- 1Vanderbilt Brain Institute, Vanderbilt University, Nashville, TN, United States
- 2Vanderbilt University Medical Center, Nashville, TN, United States
- 3Vanderbilt Kennedy Center, Nashville, TN, United States
- 4Department of Hearing and Speech Sciences, Vanderbilt University Medical Center, Nashville, TN, United States
Although fMRI studies have produced considerable evidence for differences in the spatial connectivity of resting-state brain networks in persons with autism spectrum disorder (ASD) relative to typically developing (TD) peers, little is known about the temporal dynamics of these brain networks in ASD. The aim of this study was to examine the EEG microstate architecture in children with ASD as compared to TD at rest in two separate conditions – eyes-closed (EC) and eyes-open (EO). EEG microstate analysis was performed on resting-state data of 13 ASD and 13 TD children matched on age, gender, and IQ. We found that children with ASD and TD peers produced topographically similar canonical microstates at rest. Group differences in the duration and frequency of these microstates were found primarily in the EC resting-state condition. In line with previous fMRI findings that have reported differences in spatial connectivity within the salience network (previously correlated with the activity of microstate C) in ASD, we found that the duration of activation of microstate C was increased, and the frequency of microstate C was decreased in ASD as compared to TD in EC resting-state. Functionally, these results may be reflective of alterations in interoceptive processes in ASD. These results suggest a unique pattern of EEG microstate architecture in ASD relative to TD during resting-states and also that EEG microstate parameters in ASD are susceptible to differences in resting-state conditions.
Introduction
Numerous studies of functional connectivity in ASD using fMRI have examined the network-level brain alterations in ASD at rest (“resting-state”) compared to typically developing (TD) individuals (Hull et al., 2017). While there is no signature pattern in the network alterations in ASD, studies provide evidence for both over- and under-connectivity (Cherkassky et al., 2006; Hughes, 2007; Assaf et al., 2010; Di Martino et al., 2011; Abrams et al., 2013; Supekar et al., 2013; Tyszka et al., 2014; Cerliani et al., 2015; Chen S. et al., 2017; Tomasi and Volkow, 2019). A majority of resting-state fMRI studies in persons with ASD report aberrant connectivity within the salience network (SN) (Supekar et al., 2013; Nomi and Uddin, 2015; Abbott et al., 2016; Green et al., 2016; Chen H. et al., 2017; Damiani et al., 2018). Indeed, the specificity of this effect was demonstrated in one study that showed that 78% of their sample of ASD participants could be classified accurately based on fMRI SN connectivity alone (Uddin et al., 2013). In addition, the same study found that the degree of SN activity strongly correlated with the severity of repetitive behavior phenotype in ASD, suggesting that SN activity may be important for understanding specific aspects of the ASD phenotype.
Although considerable evidence exists in ASD regarding the structural characteristics of brain networks in general (Hull et al., 2017) and the SN in particular (Uddin et al., 2013; Abbott et al., 2016; Chen H. et al., 2017), much less is known about the temporal dynamics of these networks. Reports of longer fMRI dwell times (Bressler and Seth, 2011; Barnett and Seth, 2014; Yuan et al., 2016), i.e., the duration of activation of brain networks, have been shown to be associated with the severity of ASD symptoms (Watanabe and Rees, 2017; Rashid et al., 2018). Comparable dynamics were observed by King et al., 2018, who found prolonged temporal synchrony of functional networks in ASD (King et al., 2018). One study found fewer rapid transitions between brain networks in ASD compared to TD (Watanabe and Rees, 2017). Studies by Bernas et al., 2018 and Damiani et al., 2018 have confirmed the abnormal temporal structure of the resting-state SN in ASD (Bernas et al., 2018; Damiani et al., 2018). Although these studies have shed light on the temporal dynamics of brain network activity in ASD, they have been limited by the time resolution of the BOLD fMRI signal.
To overcome these limitations in time resolution, recent studies have used EEG microstates as an approach to study the temporal dynamics of brain networks (Michel and Koenig, 2018). An advantage of considering EEG microstates as a proxy for network activation is that their timescale coincides with the sub-second range of synchronous firing of large neural networks. EEG microstates create a global representation of a functional state and are defined by the topography of electric potentials of a multichannel electrode array (Strik and Lehmann, 1993). These microstate topographies remain quasi-stable for 80–120 ms before switching to different microstate topographies (Wackermann et al., 1993; Khanna et al., 2015). The transitions between microstates can represent sequential activation of different neural networks, and in this way the dynamic nature of the neural basis of cognitive activity can be measured (Milz et al., 2016; Seitzman et al., 2017). EEG microstate analysis is rich with parameters of potential neurophysiological relevance. These parameters include: (a) The average duration of each microstate, which is the average length of time a given microstate remains stable. (b) The frequency of each microstate, which is the average number of times per second that the microstate becomes dominant during the recording period. (c) The coverage of a microstate, which is the fraction of total recording time that the microstate is dominant. (d) The transition probabilities of a microstate, which indicate switching from one class to any other in a non-random sequence (Khanna et al., 2015).
Across studies, evidence has converged for four archetypal microstates that explain most of the global topographic variance in resting-state (Dierks et al., 1997; Koenig et al., 2002; Strelets et al., 2003; Brodbeck et al., 2012; Nishida et al., 2013; Drissi et al., 2016; Atluri et al., 2017; Seitzman et al., 2017; Sverak et al., 2017; Zappasodi et al., 2017). These have been labeled as A, B, C, and D based on their topographical configuration. Simultaneous fMRI-EEG studies as well as EEG source imaging studies suggest that these microstate classes correspond to the activity of previously identified networks for phonological processing, the visual network, the saliency network, and attention network, respectively (Britz et al., 2010; Custo et al., 2017). Alterations in the expression of these microstates have been observed in a variety of neuropsychiatric conditions including depression (Atluri et al., 2017), Alzheimer’s disease (Dierks et al., 1997; Strik et al., 1997; Nishida et al., 2013), frontotemporal dementia (Strik et al., 1997; Nishida et al., 2013; Grieder et al., 2016), stroke (Zappasodi et al., 2017), and schizophrenia (Merrin et al., 1990; Stevens et al., 1997; Strelets et al., 2003; Lehmann et al., 2005; Kikuchi et al., 2007; Kindler et al., 2011; Nishida et al., 2013; Tomescu et al., 2014; Sverak et al., 2017). However, to date, only two such studies have been done in persons with ASD. Jia and Yu (2019) found that resting-state microstate parameters differed in adolescents with ASD as compared to age-matched typical controls in data containing mixed periods of eyes-open (EO) and eyes-closed (EC) conditions with increased frequency and coverage of microstate B, decreased duration of microstate A, and decreased duration and coverage of microstate C. Given that the two eye conditions result in functional differences in microstate readouts (Seitzman et al., 2017), it is necessary to investigate these two conditions separately. In the second study, D’Croz-Baron et al., 2019 also found that several microstate parameters differed in ASD adults as compared to the control group in EC resting-state (D’Croz-Baron et al., 2019). This study found an increase in frequency and coverage of microstate B, and a decrease in the duration and coverage of microstate C. However, this study used the updated meta-criterion (Custo et al., 2017; Michel and Koenig, 2018) to select more than four classes of microstates so the results of the other microstate classes from these two studies are not comparable. Neither study examined the correlation between the microstate parameters and behavioral measures in ASD. Given the importance of controlling for methodological differences in how “resting-state” is operationalized during data collection, the purpose of this study was to investigate the resting-state EEG microstate architecture in children with ASD compared to typical controls under both EC and EO resting-state conditions. We hypothesized that the temporal dynamics of microstate C would be altered in ASD reflecting atypical SN activity.
Materials and Methods
Participants
Children with a diagnosis of ASD and TD children between the ages of 8–14 years were recruited to participate in this study. All participants’ parents provided written informed consent and received monetary compensation. The study was approved by the Vanderbilt University Institutional Review Board (140436). Demographic and clinical data on both groups is provided in Table 1.
