- 1Department of Neurobiology, Northwestern University, Evanston, IL, United States
- 2Tianjin Eye Hospital, Tianjin Key Laboratory of Ophthalmology and Visual Science, Tianjin Eye Institute, Clinical College of Ophthalmology, Tianjin Medical University, Tianjin, China
- 3General Hospital, Tianjin Medical University, Tianjin, China
- 4Department of Biology and Department of Psychology, University of Virginia, Charlottesville, VA, United States
Neurons in the visual system display varying degrees of selectivity for stimulus features such as orientation and direction. Such feature selectivity is generated and processed by intricate circuit and synaptic mechanisms. A key factor in this process is the input-output transformation from membrane potential (Vm) to spikes in individual neurons. Here, we use in vivo whole-cell recording to study Vm-to-spike transformation of visual feature selectivity in the superficial neurons of the mouse superior colliculus (SC). As expected from the spike threshold effect, direction and orientation selectivity increase from Vm to spike responses. The degree of this increase is highly variable, and interestingly, it is correlated with the receptive field size of the recorded neurons. We find that the relationships between Vm and spike rate and between Vm dynamics and spike initiation are also correlated with receptive field size, which likely contribute to the observed input-output transformation of feature selectivity. Together, our findings provide useful information for understanding information processing and visual transformation in the mouse SC.
Introduction
Each neuron functions as an information processing unit that transforms synaptic input to spiking output. How individual neurons convert membrane potential (Vm) dynamics to spiking activity in this transformation process is a key factor in determining how they encode sensory stimuli or motor commands. Several biophysical factors underlying Vm-to-spike transformation have been revealed to contribute to response selectivity in the visual cortex, where neurons are tuned to features such as stimulus orientation and motion direction (Hubel and Wiesel, 1959, 1962; Ferster and Miller, 2000; Priebe, 2016). For example, spiking threshold enhances feature selectivity of the spike response over that of the membrane potential (Carandini and Ferster, 2000; Priebe and Ferster, 2008, 2012; Tan et al., 2011). Interestingly, spiking threshold is not a fixed value, but depends on the rate of Vm depolarization and firing history (Azouz and Gray, 2000; Wester and Contreras, 2013; Fontaine et al., 2014). In addition, trial-to-trial response variability and dendritic processing are also known to play important roles in enhancing feature selectivity of neuronal output in visual cortex (Anderson et al., 2000; Miller and Troyer, 2002; Carandini, 2007; Finn et al., 2007; Smith et al., 2013).
In recent years, the mouse superior colliculus (SC) has become a productive model for investigating neural processing in the brain (Cang et al., 2018; Ito and Feldheim, 2018). Neurons in its superficial layers, including the stratum griseum superficiale (SGS) and stratum opticum (SO), display diverse visual feature selectivity (Wang et al., 2010; Gale and Murphy, 2014; Inayat et al., 2015). Importantly, even though direction and orientation selectivity are seen in the mouse SC, their underlying circuit and synaptic mechanisms are likely different from those in the visual cortex. Orientation and direction selectivity in cat V1 arise from the precise alignment of non-selective thalamic receptive fields (RFs; Ferster and Miller, 2000; Lampl et al., 2001; Priebe and Ferster, 2005, 2008). A similar feedforward model seems to also underlie orientation selectivity in layer 4 of mouse V1 (Li et al., 2013; Lien and Scanziani, 2013), even though many of the thalamic inputs are already selective (Marshel et al., 2012; Piscopo et al., 2013; Scholl et al., 2013; Zhao et al., 2013a; Sun et al., 2016). In contrast, in the mouse SC, we recently revealed that the direction selectivity (DS) in the SC originates from the direction selective ganglion cells in the retina (Shi et al., 2017). In addition, another prominent characteristic of the SC is that it contains a much higher proportion of inhibitory neurons than in cortex (Mize, 1988, 1992; Endo et al., 2003; Inayat et al., 2015). Together, these important differences between visual cortex and the SC argue for the need to study Vm-to-spike transformation in the mouse SC and how it contributes to feature selectivity.
In this study, we set out to describe the feature selectivity transformation from Vm to spikes in mouse SGS neurons and to reveal underlying contributing factors. With in vivo whole-cell recording, we show that the transformation of direction and orientation selectivity from Vm to spikes is highly variable in the mouse SGS. Interestingly, the degree of this variable transformation is correlated with RF size. Several biophysical factors, including Vm-to-spike-rate relationship and the Vm-rising slope for spike initiation, but not dendritic processing, are correlated with RF size and likely contribute to the observed input-output transformation of feature selectivity. Together, these findings provide useful information for understanding information processing and visual transformation in the SC.
Materials and Methods
Animal Preparation
C57BL/6 wild type (n = 23) and transgenic mice (n = 18) of both sexes between postnatal day 45 and 90 were used in this study. Gad2-IRES-cre (Stock no. 010802) and Ai32 (RCL-ChR2(H134R)/EYFP, Stock no. 012569) mice were acquired from Jackson Laboratory and crossed to generate heterozygous offspring that express ChR2 in glutamate decarboxylase 2 positive (GAD2+) cells for in vivo optogenetic experiments. All mice were kept on a 12 h light:12 h dark cycle, 1–5 mice per cage. All experimental procedures were approved by the Northwestern University Institutional Animal Care and Use Committee.
Mice were anesthetized with urethane (1.2 g/kg in 10% saline solution, i.p.) and then sedated by chlorprothixene (10 mg/kg in water, i.m.) as described before (Zhao et al., 2014; Shi et al., 2017). Atropine (0.3 mg/kg in 10% saline) and dexamethasone (2 mg/kg in 10% saline) were administrated subcutaneously. The animal’s body temperature was monitored through a rectal thermos-probe and maintained at 37°C through a feedback heater control module (Frederick Haer Company, Bowdoinham, ME, USA). Additional urethane (0.2–0.3 g/kg) was administered when necessary according to the depth of anesthesia monitored with toe-pinch reflex test during experiments. After the mice were anesthetized, the scalp was shaved and skin removed to expose the skull. A metal plate was mounted on top of the skull with Metabond (Parkell, Edgewood, NY, USA) mixed with black ink. The plate was then mounted to a steel stand on the vibration isolation table. A thin layer of silicon oil was applied on both eyes to prevent from drying. A craniotomy (~4.0 × 2.0 mm2) was performed on the left hemisphere, and the tissues including the entire V1 overlaying the SC was removed by aspiration to expose the SC.
In Vivo Whole-Cell Recording
Blind whole-cell patch clamp was performed to record SGS neurons intracellularly, following procedures previously described (Shi et al., 2017). K+-based internal solution used in this study contained 135 mM K-gluconate, 7 mM KCl, 0.5 mM EGTA, 10 mM HEPES, 10 mM Na-phosphocreatine, 4 mM Mg-ATP, 0.4 mM Na-GTP and 0.5% biocytin with pH adjusted to 7.25. Glass pipettes were advanced perpendicularly to the horizontal plane of the mouse head until just touching the SC surface. 2% agarose in artificial cerebrospinal fluid solution (ACSF, containing 140 mM NaCl, 2.5 mM KCl, 11 mM Glucose, 20 mM HEPES, 2.5 mM CaCl2, 3 mM MgSO4, 1 mM NaH2PO4) was then added onto the exposed SC. Pipettes were then inserted into the SC.
Electrical signals were amplified using MultiClamp 700B (Axon Instruments, CA, USA), and acquired with System 3 workstation (Tucker Davis Technologies, Alachua, FL, USA) at 10 kHz. Pipette capacitance and the electrode resistance were compensated initially. Only responsive cells with stable resting membrane potentials and series resistances lower than 80 MΩ across the duration of the recordings were included in this study. The depths of recorded cells (reading from the micromanipulator) were between 0 μm and 300 μm from the point where the pipette broke into the thin membrane on the SC.
After recordings, mice were euthanized with 50 mg/kg pentobarbital and perfused with PBS and then 4% paraformaldehyde (PFA). The brain was immersed in 4% PFA overnight. Coronal slices of 150 μm were cut from the fixed brain using a vibrating blade microtome (VT1000S, Leica Microsystems). The labeled cells were revealed by visualizing biocytin with streptavidin-Alex Fluor 488 conjugate (Invitrogen). Images were captured using a Zeiss LSM5 Pascal confocal microscope (Carl Zeiss, Jena, Germany).
