- 1 Department of Psychology, Faculty of Arts and Social Sciences, National University of Singapore, Singapore
- 2 Department of Psychology, University of Calgary, Calgary, AB, Canada
There is considerable evidence (e.g., Pexman et al., 2008) that semantically rich words, which are associated with relatively more semantic information, are recognized faster across different lexical processing tasks. The present study extends this earlier work by providing the most comprehensive evaluation to date of semantic richness effects on visual word recognition performance. Specifically, using mixed effects analyses to control for the influence of correlated lexical variables, we considered the impact of number of features, number of senses, semantic neighborhood density, imageability, and body–object interaction across five visual word recognition tasks: standard lexical decision, go/no-go lexical decision, speeded pronunciation, progressive demasking, and semantic classification. Semantic richness effects could be reliably detected in all tasks of lexical processing, indicating that semantic representations, particularly their imaginal and featural aspects, play a fundamental role in visual word recognition. However, there was also evidence that the strength of certain richness effects could be flexibly and adaptively modulated by task demands, consistent with an intriguing interplay between task-specific mechanisms and differentiated semantic processing.
Although the ultimate goal of reading is to extract meaning from visually printed words, the effect of meaning-level influences on lexical processing is surprisingly poorly understood (see Pexman, in press, for a recent review; see also Balota et al., 1991). For the most part, the empirical literature has focused on how sublexical (see Carreiras and Grainger, 2004) and lexical (see Balota et al., 2006) representations influence visual word recognition. Likewise, despite their complexity and theoretical sophistication, influential computational models of visual word recognition (e.g., Perry et al., 2007) are silent on the role of semantic information (but see Harm and Seidenberg, 2004). Indeed, semantic effects are difficult to reconcile with classic logogen-based models of word recognition, which implicitly assume that lexical processing latencies tap a magic moment (Balota, 1990), i.e., a discrete moment in time when the lexical entry for a word has been identified but meaning-level information has not yet been accessed.
A number of studies (e.g., Buchanan et al., 2001; Cortese and Fugett, 2004; Duñabeitia et al., 2008; Pexman et al., 2008; Siakaluk et al., 2008; Yap et al., 2011) suggests that a word associated with relatively more semantic information can be considered to be semantically richer, and word recognition is generally facilitated for such words. A number of dimensions have been identified that appear to tap a word’s semantic richness, including: (1) the number of features (NF) associated with its referent, (2) its semantic neighborhood density (SND) in high-dimensional semantic space, (3) the number of distinct first associates (NoA) elicited by the word in a free-association task (Nelson et al., 1998), (4) imageability, the extent to which a word evokes mental imagery of things or events (imageability), (5) number of senses (NS), the number of meanings associated with a word, and (6) body–object interaction (BOI), the extent to which a human body can physically interact with the word’s referent. Specifically, word recognition is typically faster for words when their referents are associated with many semantic features (Pexman et al., 2003, 2008), when they are located in dense semantic neighborhoods (Buchanan et al., 2001; Shaoul and Westbury, 2010), when they elicit many associates (Duñabeitia et al., 2008), when they evoke more imagery (Cortese and Fugett, 2004), when they possess multiple meanings (Yap et al., 2011), and when they evoke more sensorimotor information (Siakaluk et al., 2008). That each of these variables affects word recognition behavior suggests that each taps an important aspect of semantic representation.
These findings can be accommodated by the embellished interactive activation framework suggested by Balota (1990; see also Balota et al., 1991), where there is bidirectional cascaded processing between letter-level, lexical-level, and semantic-level representations. Importantly, one could assume that there is stronger top-down feedback from semantic-level to orthographic-level representations for semantically rich words, facilitating lexical access for such words. The interactive activation framework is based on the assumption that lexical processing is subserved by mental lexicons containing localist units that represent the spelling, sound, and meaning of a word (see Coltheart et al., 2001), and that orthographic and semantic processing are handled by separate systems.
An important theoretical alternative to localist models is represented by parallel distributed processing (PDP) models, which do not assume the existence of mental lexicons. Instead, orthographic, phonological, and semantic information are respectively represented by distributed patterns of activity over separate layers of orthographic, phonological, and semantic neuron-like processing units (Plaut et al., 1996). In the classic connectionist triangle model of lexical processing (e.g., Seidenberg and McClelland, 1989), when a word is presented, activation flows from the orthographic to the semantic layer (either directly or mediated by the phonological layer) via weighted connections that reflect the network’s knowledge of the mappings between orthography and semantics. Semantically rich words, which possess more stable and more readily computable meanings (Strain et al., 1995), are represented by the activation of more semantic units, hence yielding greater feedback activation from the semantic to the orthographic layer; this facilitates word recognition (see Hino et al., 2002, for more discussion of how PDP models can handle semantic richness effects).
Importantly, within the PDP approach, there is no sharp delineation between lexical and semantic processing. Instead, word recognition is assumed to rely on a common cognitive system where lexical and semantic knowledge interdependently and concurrently influence word recognition (Dilkina et al., 2008, 2010). Indeed, this view meshes well with the literature on semantic dementia, a variant of frontotemporal lobe dementia marked by deficits in conceptual and lexical knowledge (see Hodges et al., 1998, for a review). Although the finer details of this literature are beyond the scope of the present report, there is broad support for a positive correlation between lexical and conceptual deficits in semantic dementia patients, which is consistent with a single system that mediates both lexical and semantic processing (see Dilkina et al., 2010, for a connectionist model of lexical/semantic processing that explains the patient data).
Semantic Richness Effects are Multidimensional and Task-Dependent
Importantly, although all the measures described ostensibly reflect aspects of a word’s semantic richness, it is clear that they do not tap a common undifferentiated construct. For example, NF and NS seem to primarily reflect the complexity of a word’s semantic representation, while SND and NoA may tap the extent to which that representation is interconnected with those of other words. Likewise, while imageability effects (Strain et al., 1995; Cortese et al., 1997; Cortese and Fugett, 2004) could be mediated by the interactions between lexical and visual (Paivio, 1991) or contextual (Schwanenflugel, 1991) information, BOI effects appear to implicate embodied sensorimotor representations (Siakaluk et al., 2008; Tillotson et al., 2008; Bennett et al., 2011; Wellsby et al., 2011). Consistent with this, the bivariate correlations between the different semantic richness variables are relatively modest, and the measures are also able to account for unique variance in word recognition performance (Pexman et al., 2008).
