- 1Department of Neurosurgery, Hangzhou First People's Hospital, School of Medicine, Zhejiang University, Hangzhou, China
- 2Department of Neurosurgery, The Second Affiliated Hospital, School of Medicine, Zhejiang University, Hangzhou, China
- 3Department of Neurosurgery, The Fourth Affiliated Hospital, School of Medicine, Zhejiang University, Yiwu, China
Background: This study sought to develop and validate a dynamic nomogram chart to assess the risk of acute kidney injury (AKI) in patients with acute ischemic stroke (AIS).
Methods: These data were drawn from the Medical Information Mart for Intensive Care III (MIMIC-III) database, which collects 47 clinical indicators of patients after admission to the hospital. The primary outcome indicator was the occurrence of AKI within 48 h of intensive care unit (ICU) admission. Independent risk factors for AKI were screened from the training set using univariate and multifactorial logistic regression analyses. Multiple logistic regression models were developed, and nomograms were plotted and validated in an internal validation set. Based on the receiver operating characteristic (ROC) curve, calibration curve, and decision curve analysis (DCA) to estimate the performance of this nomogram.
Results: Nomogram indicators include blood urea nitrogen (BUN), creatinine, red blood cell distribution width (RDW), heart rate (HR), Oxford Acute Severity of Illness Score (OASIS), the history of congestive heart failure (CHF), the use of vancomycin, contrast agent, and mannitol. The predictive model displayed well discrimination with the area under the ROC curve values of 0.8529 and 0.8598 for the training set and the validator, respectively. Calibration curves revealed favorable concordance between the actual and predicted incidence of AKI (p > 0.05). DCA indicates the excellent net clinical benefit of nomogram in predicting AKI.
Conclusion: In summary, we explored the incidence of AKI in patients with AIS during ICU stay and developed a predictive model to help clinical decision-making.
Introduction
Stroke remains the second most common disease threatening humans worldwide (1). Stroke places a tremendous burden on patients, families, and society, especially in developing countries (2). The American Heart Association and the American Stroke Association project that the total medical cost of stroke will reach $1,841 billion from 2012 to 2030 (3). Ischemic stroke, usually caused by a blood clot blocking an artery in the brain, is the leading type of stroke (4). Tissue plasminogen activator (tPA) is the only drug currently approved by the United States Food and Drug Administration (FDA) for the treatment of ischemic strokes. Patients outside of the time window for tPA use may be treated with other therapies, such as thrombectomy, anticoagulants, blood pressure-lowering, and cholesterol-lowering drugs. Nevertheless, the effectiveness of treatment for ischemic stroke is unsatisfactory. Stroke kills ~6 million people each year, accounting for more than 10% of all deaths (5). Therefore, in the management of ischemic stroke, early identification of patients who may have a poor prognosis and timely intervention is important to improve the quality of patient survival later in life.
Acute kidney injury (AKI) is a common condition in critically ill patients and occurs in ~30–50% of intensive care unit (ICU) patients (6). High mortality is one of its characteristics, with ~20–25% of patients dying during hospitalization (7, 8). AKI manifests itself as a dramatic deterioration of renal function and a decrease in urine output, which leads to water and electrolyte disturbances and high circulating blood volume, resulting in a series of negative effects on other organs (9). After the stroke, activation of the sympathetic nervous system, the renin-angiotensin-aldosterone pathway impairs the self-regulatory function of the kidney (10). Moreover, the immune response induced by stroke leads to a massive release of inflammatory factors, resulting in decreased renal function (11). Therefore, early identification, recognition, and intervention can reduce the probability of AKI and slow down its progression (12).
However, there are no validated clinical models to predict the occurrence of AKI in patients with acute ischemic stroke (AIS). Therefore, there is a need to effectively predict the occurrence of AKI or screen for potential risk factors to guide early clinical intervention to improve prognosis. Based on several key variables and parameters, nomograms, especially dynamic nomograms, provide a powerful and easy-to-use method to predict the outcomes of individual events (13–16). The main objective of this study was to identify factors that independently predict the occurrence of AKI in patients with AIS. The patient cohort and factors were selected from the Medical Information Mart for Intensive Care III (MIMIC-III) database, and the nomogram was concurrently developed to predict the incidence of AKI in the AIS population during ICU (17).
Materials and methods
Data source
All data for this study were obtained from a publicly available, large critical care database named MIMIC-III (17). It included 46,520 patients who were admitted to the ICU at Beth Israel Deaconess Medical Center between June 2001 and October 2012. The study was approved by the Institutional Review Board of Beth Israel Deaconess Medical Center and the Massachusetts Institute of Technology. Because data from the MIMIC-III study are publicly available and patient's personal information has been anonymized, the requirement to obtain individual patient consent was waived. After passing an exam called Protecting Human Research Participants (certification number 43391018), we were granted access to the database and extracted data using the structured query language of PostgreSQL 9.6.
Study patients
Patients admitted with a diagnosis of AIS, defined as the International Classification of Diseases-Ninth (ICD-9) codes 43301, 43311, 43321, 43331, 43381, 43391, 43401, 43411, and 43491, were included in the study population. AKI was diagnosed using The Kidney Disease Improving Global Outcomes (KDIGO) criteria (18): blood creatinine ≥26.5 μmol/l increased within 48 h or increased to 1.5 times the baseline level and urine output <0.5 ml/kg/h for more than 6 h. For patients with multiple admission records, only information on the first ICU admission was retained. The age range was set at 18–89 years because the actual age of patients older than 89 years was not available. People receiving renal replacement therapy or continuous renal replacement therapy and those with a prior history of renal failure or chronic kidney disease were excluded. In addition, patients with an ICU stay of <24 h were also excluded. Ultimately, 1,132 patients were entered into the study and randomized in a 7:3 ratio into the training set (n = 792) and validation set (n = 340).