Children With ASD
A total of 25 children with ASD were recruited for this study through a variety of resources (email distribution service, ResearchMatch.org database, local autism support groups, and flyers posted in autism clinics). Participants were required to have an existing clinical diagnosis of ASD from a licensed clinician. All diagnoses were also confirmed during the study visit by the administration of the Autism Diagnostic Observation Schedule-2 [ADOS-2 (Lord et al., 2012)] by trained research personnel. Exclusion criteria included a history of comorbid psychiatric disorders, epilepsy (one child), brain abnormalities (one child: cortical dysplasia), ADOS-2 score below 7 (one child: ADOS score 4), left-handedness, insufficient or noisy EEG data (six children), and no data for either of the two eye conditions (three children). This resulted in the final sample of 13 children in the ASD group (two females) with a mean age of 9.7 years (SD = 1.5) and an age range between 8 years 2 months and 12 years 9 months. Within the final sample, 6 participants took various ADHD medications. 2 of the 6 also took SSRIs and 1 of the 6 also took risperidone. All six participants on medication were on a stable dose for at least 2 months. On the day of data acquisition, participants took their medications as prescribed.
TD Children
A total of 38 typically developing children were recruited to serve as the control group via an email sent to the university faculty and staff. Exclusion criteria included a history of psychiatric or developmental disorders (one child), current use of psychotropic medication, epilepsy, brain abnormalities, diagnosis of ASD in an immediate family member, a score above the ASD cutoff on the Social Responsiveness Scale (SRS), left-handedness (three children), no data for either of the two eye conditions (nine children), and noisy or insufficient EEG data (three children). This resulted in a pool of 22 children from which a final sample of 13 children (two females) was selected based on age, gender, and IQ matching with the ASD group. This TD group had a mean age of 10.4 years (SD = 1.4) and an age range between 8 years 0 months and 12 years 5 months.
Procedure
Five minutes of resting-state EEG was collected in two eye conditions: EO and EC, with a short (2–5 min) break between each condition. The order in which participants performed these conditions was counterbalanced. For participants with ASD up to 10 min of each resting condition was recorded when possible to maximize the availability of artifact-free data. Participants were asked to remain still and awake and allow their minds to wander. To minimize eye movements during the EO condition, participants were asked to fixate on a white cross in the center of a black background on the computer screen. To minimize eye movements during the EC condition, participants were asked to close their eyes while fixated on the cross on the screen and keep their eyes in the same position as if still gazing at the fixation cross. Participants were seated an average of four feet from the monitor.
EEG data was monitored online during recording to ensure quality. If the experimenter noticed obvious deviations from study protocol (e.g., tapping fingers, clenching the jaw, raising eyebrows), the task was paused and the participant was given verbal feedback regarding adhering to instructions. During instances when participants failed to follow the instructions, data collection was stopped and the participants were reinstructed on the task before resuming data collection. Data collection was aborted if a participant required two or more task interruptions plus re-instructions and that participant was excluded from further data analysis (see exclusion criteria).
EEG
Continuous EEG was recorded from the scalp at a sampling rate of 1000 Hz using a high-density array of 128 Ag/AgCl electrodes embedded in soft sponges (Geodesic Sensor Net, EGI, Inc., Eugene, OR, United States) using NetStation 5.3 software. Recording began following the adjustment of electrode impedances to below 50 kΩ. During online recording, data was referenced to vertex with onscreen filters set at 0.1–100 Hz.
Signal Processing
Continuous EEG data were preprocessed using the NetStation Waveform Tools software. (i) Data were down-sampled to 125 Hz to reduce computational load, (ii) bandpass filtered between 2 and 20 Hz, (iii) notch filtered to remove 60 Hz noise, (iv) segmented into 2 s epochs, (v) processed for artifacts using NetStation Waveform Tools algorithms, (vi) all epochs were manually inspected for artifacts and those containing obvious eye-blink, saccades, muscle noise, or non-physiological artifacts not already excluded by the artifact detection algorithms in NetStation were rejected. Bad channels were replaced using the spherical spline interpolation algorithm (Perrin et al., 1989). The data were then re-referenced to the average reference and baseline corrected. Data were exported from NetStation 5.3 for offline analysis in the MATLAB® (Version 2018a, The Mathworks, Natick, MA, United States) toolbox EEGLab (Version 14.1.2.0b)1. EEG microstates were extracted using the EEGLAB plugin developed by Dr. Thomas Koenig.2
Microstate Analysis
Following the methods of Koenig et al., 2002, the first 10 artifact-free epochs for each condition were analyzed (Koenig et al., 2002). For each of these datasets, the global field power (GFP) curve, equivalent to the standard deviation of amplitude across the entire average-referenced electrode montage for each sample of time (Skrandies, 1990), was calculated. The topographic maps occurring at the peaks of the GFP curve were entered into a Topographic Atomize and Agglomerate Hierarchical Clustering (T-AAHC) algorithm to isolate four microstate clusters within each dataset. We a priori chose to extract four group-level microstate clusters in order to remain consistent with previous studies that report the four canonical microstates. The group-level microstate classes were then identified for both participant groups for the EO and EC conditions separately. These group-level maps were sorted according to the normative maps published in Koenig et al. (2002). The order of the individual participant microstate template maps was determined by the spatial correlation with the group-level microstate classes. The original EEG data of each participant for both resting-state conditions were re-expressed as the microstate class that they were most similar to with respect to the group level maps, as determined by Pearson’s correlation. From this time series, the microstate parameters such as global explained variance (GEV), duration, frequency, coverage, and GFP were computed from the maps in their center-specific configurations.
Psychometric Measures
Autism Diagnostic Observation Schedule – 2 (ADOS-2) (Lord et al., 2012): The ADOS-2 is a semi-structured, play-based assessment to evaluate the core features of ASD. In addition to providing a score to measure against diagnostic thresholds, the score provided by the ADOS-2 is a marker of ASD severity (Gotham et al., 2009).
Social Communication Questionnaire (SCQ) (Rutter et al., 2003): The SCQ is a parent report questionnaire used to evaluate the communication skills and social functioning of children with ASD.
Social Responsiveness Scale (SRS) (Constantino and Gruber, 2002): The SRS is a parent report questionnaire intended to measure social behavior impairments such as social awareness, social information processing, capacity for reciprocal social communication, and social anxiety/avoidance in children between the ages of 4–18 years. An additional section of the SRS contains questions regarding preoccupations and traits related to autism.
Repetitive Behavior Scale – Revised (RBS-R) (Bodfish et al., 1999): The RBS-R is a parent report questionnaire that assesses five categories of repetitive behaviors, namely motor stereotypy, repetitive self-injury, compulsions, routines/sameness, and restricted interests.
Stanford Binet-5 Abbreviated IQ Test (SB5) (Roid, 2003): The Stanford Binet is an IQ test developed to measure developmental or intellectual disabilities in children. The five factors tested are knowledge, quantitative reasoning, visual-spatial processing, working memory, and fluid reasoning. The present study used the abbreviated version to obtain an estimate of each child’s developmental level.
Statistical Analysis
Statistical analyses were performed in SPSS v. 26.0 (IBM Corp., Armonk, NY, United States). Separate repeated-measures (RM) analyses of variance (ANOVAs) were conducted for each microstate for the following parameters: GEV, duration, occurrence, coverage, and GFP. Each ANOVA contained one between-subject factor for group (ASD or TD), and one within-subject factor for eye condition (EC or EO). Significant interaction effects (group × eye condition) were decomposed using post hoc analyses to identify the direction of the effect. Statistical significance was set at a p-value less than 0.05 for the RM-ANOVAs. The significance levels of these post hoc comparisons were corrected for multiple comparisons using the Bonferroni method (statistically significant p-value set at 0.0125).
