- 1 RIKEN Brain Science Institute, Wako, Japan
- 2 Research Fellow of the Japan Society for the Promotion of Science, Tokyo, Japan
- 3 Institute of Neuroscience and Medicine (INM-6), Computational and Systems Neuroscience, Research Center Juelich, Juelich, Germany
- 4 Brain and Neural Systems Team, RIKEN Computational Science Research Program, Wako, Japan
- 5 Faculty of Biology III, Albert-Ludwigs-University Freiburg, Freiburg, Germany
- 6 Core Research of Evolutional Science and Technology, Japan Science and Technology Agency, Kawaguchi, Japan
A vast amount of information about the external world continuously flows into the brain, whereas its capacity to process such information is limited. Attention enables the brain to allocate its resources of information processing to selected sensory inputs for reducing its computational load, and effects of attention have been extensively studied in visual information processing. However, how the microcircuit of the visual cortex processes attentional information from higher areas remains largely unknown. Here, we explore the complex interactions between visual inputs and an attentional signal in a computational model of the visual cortical microcircuit. Our model not only successfully accounts for previous experimental observations of attentional effects on visual neuronal responses, but also predicts contrasting differences in the attentional effects of top-down signals between cortical layers: attention to a preferred stimulus of a column enhances neuronal responses of layers 2/3 and 5, the output stations of cortical microcircuits, whereas attention suppresses neuronal responses of layer 4, the input station of cortical microcircuits. We demonstrate that the specific modulation pattern of layer-4 activity, which emerges from inter-laminar synaptic connections, is crucial for a rapid shift of attention to a currently unattended stimulus. Our results suggest that top-down signals act differently on different layers of the cortical microcircuit.
Introduction
Neocortical microcircuits have a stereotyped structure, which is a six-layered network of excitatory pyramidal neurons and inhibitory interneurons. The structure is preserved across all neocortical regions, and is considered to represent the functional module of cortical information processing. Uncovering how neurons in the different layers process information is a key to understand the principles of cortical computation. Various anatomical, electrophysiological and computational studies have attempted to clarify the structure and function of cortical microcircuits. The basic design of their computation, however, remains largely unknown.
Large-scale simulation is a powerful modern tool to study computation in biological neuronal networks (Markram, 2006). For example, such simulations will allow us to study dynamical interactions in a brain-scale neural network, such as a large-scale network consisting of multiple cortical areas. As a step toward this direction of research, here we model the microcircuit of the visual cortex and explore differential functions of the individual cortical layers in attentional processing of sensory stimuli by top-down signals. Since interactions between top-down signals and bottom-up sensory inputs first occur within cortical microcircuits, the analysis of the laminar dependence of attentional modulations will provide a cue to understand the flow of information within or between cortical microcircuits, and hence the basic design of cortical computation.
A neuron in V2 or V4 is known to respond strongly to a bar presented in a neuron’s receptive field if the bar is aligned with the neuron’s preferred orientation (Reynolds et al., 1999). This neuronal response is markedly suppressed if the bar is accompanied by a second bar oriented toward a non-preferred orientation. Interestingly, however, if attention is prompted at the spatial location of the preferred stimulus prior to the presentation of the visual stimuli, the intensity of the neuronal response recovers at the original level. Whether similar attentional modulations occur in V1 remains unknown: small receptive fields of V1 neurons precluded such experiment (Luck et al., 1997). Lateral inhibition between neurons may account for the suppressed response to a preferred stimulus. By contrast, the attention-induced response modulation seems to require complex interactions between bottom-up sensory and top-down attentional inputs within cortical microcircuits (Martinez-Trujillo and Treue, 2004; Tiesinga and Sejnowski, 2009). Such a top-down control of attention has been suggested for the V4 responses evoked by stimulation to the frontal eye field (Armstrong et al., 2006).
Network models were previously proposed to explain the attentional enhancement of visual responses (Reynolds et al., 1999; Boynton, 2005; Buia and Tiesinga, 2008). However, the laminar dependence of attentional modulations by top-down signals has not been studied in cortical microcircuit models with biologically plausible layer structure. By the same token, it remains unclear how a bottom-up sensory input and a top-down attentional input may interact with each other in the laminar structure and produce the neural modulations observed in visual areas. Here, we model the microcircuits of the visual cortex to explore the attentional mechanism of selecting sensory inputs. Our model attempts to replicate the layered network structure of visual cortical columns, expressed in the connectivity patterns between excitatory and inhibitory integrate-and-fire neurons. Several biological features, such as the morphology of neurons and interneuron subtypes, are ignored due to limitations on experimental data and computational resources. Thus, our model focuses on a description of intra- and inter-laminar information flows during attention.
In sensory cortices, the bottom-up input carrying sensory information projects to cortical layer 4 (L4), whereas the top-down input from higher cortical areas to L2/3 and L5, avoiding L4 (Felleman and van Essen, 1991; Reid et al., 2009; Tiesinga and Sejnowski, 2009; Noudoost et al., 2010). Then, the output to higher cortical areas arises from L2/3, L5, and L6 of lower cortical areas. The laminar-specific input–output organization of the cortical microcircuit suggests that the microcircuit processing of sensory information is layer-dependent. Our model demonstrates that the response modulations in L2/3 and L5 are consistent with those observed in V2 and V4 (Luck et al., 1997; Reynolds et al., 1999). Interestingly, the response of L4 neurons in our model is modulated in a significantly different manner. Analyzing our numerical data, we propose distinct roles of top-down signals in attention modulations at L4 (input terminal of bottom-up signals) and L2/3 and L5 (output terminals to higher cortical areas) of the cortical microcircuit.
Materials and Methods
Microcircuit Model of Visual Cortical Columns
Figure 1 displays the major neuronal and synaptic components of our model cortical microcircuit, which consists of two columnar circuits, each representing the basic processing unit of the visual cortex and sharing their receptive fields. The columnar circuit has L2/3, L4, L5, and L6, and each layer consists of an excitatory neuron pool and an inhibitory neuron pool (see Table 1 for details). Layer 1 is not modeled explicitly as it primarily contains dendritic fibers of neurons in the other layers. Arrows on Figure 1A present major neuronal connections of this cortical microcircuit. Thick arrows show dense connections with a connection probability >0.08, while thin arrows represent connections with a connection probability <0.08 and >0.04. Other sparse connections are not shown. The detailed connection probabilities are listed in Table 2. For simplicity, all intra-columnar connections have the same synaptic weight. The full network consisting of two columns comprises in total around 80,000 integrate-and-fire neurons and 300 million synapses. See Tables A1 and A2 in Appendix for details of the neuron models and specific values of the parameters.
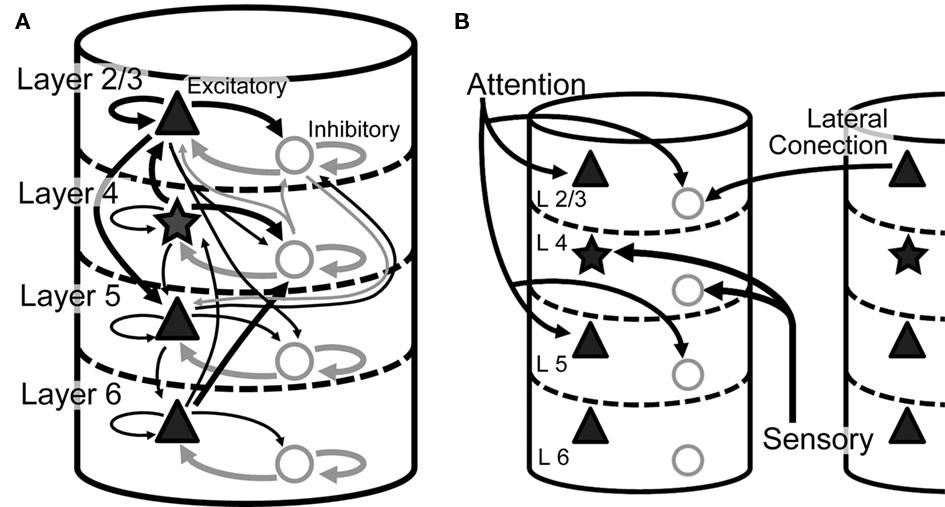
Figure 1. Model architecture. (A) Intra-columnar connections. The microcircuit model has two columns, each of which comprises 40,000 integrate-and-fire neurons and constitutes L2/3, L4, L5, and L6. Triangles and stars represent excitatory pyramidal and stellate neurons, respectively, whereas circles represent inhibitory neurons. All neurons are described by integrate-and-fire models in the present microcircuit model. Thick arrows represent strong synaptic connections with connection probability C > 0.08, and narrow arrows synaptic connections with C > 0.04. Other weaker synaptic connections are not shown. (B) Inter-columnar and external inputs. Layer 2/3 mediates reciprocal lateral inhibition between the columns through projections from excitatory neurons in one column to inhibitory in the other. Bottom-up sensory input projects to L4, while top-down attentional input projects to L2/3 and L5.
