- 1Department of Chemistry and Biochemistry, Texas Tech University, Lubbock, TX, United States
- 2Program for Neurotrauma, Neuroproteomics and Biomarkers Research, Department of Emergency Medicine, University of Florida, Gainesville, FL, United States
- 3Department of Biomedical and Dental Sciences and Morphofunctional Imaging, University of Messina, Messina, Italy
Neurological diseases affect millions of peopleochemistryorldwide and are continuously increasing due to the globe’s aging population. Such diseases affect the nervous system and are characterized by a progressive decline in brain function and progressive cognitive impairment, decreasing the quality of life for those with the disease as well as for their families and loved ones. The increased burden of nervous system diseases demands a deeper insight into the biomolecular mechanisms at work during disease development in order to improve clinical diagnosis and drug design. Recently, evidence has related glycosylation to nervous system diseases. Glycosylation is a vital post-translational modification that mediates many biological functions, and aberrant glycosylation has been associated with a variety of diseases. Thus, the investigation of glycosylation in neurological diseases could provide novel biomarkers and information for disease pathology. During the last decades, many techniques have been developed for facilitation of reliable and efficient glycomic analysis. Among these, mass spectrometry (MS) is considered the most powerful tool for glycan analysis due to its high resolution, high sensitivity, and the ability to acquire adequate structural information for glycan identification. Along with MS, a variety of approaches and strategies are employed to enhance the MS-based identification and quantitation of glycans in neurological samples. Here, we review the advanced glycomic tools used in nervous system disease studies, including separation techniques prior to MS, fragmentation techniques in MS, and corresponding strategies. The glycan markers in common clinical nervous system diseases discovered by utilizing such MS-based glycomic tools are also summarized and discussed.
Impact of neurological diseases and urgent need for clinical markers
At present, more than 47 million people are suffering from dementia, and this number is expected to exceed 130 million by 2050 as the world’s population ages (Alzheimers Association, 2020). Increased longevity, the result of modern medical and public health advances, dramatically increases the risk of neurodegenerative diseases. Such diseases, affecting the nervous system and characterized by a progressive decline in brain function and progressive cognitive impairment, decrease the quality of life for those afflicted as well as their families and loved ones (Super et al., 2019). In fact, the great majority of these patients are cared for by family members who assist in most, if not all, facets of daily living activities (Nikzad-Terhune et al., 2010). Caregiving for dementia patients has also been shown to negatively affect both the physical health and psychological wellbeing of those providing care. A meta-analysis conducted in 2003 revealed that dementia caregivers have elevated levels of stress hormones which put them at greater risk for developing cardiovascular diseases, hypertension, and type II diabetes (Vitaliano et al., 2003). Moreover, spouses caring for dementia patients have an elevated risk of receiving antidepressant treatment, due to the increased probability of depression affecting them compared to non-caregivers (Richardson et al., 2013). The factors that may influence caregiver burden include the severity of the patient’s dementia, the behavioral disturbances of the patient, and the levels of neuropsychiatric symptoms such as delusions and hallucinations (Etters et al., 2008).
Glycosylation
General concepts and association with diseases
Glycans, which are mostly found on the outermost surfaces of cellular and secreted macromolecules, are widely distributed in nature and are highly diverse in both prokaryotes and eukaryotes (Kristic and Lauc, 2017). The importance of glycans is not just restricted to the structural features they convey; in fact, they display regulatory activity and play an important role in a variety of functions in both physiological and pathophysiological states (Varki, 2017). In this section, we discuss the main types of glycosylation, the role that glycans play in some diseases, and the possibility to use them as biomarkers of pathogenesis.
Many of our system’s proteins are modified by the addition, through β-1N linkage, of an N-acetylglucosamine (GlcNAc) to an Asn side chain, a process known as N-glycosylation. This attachment allows for additional modifications such as galactosylation, GlcNAcylation, sialylation, and fucosylation; depending on the nature of the latter modification, the final obtained structure is a high mannose N-glycan, a hybrid N-glycan, or a complex N-glycan (Richard et al., 1983). The synthesis of N-glycans begins in the endoplasmic reticulum where the transfer of the ligand-glycan precursor GlcNAc to Asp is achieved through oligosaccharyltransferase (OST) activity. Then, after sequential removal of the glucose residues and the Man residue by α-glycosidases (α-Glc I-II) and α-mannosidase (ER α-Man), respectively, the glycoprotein will be further modified through trimming by specific mannosidases and/or additional glycan modifications occurring in the Golgi apparatus (Parodi, 2000).
