Multi-sensory weights depend on contextual noise in reference frame transformations
- 1 Centre for Neuroscience Studies, Queen’s University, Kingston, ON, Canada
- 2 Canadian Action and Perception Network (CAPnet), Canada
During reach planning, we integrate multiple senses to estimate the location of the hand and the target, which is used to generate a movement. Visual and proprioceptive information are combined to determine the location of the hand. The goal of this study was to investigate whether multi-sensory integration is affected by extraretinal signals, such as head roll. It is believed that a coordinate matching transformation is required before vision and proprioception can be combined because proprioceptive and visual sensory reference frames do not generally align. This transformation utilizes extraretinal signals about current head roll position, i.e., to rotate proprioceptive signals into visual coordinates. Since head roll is an estimated sensory signal with noise, this head roll dependency of the reference frame transformation should introduce additional noise to the transformed signal, reducing its reliability and thus its weight in the multi-sensory integration. To investigate the role of noisy reference frame transformations on multi-sensory weighting, we developed a novel probabilistic (Bayesian) multi-sensory integration model (based on Sober and Sabes, 2003) that included explicit (noisy) reference frame transformations. We then performed a reaching experiment to test the model’s predictions. To test for head roll dependent multi-sensory integration, we introduced conflicts between viewed and actual hand position and measured reach errors. Reach analysis revealed that eccentric head roll orientations led to an increase of movement variability, consistent with our model. We further found that the weighting of vision and proprioception depended on head roll, which we interpret as being a result of signal dependant noise. Thus, the brain has online knowledge of the statistics of its internal sensory representations. In summary, we show that sensory reliability is used in a context-dependent way to adjust multi-sensory integration weights for reaching.
Introduction
We are constantly presented with a multitude of sensory information about ourselves and our environment. Using multi-sensory integration, our brains combine all available information from each sensory modality (e.g., vision, audition, somato-sensation, etc.) (Landy et al., 1995; Landy and Kojima, 2001; Ernst and Bulthoff, 2004; Kersten et al., 2004; Stein and Stanford, 2008; Burr et al., 2009; Green and Angelaki, 2010). Although this tactic seems redundant, considering that the senses often provide similar information, having more than one sensory modality contributing to the representation of ourselves in the environment reduces the chance of processing error (Ghahramani et al., 1997). It becomes especially important when the incoming sensory representations we receive are conflicting. When this occurs, the reliability assigned to each modality determines how much we can trust the information provided. Here we explore how context-dependent sensory-motor transformations affect the modality-specific reliability.
Multi-sensory integration is a process that incorporates sensory information to create the best possible representation of ourselves in the environment. Our brain uses knowledge of how reliable each sensory modality is, and weights the incoming information accordingly (Stein and Meredith, 1993; Landy et al., 1995; Atkins et al., 2001; Landy and Kojima, 2001; Kersten et al., 2004; Stein and Stanford, 2008). Bayesian integration is an approach that assigns these specific weights in a statistically optimal fashion based on how reliable the cues are (Mon-Williams et al., 1997; Ernst and Banks, 2002; Knill and Pouget, 2004). For example, when trying to figure out where our hand is, we can use both visual and proprioceptive (i.e., sensed) information to determine its location (Van Beers et al., 2002; Ren et al., 2006, 2007). When visual information is available it is generally weighted more heavily than proprioceptive information due to the higher spatial accuracy that is associated with it (Hagura et al., 2007).
Previous studies have used reaching tasks to specifically examine how proprioceptive and visual information is weighted and integrated (Van Beers et al., 1999; Sober and Sabes, 2003, 2005). When planning a reaching movement, knowledge about target position relative to the starting hand location is required to create a movement vector. This movement vector is then used to calculate how joint angles have to change for the hand to move from the starting location to the target position using inverse kinematics and dynamics (Jordan and Rumelhart, 1992; Jordan et al., 1994). The assessment of target position is generally obtained through vision, whereas initial hand position (IHP) can be calculated using both vision and proprioception (Rossetti et al., 1995). Although it is easy to recognize what different sources of information are used to calculate IHP, knowing how this information is weighted and integrated is not.
The problem we are addressing in this manuscript is that visual and proprioceptive information are encoded separately in different coordinate frames. If both of these cues are believed to have the same cause then they can be integrated into a single estimate. However if causality is not certain then the nervous system might treat both signals separately; the degree of causal belief can thus affect multi-sensory integration (Körding and Tenenbaum, 2007). An important aspect that has never been considered explicitly is that in order for vision and proprioception to be combined, they must be represented in the same coordinate frame (Buneo et al., 2002). In other words, one set of information will have to be transformed into a representation that matches the other. Such a coordinate transformation between proprioceptive and visual coordinates depends on the orientation of the eyes and head and is potentially quite complex (Blohm and Crawford, 2007). The question then becomes, what set of information will be encoded into the other? In reaching, it is thought that this transformation depends on the stage of reach planning. Sober and Sabes (2003, 2005) proposed a dual-comparison hypothesis describing how information from vision and proprioception could be combined during a reaching task. They suggest that visual and proprioceptive signals are combined at two different stages. First, when the movement plan is being determined in visual coordinates; and second, when the visual movement plan is transformed into a motor command (proprioceptive coordinates). The latter requires knowledge of IHP in joint coordinates. They showed that estimating the position of the arm for movement planning relied mostly on visual information, whereas proprioceptive information was more heavily weighted when determining current joint angle configuration to compute the inverse kinematics. The reason why there should be two separate estimates (one in visual and one in proprioceptive coordinates) lies in the mathematical fact that the maximum likelihood estimate is different in both coordinates systems (Koerding and Tenenbaum, 2007; McGuire and Sabes, 2009). Therefore, having two distinct estimates reduces the overall estimation uncertainty because no additional transformations that might introduce noise are required.
The main hypothesis of this previous work was that the difference in sensory weighting between reference frames arises from the cost of transformation between reference frames. This idea is based on the assumption that any transformation induces noise to the transformed signal. In general, noise can arise from at least two distinct sources, i.e., from variability in the sensory readings and from the stochastic behavior of spike-mediated signal processing in the brain. Adding noise in the reference frame transformation thus increases uncertainty in coordinate alignment (Körding and Tenenbaum, 2007) resulting in lower reliability of the transformed signal and therefore lower weighting (Sober and Sabes, 2003; McGuire and Sabes, 2009). While it seems unlikely that neuronal noise from the stochastic behavior of spike-mediated signal processing changes across experimental conditions (this is believed to be a constant in a given brain area), uncertainty of coordinate alignment should increase with head roll. This is based on the hypothesis that the internal estimates of the head orientation signals themselves would be more variable (noisy) for head orientations away from primary (up-right) positions (Wade and Curthoys, 1997; Van Beuzekom and Van Gisbergen, 2000; Blohm and Crawford, 2007). This variability could be caused by signal-dependent noise in muscle spindle firing rates, or in vestibular neurons signaling head orientation (Lechner-Steinleitner, 1978; Scott and Loeb, 1994; Cordo et al., 2002; Sadeghi et al., 2007; Faisal et al., 2008).
To evaluate the notion that multi-sensory integration occurs, subjects performed a reaching task where visual and proprioceptive information about hand position differed. We expanded Sober and Sabes (2003, 2005) model into a fully Bayesian model to test how reference frame transformation noise affects multi-sensory integration. To behaviorally test this, we introduced context changes by altering the subject’s head roll angle. Again, the rationale was that head roll would affect the reference frame transformations that have to occur during reach planning (Blohm and Crawford, 2007) but would not affect the reliability of primary sensory information (i.e., vision or proprioception). Importantly, we hypothesized that larger head roll noise would lead to noisier reference frame transformations, which in turn would render any transformed signal less reliable. Our main goal was to determine the effect of head roll on sensory transformations and its consequences for multi-sensory integration weights.