More relevantly for the purposes of the present study, the effects of semantic richness dimensions are flexibly and adaptively modulated by the specific demands of different lexical processing tasks (see Balota and Yap, 2006). For example, semantic richness variables are better predictors of semantic classification, compared to lexical decision, performance. In semantic classification, participants are required to discriminate between words from different semantic categories (e.g., is CABBAGE concrete or abstract?), while in lexical decision, they have to discriminate between real words and made-up words (e.g., FLIRP). Semantic processing is implicated to a greater extent in semantic classification because participants have to resolve the specific meaning of a word in order to make a correct response, whereas they can rely heavily on familiarity-based information to drive a lexical decision response (Balota and Chumbley, 1984).
Similarly, semantic effects are typically stronger in lexical decision than in speeded pronunciation performance. For example, early work by Chumbley and Balota (1984) reported that semantic variables such as instance dominance (the likelihood that a word will be given as an example to a category in response to the category name), number of associates, and NS produced reliable effects in lexical decision, but not in speeded pronunciation. More recent studies employing larger sets of stimuli (e.g., Balota et al., 2004) indicate that semantic effects (e.g., imageability effects) can be reliably detected in the pronunciation task, although they are greatly attenuated (see also Yap and Balota, 2009). These findings suggest that semantic information plays a stronger role in lexical decision, compared to pronunciation, because semantic information can be recruited to drive the familiarity-based word/non-word discrimination process that is specific to lexical decision (Balota and Chumbley, 1984; Chumbley and Balota, 1984).
The multidimensionality and task-specificity of semantic richness effects are also evident at a more fine-grained level. For example, although high-NF words are recognized faster in both lexical decision and semantic classification (Pexman et al., 2008), a denser semantic neighborhood is associated with faster lexical decision performance, but has no effect on semantic classification performance (Yap et al., 2011). While lexical decision is facilitated by stronger semantics → orthography feedback for words from dense neighborhoods, the opposing effects of nearby (facilitatory) and distant (inhibitory) neighbors may cancel each other out (Mirman and Magnuson, 2006) in tasks which emphasize semantic processing (e.g., semantic classification).
Intriguingly, the effect of semantic ambiguity is also very different in lexical decision and semantic classification. Specifically, although there is an ambiguity advantage (i.e., better performance for words with many senses) in lexical decision (e.g., Borowsky and Masson, 1996; Hargreaves et al., 2011), there is either a null ambiguity effect or an ambiguity disadvantage in semantic classification (Piercey and Joordens, 2000; Hino et al., 2002, 2006; Pexman et al., 2004; Hargreaves et al., 2011; Yap et al., 2011). Similar to neighborhood density, multiple meanings produce greater semantic feedback, which is helpful for lexical decision. However, a task which requires more focus on semantic processing can be slowed down by ambiguity, due to one-to-many mappings between orthography and semantics for ambiguous words (Borowsky and Masson, 1996), by increased competition between the different activated meanings (Grainger et al., 2001), or by competition between the activated meanings and the required response (Pexman et al., 2004).
The foregoing findings underscore the importance of examining semantic richness effects across a constellation of lexical processing paradigms, as effects are not process-pure but instead reflect both task-specific and task-general processing (Balota and Chumbley, 1984; Grainger and Jacobs, 1996). In line with this principle, Pexman et al. (2008) used hierarchical multiple regression to explore the effects of various semantic richness dimensions on lexical decision and semantic classification performance, basing their analyses on a common set of 514 concrete words from the McRae et al. (2005) norms. Yap et al. (2011) extended this work by also considering richness effects on speeded pronunciation (i.e., read words aloud) performance. Collectively, these studies yielded a number of the intriguing task-specific findings discussed earlier. In the same vein, Duñabeitia et al. (2008) studied the effect of NoA across different visual word recognition tasks, including speeded pronunciation, lexical decision, eye movements during sentence reading (see Rayner, 1998, for a review), and progressive demasking (Dufau et al., 2008). Eye movement data provide on-line, moment-to-moment measures of cognitive processes implicated in reading, while progressive demasking is a relatively novel perceptual identification task where a word gradually emerges from a mask over time, and the time taken to identify the specific word being presented is measured.
The Present Research
The primary objective of the present study is to provide the most comprehensive evaluation to date of the impact of extant semantic richness dimensions (NF, SND, imageability, NS, BOI) across different visual word recognition tasks. In addition to the ubiquitous traditional tasks (i.e., lexical decision, speeded pronunciation, semantic classification) examined by Pexman et al. (2008) and Yap et al. (2011), we also include newer tasks such as the progressive demasking task (PDT; Dufau et al., 2008) and go/no-go lexical decision (Gordon, 1983; Perea et al., 2002). In the progressive masking task, a word stimulus (e.g., DOG) is rapidly alternated with a mask (e.g., ###), and through successive display changes, the word gradually emerges from the mask. Participants make a button press as soon as they can identify the stimulus, hence yielding response time (RT) measures for a perceptual identification paradigm based on the presentation of visually degraded stimuli. Since it is a perceptual identification task, one might argue that unique stimulus identification is mandatory in progressive demasking; hence, progressive demasking latencies might provide a purer measure of lexical processing (Carreiras et al., 1997). Methodologically speaking, progressive demasking has important advantages over lexical decision and speeded pronunciation, as the experimenter does not need to create non-word distracters and performance is unaffected by articulatory factors (Ferrand et al., 2011). Finally, because of the way it is set up, progressive demasking slows down and stretches out the recognition process, potentially making this task more sensitive to underlying perceptual (Dufau et al., 2008) and semantic (Ferrand et al., 2011) processing.
The go/no-go lexical decision task1 is an interesting variation on the standard task in which participants respond by pressing a button when a word is presented but withhold their response when a non-word is presented. The go/no-go task possesses a number of advantages. According to Perea et al. (2002), in addition to yielding faster, more accurate, and less noisy performance, go/no-go lexical decision also reduces task-specific processing demands (e.g., response competition during the decision process). In order to rigorously rule out the influence of correlated variables, which may spuriously inflate the predictive power of semantic richness variables (see Gernsbacher, 1984), linear mixed effects analyses (Baayen et al., 2008) will be used in the present study to control for phonological onsets (Balota et al., 2004) and established lexical variables (Balota et al., 2004; Yap and Balota, 2009); linear mixed models also allow us to generalize across both participants and items using a single model. All five datasets (LDT, go/no-go LDT, pronunciation Task, PDT, and SCT) are new, collected for the purposes of the present paper, and details of those datasets will be provided in the Methods section.
Method
Participants
Participants in all five tasks were undergraduate students at the University of Calgary who received course credit for participating. All participants reported that English was their first language and that they had normal or corrected-to-normal vision. There were 38 participants in the SCT, 31 participants in the LDT, and 30 in each of the other tasks.
