- 1Department of Neurology, Cedars-Sinai Medical Center, Los Angeles, CA, United States
- 2Department of Biomedical Sciences, Center for Neural Science and Medicine, Cedars-Sinai Medical Center, Los Angeles, CA, United States
- 3Bioengineering Graduate Program, Department of Bioengineering, Henry Samueli School of Engineering, University of California, Los Angeles, Los Angeles, CA, United States
- 4Department of Medicine, David Geffen School of Medicine, University of California, Los Angeles, Los Angeles, CA, United States
Sleep is known to promote recovery post-stroke. However, there is a paucity of data profiling sleep oscillations in the post-stroke human brain. Recent rodent work showed that resurgence of physiologic spindles coupled to sleep slow oscillations (SOs) and concomitant decrease in pathological delta (δ) waves is associated with sustained motor performance gains during stroke recovery. The goal of this study was to evaluate bilaterality of non-rapid eye movement (NREM) sleep-oscillations (namely SOs, δ-waves, spindles, and their nesting) in post-stroke patients vs. healthy control subjects. We analyzed NREM-marked electroencephalography (EEG) data in hospitalized stroke-patients (n = 5) and healthy subjects (n = 3). We used a laterality index to evaluate symmetry of NREM oscillations across hemispheres. We found that stroke subjects had pronounced asymmetry in the oscillations, with a predominance of SOs, δ-waves, spindles, and nested spindles in affected hemisphere, when compared to the healthy subjects. Recent preclinical work classified SO-nested spindles as restorative post-stroke and δ-wave-nested spindles as pathological. We found that the ratio of SO-nested spindles laterality index to δ-wave-nested spindles laterality index was lower in stroke subjects. Using linear mixed models (which included random effects of concurrent pharmacologic drugs), we found large and medium effect size for δ-wave nested spindle and SO-nested spindle, respectively. Our results in this pilot study indicate that considering laterality index of NREM oscillations might be a useful metric for assessing recovery post-stroke and that factoring in pharmacologic drugs may be important when targeting sleep modulation for neurorehabilitation post-stroke.
Introduction
Stroke is a leading cause of motor disability world-wide. Despite advances in neurorehabilitation, there is a lack of widely adopted therapies that target plasticity post-stroke, and functional outcomes remain inconsistent (1–3). Sleep is known to play a major role in regulating plasticity (4–12) and accordingly, there has been an interest in modulating sleep for stroke motor rehabilitation (13, 14). To optimize efforts for effective sleep modulation, there is a need to better understand neural processing during sleep. Additionally, it is important to consider co-morbidities and concurrent pharmaceuticals that may impact excitatory/inhibitory neural transmission. Previous animal and human studies have shown that sleep can influence motor recovery post-stroke (2, 14–23), however more work is needed to understand how sleep neurophysiology is affected in stroke. This has become all the more important with advances in our understanding of sleep neurophysiology linking nested non-rapid eye movement (NREM) oscillations to plasticity, motor memory consolidation, and motor recovery (4, 6, 14, 24).
Sleep-dependent neural processing is crucial for memory consolidation, which is the process of transferring newly learned information to stable long-term memory (9, 25). Initial investigations looked at sleep’s role in declarative memory (26, 27), but recent studies have underscored sleep’s role in motor skill consolidation (5, 6, 28). Specifically, NREM sleep has been linked to the reactivation of awake motor-practice activity and performance gains in a motor skill after sleep (4–6). There is now a consensus that this consolidation occurs during temporal coupling of sleep spindles (10–16 Hz) to larger amplitude slow oscillations (SOs, 0.1–1 Hz) (6, 25, 29–31). Recent work in rodents has shown that these SOs nested with spindles decline immediately post-stroke and increase during motor recovery (14). This work also showed that delta waves (δ waves, 1–4 Hz), along with δ wave-nested spindles increased post-stroke and reduced during recovery. These two nested oscillations (namely, SO-nested spindles vs. δ wave-nested spindles) were shown to have a competing role during recovery. Pharmacological reduction of tonic γ-aminobutyric acid (GABA) neurotransmission shifted the balance toward restorative SO-nested spindles in the brain and increased the pace of recovery. The chief goal of our study was to see if NREM oscillations and their nesting were affected post-stroke in human patients within a hospital setting. Specifically, we wanted to check for laterality of NREM oscillations’ densities in stroke vs. contralateral hemisphere and compare it to healthy subjects.
Our study showed that, acutely post-stroke, there is an increase in SOs, δ waves, and spindles on stroke electrodes when compared to contralateral hemisphere electrodes, whereas healthy subjects had symmetrical density of these oscillations. Our linear mixed effect model revealed that there were significant fixed effects of stroke vs. contralateral electrodes for SOs and δ waves with overall medium effect sizes, including random effects of concurrent pharmacologic drugs. We also observed a large effect size of the linear mixed model for δ wave-nested spindles. Finally, we found that the proportion of SO-nested spindles to δ-wave-nested spindles was lower in stroke subjects compared to healthy subjects. Our work here in a pilot dataset suggests that laterality of NREM sleep oscillations could be a useful marker for physiological sleep activity post-stroke. Future work that confirms our findings in a larger dataset can inform acute stroke care management that also incorporates pharmacologic drug interactions and their effects on laterality of ‘restorative’ sleep oscillations.
Patients and methods
This research was conducted in accordance with and approval of the Cedars-Sinai Medical Center Institutional Review Board (IRB). All research participants and/or their surrogates provided informed consent to participate in the study.
Inclusion/exclusion criteria
Retrospective chart review of the Cedars Sinai EEG database was done to identify patients with acute middle cerebral artery strokes (MCA strokes; with high probability of stroke lesion affecting sensorimotor regions in the brain) who also received EEG monitoring as part of their hospital stay. We selected patients who received EEG in the acute period (2–3 days) post-stroke. Other inclusion criteria were that this should be the first stroke for the patient, they should be within 50–80 years of age, and the patients should not have any sleep disorders or circadian /diurnal rhythm disruption. Subjects were excluded if they were pregnant or diagnosed with uncontrolled medical conditions. Five patients were retrospectively identified for this study, with notable limited availability of EEG studies done within 2–3 days after an MCA distribution stroke. Of the 5 patients, 3 were female and 2 males, all within the age range of 50–80 years old (see Table 1 for other details regarding demographic and clinical information). Indications for EEG were universal for altered mental status after acute stroke. P1 was noted to be on continuous infusion of propofol (<10 mcg total) and infusions of dexamethasone every 4 h. P2 and P5 were treated with levetiracetam 500 mg twice daily. P2 was also on acyclovir which was discontinued after cerebrospinal fluid (CSF) evaluated negative for meningitis; and P5 was administered nonepinephrine due to being in shock acutely and improved within 24 h. P3 and P4 were not given propofol, dexamethasone, or levetiracetam. Unlike all other patients, P4 had subcortical involvement in stroke. It is important to note that spindle oscillations are postulated to have a subcortical (thalamocortical) origin (32). P5 had a hemorrhagic stroke (ruptured right MCA aneurysmal stroke). P2 had partial status epilepticus involving the right temporal lobe. We excluded seizure related epochs based on manual inspection of recordings. This inspection was done by epileptologist (C.M.R.) and seizures were excluded based on no evolving seizure pattern across electrodes (10–20 EEG system). Hence, all our presented data was from sleep periods in all the five patients (even in the patient with status epilepticus). An average of ~5.98 ± 1.26 h [or 358.80 ± 75.40 min, mean ± standard error of mean (s.e.m.)] of NREM sleep was identified and analyzed in each of the five patients. We were not able to analyze REM/ wake periods in these recordings due to the lack of EMGs/ video recordings. Additionally, healthy subjects’ dataset from Cox et al., Sleep Medicine Reviews, 2020 (33, 34) with average NREM sleep of 3.07 ± 0.14 h (or 183.91 ± 8.38 min) was analyzed for 3 subjects.
EEG analysis and identification of NREM oscillations
Patients with overnight EEG recordings 2 to 3 days post-stroke were included. The data, obtained by a Natus Xltek EEG and Sleep System, was de-identified and made compatible for analysis with MATLAB. Each 30-s epoch was manually marked for NREM sleep by an expert scorer (C.M.R. and B.K.S.). EEG epochs were analyzed for NREM sleep in a bipolar montage. In the stroke patients, the following analyses were done with EEG data in a referential montage, referenced to the auricle electrodes. Spindles, SOs, and δ waves were extracted from these NREM epochs using custom code in MATLAB (details below). This allowed for the identification of specific sleep waveforms and how they nested temporally and topographically during NREM sleep. We assessed spindles and their nesting to SOs and δ waves. Topographical maps of the average density of these sleep oscillations allowed us to visualize the average densities with respect to electrode location, especially their lateral symmetry between hemispheres.