Materials and Methods
Participants
Experiments were performed on seven participants between 20 and 24 years of age, all of whom had normal or corrected to normal vision. Participants performed the reaching task with their dominant right hand. All of the participants gave their written informed consent to the experimental conditions that were approved by the Queen’s University General Board of Ethics.
Apparatus
While seated, participants performed a reaching task in an augmented reality setup (Figure 1A) using a Phantom Haptic Interface 3.0L (Sensable Technologies; Woburn, MA, USA). Their heads were securely positioned using a mounted bite bar that could be adjusted vertically (up/down), tilted forward and backward (head pitch), and rotated left/right (head roll to either shoulder). Subjects viewed stimuli that were projected onto an overhead screen through a semi-mirrored surface (Figure 1A). Underneath this mirrored surface was an opaque board that prevented the subjects from viewing their hand. In order to track reaching movements, subjects grasped a vertical handle (attached to the Phantom Robot) mounted on an air sled that slid across a horizontal glass surface at elbow height.
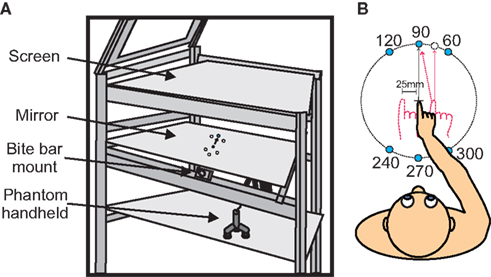
Figure 1. Experimental set up and apparatus. (A) Experimental apparatus. Targets were displayed on semi-silvered mirror. Subjects head position was kept in place using a bite bar. Reaches were made below the semi-silvered mirror using the Phantom robot. (B) A top view of the subject with all possible target positions. Initial hand positions are shown (−25, 0, and 25 mm). Subjects began each trial by aligning the visual cue representing their hand with the center cross, and then continued by reaching to one of six targets that would appear (see text for details).
Eye movements were recorded using electrooculography (EOG), (16-channel Bagnoli EMG system; DELSYS; Boston, MA, USA). Two pairs of electrodes were placed on the face (Blue Sensor M; Ambu; Ballerup, Denmark). The first pair was located on the outer edges of the left and right eyes to measure horizontal eye movements. The second pair was placed above and below one of the subject’s eyes to measure vertical eye movements. An additional ground electrode was placed on the first lumbar vertebrae, to record external electrical noise (Dermatrode; American Imex; Irvine, CA, USA).
Task Design
Subjects began each trial by aligning a blue dot (0.5 cm) on the display that represented their unseen hand position with a start position (cross) that was positioned in the center of the display field. A perturbation was introduced such that the visual position of the IHP was constant but the actual IHP of the reach varied among three positions (−25, 0, and 25 mm horizontally with respect to visual start position – VSP). The blue dot representing the hand was only visible when hand position was within 3 cm of the central cross. Once the hand was in this position, one of six peripheral targets (1.0 cm white dots) would randomly appear 250 ms later. The appearance of a target was accompanied by an audio cue. At the same time the center cross turned yellow. Once the subject’s hand began to move the hand cursor disappeared. Subjects were instructed to perform rapid reaching movements toward the visual targets while keeping gaze fixated on the center position (cross). Targets were positioned at 10-cm distance from the start position cross at 60, 90, 120, 240, 270, and 300° (see Figure 1B).
Once the subject’s reach crossed the 10-cm target circle, an audio cue would indicate that they successfully completed the reach, and the center cross would disappear. If subjects were too slow at reaching this distance threshold (more than 750 ms after target onset), a different audio cue was played, indicating that the trial was aborted and would have to be repeated. At the end of each reach subjects had to wait 500 ms to return to the start position, an audio cue indicated the end of the trial, and the center cross reappeared. This was to ensure subjects received no visual feedback of their performance. Subjects were instructed to fixate the central start position cross (VSP cross) throughout the trial.
Subjects completed the task at three different head roll positions, −30 (left), 0, and 30° (right) head roll toward the shoulders (mathematical angle convention from behind subject view). Throughout each head roll condition the proprioceptive information about hand position was altered at random trials, 2.5-cm left or right of the visual hand marker. For example, subjects would align the visual circle representing their hand with the start cross, but their actual hand position may be shifted to the right or left, 2.5 cm. Subjects were not aware of the IHP shift when asked after the experiment. We introduced this discrepancy between visual and actual hand position to gain insight into the relative weighting of both signals in the multi-sensory integration process. For each hand offset subjects reached to each target twenty times, and they did this for each head roll. Subjects completed 360 trials at each head position, for a total of 1080 reaches. Head roll was constant within a block of trials.
Data Analysis
Eye and hand movements were monitored online at a sampling rate of 1000 Hz (16-channel Bagnoli EMG system, Delsys; Boston, MA, USA; Phantom Haptic Interface 3.0L; Sensable Technologies; Woburn, MA, USA). Offline analyses were performed using Matlab (The Mathworks, Natick, MA, USA). Arm position data was low-pass filtered (autoregressive forward–backward filter, cutoff frequency = 50 Hz) and differentiated twice (central difference algorithm) to obtain hand velocity and acceleration (Figure 2). Each trial was visually inspected to ensure that eye movements did not occur while the target was presented (Figure 2C). If they did occur, the trial was removed from the analysis. Approximately 5% of trials (384 of 7560 trials) were removed due to eye movements. Hand movement onset and offset were identified based on a hand acceleration criterion (500 mm/s2), and could be adjusted after visual inspection (Figure 2E). The movement angle was calculated through regression of the data points from the initial hand movement until the hand crossed the 10-cm circle around the IHP cross. Directional movement error was calculated as the difference between overall movement angle and visual target angle.
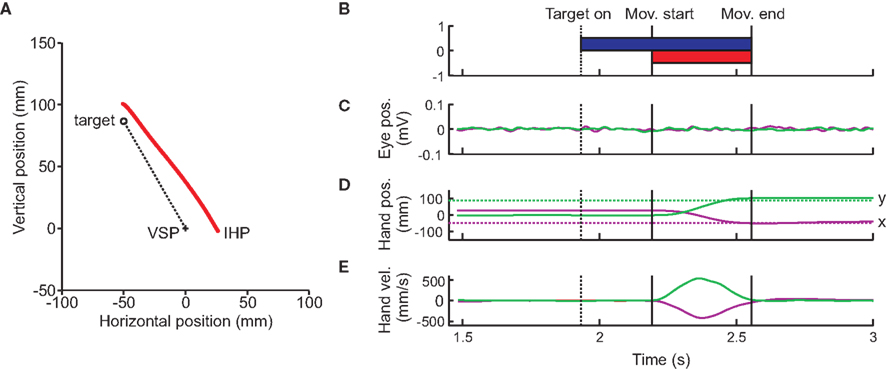
Figure 2. Typical subject trial. (A) Raw reach data from a typical trial displayed. The viewed required reach (dotted line) begins at the cross (visual start position, VSP), and ends at the target (open circle). The red line represents the subjects actual hand position. The subject starts this reach with an initial hand position (IHP) offset to the right by 25 mm. (B) Target onset and display. Timing of the trial begins when the subject aligns the hand cursor with the visual start position. The target then appears, and remains on until the end of the trial. Movement onset, as well as offset times are shown by the vertical lines. (C) Eye movement traces. Horizontal (purple) and vertical (green) eye movement traces, from EOG recordings. Subjects were instructed to keep the eyes fixated on the VSP for the entire length of the trial. Black vertical lines indicate arm movement start and end. (D) Hand position traces. Horizontal (purple) and vertical (green) hand positions (solid lines) as well as the horizontal and vertical target position (dotted lines) are plotted over time. (E) Hand velocity traces relative to time.