Materials
The word stimuli for this study were 514 concrete words2 from McRae et al.’s (2005) norms. The stimuli also included 514 non-words used in the LDT and go/no-go LDT (which were matched to the words for length), and 514 abstract words which served as fillers in the SCT. The variables in the analyses were divided into three clusters: surface, lexical, and semantic variables (see Table 1 for descriptive statistics of predictors and measures for items included in the analyses).
Surface variables
Dichotomous variables were used to code the initial phoneme of each word (1 = presence of feature; 0 = absence of feature) on 13 features: affricative, alveolar, bilabial, dental, fricative, glottal, labiodental, liquid, nasal, palatal, stop, velar, and voiced (Balota et al., 2004). These control for the variance associated with voice key biases in speeded pronunciation.
Lexical variables
These included log frequency (Brysbaert and New, 2009), number of morphemes, and number of letters. In order to address the high correlations between orthographic (Coltheart et al., 1977) and phonological (Yates, 2005) neighborhood size (r = 0.79), and between orthographic (Yarkoni et al., 2008) and phonological (Yap and Balota, 2009) Levenshtein distance (LD; r = 0.92), we used principal component analysis to reduce the two neighborhood size measures and the two LD measures to a neighborhood size (N) and LD component respectively (see Yap et al., 2011).
Semantic variables
Imageability ratings were obtained for 473 of the words from the MRC norms (Coltheart, 1981) and from the norms collected by Cortese and colleagues(Cortese and Fugett, 2004; Schock et al., in press) and Bennett et al. (2011). BOI ratings for 459 of the words were obtained from the Bennett et al. (2011) norms and BOI ratings for the remaining 55 words were collected at the University of Calgary from another separate group of 38 undergraduate students. NF values were taken from the McRae norms, and NS values were log-transformed and were from Miller (1990). Finally, SND values were based on ARC (average radius of co-occurrence) values from Shaoul and Westbury (2010); words whose closest neighbors are more similar to them have higher ARC values.
Procedure
In all tasks, testing began with a short series of practice trials with verbal feedback provided by the experimenter. Each of the tasks followed the same general procedure: on each trial, a word was presented in the center of a 20″ monitor controlled by a desktop computer. The LDT, go/no-go LDT, pronunciation task, and SCT were all conducted using E-Prime software (Schneider et al., 2002), while the PDT was run using Windows executable software for the PDT (Dufau et al., 2008).
On each trial in the LDT, participants classified each item as a word or non-word by pressing the far right or far left button on a response box, respectively. On each trial in the go/no-go LDT, participants pressed the far right button to classify an item as a word, and made no response to non-words. On each trial in the pronunciation task, participants read the word aloud into a microphone connected to the response box. Participants’ pronunciation responses were recorded with a digital recorder and later coded for accuracy. In the PDT, each item (e.g., TABLE) was presented alternating with a mask (#####), with each trial consisting of a series of repeated cycles. Initially the mask was presented on the screen for 195 ms and the stimulus for 15 ms, and over subsequent cycles the mask presentation time decreased while the stimulus presentation time increased (e.g., in the next cycle the mask would be presented for 180 ms and the stimulus for 30 ms). Participants were instructed to press the space bar on the keyboard as soon as they could determine what the word was. (If no response was made after 2700 ms, the mask disappeared and the stimulus remained on the screen until the participant pressed the space bar.) Participants then typed the word and pressed the enter key to advance to the next trial. Finally, in the SCT, participants classified each word as concrete or abstract by pressing the far right or far left button, respectively.
Results
We excluded trials for which the response was incorrect (7.52% in the LDT, 2.60% in the go/no-go LDT, 3.48% in the pronunciation task, 5.05% in the PDT, and 5.18% in the SCT), as well as responses that were faster than 200 ms or slower than 3000 ms (<1% of trials in all tasks). We also excluded trials for which the RT exceeded 2.5 SD from each participant’s mean (2.74, 2.94, 2.55, 2.00, and 2.91% respectively).
There were 473 items for which we had values on each of the lexical and semantic variables examined. For these items, intercorrelations between predictors and dependent measures are presented in Table 2. As illustrated in Table 2, although there were several significant correlations between the richness variables, most of the correlations were relatively modest (rs between 0.05 and 0.28), with the exception of the relationship between NS and SND (r = 0.49). However, it should be noted that word frequency is relatively highly correlated with both NS (r = 0.51) and SND (r = 0.77), suggesting that frequency is driving the NS–SND correlation. Indeed, when one partials out the effect of frequency, the correlation becomes 0.17. Generally, the modest correlations between richness measures suggest that these dimensions do not all tap the same underlying construct, and that semantic richness is multidimensional.
All data were analyzed using R (R Development Core Team, 2011). Linear mixed effects models were fitted to the RT data3 from each task, using the lme4 package (Bates et al., 2012); p-values for fixed effects were computed using the languageR package (Baayen, 2012). The influence of surface, lexical, and semantic richness variables were treated as fixed effects, while participants and items were treated as random variables. The results of these analyses are presented in Table 3 and Figure 1. Clearly, even when lexical variables were controlled for, semantic richness effects were observed in all tasks. At the same time, the pattern of significant richness effects varied across tasks. Imageability and NF were significant predictors in all tasks (although the NF effect was marginal in the PDT). SND was a significant predictor in the standard and go/no-go LDT, while BOI was a significant predictor in all tasks except the PDT. Finally, with the exception of the go/no-go LDT, NS did not significantly predict performance on tasks. Notably, in all cases where significant relationships were observed between latencies and semantic richness measures, the direction of the relationships was the same: relatively greater richness (whether in terms of more senses, or a more highly imageable referent, or more bodily experience, or a denser neighborhood, or more features) was associated with relatively faster latencies.