From the healthy subjects dataset, we used the common linked mastoids referenced data (33) and analyzed NREM sleep. We selected 20 electrode channels in similar locations as stroke patient data for further analysis (because the healthy subject data had more electrodes than stroke patient dataset). Similar to stroke EEG data; spindles, SOs, and δ waves were extracted from these NREM epochs using custom code in MATLAB and analyzed.
EEG data processing
For stroke patients, NREM-marked EEG data from all channels was referenced with respect to the average of the auricular electrodes (A1 & A2, Figure 1A) while the heathy control dataset had common linked mastoids referenced EEG data. Any high amplitude artifact in the differential EEG signal was removed. We utilized previously-used methods for automatic detection of these NREM oscillations (6, 14, 35). For δ/SOs detection, signal was first passed through a 0.1 Hz high-pass filter and then a 4 Hz low-pass Butterworth filter. All positive-to-negative zero crossings, previous peaks, following troughs, and negative-to-positive zero crossings were identified. A wave was considered a δ wave if its trough was lower than the negative threshold and preceded by a peak that was lower than the positive threshold, within 500 ms (Figures 1B,E,H). SOs were classified as waves with troughs lower than a negative threshold (the bottom 40 percentile of the troughs) and preceding peaks higher than a positive threshold (the top 15 percentile of the peaks; Figures 1C,F,I). Duration between peaks and troughs was between 150 ms and 500 ms. For spindle detection, EEG data was filtered using a 10 Hz high-pass Butterworth filter and a 16 Hz low-pass Butterworth filter. A smoothed envelope of this signal was calculated using the magnitude of the Hilbert transforms with convolving by a Gaussian window (200 ms). Epochs with signal amplitude higher than the upper threshold (mean, μ + 2.5* standard deviation (s.d.), σ) for at least one sample and amplitude higher than the lower threshold (μ + 1.5*σ) for at least 500 ms were considered spindles (Figures 1D,G,J). The lower threshold was used to define the duration of the spindle. Nested SO-spindles (parallel to k-complexes studied in humans) were identified as spindle peaks following SO peaks within 1.5 s duration (Figure 1K). The same criterion was used to identify δ wave-nested spindles (Figure 1L).
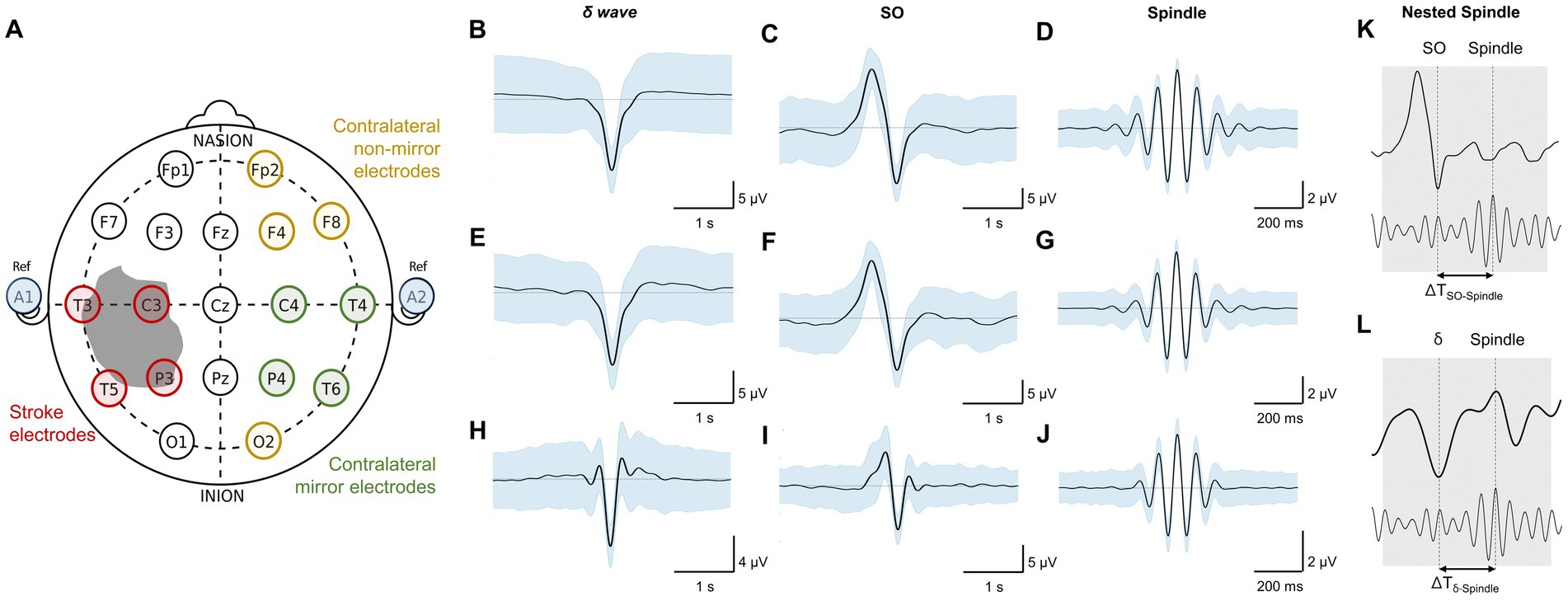
Figure 1. Stroke vs. contralateral mirror/non-mirror electrode assignment and NREM sleep oscillations. (A) 10–20 system for EEG (used in stroke patients) showing locations of all electrode locations recorded with an illustration of stroke. Gray shaded area shows a representative stroke perilesional region. Blue shaded circles represent auricular electrodes (A1, A2) that were used for referencing in the stroke patients. Red circles indicate identified stroke electrodes based on proximity to the perilesional area. Green circles indicate identified contralateral mirror (CM) electrodes which are contralateral and mirrored to identified stroke electrodes. Yellow circles indicate identified contralateral non-mirror (CNM) electrodes which are electrodes other than contralateral mirror (CM) electrodes in non-stroke hemisphere. (B) Mean δ–wave along with s.e.m. (standard error of mean) bands (blue) for all identified δ–waves from an example stroke electrode channel from EEG data recording for one stroke patient. (C) Same as (B) for SO waveforms. (D) Same as (B) for spindle waveforms. (E–G) Same as (B–D) for one example contralateral mirror electrode channel for a stroke patient. (H–J) Same as (B–D) for one example channel for a healthy subject. All waveforms are centered around the detected states. (K) Illustration of SO-spindle nesting. Nesting window was −0.5 to +1.0 s from SO’s UP state as shown. (L) Illustration of δ–wave-spindle nesting. Nesting window was −0.5 to +1.0 s from δ UP state as depicted.
Data analysis
We generated topographical maps of these different waveforms using plot_topography function in MATLAB (36) as shown in Figure 2. The patients were separated into three groups based on concurrent medications, as detailed in Table 1. Patient 1, assigned to Group 1, was on continuous propofol and dexamethasone injections every 4 h. Group 2 (patients 2 and 5) was administered levetiracetam (Keppra) twice daily; and Group 3 (patients 3 and 4) was not on medications known to significantly modulate excitatory/inhibitory neural transmission.