Modeling the Initial Movement Direction
The data was fitted to two models, one previously published velocity command model (Sober and Sabes, 2003) and a second fully Bayesian model that had processing steps similar to Sober and Sabes (2003). In addition the second, new model includes explicit reference frame transformations and – more importantly – explicit transformations of the sensory noise throughout the model. Explicit noise has previously been use to determine multi-sensory integration weights (McGuire and Sabes, 2009); however, they only considered one-dimensional cases (we model the problem in 2D). Furthermore they did not model reference frame transformations explicitly nor model movement variability in the output (nor analyze movement variability in the data). Below, we outline the general working principle of the model; please refer to Appendix 1 for model details.
The purpose of these models was to determine the relative weighting of both vision and proprioception during reach planning, separately for each head roll angle. Sober and Sabes (2003, 2005) proposed that IHP is computed twice, once in visual and once in proprioceptive coordinates (Figure 3A). In order to determine the IHP in visual coordinates (motor planning stage, left dotted box in Figure 3A), proprioceptive information about the hand must be transformed into visual coordinates (Figure 3A, red “T” box) using head orientation information both the visual and the transformed proprioceptive information are weighted based on reliability, and IHP is calculated. This IHP can then be subtracted from the target position to create a desired movement vector (Δx). If the hand position is misestimated (due to IHP offset), then there will be an error associated with the desired movement vector.
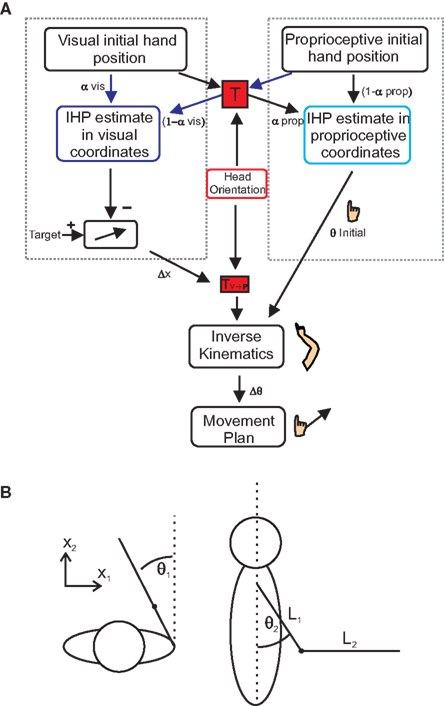
Figure 3.Multi-sensory integration model. (A) Model for multi-sensory integration for reach planning. In order to successfully complete a reach, hand position estimates have to be calculated in both proprioceptive (right dotted box) and visual coordinates (left dotted box). Initial hand position estimates in visual coordinates are computed by transforming proprioceptive information into visual coordinates (transformation “T”). Visual and proprioceptive information is then weighted and combined (visual weights α; proprioceptive weights1 −?α). The same processes take place for proprioceptive IHP estimates; only this time visual information is transformed into proprioceptive coordinates. Subtracting visual IHP from the visual target location, a movement vector can be created. Using inverse kinematics, the movement vector is combined with the calculation of initial joint angles, derived from the IHP in proprioceptive coordinates to create a movement plan based on changes in joint angles. (B) Spatial arm position (x) can be characterized in terms of two joint angles, deviation from straight ahead (θ1) and upper arm elevation (θ2). The arm and forearm lengths are represented by “L” (see text for details).
As a final processing step, this movement vector will undergo a transformation to be represented in a shoulder based reference frame (Figure 3A, TV→P box). Initial joint angles are calculated by transforming visual information about hand location into proprioceptive coordinates (Figure 3A, rightward arrows through red “T” box). This information is weighted along with the proprioceptive information, to calculate IHP in proprioceptive coordinates (right dotted box in Figure 3A) and is used to create an estimate of initial elbow and shoulder joint angles (θ initial). Using inverse kinematics, a change in joint angles (Δθ), from the initial starting position to the target is calculated based on the desired movement vector. Since the estimate of initial joint angles (θ initial) is needed to compute the inverse kinematics, misestimation of initial joint angles will lead to errors associated with the inverse kinematics, and therefore error in the movement. We wanted to see how changing head roll would affect the weighting of visual and proprioceptive information. As can be seen from Figure 3A, our model reflects the idea that head orientation affects this transformation. This is because we hypothesize (and hope to demonstrate through our data) that transformations add noise to the transformed signal and that the amount of this noise depends on the amplitude of the head roll angle. Therefore, we predict that head roll has a significant effect on the estimations of IHP, thus changing the multi-sensory integration weights and in turn affecting the accuracy of the movement plan.
Results
To test the model’s predictions, we asked participants to perform reaching movements while we varied head roll and dissociated visual and proprioceptive IHPs.
General Observations
A total of 7560 trials were collected, with 384 trials being excluded due to eye movements. Subjects were unaware of the shifts in IHP. We used reaching errors to determine how subjects weighted visual and proprioceptive information. Reach error (in angular degrees) was computed as the angle between the movement and the visual hand–target vector, where 0° error would mean no deviation from the visual hand–target direction. As a result of the shifts in the actual starting hand locations, a situation was created where the subject received conflicting visual and proprioceptive information (Figure 2). Based on how the subject responded to this discrepancy, we could determine how information was weighted and integrated.
Figure 4 displays nine sets of raw data reaches from a typical subject, depicting 10 reaches to each target. Every tenth data point is plotted for each reach, i.e., data points are distant in time by 10 ms, allowing the changes in speed to be visually identifiable. The targets are symbolized by black circles, with the visual start position marked by a cross. Each set of reaches is representative of a particular head roll angle (rows) and IHP (columns). One can already observe from these raw traces that this subject weighted visual IHP more than proprioceptive information resulting in a movement path that is approximately parallel to a virtual line between the visual cross and target locations.
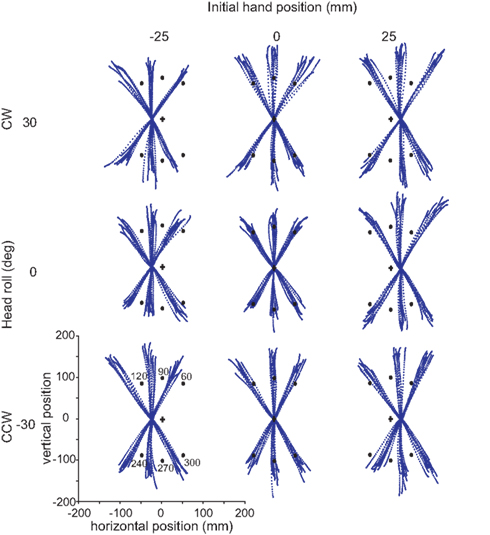
Figure 4. Raw reaches from a typical subject. Each grouping of reaches corresponds to a particular head orientation (30°, 0°, −30°) and initial hand position (−25, 0, 25 mm). In each block, ten trials are plotted for each target (black dots). Target angles are 60°, 90°, 120°, 240°, 270°, and 300°. The data points for each reach trajectory represent every tenth data point.
To further analyze this behavior, we compared the reach error (in degrees) for each hand offset condition (Figure 5A). This graph also displays a breakdown of the data for each target angle and shows a shift in reach errors between the different IHPs. The difference in reach errors between the each of the hand offsets indicates that both visual and proprioceptive information were used during reach planning. Figure 5B shows a fit from Sober and Sabes’ (2003, 2005) previously proposed model to the normalized data from Figure 5A (see also Appendix 1 for model details). The data from Figure 5A were normalized to 0 by subtracting the 0 hand offset from the IHPs 25 and −25 mm. Sober and Sabes’ (2003) previously proposed velocity command model fit our data well. In Figure 5B, it is clear that the normalized data points for each hand position follow the same pattern as the model predicted error, represented by the dotted lines. Based on this close fit of our data to the model, we can now use this model in a first step to investigate how head roll affects the weighting of vision and proprioceptive information about the hand.