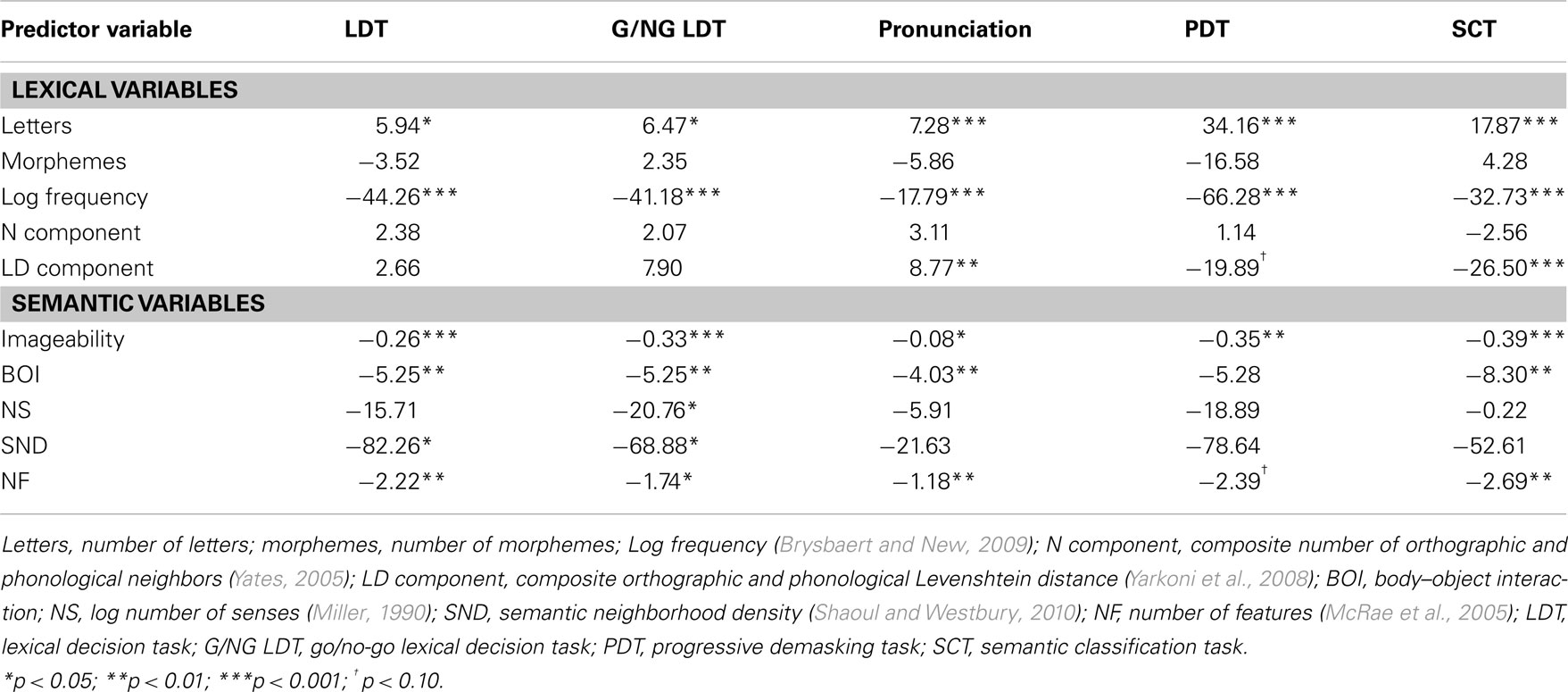
Table 3. Estimates for lexical and semantic fixed effects parameters, along with p-values based on the t-statistic (n = 473).
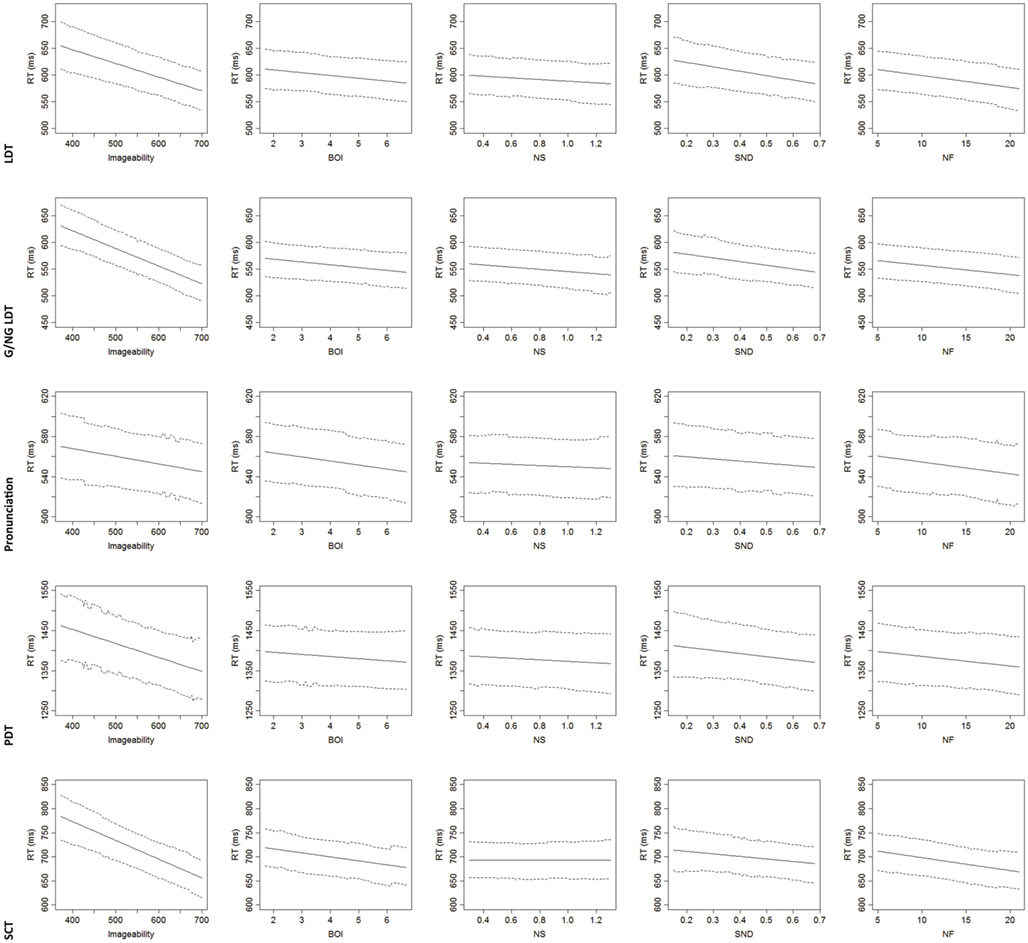
Figure 1. Partial effects plots of semantic richness effects, adjusted for the median value of the other numerical predictors in the model, as a function of task. 95% highest posterior density intervals are provided. Note. BOI, body–object interaction; Senses, log number of senses (Miller, 1990); SND, semantic neighborhood density (Shaoul and Westbury, 2010); Features, number of features (McRae et al., 2005); LDT, lexical decision task; G/NG LDT, go/no-go lexical decision task; PDT, progressive demasking task; SCT, semantic classification task.