Experimental data currently available for the construction of microcircuits of visual cortices (V2 and V4) of macaque are limited. It is also difficult to obtain all necessary data from literature on a single animal species. Therefore, we construct a columnar circuit model primarily based on anatomical data of cat primary visual cortex (Binzegger et al., 2004) and also on electrophysiological data of cat and rat cortices (Thomson and Morris, 2002; Thomson et al., 2002). To compensate for shortcomings and inconsistencies of the individual data sets, we attempt to derive the connectivity from the two data sets consistently by carefully taking methodological limitations of anatomical and electrophysiological studies into account (Potjans and Diesmann, 2011). See the Section “Appendix” for the detailed estimation of intracolumnar synaptic connections and a complete model description.
Cortical L2/3 has rich recurrent synaptic connections within the same layer (Callaway, 1998). We introduced lateral inhibition between the L2/3 networks of the two columns to induce competition between them (Lübke et al., 2003; Chalupa and Werner, 2004; Smith et al., 2006; Adesnik and Scanziani, 2010). We model the lateral inhibition by projections from L2/3 excitatory neurons in one column to L2/3 inhibitory neurons in the other (Figure 1B), since typically only excitatory neurons make long-range connections and cross-orientation suppression is blocked by the application of GABA antagonist bicuculline (Morrone et al., 1987). Lateral connections in cortical networks remain largely unknown, and whether long-range excitatory connections exist between regions with orthogonal orientation preferences is under debate (Li et al., 2006; Priebe and Ferster, 2006). Some evidence, however, suggests the existence of such connections in the visual cortex (Kisvárday et al., 1997; Kimura and Ohzawa, 2009). We also tested another model with lateral inhibition from L2/3 inhibitory neurons in one column to L2/3 excitatory neurons in the other, and found that such a model does not produce significantly different results. Therefore, we will not present the results of this model in the present paper. The connection probability of the inter-columnar synaptic connections was set to 0.1. Columns of similar orientation specificity have long-range horizontal excitatory connections (Gilbert and Wiesel, 1983, 1989; Callaway and Katz, 1991). However, we do not introduce inter-columnar connections between excitatory neurons since the two columns of our model have different preferred orientations.
The parameterization of a previous single columnar model (Potjans and Diesmann, 2011) according to the mono-layered balanced random network model (Amit and Brunel, 1997) yielded spontaneous firing rates as observed in awake animals (de Kock and Sakmann, 2009, and references therein). Due to the lateral inhibition, L2/3 of the multicolumnar model tends to show spontaneous firing of lower frequencies in comparison with the single-columnar model. To compensate for this decrease in firing rate, we slightly increase the connection probabilities of projections from L4 excitatory neurons to L2/3 excitatory or inhibitory neurons and decrease the probabilities of projections from L4 inhibitory neurons to L2/3 excitatory neurons. With these modifications, the spontaneous firing rate of each layer falls down within a physiologically realistic range (L2/3 ∼1.4 Hz, L4 ∼2.5 Hz, L5 ∼12 Hz, L6 ∼0.5 Hz for pyramidal cells; L2/3 ∼5 Hz, L4 ∼5 Hz, L5 ∼9 Hz, L6 ∼6.5 Hz for inhibitory cells).
Numerical Experiments
Excitatory and inhibitory neurons in L4 of the model columnar circuits receive bottom-up visual inputs mimicking vertical and horizontal bars in experiment. When these visual stimuli are presented in the receptive field of the two columns, they respond preferentially to one of the two stimuli. Throughout this study, the vertical bar represents the preferred stimulus of column 1, whereas the horizontal bar its non-preferred stimulus. Column 2 has an opposite preference. The preferred and non-preferred inputs are sets of independent Poisson spike trains of 20 or 2 Hz, respectively. Table 3 lists the probabilities that a L4 neuron receives these sensory inputs in our model. We set the population size for these bottom-up visual stimuli to about 450 fibers (Potjans and Diesmann, 2011).
The L2/3 and L5 of the model receive excitatory inputs representing the top-down projection mediating selective attention (Peters and Rockland, 1994; Reynolds and Desimone, 2003; Ogawa and Komatsu, 2004; Reynolds, 2008; Tiesinga and Sejnowski, 2009; Noudoost et al., 2010). In our model, this top-down signal projects to both excitatory and inhibitory neurons although controversial arguments exist for the target cell types of the attentional input (Tiesinga and Sejnowski, 2009). Simultaneous input to excitatory and inhibitory neurons makes the neuronal response of the present model multiplicative (Salinas and Abbott, 1996; Chance et al., 2002), better explaining experimental observations. The top-down input is a set of low-frequency Poisson spike trains of 5 Hz, which is much smaller than the rate of the preferred stimulus. The weak top-down signal seems reasonable as it prevents the lower order cortices from being a mere slave to the higher order cortices (Deco and Lee, 2004). In each simulation trial, we applied the attention signal to one of the two columns that prefers the attended stimulus (Buia and Tiesinga, 2008). Actually, to direct attention to multiple features or locations at the same time is known to be difficult (Eriksen and Yeh, 1985; Posner and Petersen, 1990). The number of spike trains in the top-down projection is set to 600 in both L2/3 and L5, and the projection probabilities of the top-down connections are given in a physiologically realistic range (Table 3). The way the weak top-down input modulates the neuronal activity in L2/3 and L5 is demonstrated in Figure A1.
In some simulations, we compare the above top-down attention model with a bottom-up attention model, in which the top-down inputs to L2/3 and L5 are not included and the bottom-up input corresponding to an attended bar is enhanced by 25% compared to non-attended bottom-up inputs. All simulation results presented here were produced with the NEST 2 Simulation Tool (Gewaltig and Diesmann, 2007), using 8 cores [Xeon(R) 2.26 GHz] and MPI for the parallel computation.
Results
The details of our cortical microcircuit model are explained in Materials and Methods as well as in Figure 1. We investigated the responses of our model in simulation trials that mimicked the experimental setting of Reynolds et al. (1999), in which vertical and horizontal bars were separately or simultaneously presented in the receptive field of visual cortical neurons (Figure 2A). In some trials, the expected location of one of the stimuli (circled in Figure 2B) was prompted by a visual cue prior to the presentation of oriented bars in order to direct monkeys’ attention to the stimulus shown at the prompted location. Our layered network model represents two mutually interacting cortical columns consisting of cortical layers L2/3, L4, L5 and L6. The two columns interact with one another through lateral inhibition implemented at L2/3 by projections from excitatory neurons in one column to inhibitory in the other (Figure 1). L4 of each column receives two bottom-up external inputs corresponding to vertical and horizontal bars in the experiment. The bottom-up input representing a vertical bar strongly projects to the L4 of column 1 (thick arrow in Figure 2C), while that representing a horizontal bar projects only weakly to this column (a thin arrow in Figure 2C). Column 2 has opposite preferences to vertical and horizontal bars. In addition to the bottom-up sensory inputs, each column receives a top-down signal to L2/3 and L5 when its preferred stimulus is attended (Figure 2D).
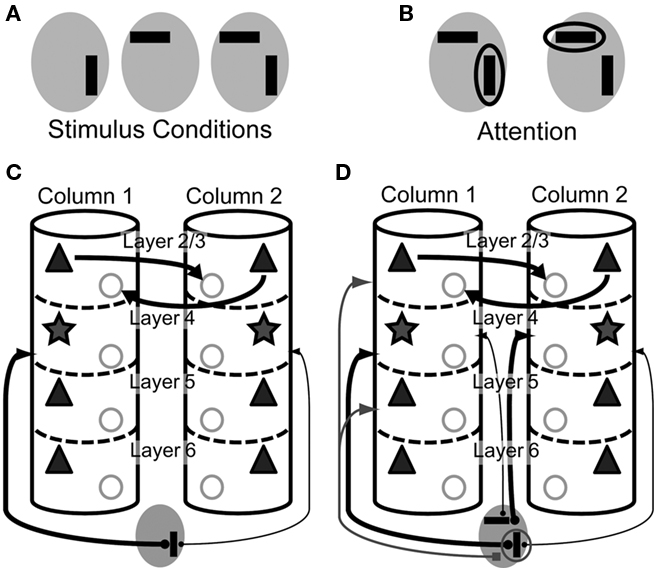
Figure 2. Visual stimuli and top-down signals in our model. A total of five different stimulus patterns are used in the numerical simulations. (A) Visual stimuli mimicking vertical and horizontal bars are presented to the model in three different combinations. Gray ellipses represent the overlapping receptive field of the two columns. (B) Top-down signal is applied to either the vertical or the horizontal bar to be attended. The empty ellipse refers to the stimulus attended. (C) Visual inputs to the two columns are schematically illustrated in a situation where a vertical bar is presented in the receptive field. The vertical orientation is the preferred orientation of column 1. The thickness of each projection represents its strength. (D) Visual and top-down inputs to the two columns are shown when a vertical bar and a horizontal bar are simultaneously presented in the receptive field and the former is attended. The horizontal orientation is the preferred orientation of column 2.