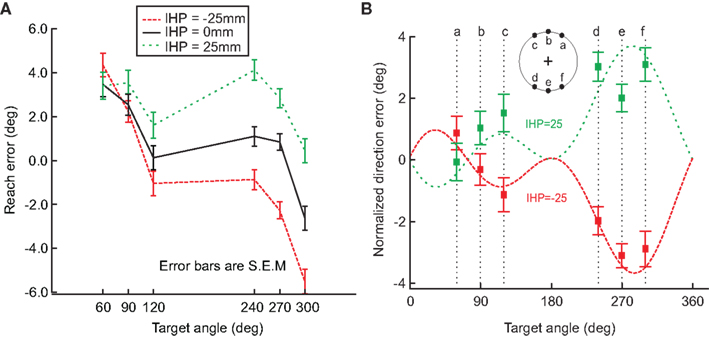
Figure 5. Hand offset effects. (A) This graph demonstrates a shift in reach errors between each initial hand position (−25, 0, 25 mm), suggesting that visual and proprioceptive information are both used when reaching. (B) Model fit. The data from A was normalized to 0, and plotted against the model fit (dotted lines; initial hand position 25 (green) and −25 (red)). The squared points represent the normalized direction error for each initial hand position at each target angle. This graph demonstrates that the model previously proposed by Sober and Sabes (2003) fits our data. Error bars represent standard error of the mean.
Head Roll Influences on Reach Errors
As mentioned before, subjects performed the experiment described above for each head roll condition, i.e. −30, 0, and 30° head roll (to the left shoulder, upright and to the right shoulder respectively). We assumed that if head roll was not taken into account, there would be no difference in the reach errors between the head roll conditions. Alternatively, if head roll was accounted for, then we would expect at least two distinct influences of head roll. First, head roll estimation might not be accurate, which would lead to an erroneous rotation of the visual information into proprioceptive coordinates. This would be reflected in an overall shift of the reach error curve up/downward for eccentric head roll angles compared to the head straight-ahead. Second, head roll estimation might not be very precise, i.e., not very reliable. In that case, variability in the estimation should affect motor planning and thus increase movement variability overall and multi-sensory integration weights in particular. We will test these predictions below. Figure 6 shows differences in reach errors between the different head roll conditions, indicating that head roll was a factor influencing reach performance. This is a novel finding that has never been considered in any previous model.
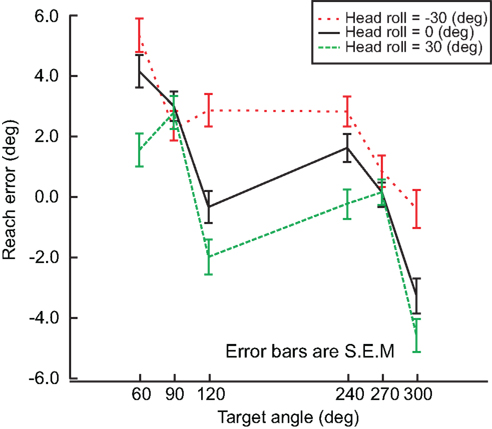
Figure 6. Head roll effects. Reach errors are plotted for each head roll (−30°, 0°, 30°) at each target angle (60°, 90°, 120°, 240°, 270°, 300°). The difference in reach error indicates that information about head roll in taken into account. Error bars represent standard error of the mean.
From our model (Figure 3A), we predicted that as head roll moves away from 0, more noise would be associated with the signal (Scott and Loeb, 1994; Blohm and Crawford, 2007; Tarnutzer et al., 2009). This increase in noise should affect the overall movement variability (i.e., standard deviation, SD) because more noise in the head roll signal should result in more noise added during the reference frame transformation process. Figure 7 plots movement variability for trials where the head was upright compared to rolled to the left or right combined.
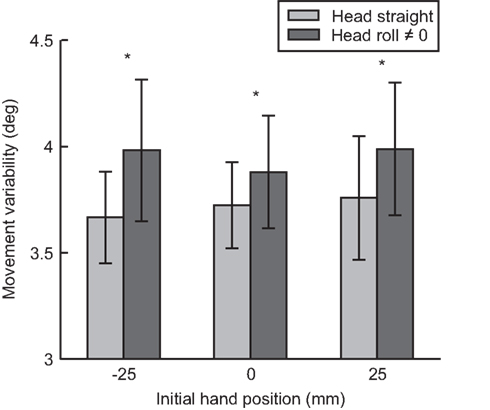
Figure 7. Movement variability as a function of head roll. For each initial hand position (−25, 0, 25 mm) movement variability (standard deviation) is compared between head straight and head roll conditions 0, ≠0. Reaches when the head is rolled had significantly more variability compared to reaches when the head is straight.
We performed a paired t-test between head roll and no head roll conditions across all seven subjects and all hand positions (21 standard deviation values per head roll conditions). Across all three IHPs, movement variability was significantly greater when the head was rolled compared to when the head was straight t(20) = −3.512, p < 0.01. This was a first indicator that head roll introduced signal-dependent noise to motor planning, likely through noisier reference frame transformations (Sober and Sabes, 2003, 2005; Blohm and Crawford, 2007).
If changing the head roll angle ultimately affects reach variability, then we would expect that the information associated with the increased noise would be weighted less at the multi-sensory integration step. To test this, we fitted Sober and Sabes’ (2003) model on our data independently for each head orientation. The visual weights, of IHPs represented in visual (dark blue, αvis) and proprioceptive (light blue, αprop) coordinates are displayed in Figure 8A. The visual weights of IHP in visual and proprioceptive coordinates were significantly different when the head was rolled compared to the head straight condition (t(20) = −4.217, p< 0.01). Visual information was weighted more heavily when IHP was calculated in visual coordinates compared to proprioceptive coordinates. Furthermore, visual information was weighted significantly more for IHP in visual coordinates for head rolled conditions, compared to head straight. In contrast, visual information was weighted significantly less when the IHP was calculated in proprioceptive coordinates for head rolled conditions compared to head straight. This finding is representative of the fact that information that undergoes a noisy transformation is weighted less due to the noise added by this transformation, e.g., vision is weighted less in proprioceptive as opposed to visual coordinates (Sober and Sabes, 2003, 2005). An even further reduction of weighting of the transformed signal will occur if head roll is introduced, presumably due to signal dependant noise (see Discussion section).
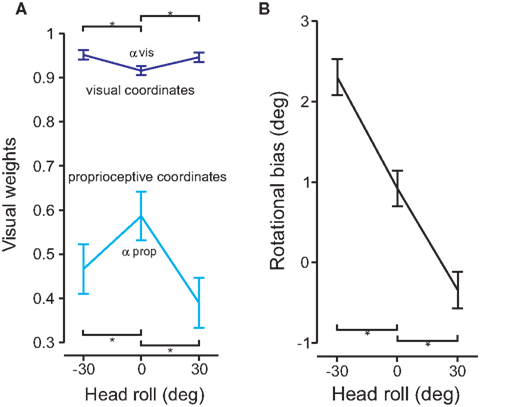
Figure 8. Model fit and rotational biases. (A) The model was fit to the data for each head roll condition. The visual weights for initial hand position estimates are plotted for both visual (dark blue) and proprioceptive (light blue) coordinate frames. Significant differences are denoted by the *(p < 0.05). (B) Rotational biases are plotted and compared for each head roll position (−30°, 0°, 30°).
In addition to accounting for head roll noise, the reference frame transformation also has to estimate the amplitude of the head roll angle. Any misestimation in head roll angle will lead to a rotational movement error. Figure 8B plots the rotation biases (i.e., the overall rotation in movement direction relative to the visual hand–target vector) for each head roll position. The graph shows that there is a rotational bias for reaching movements even for 0° head roll angle. This bias changes depending on head roll. There were significant differences between the rotational biases for head roll conditions compared to head straight (t(20) > 6.891, p < 0.01).