Discussion
The present study represents the most comprehensive large-scale study of semantic richness effects to date. Using the McRae et al. (2005) concrete words, we investigated the influence of five theoretically influential semantic richness dimensions4 (imageability, BOI, NS, SND, NF) on lexical processing, using five different word recognition tasks (LDT, go/no-go LDT, speeded pronunciation, PDT, SCT), extending earlier studies (e.g., Duñabeitia et al., 2008; Pexman et al., 2008; Yap et al., 2011) which have considered fewer variables across fewer tasks. It is noteworthy that semantic richness effects could be reliably detected in all tasks of lexical processing, even in speeded pronunciation, where meaning does not need to be computed and there is no emphasis on familiarity-based information (see Balota and Chumbley, 1984). More importantly, the present analyses yield a particularly stringent and conservative test of richness effects, given the large number of lexical variables controlled for and the fact that the unique predictive power of each richness dimension was assessed while holding the other four dimensions constant. However, as a counterpoint to the task-generality of semantic richness effects, there was also evidence of clear and systematic task-specificity. For example, SND effects were reliable only in the standard and go/no-go LDT, while BOI effects were absent in the PDT. We will now consider the implications of these findings in greater detail.
Semantic Richness Effects are Task-General
In line with previous investigations, the present study provides further evidence that semantic richness effects generalize across disparate word recognition tasks, broadly consistent with the idea that feedback activation from semantics to orthography and phonology is a pervasive aspect of lexical processing (Hino and Lupker, 1996; Pexman and Lupker, 1999; Pexman et al., 2001; Siakaluk et al., 2008). In addition to examining established word recognition measures (i.e., lexical decision, pronunciation, semantic classification), we are the first to assess the influence of multiple richness dimensions on newer paradigms such as the go/no-go LDT and PDT. Researchers have suggested that the latter tasks may help magnify the size of effects of interest by slowing down the recognition process (PDT; Dufau et al., 2008) or by minimizing the role of task-specific decision processes (go/no-go LDT; Perea et al., 2002). Compared to the standard LDT (see Table 1), it is clear that participants were indeed much slower on the PDT, and also faster and more accurate on go/no-go LDT. However, there was no evidence that these two tasks were particularly sensitive to semantic richness effects (cf. Ferrand et al., 2011), compared to the standard LDT. In general, our PDT results are compatible with findings from a recent megastudy that compared performance on lexical decision, pronunciation, and progressive demasking for the same set of 1,482 monosyllabic monomorphemic French words (Ferrand et al., 2011). In that study, Ferrand et al. reported that progressive demasking performance was primarily influenced by perceptual/visual factors such as number of letters, and that the PDT did not provide substantive additional insights beyond those provided by the LDT (but see Carreiras et al., 1997, who reported opposite effects of neighborhood density, a measure of orthographic richness, in the two tasks).
Although we have claimed that semantic richness effects generalize across tasks, we need to carefully qualify this by acknowledging that not all effects are reliable in all tasks. Specifically, when a large number of lexical and semantic factors were controlled for, the only two richness variables that produced reliable (or borderline reliable) effects on every task were imageability and NF; word recognition was faster for highly imageable words and words for which referents had more features. This suggests that feedback from semantics to orthography during lexical processing is most consistently and robustly mediated by the imaginal and featural aspects of semantic representations. Such a finding is also consistent with frameworks that model semantics via a distributed attractor network (e.g., Plaut and Shallice, 1993), which yields faster settling times for the semantic representations of high-imageability or high-NF words, since these are associated with the activation of more semantic feature units.
It is also noteworthy that BOI effects (Siakaluk et al., 2008) were significant in every task except the PDT; this task-generality is consistent with recent work by Bennett et al. (2011). The pervasiveness of BOI effects strongly supports the idea that the relative availability of sensorimotor information associated with a word contributes to lexical-semantic processing (see also Juhasz et al., 2011), possibly through the recruitment of modality-specific systems (see Hargreaves et al., 2012, for more discussion). Compared to imageability, NF, and BOI, the other richness measures showed more evidence of task-specificity. For example, SND produced reliable effects in the standard and go/no-go LDT, but not in the other three tasks. Likewise, NS did not predict variance on any task except the go/no-go LDT. We will discuss these intriguing between-task dissociations in greater detail in the next section.
Semantic Richness Effects are Task-Specific
One of the most intriguing aspects of the semantic richness literature is how the strength and even direction of certain semantic richness effects can be systematically and adaptively modulated by the specific demands of a given lexical processing task (see Balota and Yap, 2006). For example, as already observed by Pexman et al. (2008) and Yap et al. (2011), semantic variables collectively account for relatively much more variance in the SCT, compared to other tasks where semantic processing is not the primary basis for a response. At a more fine-grained level, the influence of SND, which provides support for models which structure semantics via lexical co-occurrence (e.g., Shaoul and Westbury, 2010), was evident in the two LDTs, but not in the other three tasks. This replicates the pattern reported by both Pexman et al. (2008) and Yap et al. (2011), and suggests that neighborhood density effects are more reliable in tasks where familiarity-based information is emphasized. Moreover, when a task (i.e., SCT) requires participants to compute the specific meaning of presented words, there might be a trade-off between the facilitatory effects of close neighbors and inhibitory effects of distant neighbors (Mirman and Magnuson, 2006), resulting in null neighborhood effects.
Turning to NS, we were surprised to note that this variable predicted unique variance only in the go/no-go LDT (see Table 3), given that previous studies (e.g., Borowsky and Masson, 1996; Hargreaves et al., 2011) reported an ambiguity advantage using the standard LDT. When we examined the zero-order correlations between NS and RT, the relationships were clearly negative (i.e., facilitatory) across all tasks. However, in the mixed effects analyses, when lexical and semantic predictors were more stringently controlled, NS reliably predicted additional unique word recognition variance only in the go/no-go LDT, possibly because performance on that task is inherently less noisy and contaminated by task-specific processing demands. We should clarify that ambiguity was operationally defined in the present study using WordNet (Miller, 1990) NS. However, in this metric, the multiple senses of a word may or may not be related to one another.
Rodd et al. (2002) have argued that it is important to distinguish between words with multiple related senses (i.e., polysemes, e.g., TWIST) and words with multiple unrelated meanings (i.e., homonyms, e.g., BARK). Interestingly, Rodd et al. reported that in lexical decision, there is an ambiguity advantage for polysemes (related senses) but an ambiguity disadvantage for homonyms (unrelated senses; see also Klepousniotou and Baum, 2007). This is consistent with the idea that the semantic richness of a representation is reinforced by multiple related senses but is undermined by multiple unrelated senses through lexical competition (see Rodd et al., 2002, for more discussion). Because our measure of ambiguity does not distinguish between related and unrelated senses, it is possible that there is a trade-off between the facilitatory effects of related senses and the inhibitory effects of unrelated senses. Of course, it is also possible that this particular metric of NS (counting the number of dictionary senses), despite its objectivity, is a relatively coarse proxy for variability in a word’s core meaning (Hoffman et al., 2011).