O-glycosylation usually occurs on oxygen atoms of Ser and Thr, amino acids characterized by the presence of functional hydroxyl groups in their structure (Ten Hagen et al., 2001). Classification of the end O-glycans formed depends on the initial sugar that was attached to the protein and on the other sugar structures which are subsequently added; the addition of N-acetyl-galactosamine on Ser or Thr, known as the mucin-type linkage, is the most widespread type of O-glycosylation (Carraway and Hull, 1991). In fact, glycosyltransferases will expand the existing structure that was formed in the endoplasmic reticulum with galactose (Lis and Sharon, 1993), GlcNAc, sialic acid, and fucose (Harris et al., 1991) in the Golgi apparatus. This is similar to the N-glycosylation process, with one important distinction: O-glycans do not undergo trimming like N-glycans.
N-glycosylation and O-glycosylation are not the only two types of glycosylation. The addition of glycosylphosphatidylinositol (GPI) anchors at the carboxyl terminus of some proteins, a process termed glypiation, is omnipresent in eukaryotic organisms (Ferguson, 1992). This type of glycosylation, performed by a transamidase found in the endoplasmic reticulum, has been found to play a role in transmembrane signaling and folate uptake (Ferguson, 1991). However, additional studies are still required to investigate glypiation and C-mannosylation, another lesser-targeted glycosylation, and the effects that such types of glycosylation have on physiological processes.
Research over the years has revealed that genetic defects in glycosylation lead to embryonic lethality, exposing the vital role of glycans, with the identification of more than a hundred congenital disorders of glycosylation (CDGs) resulting from glycogenes’ deficiencies (Haeuptle and Hennet, 2009). Because glycans play an essential role in muscular, developmental, and neurological processes, CDGs are typically severe in their manifestations. Hence, studying glycans as biomarkers of certain diseases seems like a promising tool that has interested many scientists in the field.
The most common form of CDG is the N-glycan-related PMM2-CDG, with phosphomanomutase 2 (PMM2) being responsible for the conversion of Man-6-P to Man-1-P. Because Man-1-P is essential for the production of the precursor of an N-glycan, this CDG presents with a neurological or multi-system phenotype depending on the type of mutation affecting the gene (Witters et al., 2019). O-glycan-related CDGs have been associated with the development of congenital muscular dystrophies as a result of abnormal Man O-glycosylation of the alpha-dystroglycan protein. The six genes that were identified to cause muscular dystrophy (POMT1, POMT2, POMGnT1, FCMD, FKRP, and LARGE) are all associated with an under-glycosylation of α-dystroglycan (Martin, 2007). Also, the overexpression of the LARGE gene increases dystroglycan glycosylation and allows the restoration of its function (Barresi et al., 2004). This confirms the important role that O-glycosylation plays in avoiding the development of such a disease.
The successful story of glyco-biomarkers in other diseases
Because glycosylation is greatly affected by inflammation and diseases such as cancer and diabetes, the use of glycans as biomarkers in the study of diseases is a promising tool. The synthesis of glycans has been shown to be more affected by disease states than protein synthesis, and quantifying oligosaccharide expression is easier than investigating protein expression, which supports the use of glycans as biomarkers.
Glycosylation biomarkers are already in use in the cancer field as a means to identify cells with stem-cell-like phenotypes. In fact, abnormal core fucosylation and increased N-glycan branching are two common features that are observed in cancer cells (Pinho and Reis, 2015). Also, elevated α-2,6-linked sialic acid in N-glycans seems to be a characteristic of pancreatic and colon cancers and is used as a glycosylation-related biomarker (Wilson and Massey, 1987).
Moreover, alterations in the glycosylation of IgG accompanied by an elevated amount of bisecting GlcNAc and core fucose on the antigen-binding fragment (Fab)-associated glycans seem to be a critical component of rheumatoid arthritis pathogenesis (Youings et al., 1996). Abnormal O-linked N-acetylglucosamine-mediated signaling and enhanced glycation of multiple proteins such as AKT (Liu et al., 2000) seem to be glycosylation-biomarkers of diabetes mellitus.
Many proteomics studies have been conducted to identify biomarkers of neurodegenerative disorders (Zhang et al., 2005; Shi et al., 2009, 2015; Vanguilder and Freeman, 2011), yet few reports are to be found on the CSF N-glycoproteome study. Considering that many neurodegenerative disorders are associated with alterations in glycosylation patterns (Hwang et al., 2010), and taking into account the success that glycosylation-based biomarker discovery has achieved in other fields (and especially in cancer research), discovering glycan markers for dementia-related diseases seems promising for the diagnosis, prognosis, and even treatment of such burdensome diseases (Chen et al., 2010a; Ahmed et al., 2014). Some of the main findings from diverse in vitro and in vivo studies revealing the important role that glycosylation plays in the development of neurodegenerative disorders are summarized in Table 1.