A general assumption here is that attention is mediated by an excitatory signal from the higher cortical circuits (e.g., see Noudoost et al., 2010). However, there is also ample evidence for other possible mechanisms of attention. For instance, neuromodulatory inputs, such as Ach (see Klinkenberg et al., 2011 for review) and dopamine (Noudoost and Moore, 2011), are known to mediate attentional modulations. Furthermore, thalamocortical input may be also influential in attentional modulations (see Saalmann and Kastner, 2009 for review). The present model without neuromodulatory and/or thalamic attentional inputs is sufficient for a rate-based description of the attentional modulations found in the monkey visual cortices (Reynolds et al., 1999) and yields testable predictions.
Responses of the Multicolumnar Microcircuit Model to Bottom-Up and Top-Down Inputs
Figure 3A summarizes the mean firing rates of excitatory and inhibitory neurons in each layer of column 1 of our microcircuit model in various stimulus conditions. This column responded strongly to a preferred stimulus (vertical bar) and weakly to a non-preferred stimulus (horizontal bar). In L2/3 and L5 of the model, a simultaneous presentation of two stimuli suppresses neuronal responses compared with those to the preferred stimulus, especially in excitatory neurons. Then, a top-down input enhances the responses evoked in these layers up to the levels of the responses to the preferred stimulus presented alone. By contrast, attention to the non-preferred stimulus, i.e., an attentional input to column 2, suppresses neuronal responses in the two layers. The attentional effects on excitatory neurons in L2/3 and L5 are statistically significant compared to neuronal responses in the absence of attention. All the response patterns mentioned above are consistent with the previous experimental results (c.f. Figures 6–8 in Reynolds et al., 1999). However, we note that a simultaneous presentation of two visual stimuli enhances the responses of excitatory neurons in L4, whereas attention to a preferred stimulus significantly reduces the responses. These modulation patterns are opposite to those in L2/3 and L5.
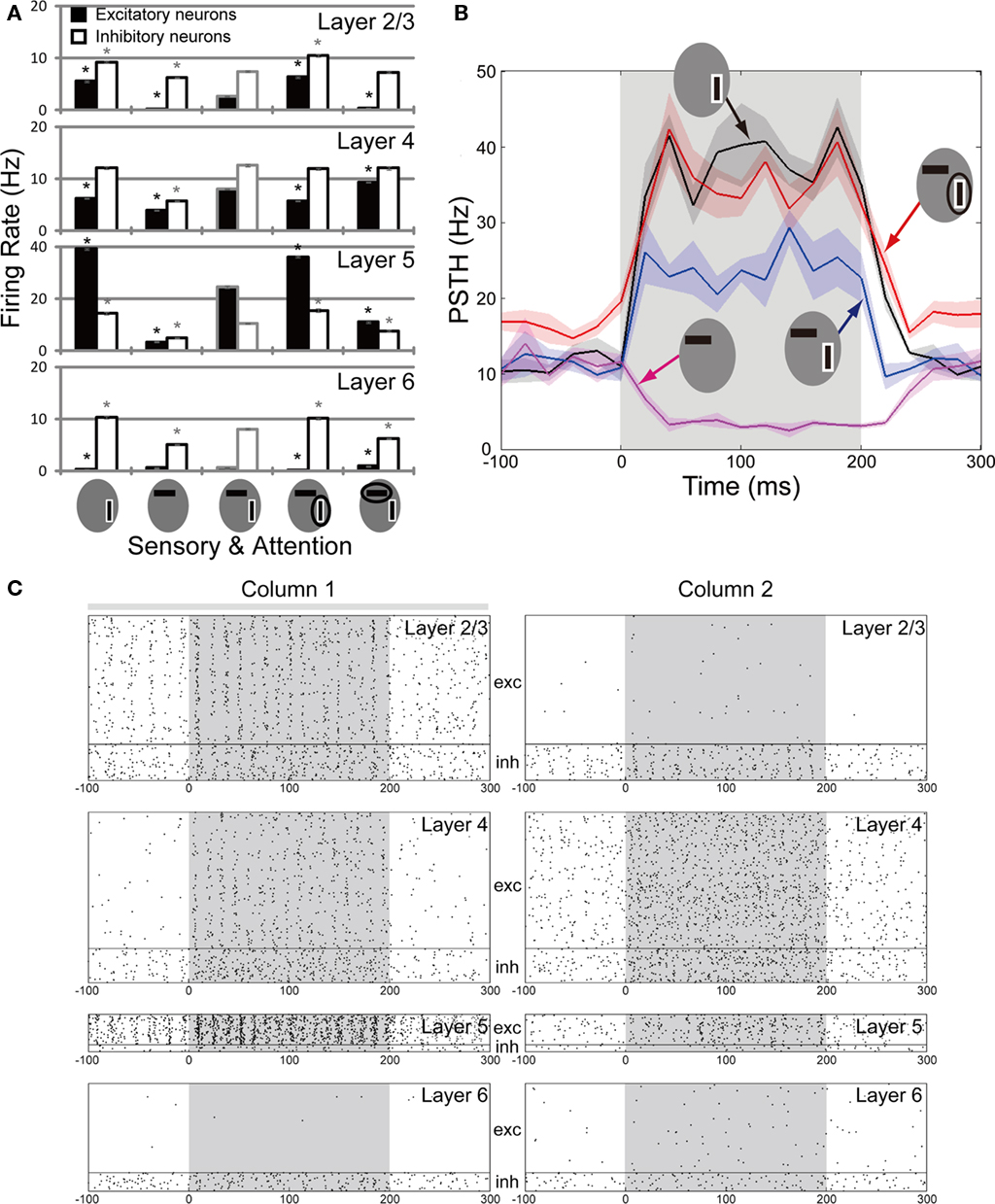
Figure 3. Neuronal responses of the multicolumnar model. (A) The population firing rates of excitatory (filled bars) and inhibitory (empty bars) neurons from 20 trials are shown for each layer of column 1 for various combinations of visual and top-down inputs. The preferred stimulus of column 1 is bordered white. An attended stimulus is circled. Asterisks indicate the firing rates of excitatory (black) and inhibitory (gray) neurons that are significantly different (p < 0.05) from those in the stimulus condition with two oriented bars and without attentional bias. (B) Time evolution of the average firing rate is shown for the excitatory neuron population in L5 of the multicolumnar model. Stimuli are presented during the gray shaded period (0–200 ms). The PSTH is computed with 20 ms time bins. Shadings of curves show the SE of the mean obtained from 5 simulation trials. (C) Responses of the multicolumnar model to vertical and horizontal bars with an attentional input applied to column 1 (gray bar). The attentional input to column 1 increases the activities of L2/3 and L5 in column 1, while suppressing the activities of the same layers in column 2. Layers 4 and also layer 6 exhibit an opposite activation pattern.
The L2/3 and L5 of the bottom-up attention model also displayed attentional modulations similar to those observed in the top-down model. The L4 of the bottom-up model, however, did not exhibit significant attentional modulation (Figure A2). The weak modulation of L4 is understood as effects of inhibitory feedback from L2/3 to L4. Thus, the attentional modulation in L4 is characteristic to the case where visual attention is mediated by top-down signals to L2/3 and L5.
Figure 3B shows the time course of averaged peri-stimulus-time-histograms (PSTHs) of L5 excitatory neurons of column 1 in the different stimulus conditions. The consistency between our model and the experiment by Reynolds is most clearly demonstrated in the neuronal responses in L5. Since extracellular recordings from the cortex of behaving monkeys do not allow us to morphologically identify recorded neurons, it remains unclear how strongly this coincidence with the experimental results supports the model. However, it is possible that extracellular recording data contains L5 neurons more often than others owing to their relatively high firing rate. Therefore, the consistency between the model’s L5 activity and experiment is particularly interesting.
In addition to the rate modulations, the activity evoked in L2/3, L4 and L5 of column 1 tends to exhibit synchronized oscillations in the gamma frequency range. To see this, we display a typical example of spike raster of our microcircuit model in Figure 3C, where it receives sensory inputs to columns 1 and 2 (shaded intervals) and an attentional input to column 1 (gray bar above the spike raster). Therefore, the raster corresponds to the red trace shown in Figure 3B. We found that the gamma oscillations emerge strongly when L2/3 has strong recurrent excitatory connections. The gamma oscillations are considered to play an active role in visual attention (Fries, 2009; Womelsdorf et al., 2006; Khayat et al., 2010). We, however, do not enter into a detailed analysis of the gamma oscillations since the dynamical property of such synchronization depends significantly on the biological details of fast-spiking interneurons (Lewis et al., 2003; Nomura et al., 2003; Mancilla et al., 2007) that are not implemented in the present model.