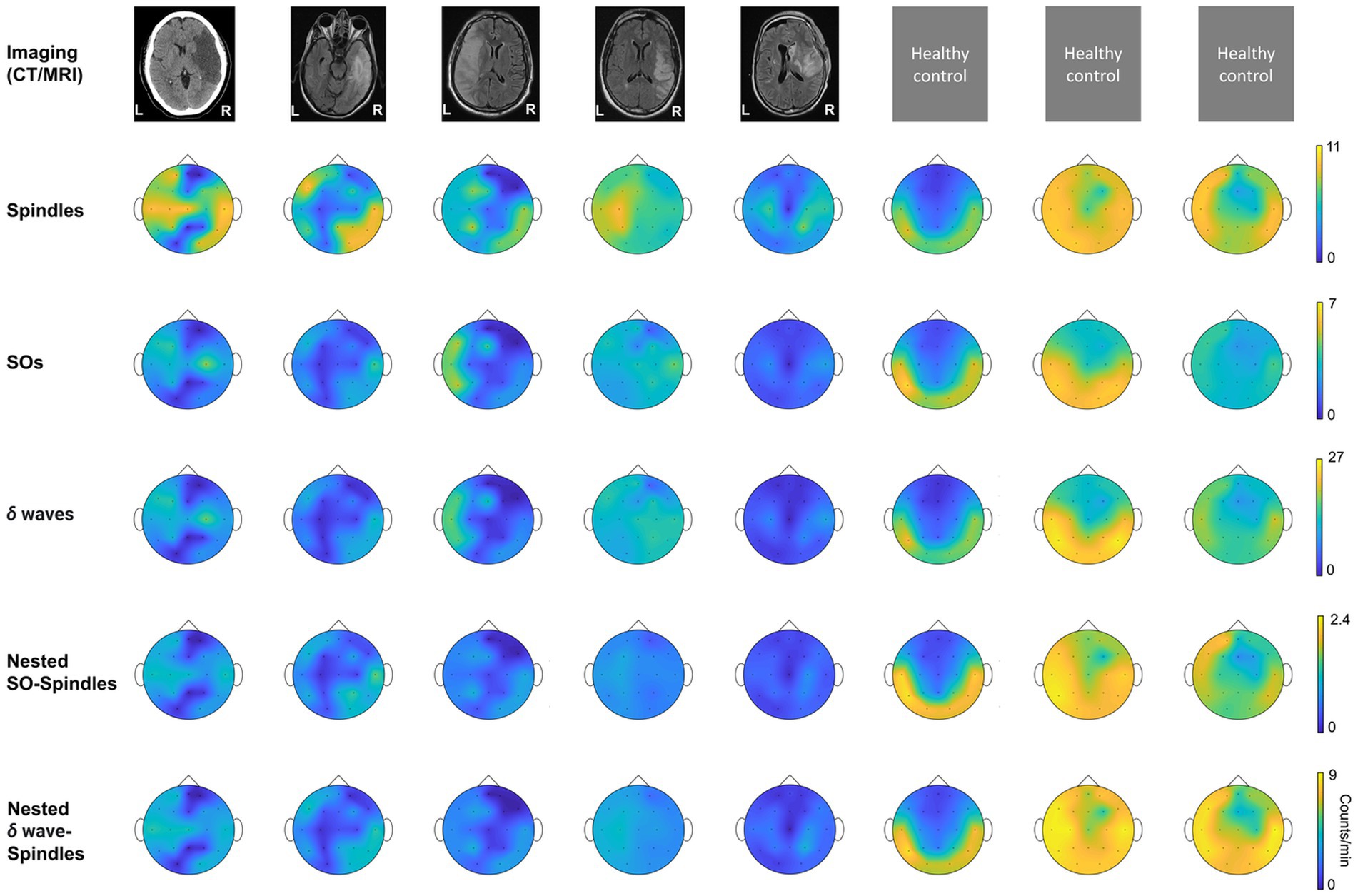
Figure 2. Imaging data and topographical density plots for different NREM oscillations. Top to bottom: Imaging data: CT (computed tomography) image for patient P1, T2 sequences of MRI (magnetic resonance imaging) images for patients P2–P5; no imaging data available for healthy subjects (P6–P8). Radiologic imaging has been flipped horizontally to align with topographic density maps; i.e., image left, and right are ipsilateral to patient left and right. Left and right are marked in imaging figures (P1–P5) and apply to density topographical maps below them; Topographical maps for detected spindle density (count/min) during NREM sleep for all subjects; Topographical maps for detected SO density (count/min) during NREM sleep for all subjects; Topographical maps for detected δ waves’ density (count/min) during NREM sleep for all subjects; Topographical maps for detected nested SO-spindle density (count/min) during NREM sleep for all subjects; Topographical maps for detected δ wave-nested-spindle density (count/min) during NREM sleep for all subjects. Color map shown at right for all the panels in a row.
Perilesional electrodes were identified by analyzing post-stroke magnetic resonance imaging (MRI) and computer tomography (CT) neuroimaging. We marked Stroke electrodes as the electrodes covering the perilesional region of the brain as shown in Figure 1A. The mirror opposite electrodes on the contralateral side were marked as Contralateral mirror (CM) electrodes for further analysis (Figure 1A). The non-mirror opposite electrodes on the contralateral side were marked as Contralateral non-mirror (CNM) electrodes.
We compared the symmetry in NREM oscillations’ density across hemispheres for stroke patients and healthy control using a laterality index (Figures 3A–F). Laterality index of 1 meant the average density being analyzed for electrode locations selected across hemisphere is equal. For stroke patients, laterality index was defined as the ratio of mean of stroke electrodes’ NREM densities to all contralateral electrodes’ NREM densities. For healthy subjects, laterality index was defined as the ratio of the mean of left hemisphere electrodes’ NREM densities to right hemisphere electrodes’ NREM densities. We also compared the ratio of SO-nested spindles laterality index to δ wave-nested spindles laterality index for stroke vs. healthy subjects.
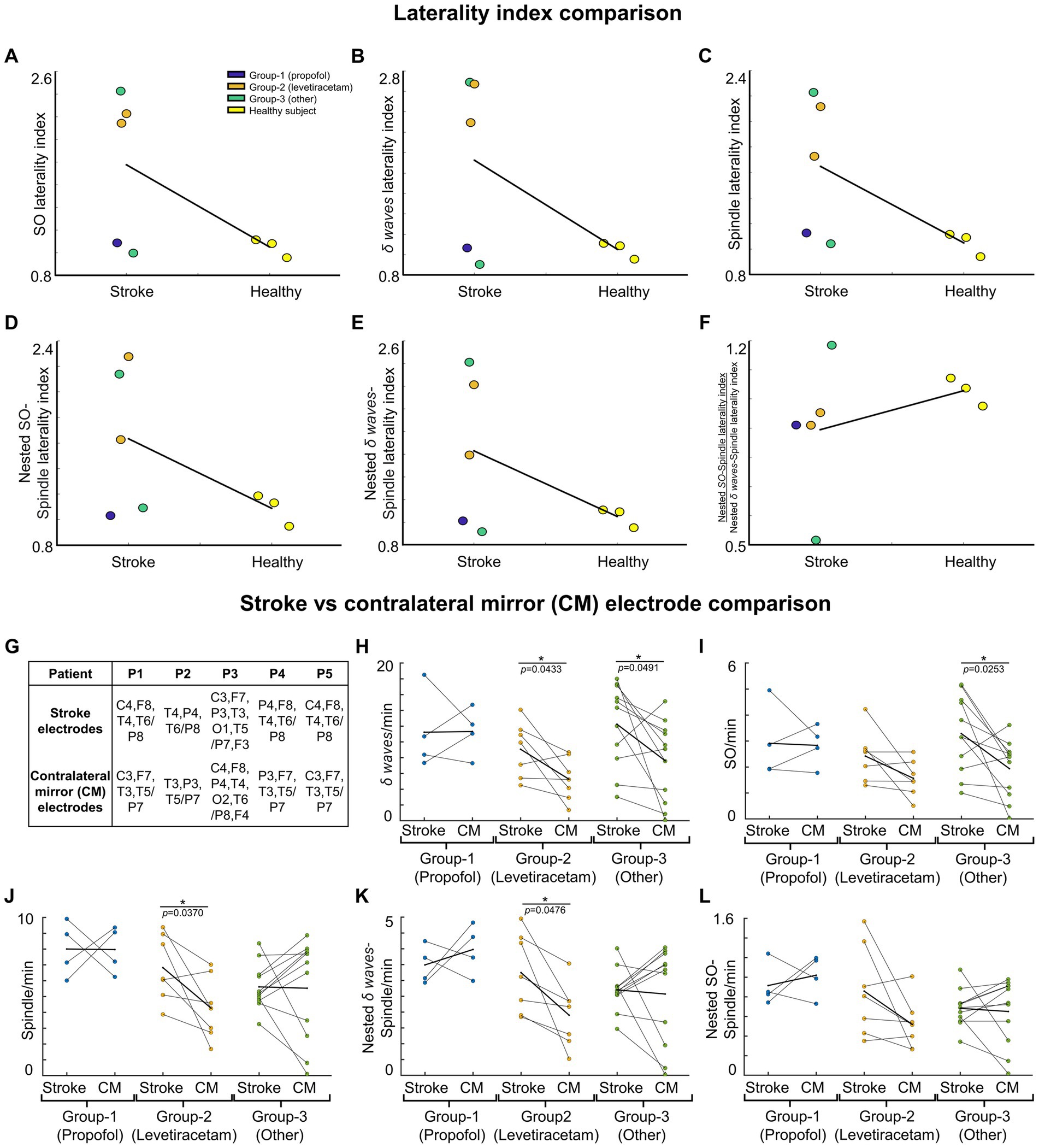
Figure 3. NREM oscillations’ laterality in stroke patient’s vs. healthy controls; and NREM oscillations’ densities for different patient groups on stroke verses contralateral mirror (CM) electrodes. For stroke patients’ laterality index (LI) is defined as ratio of mean of stroke electrode NREM densities to all contralateral electrode NREM densities. For healthy subjects’ laterality index is defined as ratio of mean of left hemisphere electrode NREM densities to right hemisphere electrode NREM densities. (A) LI for SO density for stroke patients and healthy controls. Black line connects the mean of stroke and control group. Dots represent different patients/subjects; blue dots: Patients in propofol medication group; orange dots: Patients in levetiracetam medication group; green dots: Stroke patients in other medication group; yellow dots: Healthy subjects. (B) Same as (A) for δ wave density LI. (C) Same as (A) for spindle density LI. (D) Same as (A) for nested SO-spindle density LI. (E) Same as (A) for Nested δ wave-spindle density LI. (F) Ratio of LI for nested SO-spindle density and nested δ wave-spindle density. (G) Table showing selected stroke and contralateral mirror electrodes (CM) for all patients. (H) Comparison of δ wave density (count/min) on stroke vs. CM electrodes for patients on different medications. Thick black line shows the mean values within the group. Thinner black lines join pair of stroke and CM electrode. Dots represent the NREM oscillations’ density for single electrode. (I) Same as (H) for SO density. (J) Same as (H) for spindle density. (K) Same as (H) for nested δ wave-nested spindle density. (L) Same as (H) for SO-nested spindle density. *statistically significant p values for two-tailed t-test.