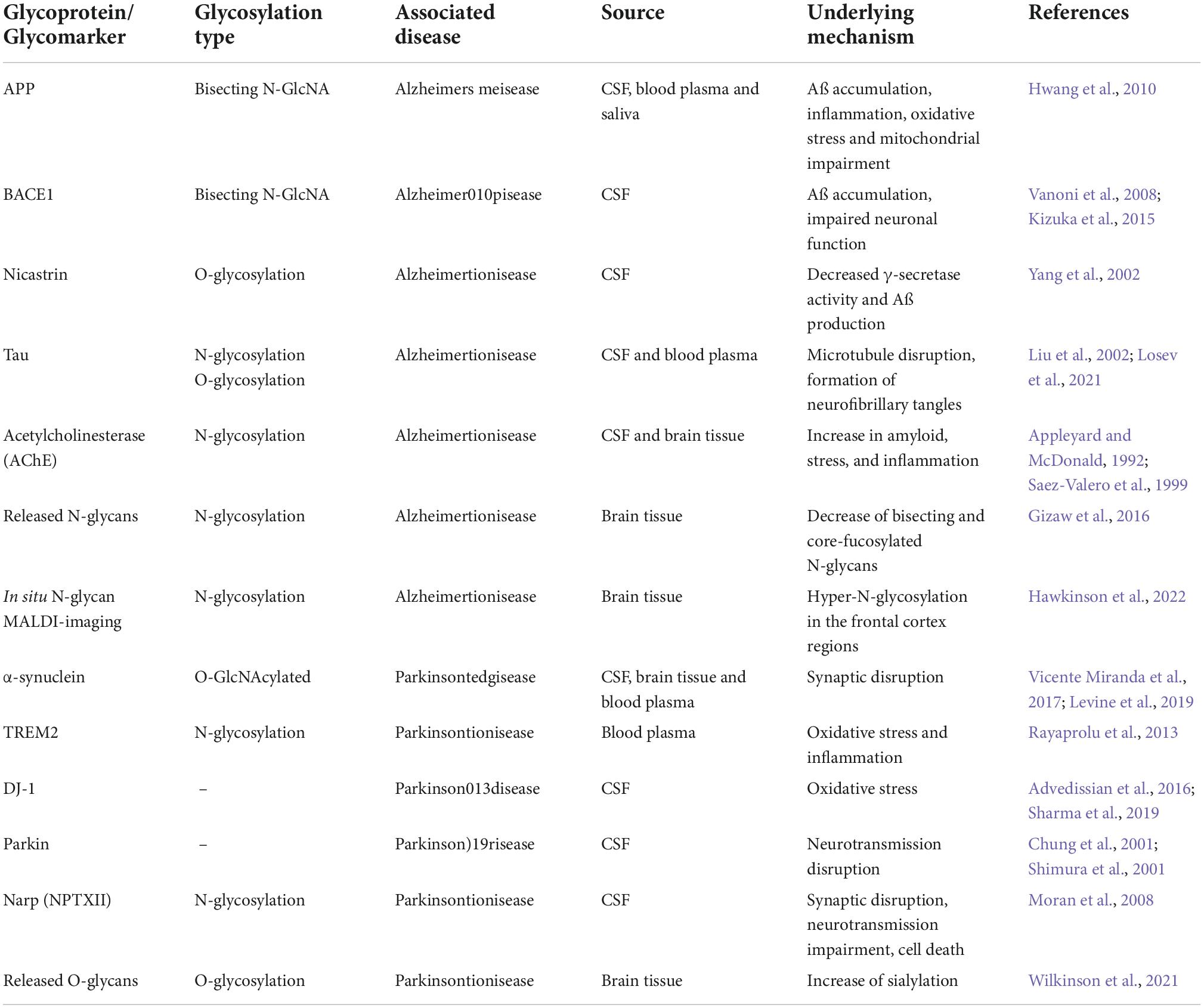
Table 1. Summary of glycoproteins, corresponding pathologies resulting from glycosylation modifications, and their suggested underlying mechanisms.
Mass spectrometry-based separation techniques facilitate glycomics studies in neurological diseases
MS has become a common tool in glycomics analysis due to its high resolution and ability to acquire rich structural information. However, MS alone sometimes faces sensitivity issues due to the competitive ionization that hinders the characterization of low abundant glycans. Therefore, numerous separation techniques have been widely coupled to MS to reveal glycan microheterogeneity and biological functions. Of these techniques, the most common include pre-ionization separations such as reverse phase chromatography (RPLC), hydrophilic interaction chromatography (HILIC), and capillary electrophoresis (CE), as well as post-ionization separations such as ion mobility (IM). In pre-ionization separation techniques, any ionization competition is addressed since glycans are separated and ionized separately; in post-ionization separation techniques, however, this issue still requires consideration. Although separation techniques offer advantages in glycomics, MS-based strategies such as matrix assisted laser desorption/ionization (MALDI) are still common for clinical glycomics analysis because of the simple sample preparation and high throughput.