Role of L2/3-to-L4 Projections in Attentional Processing by Cortical Microcircuits
Laminar-specific neuronal modulations provide experimentally testable predictions of our model. Of particular interest are the response modulations in L4, which show a remarkable contrast to those of L2/3 and L5. In fact, an attentional input gives opposite effects on the response of L4 excitatory neurons, which exhibit suppressed responses when their preferred stimulus is attended. In other words, their responses are enhanced when their non-preferred stimulus is attended (see Figure 3A). This tendency is also seen from the spike raster of L4 neurons in Figure 3C. Thus, our model predicts that the top-down input plays differential roles in attentional processing at the input (L4) and output (L2/3 and L5) stations of the cortical microcircuit.
The underlying mechanism of the modulation pattern of L4 can be understood as follows. As shown in Figure 1A, the major inter-laminar projections to L4 are those to L4 inhibitory neurons from L2/3 excitatory or L6 excitatory neurons. When a vertical bar (the preferred stimulus of column 1) is attended, L2/3 excitatory neurons are activated more strongly in column 1 than in column 2 due to the inter-columnar competition. This means that the L4 activity is suppressed more strongly in column 1 than in column 2 by the inhibitory effect of the projections from L2/3 (Figure 4A). This explains why the pattern of response modulations is reversal in L4 excitatory neurons. Strong projections from L6 excitatory to L4 inhibitory neurons work essentially in the same fashion, although they only play a minor role due to a relatively low activation of L6 (Figure 3A). To confirm these arguments and predictions, we reduce the connection probabilities of the projection from L2/3 excitatory to L4 inhibitory neurons as 0.069 → 0.015. To avoid too strong winner-take-all behavior, we simultaneously weaken the inter-columnar lateral inhibition as 0.1 → 0.08. As expected, this modified model does not exhibit strong modulations of activity of L4 excitatory neurons in column 1 when a vertical bar is attended (Figure 4B). Weakening the projections from L6 excitatory to L4 inhibitory neurons also gives similar results (not shown), as expected.
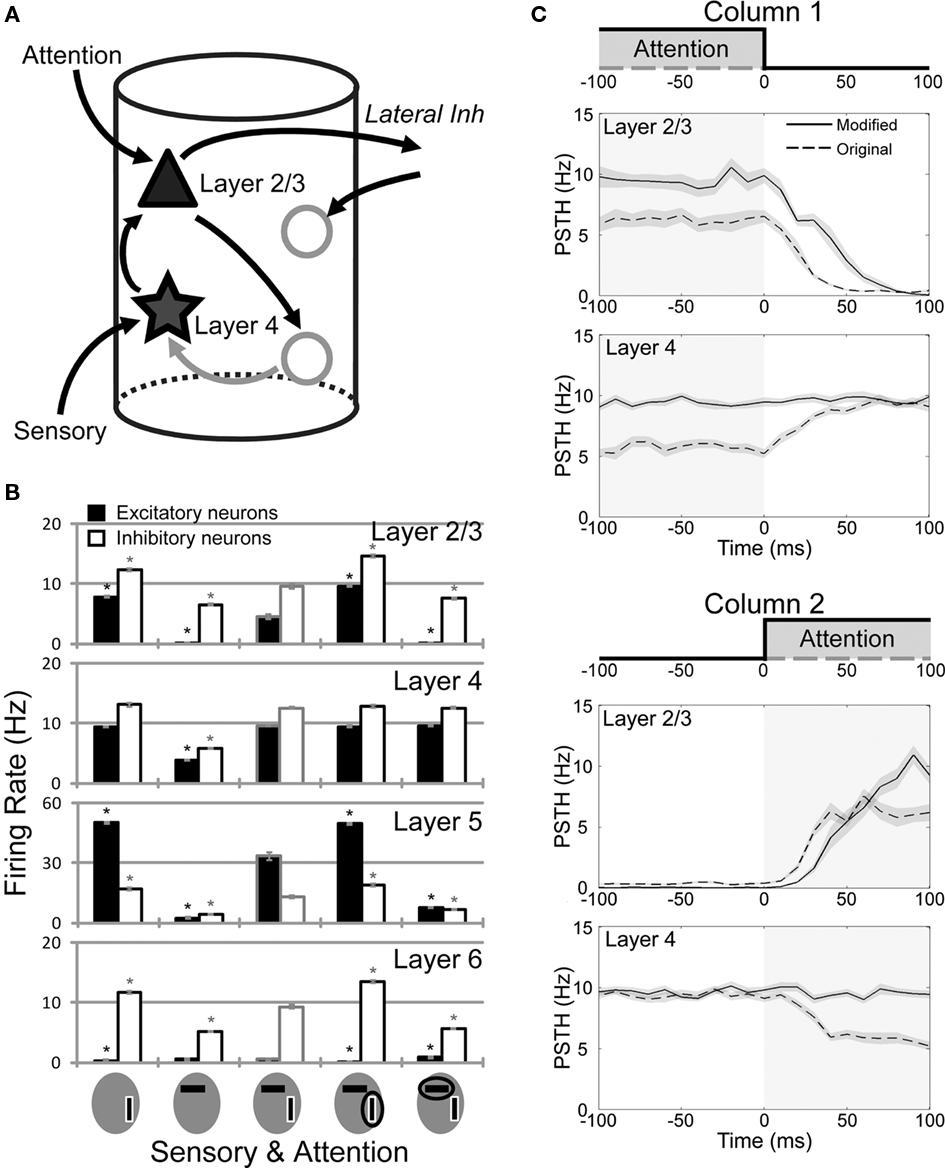
Figure 4. Role of the attention-induced suppression of L4 activity in attention shift. (A) The circuit mechanism of the response modulation in L4 is explained schematically. When the L2/3 activity is increased, connections from L2/3 excitatory to L4 inhibitory neurons suppress the L4-to-L2/3 excitatory drive, which in turn weakens the inter-columnar competition and leads to the attentional suppression of L4. (B) The averaged firing rates obtained from twenty simulation trials of a microcircuit model with a reduced connection probability from L2/3 excitatory to L4 inhibitory neurons. Activity of L4 neurons is not much modulated by a top-down input, while L2/3 and L5 display response modulations similar to those in the original model (see Figure 3A). Asterisks indicate statistically significant differences in firing rate compared to the responses to horizontal and vertical bars presented simultaneously. (C) Responses of L2/3 and L4 of the original and modified models before and after the attentional shift at 0 ms. Upper and lower two panels show activities of columns 1 and 2, respectively. At 0 ms, the attentional input switches off in column 1, while it switches on in column 2.
Rapid Switching of Attention by Top-Down Signals in the Cortical Microcircuit Model
Comparison between the models shown in Figure 3A (original model) and Figure 4B (modified model) allows us to address functional implications of the attention-induced suppression in L4. To this end, we investigated the transient behavior of these microcircuit models when top-down attention is suddenly shifted from a vertical bar to a horizontal bar, as illustrated by shaded areas in Figure 4C. The transient responses of L2/3 and L4 of both models are compared in Figure 4C. We first explain the behavior of the original model. When a vertical bar (the preferred stimulus of column 1) is attended, column 1 becomes a winner. The activity of L2/3 of column 1, however, is lower in the original model than in the modified model since L4 of the column, which sends strong excitatory projections to L2/3, is suppressed by inhibitory influences from L2/3 during attention to a vertical bar (Figure 3A). After attention is shifted to a horizontal bar, L2/3 activity decays rapidly in column 1, as it was initially low. This implies that lateral inhibition from column 1 to column 2 also decreases rapidly, and hence L2/3 activity can grow rapidly in column 2.
By contrast, in the modified model attention to a vertical bar does not suppress the L4 activity of column 1 (Figure 4B), so the L4-to-L2/3 excitatory projection keeps the L2/3 activity also high (Figure 4C). Because L2/3 is highly activated in column 1, inhibition from column 1 to column 2 remains strong for a sufficiently long time and prevents a rapid activation of the L2/3 of column 2 after the shift of attention.
Thus, the L2/3 of column 2 is activated after an attentional shift about 10 to 15 ms earlier in the original model than in the modified one. Activities of L5 also exhibit similar differences between the two columns (results not shown). Thus, the suppression of L4 activity induced by the top-down signal regulates the inter-columnar competition in a functionally useful way. Consequently, the suppressed L4 activity enables the microcircuits of lower visual cortices to immediately respond to a newly attended stimulus and output the relevant information to higher visual areas.
