Visualizing the engram: learning stabilizes odor representations in the olfactory network. J. Neurosci. 34, 15394–15401. doi: 10.1523/JNEUROSCI.3396-14.2014
Pubmed Abstract | Pubmed Full Text | CrossRef Full Text | Google Scholar
Shakhawat, A. M., Harley, C. W., and Yuan, Q. (2014b). Arc visualization of odor objects reveals experience-dependent ensemble sharpening, separation and merging in anterior piriform cortex in adult rat. J. Neurosci. 34, 10206–10210. doi: 10.1523/jneurosci.1942-14.2014
Pubmed Abstract | Pubmed Full Text | CrossRef Full Text | Google Scholar
Slotnick, B., and Bisulco, S. (2003). Detection and discrimination of carvone enantiomers in rats with olfactory bulb lesions. Neuroscience 121, 451–457. doi: 10.1016/s0306-4522(03)00402-0
Pubmed Abstract | Pubmed Full Text | CrossRef Full Text | Google Scholar
Slotnick, B., Cockerham, R., and Pickett, E. (2004). Olfaction in olfactory bulbectomized rats. J. Neurosci. 24, 9195–9200. doi: 10.1523/jneurosci.1936-04.2004
Pubmed Abstract | Pubmed Full Text | CrossRef Full Text | Google Scholar
Soucy, E. R., Albeanu, D. F., Fantana, A. L., Murthy, V. N., and Meister, M. (2009). Precision and diversity in an odor map on the olfactory bulb. Nat. Neurosci. 12, 210–220. doi: 10.1038/nn.2262
Pubmed Abstract | Pubmed Full Text | CrossRef Full Text | Google Scholar
Stettler, D. D., and Axel, R. (2009). Representations of odor in the piriform cortex. Neuron 63, 854–864. doi: 10.1016/j.neuron.2009.09.005
Pubmed Abstract | Pubmed Full Text | CrossRef Full Text | Google Scholar
Stopfer, M., Jayaraman, V., and Laurent, G. (2003). Intensity versus identity coding in an olfactory system. Neuron 39, 991–1004. doi: 10.1016/j.neuron.2003.08.011
Pubmed Abstract | Pubmed Full Text | CrossRef Full Text | Google Scholar
Sullivan, R. M., Stackenwalt, G., Nasr, F., Lemon, C., and Wilson, D. A. (2000). Association of an odor with activation of olfactory bulb noradrenergic beta-receptors or locus coeruleus stimulation is sufficient to produce learned approach responses to that odor in neonatal rats. Behav. Neurosci. 114, 957–962. doi: 10.1037//0735-7044.114.5.957
Pubmed Abstract | Pubmed Full Text | CrossRef Full Text | Google Scholar
Thum, A. S., Jenett, A., Ito, K., Heisenberg, M., and Tanimoto, H. (2007). Multiple memory traces for olfactory reward learning in Drosophila. J. Neurosci. 27, 11132–11138. doi: 10.1523/jneurosci.2712-07.2007
Pubmed Abstract | Pubmed Full Text | CrossRef Full Text | Google Scholar
Uchida, N., and Mainen, Z. F. (2003). Speed and accuracy of olfactory discrimination in the rat. Nat. Neurosci. 6, 1224–1229. doi: 10.1038/nn1142
Pubmed Abstract | Pubmed Full Text | CrossRef Full Text | Google Scholar
Vazdarjanova, A., Ramirez-Amaya, V., Insel, N., Plummer, T. K., Rosi, S., Chowdhury, S., et al. (2006). Spatial exploration induces ARC, a plasticity-related immediate-early gene, only in calcium/calmodulin-dependent protein kinase II-positive principal excitatory and inhibitory neurons of the rat forebrain. J. Comp. Neurol. 498, 317–329. doi: 10.1002/cne.21003
Pubmed Abstract | Pubmed Full Text | CrossRef Full Text | Google Scholar
Vogels, T. P., Sprekeler, H., Zenke, F., Clopath, C., and Gerstner, W. (2011). Inhibitory plasticity balances excitation and inhibition in sensory pathways and memory networks. Science 334, 1569–1573. doi: 10.1126/science.1211095
Pubmed Abstract | Pubmed Full Text | CrossRef Full Text | Google Scholar
Wachowiak, M., and Cohen, L. B. (2001). Representation of odorants by receptor neuron input to the mouse olfactory bulb. Neuron 32, 723–735. doi: 10.1016/s0896-6273(01)00506-2
Pubmed Abstract | Pubmed Full Text | CrossRef Full Text | Google Scholar
Wesson, D. W., Carey, R. M., Verhagen, J. V., and Wachowiak, M. (2008). Rapid encoding and perception of novel odors in the rat. PLoS Biol. 6:e82. doi: 10.1371/journal.pbio.0060082