Matrix assisted laser desorption/ionization-mass spectrometry in neurological glycomics
MALDI-MS is one of the most common ways to study glycomic alterations in biological samples (Harvey, 1999), and improving sensitivity is of great importance in MALDI-MS. During the last few decades, several MALDI matrices have been developed to achieve an efficient glycomic analysis (Stahl et al., 1991, 1994; Karas et al., 1993; Metzger et al., 1994; Mohr et al., 1995; Mechref and Novotny, 1998; Jiao et al., 2015), including dihydroxybenzoic acid (DHB) (Jensen et al., 2013; Inyoung et al., 2016; Chen et al., 2020), and nanomaterials such as glutathione-capped iron oxide and carbon nanoparticles that have recently been used as a co-matrix to enhance sensitivity (Liang et al., 2014; Banazadeh et al., 2018).
Matrix assisted laser desorption/ionization-mass spectrometry
MALDI is one of the soft ionization techniques that enable less in-source fragmentation for an efficient structural elucidation. MALDI has successfully profiled glycans in many diseases such as liver cancer (Kamiyama et al., 2013; Kim et al., 2015), breast cancer (Kyselova et al., 2008; de Leoz et al., 2011), ovarian cancer (Biskup et al., 2013, 2017), colorectal cancer (Kaprio et al., 2015; Liu et al., 2018), and more (Qin et al., 2017; Wang et al., 2018). Time of flight (TOF) MS is often coupled to MALDI for its fast scan speed and theoretically unlimited m/z range, which together enhance the efficiency in analysis of large biological cohorts (Everest-Dass et al., 2018). However, the sensitivity remains a challenge even with the introduction of a different matrix and co-matrix. Therefore, derivatization strategies, such as Girard’s reagent P/T (Kim et al., 2015; Zhang et al., 2019, 2020a) and permethylation (Kang et al., 2005; Shubhakar et al., 2016), have been used to improve the ionization efficiency. In addition, sialic acid derivatization methods, such as amidation (de Haan et al., 2015), esterification (Gomes de Oliveira et al., 2015; Powers et al., 2015), and alkylamidation (Nishikaze et al., 2017; Hanamatsu et al., 2018; Suzuki et al., 2019), were used to distinguish sialic acid linkage isomers and stabilize labile sialic acid structures. Among these, sialic acid linkage specific alkylamidation (SALSA) is the newest efficient and stable linkage specific derivatization method (Nishikaze et al., 2017). The following methods were mainly modifications based on SALSA. With the demonstration of these MALDI-MS strategies in many diseases, MALDI-MS has also been widely applied in glycomic analysis of central system diseases.
Early in 2013, MALDI-TOF/TOF (Applied Biosystems 4800) was applied to profile the permethylated glycans derived from congenital disorders of glycosylation (CDGs) patient sera and proved to be able to differentiate CDG from controls (Xia et al., 2013). Recently, Duvet et al. (2018) investigated the serum N-glycome expressions in MAN1B1-CDG patients using the same MAIDI-TOF/TOF system in combination with an Endo-β-N-acetylglucosaminidase H (Endo H) digestion. Since the Endo H can only release high-mannose and hybrid structures, a straightforward investigation of MAN1B1 deficit was achieved. The MALDI-MS successfully identified the accumulation of several hybrid structures and alteration of high-mannose structures in MAN1B1-CDG patients.
Gizaw et al. (2016) used a glycoblotting method (BlotGlyco® H beads, Sumitomo Bakelite, Tokyo, Japan) to enrich released N-glycans followed by an on-beads methyl esterification to investigate the glycan expression changes in AD patients’ brain tissue using MALDI-TOF-MS. This analytical method allowed the identification of 72 N-glycan compositions from brain tissues of AD patients. The decrease of bisecting and core-fucosylated N-glycans in the frontal cortex of AD patients was observed, which could be explained by the downregulation of glycosyltransferases GnTIII and FUT8 in the brain. This method was also demonstrated on AD serum and CSF N-glycomics.
Recently, Palmigiano et al. (2018) introduced a MALDI-TOF/TOF (Applied Biosystems 4800) based method for the analysis of permethylated glycans in Alzheimer’s Disease (AD), which allowed the identification of 90 N-glycan structures. Later, permethylated glycan profiling using a 4800 MALDI-TOF/TOF mass spectrometer (PerSeptive Biosystems) revealed an increase in the levels of bisecting Lewis × epitopes in certain glycans (Schedin-Weiss et al., 2020). Besides CDG and AD, MALDI-TOF-MS was also employed in glycomics analysis of other neurological diseases such as amyotrophic lateral sclerosis (ALS) (Edri-Brami et al., 2012; Costa et al., 2019) and Huntington’s Disease (HD) (Gizaw et al., 2015).