Effects of Various Intra-Laminar Connections on the Microcircuit Output
In the following, we investigate the influence of different intra-columnar connections on neuronal responses. We have shown above that projections from L4 excitatory to L2/3 excitatory neurons play an important role in accelerating the attentional shift. However, too much increase in the connection probability of such projections (0.0846 → 0.1265) results in over-excitation and bursting activity in L2/3 of the microcircuit responding to bottom-up sensory inputs (Figure 5A). In such a case, inter-columnar lateral inhibition induces synchronous and alternating (between the columns) activation of L2/3 neurons, which enslaves also other layers. Thus, the excitatory drive on L2/3 by L4 should be kept in an appropriate range.
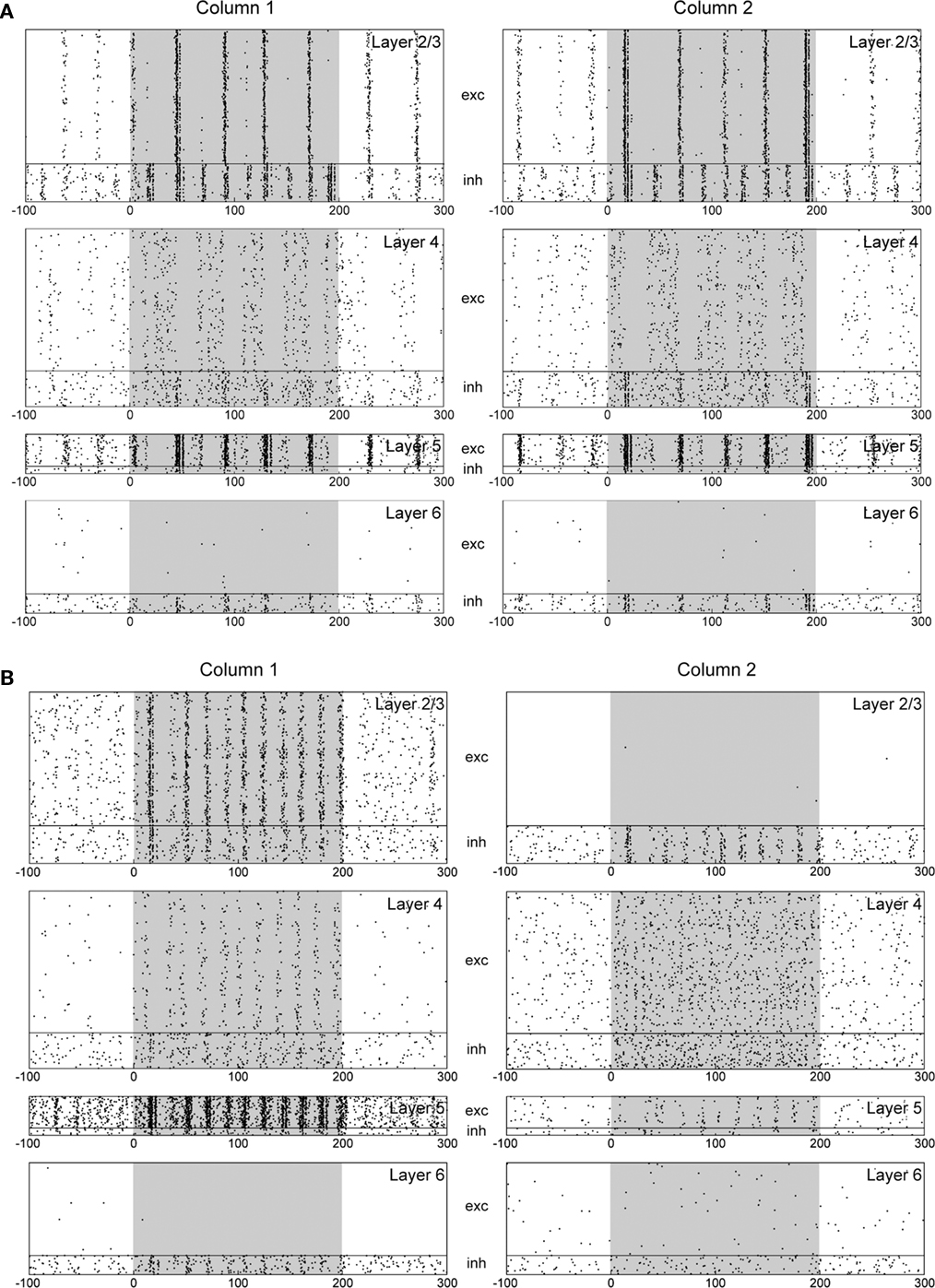
Figure 5. Influences of interlaminar connections on the response of the multicolumnar model. Both columns receive visual stimuli (shaded), but no attentional input. (A) For an increased connection probability from L4 excitatory to L2/3 excitatory neurons. (B) For a decreased connection probability from L5 excitatory to L2/3 inhibitory neurons. Even without attentional input, the two columns compete with one another strongly to determine a winner just by chance.
Since L2/3 is the source of mutual inhibition, we also investigate the effects of other intra-columnar connections that may influence activity of the L2/3 network. First, we decrease the connection probability from L5 excitatory to L2/3 inhibitory neurons (0.0755 → 0.0485) to enhance the L2/3 activity. As seen from Figure 5B, this modification induces too strong winner-take-all competition between the two columns even without sensory stimuli, where a winner is essentially selected by chance. Such a microcircuit model cannot adequately process sensory stimuli.
Next, decreasing the connection probability of excitatory-to-inhibitory synaptic connections within L2/3 (0.1008 → 0.0504) also results in a rather strong inter-columnar competition in response to sensory inputs (Figure 6A). A model with decreased L2/3 inhibitory-to-excitatory connections or with decreased L4 inhibitory to L2/3 excitatory connections displays similar responses (data not shown).
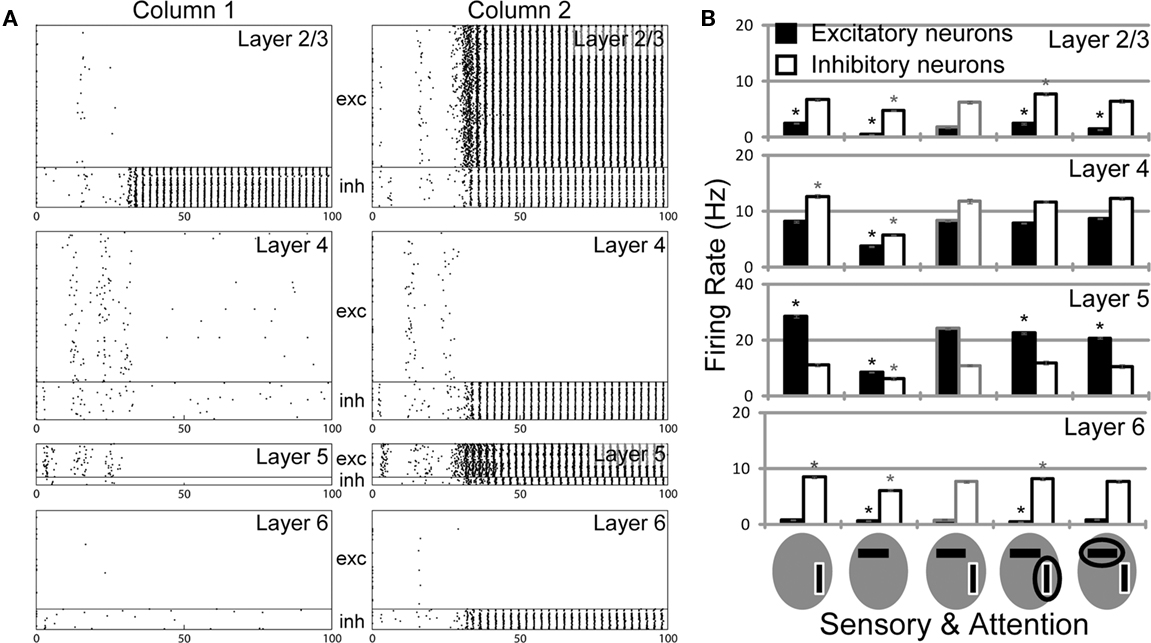
Figure 6. Influences of L2/3 intralaminar connections on the response of the multicolumnar model. Both columns receive visual stimuli from 0 ms onward, but not an attentional input. (A) A model with a decreased connection probability of L2/3 excitatory-to-inhibitory synapses exhibits a rather strong inter-columnar competition. In such a situation, an attentional input does not play a meaningful role. (B) Attentional response modulations are shown for the column 1 of a model with a decreased connection probability of L2/3 excitatory-to-excitatory recurrent synapse. Asterisks indicate the statistical significance of rate changes compared to the responses to horizontal and vertical bars presented simultaneously. The modulations of excitatory activity are statistically significant in both L2/3 and L5 of column 1. However, the magnitudes of these modulations are very small.
Finally, a model with decreased L2/3 excitatory-to-excitatory recurrent connections (0.1184 → 0.0592) significantly weakens the effects of top-down signals on attentional modulations (Figure 6B) compared to those shown in Figure 3A, implying that competition between the columns is too weak. Thus, L2/3 excitatory neurons should be activated sufficiently strong (but not too strong) to make the inter-columnar inhibition effective.