Hoffman et al. have developed an intriguing new ambiguity measure, semantic diversity (SD), using lexical co-occurrence data. Although a full description of their approach is beyond the scope of the present report, they essentially considered all the contexts a word could appear in and computed the similarity between these contexts. A word that can appear in very diverse linguistic contexts (e.g., PART) is considered to be high-SD (i.e., high in ambiguity), while a word that can only occur in a restricted range of contexts (e.g., GASTRIC) is considered low-SD (i.e., low in ambiguity). Interestingly, although the correlation between NS and SD is positive and moderate in strength (r = 0.41), words judged explicitly to have few senses nevertheless varied greatly on their SD values (Hoffman et al., 2011), suggesting that this is a more sensitive measure of ambiguity that could be exploited by researchers in future work.
An important limitation of the present work is the item set used. Several of the semantic richness measures are available for only a limited set of items; these tend to be words (nouns) that refer to highly concrete referents. As such, although we examined a large set of these items our results may not necessarily generalize to other item sets. Future research should examine semantic richness effects in other word sets and should extend the study of semantic richness to other word types, such as verbs, in order to gain additional insights about lexical-semantic representation. Future work can also be directed toward exploring non-linear effects of semantic richness dimensions, and possible interactions between these variables.
Conclusion
The present study examined the influence of multiple semantic richness dimensions across various tasks of lexical processing. The fact that semantic richness effects could be reliably detected on all tasks attests to their robustness and generality. At the same time, there was ample evidence supporting the multidimensional nature of semantic richness and demonstrating that these dimensions are selectively modulated by task demands. In order to be more fully specified, emerging theories of semantic representation need to take into account this complex interplay between lexical-semantic processes and task-specific mechanisms.
Conflict of Interest Statement
The authors declare that the research was conducted in the absence of any commercial or financial relationships that could be construed as a potential conflict of interest.
Acknowledgments
This work was supported in part by a Natural Sciences and Engineering Research Council (NSERC) of Canada Discovery Grant to Penny M. Pexman. We thank Dave Balota, Mike Cortese, Jon Duñabeitia, and Paul Siakaluk for their very useful comments during the preparation of this manuscript.
Footnotes
- ^The go/no-go lexical decision task should be distinguished from the go/no-go speeded pronunciation task (e.g., Hino and Lupker, 1998, 2000). In the latter task, participants name a stimulus aloud only if it is a word and withhold their response if it is a non-word. One of the reviewers made the interesting suggestion that this task could potentially provide important new insights into the task-specificity of semantic effects. Specifically, in go/no-go pronunciation, the response is the same as the one required in the standard pronunciation task but there is also a lexical decision involved. Performance on the go/no-go pronunciation task could then address the question of whether the increased influence of semantics on lexical decision is due to differences in the response modality (i.e., vocal response vs. button press) or the fact that lexical decision involves a word/non-word discrimination.
- ^The McRae et al. (2005) concrete nouns were selected as stimuli because number of semantic features is available for this set of words.
- ^There were too few response errors in most of the tasks to warrant parallel analyses of the accuracy data.
- ^Although number of associates (Duñabeitia et al., 2008; Müller et al., 2010) is clearly an important semantic richness variable, including this predictor would have greatly reduced the statistical power of our analyses, given that NoA values were available for only 377 (out of 473) of our stimuli. We did conduct additional analyses examining NoA effects for this subset of words, and found that with the exception of speeded pronunciation, NoA effects were not significant on any task (see also Yap et al., 2011). However, it is possible that these results are specific to the items used in the present study (i.e., concrete nouns).
References
Baayen, R. H. (2012). languageR: Data Sets and Functions with “Analyzing Linguistic Data: A Practical Introduction to Statistics,” R Package Version 1.4. Vienna: R Foundation for Statistical Computing.
Baayen, R. H., Davidson, D. J., and Bates, D. M. (2008). Mixed-effects modeling with crossed random effects for subjects and items. J. Mem. Lang. 59, 390–412.
Balota, D. A. (1990). “The role of meaning in word recognition,” in Comprehension Processes in Reading, eds D. A. Balota, G. B. Flores d’Arcais, and K. Rayner (Hillsdale, NJ: Lawrence Erlbaum Associates), 9–32.
Balota, D. A., and Chumbley, J. I. (1984). Are lexical decisions a good measure of lexical access? The role of word frequency in the neglected decision stage. J. Exp. Psychol. Hum. Percept. Perform. 10, 340–357.
Balota, D. A., Cortese, M. J., Sergent-Marshall, S., Spieler, D. H., and Yap, M. J. (2004). Visual word recognition of single-syllable words. J. Exp. Psychol. Gen. 133, 336–345.
Balota, D. A., Ferraro, R. F., and Connor, L. T. (1991). “On the early influence of meaning in word recognition: a review of the literature,” in The Psychology of Word Meanings, ed. P. J. Schwanenflugel (Hillsdale, NJ: Erlbaum), 187–222.
Balota, D. A., and Yap, M. J. (2006). “Attentional control and flexible lexical processing: explorations of the magic moment of word recognition,” From Inkmarks to Ideas: Current Issues in Lexical Processing, ed. S. Andrews (New York: Psychology Press), 229–258.
Balota, D. A., Yap, M. J., and Cortese, M. J. (2006). “Visual word recognition: the journey from features to meaning (a travel update),” in Handbook of Psycholinguistics, 2nd Edn, eds M. Traxler and M. A. Gernsbacher (Amsterdam: Academic Press), 285–375.
Bates, D. M., Maechler, M., and Dai, B. (2012). lme4: Linear Mixed-Effect Models Using S4 Classes, R Package Version 0.999375-42. Vienna: R Foundation for Statistical Computing.
Bennett, S. D. R., Burnett, A. N., Siakaluk, P. D., and Pexman, P. M. (2011). Imageability and body-object interaction ratings for 599 multisyllabic nouns. Behav. Res. Methods 43, 1100–1109.
Borowsky, R., and Masson, M. E. J. (1996). Semantic ambiguity effects in word identification. J. Exp. Psychol. Learn. Mem. Cogn. 22, 63–85.
Brysbaert, M., and New, B. (2009). Moving beyond Kucera and Francis: a critical evaluation of current word frequency norms and the introduction of a new and improved word frequency measure for American English. Behav. Res. Methods 41, 977–990.