Although MALDI-MS has been applied in many neurological glycomics studies, the technique’s sensitivity was limited. Compared to other methods, there were usually fewer glycan structures identified in MALDI-MS (Huffman et al., 2014). However, because of the high throughput and simple sample preparation, it is promising to couple MALDI-MS with in situ glycomics techniques such as mass spectrometry imaging (MSI).
Matrix assisted laser desorption/ionization imaging
In the last decade, a major application of MALDI-MS has been MALDI-MSI, which can visualize the in situ distributions of different glycans on a tissue slide. The key factors in MALDI-MSI are the raster-scan resolution and the MS resolution and accuracy. The scan resolution determines the spot (pixel) number measured by MS (with a spatial resolution down to 5–20 μm), while the MS resolution and accuracy determines the molecules that can be analyzed (Aichler and Walch, 2015). It enables a label-free in situ characterization of hundreds of glycans on a single tissue section (Hasan et al., 2021). The most common MS techniques employed in MALDI-MSI are FTICR for high-resolution analysis or TOF for rapid and high mass range analysis. MALDI-MSI has been used to investigate glycans from neurological tissue samples for years.
The MALDI-MSI analysis of N-glycans on a brain tissue was first introduced by Powers et al. (2013). The typical in situ N-glycomic imaging protocol has also been summarized (Drake et al., 2018; Stanback et al., 2021). Toghi Eshghi et al. (2014) utilized this strategy to analyze the N-glycan distributions in a glioblastoma tumor transplanted mouse brain using a MALDI-QIT-TOF MS (AXIMA Resonance, Shimadzu). In this study, a 100 μm imaging resolution was employed, resulting in identification of 42 N-glycans. The different distributions of several glycans in tumor vs. normal brain tissues were identified. In another study, Malaker et al. (2022) employed a RapiFlex MALDI-TOF MS (Bruker) together with a MALDI LTQ orbitrap XL (Thermo Fisher Scientific) to map the N-glycan distribution of canine glioma samples, and identified the increase of a bi-sialylated bi-antennary structure in canine glioma.
Most recently, Rebelo et al. (2021) investigated the glycosylation changes during neuroinflammation in the rodent brain. The rat striatum was injected by lipopolysaccharide (LPS) to establish the striatal neuroinflammation model. The N-glycan imaging was performed using a MALDI timsTOF fleX trapped ion mobility QTOF MS (Bruker) with a laser spot of 20 μm and a raster width of 40 μm between spots. Overall, 52 N-glycans were detected by MALDI-MSI. Some significant glycan expression changes were observed in the injected site that were directly related to the inflammatory reaction, as shown in Figure 1. These significant N-glycans were mainly highlighted by the increase of high-mannose, bisecting, and α-galactosylated glycans in the lesion core. Meanwhile, some fucosylated and sialylated structures were observed to have decreased in the injected side. Later, Hawkinson et al. (2022) spatially investigated N-glycan heterogeneity in both mouse and human AD brain samples and corresponding age-related controls using an optimized in situ MALDI-MSI workflow. The imaging was acquired using a Waters SynaptG2-Xs MS coupled to traveling wave ion mobility. The spatially heterogeneous N-glycans were identified as a potential AD clinical hallmark, and the hyperglycosylation as a phenotype of AD mouse models and human frontal cortex specimens were discovered. These studies demonstrate the potential for glyco-marker discovery with MALDI-MSI. However, the majority of the studies were on animal models or limited biomedical samples. An increased biological cohort should be considered in future works.