Data extraction
All data were extracted within 24 h of the patient's admission to the ICU. The variables extracted included demographic characteristics, vital signs, interventions, comorbidities, laboratory indicators, and scoring systems (19, 20). Demographic characteristics included age, sex, race, and type of admission. Vital signs measured on admission for the first time were recorded, such as heart rate (HR), respiratory rate (RR), systolic blood pressure (SBP), diastolic blood pressure (DBP), body temperature, and peripheral hemoglobin oxygen saturation (SPO2). Interventions, such as vasopressin, mannitol, colloidal injections, aminoglycoside antibiotics, vancomycin, mechanical ventilation, angiotensin-converting enzyme inhibitors (ACEIs), angiotensin receptor blockers (ARBs), intravenous thrombolysis, and endovascular mechanical thrombectomy, were recorded within 24 h of admission. Secondary prevention medications for ischemic stroke were also documented that included antiplatelet agents (e.g., aspirin, clopidogrel, ticlopidine, cilostazol, tegretol, prasugrel, and disulfiram) and anticoagulants (e.g., warfarin, dabigatran, apixaban, rivaroxaban, and edoxaban). Laboratory parameters, such as hemoglobin, white blood cell (WBC), platelet count, blood glucose, sodium, potassium, blood urea nitrogen (BUN), red blood cell distribution width (RDW), prothrombin time (PT), and activated partial thromboplastin time (APTT), recorded in the laboratory event table. In addition, the Oxford Acute Severity of Illness Score (OASIS) and ICU length of stay (ICUlos) were recorded. OASIS is a new score developed using machine-learning algorithms that have been widely used in prognostic prediction models for a range of diseases (21, 22). All comorbidities were identified according to the ICD-9 code records.
Statistical analysis
Continuous variables were compared by t-test and Kruskal-Wallis rank sum test, expressed as median and quartiles (Q1 and Q3). Categorical variables were compared using the χ2 test or Fisher's exact test. The presence of multicollinearity between covariates was determined by the variance inflation factor (VIF). VIF ≥ 5 was considered to have multicollinearity (23). Univariate logistic analysis was used to assess the association between covariates and outcomes. Variables with p < 0.05 were included in the multifactorial regression. In a stepwise backward regression according to the Akaike Information Criterion (AIC), the optimal model holds the minimum AIC value. A nomogram predicting the risk of AKI in patients with AIS was created based on the results of the multifactorial regression. The performance of the nomogram was verified in the validation set using the following methods. The area under the curve (AUC) of the receiver operating characteristic (ROC) curve was used to evaluate the discriminatory ability of the nomogram. Calibration plots and Hosmer-Lemeshow goodness-of-fit tests (HL tests) were used by comparing the accuracy of the nomogram. Decision curve analysis (DCA) was performed to evaluate the clinical applicability of the nomogram. p < 0.05 was considered to be statistically significant. All statistical analyses were performed with R statistical software (V.4.1.2) and Stata (V.16.0). The flow chart of the study is shown in Figure 1.
Results
Baseline characteristics
The median age of patients with AKI was 72.2 (59.3 and 81.3), older than patients with non-AKI at 68.5 (55.9 and 78.8). The baseline characteristics of all patients are shown in Table 1. In terms of comorbidities, patients with AKI were more likely to have congestive heart failure (CHF), liver disease, coagulopathy, and diabetes than patients with non-AKI. In terms of therapeutic measures received, the AKI group received more aminoglycoside antibiotics, furosemide, vancomycin, antiplatelet agent, and mechanical ventilation as compared to the non-AKI group. In addition, the AKI group received contrast-related tests more frequently, whereas the non-AKI group was more likely to receive intravenous thrombolysis. Furthermore, patients with AKI had higher HR, RR, blood glucose, creatinine, BUN, red blood cell width (RDW), OASIS, and ICUlos and lower SBP, DBP, urine output, and hemoglobin. Comparatively, patients in the non-AKI group usually had higher SBP, DBP, urine output, and hemoglobin. Similar results were obtained in the training set (Table 2). There was no significant difference in the baseline characteristics of the training and validation set populations for each clinical factor as shown in Supplementary material S1. The number of people with AKI was 104 (13.13%) and 48 (14.12%), respectively.
Variables selection and nomogram construction
Multiple covariance tests revealed no significant covariance among the variables as shown in Supplementary material S2, so all variables were included in the univariate logistic regression. Based on the univariate regression results, 29 variables were significantly associated with the occurrence of AKI in patients with AIS (Table 3). Then, based on AIC, 9 factors were selected: BUN, creatinine, RDW, HR, the use of vancomycin, contrast agent, mannitol agent, the history of CHF, and OASIS. Multivariate logistic regression analysis showed risk factors that were independently associated with the development of AKI in patients with AIS (Table 4). A model was constructed using the above 9 factors, on the basis of which a nomogram was constructed to predict the risk of AKI in patients with AIS (Figure 2). In addition, the dynamic nomogram can be accessed through https://dynnomo.shinyapps.io/PREDICTAKI/.
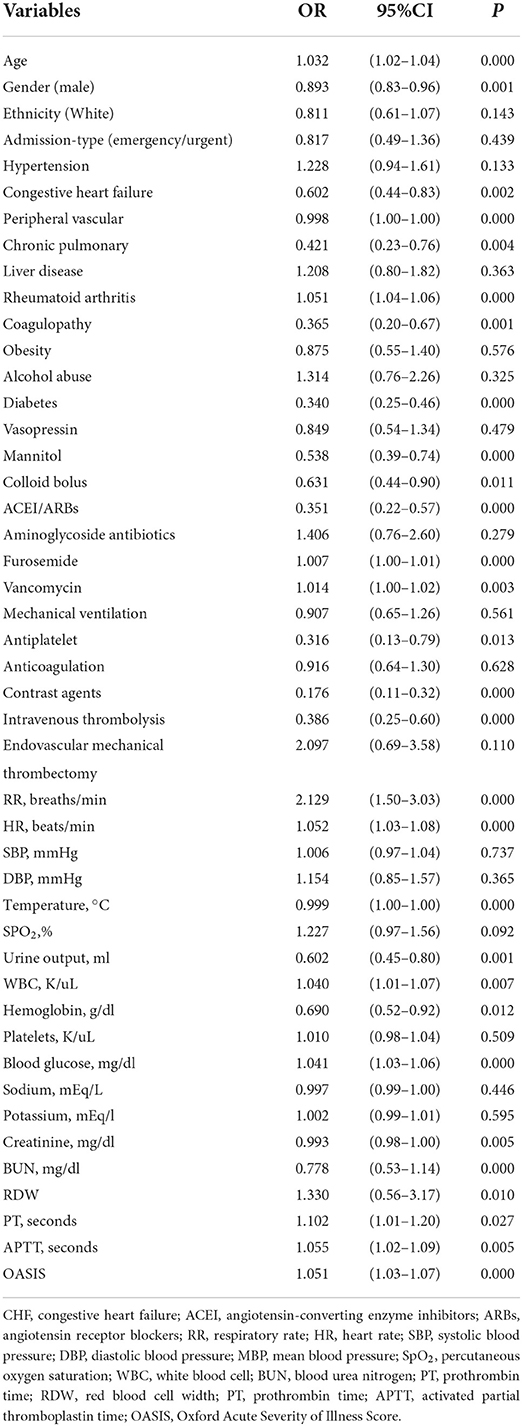
Table 3. Univariate logistic regression analysis of predictive variables of acute kidney injury (AKI) in the training cohort.