Modeling Noisy Reference Frame Transformations
We developed a full Bayesian model of multi-sensory integration for reach planning. This model uses proprioceptive and visual IHP estimates and combines them in a statistically optimal way, separately in two different representations (Sober and Sabes, 2003, 2005): proprioceptive coordinates and visual coordinates (Figure 3B). The IHP estimate in visual coordinates is compared to target position to compute the desired movement vector while the IHP estimate in proprioceptive coordinates in needed to translate (through inverse kinematics) this desired movement vector into a change of joint angles using a velocity command model. For optimal movement planning, not only are [the point estimates in these two reference] frames are required, but the expected noise in those estimates is also needed (see Appendix).
Compared to previous models (Sober and Sabes, 2003, 2005), our model includes two crucial additional features. First, we explicitly include the required reference transformations (Figure 3A, “T”) from proprioceptive to visual coordinates (and vice versa), including the forward/inverse kinematics for transformation between Euclidean space and joint angles as well as for movement generation. The reference frame transformation T depends on an estimate of body geometry, i.e., head roll angle (Figure 3A, “H”) in our experiment. Second, in addition to modeling the mean behavior, we also include a full description of variability. Visual and proprioceptive sensory information have associated noise, i.e., proprioceptive and visual IHP as well as head roll angle. As a consequence, covariance matrices of all variables also have to undergo the above-mentioned transformations. In addition, these transformations themselves are noisy, i.e., they depend on noisy sensory estimates.
To illustrate how changes in transformation noise, visual noise, and joint angle variability affect predicted reach error, we used the model to simulate these conditions. We did this first to demonstrate that our model can reproduce the general movement error pattern produced by previous models (Sober and Sabes, 2003) and second to show how different noise amplitudes in the sensory variables change this error pattern. Figure 9A displays the differences in predicted error between high, medium and low noise in the reference frame transformation. As the amount of transformation noise increases, the reach error decreases. The transformed signal in both visual and proprioceptive coordinates is weighted less in the presence of higher transformation noise. However, the misestimation of IHP in visual coordinates has a bigger impact on movement error than the IHP estimation in proprioceptive coordinates (Sober and Sabes, 2003, 2005). As a consequence, the gross effect of higher transformation noise is a decrease in movement error because the proprioceptive information will be weighted relatively less after it is converted into visual coordinates.
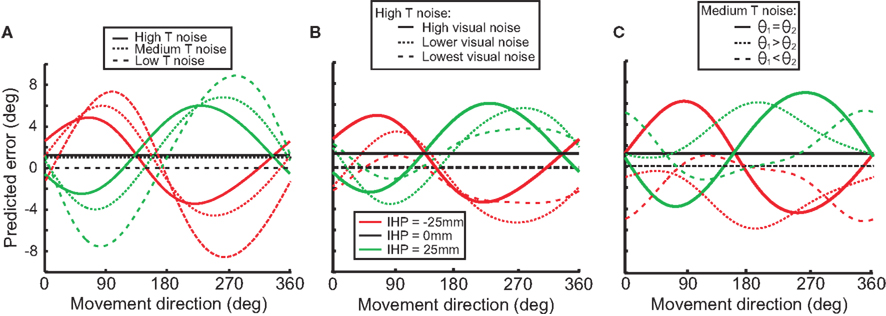
Figure 9. Model simulations. Each graph illustrates how different stimulus parameters affect the predicted reach error for each of the initial hand positions (−25, 0, 25). (A) Low, medium, and high transformation noise is compared. (B) Different magnitudes of visual noise affect predicted error in the high transformation noise condition. (C) Different amounts of noise associated with separate joint angles affects predicted error in the medium transformation noise condition.
Figure 9B illustrates the effect of visual sensory noise (e.g. in situations such as seen versus remembered stimuli) on predicted error in a high transformation noise condition. When the amount of visual noise increases (visual reliability decreases), proprioceptive information will be weighted more, and predicted error will increase. Conversely, as visual noise decreases (reliability increases), predicted error will decrease as well. Differences seen between different movement directions (forward and backward) are due to an interaction effect of transformations for vector planning (visual coordinates) and movement execution (proprioceptive coordinates).
Not only does visual noise impact the predicted error, but proprioceptive information does as well. Noise associated with different joint angles will result in proprioceptive information being weighted less than visual information, and as a result there will be a decrease in predictive error (Figure 9C). Figure 9C displays how changing the amount of noise associated with one joint angle over another can change the predicted error. For example with θ1 > θ2, the signals indicating the arm deviations from the straight-ahead position are noisier than the signals indicating upper arm elevation. With this situation, visual error will be smaller when the targets are straight ahead or behind because the proprioceptive signals for the straight-ahead position are noisier and thus will be weighted less.
Figure 10 displays the model fits to the data for both error (top panels) and variability (lower panels) graphs for each IHP (−25, 0, 25 mm), comparing the different head roll effects. The solid lines represent the model fit for each IHP, with the squared nodes representing the behavioral data for each target. The model fits are different for each head roll position, with 0 head roll falling in between the tilted head positions. The model predicts that −30° head roll and 30° head roll would have reach errors in opposite directions; this is consistent with the data. Furthermore, the model presents 0 head roll as having the least variability when reaching towards the visual targets, with the behavioral data following the same trend.
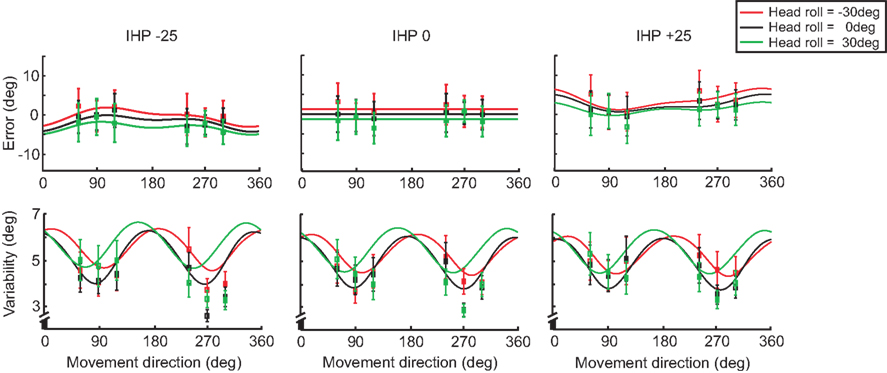
Figure 10. Model fit comparing head roll conditions. For each initial hand position (−25, 0, 25 mm) both reaching error and variability are plotted. The solid lines represent the model fit for each head roll; −30° (red), 0° (black), and 30° (green). The squared points represent the data sampled at each target angle (60°, 90°, 120°, 240°, 270°, 300°).
In addition to modeling the effect of head roll on error and variability, we plotted the differences for IHP as well. Figure 11 displays both error and variability graphs for each head roll condition (same plots as in Figure 10, but re-arranged according to head roll conditions). The reach errors for different IHPs changed in a systematic way; however differences in variability between the IHPs are small and show a similar pattern of variability across movement directions.
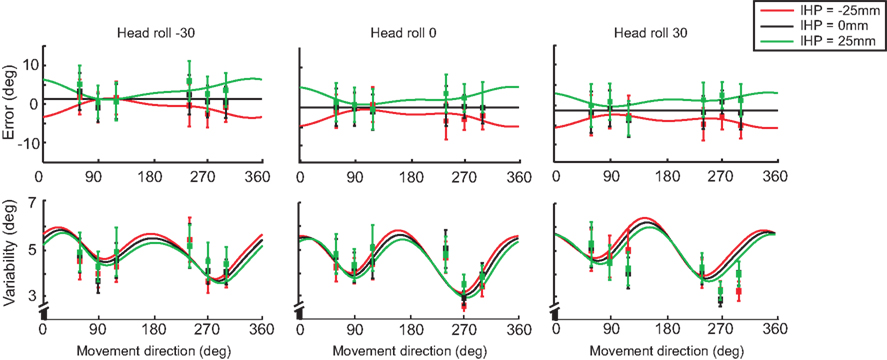
Figure 11. Model fit comparing different initial hand positions. For each head roll angle (−30°, 0°, 30°) both reaching error and variability are plotted. The solid lines represent the model fit for each initial hand position; −25 (red), 0 (black), and 25 mm (green). The squared points represent the data sampled at each target angle (60°, 90°, 120°, 240°, 270°, 300°). Data re-arranged from Figure 10.