Visual Stimulation
Visual stimuli were generated with Matlab Psychophysics toolbox on a CRT monitor (40 cm × 30 cm, 60 Hz, ~35 cd/m2 luminance). The monitor was placed 25 cm away from the right eye (contralateral to the recording site), and slightly adjusted for each cell so that its RF was completely covered. The left eye was covered throughout the experiments. Sweeping white bars on a gray background, 5° wide drifting at a speed of 30°/s, were used in the experiments to determine the direction selectivity or orientation selectivity. The drifting directions were varied between 0° and 330° (12 steps, 30° spacing), which were presented in a pseudorandom sequence together with a “blank stimulus” (gray screen at the mean luminance). The inter-stimulus interval was 0.5 or 1 s. Each stimulus was repeated 5–8 times.
Optogenetic Determination of Cell Types
An optic fiber (0.2 mm core diameter) driven by a blue LED (470 nm, Doric Lenses) was placed ~0.5 mm above the exposed SC to photostimulate ChR2-expressing cells. The intensity of LED light was ~160 mW/mm2 at the tip of the optic fiber in all recordings, which was confirmed to be reliably effective in activating ChR2-expressing neurons (Shi et al., 2017).
Data Analysis
Whole-cell recording data were first analyzed using a custom MATLAB program (Shi et al., 2017). Briefly, spikes were detected when the first derivative of raw voltage traces (dV/dt) reached a manually set positive threshold. Individual traces were carefully inspected to ensure proper spike detection. To precisely determine the start of a spike (spike initiation), the second derivative of raw voltage traces (d2V/dt2) were calculated. The spike initiation time point was the time of the first peak of d2V/dt2 of the spike (Platkiewicz and Brette, 2010). The peri-stimulus spike time histograms (PSTHs) were calculated by trial-averaging the spike counts in each 50 ms time bin. Subthreshold Vm were extracted by removing spikes from the raw voltage traces by a 6 ms median filter. The subthreshold Vm traces were trial-averaged for each stimulus condition. The trial-averaged Vm trace for the blank stimulus (i.e., gray screen) was used to calculate the Vm baseline and the standard deviation of spontaneous Vm fluctuations. The Vm baseline was then subtracted from the trial-averaged Vm trace for each visual stimulus condition.
For analyzing Vm, we determined time windows of responses to the sweeping bars as described in a previous article (Shi et al., 2017). Briefly, we first calculated a cutoff threshold, which was the Vm level 2 standard deviations away from the baseline fluctuation. The widest segment of the traces that were above the threshold was determined as the response time window for each stimulus condition. This time window was expanded if there were any short above-threshold segments within 150 ms of the two sides. Next, the conditions that evoked wider time windows were used to guide the analysis of other conditions, to ensure that the estimation of response window was not too conservative or inaccurate for non-preferred directions. Specifically, for conditions where the window was narrower than 1/3 of the widest window of this cell (or 333 ms if the widest window is bigger than 1 s), the response time window determined from the opposite direction, reversed in timing, was used. All traces were checked visually to confirm that the time windows were determined properly. Peak Vm and spike rate were calculated for the response time window of each stimulus condition, subtracting the mean values of blank condition. The RF size was determined using the widest time window of all the 12 stimulus conditions, i.e., the size of the long axis of the RF. We also calculated the area of RF using a fitting method and observed similar correlations as using the width of the long axis. These analyses were thus not presented in the article.
To quantify the degree of direction selectivity and orientation selectivity, we calculated a global direction selectivity index (gDSI) and a global orientation selectivity index (gOSI). gDSI or gOSI is the vector sum of responses normalized by the scalar sum of responses in 12 directions or six orientations (Mazurek et al., 2014; Inayat et al., 2015; Shi et al., 2017): , , where Rθ is the response magnitude of spikes or Vm, at θ direction of bars. DS transformation index (DSTI) is the difference of gDSI of spike rate and Vm: DSTI = gDSI-Spike − gDSI-Vm. OS transformation index (OSTI) is the difference of gOSI of spike rate and Vm: OSTI = gOSI-Spike − gOSI-Vm.
To quantify the relationship between Vm and spike rate, we fitted the data with a power law function (Hansel and van Vreeswijk, 2002; Miller and Troyer, 2002; Priebe et al., 2004): R(Vm) = k(Vm − Vrest)p, where R(Vm) is the average spike rate at Vm, k is a gain factor, Vrest is the resting membrane potential, and p is the exponent of the fitted function, referred to as power-law index in this study.
Statistics
All pooled data were presented as mean ± SEM unless otherwise stated. Statistical significance was calculated using two-sided student’s t test or non-parametric, two-sided, Kolmogorov-Smirnov (K-S) test as mentioned in the text. All analyses and graph plotting were performed in MATLAB (MathWorks) or Prism (GraphPad Software Inc). No statistical methods were used to predetermine sample sizes, but our sample sizes are similar to those reported in the field.
Results
Feature Selectivity Transformation From Vm to Spike Rate in SGS Neurons
To study how membrane potential (Vm)-to-spike transformation in SGS neurons impact their feature selectivity, we carried out in vivo intracellular whole-cell recording in urethane-anesthetized mice. We recorded SGS neurons under current clamp to reveal their spiking and the underlying Vm changes in response to sweeping bars in different directions (Figures 1A–D). As described in previous studies (Wang et al., 2010; Inayat et al., 2015), SGS neurons displayed diverse feature selectivity. To moving bars, some cells showed orientation selectivity (OS; Figure 1C), and others showed DS (Figure 1D). We separately analyzed the recorded cells’ Vm and spike responses and examined their tuning properties (see “Materials and Methods” section for details; e.g., Figures 1E–H). Using the normalized vector sum as an index (see “Materials and Methods” section for details), which we refer to as gDSI and gOSI, we quantified the degree of DS and OS of spiking and Vm responses, respectively, of the recorded SGS neurons. As expected from the thresholding effect (Carandini and Ferster, 2000), the gDSI or gOSI of spiking responses were greater than those of Vm responses for individual cells (e.g., Figures 1G,H) and across the population (Figures 2A–D).
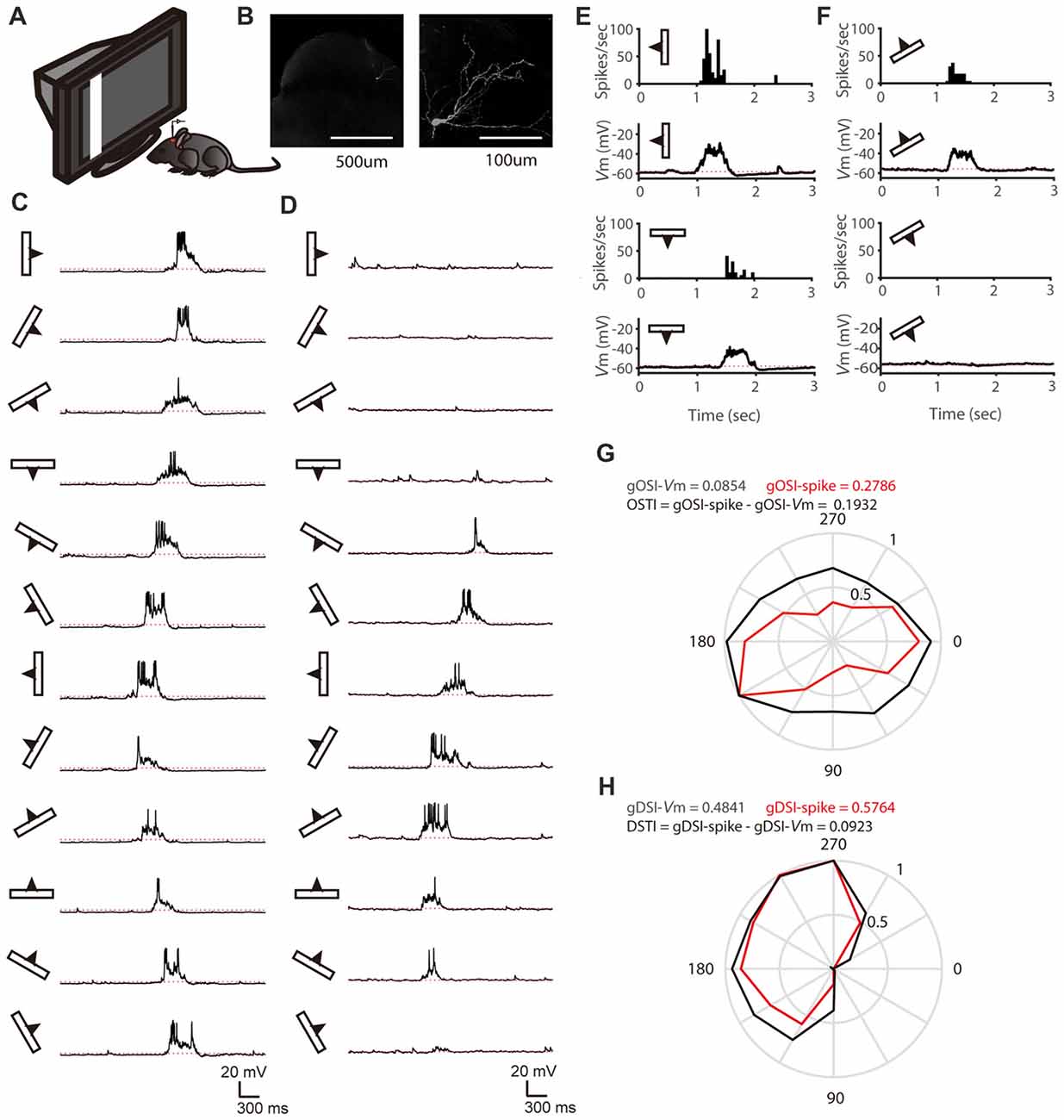
Figure 1. In vivo whole-cell recording of stratum griseum superficiale (SGS) neurons. (A) A schematic of the experimental setup. (B) Morphology of an example SGS neuron with low (left) and high (right) magnification. (C,D) Membrane potential (Vm) traces of an orientation selectivity (OS) cell (C) and a direction selectivity (DS; D) cell in response to sweeping bars. The movement direction is diagramed by the bar and arrow to the left of each trace. Action potentials are truncated at −10 mV to better reveal visually-evoked Vm responses. The red dotted lines indicate the resting membrane potential of −58 mV in the OS cell and −56 mV in the DS cell. (E) The mean spike and Vm responses to bars moving along its preferred and opposite orientations of the same cell in (C). Peri-stimulus spike time histograms (top) and trial-averaged Vm (bottom) are shown. The red dotted lines indicate the resting Vm. (F) The mean spike and Vm responses to bars moving along its preferred and opposite directions of the same cell in (D). (G) Orientation tuning for peak Vm (black) and spike rate (red) of the same cell in (C). (H) Direction tuning for peak Vm (black) and spike rate (red) of the same cell in (D).