Buchanan, L., Westbury, C., and Burgess, C. (2001). Characterizing semantic space: neighborhood effects in word recognition. Psychon. Bull. Rev. 8, 531–544.
Carreiras, M., and Grainger, J. (2004). Sublexical units and the “front end” of visual word recognition. Lang. Cogn. Process. 19, 321–331.
Carreiras, M., Perea, M., and Grainger, J. (1997). Effects of orthographic neighborhood in visual word recognition: cross-task comparisons. J. Exp. Psychol. Learn. Mem. Cogn. 23, 857–871.
Chumbley, J. I., and Balota, D. A. (1984). A word’s meaning affects the decision in lexical decision. Mem. Cognit. 12, 590–606.
Coltheart, M., Davelaar, E., Jonasson, J. T., and Besner, D. (1977). “Access to the internal lexicon,” in Attention and Performance VI, ed. S. Dornic (Hillsdale, NJ: Erlbaum), 535–555.
Coltheart, M., Rastle, K., Perry, C., Langdon, R., and Ziegler, J. (2001). DRC: a dual route cascaded model of visual word recognition and reading aloud. Psychol. Rev. 108, 204–256.
Cortese, M. J., and Fugett, A. (2004). Imageability ratings for 3,000 monosyllabic words. Behav. Res. Methods Instrum. Comput. 36, 384–387.
Cortese, M. J., Simpson, G. B., and Woolsey, S. (1997). Effects of association and imageability on phonological mapping. Psychon. Bull. Rev. 4, 226–231.
Dilkina, K., McClelland, J. L., and Plaut, D. C. (2008). A single-system account of semantic and lexical deficits in five semantic dementia patients. Cogn. Neuropsychol. 25, 136–164.
Dilkina, K., McClelland, J. L., and Plaut, D. C. (2010). Are there mental lexicons? The role of semantics in lexical decision. Brain Res. 1365, 66–81.
Dufau, S., Stevens, M., and Grainger, J. (2008). Windows executable software for the progressive demasking task. Behav. Res. Methods 40, 33–37.
Duñabeitia, J. A., Avilés, A., and Carreiras, M. (2008). NoA’s Ark: influence of the number of associates in visual word recognition. Psychon. Bull. Rev. 15, 1072–1077.
Ferrand, L., Brysbaert, M., Keuleers, E., New, B., Bonin, P., Méot, A., Augustinova, M., and Pallier, C. (2011). Comparing word processing times in naming, lexical decision, and progressive demasking: evidence from Chronolex. Front. Psychol. 2:306. doi:10.3389/fpsyg.2011.00306
Gernsbacher, M. A. (1984). Resolving 20 years of inconsistent interactions between lexical familiarity and orthography, concreteness, and polysemy. J. Exp. Psychol. Gen. 113, 256–281.
Gordon, B. (1983). Lexical access and lexical decision: mechanisms of frequency sensitivity. J. Mem. Lang. 22, 24–44.
Grainger, J., and Jacobs, A. M. (1996). Orthographic processing in visual word recognition: a multiple read-out model. Psychol. Rev. 103, 518–565.
Grainger, J., Van Kang, M., and Segui, J. (2001). Cross-modal priming from heterographic homophones. Mem. Cognit. 29, 53–61.
Hargreaves, I. S., Leonard, G. A., Pexman, P. M., Pittman, D. J., Siakaluk, P. D., and Goodyear, B. G. (2012). The neural correlates of the body-object interaction effect in semantic processing. Front. Hum. Neurosci. 6:22. doi:10.3389/fnhum.2012.00022
Hargreaves, I. S., Pexman, P. M., Pittman, D. J., and Goodyear, B. G. (2011). Tolerating ambiguity: ambiguous words recruit the left inferior frontal gyrus in absence of a behavioral effect. Exp. Psychol. 58, 19–30.
Harm, M. W., and Seidenberg, M. S. (2004). Computing the meanings of words in reading: cooperative division of labor between visual and phonological processes. Psychol. Rev. 111, 662–720.
Hino, Y., and Lupker, S. J. (1996). Effects of polysemy in lexical decision and naming: an alternative to lexical access accounts. J. Exp. Psychol. Hum. Percept. Perform. 22, 1331–1356.
Hino, Y., and Lupker, S. J. (1998). The effects of word frequency for Japanese kana and kanji words in naming and lexical decision: can the dual-route model save the lexical-selection account? J. Exp. Psychol. Hum. Percept. Perform. 24, 1431–1453.
Hino, Y., and Lupker, S. J. (2000). The effects of word frequency and spelling-to-sound regularity in naming with and without preceding lexical decision. J. Exp. Psychol. Hum. Percept. Perform. 26, 166–183.
Hino, Y., Lupker, S. J., and Pexman, P. M. (2002). Ambiguity and synonymy effects in lexical decision, naming and semantic categorization tasks: interactions between orthography, phonology and semantics. J. Exp. Psychol. Learn. Mem. Cogn. 28, 686–713.
Hino, Y., Pexman, P., and Lupker, S. (2006). Ambiguity and relatedness effects in semantic tasks: are they due to semantic coding? J. Mem. Lang. 55, 247–273.
Hodges, J. R., Garrard, P., and Patterson, K. (1998). “Semantic dementia,” in Pick’s Disease and Pick Complex, eds A. Kertesz and D. G. Munoz (New York: Wiley-Liss), 83–104.
Hoffman, P., Rogers, T. T., and Lambon Ralph, M. A. (2011). Semantic diversity accounts for the “missing” word frequency effect in stroke aphasia: insights using a novel method to quantify contextual variability in meaning. J. Cogn. Neurosci. 23, 2432–2446.
Juhasz, B. J., Yap, M. J., Dicke, J., Taylor, S. C., and Gullick, M. M. (2011). Tangible words are recognized faster: the grounding of meaning in sensory and perceptual systems. Q. J. Exp. Psychol. 64, 1683–1691.
Klepousniotou, E., and Baum, S. R. (2007). Disambiguating the ambiguity advantage effect in word recognition: an advantage for polysemous but not homonymous words. J. Neurolinguistics 20, 1–24.
McRae, K., Cree, G. S., Seidenberg, M. S., and McNorgan, C. (2005). Semantic feature production norms for a large set of living and nonliving things. Behav. Res. Methods 37, 547–559.
Mirman, D., and Magnuson, J. S. (2006). “The impact of semantic neighborhood density on semantic access,” in Proceedings of the 28th Annual Conference of the Cognitive Science Society, eds R. Sun and N. Miyake (Mahwah, NJ: Erlbaum), 1823–1828.