Determining how head roll affects multi-sensory weights was the main goal of this experiment. Previously in this section we fitted Sober and Sabes’ (2003) original model to the data, and displayed the visual weights for IHP estimates for both visual and proprioceptive coordinate frames (Figure 8A). In our model, we did not explicitly fit those weights to the data; however, from the covariance matrices of the sensory signals, we could easily recover the multi-sensory weights (see Appendix 1). Since our model uses two-dimensional covariance matrices (a 2D environment allows a visual coordinate frame to be represented in x and y, and proprioceptive coordinates to be displayed by two joint angles), the recovered multi-sensory weights were also 2D matrices. We used the diagonal elements of those weight matrices as visual weights in visual (x and y) and proprioceptive (joint angles) coordinates. Figure 12 displays significant differences (t(299) < −10, p < 0.001) for all visual weights between head straight and head rolled conditions, except for θ2. Visual weights were higher for visual coordinates when the head is rolled. In contrast, visual weights decrease in proprioceptive coordinates when the head is rolled compared to the head straight condition. These results were very similar to the original model fits performed in Figure 8A. Thus, our model was able to simulate head roll dependent noise in reference frame transformations underlying reach planning and multi-sensory integration. More importantly, our data show that head roll dependent noise can influence multi-sensory integration in a way that is explained through context-dependent changes in added reference frame transformation noise.
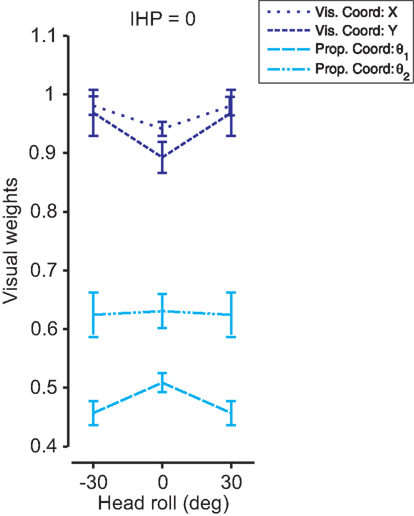
Figure 12. 2D model fit for each head roll. The model was fit to the data for each head roll condition. The visual weights for initial hand position estimates are plotted for visual (dark blue) and proprioceptive (light blue) coordinate frames with standard deviations. A 2D environment allows visual x and y and proprioceptive θ1 and θ2 to be weighted separately. There were significant differences in visual weighting between head straight and head roll conditions for all coordinate representations except proprioceptive θ2.
Discussion
In this study, we analyzed the effect of context-dependent head roll on multi-sensory integration during a reaching task. We found that head roll influenced reach error and variability in a way that could be explained by signal-dependent noise in the coordinate matching transformation between visual and proprioceptive coordinates. To demonstrate this quantitatively, we developed the first integrated model of multi-sensory integration and reference frame transformations in a probabilistic framework. This shows that the brain has online knowledge of the reliability associated with each sensory variable and uses this information to plan motor actions in a statistically optimal fashion (in the Bayesian sense).
Experimental Findings
When we changed the hand offset, we found reach errors that were similar to previously published data in multi-sensory integration tasks (Sober and Sabes, 2003, 2005; McGuire and Sabes, 2009) and were well described by Sober and Sabes’ (2003) model. In addition we also found changes in the pattern of reach errors across different head orientations. This was a new finding that previous models did not explore. There were multiple effects of head roll on reach errors. First, there was a slight rotational offset for the head straight condition, which could be a result of biomechanical biases, e.g., related to the posture of the arm. In addition, our model-based analysis showed that reach errors shifted with head roll. Our model accounted for this shift by assuming that head roll was over-estimated in the reference frame transformation during the motor planning process. The over-estimation of head roll could be explained by ocular counter-roll. Indeed, when the head is held in a stationary head roll position, ocular counter-roll compensates for a portion of the total head rotation (Collewijn et al., 1985; Haslwanter et al., 1992; Bockisch and Haslwanter, 2001). This means that the reference frame transformation has to rotate the retinal image less than the head roll angle. Not taking ocular counter-roll into account (or only partially accounting for it) would thus result in an over-rotation of retinal image, similar to what we observed in our data. However, since we did not measure ocular torsion, we cannot evaluate this hypothesis.
Alternatively, an over-estimation of head roll could in theory be related to the effect of priors in head roll estimation. If for some reason the prior belief of the head angle is that head roll is large, then Bayesian estimation would predict a posterior in head roll estimation that is biased toward larger than actual angles. However, a rationale for such a bias is unclear and would be contrary to priors expecting no head tilt such as reported in the subjective visual verticality perception literature (Dyde et al., 2006).
The second effect of head roll was a change in movement variability. Non-zero head roll angles produced reaches with higher variability compared to reaches during upright head position. This occurred despite the fact that the quality of the sensory input from the eyes and arm did not change. We took this as evidence for head roll influencing the sensory-motor reference frame transformation. Since we assume head roll to have signal-dependent noise (see below), different head roll angles will result in different amounts of noise in the transformation.
Third and most importantly, head roll changed the multi-sensory weights both at the visual and proprioceptive processing stages. This finding was validated independently by fitting Sober and Sabes’ (2003) original model and our new full Bayesian reference frame transformation model to the data. This is evidence that head roll variability changes for different head roll angles and that this signal-dependent noise enters the reference frame transformation and adds to the transformed signal, thus making it less reliable. Therefore, the context of body geometry influences multi-sensory integration through stochastic processes in the involved reference frame transformations.
Signal-dependent head roll noise could arise from multiple sources. Indeed, head orientation can be derived from vestibular signals as well as muscle spindles in the neck. The vestibular system is an essential component for determining head position sense; specifically the otolith organs (utricle and saccule) respond to static head positions in relation to gravitational axes (Fernandez et al., 1972; Sadeghi et al., 2007). We suggest that the noise from the otoliths varies for different head roll orientations; such signal-dependent noise has previously been found in the eye movement system for extraretinal eye position signals (Gellman and Fletcher, 1992; Li and Matin, 1992). In addition, muscle spindles are found to be the most important component in determining joint position sense (Goodwin et al., 1972; Scott and Loeb, 1994), with additional input from cutaneous and joint receptors (Clark and Burgess, 1975; Gandevia and McCloskey, 1976; Armstrong et al., 2008). Muscles found in the cervical section of the spine contain high densities of muscle spindles, enabling a relatively accurate representation of head position (Armstrong et al., 2008). In essence, as the head moves away from an upright position, more noise should be associated with the signal due to an increase in muscle spindle firing (Burke et al., 1976; Edin and Vallbo, 1990; Scott and Loeb, 1994; Cordo et al., 2002). However, due to the complex neck muscle arrangement, a detailed biomechanical model of the neck (Lee and Terzoloulos, 2006) would be needed to corroborate this claim.
Model Discussion
We have shown that noise affects the way reference frame transformations are performed in that transformed signals have increased variability. A similar observation has previously been made for eye movements (Li and Matin, 1992; Gellman and Fletcher, 1992) and visually guided reaching (Blohm and Crawford, 2007). This validates a previous suggestion that any transformation of signals in the brain has a cost of added noise (Sober and Sabes, 2003, 2005). Therefore, the optimal way for the brain to process information would be to minimize the number of serial computational (or transformational) stages. The latter point might be the reason why multi-sensory comparisons could occur fewer times but in parallel at different stages in the processing hierarchy and in different coordinate systems (Körding and Tenenbaum, 2007).