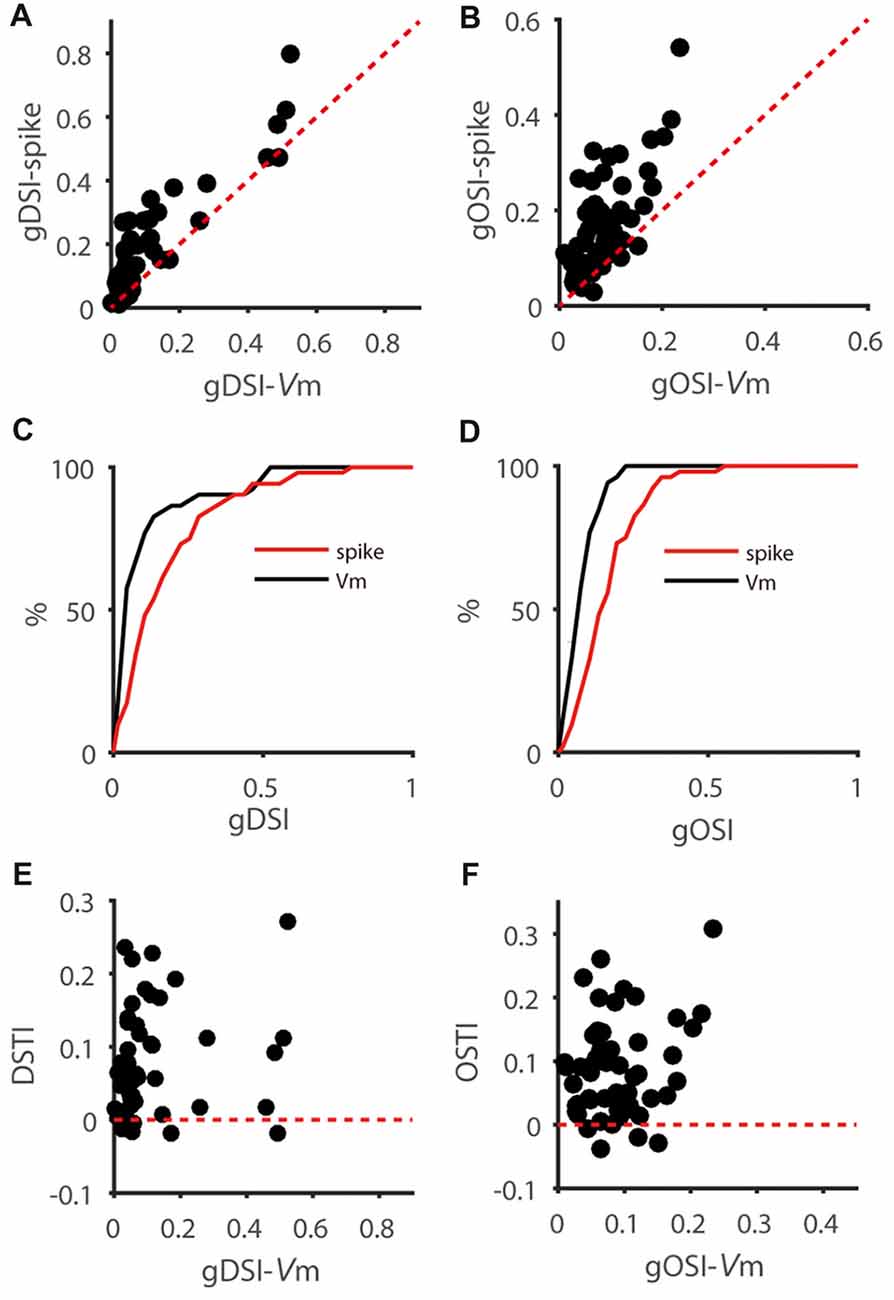
Figure 2. Feature selectivity transformation from Vm to spikes is highly variable in SGS neurons. (A) Scatter plot of global direction selectivity index (gDSI)-Spike vs. gDSI-Vm (n = 52 cells from 41 mice). The red dotted line is the line of unity. Note that this plot is adapted from Figure 1F of Shi et al., 2017. (B) Scatter plot of global orientation selectivity index (gOSI)-Spike vs. gOSI-Vm of the same neurons. The red dotted line is the line of unity. (C,D) Cumulative distributions of gDSI (C) and gOSI (D) for Vm and spike rate, indicating that spike rate has higher gDSI and gOSI values than Vm (P < 0.0001 for gDSI and <0.0001 for gOSI, K-S statistic = 0.46 for gDSI and 0.5 for gOSI, Kolmogorov-Smirnov test; n = 52 cells from 41 mice). (E) Scatter plot of gDSI for Vm vs. DS transformation index (DSTI). The red dotted line is the line of zero. Note that the DSTI is highly variable for different degree of gDSI. (F) Scatter plot of gOSI for Vm vs. OS transformation index (OSTI). The red dotted line is the line of zero.
We then quantified the transformation of direction/orientation selectivity from Vm to spiking responses by calculating a DS transformation index (DSTI = gDSI-Spike − gDSI-Vm) and an OS transformation index (OSTI = gOSI-Spike − gOSI-Vm). Most of OSTI and DSTI were positive, reflecting the sharpening of OS and DS from SGS neurons’ input (Vm) to output (spikes). Furthermore, the degree of this sharpening was highly variable among the recorded cells, with OSTI ranging from −0.04 to 0.31, and DSTI from −0.02 to 0.27, respectively. No correlation between DSTI and gDSI-Vm or OSTI and gOSI-Vm were found in the recorded cells (Figures 2E,F), suggesting that the feature selectivity of input itself does not determine the degree of its transformation from input to output.
Receptive Field Size Constrains Vm-to-Spike Transformation of Feature Selectivity
We next asked whether other characteristic of the input (as reflected by Vm) constrains the feature selectivity transformation from Vm to spikes. One possibility is that the RF size of input may have an impact on the DS or OS transformation from Vm to spiking responses, since cells with big or small RF may take different roles in visual processing. To explore this possibility, we measured the RF size of Vm of each recorded neuron (see “Materials and Methods” section), which is larger than that of spiking responses and reflect the full range of visual field to which the recorded neurons respond, including subthreshold responses. Neither gDSI or gOSI itself was significantly correlated with RF size (gDSI-Spike, r = −0.26, P = 0.07; gDSI-Vm, r = −0.10, P = 0.48; gOSI-Spike, r = −0.25, P = 0.07; gOSI-Vm, r = 0.04, P = 0.76), but the increase of gDSI and gOSI (i.e., the “transformation index”, DSTI and OSTI) were negatively correlated with the RF size in the recorded cells (DSTI: r = −0.40, P < 0.01, Figure 3A; OSTI: r = −0.38, P < 0.01, Figure 3B; n = 52 cells from 41 mice). Furthermore, using transgenic mice that expressed Channelrhodopsin-2 (ChR2) in GABAergic inhibitory neurons, we were able to identify whether the recorded cells were excitatory or inhibitory by their responses to LED photoactivation (Shi et al., 2017). The correlation between RF size and the transformation index was seen for both cell types and for both DSTI (r = −0.74, P < 0.05 for excitatory cells, n = 9 cells from 8 mice, Figure 3C; r = −0.84, P < 0.01 for inhibitory cells, n = 10 cells from 10 mice, Figure 3E) and OSTI (r = −0.87, P < 0.01 for excitatory cells, Figure 3D; r = −0.72, P < 0.05 for inhibitory cells, Figure 3F).