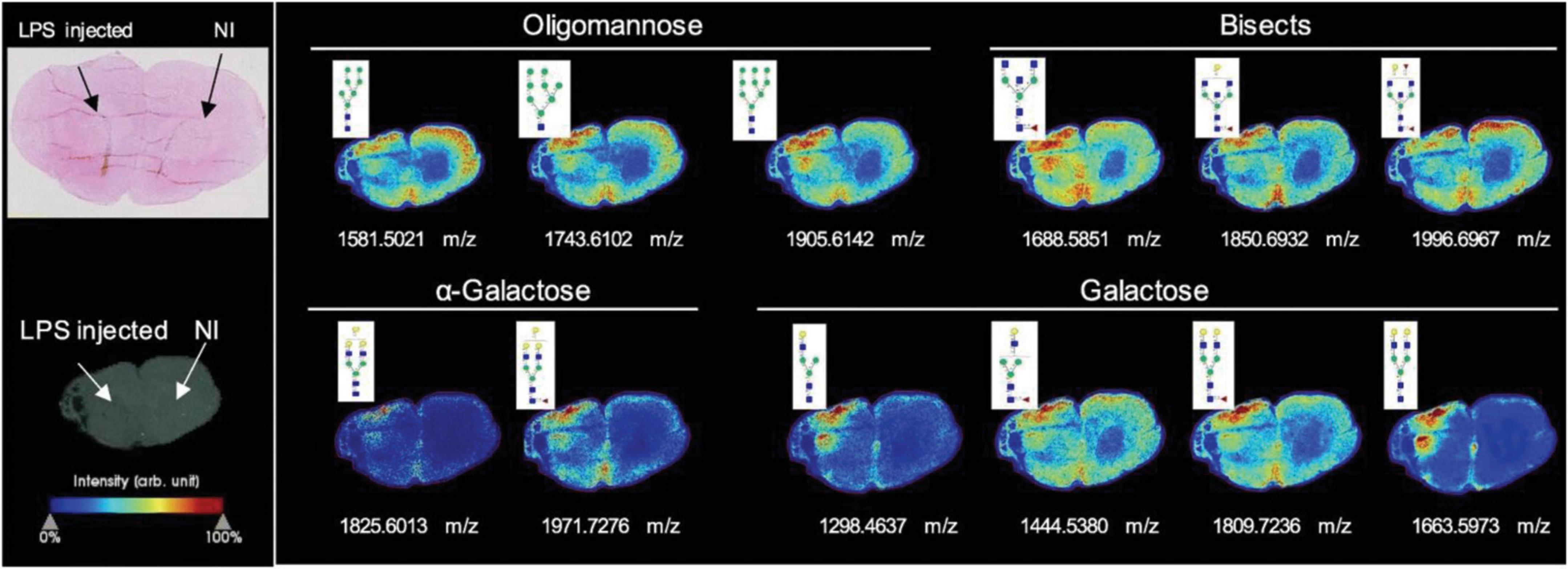
Figure 1. N-glycan imaging of the brain of LPS-injected rats. Main N-glycan structures that are visualized in the lesion core belong to specific groups: oligomannose, bisected structures, and galactosylated glycans, mainly with α-galactose. Each image is accompanied by the putative structures determined by combinations of accurate m/z, CID fragmentation patterns, and glycan database structure. NI stands for none injected area. Reprinted with the permission from Rebelo et al. (2021).
A new laser-induced postionization technique (MALDI-2) has been introduced to MALDI-MSI to enhance the sensitivity through a wavelength-tunable postionization laser in the gas phase of ionized plume (Soltwisch et al., 2015). This technique initializes a secondary MALDI-like ionization process to increase the signal intensity and was successfully utilized on brain tissue glycome imaging, which enhanced the sensitivity by 10 times relative to the current standard method (Heijs et al., 2020). Although MALDI-2 imaging has not yet been used in glycomics analysis of nervous system diseases, the greater sensitivity makes it a promising alternative in future studies.
Although quantitative MSI (qMSI) of metabolites and peptides have been illustrated as a result of significant advances in MSI (Tobias and Hummon, 2020), qMSI of glycans is still a challenging analytical task. Generally, qMSI has been difficult to attain due to matrix crystallization heterogeneity, tissue heterogeneity, insufficient analyte extraction, and ion suppression effect. Ellis et al. (2014), Rzagalinski and Volmer (2017), and more recently Tobias and Hummon (2020) have highlighted the challenges of qMSI for drugs, lipids and peptides. qMSI of glycan is achieved by attending to the same sources of variability that are commonly associated with MALDI-MS measurement, including ion suppression, sample heterogeneity, spot-to-spot variability, sample preparation, matrix choice, matrix application and analyte recovery.
Like any analytical measurement, qMSI requires multiple replicates. Multiple tissue sections should be mounted on the same target plate to allow multiple measurements of the same biological or chemical conditions. Replicate analyses are critical to minimize any potential measurements variabilities originating from sample treatment or matrix deposition. Triplicates are also required to construct calibration curves. Biological replicates should be also considered when constructing calibration curves. When possible, a minimum of three biological replicates should be considered to ensure that the observed variation is due to the condition under investigation and not because of random variation (Tobias and Hummon, 2020).
Analytical sensitivity in qMSI is directly dependent on sample preparation and correct choice of matrix. Sample preparation such as washing is critical to ensure the removal of any materials that could interfere with the proper crystallization of the matrix or the suppression of the MS signal of the analytes. Several studies have detailed sample preparation for the MSI of proteins (Groseclose et al., 2007; Seeley and Caprioli, 2008; Heijs et al., 2016; Zubair et al., 2016) and gangliosides (Angel et al., 2012; Yang et al., 2019). The choice of matrix in qMSI is driven by sensitivity, MS-mode of analysis and suitability of the matrix for the lengthy time of analysis commonly associate with MSI. Also, the use of robotic sprayer is highly recommended to ensure uniform application of matrix and internal standards (needed for absolute quantification). Thus far, no studies have addressed sample preparation needed for MSI of glycans; however, several of the steps discussed for the sample preparation of biomolecules are applicable to glycan MSI. Glycan MSI has been mainly conducted using α-cyano-4-hydroxycinnamic acid (CHCA) as a matrix (Shi et al., 2019).