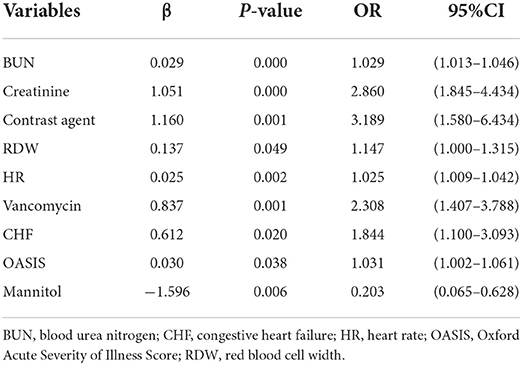
Table 4. Multivariate logistic regression analysis of candidate factors of acute kidney injury (AKI) in the training cohort.
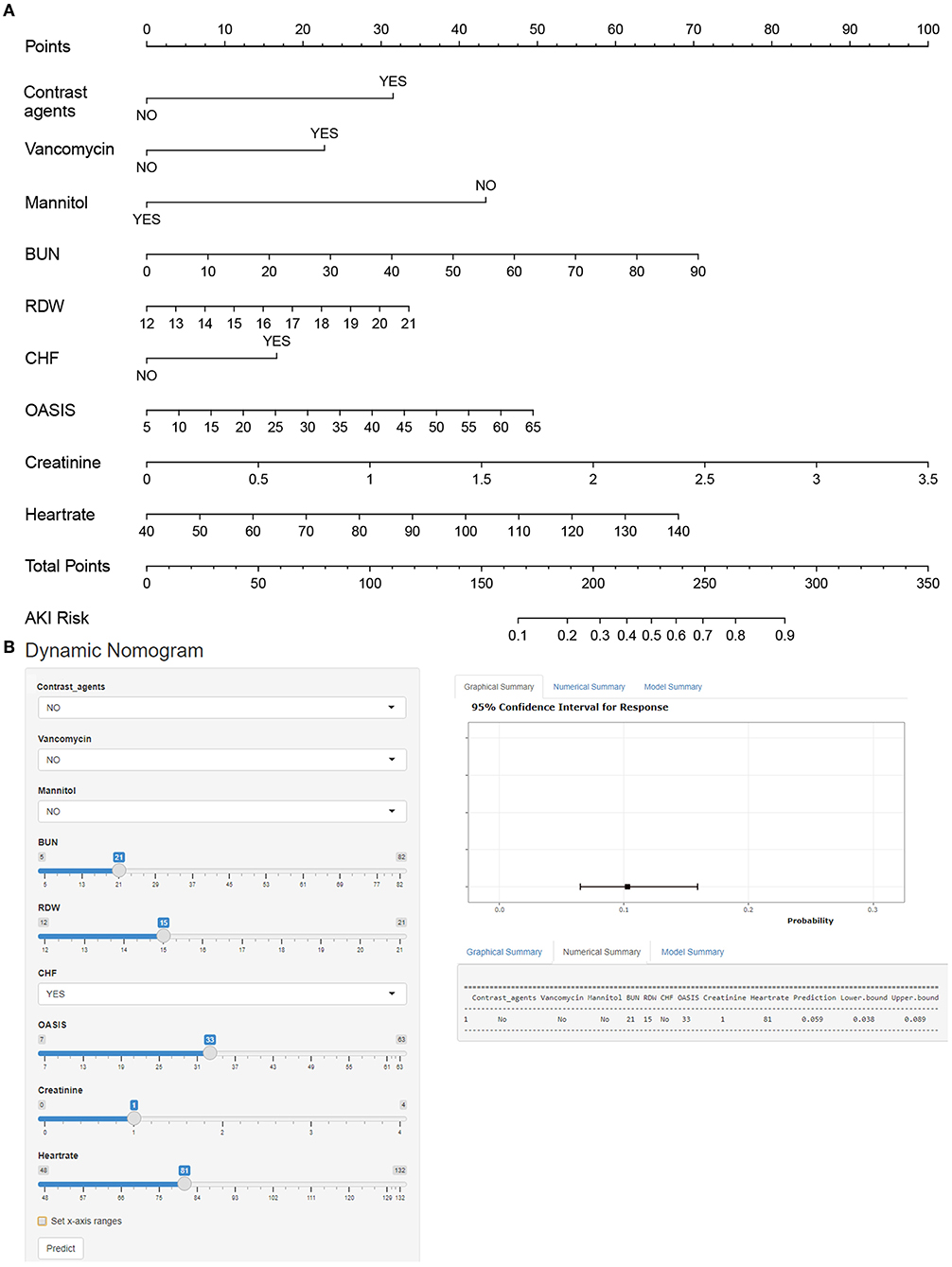
Figure 2. (A) Nomogram to identify the risk of acute kidney injury (AKI) in patients with AIS, based on logistic regression analysis. To acquire the corresponding scores for each variable, draw a vertical line upward to the “Points” axis. Sum the score for all predictors and locate the final value on the “Total Points” axis. Draw a line straight down to the “Risk” axis to determine the risk of AKI. (B) Dynamic nomogram line graph interface. BUN, blood urea nitrogen; RDW, red blood cell width; CHF, congestive heart failure; OASIS, Oxford Acute Severity of Illness Score.