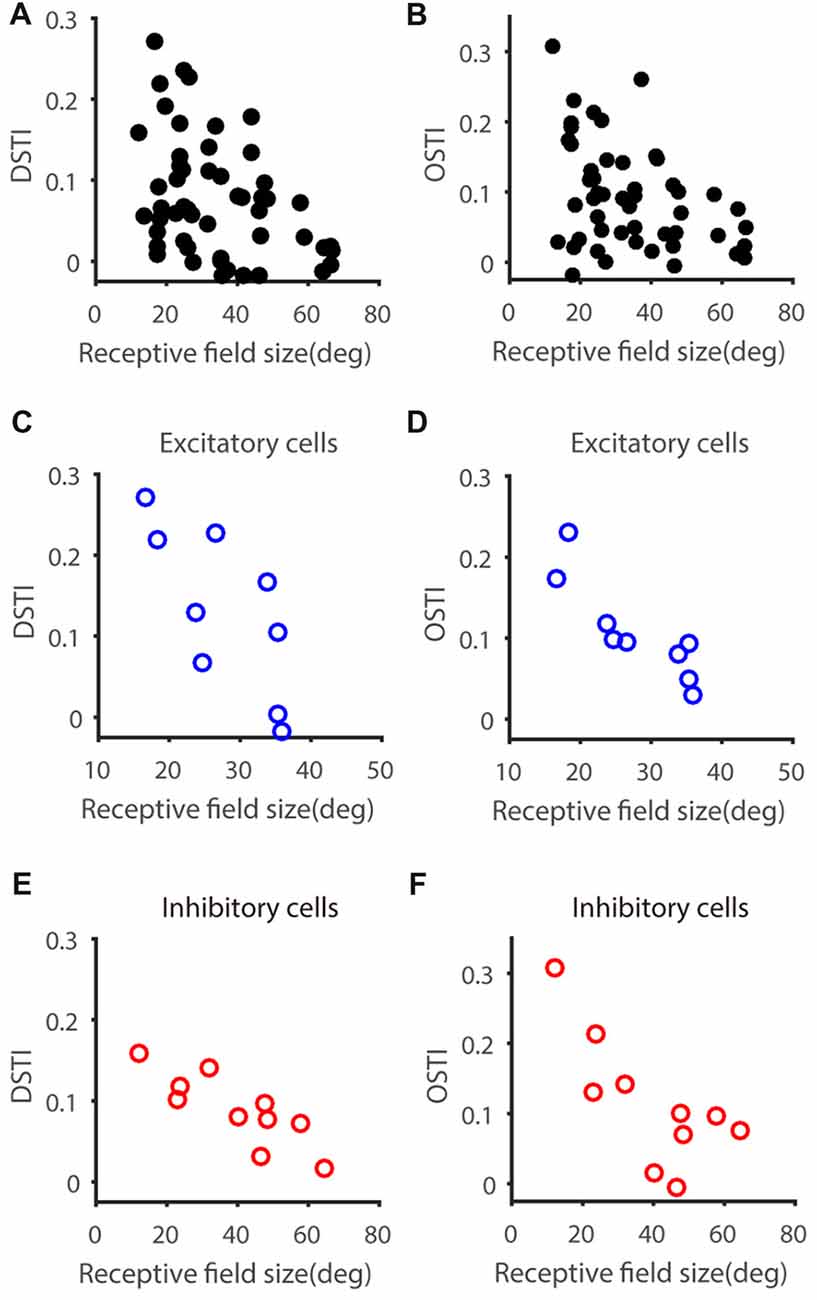
Figure 3. Relationship between feature transformation and receptive field (RF) size. (A,B) Scatter plot of RF size vs. Vm-to-spike-rate DSTI (A) and OSTI (B), indicating significant correlation (n = 52 cells from 41 mice, r = −0.40, P < 0.01 for panel A, r = −0.37, P < 0.01 for panel B). (C,D) Scatter plot of RF size vs. DSTI (C) and OSTI (D) of identified excitatory cells (n = 9; r = −0.74, P < 0.05 for panel C, r = −0.87, P < 0.01 for panel D). (E,F) Scatter plot of RF size vs. DSTI (E) and OSTI (F) of identified inhibitory cells (n = 10; r = −0.84, P < 0.01 for panel E, r = −0.73, P < 0.05 for panel F).
Vm-Spike Relationship in SGS Neurons
We next sought insights on what biophysical properties might contribute to the observed correlation between feature selectivity transformation and RF size. Previous studies in visual cortex have shown that Vm-spike transformation follows a power-law nonlinearity (Priebe and Ferster, 2008) and neurons with higher power-law index tended to have a greater OSI increase from Vm to spike rate than neurons with lower index (Tan et al., 2011). We thus examined this factor by fitting power-law functions to the data of spike rate vs. Vm (see “Materials and Methods” section). As in previous studies, we used the exponent of the fitted function, referred to as power-law index (Priebe and Ferster, 2008; Tan et al., 2011), to quantify the Vm-spike transformation (Figures 4A,B). No significant difference in the power law index was seen between excitatory (3.38 ± 0.33, n = 9) and inhibitory cells (3.01 ± 0.41, n = 10; t = 0.70; P = 0.49). Interestingly, the power-law index was indeed negatively correlated with the RF size (Figure 4C, r = −0.45, P < 0.001), where cells with larger RFs tended to have smaller exponents (e.g., Figure 4A) and smaller RFs larger exponents (e.g., Figure 4B). Consequently, both DSTI (Figure 4D, r = 0.38, P < 0.01) and OSTI (Figure 4E, r = 0.61, P < 0.0001) were positively correlated with the power-law index.