Discussion
Visual attention is a function that boosts the activity of neurons (Treue and Maunsell, 1999; Reynolds et al., 2000; Martinez-Trujillo and Treue, 2004; Sommer, 2007; Ogawa and Komatsu, 2009; Tiesinga and Sejnowski, 2009). It enables the brain to attend the most important information at the moment (Itti and Koch, 2001), enhancing perception in a number of aspects (Posner, 1980; Carrasco et al., 2004; Wagatsuma et al., 2008). To explore the circuit mechanism of visual attention, we have constructed a detailed model of the visual cortical microcircuit consisting of two multi-layer columns of about 40,000 integrate-and-fire neurons (about 80,000 neurons in total). The two columns receive different preferred bottom-up sensory inputs at L4 as well as top-down inputs representing the attentional bias at L2/3 and L5, and interact with each other via the lateral inhibition implemented within L2/3. We also tested a model with additional lateral inhibition from L2/3 excitatory to L5 inhibitory neurons (Adesnik and Scanziani, 2010), and found that the model does not produce essential differences (data not shown). The layers 2/3 and 5 of our multicolumnar model successfully replicated the responses of visual cortical neurons and their attentional modulations reported by experiments (Reynolds et al., 1999). In single unit recordings from behaving animals, a random sampling of neuronal activity likely results in a biased sampling of L5 neurons, as they are more active and larger than neurons in the superficial layers. Therefore, the attentional modulations in L2/3 and L5 of our model are likely to be consistent with experimental findings. More interestingly, our model has predicted that attention mediated by top-down signals induces in L4 a response modulation pattern opposite to that in L2/3 and L5.
Mechanism and Implications of the Attention-Induced Response Suppression in Layer 4
In our model, top-down attention on a preferred stimulus suppresses, rather than enhances, the response of L4 neurons (Figure 3). Our numerical analysis has shown that bi-directional synaptic interactions between L2/3 and L4 underlie the attention-induced suppression of the L4 activity in the following manner. Sensory input received at L4 is routed by excitatory-to-excitatory connections to L2/3, which implements a winner-take-all competition between columns. If attention is given to the preferred stimulus of a column, this column becomes a winner and its L2/3 exhibits an elevated activity. Then, the feedback projection from L2/3 excitatory to L4 inhibitory neurons increases the activity of latter neurons and hence suppresses activity of L4 excitatory neurons in the winner column (Figure 1). In contrast, attention to a non-preferred stimulus of a column makes this column a looser for the inter-columnar competition, hence suppressing its L2/3 activity. Consequently, its L4 excitatory neurons are released from inhibitory influences (via L4 inhibitory neurons) of L2/3 excitatory neurons, and exhibit an enhanced response to the stimulus (Figures 4A,B).
While the attention-induced enhancement of output from L2/3 and L5 is considered to facilitate the analysis of the attended object by higher visual areas (Felleman and van Essen, 1991; Noudoost et al., 2010), the role of the attention-induced suppression of L4 activity is not so obvious. We have suggested that the suppression is advantageous for a rapid exchange of the roles of winner and looser between the columns when attention is shifted to one visual stimulus to another (Figure 4C). Too strong activation of L4 in a winner column innervates its L2/3 very strongly, and slows down the exchange of L2/3 activities between the columns that follows the shift of top-down signals for attention (Figure 4C). Thus, an adequate regulation of L4 activity by inhibitory feedback from L2/3 excitatory neurons is necessary for the attentional processing of bottom-up sensory stimuli by top-down input (Figure 5A). In fact, cortical microcircuits would not stably response to sensory stimuli if L2/3 had strong excitatory projections to L4, hence closing an excitatory feedback loop between L2/3 and L4.
Influences of Various Intra- and Inter-Laminar Connections on Inter-Columnar Competition
Like many other models of visual attention, the response of our microcircuit model is determined by the dynamical competition between mutually inhibiting columns. How various intra- and inter-laminar connections influence this competition is well understood from the previous results of multiple-winner selection by inhibitory neural networks (Fukai and Tanaka, 1997), which are summarized as follows. When lateral inhibition between inhibitory neurons dominates over self-inhibition, a unique winner survives for the competition (winner-take-all behavior). In contrast, if self-inhibition dominates over the lateral inhibition, multiple winners can survive (winners-share-all). The different dynamical behaviors are schematically illustrated for a competitive network consisting of two inhibitory neurons (Figure 7A).
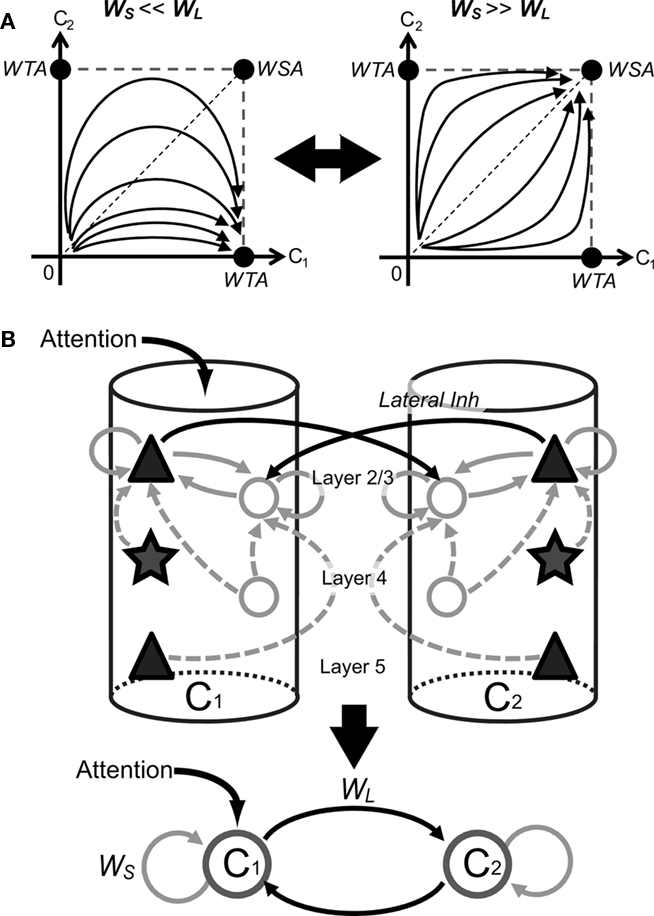
Figure 7. Schematic illustrations of the underlying mechanism of attentional modulations by top-down signals. (A) The number of winners surviving the competition in a mutually inhibiting neural network can be determined analytically by the balance between lateral and self-inhibition. Multiple winners can survive if the self-inhibition dominates over lateral inhibition (Winners-Share-All), whereas only a single winner survives in the opposite case (Winner-Take-All). The two situations are illustrated in the simplest network with only two inhibitory neurons. (B) The above results hold for the present microcircuit model if we regard the L2/3 network in each column as a single processing unit. Black solid lines represent intercolumnar connections, while gray sold and dashed lines show intracolumnar connections within and toward L 2/3, respectively.
These results can be applied to the present model if we regard the entire L2/3 neuronal network as an effective processing unit (Figure 7B). For instance, excitatory-to-inhibitory connections within L2/3 of each column represent self-inhibition on the processing unit. Therefore, weakening these connections leads to a stronger winner-take-all competition between columns (Figure 6A). Similarly, weakening inhibitory-to-excitatory connections in L2/3, connections from L5 excitatory to L2/3 inhibitory neurons (data not shown), and recurrent excitatory connections in L2/3 (Figure 6B) all weaken the self-inhibition, hence inducing a stronger inter-columnar competition. Thus, the strengths of various inter- and intra-laminar connections must be adequately balanced for the proper processing of bottom-up sensory and top-down attentional inputs by the present cortical microcircuit model.
Limitation of the Present Model
Our microcircuit model is primarily based on the anatomical (Binzegger et al., 2004) and electrophysiological data (Thomson et al., 2002) obtained from the visual cortices of cat and rat. Although visual cortices of both cat and monkey have a columnar structure, whether our model gives a reasonable approximation of the cortical microcircuit of monkey visual cortex, where experimental data were collected (Reynolds et al., 1999), remains unclear at present. Similarities and dissimilarities in the structure and function of cortical microcircuits between different animal species have to be further explored.
Firing rates of neurons decreased rapidly in our model if sensory input to layer 4 was removed. In experiments, however, the firing rate decayed slowly in a few hundreds of milliseconds after the disappearance of visual stimuli. We can think of several reasons for this discrepancy. Cortical microcircuits in V2 or V4 may receive more complex subcortical or cortical inputs that were not incorporated into the present model. Another possible reason is that our network model does not contain NMDA receptor-mediated currents at recurrent synapses due to a limitation on our computational resource. In fact, NMDA receptors can generate asynchronous persistent firing in recurrent cortical networks (Wang, 1999), so the inclusion of NMDA receptors would slow the activity decay in our microcircuit model. However, the slow decay of activity after stimulation was not the focus of this work and a large number of excitatory synapses in the model make such modifications computationally very costly.