Nomogram validation
Nomograms showed good differentiating value for combined AKI in patients with AIS. The AUC values of AKI plots in the training and validation sets were 0.8529 (95% CI: 0.8036–0.8954) and 0.8598 (95% CI: 0.8017–0.8806), respectively (Figure 3). The calibration curves showed good calibration for both cohorts (Figure 4). In addition, the p-value of the Hosmer-Lemeshow goodness-of-fit test in the training set was 0.8814, and the corresponding value in the validation set was 0.4835. Both the calibration curve and the Hosmer-Lemeshow goodness-of-fit test indicated that the predicted results were highly consistent with the actual results. The DCA of the AKI nomogram was performed in both the training (Figure 5A) and validation sets (Figure 5B). The horizontal axis indicates that no one received the intervention, and the net gain was 0. The diagonal line indicates that everyone received the intervention. The net returns range from 0 to 12% and 0 to 13% in the training and validation sets when the prediction thresholds are in the range of 0–100% and 0–64%, respectively. The smaller the threshold, the greater the net gain. Interventions that avoided curves show a higher net reduction in interventions per 100 patients as the prediction threshold increases in the range of 0.01–1 in both the training (Figure 5C) and validation sets (Figure 5D). For example, in the training set, a prediction threshold of 0.24 reduces the intervention rate for patients by 60% without missing a diagnosis. The DCA and interventions that avoided curves visualize that the nomogram has significant predictive value.
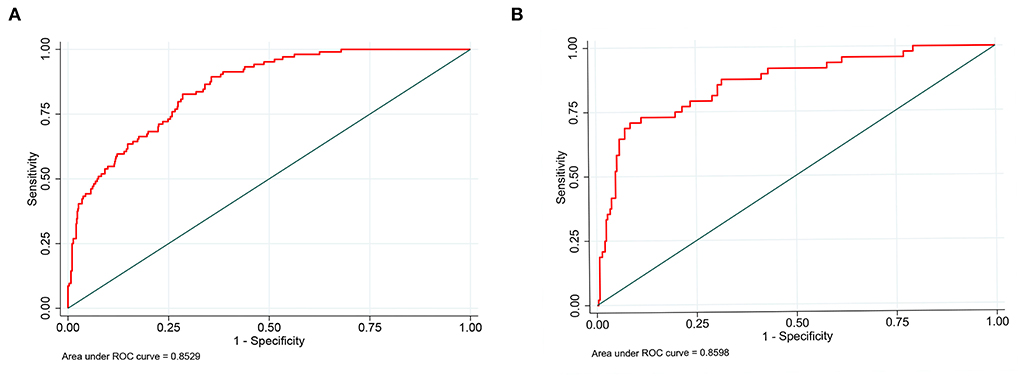
Figure 3. Receiver operating characteristic curve of the nomogram. The an area under the curve (AUC) of the nomogram for the prediction of acute kidney injury (AKI) in patients with AIS was 0.8529 in the training set (A) and 0.8598 in the validation set (B).
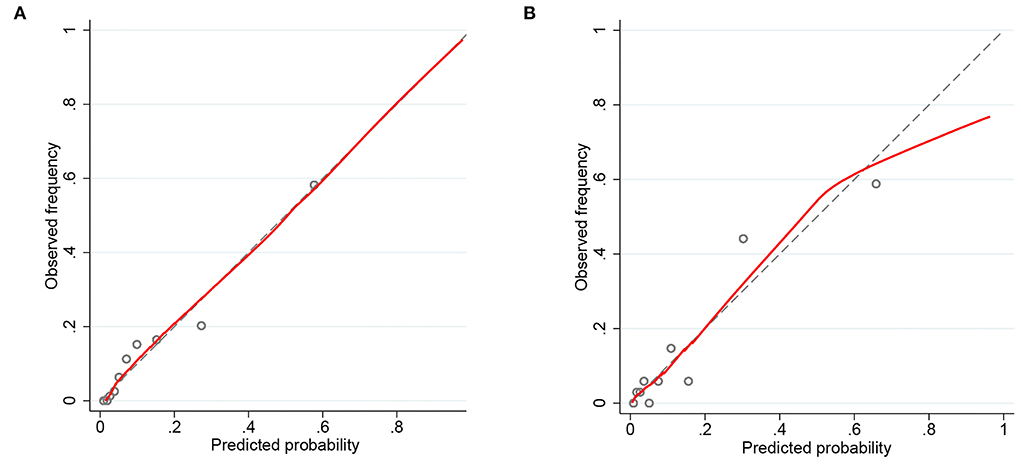
Figure 4. Calibration curves of the predicted nomogram in the training set (A) and validation set (B). The x-axis represents the predicted probability calculated by the nomogram, and the y-axis is the observed actual probability of acute kidney injury (AKI). Results of the Hosmer-Lemeshow test demonstrate that the p-value of the training set is 0.8814 and the validation set is 0.4835, respectively.