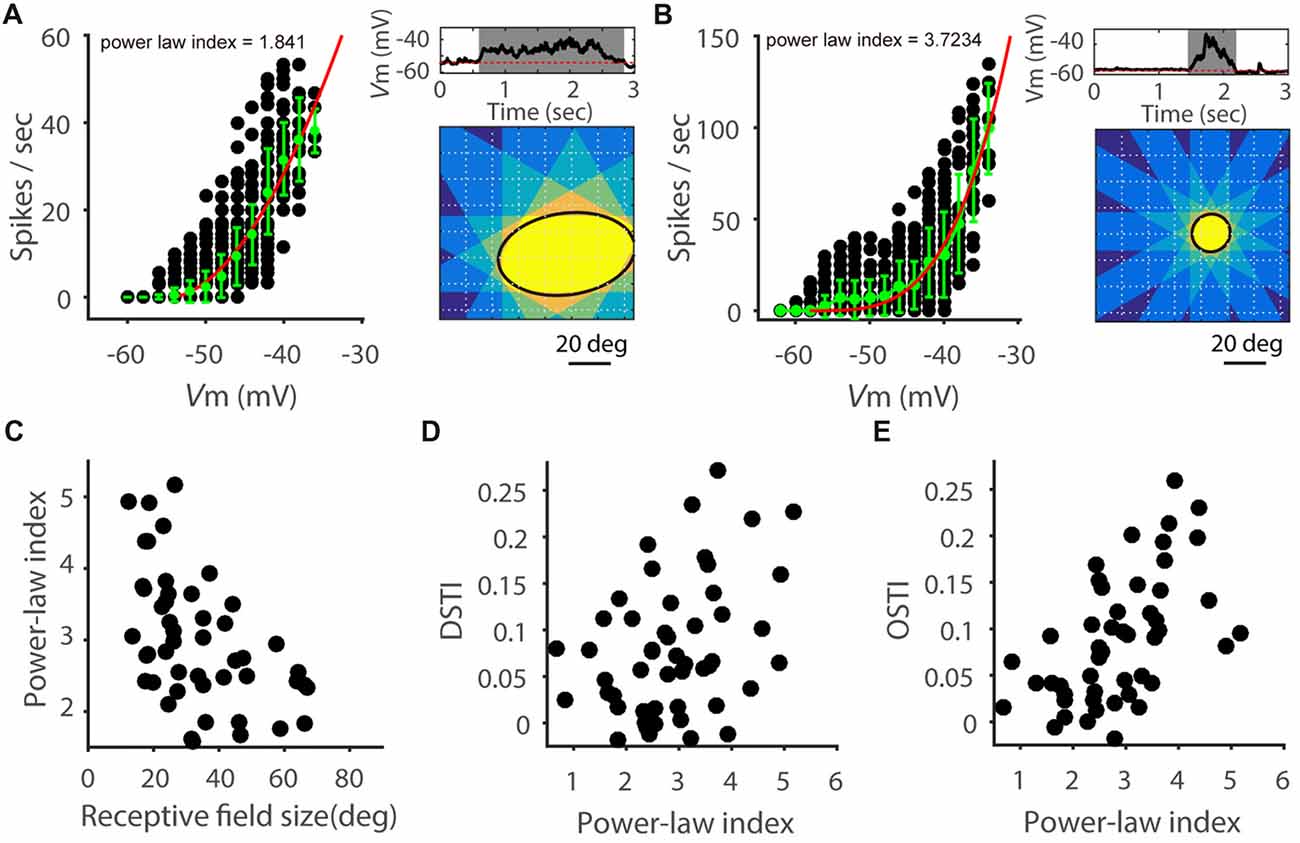
Figure 4. Power-law relationship of Vm-to-spike transformation. (A,B) Relationship between Vm and spike rate in two example SGS neurons. On the left of each panel, each black point represents the spike rate and corresponding Vm response (grouped in 2 mV intervals) for one 50 ms epoch of a trial-averaged response. Green points and error bars are means and STDs of all the black points in the corresponding Vm range. Red curve is a fit of the green points to a power-law function. Right up: trial-averaged Vm response with the widest time window to the sweeping bar. Gray shadow indicates the response time window. Red dotted line indicates the resting Vm. Right bottom: ellipse fitted RF (black solid line) measured with superimposed time windows of responses to 12 directions of moving bars. Note that the power-law index of the cell in panel (A) is smaller than that of (B), and its RF size is larger than that of the cell in panel (B). (C) Scatter plot of power-law index vs. RF size, indicating negative correlation (n = 52 cells from 41 mice, r = −0.45, P < 0.001). (D) Scatter plot of DSTI vs. power law index (r = 0.38, P < 0.01). (E) Scatter plot of OSTI vs. power-law index (r = 0.62, P < 0.0001).
In performing this analysis, we noted that not all Vm-spike relationship could be best fitted by the power-law nonlinearity, possibly due to sublinear effects at more depolarized Vm (Zhao et al., 2013b). We thus carried out additional analysis and separated the recorded cells into “superlinear” and “sublinear” cells based on their behavior at the most depolarized membrane potentials. Specifically, we calculated spike rate change in the two most depolarized Vm ranges (shown as red arrows in Figures 5A,B). Cells with increasing rate change with Vm were considered “superlinear cells” (e.g., Figure 5A) and cells with decreasing rate changes were “sublinear cells” (e.g., Figure 5B). Overall, the “superlinear cells” had smaller RFs than the “sublinear cells” (Figure 5C). The above analyses thus suggest that the Vm-spike relationship in SGS cells is correlated with their RF size, and likely contributes to the RF-dependent feature selectivity transformation.
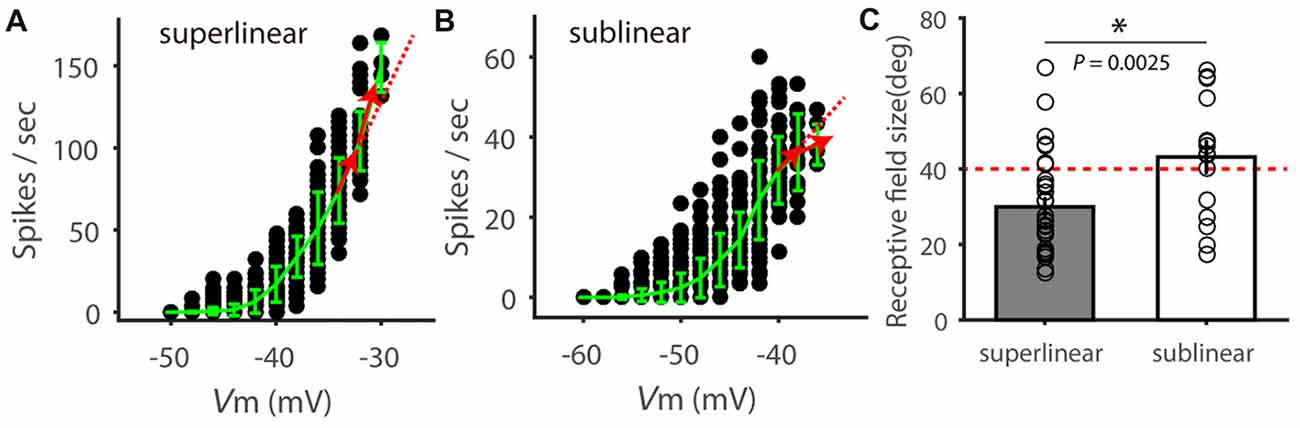
Figure 5. Superlinear and sublinear Vm-spike transformation in SGS neurons. (A) Relationship between Vm and spike rate in an example SGS neuron. Red arrows show the instant change of spike rate with Vm increasing, which is higher than that at lower Vm (dotted red line), indicating superlinear change of spike rate with Vm. (B) Relationship between Vm and spike rate in another example SGS neuron, indicating sublinear change of spike rate at the more depolarized Vm. (C) Comparison of RF size between SGS neurons with superlinear and sublinear Vm-spike-transformation. There is significant difference between these two groups (t = 3.18, P < 0.01). Note that most “superlinear” cells (80.0%, 28/35) had RF size smaller than 40 degrees (red dotted line), and most “sublinear” cells (64.7%, 11/17) had RF size larger than 40 degrees. Error bars represent STD in (A,B) and SEM in (C). * represents P < 0.01.
Spike Initiation in SGS Neurons
The spike threshold is highly variable in vivo (Azouz and Gray, 2000; Fontaine et al., 2014), which could be stimulus dependent and affect feature selectivity in cortical neurons (Wilent and Contreras, 2005). Moreover, spike initiation not only depends on the absolute Vm level but also on other features such as the rate of Vm change (Wester and Contreras, 2013). We thus calculated the slope of the Vm during the 1 ms window before each spike (Figure 6A) for individual neurons. For each cell, we plotted the distribution of this “pre-spike slope” (Figure 6B) and saw no obvious segregation of these distributions according to RF size. Similarly, distributions of spike initiating Vm also showed no difference between the two groups (Figure 6C). We then averaged the pre-spike slopes of all the spikes for each cell. Again, no correlation was observed between the average pre-spike slope and RF size; and the pre-spike slope in the cells with small RF was not different from that of the cells with large RF (Figure 6D). Accordingly, the DS and OS transformation index (DSTI and OSTI) did not show correlation with pre-spike slope in the recorded SGS cells (Figures 6E,F). Because small pre-spike slope is often associated with dendritic spikes which has been found in wide-field SGS cells (Gale and Murphy, 2016), our results suggest that it is unlikely that dendritic processing has much effect on feature selectivity transformation from Vm to spikes in mouse SGS neurons.