Statistical analysis
We performed a linear mixed effect analysis for all patients comparing the Stroke electrodes density vs. Contralateral (CM/CNM) electrodes density for different waveforms using the fitlmematrix function in MATLAB. The linear mixed effect model was fitted by maximum likelihood using the formula below (1) for all the different waveforms identified during EEG data processing. Medication groups were defined as the three groups mentioned earlier. This model considered fixed effects of stroke vs. contralateral (CM/CNM) electrodes, and the random effect of electrodes and medication groups depending on the patient and was represented as:
Waveform Density ~ Intercept + Electrode + (Intercept + Electrode + Medication Groups | Patient).
The above formula/equation is written in a format like the documentation for fitlmematrix Matlab function. We compared the Stroke electrodes density vs. contralateral (CM/CNM) electrodes density within each medication group using a two-tailed t-test. Contralateral electrodes chosen were mirrored electrodes (Figures 3G–L) or non-mirrored (Supplementary Figures S2A–F). One-way ANOVA was used to compare the stroke electrodes’ NREM oscillations’ density of the three different medication groups.
We calculated r-squared (R2) and the Cohen’s d values for the overall linear mixed effect model generated. However, the p-values were specifically assessed for fixed effect of electrodes (stroke vs. CM/CNM). Cohen’s d was used to evaluate if the nested data (all data combined) for NREM oscillations had a small, medium or large experimental effect (Cohen’s d = 0.20, 0.50 or 0.80, respectively) (37). Effect size indicates if research findings have practical significance. Metrics such as Cohen’s d are better at the planning stage for pilot studies, like the one here, to determine optimal sample sizes for sufficient power in bigger clinical trials (38). We summarized the linear mixed effects models results in the tables in the Supplementary Information (Supplementary Tables S1, S2).
Results
One of the limitations of retrospectively analyzing EEG data gathered from clinical EEG was the heterogeneity encountered across the subjects studied, a contrast from the controlled setting of related rodent studies. With this in mind, we noted that one important similarity across the study population was the indication for EEG: concern for underlying seizure in the setting of altered mental status and recent hemispheric stroke. Accordingly, the patients were all hospitalized, and our analysis benefited from close pharmacologic documentation. We observed differences in laterality of NREM oscillations in stroke patients. We observed higher SOs, δ waves, spindles and spindles nested to SOs and δ waves in the stroke hemisphere. For the patient with subcortical involvement in stroke, we observed a decrease in spindles in the stroke hemisphere. We also observed effects of concurrent medications, particularly medications that might influence neural transmission.
NREM oscillation densities symmetry is disturbed acutely in stroke
We found that stroke patients had laterality differences (higher or lower densities in stroke hemisphere) for all NREM oscillations, while the healthy subject NREM oscillation density looked more symmetrical across hemispheres (Figure 2). Comparing the laterality index (LI) (as defined in methods), we found that the LI was closer to 1 on average with low variance for healthy subjects. For stroke patients, LI was higher than 1 on average with high variance. SO density LI’s were: stroke: 1.78 ± 0.34 and healthy: 1.05 ± 0.06 (Figure 3A). δ wave density LI’s were: stroke: 1.93 ± 0.44 and healthy: 1.05 ± 0.06 (Figure 3B). Spindle density LI’s were: stroke: 1.65 ± 0.27 and healthy: 1.05 ± 0. 0.07 (Figure 3C). SO-nested spindles LI’s were: stroke: 1.63 ± 0.30 and healthy: 1.09 ± 0.09 (Figure 3D). δ wave-nested spindles LI’s were: stroke: 1.63 ± 0.34 and healthy: 1.05 ± 0.06 (Figure 3E). The ratios of nested SO-spindles LI’s and δ wave-nested spindle LI’s were: stroke: 0.90 ± 0.12 and healthy: 1.03 ± 0.03 (Figure 3F).
SO and δ wave density increased in perilesional electrodes
Next, we wanted to look at stroke-affected electrodes in stroke patients vis-à-vis the contralateral hemisphere electrodes. In the contralateral hemisphere, we looked at mirrored electrodes (CM, as defined in the methods; Figure 3G), or non-mirrored electrodes (CNM, as defined in methods; Supplementary Figure S2A). Consistent with previous reports, we found that stroke electrodes had increased low-frequency (< 4 Hz) oscillations (Figures 3H,I; Supplementary Figures S2B,C) (39). Our mixed-effects model showed a significant fixed effect of stroke vs. CM and CNM electrodes for a subset of NREM oscillations and overall medium to large effect sizes which included random effects of concurrent pharmaceuticals. We observed higher δ wave density in the perilesional electrodes (Figure 3H; Supplementary Figure S2B; Supplementary Tables S1, S2 provide statistical details for stroke vs. CM or CNM: p-value is provided for the fixed effect (“electrode”), R2 and Cohen’s d are for the overall model with fixed and random effects, conventions same henceforth). Our comparison of LI’s of SOs and δ wave showed that LI’s were higher in stroke patients compared to healthy subjects: Mean LI’s for SOs were: stroke: 1.78 ± 0.34 and healthy: 1.05 ± 0.06; mean LI’s for δ wave were: stroke: 1.91 ± 0.44 and healthy: 1.05 ± 0.06. We also observed that Group-1 (propofol and dexamethasone) and Group-3 (others) both had higher δ wave density on stroke electrodes than Group-2 (levetiracetam) (Figure 3H; Supplementary Figure S2B; stroke electrodes’ δ wave density- Group 1: 11.23 ± 2.53 counts min−1 (mean ± s.e.m.); Group 2: 9.07 ± 1.32 counts min−1; Group 3: 12.25 ± 1.59 counts min−1, see Supplementary Table S3 for details). Group-2 and Group-3 showed a high density of δ waves in the stroke electrodes vs. CM/CNM electrodes (Figure 3H; Supplementary Figure S2B). For SOs, there was a significant fixed effect of stroke vs. contralateral electrodes (Figure 3I; Supplementary Figure S2C; Supplementary Tables S1, S2 provide p-value and Cohen’s d). We observed that the patients in Group-1 did not show a significant difference between stroke or contralateral electrode SO density, while patients in Group-2 showed elevation in SO on stroke electrodes when compared to CM electrodes (Figure 3I). The patients in Group-3 showed increased SOs on stroke electrodes when compared to CM/CNM electrodes (Figure 3I; Supplementary Figure S2C; stroke electrodes’ SO density: Group 1: 2.91 ± 0.71 counts min−1; Group 2: 2.42 ± 0.37 counts min−1; Group 3: 3.29 ± 0.45 counts min−1; see Supplementary Table S3 for details).
For spindle oscillations, LI’s were higher in stroke patients (Mean LI spindles, stroke: 1.65 ± 0.27 and healthy: 1.05 ± 0. 0.07). Interestingly, in one patient with subcortical involvement with stroke (P4), spindles were higher in the contralesional hemisphere (Figure 3J). Linear mixed-effects model did not show a significant fixed effect for spindle density on stroke vs. contralateral electrodes; overall, it was a medium effect size based on the Cohen’s d (Figure 3J; Supplementary Figure S2D; see Supplementary Tables S1, S2 for p-value and Cohen’s d). Spindle density was found to be the highest on the stroke electrodes in the patient in Group-1 (8 ± 0.88 counts min−1), followed by the patients in Group-2 (6.83 ± 0.79 counts min−1), and then patients in Group-3 (5.61 ± 0.44 counts min−1) (Figure 3J; Supplementary Figure S2D; see Supplementary Table S3 for details).