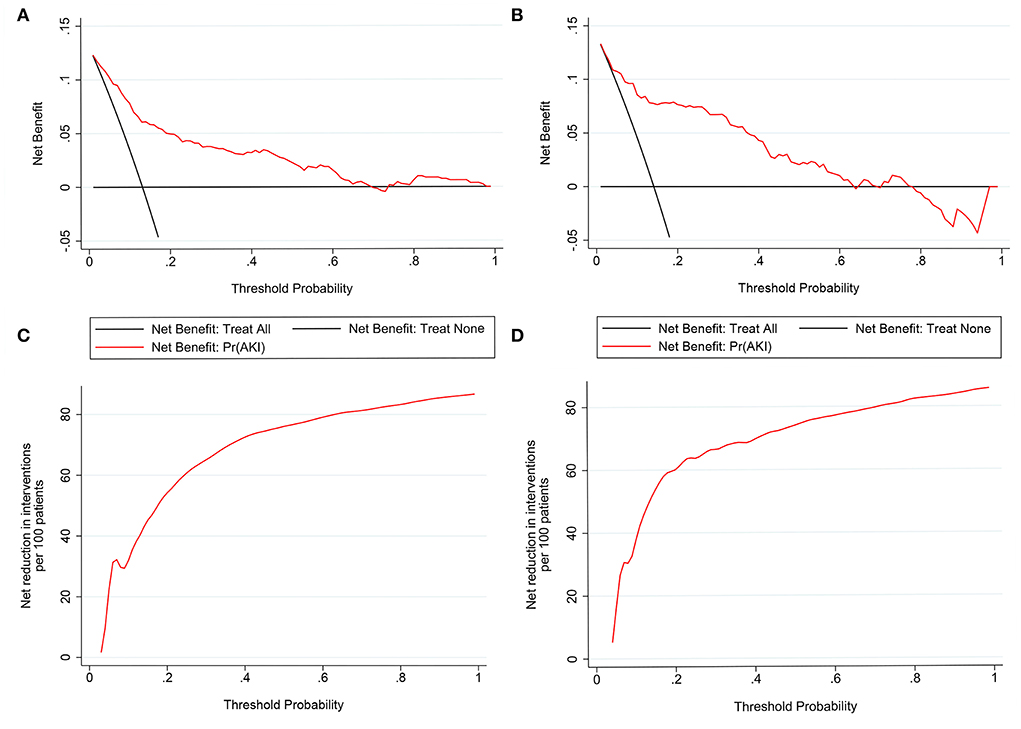
Figure 5. Decision curve analysis (DCA) of the nomogram in the training set (A) and the validation set (C). The horizontal line indicates no patients develop acute kidney injury (AKI), and the gray oblique line indicates patients develop AKI. The blue solid line represents the AKI risk nomogram. In DCA, the nomogram shows a more net benefit than full or no treatment across a threshold probability range. Interventions avoided the curve of the nomogram in the training set (B) and the validation set (D). The curve represents the net reduction in the number of interventions per 100 patients at a given threshold.
Discussion
This study predicts the short-term probability of AKI in patients with AIS by using routine information available in the ICU to construct a simple and easy-to-use nomogram. Patients with AKI receive antibiotic therapy and mechanical ventilation more frequently and have longer ICU stays, suggesting that patients with AKI typically suffer more adverse effects and financial burden. A previous study on patients with sepsis constructed a predictive model for AKI that included 5 indicators, such as baseline serum creatinine and glucose. The C index of the nomogram was 0.752, which had a good predictive performance. In addition, the prediction model was also developed to predict the risk of AKI in patients with diabetic ketoacidosis. The predictors used to construct the nomogram included diabetes, obesity, simplified acute physiology score II, bilirubin, creatinine, and BUN. The model has good discriminatory power, with area under the ROC curve values of 0.756 and 0.760 in the training and validation cohorts, respectively. Recently, Liu et al. constructed a glycemic-based predictive model for predicting the occurrence of AKI in stroke patients treated with endovascular treatment (EVT) to provide evidence for the glycemic management of patients (24). They screened stroke patients receiving EVT and included many glycemic-related factors, such as acute glycemia, hemoglobin bA1c (HbA1c), and average chronic glycemia, from the perspective of glycemic management. The study is more focused, especially on stroke patients with diabetes mellitus. However, it is important to construct a more generalizable predictive model for all patients with AIS. Therefore, our nomogram identifies variables that are easily accessible in the clinical setting. Moreover, the portability of the dynamic nomogram makes it possible for clinicians to predict the probability of AKI in patients with AIS at any time. The construction of the nomogram was performed well in terms of predictive performance, calibration, and clinical application, providing valuable information for selecting individualized treatment plans for different patients. More importantly, it bridges the gap between the high incidence of AKI after ischemic stroke and the absence of credible predictive modeling.
Our results showed that the probability of AKI in patients with AISS was 13.43%, with an incidence similar to that reported in previously published studies (25–27). Based on a meta-analysis in the United Kingdom, the combined incidence of AKI after ischemic stroke was 9.62% (28). Two pathways typically exist to influence the development of AKI after brain injury, such as neuroendocrine pathways, along with inflammatory and immune pathways. After a stroke, the hypothalamic-pituitary-adrenal axis activates, stimulating the adrenal glands to release glucocorticoids into the bloodstream (29). Prolonged high levels of hormonal state impair glomerular and tubular function. In addition, the sympathetic excitation that accompanies a stroke promotes the release of catecholamines from the adrenal glands, resulting in increased norepinephrine levels. Although the hyperadrenergic state facilitates the delivery of adequate oxygen to damaged tissues, and renal vasoconstriction, decreased renal perfusion, and reduced glomerular filtration rate appear to be unavoidable (30). Various studies in vivo and in vitro suggest that the renin-angiotensin-aldosterone system (RAAS) may play an important role in the development of kidney injury (31). Furthermore, the autoregulatory function of the kidney is impaired after stroke. Studies have shown a significant correlation between a decrease in estimated glomerular filtration rate (eGFR) and a decrease in autoregulation in the brain (32). Secondary alterations, such as hemorrhagic transformation and the presence of cerebral edema, may lead to worse stroke outcomes. Similarly, they have also been shown to be connected with reduced eGFR and poorer cerebral autoregulation (33). On the other hand, the compromised blood-brain barrier allows inflammatory cells that enter the brain to enter further into the systemic circulation, thereby causing secondary systemic inflammation (30). Infiltration of immune cells (M1-type macrophages) and activation of inflammatory factors (C-reactive protein, interleukin (IL)-6, IL-1β, reactive oxygen species [ROS], etc.) are important factors in the development of AKI (34).