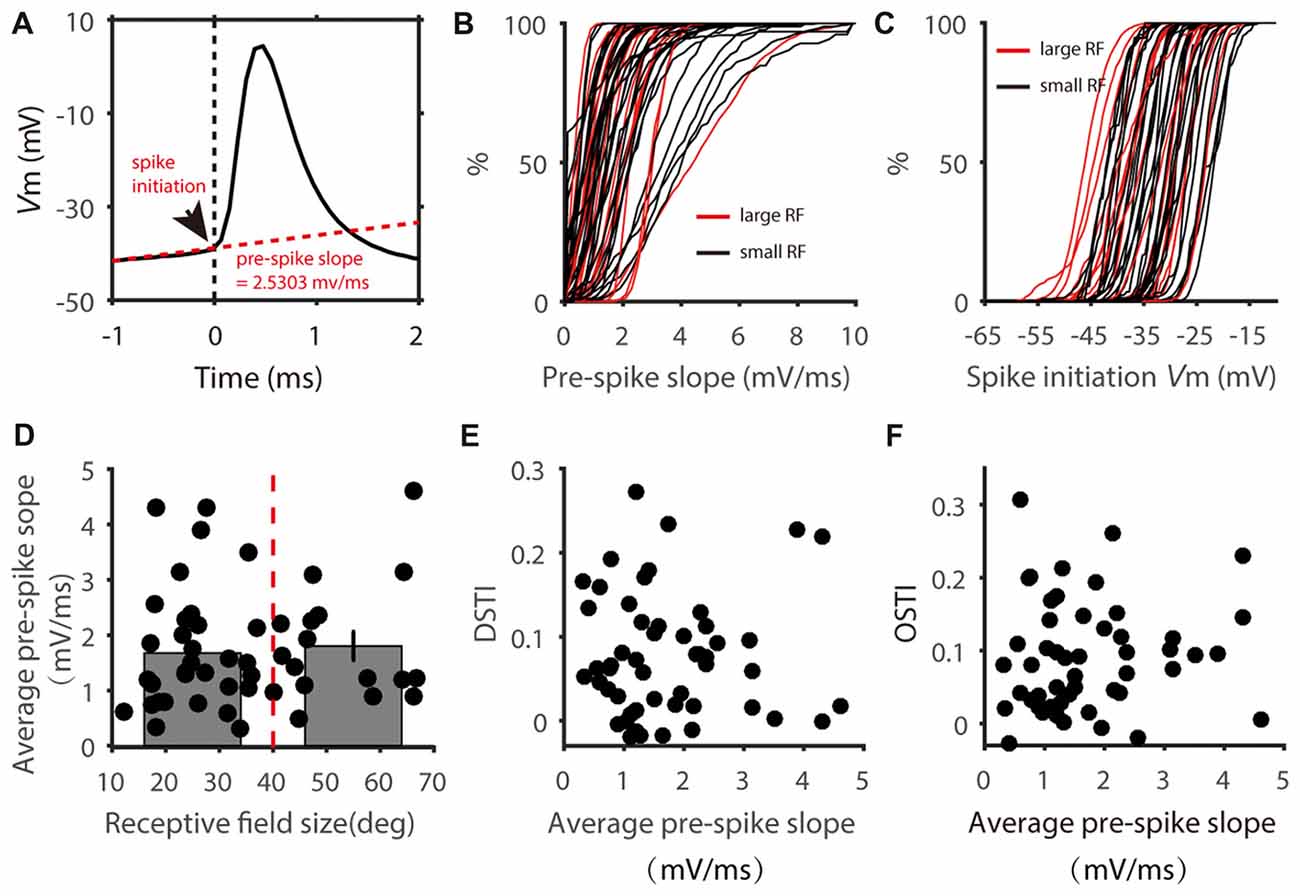
Figure 6. Distribution of pre-spike Vm slopes. (A) Calculation of pre-spike Vm slope. Arrow head shows the point of spike initiation. The slope of the red dotted line shows the mean pre-spike Vm slope during the 1 ms window before spike onset. (B) Cumulative distributions of pre-spike Vm slope of cells with small (black) and large (red) RFs. Each line represent all spikes from one cell. (C) Cumulative distributions of spike initiating Vm of the two groups of cells. (D) Scatter plot of average pre-spike slope vs. RF size. The average pre-spike slopes of two groups divided at 40 degrees of RF size (red dotted line) showed no significant difference (t = 0.37, P = 0.71). (E,F) Scatter plot of DSTI (E) and OSTI (F) vs. average pre-spike slope.
Finally, we analyzed the relationship between pre-spike slope and spike-initiating Vm for each cell. In some cells, a negative correlation was seen between the pre-spike slope and Vm threshold (e.g., Figure 7A), indicating a faster Vm rise is needed for spike initiation at more hyperpolarized Vm. In other cells, their relationship is rather flat (e.g., Figure 7B). To quantify this relationship, we compared the average pre-spike slopes between the 0%–25% and 75%–100% of the observed Vm range for spike initiation (red circles in Figures 7A,B), and normalized their difference by the corresponding Vm difference. Notably, this “slope-changing rate” was well correlated with the RF size (r = 0.60, P < 0.0001, Figure 7C). Most cells with smaller RFs had negative values (Figure 7A for an example cell, Figures 7C,D for the population), while cells with larger RFs had values around zero (Figure 7B for an example cell, Figures 7C,D for the population; t = 4.35, P < 0.0001 for comparison between the two groups). In other words, for cells with smaller RFs, their spike initiation would become “easier” at more depolarized Vm because smaller Vm rise rate is needed, which would then contribute to their superlinear behavior in Vm-spike transformation (Figure 5C) and higher power-law index than bigger RF neurons (Figures 7E,F). Overall, these results suggest that RF-size-dependent spike initiation is another factor contributing to feature selectivity transformation from Vm to spikes.
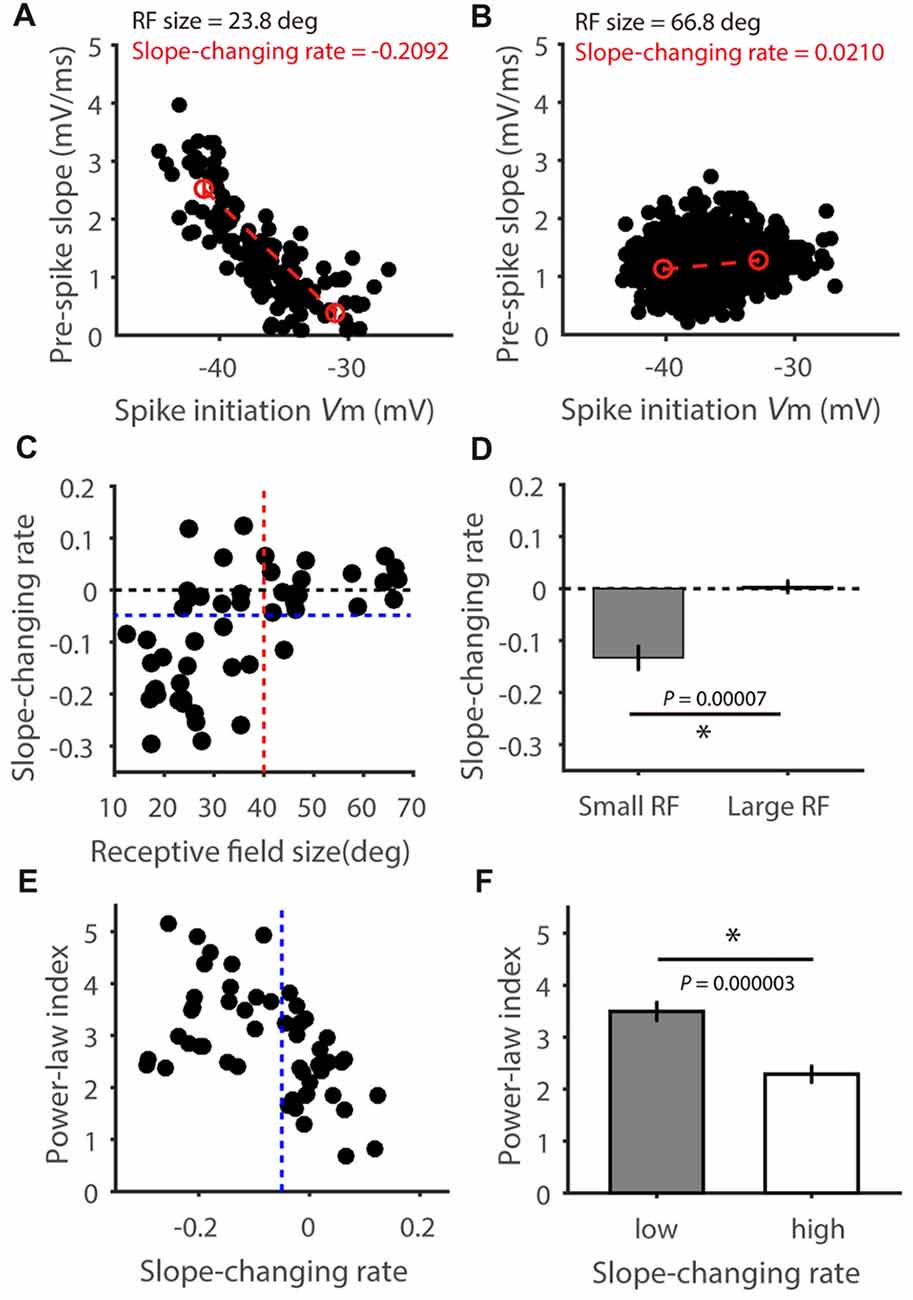
Figure 7. The relationship between pre-spike Vm slope and spike-initiating Vm. (A,B) Relationship between pre-spike Vm slope and spike initiating Vm level in two example cells, one with small RF (A) and the other large (B). Red circles indicate average pre-spike slopes for all spikes in the 0%–25% and 75%–100% spike initiating Vm range. Red dotted lines indicate the pre-spike slope changing rates. (C) Scatter plot of the “slope-changing rate” vs. RF size, indicating positive correlation between them (r = 0.60, P < 0.0001). Note that most of the cells (31/34, 91.2%) with RF smaller than 40 degrees (red dotted line) had values smaller than zero, and most of the cells with larger RFs (17/18, 94.4%) had values around zero and larger than −0.5 (blue dotted line). (D) The “slope-changing rate” is significantly different between the two groups of cells (t = 4.35, P < 0.0001). (E) Scatter plot of power-law index vs. slope changing rate, indicating negative correlation between them (r = 0.49 P = 0.0002), and (F) the means were significantly different between cells with high and low changing rate (t = 5.29, P < 0.0001). Error bars represent SEM. * represents P < 0.0001.