Pubmed Abstract | Pubmed Full Text | CrossRef Full Text | Google Scholar
Wilson, D. A., and Sullivan, R. M. (2011). Cortical processing of odor objects. Neuron 72, 506–519. doi: 10.1016/j.neuron.2011.10.027
Pubmed Abstract | Pubmed Full Text | CrossRef Full Text | Google Scholar
Woo, C. C., Coopersmith, R., and Leon, M. (1987). Localized changes in olfactory bulb morphology associated with early olfactory learning. J. Comp. Neurol. 263, 113–125. doi: 10.1002/cne.902630110
Pubmed Abstract | Pubmed Full Text | CrossRef Full Text | Google Scholar
Woo, C. C., and Leon, M. (1991). Increase in a focal population of juxtaglomerular cells in the olfactory bulb associated with early learning. J. Comp. Neurol. 305, 49–56. doi: 10.1002/cne.903050106
Pubmed Abstract | Pubmed Full Text | CrossRef Full Text | Google Scholar
Xu, F., Greer, C. A., and Shepherd, G. M. (2000). Odor maps in the olfactory bulb. J. Comp. Neurol. 422, 489–495. doi: 10.1002/1096-9861(20000710)422:4<489::aid-cne1>3.0.co;2-#
Pubmed Abstract | Pubmed Full Text | CrossRef Full Text | Google Scholar
Yassin, L., Benedetti, B. L., Jouhanneau, J. S., Wen, J. A., Poulet, J. F., and Barth, A. L. (2010). An embedded subnetwork of highly active neurons in the neocortex. Neuron 68, 1043–1050. doi: 10.1016/j.neuron.2010.11.029
Pubmed Abstract | Pubmed Full Text | CrossRef Full Text | Google Scholar
Yu, Y., Migliore, M., Hines, M. L., and Shepherd, G. M. (2014). Sparse coding and lateral inhibition arising from balanced and unbalanced dendrodendritic excitation and inhibition. J. Neurosci. 34, 13701–13713. doi: 10.1523/jneurosci.1834-14.2014
Pubmed Abstract | Pubmed Full Text | CrossRef Full Text | Google Scholar
Yuan, Q., and Harley, C. W. (2012). What a nostril knows: olfactory nerve-evoked AMPA responses increase while NMDA responses decrease at 24-h post-training for lateralized odor preference memory in neonate rat. Learn. Mem. 19, 50–53. doi: 10.1101/lm.024844.111
Pubmed Abstract | Pubmed Full Text | CrossRef Full Text | Google Scholar
Zhan, C., and Luo, M. (2010). Diverse patterns of odor representation by neurons in the anterior piriform cortex of awake mice. J. Neurosci. 30, 16662–16672. doi: 10.1523/jneurosci.4400-10.2010
Pubmed Abstract | Pubmed Full Text | CrossRef Full Text | Google Scholar
Keywords: anterior piriform cortex, olfactory bulb, early odor preference learning, odor discrimination learning, Arc, viability, sparse coding
Citation: Yuan Q and Harley CW (2014) Learning modulation of odor representations: new findings from Arc-indexed networks. Front. Cell. Neurosci. 8:423. doi: 10.3389/fncel.2014.00423
Received: 05 August 2014; Accepted: 23 November 2014;
Published online: 19 December 2014.
Edited by:
Arianna Maffei, State University of New York at Stony Brook, USAReviewed by:
Christiane Linster, Cornell University, USADaniel W. Wesson, Case Western Reserve University, USA
Copyright © 2014 Yuan and Harley. This is an open-access article distributed under the terms of the Creative Commons Attribution License (CC BY). The use, distribution and reproduction in other forums is permitted, provided the original author(s) or licensor are credited and that the original publication in this journal is cited, in accordance with accepted academic practice. No use, distribution or reproduction is permitted which does not comply with these terms.
*Correspondence: Qi Yuan, Division of Biomedical Sciences, Faculty of Medicine, Memorial University of Newfoundland, Health Science Center 5340, 300 Prince Philip Dr., St. John’s, NL A1B 3V6, Canada e-mail:cWkueXVhbkBtZWQubXVuLmNh;
Carolyn W. Harley, Department of Psychology, Faculty of Science, Memorial University of Newfoundland, 300 Prince Philip Dr., St. John’s, NL A1B 3X9, Canada e-mail:Y2hhcmxleUBwbGF5LnBzeWNoLm11bi5jYQ==