To assess the relationships between microstate measures and psychometric data in ASD, Pearson’s r was employed. To adjust for the skewness in the distribution of psychometric data, each variable was log transformed by a factor of log(x+1). To reduce the number of correlations examined, and in line with the a priori hypotheses of the study, the correlation analyses of microstate parameters with psychometric measures were restricted to examining microstate C frequency in the EC condition in ASD and age, IQ, SRS-Total, and RBSR-Total (with pairwise error rate corrected using the Bonferroni method).
Results
The topographies of the four microstate classes are displayed in Figure 1. The group level maps resemble those of the normative data (Koenig et al., 2002) and those reported in previous studies (Koenig et al., 2002; Britz et al., 2010). The four microstate classes were labeled A, B, C, and D, respectively, in accordance with their topographic shapes. Microstate A has a right frontal, left occipital orientation, Microstate B has a left frontal, right occipital orientation, Microstate C has an anterior-posterior orientation, and Microstate D has a fronto-central maximum. Table 2 shows the summary statistics for all the parameters of these microstates. The four microstate classes display different patterns in temporal dynamics across the two groups and eye conditions. These differences were statistically tested using RM-ANOVAs. The results of the RM-ANOVAs are displayed in Table 3.
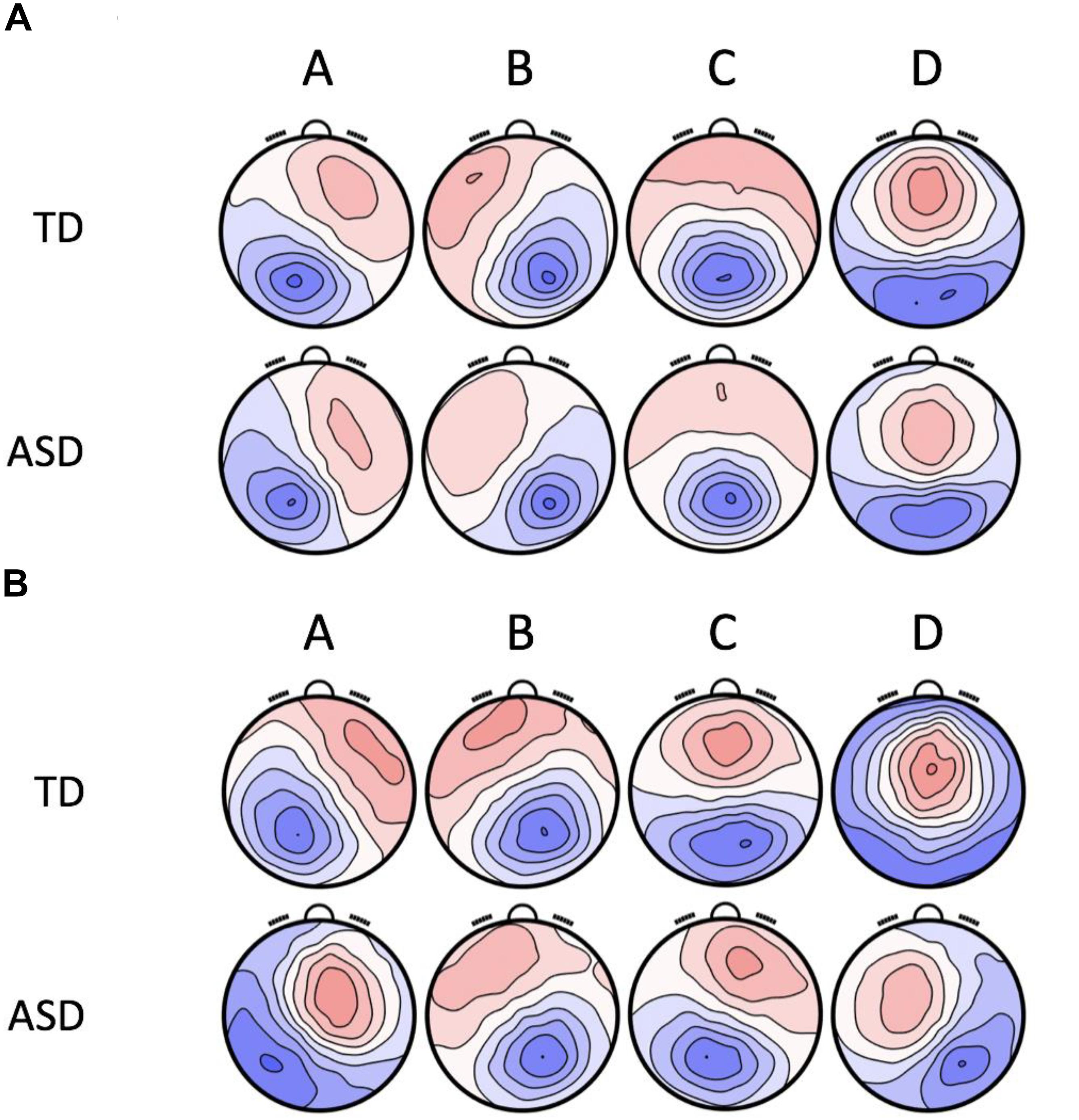
Figure 1. The group-level maps of the four microstate classes (A–D) in ASD and TD, independently computed for the two resting-state conditions. (A) Group Level Microstate Maps in Eyes Closed Resting-state. (B) Group Level Microstate Maps in Eyes Open Resting-state.
GEV
The total GEV is a measure of how well all four microstate template maps together explain the topographies for every moment in time for each dataset (Murray et al., 2008). There were no significant interactions between the group × eye condition for GEV [F(1,24) = 3.175, p = 0.088, = 0.117]. There was a main effect of group [F(1,24) = 4.981, p = 0.035, = 0.172] with greater GEV in ASD (p = 0.005) and a main effect of eye condition [F(1,24) = 5.450, p = 0.028, = 0.185]. However, the GEV for the two conditions was not significantly different after correcting for multiple comparisons (p = 0.233).
Microstate A
There were no significant interactions between group × eye condition for duration, frequency, coverage, or GFP. There was a main effect of eye condition for frequency of microstate A [F(1,24) = 5.605, p = 0.026, = 0.189] with increased frequency of microstate A in EO (p = 0.012). There were no main effects of group or eye condition for duration and coverage. For the GFP of microstate A, there was a main effect of group [F(1,24) = 24.3, p < 0.0001, = 0.537] with decreased GFP in ASD (p < 0.0001) and a main effect of eye condition [F(1,24) = 52.3, p < 0.0001, = 0.713] with decreased GFP in the EO condition (p = 0.012).
Microstate B
There was a significant interaction between group × eye condition for duration [F(1,24) = 5.417, p = 0.029, = 0.184]. Post hoc tests showed that within the ASD group, the duration of microstate B was greater in EC (p = 0.0051) than in EO (Figure 2A). Across the groups, the duration of microstate B was greater in ASD than in TD in the EC condition (p = 0.0039). There was a main effect of group for duration [F(1,24) = 5.571, p = 0.027, = 0.188] with increased duration of microstate B in ASD (p = 0.008). There was no main effect of eye condition for the duration of microstate B.