Discussion
Feature selectivity is a fundamental property that allows neurons in the sensory systems to extract and encode external information. The circuit and synaptic mechanisms underlying feature selectivity have been extensively studied in the visual system, where neurons are tuned to specific stimulus orientation or moving direction. These studies have revealed precise neural circuits that give rise to orientation and direction selectivity in different visual structures. For example, direction selective ganglion cells (DSGCs) in the retina acquire their selectivity from the intricate spatial and temporal interactions between synaptic excitation and inhibition (Wei and Feller, 2011; Vaney et al., 2012). Similarly, orientation selectivity in the visual cortex arises from the excitatory thalamic inputs that are precisely aligned in their spatial RFs (Ferster and Miller, 2000; Priebe and Ferster, 2008; Priebe, 2016). On the other hand, we have recently discovered that SGS neurons in the SC inherit their direction selectivity from the retina by precisely converging inputs of similarly-tuned DSGCs (Shi et al., 2017).
In addition to the precise circuit connectivity, the transformation from synaptic input to spike output is another key mechanism in processing feature selectivity. Here, we performed in vivo whole cell recording of mouse SGS neurons to study factors important for orientation and direction selectivity transformation from Vm to spikes. We found that the selectivity increased from the Vm to spike responses, and the degree of this increase was highly variable among different neurons. Interestingly, the variability was not random, but correlated with the size of the neurons’ RFs. Our subsequent analyses indicate that the power-law relationship between Vm and spike rate and the relationship between Vm dynamics and spike initiation, but not dendritic processing, are RF size dependent and likely contribute to the observed input-output transformation of feature selectivity.
The power-law index, i.e., the exponent of the fitted power-law function, has been used to estimate the degree of Vm-to-spike transformation in previous studies (Finn et al., 2007; Priebe and Ferster, 2008, 2012; Tan et al., 2011). Similar as in visual cortex (Tan et al., 2011), our studies showed that the power-law index is positively correlated with the level of increase of feature selectivity from Vm to spikes. In other words, the higher the index, the greater degree of the Vm-to-spike sharpening of orientation/direction selectivity will be. Previous studies in cat V1 demonstrated that the power-law relationship between Vm and spike rate could be explained by trial-to-trial response variability (Hansel and van Vreeswijk, 2002; Miller and Troyer, 2002; Carandini, 2004; Priebe et al., 2004). Our finding that the power-law index is correlated with RF size, which would not affect response variability, suggests that additional mechanisms may be also involved. One possible factor is the relationship between spike initiating Vm and the required depolarizing rate, which we found to be correlated with RF size in mouse SGS neurons. Cells with smaller RFs display a negative correlation, where spike initiation becomes “easier” at more depolarized Vm because smaller Vm rise rate is needed. These cells would likely show higher power-law index and superlinear behavior in Vm-spike transformation. In contrast, cells with larger RFs require similar depolarization rate for spike initiation at different Vm, thus displaying smaller power-law index than smaller RF cells.
What causes the differences in the relationship between Vm and spike rate and between Vm dynamics and spike initiation among cells with different RF sizes is still unknown. One possibility is that cells with different RFs may represent distinct cell types. We were not able to distinguish the recorded cells into subtypes because of the limited dataset in reconstructed morphology. But it was shown that narrow field (NF) vertical cells, wide field (WF) vertical and stellate cells are the three types of excitatory cells and they show different RF size (Gale and Murphy, 2014). It is possible that these cells have different biophysical features in their membrane properties that underlie their difference in Vm-to-spike transformation. On the other hand, horizontal cells represent possibly the only inhibitory cell type in the mouse SGS (Gale and Murphy, 2014), and our optogenetic experiments demonstrate that they also display RF-size-dependent Vm-to-spike transformation. This result suggests that additional factors within a cell type could contribute to the observed difference as well.
Finally, the RF-dependent differences in Vm-to-spike transformation may have functional implications. For small visual objects such as a prey that is best responded to by cells with small RFs, a higher input-output transformation could augment the saliency of the objects. For large visual stimuli such as a moving background, a lower transformation could maintain the wider tuning of the input, potentially helping keep the fidelity of the signal. These speculations may be testable one day under more natural conditions with ethologically-relevant visual stimulation. Our current study thus provides the basic information for future studies that will attempt to link sensory input to behavioral output.
Author Contributions
XS and JC designed the experiments. XS performed in vivo whole-cell recording experiments and analyzed the data. YJ performed histology. JC guided data analysis and oversaw the project. All authors discussed the results and wrote the manuscript.
Conflict of Interest Statement
The authors declare that the research was conducted in the absence of any commercial or financial relationships that could be construed as a potential conflict of interest.
Acknowledgments
We thank Xinyu Zhao and Hui Chen for their help with data analysis. This research was supported by US National Institutes of Health (NIH) grants (R01EY026286 and R01EY020950 to JC), National Natural Science Foundation of China (NSFC) grant (81371049 and 81770956 to XS), and Tianjin Science Fund for Distinguished Young Scholars (17JCJQJC46000 to XS).
References
Anderson, J. S., Lampl, I., Gillespie, D. C., and Ferster, D. (2000). The contribution of noise to contrast invariance of orientation tuning in cat visual cortex. Science 290, 1968–1972. doi: 10.1126/science.290.5498.1968
Azouz, R., and Gray, C. M. (2000). Dynamic spike threshold reveals a mechanism for synaptic coincidence detection in cortical neurons in vivo. Proc. Natl. Acad. Sci. U S A 97, 8110–8115. doi: 10.1073/pnas.130200797
Cang, J., Savier, E., Barchini, J., and Liu, X. (2018). Visual Function, Organization, and Development of the Mouse Superior Colliculus. Annu. Rev. Vis. Sci. doi: 10.1146/annurev-vision-091517-034142 [Epub ahead of print].