Attention is known to involve cholinergic modulation on neuronal activity (Bentley et al., 2003; Herrero et al., 2008), and acetylcholine enhances gamma-band oscillations of visual cortical neurons (Rodriguez et al., 2004). Gamma-band synchronized oscillations are likely to be crucial for attention (Fries, 2009; Tiesinga and Sejnowski, 2009; Ardid et al., 2010). Our microcircuit model also exhibits such activity (Figure 3C), if excitatory connections, particularly recurrent excitatory connections in layer 2/3, are sufficiently strong. The present model, however, is not realistic enough to address the role of gamma-band synchronization in the microcircuitry processing of attention. For this purpose, we should use a more realistic model of fast-spiking interneurons since they strongly influence the dynamics of such synchronization (Lewis and Rinzel, 2003; Nomura et al., 2003; Cardin et al., 2009). Some hypothesis says that acetylcholine levels modulate the interaction between top-down and bottom-up processing to generate appropriate neural representations for inputs (Yu and Dayan, 2002). Testing such a hypothesis in a microcircuit model similar to the present one seems to be an intriguing future problem.
Usefulness of Large-Scale Simulation Models
In this study, we have interpreted the dynamical behavior of the microcircuit model only on a rate-based level, and have not explored the temporal structure of simulated activities. We could have achieved a similar insight into the microcircuit dynamics using a rate-based model or a reduced mean-field model without simulations of spiking neurons. However, to derive a reliable reduced model, we need a correct mapping of biological parameters onto the space of reduced variables and intuition about what would occur in the original complex network of spiking neurons. For example, we have shown that the reciprocal interactions between L2/3 and L4 in single columns are crucial for the rapid switching of top-down attention between multiple sensory signals (Figure 4). It would be difficult to derive the theoretical prediction from a reduced model without simulating the microcircuit model having a connectivity matrix constructed as realistic as possible according to known anatomical and physiological data. We also note that a discovery in a rate-based model should be cross-validated in a more realistic spiking model.
In addition, large-scale simulation of spiking neurons is useful for studying spike-based learning in complex biological neural networks. For example, we may use a microcircuit model with spike-timing-dependent plasticity for investigating the activity-dependent development of a fine-scale network structure in specific cortical areas. To make such studies technically tractable, we have developed techniques for efficient simulations of large-scale spiking neuron networks, providing a proof of concept, merit and constraint for the method.
Comparison to Previous Models
Several models were proposed to account for the attentional modulations of visual responses studied here. First, Reynolds et al. (1999) proposed a simple feed-forward network model in which attention strengthened the synapses mediating the attended stimulus. However, modifications of synaptic weights or neuronal excitability are generally slow and the rapid modification may be somewhat unrealistic. Deco and Lee (2004) proposed that interactions between lower- and higher-visual areas mediate the attentional modulations, without modeling the microscopic circuit-level mechanism. Buia and Tiesinga (2008) constructed a simple network model representing two parallel visual pathways, each of which comprises one excitatory and two inhibitory neurons, and mutually inhibiting one another. These are the minimal number of elements required to produce the physiological findings. Attention essentially releases a visual pathway by suppressing the lateral inhibition on it. Our model is closely related to this model, and demonstrates how lateral (and self-) inhibition is controlled by the realistic connectivity of the cortical microcircuit. Most interestingly, our model revealed layer-specific effects of top-down input on attentional processing that are experimentally testable.
The present attentional modulations may be also modeled in terms of bottom-up spatial attention on the location of a visual object. Bottom-up spatial attention increases the contrast gain in early visual areas (Lee et al., 1999; Carrasco et al., 2004; Peters et al., 2005), and such attention was previously modeled as a slight increase in sensory input at the locus of attention (Buia and Tiesinga, 2008). If we model the spatial attention in this fashion, the responses of L2/3 and L5 of the bottom-up microcircuit model were quite similar to those of the present top-down model, whereas the L4 of the bottom-up model exhibited no significant response modulations (Figure A2). In reality whether the spatial attention works at a resolution less than the size of the cell’s receptive field remains to be further studied.
Conclusion
We have demonstrated that a layered cortical microcircuit model based on an integrated connectivity map derived from anatomical and electrophysiological data can account for the previous experimental observations on neuronal responses to visual stimuli with and without attention. Our multicolumnar model provides a canonical microcircuit model for the dynamic selection of multiple sensory inputs in the visual cortices, and made several testable predictions about the layer-dependence of the response modulations. In particular, our model predicted that attention to a preferred stimulus of a L4 neuron should suppress its response in order to prepare for a shift of attention to a different sensory stimulus.
Conflict of Interest Statement
The authors declare that the research was conducted in the absence of any commercial or financial relationships that could be construed as a potential conflict of interest.
Acknowledgments
This work was partly supported by Grants-in-Aid for Scientific Research on Innovative Areas (no. 22115013), the Next-Generation Supercomputer Project of MEXT, Japan, EU Grant 15879 (FACETS), EU Grant 269921 (BrainScaleS), BMBF Grant 01GQ0420 to BCCN Freiburg and the Helmholtz Alliance on Systems Biology. We are grateful to Yumiko Yoshimura of National Institute of Physiology for valuable discussion about the laminar organization of cortical microcircuits. We also benefited from critical discussions with Tadaharu Tsumoto, Ko Sakai, Tadashi Sugihara, and Zhaoping Li.
References
Adesnik, H., and Scanziani, M. (2010). Lateral competition for cortical space by layer-specific horizontal circuits. Nature 464, 1155–1160.
Amit, D. J., and Brunel, N. (1997). Model of global spontaneous activity and local structured activity during delay periods in the cerebral cortex. Cereb. Cortex 7, 237–252.
Ardid, S., Wang, X. J., Gomez-Cabrero, D., and Compte, A. (2010). Reconciling coherent oscillation with modulation of irregular spiking activity in selective attention: gamma-range synchronization between sensory and executive cortical areas. J. Neurosci. 30, 2856–2870.
Armstrong, K. M., Fitzgerald, J. K., and Moore, T. (2006). Changes in visual receptive fields with microstimulation of frontal cortex. Neuron 50, 791–798.
Bentley, P., Vuilleumier, P., Thiel, C. M., Driver, J., and Dolan, R. J. (2003). Cholinergic enhancement modulates neural correlates of selective attention and emotional processing. Neuroimage 20, 58–70.
Binzegger, T., Douglas, R. J., and Martin, K. A. C. (2004). A quantitative map of the circuit of cat primary visual cortex. J. Neurosci. 24, 8441–8453.
Buia, C. I., and Tiesinga, P. H. (2008). Role of interneuron diversity in the cortical microcircuit for attention. J. Neurophysiol. 99, 2158–2182.
Callaway, E., and Katz, L. C. (1991). Effects of binocular deprivation on the development of clustered horizontal connections in cat striate cortex. Proc. Natl. Acad. Sci. U.S.A. 88, 745–749.
Callaway, E. M. (1998). Local circuits in primary visual cortex of the macaque monkey. Annu. Rev. Neurosci. 21, 47–74.
Cardin, J. A., Carlén, M., Meletis, K., Knoblich, U., Zhang, F., Deisseroth, K., Tsai, L. H., and Moore, C. I. (2009). Driving fast-spiking cells induces gamma rhythm and controls sensory responses. Nature 459, 663–667.
Carrasco, M., Ling, S., and Read, S. (2004). Attention alters appearance. Nat. Neurosci. 7, 308–313.
Chance, F. S., Abbott, L. F., and Reyes, A. D. (2002). Gain modulation from background synaptic input. Neuron 35, 773–782.
de Kock, C. P. J., and Sakmann, B. (2009). Spiking in primary somatosensory cortex during natural whisking in awake head-restrained rats is cell-type specific. Proc. Natl. Acad. Sci. U.S.A. 106, 16446–16450.
Deco, G., and Lee, T. S. (2004). The role of early visual cortex in visual integration: a neural model of recurrent interaction. Eur. J. Neurosci. 20, 1089–1100.
Eriksen, C. W., and Yeh, Y. Y. (1985). Allocation of attention in the visual field. J. Exp. Psychol. Hum. Percept. Perform. 11, 583–597.
Felleman, D. J., and Van Essen, D. C. (1991). Distributed hierarchical processing in the primate cerebral cortex. Cereb. Cortex 1, 1–47.
Fries, P. (2009). Neuronal gamma-band synchronization as a fundamental process in cortical computation. Annu. Rev. Neurosci. 32, 209–224.
Fukai, T., and Tanaka, S. (1997). A simple neural network exhibiting selective activation of neuronal ensembles: from winner-take-all to winners-share-all. Neural. Comput. 9, 77–97.