It has been suggested that in cases of virtual reality experiments, the visual cursor used to represent the hand could be considered as a tool attached to the hand (Körding and Tenenbaum, 2007). As a consequence, there is additional uncertainty as to the tool length. This uncertainty adds to the overall uncertainty of the visual signals. We have not modeled this separately, as tool-specific uncertainty would simply add to the actual visual uncertainty (the variances add up). However, the estimated location of the cursor tool itself could be biased toward the hand; an effect that would influence the multi-sensory integration weights but that we cannot discriminate from our data.
In our model, multi-sensory integration occurred in specific reference frames, i.e. in visual and proprioceptive coordinates. Underlying this multiple comparison hypothesis is the belief that signals can only be combined if they are represented in the same reference frame (Lacquaniti and Caminiti, 1998; Cohen and Andersen, 2002; Engel et al., 2002; Buneo and Andersen, 2006; McGuire and Sabes, 2009). However, this claim has never been explicitly verified and this may not be the way neurons in the brain actually carry out multi-sensory integration. The brain could directly combine different signals across reference frames in largely parallel neural ensembles (Denève et al., 2001; Blohm et al., 2009), for example using gain modulation mechanisms (Andersen and Mountcastle, 1983; Chang et al., 2009). Regardless of the way the brain integrates information, the behavioral output would likely look very similar. A combination of computational and electro-physiological studies would be required to distinguish these alternatives.
Our model is far from being complete. In transforming the statistical properties of the sensory signals through the different processing steps of movement planning, we only computed first-order approximations and hypothesized that all distributions remained Gaussian. This is of course a gross over-simplification; however, no statistical framework for arbitrary transformations of probability density functions exists. In addition, we only included relevant 2D motor planning computations. In the real world, this model would need to be expanded into 3D with all the added complexity (Blohm and Crawford, 2007), i.e., non-commutative rotations, offset between rotation axes, non-linear sensory mappings and 3D behavioral constraints (such as Listing’s law).
Implications
Our findings have implications for behavioral, perceptual, electrophysiological and brain imaging experiments. First, we have shown behaviorally, that body geometry signals can change the multi-sensory weightings in reach planning. Therefore, we also expect other contextual variables to have potential influences, such as gaze orientation, task/object value, or attention (Sober and Sabes, 2005). Second, we have shown contextual influences on multi-sensory integration for action planning, but the question remains whether this is a generalized principle in the brain that would also influence perception.
Finally, our findings have implications for electrophysiological and brain imaging studies. Indeed, when identifying the function of brain areas, gain-like modulations in brain activity are often taken as an indicator for reference frame transformations. However, as previously noted (Denève et al., 2001), such modulations could also theoretically perform all kinds of other different functions involving the processing of different signals, such as attention, target selection or multi-sensory integration. Since all sensory and extra-sensory signals involved in these processes can be characterized by statistical distributions, computations involving these variables will evidently look like probabilistic population codes (Ma et al., 2006) – the suggested computational neuronal substrate of multi-sensory integration. Therefore, the only way to determine if a brain area is involved in multi-sensory integration is to generate sensory conflict and analyze the brain activity resulting from this situation in conjunction with behavioral performance (Nadler et al., 2008).
Conclusions
In examining the effects of head roll on multi-sensory integration, we found that the brain incorporates contextual information about head position during a reaching task. We developed a new statistical model of reach planning combining reference frame transformations and multi-sensory integration to show that noisy reference frame transformations can alter the sensory reliability. This is evidence that the brain has online knowledge about the reliability of sensory and extra-sensory signals and includes this information into signal weighting, to ensure statistically optimal behavior.
Conflict of Interest Statement
The authors declare that the research was conducted in the absence of any commercial or financial relationships that could be construed as a potential conflict of interest.
Acknowledgments
This work was supported by NSERC (Canada), CFI (Canada), the Botterell Fund (Queen’s University, Kingston, ON, Canada) and ORF (Canada).
References
Andersen, R. A., and Mountcastle, V. B. (1983). The influence of the angle of gaze upon the excitability of the light-sensitive neurons of the posterior parietal cortex. J. Neurosci. 3, 532–548.
Armstrong, B., McNair, P., and Williams, M. (2008). Head and neck position sense. Sports Med. 38, 101–117.
Atkins, J. E., Fiser, J., and Jacobs, R. A. (2001). Experience-dependent visual cue integration based on consistencies between visual and haptic precepts. Vision Res. 41, 449–461.
Blohm, G., and Crawford, J. D. (2007). Computations for geometrically accurate visually guided reaching in 3-D space. J. Vis. 7, 1–22.
Blohm, G., Keith, G. P., and Crawford, J. D. (2009). Decoding the cortical transformations for visually guided reaching in 3D space. Cereb. Cortex 19, 1372–1393.
Bockisch, C. J., and Haslwanter, T. (2001). 3D eye position during static roll and pitch in humans. Vision Res. 41, 2127–2137.
Buneo, C. A., and Andersen, R. A. (2006). The posterior parietal cortex: sensorimotor interface for the planning and online control of visually guided movements. Neuropsychologia 44, 2594–2606.
Buneo, C. A., Jarvis, M. R., Batista, A. P., and Andersen, R. A. (2002). Direct visuomotor transformations for reaching. Nature 416, 632–636.
Burke, D., Hagbarth, K., Lofstedt, L., and Wallin, B. G. (1976). The responses of human muscle spindle endings to vibration during isometric contraction. J. Physiol. 261, 695–711.
Burr, D., Banks, M. S., and Marrone, M. C. (2009). Auditory dominance over vision in the perception of interval duration. Exp. Brain Res. 198, 49–52.
Clark, F. J., and Burgess, P. R. (1975). Slowly adapting receptors in cat knee joint: Can they signal joint angle? J. Neurophysiol. 38, 1448–1463.
Chang, S. W. C., Papadimitriou, C., and Snyder, L. H. (2009). Using a compound gain field to compute a reach plan. Neuron 64, 744–755.
Cohen, Y. E., and Andersen, R. A. (2002). A common reference frame for movement plans in the posterior parietal cortex. Nat. Rev. Neurosci. 3, 553–562.
Cordo, P. J., Flores-Vieira, C., Verschueren, S. M. P., Inglis, J. T., and Gurfinke. (2002). Positions sensitivity of human muscle spindles: Single afferent and population representations. J. Neurophysiol. 87, 1186–1195.
Collewijn, H., Van der Steen, J., Ferman, L., and Jansen, T. C. (1985). Human ocular counterroll: Assessment of static and dynamic properties from electromagnetic scleral coil recordings. Exp. Brain Res. 59, 185–196.
Denève, S., Latham, P.E., and Pouget, A. (2001). Efficient computation and cue integration with noisy population codes. Nat. Neurosci. 4, 826–831.
Dyde, R. T., Jenkin, M. R., and Harris, L. R. (2006). The subjective visual vertical and the perceptual upright. Exp. Brain Res. 173, 612–622.
Edin, B. B., and Vallbo, A. B. (1990). Muscle afferent responses to isometric contractions and relaxations in humans. J. Neurophysiol. 63, 1307–1313.
Engel, K. C., Flanders, M., and Soechting, J. F. (2002). Oculocentric frames of reference for limb movement. Arch. Ital. Biol. 140, 211–219.
Ernst, M. O., and Banks, M. S. (2002). Humans integrate visual and haptic information in a statistically optimal fashion. Nature 415, 429–433.
Ernst, M. O., and Bulthoff, H. H. (2004). Merging the senses into a robust percept. Trends Cogn. Sci. 8, 162–169.