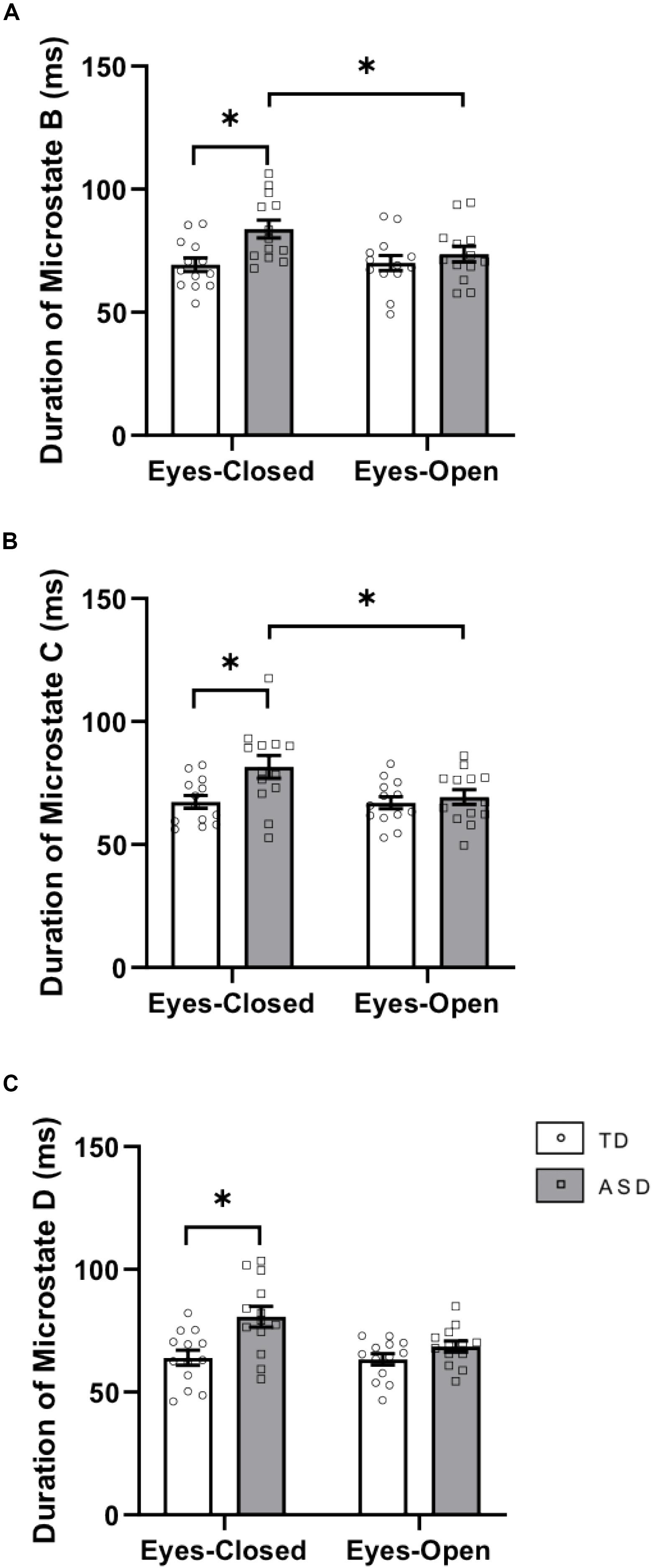
Figure 2. Durations of (A) microstate class B, (B) microstate class C, and (C) microstate class D in EC and EO for the two groups. This graph displays the mean duration of each microstate with corresponding standard error of the mean. The white and gray bars display results from the TD and ASD groups, respectively. individual participant data is plotted on each bar. All significant differences are indicated with asterisks (p < 0.0125, Bonferroni corrected).
There were no significant interactions between group × eye condition for frequency, coverage, or GFP. There was a main effect of eye condition for frequency [F(1,24) = 6.258, p = 0.020, = 0.207] with a trend toward increased frequencies of microstate B in EO (p = 0.064). For the GFP of microstate B, there was a main effect of group [F(1,24) = 22.5, p < 0.0001, = 0.506] with decreased GFPs in ASD (p < 0.0001) and a main effect of eye condition [F(1,24) = 61.9, p < 0.0001, = −0.738] with decreased GFP in the EO condition (p = 0.006).
Microstate C
There was a significant interaction between group × eye condition for duration [F(1,24) = 7.305, p = 0.012, = 0.233]. Post hoc tests showed that within the ASD group, the duration of microstate C was greater in EC (p = 0.0006) than in EO (Figure 2B). Across the groups, the duration of microstate C was greater in ASD than in TD in the EC condition (p = 0.01275). There was a main effect of eye condition for the duration of microstate C [F(1,24) = 8.129, p = 0.009, = 0.253] with a trend toward greater duration of microstate C in EC (p = 0.079).
There was a significant interaction between group × eye condition for frequency [F(1,24) = 12.248, p = 0.002, = 0.338]. Post hoc tests showed that within the ASD group, microstate C was less frequent in EC (p = 0.0019) than in EO. Across the groups, microstate C was less frequent in ASD than in TD in the EC condition (p = 0.0003) (Figure 3A). There was a main effect of group for the frequency of microstate C [F(1,24) = 8.279, p = 0.008, = 0.256] with decreased frequencies of microstate C in ASD (p = 0.0014).
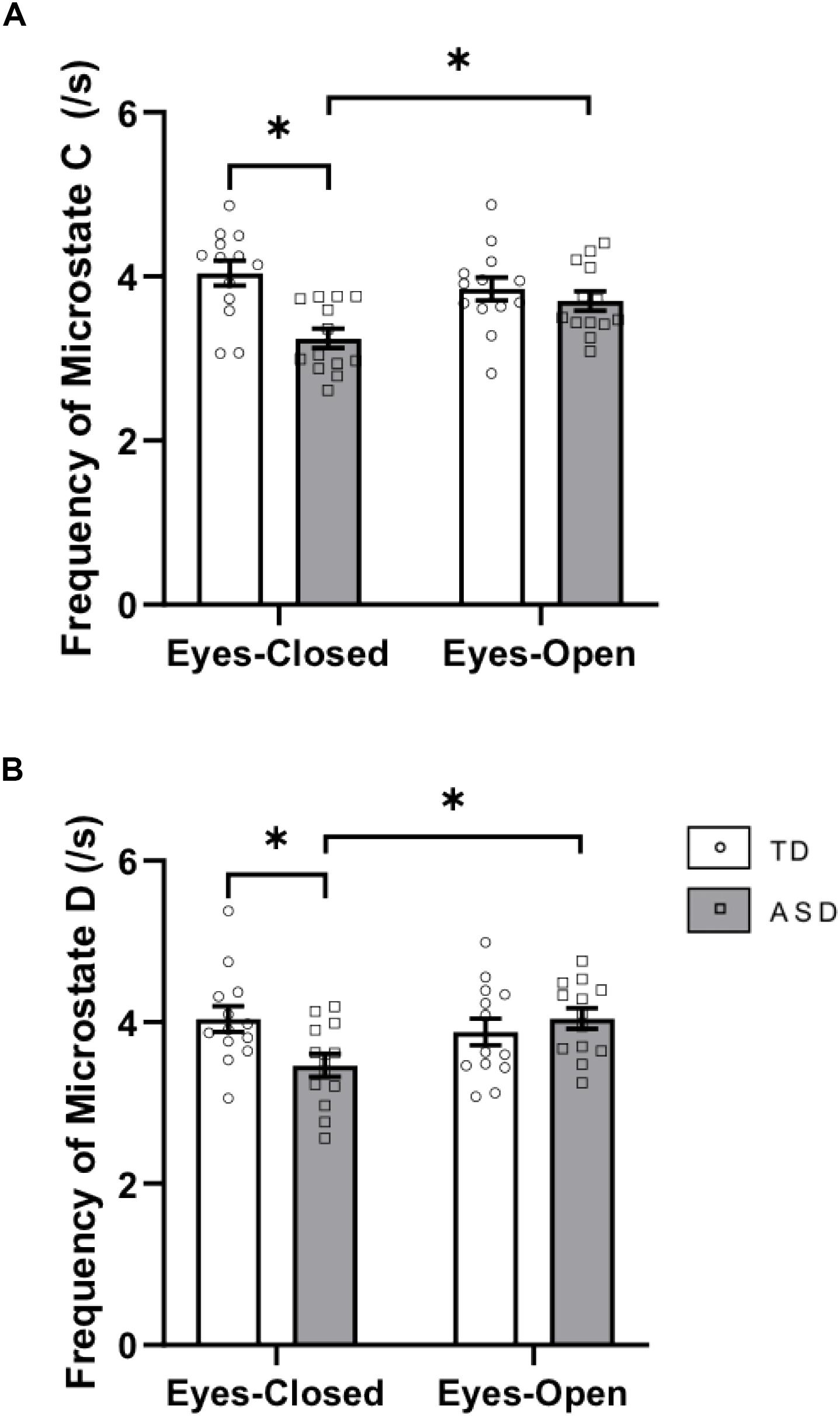
Figure 3. Frequency of (A) microstate class C and (B) microstate class D in EC and EO for the two groups. These graphs display the mean frequencies of each microstate with corresponding standard error of the mean. The white and gray bars display results from the TD and ASD groups, respectively. individual participant data is plotted on each bar. All significant differences are indicated with asterisks (p < 0.0125, Bonferroni corrected).