δ wave-nested spindles and SO-nested spindles
Next, we looked at nested oscillations, namely δ wave-nested spindles and SO-nested spindles oscillations that were recently shown to have a competing role in memory consolidation and inverse trend during stroke recovery (6, 14). LI’s for both nested oscillations were observed to be higher in stroke subjects. Mean LI’s for SO-nested spindle were: stroke: 1.64 ± 0.29 and healthy: 1.09 ± 0.09; and mean LI’s for δ wave-nested spindle were: stroke: 1.63 ± 0.34 and healthy: 1.05 ± 0.06. Linear mixed effects models of δ wave-nested spindles and SO-nested spindles did not show a significant difference between stroke and contralateral electrodes, whereas these models still had large and medium effect sizes, respectively (Supplementary Tables S1, S2; Figure 3K; Supplementary Figure S2E, δ wave-nested spindle density on stroke electrodes: Group-1: 3.49 ± 0.30 counts min−1; Group-2: 3.25 ± 0.48 counts min−1; Group-3: 2.70 ± 0.20 counts min−1, also see Supplementary Table S3; SO-nested spindle density on stroke electrodes: Group 1: 0.92 ± 0.11 counts min−1; Group 2: 0.86 ± 0.17 counts min−1; Group 3: 0.68 ± 0.06 counts min−1; see Figure 3L; Supplementary Figure S2F; Supplementary Table S3). Notably, the ratios of SO-nested spindle LI’s to δ wave-nested spindle LI’s were lower in stroke subjects compared to heathy subjects (Mean LI ratio, stroke: 0.0 ± 0.12 and healthy: 1.03 ± 0.03). This might indicate relatively increased δ wave-nested spindles when compared to SO-nested spindles (the oscillations that have a competing role in forgetting vs. strengthening, respectively) in the perilesional areas for stroke brain when compared to healthy brain.
Together, the results in this limited dataset showed that lateral symmetry of NREM oscillations is disturbed in stroke (Figures 3A–F), when compared to healthy subjects. These results also indicated that there is an elevation of SO, δ wave, spindles, and spindle nesting to SOs or δ waves in the perilesional areas post-stroke. Future work can confirm these findings on laterality of sleep oscillations in a larger dataset that also considers the pharmacologic drug interactions.
Discussion
Our results show that, post-stroke there is a disturbance in laterality of NREM sleep oscillations across ipsilesional and contralesional hemispheres. Interestingly, hemispherical differences in these nested oscillations were less pronounced in healthy subjects, and oscillations appeared mostly symmetric. We used a laterality index for comparing NREM oscillations, with an emphasis on nested oscillations, i.e., SO-nested spindle oscillations and δ wave-nested spindle oscillations. Our results here can be a precursor to future investigations studying neuromodulation of sleep for rehabilitation. While our findings are preliminary in a small pilot dataset, they report an interesting effect size, suggesting a roadmap for delineating pathological sleep in larger cohorts and optimal therapeutic modulation to promote recovery.
Sleep and plasticity post-stroke
Preclinical and clinical studies that have evaluated local-field potentials (LFPs) in animals (40, 41) and EEG in human patients (22, 42, 43) have found increased low-frequency power during awake, spontaneous periods after a stroke. These studies postulate that this increased low-frequency activity could be a marker of cortical injury and loss of subcortical inputs (44). Our findings on increased SOs and δ waves on stroke electrodes are indicative of similar phenomena. We also found an increase in SO-nested spindles and δ wave-nested spindles on stroke electrodes along with a lower ratio of SO-nested spindle LI’s to δ wave-nested spindle LI’s (Figure 3F). There is growing evidence that temporal coupling of spindles to SOs is a primary driver of sleep-related plasticity and memory consolidation (6, 30, 31, 45–48). SO-nested spindles are linked to spike-time dependent plasticity (49). These events are also related to reactivation of awake experiences (30, 47, 50). Importantly, disruption of this coupling can impair sleep-related memory consolidation of awake experiences (6). This same work showed that SO-nested spindles and δ wave-nested spindles compete to either strengthen or forget a memory. Our results indicate that balance of SO-nested spindle density and δ wave-nested spindle density is disturbed across hemispheres in stroke patients compared to healthy subjects. These disruptions might be related to impaired sleep-processing that impact recovery. Interestingly, we observed large to medium effect sizes in our linear mixed-effects models for δ wave-nested spindle and SO-nested spindle where we considered fixed effects of electrodes and random effects of drugs and patients. It is worth noting that drugs like propofol can impact such nested sleep oscillations (51, 52). It may be important to consider the effects of drugs on sleep oscillations when modulating sleep for stroke recovery.
Propofol and levetiracetam: effect on sleep
We made observations on different medications that stroke patients received during sleep EEG recordings. Group-1 received propofol, which is one of the most commonly used anesthetics in neurologic intensive care units after stroke or traumatic brain injury (53). It exerts its action by potentiating the activity of chloride currents through GABA receptors while blocking voltage-gated sodium channels (54–56). The patient on propofol received less than 10 mcg dose of propofol which is not known to impact sleep (57, 58). Group-2 received levetiracetam (Keppra), which is a newer anti-seizure drug. The exact mechanism for its anti-seizure function is unclear, but it is believed to exert its effect through synaptic vesicle glycoprotein 2A (59). Through this mechanism, levetiracetam is capable of modulating neurotransmission by inhibiting calcium currents (60). A study has shown that levetiracetam has minimal effects on sleep parameters like total sleep duration, sleep latency, and sleep efficiency in both healthy humans and partial epilepsy patients (61). However, observations have been made that levetiracetam can reduce motor activity and cause daytime drowsiness in patients (61, 62). Propofol, by its GABAergic action, causes greater loss of faster frequencies during induction with a shift in alpha frequencies to the frontal regions that reverses post-awakening (63–65). Since our linear mixed-effects model had large to medium effect sizes when considering random effects of drugs on all NREM oscillation, it may be useful to explore the impact of drugs on NREM sleep densities with larger patient cohorts in the future.
Sleep processing and stroke rehabilitation
Recent rodent work profiled SO-nested and δ wave-nested spindles during the course of stroke recovery and found links between these nested structures and motor performance gains during recovery (6). This work specifically looked into reach task, but clinical rehabilitation approaches can be varied (66–68). It is likely that the sleep features of nested oscillations and their putative pathological or physiological roles need to be factored in when considering timing for rehabilitation, irrespective of training type. Previous human and rodent studies have also suggested critical periods in training that can offer long-term benefits (69–71). Past studies that have found low-frequency power in awake state in stroke patients might be related to our findings of increased SO and δ waves densities. Future studies where EEG data is captured over longer periods may delineate a transition of δ wave LI, SOs LI, δ wave-nested spindles LI (pathological sleep) and SO-nested spindle LI (physiological sleep), and its relation to critical periods post-stroke for optimal timing of rehabilitation. For example, SO-nested spindles LI and δ wave-nested spindles LI proportions between hemispheres could be targeted to be brought closer to unity as in healthy subjects, to accelerate recovery.
Modulation of sleep as a therapeutic intervention
The results we have presented can form the basis of translational studies in the future that target modulation of sleep post-stroke. Animal studies have suggested that modulation of GABAergic transmission (specifically GABAA-receptor mediated tonic inhibition) in the perilesional cortex can serve as a therapeutic target to promote recovery, and that blocking of GABAA-mediated tonic inhibition promoted motor recover maximally in the first 1 to 2 weeks post-stroke (72, 73). Both short-term (acute) and long-term chronic infusion of GABAA inhibiting compounds have been tested, and long-term infusion was shown to be better (72). Long-term pharmacologic modulation, as shown by Clarkson and colleagues, may be essential to achieve observable motor benefits in human patients. Benefits of long-term infusion include the effect of the drug not only with rehabilitation-specific online (awake) training, but also during offline memory consolidation during sleep.
Studies such as ours can also help guide electric stimulation-based neuromodulation for augmenting recovery. SOs and δ waves can be easily monitored using EEG in stroke patients. Non-invasive brain stimulation during sleep (30, 47, 74, 75) can be used to modulate specific NREM oscillations. Invasive stimulation approaches, such as epidural stimulation (76), can also focus on sleep state to optimize sleep neural processing. Similar approaches have shown that direct epidural motor cortical electric stimulation can enhance awake performance and neural activity (77, 78) and epidural stimulation of subcortical regions can also modulate low-frequency oscillations in the motor cortex (79). However, such approaches have not been applied during sleep. A recent study suggested that modulating UP states during sleep can enhance recovery (18). It is plausible that future approaches targeting sleep, when delivered in a closed-loop fashion, optimize both awake task performance and its consequent sleep processing, and may lead to greater long-term benefits during rehabilitation. Indices such as laterality index that we pursued here may serve a utilitarian purpose in long-term sleep evaluation post-stroke with different treatments. Our pilot observations here also suggest that concurrent pharmacologic drugs may affect NREM oscillations. Future work can confirm these effects in larger cohorts and if medication effects should be considered when personalizing sleep stimulation.