Faisal, A. A., Selen, L. P., and Wolpert, D. M. (2008). Noise in the nervous system. Nat. Rev. Neurosci. 9, 292–303.
Fernandez, C., Goldberg, J. M., and Abend, W. K. (1972). Response to static tilts of peripheral neurons innervating otolith organs of the squirrel monkey. J. Neurophysiol. 35, 978–987.
Gandevia, S. C., and McCloskey, D. I. (1976). Joint sense, muscle sense and their combination as position sense, measured at the distal interphalangeal joint of the middle finger. J. Physiol. 260, 387–407.
Gellman, R. S., and Fletcher, W. A. (1992). Eye position signals in human saccadic processing. Exp. Brain Res. 89, 425–434.
Ghahramani, Z., Wolpert, D. M., and Jordan, M. I. (1997). “Computational models of sensorimotor integration,” in Self-organization, Computational Maps and Motor Control, eds P. G. Morasso and V. Sanguineti (Amsterdam: North Holland), 117–147.
Goodwin, G. M., McCloskey, D. I., and Mathews, P. B. C. (1972). The contribution of muscle afferents to kinesthesis shown by vibration induced illusions of movement and by the effect of paralysing joint afferents. Brain 95, 705–748.
Green, A. M., and Angelaki, D. E. (2010). Multisensory integration: resolving sensory ambiguities to build novel representations. Curr. Opin. Neurobiol. 20, 353–360.
Hagura, N., Takei, T., Hirose, S., Aramaki, Y., Matsumura, M., Sadata, N., and Naito, E. (2007). Activity in the posterior parietal cortex mediates visual dominance over kinesthesia. J. Neurosci. 27, 7047–7053.
Haslwanter, T., Straumann, D., Hess, B. J., and Henn, V. (1992). Static roll and pitch in the monkey: shift and rotation of listing’s plane. Vision Res. 32, 1341–1348.
Jordan, M. I., Flahs, T., and Arnon, Y. (1994). A model of the learning of arm trajectories from spatial deviations. J. Cogn. Neurosci. 6, 359–376.
Jordan, M. I., and Rumelhart, D. E. (1992). Forward models: Supervised learning with a distal teacher. Cogn. Sci. 16, 307–354.
Kersten, D., Mamassian, P., and Yuille, A. (2004). Object perception as Bayesian inference. Annu. Rev. Psychol. 55, 271–304.
Knill, D. C., and Pouget, A. (2004). The Bayesian brain: the role of uncertainty in neural coding and computation. Trends Neurosci. 27, 712–719.
Körding, K. P., and Tenenbaum, J. B. (2007). “Casual inference in sensorimotor integration. NIPS 2006 conference proceedings,” in Advances in Neural Information Processing Systems, Vol. 1, eds. B. Schölkopf, J. Platt, and T. Hoffman (Cambridge, MA: MIT Press), 641–647.
Lacquaniti, F., and Caminiti, R. (1998). Visuo-motor transformations for arm reaching. Eur. J. Neurosci. 10, 195–203.
Landy, M. S., and Kojima, H. (2001). Ideal cue combination for localizing texture-defined edges. J. Opt. Soc. Am. A. 18, 2307–2320.
Landy, M. S., Maloney, L., Johnston, E. B., and Young, M. (1995). Measurement and modeling of depth cue combination: in defense of weak fusion. Vision Res. 35, 389–412.
Lechner-Steinleitner, S. (1978). Interaction of labyrinthine and somatoreceptor inputs as determinants of the subjective vertical. Psychol. Res. 40, 65–76.
Lee, S., and Terzoloulos, D. (2006). Heads up!: Biomechanical modeling and neuromuscular control of the neck. ACM Trans. Graph. 25, 1188–1198.
Li, W., and Matin, L. (1992). Visual direction is corrected by a hybrid extraretinal eye position signal. Ann. N.Y. Acad. Sci. 656, 865–867.
Ma, W. J., Beck, J. M., Latham, P. E., and Pouget, A. (2006). Bayesian inference with probabilistic population codes. Nat. Neurosci. 9, 1432–1438.
McIntyre, J., Stratta, F., and Lacquaniti, F. (1997). Viewer-centered frame of reference for pointing to memorized targets in three-dimensional space. J. Neurophysiol. 78, 1601–1618.
McGuire, L. M., and Sabes, P. N. (2009). Sensory transformations and the use of multiple reference frames for reach planning. Nat. Neurosci. 12, 1056–1061.
Mon-Williams, M., Wann, J. P., Jenkinson, M., and Rushton, K. (1997). Synaesthesia in the normal limb. Proc. Biol. Sci. 264, 1007–1010.
Nadler, J. W., Angelaki, D. E., and DeAngelis, G. C. (2008). A neural representation of depth from motion parallax in macaque visual cortex. Nature 452, 642–645.
Ren, L., Blohm, G., and Crawford, J. D. (2007). Comparing limb proprioception and oculomotor signals during hand-guided saccades. Exp. Brain Res. 182, 189–198.
Ren, L., Khan, A. Z., Blohm, G., Henriques, D. Y. P., Sergio, L. E., and Crawford, J. D. (2006). Proprioceptive guidance of saccades in eye-hand coordination. J. Neurophysi. 96, 1464–1477.
Rossetti, Y., Desmurget, M., and Prablanc, C. (1995). Vectorial coding of movement: vision, proprioception, or both? J. Neurophysiol. 74, 457–463.
Sadeghi, S. G., Chacron, M. J., Taylor, M. C., and Cullen, K. E. (2007). Neural variability, detection thresholds, and information transmission in the vestibular system. J. Neurosci. 27, 771–781.
Scott, S. H., and Loeb, G. E. (1994). The computation of position-sense from spindles in mono- and multi-articular muscles. J. Neurosci. 14, 7529–7540.
Sober, S. J., and Sabes, P. N. (2003). Multisensory integration during motor planning. J. Neurosci. 23, 6982–6992.
Sober, S. J., and Sabes, P. N. (2005). Flexible strategies for sensory integration during motor planning. Nat. Neurosci. 8, 490–497.
Stein, B. E., and Stanford, T. R. (2008). Multisensory integration: current issues from the perspective of the single neuron. Nat. Rev. Neurosci. 9, 255–266.
Tarnutzer, A. A., Bockisch, C., Straumann, D., and Olasagasti, I. (2009). Gravity dependence of subjective visual vertical variability. J. Neurophysiol. 102, 1657–1671.
Van Beers, R. J., Sittig, A. C., and Denier Van Der Gon, J. J. (1999). Integration of proprioception and visual position-information: an experimentally supported model. J. Neurophysiol. 81, 1355–1364.
Van Beers, R. J., Wolpert, D. M., and Haggard, P. (2002). When feeling is more important than seeing in sensorimotor adaptation. Curr. Biol. 12, 824–837.
Van Beuzekom, A. D., and Van Gisbergen, J. A. M. (2000). Properties of the internal representation of gravity inferred from spatial-direction and body-tilt estimates. J. Neurophysiol. 84, 11–27.
Keywords: reaching, Bayesian integration, multi-sensory, proprioception, vision, context, head roll, reference frames
Citation: Blohm G and Burns JK (2010) Multi-sensory weights depend on contextual noise in reference frame transformations. Front. Hum. Neurosci. 4:221. doi: 10.3389/fnhum.2010.00221
Received: 19 August 2010;
Accepted: 04 November 2010;
Published online: 07 December 2010.
Edited by:
Francisco Barcelo, University of Illes Balears, SpainCopyright: © 2010 Blohm and Burns. This is an open-access article subject to an exclusive license agreement between the authors and the Frontiers Research Foundation, which permits unrestricted use, distribution, and reproduction in any medium, provided the original authors and source are credited.
*Correspondence: Gunnar Blohm, Centre for Neuroscience Studies, Queen’s University, Kingston, ON, Canada K7L 3N6. e-mail: gunnar.blohm@queensu.ca