There were no significant interactions between group × eye condition for coverage. For the GFP of microstate C, there was a main effect of group [F(1,24) = 22.2, p < 0.0001, = 0.481] with decreased GFP in ASD (p < 0.0001) and a main effect of eye condition [F(1,24) = 63.1, p < 0.0001, = 0.725] with decreased GFP in the EO condition (p = 0.0049).
Microstate D
There was a significant interaction between group × eye condition for duration [F(1,24) = 4.538, p = 0.044, = 0.159]. Post hoc tests showed that within the ASD group, the duration of microstate D was greater in EC (p = 0.0040) than in EO. Across the groups, microstate D had a greater duration in ASD than in TD in the EC condition (p = 0.0040). There was a main effect of eye condition for the duration of microstate D [F(1,24) = 5.609, p = 0.026, = 0.189] with a trend toward greater duration of microstate D in EC (p = 0.073). There was also a main effect of group for the duration of microstate D [F(1,24) = 10.3, p = 0.004, = 0.301] with a greater duration of microstate D in ASD (p = 0.001) (Figure 2C).
There was a significant interaction between group × eye condition for frequency [F(1,24) = 11.291, p = 0.003, = 0.320]. Post hoc tests showed that within the ASD group, microstate D was less frequent in EC than in EO (p = 0.0010). Across the groups, microstate D trended toward being less frequent in ASD than in TD in EC (p = 0.0135) (Figure 3B). There were no main effects of group or eye condition for frequency of microstate D.
There were no significant interactions between group × eye condition for coverage [F(1,24) = 0.523, p = 0.476, = 0.021] or GFP [F(1,24) = 3.331, p = 0.081, = 0.126]. For the GFP of microstate D, there was a main effect of group [F(1,24) = 22.2, p < 0.0001, = 0.482] with decreased GFP in ASD (p < 0.0001) and a main effect of eye condition [F(1,24) = 63.1, p < 0.0001, = 0.752] with decreased GFP in the EO condition (p = 0.0039).
Correlations of Microstate Parameters and Psychometric Measures
No significant correlation was found between microstate C frequency and these psychometric measures with and without correction using the Bonferroni method for multiple comparisons.
Discussion
The purpose of this study was to investigate the resting-state EEG microstate architecture in ASD under EC and EO conditions. Based on established findings for SN activity in ASD using fMRI, we hypothesized that the temporal dynamics of microstate C would be altered in ASD in both eye conditions reflecting atypical activity. ASD and TD children between the ages of 8–14 years performed two resting-state tasks for 5 min, keeping their eyes either open or closed during data collection. The main outcomes of this study were that children with ASD produced topographically similar canonical microstates at rest in comparison to their TD peers. The differences between these groups in the parameters of these microstates were largely restricted to the eyes closed resting-state condition.
The four microstate maps (labeled A, B, C, and D) isolated for both resting-state conditions in the two groups were topographically similar to the canonical maps described in the normative study of adults by Koenig et al., 2002. In the EC resting-state, they accounted for 73.2 and 72.8% of the explained variance in TD and ASD, respectively. In the EO resting-state, these microstate maps accounted for 77.7 and 75.0% of the explained variance in TD and ASD, respectively. According to prior studies (Britz et al., 2010), microstate A reflects the activity of the bilateral superior temporal and parietal cortex, interpreted as the auditory cortex, microstate B reflects the activity of the striate and extrastriate cortex or the visual network, microstate C reflects the activity of the bilateral insular and anterior cingulate cortex or the SN, and microstate D reflects the activity of the fronto-parietal attentional network.
The findings of this study supported our hypothesis that the parameters of microstate C are altered in ASD at rest. This hypothesis was based on fMRI findings which described aberrant SN activity in ASD (Supekar et al., 2013; Nomi and Uddin, 2015; Abbott et al., 2016; Green et al., 2016; Chen H. et al., 2017; Damiani et al., 2018) and numerous studies that have linked microstate C to the SN (Stevens et al., 1997). Functionally, the SN has been presumed to have two roles: (1) a bottom-up process for the extraction of salient sensory stimuli from the external or internal environment and (2) a top-down mechanism for focusing one’s spotlight of attention on a goal-directed behavior (Menon and Uddin, 2010). In this study, at rest in the EC condition, we found that the duration of microstate C was increased, and the frequency of microstate C was decreased in children with ASD as compared to TD. Functionally, in ASD, this would imply that the SN remains activated for longer durations and is activated less frequently. This may explain the difficulty in processing complex but subtle environmental stimuli such as social cues in ASD individuals. Clinically, these difficulties may lead to impaired social and communication skills. We also found that the changes in the microstate parameters in ASD were not restricted to microstate C. The durations of microstates B and D were also increased in ASD in EC, which is consistent with the finding of longer dwell times across all brain networks in children with ASD using fMRI (Dierks et al., 1997; Atluri et al., 2017). This is also consistent with various reports of hyper-connectivity across brain networks using fMRI in ASD at the whole brain level (Chen S. et al., 2017; Damiani et al., 2018). Similarly, the frequencies of microstates A and B were significantly decreased in ASD in EC. This is consistent with findings in fMRI studies showing decreased switching between global brain networks in autism (Chen H. et al., 2017).
Our findings showing altered microstate dynamics in ASD are similar to observations by Jia and Yu (2019). However, the specific group differences were not the same. Whereas the duration of microstates C and A were significantly reduced in ASD compared to TD in the previous study, we found increased duration of microstate C as well as microstates B and D. Similarly, the previous study reported an increase in the frequency of microstate B in ASD as compared to TD, whereas we found that the frequencies of microstates A, B, and C were significantly reduced in ASD as compared to TD. The differences in microstate dynamics between these two studies may be explained by differences in the data collection methods as well as differences in the age range of the participants included. Whereas the previous study combined EC and EO resting-state data, we collected data from these two conditions as separate instances and analyzed them independently. This was based on a previous study by Seitzman et al., 2017 that found differences in EC and EO resting-state microstate dynamics, and also on several previous fMRI resting-state studies that have reported differences in network activity across resting-state conditions. Consistent with Seitzman et al., 2017, we found that the frequency of microstate B was increased and the durations of microstates A and D were decreased in the EO condition across groups.
In this study, we found that ASD-related differences in EEG microstate architecture were largely restricted to the EC resting-state condition. fMRI studies have suggested differences in the topological organizations of functional neural networks for exteroceptive and interoceptive processing during EO and EC resting-states (Xu et al., 2014). These studies observed an increase in interoceptive and self-referential processes in the EC condition as compared to the EO condition, which is reflected by an increase in the activity of the default mode network (DMN) (Marx et al., 2004; Xu et al., 2014). In addition to the SN, microstate C may also represent the activity of the anterior DMN (Britz et al., 2010; Milz et al., 2016; Michel and Koenig, 2018), which is implicated in self-referential processes as well as the integration of interoceptive information with emotional salience to form a subjective representation of a subject’s own body (Taylor et al., 2009; Andrews-Hanna, 2012). The group differences in microstate C parameters observed in the EC resting-state may be a result of differences in these interoceptive processes in ASD. Several studies have suggested an increased attention to internal rather than external cues in ASD (Schauder et al., 2015; Noel et al., 2018). During the EO resting-state, the differences in microstate parameters between the two groups may no longer be apparent due to the suppression of these interoceptive processes as resources are allocated to exteroceptive processes. The neural generators of microstate C are an area of active debate and require further exploration (Michel and Koenig, 2018).