Defining a quality control (QC) sample is an excellent mean to address sources of variability in MSI; however, identifying such a QC sample might not be an easy task especially for qMSI glycomics. Recently, the mean signal intensities of peptides associated with egg white section as a QC sample has been used to monitor the performance of a large scale formalin-fixed paraffin embedded formalin-fixed paraffin embedded (FFPE) MALDI-MSI study using a TOF/TOF instrument (Condina et al., 2019). Also, mimetic tissue model spiked with internal standard has been used to monitor the performance of qMSI in multicenter study (Barry et al., 2019).
The same guidelines considered for absolute quantification in LC-MS and GC-MS are applied to qMSI of peptides and metabolites (Tobias and Hummon, 2020). Several calibrants should be considered when constructing the calibration curve. The calibration points should be evenly distributed in the linear working range of the curve. The signal should be averaged over the region of interest (ROI). The signals of the different adducts formed should be plotted against the applied concentration (Tobias and Hummon, 2020). The signal of the analyte generated from the real samples can be then determined using the constructed calibration curve. Although no studies thus far have highlighted absolute quantitation of glycan through qMSI, the steps summarized up for other molecules can be utilized. Cambridge Isotope Laboratories Inc. (Tewksbury, MA) is offering several isotopically labeled glycans that could be potentially used as both internal standards and calibrants for absolute qMSI of glycans. GlycoScientific, LLC (Athens, GA) is offering iGlycoMab which is a murine IgG2b monoclonal antibody whose glycans are labeled with the stable isotope (15N).
Matrix assisted laser desorption/ionization-mass spectrometry based isomeric glycomics
Without separation, isomeric characterization becomes a challenge for MALDI-MS. Thus, linkage specific derivatization methods have been widely applied in MALDI-MSI to distinguish in situ sialic acid linkages. Differential derivatization, including amidation (de Haan et al., 2015) and esterification (Gomes de Oliveira et al., 2015; Powers et al., 2015), can introduce different mass shift of salic acids with different linkages. These mass differences can be differentiated by MALDI-MS, thus achieving the characterization of sialic acid linkage isomers. The earliest derivatization method was introduced by Wheeler et al. (2009), then improved by Reiding et al. (2014). Later, a two-step derivatization method was developed by Holst et al. (2016) and further modified as the sialic acid linkage specific alkylamidation (SALSA) method (Nishikaze et al., 2017; Hanamatsu et al., 2018; Suzuki et al., 2019) to increase the labeling efficiency; this has been used with the isotopic labeling technique (iSALSA) in liver disease using MALDI-TOF to characterize sialylated glycan isomers (Hanamatsu et al., 2019). Recently, an enzymatic method to distinguish core- and branch-fucosylation was introduced (West et al., 2020), where the endoglycosidase F3 (Endo F3) was utilized to introduce 349.137 Da mass shift between Endo F3 digested and PNGase F digested core-fucosylated N-glycans. These derivatization methods have been employed in MALDI-MSI for in situ isomeric glycomics of multiple diseases (Powers et al., 2013; Holst et al., 2016; Herrera et al., 2019; Blaschke et al., 2020; West et al., 2020). However, isomeric differential derivatization methods have not been widely applied in CNS diseases. The fact that glycan isomers have been demonstrated to be involved in many diseases prompts a need to use these methods in MALDI-MS-based isomeric glycomics in future studies.
Although MALDI-MS and MALDI-MSI provide rapid analytical speed, high throughput, and ability of visualization, the relatively low resolution and sensitivity hinders its application in analyzing low abundant glycans from precious biomedical samples. Therefore, different separation techniques are commonly employed prior to MS to achieve better sensitivity and selectivity of glycans.