Logistic regression analysis showed that BUN, creatinine, RDW, HR, the use of vancomycin, contrast agents, mannitol agent, the history of CHF, and OASIS were significantly associated with the occurrence of AKI in patients with AIS. AKI is characterized by a rapid decline in renal function or the development of significant renal failure accompanied by a significant increase in BUN and creatinine (35). Despite being susceptible to interference by age, sex, race, and weight, BUN and creatinine remain the most commonly used biomarkers of impaired kidney function. Studies have noted that mild increments in BUN and creatinine are associated with an increased incidence of AKI in patients in the ICU. In addition, patients with higher creatinine typically have longer hospital stays and higher mortality rates (36). In addition, it is inevitable that stroke patients need to undergo examinations with contrast agents, such as iodinated alcohol. Contrast-induced AKI is a very common cause of hospital-acquired AKI and usually occurs within 48–72 h after contrast injection (37). Although the mechanism is not fully understood, contrast agents eventually lead to epithelial and endothelial cell apoptosis, which in turn causes a decrease in glomerular filtration rate. Adequate blood volume expansion and the use of hypotonic contrast media can help to prevent AKI (38). CHF leads to complex interactions between the heart and the kidneys (39). Patients with a history of CHF are usually at increased risk of AKI due to inadequate renal reserve function and reduced renal perfusion (40). Moreover, our study shows that patients with AIS who develop AKI are usually associated with a higher HR. An excessively fast HR decreases cardiac output per beat, which decreases renal blood perfusion and fosters the development of AKI (41). Mannitol is an osmotic diuretic that is commonly used to relieve cerebral edema after cerebrovascular accidents. Despite conflicting data, mannitol is still commonly used in clinical practice to prevent AKI (42). Fisher et al. showed that patients' renal function is preserved when mannitol is administered in open-heart surgery, which may be associated with reduced cell swelling and deposition of cellular debris in the renal tubules (43). Further studies are needed in the future to determine the specific mechanisms by which mannitol is used to prevent AKI. OASIS reflects the severity of the patient's condition and may be an independent predictor of mortality 1 year after ICU admission. Similar to previous reports in the literature (44), our study showed that patients in the AKI group had higher OASIS scores than the patients with non-AKI and that higher OASIS scores were associated with a higher risk of developing AKI during ICU. RDW has value in predicting AKI in patients treated in a coronary care unit and in patients undergoing initial percutaneous coronary intervention (45, 46). The underlying mechanisms between RDW and AKI have not been completely elucidated, and the levels of systemic inflammation and oxidative stress in the kidney and systemic nutritional status reflected by RDW may be related to the development of AKI (47).
Here we give an example to illustrate how to use a nomogram. Suppose a patient with AIS, with a history of CHF, who was treated with vancomycin and mannitol during the treatment period, had an HR of 90 beats per min, an RDW of 18, a BUN of 20 mg/dl, a creatinine of 1 mg/dl, and an OASIS score of 30. According to Figure 2, the score corresponding to each individual parameter is obtained from the first row (the “Point” axis). Finally, the overall score was calculated as the sum of points for all parameters [30 (contrast agents) + 15 (CHF) + 0 (mannitol) + 21 (vancomycin) + 35 (HR) + 21 (RDW) + 27 (creatinine) + 23 (BUN) + 20 (OASIS) = 192]. This score corresponded to a risk of developing AKI at an ~22.3% level. More notably, the dynamic nomogram eliminates this step of the calculation. All that is required is to manually enter the patient's various laboratory parameters, medical history, and treatment received, depending on the actual situation. A dynamic nomogram will automatically output predicted values for the occurrence of AKI.
There are some limitations to this study. First, the patients included in the study were from only one medical center from 2001 to 2012, and the selection bias of the population and the continuous updating of treatment regimens may lead to a decrease in predictive performance, and multi-center data may be needed to validate the accuracy of the model in the future. Second, the data in this study were collected within 24 h of ICU admission, where there may be differences in laboratory variables between the emergency department and the general ward. Therefore, further studies are needed to confirm the validity of our findings. Finally, we constructed the nomogram. Since the nomogram was constructed based on 13 indicators, the sensitivity of the model performance may be reduced if data for one or two indicators of a patient are missing.
Conclusion
Blood urea nitrogen, creatinine, red blood cell distribution width, heart rate, Oxford Acute Severity of Illness Score, the history of congestive heart failure, the use of vancomycin, contrast agent, and mannitol agents were identified as risk factors for AKI in patients with AIS. Furthermore, a dynamic nomogram was developed based on multivariate logistic regression of these factors. This is a simple scoring method to predict the risk of AKI in patients with AIS and helps to identify and intervene in high-risk patients earlier.
Data availability statement
The data analyzed in this study was obtained from the Medical Information Mart for Intensive Care III (MIMIC-III) Clinical Database, the following licenses/restrictions apply: To access the data you must be a credentialed user, complete the required training (CITI Data or Specimens Only Research) and sign the data use agreement for the project. Requests to access these datasets should be directed to PhysioNet, https://physionet.org/, https://doi.org/10.13026/C2XW26.
Ethics statement
Ethical review and approval was not required for the study on human participants in accordance with the local legislation and institutional requirements. Written informed consent for participation was not required for this study in accordance with the national legislation and the institutional requirements.
Author contributions
GZ: conceptualization, data curation, and writing—original draft. ZF: investigation. TJ: methodology. XX and JW: software and supervision. LC: validation. WY: writing—review and editing. All authors contributed to the article and approved the submitted version.
Acknowledgments
The authors would like to thank everyone who participated in the creation of the MIMIC database.
Conflict of interest
The authors declare that the research was conducted in the absence of any commercial or financial relationships that could be construed as a potential conflict of interest.
Publisher's note
All claims expressed in this article are solely those of the authors and do not necessarily represent those of their affiliated organizations, or those of the publisher, the editors and the reviewers. Any product that may be evaluated in this article, or claim that may be made by its manufacturer, is not guaranteed or endorsed by the publisher.
Supplementary material
The Supplementary Material for this article can be found online at: https://www.frontiersin.org/articles/10.3389/fneur.2022.987684/full#supplementary-material
Supplementary material S1. Baseline characteristics of the training and validation set populations.
Supplementary material S2. Results of multiple covariance tests.