Müller, O., Duñabeitia, J. A., and Carreiras, M. (2010). Orthographic and associative neighborhood density effects: what is shared, what is different? Psychophysiology 47, 455–466.
Nelson, D. L., McEvoy, C. L., and Schreiber, T. A. (1998). The University of South Florida Word Association, Rhyme, and Word Fragment Norms. Available at: http://www.usf.edu/FreeAssociation/ [retrieved March 9, 2011].
Perea, M., Rosa, E., and Gómez, C. (2002). Is the go/no-go lexical decision task an alternative to the yes/no lexical decision task? Mem. Cognit. 30, 34–45.
Perry, C., Ziegler, J. C., and Zorzi, M. (2007). Nested incremental modeling in the development of computational theories: the CDP+ model of reading aloud. Psychol. Rev. 114, 273–315.
Pexman, P. M. (in press). “Meaning-level influences on visual word recognition,” in Visual Word Recognition, ed. J. S. Adelman.
Pexman, P. M., Hargreaves, I. S., Siakaluk, P., Bodner, G., and Pope, J. (2008). There are many ways to be rich: effects of three measures of semantic richness on word recognition. Psychon. Bull. Rev. 15, 161–167.
Pexman, P. M., Hino, Y., and Lupker, S. J. (2004). Semantic ambiguity and the process of generating meaning from print. J. Exp. Psychol. Learn. Mem. Cogn. 30, 1252–1270.
Pexman, P. M., Holyk, G. G., and Monfils, M. H. (2003). Number-of-features effects and semantic processing. Mem. Cognit. 31, 842–855.
Pexman, P. M., and Lupker, S. J. (1999). Ambiguity and visual word recognition: can feedback explain both homophone and polysemy effects? Can. J. Exp. Psychol. 53, 323–334.
Pexman, P. M., Lupker, S. J., and Jared, D. (2001). Homophone effects in lexical decision. J. Exp. Psychol. Learn. Mem. Cogn. 27, 139–156.
Piercey, C. D., and Joordens, S. (2000). Turning an advantage into a disadvantage: ambiguity effects in lexical decision versus reading tasks. Mem. Cognit. 28, 657–666.
Plaut, D. C., McClelland, J. L., Seidenberg, M., and Patterson, K. E. (1996). Understanding normal and impaired word reading: computational principles in quasi-regular domains. Psychol. Rev. 103, 56–115.
Plaut, D. C., and Shallice, T. (1993). Deep dyslexia: a case study of connectionist neuropsychology. Cogn. Neuropsychol. 10, 377–500.
R Development Core Team. (2011). R: A Language and Environment for Statistical Computing. Vienna: R Foundation for Statistical Computing.
Rayner, K. (1998). Eye movements in reading and information processing: 20 years of research. Psychol. Bull. 124, 372–422.
Rodd, J., Gaskell, G., and Marslen-Wilson, W. (2002). Making sense of semantic ambiguity: semantic competition in lexical access. J. Mem. Lang. 46, 245–266.
Schneider, W., Eschman, A., and Zuccolotto, A. (2002). E-Prime User’s Guide. Pittsburgh: Psychology Software Tools, Inc.
Schock, J., Cortese, M. J., and Khanna, M. M. (in press). Imageability estimates for 3000 disyllabic words. Behav. Res. Methods.
Schwanenflugel, P. J. (1991). “Why are abstract concepts hard to understand?” The Psychology of Word Meanings, ed P. J. Schwanenflugel (Hillside, NJ: Erlbaum), 223–250.
Seidenberg, M. S., and McClelland, J. L. (1989). A distributed, developmental model of word recognition and naming. Psychol. Rev. 96, 523–568.
Shaoul, C., and Westbury, C. (2010). Exploring lexical co-occurrence space using HiDEx. Behav. Res. Methods 42, 393–413.
Siakaluk, P. D., Pexman, P. M., Aguilera, L., Owen, W. J., and Sears, C. R. (2008). Evidence for the activation of sensorimotor information during visual word recognition: the body-object interaction effect. Cognition 106, 433–443.
Strain, E., Patterson, K., and Seidenberg, M. S. (1995). Semantic effects in single-word naming. J. Exp. Psychol. Learn. Mem. Cogn. 21, 1140–1154.
Tillotson, S. M., Siakaluk, P. D., and Pexman, P. M. (2008). Body-object interaction ratings for 1,618 monosyllabic nouns. Behav. Res. Methods 40, 1075–1078.
Wellsby, M., Siakaluk, P. D., Owen, W. J., and Pexman, P. M. (2011). Embodied semantic processing: the body-object interaction effect in a non-manual task. Lang. Cogn. 3, 1–14.
Yap, M. J., and Balota, D. A. (2009). Visual word recognition of multisyllabic words. J. Mem. Lang. 60, 502–529.
Yap, M. J., Tan, S. E., Pexman, P. M., and Hargreaves, I. S. (2011). Is more always better? Effects of semantic richness on lexical decision, speeded pronunciation, and semantic classification. Psychon. Bull. Rev. 18, 742–750.
Yarkoni, T., Balota, D. A., and Yap, M. J. (2008). Beyond Coltheart’s N: a new measure of orthographic similarity. Psychon. Bull. Rev. 15, 971–979.
Keywords: semantic richness, visual word recognition, imageability, semantic neighborhood density, body-object interaction, semantic classification, lexical decision, progressive demasking
Citation: Yap MJ, Pexman PM, Wellsby M, Hargreaves IS and Huff MJ (2012) An abundance of riches: cross-task comparisons of semantic richness effects in visual word recognition. Front. Hum. Neurosci. 6:72. doi: 10.3389/fnhum.2012.00072
Received: 05 January 2012; Accepted: 15 March 2012;
Published online: 17 April 2012.
Edited by:
Paul D. Siakaluk, University of Northern British Columbia, CanadaReviewed by:
Jon Andoni Duñabeitia, Basque Center on Cognition, Brain and Language, SpainDavid Balota, Washington University, USA
Michael J. Cortese, University of Nebraska at Omaha, USA
Copyright: © 2012 Yap, Pexman, Wellsby, Hargreaves and Huff. This is an open-access article distributed under the terms of the Creative Commons Attribution Non Commercial License, which permits non-commercial use, distribution, and reproduction in other forums, provided the original authors and source are credited.
*Correspondence: Melvin J. Yap, Department of Psychology, Faculty of Arts and Social Sciences, National University of Singapore, Block AS4, #02-07, Singapore 117570. e-mail:bWVsdmluQG51cy5lZHUuc2c=