Limitations
One of the limitations of our study is the lack of a link between sleep architecture and motor status. Future work that studies sleep over longer periods post-stroke and assesses motor functionality longitudinally may find more robust links between sleep processing and related gains in motor performance. It is also possible that, with more effective task performance and associated awake neural dynamics (77, 78, 80), efficacy of sleep may change. Precise disruption of sleep processing, specifically SO-spindle coupling in healthy animals, was sufficient to prevent offline performance gains, even when awake task learning was robust (6). This work also showed that precise modulation of the extent of sleep spindle-SO coupling in healthy animals could either enhance or impede sleep processing. While extension of this work in stroke animals has shown SO-spindle nesting resurges with recovery (14), future animal studies that modulate sleep microarchitecture can study if artificial manipulation of SO-nested spindles or δ wave-nested spindles after stroke are sufficient to enhance or impair motor recovery. Our work here showed that both SO-nested spindles and δ wave-nested spindles increased in stroke affected hemisphere acutely post-stroke. Future work that monitors these oscillations for longer periods can assess if SO-nested spindles should increase with respect to δ wave-nested spindles for better recovery in human stroke patients.
As a pilot retrospective study, one more limitation is a smaller sample size with varying lesion location and volume. While we focused on getting patients with cortical lesions and MCA involvement, sleep may have been impacted differently for one patient with a primarily subcortical stroke. For example, a stroke in the white matter that impacts thalamocortical networks may also impact spindles. Future work with larger sample sizes and incorporation of motor task rehabilitation training and drug manipulation, may provide stronger links to engineer sleep to benefit motor recovery post-stroke.
Data availability statement
All requests for raw and analyzed data and materials are promptly reviewed by the Cedars-Sinai Medical Center to verify if the request is subject to any intellectual property or confidentiality obligations. Patient-related data may be subject to patient confidentiality. Requests to access the datasets should be directed to TG at tanuj.gulati@csmc.edu.
Ethics statement
The studies involving humans were approved by Cedars-Sinai Institutional Review Board. The studies were conducted in accordance with the local legislation and institutional requirements. The participants provided their written informed consent to participate in this study.
Author contributions
BS, RR, CR, and TG contributed to the design of the study, interpretation of the data, and draft of the article. RR, BS, and AA contributed to the analysis of the data. BS, JC, and CR contributed to the acquisition of data. All authors contributed to the article and approved the submitted version.
Funding
The study was supported by American Heart Association (AHA; predoctoral fellowship 23PRE1018175 to RR, postdoctoral fellowship 897265 to AA and career development award 847486 to TG), National Institutes of Health (NIH)’s National Institute for Neurological Disorders and Stroke (NINDS) (R00NS097620 and R01NS128469 to TG), National Science Foundation (award 2048231 to TG) and Cedars-Sinai Medical Center. AA also received support through Cedars-Sinai’s Center for Neural Science and Medicine postdoctoral fellowship.
Acknowledgments
We thank the patients that participated in this study. We thank Daniel W. Bowen for manuscript review and edits. We thank the Cedars-Sinai Neurophysiology team that helped in EEG data acquisition and consent especially Cody Holland, Erica Quan, and Ho Duong. We thank Karunesh Ganguly and Jaekyung Kim for NREM sleep oscillations detection Matlab code.
Conflict of interest
The authors declare that the research was conducted in the absence of any commercial or financial relationships that could be construed as a potential conflict of interest.
Publisher’s note
All claims expressed in this article are solely those of the authors and do not necessarily represent those of their affiliated organizations, or those of the publisher, the editors and the reviewers. Any product that may be evaluated in this article, or claim that may be made by its manufacturer, is not guaranteed or endorsed by the publisher.
Supplementary material
The Supplementary material for this article can be found online at: https://www.frontiersin.org/articles/10.3389/fneur.2023.1243575/full#supplementary-material
References
1. Ganguly, K, and Poo, MM. Activity-dependent neural plasticity from bench to bedside. Neuron. (2013) 80:729–41. doi: 10.1016/j.neuron.2013.10.028
2. Ganguly, K, Khanna, P, Morecraft, RJ, and Lin, DJ. Modulation of neural co-firing to enhance network transmission and improve motor function after stroke. Neuron. (2022) 110:2363–85. doi: 10.1016/j.neuron.2022.06.024
3. Norrving, B, and Kissela, B. The global burden of stroke and need for a continuum of care. Neurology. (2013) 80:S5–S12. doi: 10.1212/WNL.0b013e3182762397
4. Gulati, T, Guo, L, Ramanathan, DS, Bodepudi, A, and Ganguly, K. Neural reactivations during sleep determine network credit assignment. Nat Neurosci. (2017) 20:1277–84. doi: 10.1038/nn.4601
5. Ramanathan, DS, Gulati, T, and Ganguly, K. Sleep-dependent reactivation of ensembles in motor cortex promotes skill consolidation. PLoS Biol. (2015) 13:e1002263. doi: 10.1371/journal.pbio.1002263
6. Kim, J, Gulati, T, and Ganguly, K. Competing roles of slow oscillations and Delta waves in memory consolidation versus forgetting. Cells. (2019) 179:514–526.e13. doi: 10.1016/j.cell.2019.08.040
7. Gulati, T, Ramanathan, DS, Wong, CC, and Ganguly, K. Reactivation of emergent task-related ensembles during slow-wave sleep after neuroprosthetic learning. Nat Neurosci. (2014) 17:1107–13. doi: 10.1038/nn.3759
8. Genzel, L, Kroes, MC, Dresler, M, and Battaglia, FP. Light sleep versus slow wave sleep in memory consolidation: a question of global versus local processes? Trends Neurosci. (2014) 37:10–9. doi: 10.1016/j.tins.2013.10.002
9. Stickgold, R. Sleep-dependent memory consolidation. Nature. (2005) 437:1272–8. doi: 10.1038/nature04286
10. Tononi, G, and Cirelli, C. Sleep and the price of plasticity: from synaptic and cellular homeostasis to memory consolidation and integration. Neuron. (2014) 81:12–34. doi: 10.1016/j.neuron.2013.12.025
11. de Vivo, L, Bellesi, M, Marshall, W, Bushong, EA, Ellisman, MH, Tononi, G, et al. Ultrastructural evidence for synaptic scaling across the wake/sleep cycle. Science. (2017) 355:507–10. doi: 10.1126/science.aah5982
12. Klinzing, JG, Niethard, N, and Born, J. Mechanisms of systems memory consolidation during sleep. Nat Neurosci. (2019) 22:1598–610. doi: 10.1038/s41593-019-0467-3
13. Ebajemito, JK, Furlan, L, Nissen, C, and Sterr, A. Application of transcranial direct current stimulation in neurorehabilitation: the modulatory effect of sleep. Front Neurol. (2016) 7:54. doi: 10.3389/fneur.2016.00054
14. Kim, J, Guo, L, Hishinuma, A, Lemke, S, Ramanathan, DS, Won, SJ, et al. Recovery of consolidation after sleep following stroke—interaction of slow waves, spindles, and GABA. Cell Rep. (2022) 38:110426. doi: 10.1016/j.celrep.2022.110426
15. Backhaus, W, Braass, H, Gerloff, C, and Hummel, FC. Can daytime napping assist the process of skills acquisition after stroke? Front Neurol. (2018) 9:1002. doi: 10.3389/fneur.2018.01002