References
1. Markus HS. the global burden of stroke, and fluoxetine for stroke recovery. Int J Stroke. (2020) 15:355. doi: 10.1177/1747493020919047
2. Collaborators GBDN. Global, regional, and national burden of neurological disorders, 1990–2016: a systematic analysis for the global burden of disease study 2016. Lancet Neurol. (2019) 18:459–80. doi: 10.1016/S1474-4422(18)30499-X
3. Rajsic S, Gothe H, Borba HH, Sroczynski G, Vujicic J, Toell T, et al. Economic burden of stroke: a systematic review on post-stroke care. Eur J Health Econ. (2019) 20:107–34. doi: 10.1007/s10198-018-0984-0
4. Barthels D, Das H. Current advances in ischemic stroke research and therapies. Biochim Biophys Acta Mol Basis Dis. (2020) 1866:165260. doi: 10.1016/j.bbadis.2018.09.012
5. Zhang SR, Phan TG, Sobey CG. Targeting the immune system for ischemic stroke. Trends Pharmacol Sci. (2021) 42:96–105. doi: 10.1016/j.tips.2020.11.010
6. Lewington AJ, Cerda J, Mehta RL. Raising awareness of acute kidney injury: a global perspective of a silent killer. Kidney Int. (2013) 84:457–67. doi: 10.1038/ki.2013.153
7. Susantitaphong P, Cruz DN, Cerda J, Abulfaraj M, Alqahtani F, Koulouridis I, et al. World incidence of Aki: a meta-analysis. Clin J Am Soc Nephrol. (2013) 8:1482–93. doi: 10.2215/CJN.00710113
8. Selby NM, Kolhe NV, McIntyre CW, Monaghan J, Lawson N, Elliott D, et al. Defining the cause of death in hospitalised patients with acute kidney injury. PLoS ONE. (2012) 7:e48580. doi: 10.1371/journal.pone.0048580
9. Clec'h C, Darmon M, Lautrette A, Chemouni F, Azoulay E, Schwebel C, et al. Efficacy of renal replacement therapy in critically ill patients: a propensity analysis. Crit Care. (2012) 16:R236. doi: 10.1186/cc11905
10. Zorrilla-Vaca A, Ziai W, Connolly ES Jr, Geocadin R, Thompson R, Rivera-Lara L. Acute kidney injury following acute ischemic stroke and intracerebral hemorrhage: a meta-analysis of prevalence rate and mortality risk. Cerebrovasc Dis. (2018) 45:1–9. doi: 10.1159/000479338
11. Pesonen A, Ben-Hamouda N, Schneider A. Acute kidney injury after brain injury: does it exist? Minerva Anestesiol. (2021) 87:823–7. doi: 10.23736/S0375-9393.20.14991-5
12. Kashani K, Ronco C. Acute kidney injury electronic alert for nephrologist: reactive versus proactive? Blood Purif. (2016) 42:323–8. doi: 10.1159/000450722
13. Pan Z, You H, Bu Q, Feng X, Zhao F, Li Y, et al. Development and validation of a nomogram for predicting cancer-specific survival in patients with Wilms' tumor. J Cancer. (2019) 10:5299–305. doi: 10.7150/jca.32741
14. Fan T, Wang H, Wang J, Wang W, Guan H, Zhang C. Nomogram to predict the risk of acute kidney injury in patients with diabetic ketoacidosis: an analysis of the mimic-iii database. BMC Endocr Disord. (2021) 21:37. doi: 10.1186/s12902-021-00696-8
15. Deng H, Yu X, Gao K, Liu Y, Tong Z, Liu Y, et al. Dynamic nomogram for predicting thrombocytopenia in adults with acute pancreatitis. J Inflamm Res. (2021) 14:6657–67. doi: 10.2147/JIR.S339981
16. Wang ZM, Swierzy M, Balke D, Nachira D, Gonzalez-Rivas D, Badakhshi H, et al. Dynamic nomogram for long-term survival in patients with non-small cell lung cancer after pneumonectomy. J Thorac Dis. (2021) 13:2276–87. doi: 10.21037/jtd-20-3203
17. Johnson AE, Pollard TJ, Shen L, Lehman LW, Feng M, Ghassemi M, et al. Mimic-iii, a freely accessible critical care database. Sci Data. (2016) 3:160035. doi: 10.1038/sdata.2016.35
18. Thomas ME, Blaine C, Dawnay A, Devonald MA, Ftouh S, Laing C, et al. The definition of acute kidney injury and its use in practice. Kidney Int. (2015) 87:62–73. doi: 10.1038/ki.2014.328
19. Yang J, Li Y, Liu Q, Li L, Feng A, Wang T, et al. Brief introduction of medical database and data mining technology in big data era. J Evid Based Med. (2020) 13:57–69. doi: 10.1111/jebm.12373
20. Wu WT, Li YJ, Feng AZ, Li L, Huang T, Xu AD, et al. Data mining in clinical big data: the frequently used databases, steps, and methodological models. Mil Med Res. (2021) 8:44. doi: 10.1186/s40779-021-00338-z
21. El-Manzalawy Y, Abbas M, Hoaglund I, Cerna AU, Morland TB, Haggerty CM, et al. Oasis +: leveraging machine learning to improve the prognostic accuracy of oasis severity score for predicting in-hospital mortality. BMC Med Inform Decis Mak. (2021) 21:156. doi: 10.1186/s12911-021-01517-7
22. Wang L, Zhang Z, Hu T. Effectiveness of lods, oasis, and saps ii to predict in-hospital mortality for intensive care patients with st elevation myocardial infarction. Sci Rep. (2021) 11:23887. doi: 10.1038/s41598-021-03397-3
23. Shen Y, Huang X, Zhang W. Platelet-to-lymphocyte ratio as a prognostic predictor of mortality for sepsis: interaction effect with disease severity-a retrospective study. BMJ Open. (2019) 9:e022896. doi: 10.1136/bmjopen-2018-022896
24. Liu C, Li X, Xu Z, Wang Y, Jiang T, Wang M, et al. Construction of a glycaemia-based signature for predicting acute kidney injury in ischaemic stroke patients after endovascular treatment. J Clin Med. (2022) 11. doi: 10.3390/jcm11133865
25. Covic A, Schiller A, Mardare NG, Petrica L, Petrica M, Mihaescu A, et al. The impact of acute kidney injury on short-term survival in an Eastern European population with stroke. Nephrol Dial Transplant. (2008) 23:2228–34. doi: 10.1093/ndt/gfm591
26. Tsagalis G, Akrivos T, Alevizaki M, Manios E, Theodorakis M, Laggouranis A, et al. Long-term prognosis of acute kidney injury after first acute stroke. Clin J Am Soc Nephrol. (2009) 4:616–22. doi: 10.2215/CJN.04110808