Carandini, M. (2004). Amplification of trial-to-trial response variability by neurons in visual cortex. PLoS Biol. 2:E264. doi: 10.1371/journal.pbio.0020264
Carandini, M. (2007). Melting the iceberg: contrast invariance in visual cortex. Neuron 54, 11–13. doi: 10.1016/j.neuron.2007.03.019
Carandini, M., and Ferster, D. (2000). Membrane potential and firing rate in cat primary visual cortex. J. Neurosci. 20, 470–484. doi: 10.1523/JNEUROSCI.20-01-00470.2000
Endo, T., Yanagawa, Y., Obata, K., and Isa, T. (2003). Characteristics of GABAergic neurons in the superficial superior colliculus in mice. Neurosci. Lett. 346, 81–84. doi: 10.1016/s0304-3940(03)00570-6
Ferster, D., and Miller, K. D. (2000). Neural mechanisms of orientation selectivity in the visual cortex. Annu. Rev. Neurosci. 23, 441–471. doi: 10.1146/annurev.neuro.23.1.441
Finn, I. M., Priebe, N. J., and Ferster, D. (2007). The emergence of contrast-invariant orientation tuning in simple cells of cat visual cortex. Neuron 54, 137–152. doi: 10.1016/j.neuron.2007.02.029
Fontaine, B., Peña, J. L., and Brette, R. (2014). Spike-threshold adaptation predicted by membrane potential dynamics in vivo. PLoS Comput. Biol. 10:e1003560. doi: 10.1371/journal.pcbi.1003560
Gale, S. D., and Murphy, G. J. (2014). Distinct representation and distribution of visual information by specific cell types in mouse superficial superior colliculus. J. Neurosci. 34, 13458–13471. doi: 10.1523/JNEUROSCI.2768-14.2014
Gale, S. D., and Murphy, G. J. (2016). Active dendritic properties and local inhibitory input enable selectivity for object motion in mouse superior colliculus neurons. J. Neurosci. 36, 9111–9123. doi: 10.1523/JNEUROSCI.0645-16.2016
Hansel, D., and van Vreeswijk, C. (2002). How noise contributes to contrast invariance of orientation tuning in cat visual cortex. J. Neurosci. 22, 5118–5128. doi: 10.1523/JNEUROSCI.22-12-05118.2002
Hubel, D. H., and Wiesel, T. N. (1959). Receptive fields of single neurones in the cat’s striate cortex. J. Physiol. 148, 574–591. doi: 10.1113/jphysiol.1959.sp006308
Hubel, D. H., and Wiesel, T. N. (1962). Receptive fields, binocular interaction and functional architecture in the cat’s visual cortex. J. Physiol. 160, 106–154. doi: 10.1113/jphysiol.1962.sp006837
Inayat, S., Barchini, J., Chen, H., Feng, L., Liu, X., and Cang, J. (2015). Neurons in the most superficial lamina of the mouse superior colliculus are highly selective for stimulus direction. J. Neurosci. 35, 7992–8003. doi: 10.1523/JNEUROSCI.0173-15.2015
Ito, S., and Feldheim, D. A. (2018). The mouse superior colliculus: an emerging model for studying circuit formation and function. Front. Neural Circuits 12:10. doi: 10.3389/fncir.2018.00010
Lampl, I., Anderson, J. S., Gillespie, D. C., and Ferster, D. (2001). Prediction of orientation selectivity from receptive field architecture in simple cells of cat visual cortex. Neuron 30, 263–274. doi: 10.1016/s0896-6273(01)00278-1
Li, Y. T., Ibrahim, L. A., Liu, B. H., Zhang, L. I., and Tao, H. W. (2013). Linear transformation of thalamocortical input by intracortical excitation. Nat. Neurosci. 16, 1324–1330. doi: 10.1038/nn.3494
Lien, A. D., and Scanziani, M. (2013). Tuned thalamic excitation is amplified by visual cortical circuits. Nat. Neurosci. 16, 1315–1323. doi: 10.1038/nn.3488
Marshel, J. H., Kaye, A. P., Nauhaus, I., and Callaway, E. M. (2012). Anterior-posterior direction opponency in the superficial mouse lateral geniculate nucleus. Neuron 76, 713–720. doi: 10.1016/j.neuron.2012.09.021
Mazurek, M., Kager, M., and Van Hooser, S. D. (2014). Robust quantification of orientation selectivity and direction selectivity. Front. Neural Circuits 8:92. doi: 10.3389/fncir.2014.00092
Miller, K. D., and Troyer, T. W. (2002). Neural noise can explain expansive, power-law nonlinearities in neural response functions. J. Neurophysiol. 87, 653–659. doi: 10.1152/jn.00425.2001
Mize, R. R. (1988). Immunocytochemical localization of γ-aminobutyric acid (GABA) in the cat superior colliculus. J. Comp. Neurol. 276, 169–187. doi: 10.1002/cne.902760203
Mize, R. R. (1992). The organization of GABAergic neurons in the mammalian superior colliculus. Prog. Brain Res. 90, 219–248. doi: 10.1016/s0079-6123(08)63616-x
Piscopo, D. M., El-Danaf, R. N., Huberman, A. D., and Niell, C. M. (2013). Diverse visual features encoded in mouse lateral geniculate nucleus. J. Neurosci. 33, 4642–4656. doi: 10.1523/JNEUROSCI.5187-12.2013
Platkiewicz, J., and Brette, R. (2010). A threshold equation for action potential initiation. PLoS Comput. Biol. 6:e1000850. doi: 10.1371/journal.pcbi.1000850
Priebe, N. J. (2016). Mechanisms of orientation selectivity in the primary visual cortex. Annu. Rev. Vis. Sci. 2, 85–107. doi: 10.1146/annurev-vision-111815-114456
Priebe, N. J., and Ferster, D. (2005). Direction selectivity of excitation and inhibition in simple cells of the cat primary visual cortex. Neuron 45, 133–145. doi: 10.1016/j.neuron.2004.12.024
Priebe, N. J., and Ferster, D. (2008). Inhibition, spike threshold, and stimulus selectivity in primary visual cortex. Neuron 57, 482–497. doi: 10.1016/j.neuron.2008.02.005
Priebe, N. J., and Ferster, D. (2012). Mechanisms of neuronal computation in mammalian visual cortex. Neuron 75, 194–208. doi: 10.1016/j.neuron.2012.06.011
Priebe, N. J., Mechler, F., Carandini, M., and Ferster, D. (2004). The contribution of spike threshold to the dichotomy of cortical simple and complex cells. Nat. Neurosci. 7, 1113–1122. doi: 10.1038/nn1310
Scholl, B., Tan, A. Y., Corey, J., and Priebe, N. J. (2013). Emergence of orientation selectivity in the Mammalian visual pathway. J. Neurosci. 33, 10616–10624. doi: 10.1523/JNEUROSCI.0404-13.2013
Shi, X., Barchini, J., Ledesma, H. A., Koren, D., Jin, Y., Liu, X., et al. (2017). Retinal origin of direction selectivity in the superior colliculus. Nat. Neurosci. 20, 550–558. doi: 10.1038/nn.4498
Smith, S. L., Smith, I. T., Branco, T., and Häusser, M. (2013). Dendritic spikes enhance stimulus selectivity in cortical neurons in vivo. Nature 503, 115–120. doi: 10.1038/nature12600
Sun, W., Tan, Z., Mensh, B. D., and Ji, N. (2016). Thalamus provides layer 4 of primary visual cortex with orientation- and direction-tuned inputs. Nat. Neurosci. 19, 308–315. doi: 10.1038/nn.4196
Tan, A. Y., Brown, B. D., Scholl, B., Mohanty, D., and Priebe, N. J. (2011). Orientation selectivity of synaptic input to neurons in mouse and cat primary visual cortex. J. Neurosci. 31, 12339–12350. doi: 10.1523/JNEUROSCI.2039-11.2011
Vaney, D. I., Sivyer, B., and Taylor, W. R. (2012). Direction selectivity in the retina: symmetry and asymmetry in structure and function. Nat. Rev. Neurosci. 13, 194–208. doi: 10.1038/nrn3165
Wang, L., Sarnaik, R., Rangarajan, K., Liu, X., and Cang, J. (2010). Visual receptive field properties of neurons in the superficial superior colliculus of the mouse. J. Neurosci. 30, 16573–16584. doi: 10.1523/JNEUROSCI.3305-10.2010
Wei, W., and Feller, M. B. (2011). Organization and development of direction-selective circuits in the retina. Trends Neurosci. 34, 638–645. doi: 10.1016/j.tins.2011.08.002
Wester, J. C., and Contreras, D. (2013). Biophysical mechanism of spike threshold dependence on the rate of rise of the membrane potential by sodium channel inactivation or subthreshold axonal potassium current. J. Comput. Neurosci. 35, 1–17. doi: 10.1007/s10827-012-0436-2
Wilent, W. B., and Contreras, D. (2005). Stimulus-dependent changes in spike threshold enhance feature selectivity in rat barrel cortex neurons. J. Neurosci. 25, 2983–2991. doi: 10.1523/JNEUROSCI.4906-04.2005
Zhao, X., Chen, H., Liu, X., and Cang, J. (2013a). Orientation-selective responses in the mouse lateral geniculate nucleus. J. Neurosci. 33, 12751–12763. doi: 10.1523/JNEUROSCI.0095-13.2013
Zhao, X., Liu, M., and Cang, J. (2013b). Sublinear binocular integration preserves orientation selectivity in mouse visual cortex. Nat. Commun. 4:2088. doi: 10.1038/ncomms3088
Keywords: superior colliculus, feature selectivity, receptive field, membrane potential, spike rate
Citation: Shi X, Jin Y and Cang J (2018) Transformation of Feature Selectivity From Membrane Potential to Spikes in the Mouse Superior Colliculus. Front. Cell. Neurosci. 12:163. doi: 10.3389/fncel.2018.00163
Received: 23 April 2018; Accepted: 25 May 2018;
Published: 19 June 2018.
Edited by:
Karl Farrow, Neuroelectronics Research Flanders, BelgiumReviewed by:
J. Alexander Heimel, Netherlands Institute for Neuroscience (KNAW), NetherlandsMartin Meyer, King’s College London, United Kingdom
Copyright © 2018 Shi, Jin and Cang. This is an open-access article distributed under the terms of the Creative Commons Attribution License (CC BY). The use, distribution or reproduction in other forums is permitted, provided the original author(s) and the copyright owner are credited and that the original publication in this journal is cited, in accordance with accepted academic practice. No use, distribution or reproduction is permitted which does not comply with these terms.
*Correspondence: Jianhua Cang, Y2FuZ0B2aXJnaW5pYS5lZHU=