16. Baumann, CR, Kilic, E, Petit, B, Werth, E, Hermann, DM, Tafti, M, et al. Sleep EEG changes after middle cerebral artery infarcts in mice: different effects of striatal and cortical lesions. Sleep. (2006) 29:1339–44. doi: 10.1093/sleep/29.10.1339
17. Duss, SB, Seiler, A, Schmidt, MH, Pace, M, Adamantidis, A, Müri, RM, et al. The role of sleep in recovery following ischemic stroke: a review of human and animal data. Neurobiol Sleep Circadian Rhythms. (2017) 2:94–105. doi: 10.1016/j.nbscr.2016.11.003
18. Facchin, L, Schöne, C, Mensen, A, Bandarabadi, M, Pilotto, F, Saxena, S, et al. Slow waves promote sleep-dependent plasticity and functional recovery after stroke. J Neurosci. (2020) 40:8637–51. doi: 10.1523/JNEUROSCI.0373-20.2020
19. Gao, B, Cam, E, Jaeger, H, Zunzunegui, C, Sarnthein, J, and Bassetti, CL. Sleep disruption aggravates focal cerebral ischemia in the rat. Sleep. (2010) 33:879–87. doi: 10.1093/sleep/33.7.879
20. Giubilei, F, Iannilli, M, Vitale, A, Pierallini, A, Sacchetti, ML, Antonini, G, et al. Sleep patterns in acute ischemic stroke. Acta Neurol Scand. (1992) 86:567–71. doi: 10.1111/j.1600-0404.1992.tb05488.x
21. Gottselig, JM, Bassetti, CL, and Achermann, P. Power and coherence of sleep spindle frequency activity following hemispheric stroke. Brain. (2002) 125:373–83. doi: 10.1093/brain/awf021
22. Poryazova, R, Huber, R, Khatami, R, Werth, E, Brugger, P, Barath, K, et al. Topographic sleep EEG changes in the acute and chronic stage of hemispheric stroke. J Sleep Res. (2015) 24:54–65. doi: 10.1111/jsr.12208
23. Siengsukon, CF, and Boyd, LA. Sleep to learn after stroke: implicit and explicit off-line motor learning. Neurosci Lett. (2009) 451:1–5. doi: 10.1016/j.neulet.2008.12.040
24. Lemke, SM, Ramanathan, DS, Darevksy, D, Egert, D, Berke, JD, and Ganguly, K. Coupling between motor cortex and striatum increases during sleep over long-term skill learning. elife. (2021) 10:e64303. doi: 10.7554/eLife.64303
25. Born, J, Rasch, B, and Gais, S. Sleep to remember. Neuroscientist. (2006) 12:410–24. doi: 10.1177/1073858406292647
26. Rothschild, G, Eban, E, and Frank, LM. A cortical–hippocampal–cortical loop of information processing during memory consolidation. Nat Neurosci. (2017) 20:251–9. doi: 10.1038/nn.4457
27. Sirota, A, Csicsvari, J, Buhl, D, and Buzsáki, G. Communication between neocortex and hippocampus during sleep in rodents. Proc Natl Acad Sci. (2003) 100:2065–9. doi: 10.1073/pnas.0437938100
28. Walker, MP, Brakefield, T, Morgan, A, Hobson, JA, and Stickgold, R. Practice with sleep makes perfect: sleep-dependent motor skill learning. Neuron. (2002) 35:205–11. doi: 10.1016/S0896-6273(02)00746-8
29. Buzsáki, G. Hippocampal sharp wave-ripple: a cognitive biomarker for episodic memory and planning. Hippocampus. (2015) 25:1073–188. doi: 10.1002/hipo.22488
30. Cairney, SA, Guttesen, A, el Marj, N, and Staresina, BP. Memory consolidation is linked to spindle-mediated information processing during sleep. Curr Biol. (2018) 28:948–954.e4. doi: 10.1016/j.cub.2018.01.087
31. Latchoumane, C-FV, Ngo, H-VV, Born, J, and Shin, H-S. Thalamic spindles promote memory formation during sleep through triple phase-locking of cortical, thalamic, and hippocampal rhythms. Neuron. (2017) 95:424–435.e6. doi: 10.1016/j.neuron.2017.06.025
32. Fernandez, LMJ, and Lüthi, A. Sleep spindles: mechanisms and functions. Physiol Rev. (2020) 100:805–68. doi: 10.1152/physrev.00042.2018
33. Cox, R, and Fell, J. Analyzing human sleep EEG: a methodological primer with code implementation. Sleep Med Rev. (2020) 54:101353. doi: 10.1016/j.smrv.2020.101353
34. Cox, R. Analyzing human sleep EEG [Internet]. (2020). Available at: https://zenodo.org/record/3929730 (Accessed September 1, 2023).
35. Silversmith, DB, Lemke, SM, Egert, D, Berke, JD, and Ganguly, K. The degree of nesting between spindles and slow oscillations modulates neural synchrony. J Neurosci. (2020) 40:4673–84. doi: 10.1523/JNEUROSCI.2682-19.2020
36. Martínez-Cagigal, V. Topographic EEG/MEG plot [internet]. (2023). Available at: https://www.mathworks.com/matlabcentral/fileexchange/72729-topographic-eeg-meg-plot.
37. Aarts, E, Verhage, M, Veenvliet, JV, Dolan, CV, and van der Sluis, S. A solution to dependency: using multilevel analysis to accommodate nested data. Nat Neurosci. (2014) 17:491–6. doi: 10.1038/nn.3648
38. Sullivan, GM, and Feinn, R. Using effect size—or why the p value is not enough. J Grad Med Educ. (2012) 4:279–82. doi: 10.4300/JGME-D-12-00156.1
39. Cassidy, JM, Wodeyar, A, Wu, J, Kaur, K, Masuda, AK, Srinivasan, R, et al. Low-frequency oscillations are a biomarker of injury and recovery after stroke. Stroke. (2020) 51:1442–50. doi: 10.1161/STROKEAHA.120.028932
40. Carmichael, ST, and Chesselet, MF. Synchronous neuronal activity is a signal for axonal sprouting after cortical lesions in the adult. J Neurosci. (2002) 22:6062–70. doi: 10.1523/JNEUROSCI.22-14-06062.2002
41. Gulati, T, Won, SJ, Ramanathan, DS, Wong, CC, Bodepudi, A, Swanson, RA, et al. Robust neuroprosthetic control from the stroke perilesional cortex. J Neurosci. (2015) 35:8653–61. doi: 10.1523/JNEUROSCI.5007-14.2015
42. Tu-Chan, AP, Natraj, N, Godlove, J, Abrams, G, and Ganguly, K. Effects of somatosensory electrical stimulation on motor function and cortical oscillations. J Neuroeng Rehabil. (2017) 14:113. doi: 10.1186/s12984-017-0323-1
43. van Dellen, E, Hillebrand, A, Douw, L, Heimans, JJ, Reijneveld, JC, and Stam, CJ. Local polymorphic delta activity in cortical lesions causes global decreases in functional connectivity. NeuroImage. (2013) 83:524–32. doi: 10.1016/j.neuroimage.2013.06.009
44. Topolnik, L, Steriade, M, and Timofeev, I. Partial cortical Deafferentation promotes development of paroxysmal activity. Cereb Cortex. (2003) 13:883–93. doi: 10.1093/cercor/13.8.883
45. Helfrich, RF, Mander, BA, Jagust, WJ, Knight, RT, and Walker, MP. Old brains come uncoupled in sleep: slow wave-spindle synchrony, brain atrophy, and forgetting. Neuron. (2018) 97:221–230.e4. doi: 10.1016/j.neuron.2017.11.020
46. Maingret, N, Girardeau, G, Todorova, R, Goutierre, M, and Zugaro, M. Hippocampo-cortical coupling mediates memory consolidation during sleep. Nat Neurosci. (2016) 19:959–64. doi: 10.1038/nn.4304
47. Antony, JW, Piloto, L, Wang, M, Pacheco, P, Norman, KA, and Paller, KA. Sleep spindle refractoriness segregates periods of memory reactivation. Curr Biol. (2018) 28:1736–1743.e4. doi: 10.1016/j.cub.2018.04.020
48. Staresina, BP, Bergmann, TO, Bonnefond, M, van der Meij, R, Jensen, O, Deuker, L, et al. Hierarchical nesting of slow oscillations, spindles and ripples in the human hippocampus during sleep. Nat Neurosci. (2015) 18:1679–86. doi: 10.1038/nn.4119
49. Bergmann, TO, and Born, J. Phase-amplitude coupling: a general mechanism for memory processing and synaptic plasticity? Neuron. (2018) 97:10–3. doi: 10.1016/j.neuron.2017.12.023
50. Peyrache, A, Khamassi, M, Benchenane, K, Wiener, SI, and Battaglia, FP. Replay of rule-learning related neural patterns in the prefrontal cortex during sleep. Nat Neurosci. (2009) 12:919–26. doi: 10.1038/nn.2337
51. Bhattacharya, S, Donoghue, JA, Mahnke, M, Brincat, SL, Brown, EN, and Miller, EK. Propofol anesthesia alters cortical traveling waves. J Cogn Neurosci. (2022) 34:1274–86. doi: 10.1162/jocn_a_01856
52. Soplata, AE, Adam, E, Brown, EN, Purdon, PL, McCarthy, MM, and Kopell, N. Rapid thalamocortical network switching mediated by cortical synchronization underlies propofol-induced EEG signatures: A biophysical model. J Neurophysiol. (2023) 130:86–103. doi: 10.1152/jn.00068.2022