27. Khatri M, Himmelfarb J, Adams D, Becker K, Longstreth WT, Tirschwell DL. Acute kidney injury is associated with increased hospital mortality after stroke. J Stroke Cerebrovasc Dis. (2014) 23:25–30. doi: 10.1016/j.jstrokecerebrovasdis.2012.06.005
28. Arnold J, Ng KP, Sims D, Gill P, Cockwell P, Ferro C. Incidence and impact on outcomes of acute kidney injury after a stroke: a systematic review and meta-analysis. BMC Nephrol. (2018) 19:283. doi: 10.1186/s12882-018-1085-0
29. Mracsko E, Liesz A, Karcher S, Zorn M, Bari F, Veltkamp R. Differential effects of sympathetic nervous system and hypothalamic-pituitary-adrenal axis on systemic immune cells after severe experimental stroke. Brain Behav Immun. (2014) 41:200–9. doi: 10.1016/j.bbi.2014.05.015
30. Khalid F, Yang GL, McGuire JL, Robson MJ, Foreman B, Ngwenya LB, et al. Autonomic dysfunction following traumatic brain injury: translational insights. Neurosurg Focus. (2019) 47:E8. doi: 10.3171/2019.8.FOCUS19517
31. Lopez-Novoa JM, Rodriguez-Pena AB, Ortiz A, Martinez-Salgado C, Lopez Hernandez FJ. Etiopathology of chronic tubular, glomerular and renovascular nephropathies: clinical implications. J Transl Med. (2011) 9:13. doi: 10.1186/1479-5876-9-13
32. Castro P, Azevedo E, Rocha I, Sorond F, Serrador JM. Chronic kidney disease and poor outcomes in ischemic stroke: is impaired cerebral autoregulation the missing link? BMC Neurol. (2018) 18:21. doi: 10.1186/s12883-018-1025-4
33. Castro P, Azevedo E, Serrador J, Rocha I, Sorond F. Hemorrhagic transformation and cerebral edema in acute ischemic stroke: link to cerebral autoregulation. J Neurol Sci. (2017) 372:256–61. doi: 10.1016/j.jns.2016.11.065
34. Zhao Q, Yan T, Chopp M, Venkat P, Chen J. Brain-kidney interaction: renal dysfunction following ischemic stroke. J Cereb Blood Flow Metab. (2020) 40:246–62. doi: 10.1177/0271678X19890931
35. Andreucci M, Faga T, Pisani A, Perticone M, Michael A. The ischemic/nephrotoxic acute kidney injury and the use of renal biomarkers in clinical practice. Eur J Intern Med. (2017) 39:1–8. doi: 10.1016/j.ejim.2016.12.001
36. Samimagham HR, Kheirkhah S, Haghighi A, Najmi Z. Acute kidney injury in intensive care unit: incidence, risk factors and mortality rate. Saudi J Kidney Dis Transpl. (2011) 22:464–70.
37. Vlachopanos G, Schizas D, Hasemaki N, Georgalis A. Pathophysiology of contrast-induced acute kidney injury (Ciaki). Curr Pharm Des. (2019) 25:4642–7. doi: 10.2174/1381612825666191210152944
38. Mandurino-Mirizzi A, Munafo A, Crimi G. Contrast-associated acute kidney injury. J Clin Med. (2022) 11:2167. doi: 10.3390/jcm11082167
39. Abu-Saleh N, Aronson D, Khamaisi M, Khoury EE, Awad H, Kabala A, et al. Increased intra-abdominal pressure induces acute kidney injury in an experimental model of congestive heart failure. J Card Fail. (2019) 25:468–78. doi: 10.1016/j.cardfail.2019.03.008
40. Holgado JL, Lopez C, Fernandez A, Sauri I, Uso R, Trillo JL, et al. Acute kidney injury in heart failure: a population study. ESC Heart Fail. (2020) 7:415–22. doi: 10.1002/ehf2.12595
41. Lee KJ, Kim BJ, Han MK, Kim JT, Choi KH, Shin DI, et al. Effect of heart rate on stroke recurrence and mortality in acute ischemic stroke with atrial fibrillation. Stroke. (2020) 51:162–9. doi: 10.1161/STROKEAHA.119.026847
42. Waskowski J, Pfortmueller CA, Erdoes G, Buehlmann R, Messmer AS, Luedi MM, et al. Mannitol for the prevention of peri-operative acute kidney injury: a systematic review. Eur J Vasc Endovasc Surg. (2019) 58:130–40. doi: 10.1016/j.ejvs.2019.02.003
43. Fisher AR, Jones P, Barlow P, Kennington S, Saville S, Farrimond J, et al. The influence of mannitol on renal function during and after open-heart surgery. Perfusion. (1998) 13:181–6. doi: 10.1177/026765919801300305
44. Wang N, Wang M, Jiang L, Du B, Zhu B, Xi X. The predictive value of the oxford acute severity of illness score for clinical outcomes in patients with acute kidney injury. Ren Fail. (2022) 44:320–8. doi: 10.1080/0886022X.2022.2027247
45. Hu Y, Liu H, Fu S, Wan J, Li X. Red blood cell distribution width is an independent predictor of aki and mortality in patients in the coronary care unit. Kidney Blood Press Res. (2017) 42:1193–204. doi: 10.1159/000485866
46. Akin F, Celik O, Altun I, Ayca B, Ozturk D, Satilmis S, et al. Relation of red cell distribution width to contrast-induced acute kidney injury in patients undergoing a primary percutaneous coronary intervention. Coron Artery Dis. (2015) 26:289–95. doi: 10.1097/MCA.0000000000000223
Keywords: acute ischemic stroke, acute kidney injury, nomogram, MIMIC-III database, retrospective study
Citation: Zhu G, Fu Z, Jin T, Xu X, Wei J, Cai L and Yu W (2022) Dynamic nomogram for predicting acute kidney injury in patients with acute ischemic stroke: A retrospective study. Front. Neurol. 13:987684. doi: 10.3389/fneur.2022.987684
Received: 06 July 2022; Accepted: 22 August 2022;
Published: 13 September 2022.
Edited by:
Yujie Chen, Army Medical University, ChinaReviewed by:
Chao Zhang, Army Medical University, ChinaQiwen Deng, Nanjing Medical University, China
Copyright © 2022 Zhu, Fu, Jin, Xu, Wei, Cai and Yu. This is an open-access article distributed under the terms of the Creative Commons Attribution License (CC BY). The use, distribution or reproduction in other forums is permitted, provided the original author(s) and the copyright owner(s) are credited and that the original publication in this journal is cited, in accordance with accepted academic practice. No use, distribution or reproduction is permitted which does not comply with these terms.
*Correspondence: Wenhua Yu, ywh699@126.com