These findings should be interpreted within the framework of the limitations of this study. One limitation is the amount of data used for the microstate analysis. We chose 20 s (10 epochs) of artifact-free data across participants for each of the resting-state conditions. This same amount of data was used by Koenig et al., 2002 for the generation of the normative microstate data and has been shown to be sufficient for this type of analysis (Lehmann et al., 2005; Nishida et al., 2013; Michel and Koenig, 2018). Other studies report larger amounts of data for microstate analysis per participant. However, those studies often employ artifact correction pipelines that may alter the underlying structure of the data. In contrast, our data were not modified in any manner. Another limitation of this study is that not all ASD participants were able to complete both resting-state conditions. Hence, the final sample size was considerably reduced. However, it also allowed us to systematically compare two functionally different resting-states in EC and EO conditions, which was not controlled for in the prior ASD study (Jia and Yu, 2019). Data loss in the ASD group necessitated reduction in the TD sample to ensure that the two participant groups remained closely matched at an individual level and to reduce the possibility that observed group differences in microstates could be explained by age, gender or IQ differences. Re-analysis of the full TD sample recapitulated the differences in microstate parameters observed for the smaller subgroup (Supplementary Figure S1 and Supplementary Tables S1, S2). Another potential limitation of our study is that we a priori chose to focus on four canonical microstate clusters for our analyses instead of applying a more recently described meta-criterion to generate a data-specific number of optimal clusters. The latter method resolves microstate C into two separate clusters that are thought to independently represent the SN and the DMN. However, it is still unclear if using the meta-criterion across different datasets of the same populations generates similar cluster numbers. Furthermore, using the four canonical clusters allowed us to directly compare our findings to other studies in ASD populations. We expected a correlation between the dynamics of the SN (as represented by microstate C) and the severity of ASD behaviors. However, the absence of the predicted association may reflect a need for a more nuanced functional interpretation of microstate C. Recent findings (Custo et al., 2017; Seitzman et al., 2017) suggest that it may also represent the activity of the DMN. Future studies resolving microstate C into two separate clusters for SN and DMN may help uncover their associations with the psychometric measures and address this limitation. Finally, we did not use topographic ANOVA to determine the spatial differences between the group level microstates. The group level microstate maps in the EO condition of ASD neither resemble the EC condition of ASD nor the group level maps of TD. Given that the topographies are not visually similar, the underlying networks generating these patterns can be assumed to be different from the EC condition (Murray et al., 2008). It is necessary to further investigate this finding using methods such as source localization. This could potentially explain why we were not able to detect meaningful differences between the two groups in the EO condition.
In conclusion, the current study provided preliminary evidence for alterations in EEG microstate architecture in ASD as compared to TD children, providing an understanding of the potential underlying etiology of ASD behavior in terms of brain network dynamics. These findings are consistent with previous studies in ASD using fMRI and thus add to the literature on brain network dynamics.
Data Availability Statement
The raw data supporting the conclusions of this article will be made available by the authors, without undue reservation.
Ethics Statement
The studies involving human participants were reviewed and approved by Vanderbilt University Institutional Review Board. Written informed consent to participate in this study was provided by the participants’ legal guardian/next of kin.
Author Contributions
SNK and JB contributed to the conception of the study and critically revised the manuscript and provided crucial feedback. SNK and AW recruited participants and collected data. SNK performed the pre-processing of the data, microstate analysis, statistical tests, and wrote the draft of the manuscript. AK provided expertise on EEG analyses, pre-processing, and the functional interpretation of the results. JB provided expertise on autism spectrum disorders and the interpretation of results. All authors contributed to the article and approved the submitted version.
Conflict of Interest
The authors declare that the research was conducted in the absence of any commercial or financial relationships that could be construed as a potential conflict of interest.
Supplementary Material
The Supplementary Material for this article can be found online at: https://www.frontiersin.org/articles/10.3389/fnhum.2020.00288/full#supplementary-material
Footnotes
References
Abbott, A. E., Nair, A., Keown, C. L., Datko, M., Jahedi, A., Fishman, I., et al. (2016). Patterns of Atypical Functional Connectivity and Behavioral Links in Autism Differ Between Default, Salience, and Executive Networks. Cereb. Cortex 26, 4034–4045. doi: 10.1093/cercor/bhv191
Abrams, D. A., Lynch, C. J., Cheng, K. M., Phillips, J., Supekar, K., Ryali, S., et al. (2013). Underconnectivity between voice-selective cortex and reward circuitry in children with autism. Proc. Natl. Acad. Sci. 110, 12060–12065. doi: 10.1073/pnas.1302982110
Andrews-Hanna, J. R. (2012). The Brain’s Default Network and Its Adaptive Role in Internal Mentation. Neuroscientist 18, 251–270. doi: 10.1177/1073858411403316
Assaf, M., Jagannathan, K., Calhoun, V. D., Miller, L., Stevens, M. C., Sahl, R., et al. (2010). Abnormal functional connectivity of default mode sub-networks in autism spectrum disorder patients. NeuroImage 53, 247–256. doi: 10.1016/j.neuroimage.2010.05.067
Atluri, S., Wong, W., Blumberger, D. M., Daskalakis, Z. J., and Farzan, F. (2017). 533. Insights from EEG Microstate Analysis on the Pathophysiology of Depression and Mechanisms of Seizure Therapy. Biol. Psych. 81:S216. doi: 10.1016/j.biopsych.2017.02.1141
Barnett, L., and Seth, A. K. (2014). The MVGC multivariate Granger causality toolbox: A new approach to Granger-causal inference. J. Neurosci. Methods 223, 50–68. doi: 10.1016/j.jneumeth.2013.10.018
Bernas, A., Barendse, E. M., Aldenkamp, A. P., Backes, W. H., Hofman, P. A. M., Hendriks, M. P. H., et al. (2018). Brain resting-state networks in adolescents with high-functioning autism: Analysis of spatial connectivity and temporal neurodynamics. Brain Behav. 8:e00878. doi: 10.1002/brb3.878
Bodfish, J. W., Symons, F. J., and Lewis, M. H. (1999). The repetitive behavior scale. West. Carol. Cent. Res. Rep. 37(5), 855–66.
Bressler, S. L., and Seth, A. K. (2011). Wiener-Granger Causality: A well established methodology. NeuroImage 58, 323–329. doi: 10.1016/j.neuroimage.2010.02.059
Britz, J., Van De Ville, D., and Michel, C. M. (2010). BOLD correlates of EEG topography reveal rapid resting-state network dynamics. NeuroImage 52, 1162–1170. doi: 10.1016/j.neuroimage.2010.02.052
Brodbeck, V., Kuhn, A., von Wegner, F., Morzelewski, A., Tagliazucchi, E., Borisov, S., et al. (2012). EEG microstates of wakefulness and NREM sleep. NeuroImage 62, 2129–2139. doi: 10.1016/j.neuroimage.2012.05.060
Cerliani, L., Mennes, M., Thomas, R. M., Martino, A. D., Thioux, M., and Keysers, C. (2015). Increased Functional Connectivity Between Subcortical and Cortical Resting-State Networks in Autism Spectrum Disorder. JAMA Psych. 72, 767–777. doi: 10.1001/jamapsychiatry.2015.0101
Chen, H., Uddin, L. Q., Duan, X., Zheng, J., Long, Z., Zhang, Y., et al. (2017). Shared atypical default mode and salience network functional connectivity between autism and schizophrenia: Shared atypical FC in ASD and schizophrenia. Autism Res. 10, 1776–1786. doi: 10.1002/aur.1834
Chen, S., Xing, Y., and Kang, J. (2017). Latent and Abnormal Functional Connectivity Circuits in Autism Spectrum Disorder. Front. Neurosci 11:125. doi: 10.3389/fnins.2017.00125
Cherkassky, V. L., Kana, R. K., Keller, T. A., and Just, M. A. (2006). Functional connectivity in a baseline resting-state network in autism. Neuroreport 17, 1687–1690. doi: 10.1097/01.wnr.0000239956.45448.4c
Constantino, J., and Gruber, C. P. (2002). The social responsiveness scale. Los Angel. West. Psychol. Serv. 10(10), 1663–1671.