53. Bauerschmidt, A, al-Bermani, T, Ali, S, Bass, B, Dorilio, J, Rosenberg, J, et al. Modern sedation and analgesia strategies in neurocritical care. Curr Neurol Neurosci Rep. (2023) 23:149–58. doi: 10.1007/s11910-023-01261-7
54. Kang, Y, Saito, M, and Toyoda, H. Molecular and regulatory mechanisms of desensitization and Resensitization of GABAA receptors with a special reference to Propofol/barbiturate. Int J Mol Sci. (2020) 21:563. doi: 10.3390/ijms21020563
55. Ouyang, W, Wang, G, and Hemmings, HC. Isoflurane and propofol inhibit voltage-gated sodium channels in isolated rat neurohypophysial nerve terminals. Mol Pharmacol. (2003) 64:373–81. doi: 10.1124/mol.64.2.373
56. Tang, P, and Eckenhoff, R. Recent progress on the molecular pharmacology of propofol. F1000Res. (2018) 7:123. doi: 10.12688/f1000research.12502.1
57. Kondili, E, Alexopoulou, C, Xirouchaki, N, and Georgopoulos, D. Effects of propofol on sleep quality in mechanically ventilated critically ill patients: a physiological study. Intensive Care Med. (2012) 38:1640–6. doi: 10.1007/s00134-012-2623-z
58. Yue, X-F, Wang, A-Z, Hou, Y-P, and Fan, K. Effects of propofol on sleep architecture and sleep–wake systems in rats. Behav Brain Res. (2021) 411:113380. doi: 10.1016/j.bbr.2021.113380
59. Lynch, BA, Lambeng, N, Nocka, K, Kensel-Hammes, P, Bajjalieh, SM, Matagne, A, et al. The synaptic vesicle protein SV2A is the binding site for the antiepileptic drug levetiracetam. Proc Natl Acad Sci U S A. (2004) 101:9861–6. doi: 10.1073/pnas.0308208101
60. Luz Adriana, PM, Blanca Alcira, RM, Itzel Jatziri, CG, Sergio Roberto, ZH, Juan Luis, CP, Karla Berenice, SH, et al. Effect of levetiracetam on extracellular amino acid levels in the dorsal hippocampus of rats with temporal lobe epilepsy. Epilepsy Res. (2018) 140:111–9. doi: 10.1016/j.eplepsyres.2018.01.004
61. Bell, C, Vanderlinden, H, Hiersemenzel, R, Otoul, C, Nutt, D, and Wilson, S. The effects of levetiracetam on objective and subjective sleep parameters in healthy volunteers and patients with partial epilepsy. J Sleep Res. (2002) 11:255–63. doi: 10.1046/j.1365-2869.2002.00301.x
62. Bazil, CW, Battista, J, and Basner, RC. Effects of levetiracetam on sleep in normal volunteers. Epilepsy Behav. (2005) 7:539–42. doi: 10.1016/j.yebeh.2005.08.001
63. Murphy, M, Bruno, M-A, Riedner, BA, Boveroux, P, Noirhomme, Q, Landsness, EC, et al. Propofol anesthesia and sleep: a high-density EEG study. Sleep. (2011) 34:283–91. doi: 10.1093/sleep/34.3.283
64. Yeh, W-C, Lu, S-R, Wu, M-N, Lee, KW, Chien, CF, Fong, YO, et al. The impact of antiseizure medications on polysomnographic parameters: a systematic review and meta-analysis. Sleep Med. (2021) 81:319–26. doi: 10.1016/j.sleep.2021.02.056
65. Purdon, PL, Pierce, ET, Mukamel, EA, Prerau, MJ, Walsh, JL, Wong, KF, et al. Electroencephalogram signatures of loss and recovery of consciousness from propofol. Proc Natl Acad Sci U S A. (2013) 110:E1142–51. doi: 10.1073/pnas.1221180110
66. Bernhardt, J, Hayward, KS, Kwakkel, G, Ward, NS, Wolf, SL, Borschmann, K, et al. Agreed definitions and a shared vision for new standards in stroke recovery research: the stroke recovery and rehabilitation roundtable taskforce. Neurorehabil Neural Repair. (2017) 31:793–9. doi: 10.1177/1545968317732668
67. Pearson-Fuhrhop, KM, Kleim, JA, and Cramer, SC. Brain plasticity and genetic factors. Top Stroke Rehabil. (2009) 16:282–99. doi: 10.1310/tsr1604-282
68. Ganguly, K, Byl, NN, and Abrams, GM. Neurorehabilitation: motor recovery after stroke as an example. Ann Neurol. (2013) 74:373–81. doi: 10.1002/ana.23994
69. Dromerick, AW, Lang, CE, Birkenmeier, RL, Wagner, JM, Miller, JP, Videen, TO, et al. Very early constraint-induced movement during stroke rehabilitation (VECTORS): a single-center RCT. Neurology. (2009) 73:195–201. doi: 10.1212/WNL.0b013e3181ab2b27
70. Dromerick, AW, Geed, S, Barth, J, Brady, K, Giannetti, ML, Mitchell, A, et al. Critical period after stroke study (CPASS): a phase II clinical trial testing an optimal time for motor recovery after stroke in humans. Proc Natl Acad Sci. (2021) 118:e2026676118. doi: 10.1073/pnas.2026676118
71. Krakauer, JW, Carmichael, ST, Corbett, D, and Wittenberg, GF. Getting neurorehabilitation right: what can be learned from animal models? Neurorehabil Neural Repair. (2012) 26:923–31. doi: 10.1177/1545968312440745
72. Clarkson, AN, Huang, BS, MacIsaac, SE, Mody, I, and Carmichael, ST. Reducing excessive GABA-mediated tonic inhibition promotes functional recovery after stroke. Nature. (2010) 468:305–9. doi: 10.1038/nature09511
73. He, W-M, Ying-Fu, L, Wang, H, and Peng, Y-P. Delayed treatment of α5 GABAA receptor inverse agonist improves functional recovery by enhancing neurogenesis after cerebral ischemia-reperfusion injury in rat MCAO model. Sci Rep. (2019) 9:2287. doi: 10.1038/s41598-019-38750-0
74. Marshall, L, Helgadottir, H, Molle, M, and Born, J. Boosting slow oscillations during sleep potentiates memory. Nature. (2006) 444:610–3. doi: 10.1038/nature05278
75. Ngo, H-VV, Martinetz, T, Born, J, and Mölle, M. Auditory closed-loop stimulation of the sleep slow oscillation enhances memory. Neuron. (2013) 78:545–53. doi: 10.1016/j.neuron.2013.03.006
76. Levy, RM, Harvey, RL, Kissela, BM, Winstein, CJ, Lutsep, HL, Parrish, TB, et al. Epidural electrical stimulation for stroke rehabilitation: results of the prospective, multicenter, randomized, single-blinded Everest trial. Neurorehabil Neural Repair. (2016) 30:107–19. doi: 10.1177/1545968315575613
77. Khanna, P, Totten, D, Novik, L, Roberts, J, Morecraft, RJ, and Ganguly, K. Low-frequency stimulation enhances ensemble co-firing and dexterity after stroke. Cells. (2021) 184:912–930.e20. doi: 10.1016/j.cell.2021.01.023
78. Ramanathan, DS, Guo, L, Gulati, T, Davidson, G, Hishinuma, AK, Won, SJ, et al. Low-frequency cortical activity is a neuromodulatory target that tracks recovery after stroke. Nat Med. (2018) 24:1257–67. doi: 10.1038/s41591-018-0058-y
79. Abbasi, A, Danielsen, NP, Leung, J, Muhammad, AKMG, Patel, S, and Gulati, T. Epidural cerebellar stimulation drives widespread neural synchrony in the intact and stroke perilesional cortex. J Neuroeng Rehabil. (2021) 18:89. doi: 10.1186/s12984-021-00881-9
Keywords: stroke, sleep, EEG, spindles, slow wave oscillations, non-rapid eye movement sleep, delta waves
Citation: Simpson BK, Rangwani R, Abbasi A, Chung JM, Reed CM and Gulati T (2023) Disturbed laterality of non-rapid eye movement sleep oscillations in post-stroke human sleep: a pilot study. Front. Neurol. 14:1243575. doi: 10.3389/fneur.2023.1243575
Edited by:
Roberto Rodríguez-Labrada, Cuban Neuroscience Center, CubaReviewed by:
Xiaojia Ni, Guangzhou University of Traditional Chinese Medicine, ChinaEric Landsness, Washington University in St. Louis, United States
Copyright © 2023 Simpson, Rangwani, Abbasi, Chung, Reed and Gulati. This is an open-access article distributed under the terms of the Creative Commons Attribution License (CC BY). The use, distribution or reproduction in other forums is permitted, provided the original author(s) and the copyright owner(s) are credited and that the original publication in this journal is cited, in accordance with accepted academic practice. No use, distribution or reproduction is permitted which does not comply with these terms.
*Correspondence: Tanuj Gulati, tanuj.gulati@csmc.edu
†These authors have contributed equally to this work and share first authorship
‡These authors have contributed equally to this work