Gilbert, C. D., and Wiesel, T. N. (1983). Clustered intrinsic connections in cat visual cortex. J. Neurosci. 3, 1116–1133.
Gilbert, C. D., and Wiesel, T. N. (1989). Columnar specificity of intrinsic horizontal and corticocortical connections in cat visual cortex. J. Neurosci. 9, 2432–2442.
Herrero, J. L., Roberts, M. J., Delicato, L. S., Gieselmann, M. A., Dayan, P., and Thiele, A. (2008). Acetylcholine contributes through muscarinic receptors to attentional modulation in V1. Nature 454, 1110–1114.
Itti, L., and Koch, C. (2001). Computational modelling of visual attention. Nat. Rev. Neurosci. 2, 194–203.
Khayat, P. S., Niebergall, R., and Martinez-Trujillo, J. C. (2010). Frequency-dependent attentional modulation of local field potential signals in macaque area MT. J. Neurosci. 30, 7037–7048.
Kimura, R., and Ohzawa, I. (2009). Time course of cross-orientation suppression in the early visual cortex. J. Neurophysiol. 101, 1463–1479.
Kisvárday, Z. F., Tóth, E., Rausch, M., and Eysel, U. T. (1997). Orientation-specific relationship between populations of excitatory and inhibitory lateral connections in the visual cortex of the cat. Cereb. Cortex 7, 605–618.
Klinkenberg, I., Sambeth, A., and Blokland, A. (2011). Acetylcholine and attention. Behav. Brain Res. 221, 430–442.
Lee, D. K., Itti, L., Koch, C., and Braun, J. (1999). Attention activates winner-take-all competition among visual filters. Nat. Neurosci. 2, 375–381.
Lewis, T. J., and Rinzel, J. (2003). Dynamics of spiking neurons connected by both inhibitory and electrical coupling. J. Comput. Neurosci. 14, 283–309.
Li, B., Thompson, J. K., Duong, T., Peterson, M. R., and Freeman, R. D. (2006). Origins of cross-orientation suppression in the visual cortex. J. Neurophysiol. 96, 1755–1764.
Lübke, J., Roth, A., Feldmeyer, D., and Sakmann, B. (2003). Morphometric analysis of the columnar innervation domain of neurons connecting layer 4 and layer 2/3 juvenile rat barrel cortex. Cereb. Cortex 13, 1051–1063.
Luck, S. J., Chelazzi, L., Hillyard, S. A., and Desimone, R. (1997). Neural mechanisms of spatial selective attention in areas V1, V2, and V4 of macaque visual cortex. J. Neurophysiol. 77, 24–42.
Mancilla, J. G., Lewis, T. J., Pinto, D. J., Rinzel, J., and Connors, B. W. (2007). Synchronization of electrically coupled pairs of inhibitory interneurons in neocortex. J. Neurosci. 27, 2058–2073.
Martinez-Trujillo, J. C., and Treue, S. (2004). Feature-based attention increases the selectivity of population responses in primate visual cortex. Curr. Biol. 14, 744–751.
Morrone, M. C., Burr, D. C., and Speed, H. D. (1987). Cross-orientation inhibition in cat is GABA mediated. Exp. Brain Res. 67, 635–644.
Nomura, M., Fukai, T., and Aoyagi, T. (2003). Synchrony of fast-spiking interneurons interconnected by GABAergic and electrical synapses. Neural. Comput. 15, 2179–2198.
Noudoost, B., Chang, M. H., Steinmetz, N. A., and Moore, T. (2010). Top-down control of visual attention. Curr. Opin. Neurobiol. 20, 183–190.
Noudoost, B., and Moore, T. (2011). Control of visual cortical signals by prefrontal dopamine. Nature 474, 372–375.
Ogawa, T., and Komatsu, H. (2004). Target selection in area V4 during a multidimensional visual search task. J. Neurosci. 24, 6371–6382.
Ogawa, T., and Komatsu, H. (2009). Condition-dependent and condition-independent target selection in the macaque posterior parietal cortex. J. Neurophysiol. 101, 721–736.
Peters, A., and Rockland, K. S. (1994). Cerebral Cortex: Primary Visual Cortex in Primates. New York, Plenum press.
Peters, R. J., Iyer, A., Itti, L., and Koch, C. (2005). Components of bottom-up gaze allocation in natural images. Vision Res. 45, 2397–2416.
Posner, M. I., and Petersen, S. E. (1990). The attention system of the human brain. Annu. Rev. Neurosci. 13, 25–42.
Potjans, T. C., and Diesmann, M. (2011). The cell-type specific connectivity of the local cortical network explains prominent features of neuronal activity. Neurons Cogn.arXiv:1106.5678.
Priebe, N. J., and Ferster, D. (2006). Mechanisms underlying cross-orientation suppression in cat visual cortex. Nat. Neurosci. 9, 552–561.
Reid, A. T., Krumnack, A., Wanke, E., and Kötter, R. (2009). Optimization of cortical hierarchies with continuous scales and ranges. Neuroimage 47, 611–617.
Reynolds, J. H., Chelazzi, L., and Desimone, R. (1999). Competitive mechanisms subserve attention in macaque areas V2 and V4. J. Neurosci., 19, 1736–1753.
Reynolds, J. H., and Desimone, R. (2003). Interacting roles of attention and visual salience in V4. Neuron 37, 853–863.
Reynolds, J. H., Pasternak, T., and Desimone, R. (2000). Attention increases sensitivity of V4 neurons. Neuron 26, 703–714.
Rodriguez, R., Kallenbach, U., Singer, W., and Munk, M. H. J. (2004). Short- and long-term effects of cholinergic modulation on gamma oscillations and response synchronization in the visual cortex. J. Neurosci. 24, 10369–10378.
Saalmann, Y. B., and Kastner, S. (2009). Gain control in the visual thalamus during perception and cognition. Curr. Opin. Neurobiol. 19, 408–414.
Salinas, E., and Abbott, L. F. (1996). A model of multiplicative neural responses in parietal cortex. Proc. Natl. Acad. Sci. U.S.A. 93, 11956–11961.
Smith, M. A., Bair, W., and Movshon, J. A. (2006). Dynamics of suppression in macaque primary visual cortex. J. Neurosci. 26, 4826–4834.
Thomson, A. M., and Morris, O. T. (2002). Selectivity in the inter-laminar connections made by neocortical neurones. J. Neurocytol. 31, 239–246.
Thomson, A. M., West, D. C., Wang, Y., and Bannister, A. P. (2002). Synaptic connections and small circuits involving excitatory and inhibitory neurons in layers 2-5 of adult rat and cat neocortex: triple intracellular recordings and biocytin labelling in vitro. Cereb. Cortex 12, 936–953.
Tiesinga, P., and Sejnowski, T. J. (2009). Cortical enlightenment: are attentional gamma oscillations driven by ING or PING? Neuron, 63, 727–732.
Treue, S., and Maunsell, J. H. R. (1999). Effects of attention on the processing of motion in macaque middle temporal and medial superior temporal visual cortical areas. J. Neurosci. 19, 7591–7602.
Wagatsuma, N., Shimizu, R., and Sakai, K. (2008). Spatial attention in early vision for the perception of border ownership. J. Vis. 8, 1–19.
Wang, X. J. (1999). Synaptic basis of cortical persistent activity: the importance of NMDA receptors to working memory. J. Neurosci. 19, 9587–9603.
Womelsdorf, T., Fries, P., Mitra, P. P., and Desimone, R. (2006). Gamma-band synchronization in visual cortex predicts speed of change detection. Nature 439, 733–736.
Keywords: laminar organization, computational model, large-scale simulation, orientation column, visual attention, visual cortex
Citation: Wagatsuma N, Potjans TC, Diesmann M and Fukai T (2011) Layer-dependent attentional processing by top-down signals in a visual cortical microcircuit model. Front. Comput. Neurosci. 5:31. doi: 10.3389/fncom.2011.00031
Received: 05 April 2011;
Accepted: 20 June 2011;
Published online: 08 July 2011.
Edited by:
David Hansel, University of Paris, FranceReviewed by:
Boris Gutkin, Institut Pasteur, FranceChristian Leibold, Ludwig Maximilians University, Germany
Copyright: © 2011 Wagatsuma, Potjans, Diesmann and Fukai. This is an open-access article subject to a non-exclusive license between the authors and Frontiers Media SA, which permits use, distribution and reproduction in other forums, provided the original authors and source are credited and other Frontiers conditions are complied with.
*Correspondence: Nobuhiko Wagatsuma, Laboratory for Neural Circuit Theory, RIKEN Brain Science Institute, 2-1 Hirosawa, Wako, Saitama 351-0198, Japan. e-mail:bndhZ2F0c3VtYUBicmFpbi5yaWtlbi5qcA==