Custo, A., Van De Ville, D., Wells, W. M., Tomescu, M. I., Brunet, D., and Michel, C. M. (2017). Electroencephalographic Resting-State Networks: Source Localization of Microstates. Brain Connect. 7, 671–682. doi: 10.1089/brain.2016.0476
Damiani, S., Scalabrini, A., Gomez-Pilar, J., Brondino, N., and Northoff, G. (2018). Increased scale-free dynamics in salience network in adult high-functioning autism. NeuroImage Clin. 21:101634. doi: 10.1016/j.nicl.2018.101634
D’Croz-Baron, D. F., Baker, M., Michel, C. M., and Karp, T. (2019). EEG Microstates Analysis in Young Adults With Autism Spectrum Disorder During Resting-State. Front. Hum. Neurosci. 13:173. doi: 10.3389/fnhum.2019.00173
Di Martino, A., Kelly, C., Grzadzinski, R., Zuo, X.-N., Mennes, M., Mairena, M. A., et al. (2011). Aberrant Striatal Functional Connectivity in Children with Autism. Biol. Psych. 69, 847–856. doi: 10.1016/j.biopsych.2010.10.029
Dierks, T., Jelic, V., Julin, P., Maurer, K., Wahlund, L. O., Almkvist, O., et al. (1997). EEG-microstates in mild memory impairment and Alzheimer’s disease: possible association with disturbed information processing. J. Neural. Transm. 104, 483–495. doi: 10.1007/BF01277666
Drissi, N. M., Szakács, A., Witt, S. T., Wretman, A., Ulander, M., Ståhlbrandt, H., et al. (2016). Altered Brain Microstate Dynamics in Adolescents with Narcolepsy. Front. Hum. Neurosci. 10:369. doi: 10.3389/fnhum.2016.00369
Gotham, K., Pickles, A., and Lord, C. (2009). Standardizing ADOS Scores for a Measure of Severity in Autism Spectrum Disorders. J. Autism Dev. Disord. 39, 693–705. doi: 10.1007/s10803-008-0674673
Green, S. A., Hernandez, L., Bookheimer, S. Y., and Dapretto, M. (2016). Salience Network Connectivity in Autism Is Related to Brain and Behavioral Markers of Sensory Overresponsivity. J. Am. Acad. Child Adolesc. Psych. 55, 618–626.e. doi: 10.1016/j.jaac.2016.04.013
Grieder, M., Koenig, T., Kinoshita, T., Utsunomiya, K., Wahlund, L.-O., Dierks, T., et al. (2016). Discovering EEG resting state alterations of semantic dementia. Clin. Neurophysiol. 127, 2175–2181. doi: 10.1016/j.clinph.2016.01.025
Hughes, J. R. (2007). Autism: The first firm finding = underconnectivity? Epilepsy Behav. 11, 20–24. doi: 10.1016/j.yebeh.2007.03.010
Hull, J. V., Jacokes, Z. J., Torgerson, C. M., Irimia, A., and Van Horn, J. D. (2017). Resting-State Functional Connectivity in Autism Spectrum Disorders: A Review. Front. Psych. 7:205. doi: 10.3389/fpsyt.2016.00205
Jia, H., and Yu, D. (2019). Aberrant Intrinsic Brain Activity in Patients with Autism Spectrum Disorder: Insights from EEG Microstates. Brain Topogr. 32, 295–303. doi: 10.1007/s10548-018-0685680
Khanna, A., Pascual-Leone, A., Michel, C. M., and Farzan, F. (2015). Microstates in resting-state EEG: Current status and future directions. Neurosci. Biobehav. Rev. 49, 105–113. doi: 10.1016/j.neubiorev.2014.12.010
Kikuchi, M., Koenig, T., Wada, Y., Higashima, M., Koshino, Y., Strik, W., et al. (2007). Native EEG and treatment effects in neuroleptic-naïve schizophrenic patients: Time and frequency domain approaches. Schizophr. Res. 97, 163–172. doi: 10.1016/j.schres.2007.07.012
Kindler, J., Hubl, D., Strik, W. K., Dierks, T., and Koenig, T. (2011). Resting-state EEG in schizophrenia: Auditory verbal hallucinations are related to shortening of specific microstates. Clin. Neurophysiol. 122, 1179–1182. doi: 10.1016/j.clinph.2010.10.042
King, J. B., Prigge, M. B. D., King, C. K., Morgan, J., Dean, D. C., Freeman, A., et al. (2018). Evaluation of Differences in Temporal Synchrony Between Brain Regions in Individuals With Autism and Typical Development. JAMA Netw. Open 1:e184777. doi: 10.1001/jamanetworkopen.2018.4777
Koenig, T., Prichep, L., Lehmann, D., Sosa, P. V., Braeker, E., Kleinlogel, H., et al. (2002). Millisecond by Millisecond, Year by Year: Normative EEG Microstates and Developmental Stages. NeuroImage 16, 41–48. doi: 10.1006/nimg.2002.1070
Lehmann, D., Faber, P. L., Galderisi, S., Herrmann, W. M., Kinoshita, T., Koukkou, M., et al. (2005). EEG microstate duration and syntax in acute, medication-naïve, first-episode schizophrenia: a multi-center study. Psych. Res. Neuroimaging 138, 141–156. doi: 10.1016/j.pscychresns.2004.05.007
Lord, C., Rutter, M., DiLavore, P. C., Risi, S., Gotham, K., and Bishop, S. L. (2012). Autism diagnostic observation schedule, (ADOS-2) modules 1-4. Los Angel. Calif. West. Psychol. Serv. 44(8), 1996–2012.
Marx, E., Deutschländer, A., Stephan, T., Dieterich, M., Wiesmann, M., and Brandt, T. (2004). Eyes open and eyes closed as rest conditions: impact on brain activation patterns. NeuroImage 21, 1818–1824. doi: 10.1016/j.neuroimage.2003.12.026
Menon, V., and Uddin, L. Q. (2010). Saliency, switching, attention and control: a network model of insula function. Brain Struct. Funct. 214, 655–667. doi: 10.1007/s00429-010-0262260
Merrin, E. L., Meek, P., Floyd, T. C., and Callaway, E. (1990). Topographic segmentation of waking EEG in medication-free schizophrenic patients. Int. J. Psychophysiol. Off. J. Int. Organ. Psychophysiol. 9, 231–236. doi: 10.1016/0167-8760(90)90055-i
Michel, C. M., and Koenig, T. (2018). EEG microstates as a tool for studying the temporal dynamics of whole-brain neuronal networks: A review. NeuroImage 180, 577–593. doi: 10.1016/j.neuroimage.2017.11.062
Milz, P., Faber, P. L., Lehmann, D., Koenig, T., Kochi, K., and Pascual-Marqui, R. D. (2016). The functional significance of EEG microstates—Associations with modalities of thinking. NeuroImage 125, 643–656. doi: 10.1016/j.neuroimage.2015.08.023
Murray, M. M., Brunet, D., and Michel, C. M. (2008). Topographic ERP Analyses: A Step-by-Step Tutorial Review. Brain Topogr. 20, 249–264. doi: 10.1007/s10548-008-005455
Nishida, K., Morishima, Y., Yoshimura, M., Isotani, T., Irisawa, S., Jann, K., et al. (2013). EEG microstates associated with salience and frontoparietal networks in frontotemporal dementia, schizophrenia and Alzheimer’s disease. Clin. Neurophysiol. 124, 1106–1114. doi: 10.1016/j.clinph.2013.01.005
Noel, J.-P., Lytle, M., Cascio, C., and Wallace, M. T. (2018). Disrupted integration of exteroceptive and interoceptive signaling in autism spectrum disorder: Cardiovisual temporal binding window in ASD. Autism Res. 11, 194–205. doi: 10.1002/aur.1880
Nomi, J. S., and Uddin, L. Q. (2015). Developmental changes in large-scale network connectivity in autism. NeuroImage Clin. 7, 732–741. doi: 10.1016/j.nicl.2015.02.024
Perrin, F., Pernier, J., Bertrand, O., and Echallier, J. F. (1989). Spherical splines for scalp potential and current density mapping. Electroencephalogr. Clin. Neurophysiol. 72, 184–187. doi: 10.1016/0013-4694(89)9018090186
Rashid, B., Blanken, L. M. E., Muetzel, R. L., Miller, R., Damaraju, E., Arbabshirani, M. R., et al. (2018). Connectivity dynamics in typical development and its relationship to autistic traits and autism spectrum disorder. Hum. Brain Mapp. 39, 3127–3142. doi: 10.1002/hbm.24064
Roid, G. H. (2003). Stanford-Binet Intelligence Scale. Fifth Edition. Itasca, IL: Riverside Publishing.
Rutter, M., Bailey, A., and Lord, C. (2003). Social Communication Questionnaire. Los Angeles, CA: Western Psychological Services.
Schauder, K. B., Mash, L. E., Bryant, L. K., and Cascio, C. J. (2015). Interoceptive ability and body awareness in autism spectrum disorder. J. Exp. Child Psychol. 131, 193–200. doi: 10.1016/j.jecp.2014.11.002
Seitzman, B. A., Abell, M., Bartley, S. C., Erickson, M. A., Bolbecker, A. R., and Hetrick, W. P. (2017). Cognitive manipulation of brain electric microstates. NeuroImage 146, 533–543. doi: 10.1016/j.neuroimage.2016.10.002
Skrandies, W. (1990). Global field power and topographic similarity. Brain Topogr. 3, 137–141. doi: 10.1007/BF01128870
Stevens, A., Lutzenberger, W., Bartels, D. M., Strik, W., and Lindner, K. (1997). Increased duration and altered topography of EEG microstates during cognitive tasks in chronic schizophrenia. Psychiatry Res. 66, 45–57. doi: 10.1016/S0165-1781(96)029382931
Strelets, V., Faber, P. L., Golikova, J., Novototsky-Vlasov, V., Koenig, T., Gianotti, L. R. R., et al. (2003). Chronic schizophrenics with positive symptomatology have shortened EEG microstate durations. Clin. Neurophysiol. 114, 2043–2051. doi: 10.1016/S1388-2457(03)00211216
Strik, W. K., Chiaramonti, R., Muscas, G. C., Paganini, M., Mueller, T. J., Fallgatter, A. J., et al. (1997). Decreased EEG microstate duration and anteriorisation of the brain electrical fields in mild and moderate dementia of the Alzheimer type. Psych. Res. 75, 183–191. doi: 10.1016/s0925-4927(97)00054-1
Strik, W. K., and Lehmann, D. (1993). Data-determined window size and space-oriented segmentation of spontaneous EEG map series. Electroencephalogr. Clin. Neurophysiol. 87, 169–174. doi: 10.1016/0013-4694(93)90016-O
Supekar, K., Uddin, L. Q., Khouzam, A., Phillips, J., Gaillard, W. D., Kenworthy, L. E., et al. (2013). Brain Hyperconnectivity in Children with Autism and its Links to Social Deficits. Cell Rep. 5, 738–747. doi: 10.1016/j.celrep.2013.10.001
Sverak, T., Albrechtova, L., Lamos, M., Rektorova, I., and Ustohal, L. (2017). Intensive repetitive transcranial magnetic stimulation changes EEG microstates in schizophrenia: A pilot study. Schizophr. Res 193, 451–452. doi: 10.1016/j.schres.2017.06.044
Taylor, K. S., Seminowicz, D. A., and Davis, K. D. (2009). Two systems of resting state connectivity between the insula and cingulate cortex. Hum. Brain Mapp. 30, 2731–2745. doi: 10.1002/hbm.20705
Tomasi, D., and Volkow, N. D. (2019). Reduced Local and Increased Long-Range Functional Connectivity of the Thalamus in Autism Spectrum Disorder. Cereb. Cortex 29, 573–585. doi: 10.1093/cercor/bhx340
Tomescu, M. I., Rihs, T. A., Becker, R., Britz, J., Custo, A., Grouiller, F., et al. (2014). Deviant dynamics of EEG resting state pattern in 22q11.2 deletion syndrome adolescents: A vulnerability marker of schizophrenia?. Schizophr. Res. 157, 175–181. doi: 10.1016/j.schres.2014.05.036
Tyszka, J. M., Kennedy, D. P., Paul, L. K., and Adolphs, R. (2014). Largely Typical Patterns of Resting-State Functional Connectivity in High-Functioning Adults with Autism. Cereb. Cortex 24, 1894–1905. doi: 10.1093/cercor/bht040
Uddin, L. Q., Supekar, K., Lynch, C. J., Khouzam, A., Phillips, J., Feinstein, C., et al. (2013). Salience Network-Based Classification and Prediction of Symptom Severity in Children With Autism. JAMA Psych. 70, 869–879. doi: 10.1001/jamapsychiatry.2013.104
Wackermann, J., Lehmann, D., Michel, C. M., and Strik, W. K. (1993). Adaptive segmentation of spontaneous EEG map series into spatially defined microstates. Int. J. Psychophysiol. Off. J. Int. Organ. Psychophysiol. 14, 269–283. doi: 10.1016/0167-8760(93)90041-m
Watanabe, T., and Rees, G. (2017). Brain network dynamics in high-functioning individuals with autism. Nat. Commun. 8:16048. doi: 10.1038/ncomms16048
Xu, P., Huang, R., Wang, J., Van Dam, N. T., Xie, T., Dong, Z., et al. (2014). Different topological organization of human brain functional networks with eyes open versus eyes closed. NeuroImage 90, 246–255. doi: 10.1016/j.neuroimage.2013.12.060
Yuan, H., Ding, L., Zhu, M., Zotev, V., Phillips, R., and Bodurka, J. (2016). Reconstructing Large-Scale Brain Resting-State Networks from High-Resolution EEG: Spatial and Temporal Comparisons with fMRI. Brain Connect. 6, 122–135. doi: 10.1089/brain.2014.0336
Keywords: EEG, microstates, resting-state, autism spectrum disorders, salience network
Citation: Nagabhushan Kalburgi S, Whitten AP, Key AP and Bodfish JW (2020) Children With Autism Produce a Unique Pattern of EEG Microstates During an Eyes Closed Resting-State Condition. Front. Hum. Neurosci. 14:288. doi: 10.3389/fnhum.2020.00288
Received: 04 May 2020; Accepted: 26 June 2020;
Published: 08 October 2020.
Edited by:
Teppei Matsui, The University of Tokyo, JapanReviewed by:
Giovanni Assenza, Campus Bio-Medico University, ItalyJinping Xu, Chinese Academy of Sciences, China
Copyright © 2020 Nagabhushan Kalburgi, Whitten, Key and Bodfish. This is an open-access article distributed under the terms of the Creative Commons Attribution License (CC BY). The use, distribution or reproduction in other forums is permitted, provided the original author(s) and the copyright owner(s) are credited and that the original publication in this journal is cited, in accordance with accepted academic practice. No use, distribution or reproduction is permitted which does not comply with these terms.
*Correspondence: Sahana Nagabhushan Kalburgi, c25hZ2FiaHVzaGFua2FsYnVyZ2lAY2hsYS51c2MuZWR1
†Present address: Sahana Nagabhushan Kalburgi, Children’s Hospital Los Angeles, Los Angeles, CA, United States