Liquid chromatography-mass spectrometry in neurological glycomics
Among the many separation techniques, liquid chromatography (LC) is the most widely used approach in glycomic analysis due to its high reproducibility, high sensitivity, and compatibility with MS. However, the relatively low ionization efficiency of glycans remains a challenge in glycomic analysis. To overcome this issue, a series of derivatization methods were developed to enhance ionization and separation. One of the common derivatizations is the conjugation of different reagents on the reducing end of glycans. The reactions with the glycan reducing end can be (i) reductive amination such as 2-aminobenzoic acid (2-AA) (Ruhaak et al., 2008), 2-aminobenzamide (2-AB) (Royle et al., 2008), procainamide (ProA) (Klapoetke et al., 2010; Kozak et al., 2015), 2-aminopyridine (PA) (Deguchi et al., 2008), 2-aminonaphthalene trisulfonic acid (ANTS) (Lemoine et al., 2000), and 1-aminopyrene-3,6,8-trisulfonic acid (APTS) (Laroy et al., 2006); (ii) hydrazide chemistry such as Girard’s T reagent (GT) (Gil et al., 2008; Unterieser and Mischnick, 2011), Girard’s P reagent (GP) (Kim et al., 2015), phenylhydrazine (Lattova and Perreault, 2003), and 4-phenethylbenzohydrazide (phenyl2-GPN) (Walker et al., 2011); and (iii) carbamate derivatization such as RapiFluor-MS (RFMS) (Lauber et al., 2015) and InstantPC (Xie and Butler, 2022). Another common derivatization technique of glycans is permethylation, where active hydrogens on hydroxyl and amide groups are replaced by a methyl group. Permethylation provides advantages to stabilize labile sialic acids, prevent fucose migration, and significantly increase sensitivity. Zhou et al. (2017c) compared the most common derivatization techniques including 2-AB, ProA, aminoxyTMT, RMFS, and permethylation. RFMS obtained the highest signal for neutral glycans but distorted the sialylated glycan quantitation, while permethylation gained sensitivity for both neutral and sialylated glycans and is accurate in sialylated glycan quantitation. Different derivatization strategies are employed according to different research goals and separation methods used in the studies. These labeling reagents are important to glycomic studies, since most used one of these reagents to enhance sensitivity, improve separation, or even introduce UV absorption.
Liquid chromatography-mass spectrometry analysis of native and reducing end-labeled glycans
Native and reducing end-labeled glycans are highly hydrophilic. A normal reverse phase column (such as a C18 column) cannot be efficient in separation, because reverse phase chromatography relies on hydrophobic interactions. Therefore, hydrophilic interaction chromatography (HILIC) has been widely used in the separation of native and reducing end-labeled glycans. In addition, a porous graphitic carbon (PGC) column is also used in native glycomics due to its polar interactions.
Hydrophilic interaction chromatography in neurological glycomics
HILIC-MS has been a popular choice to separate native (Wuhrer et al., 2004) or reducing end-labeled glycans (Wuhrer et al., 2004; Clarke et al., 2009). Different glycan structures can be resolved by their hydrophilicities. Therefore, the gradient used for a HILIC column is usually from high organic phase (e.g., 80% ACN in water) to high aqueous phase (e.g., 20% ACN in water) with the existence of additives such as ammonium formate (e.g., 50 mM ammonium formate in mobile phase A). HILIC-MS has been demonstrated for glycomic analysis with a variety of diseases (Abd Hamid et al., 2008; Arnold et al., 2011; Doherty et al., 2018; Terkelsen et al., 2018), thus proving its potential application in neurological diseases.
In a study of glycomic changes in aging brain nigrostriatal pathways, an in-house packed amide-80 (Tosoh Biosciences) column and glycanpac AXH-1 (Thermo Fisher Scientific) column were coupled to an LTQ Orbitrap XL MS to analyze the N-glycans and Heparan Sulfate (HS) disaccharides, respectively (Raghunathan et al., 2018). The age-related changes of nigrostriatal N-glycosylation and HS disaccharides were observed, which might provide a deeper insight to improve the viral vectors’ design for Parkinson Disease (PD). Another study of glycomics in aging and PD also employed an in-house packed nano HILIC column interfaced with an Orbitrap XL MS (Thermo Fisher Scientific) to analyze HS disaccharides (Raghunathan et al., 2020). The changes of HS and chondroitin sulfate (CS) domain structures were identified in aged and PD patients’ brains. Noticeably, this study reported the capability of HILIC in separating disaccharide isomers. These recent examples demonstrate the capability of HILIC-MS for glycomic analysis of neurological diseases, and have been applied in additional studies (Russell et al., 2017; Samal et al., 2020).
Along with glycan profiling, HILIC can provide isomeric separations of native and reducing end-labeled glycans (Ahn et al., 2010; Moravcová et al., 2021). The differences in the surface hydrophilicities of glycan isomers can be the main force to resolve glycan isomers. Recently, several studies have reported isomeric separations using HILIC-MS in glycomic analysis of diseases associated with nervous systems. Messina et al. (2021) proved the isomeric separation of N-glycans in the study of CDG glycomics, which is considered to be closely related to many neural system diseases. The glycans were labeled by RFMS and analyzed using a HILIC column (ACQUITY UPLC Glycan BEH Amide, Waters) coupled to an Exactive Orbitrap MS (Thermo Fisher Scientific). Figure 2 depicts the separations of N-glycans on the HILIC column. The separation of glycan compositions was achieved as shown in Figure 2A, demonstrating the potential ability of HILIC-MS for neurological glycomics analysis. Additionally, isomeric separations for both sialylated glycans and high-mannose glycans were observed as shown in Figures 2B,C, respectively. The isomeric structures were assigned based on retention times and comparison to MS databases and literatures. With the separation of isomers, distinct isomeric distribution changes could be observed among different CDG patients and controls.
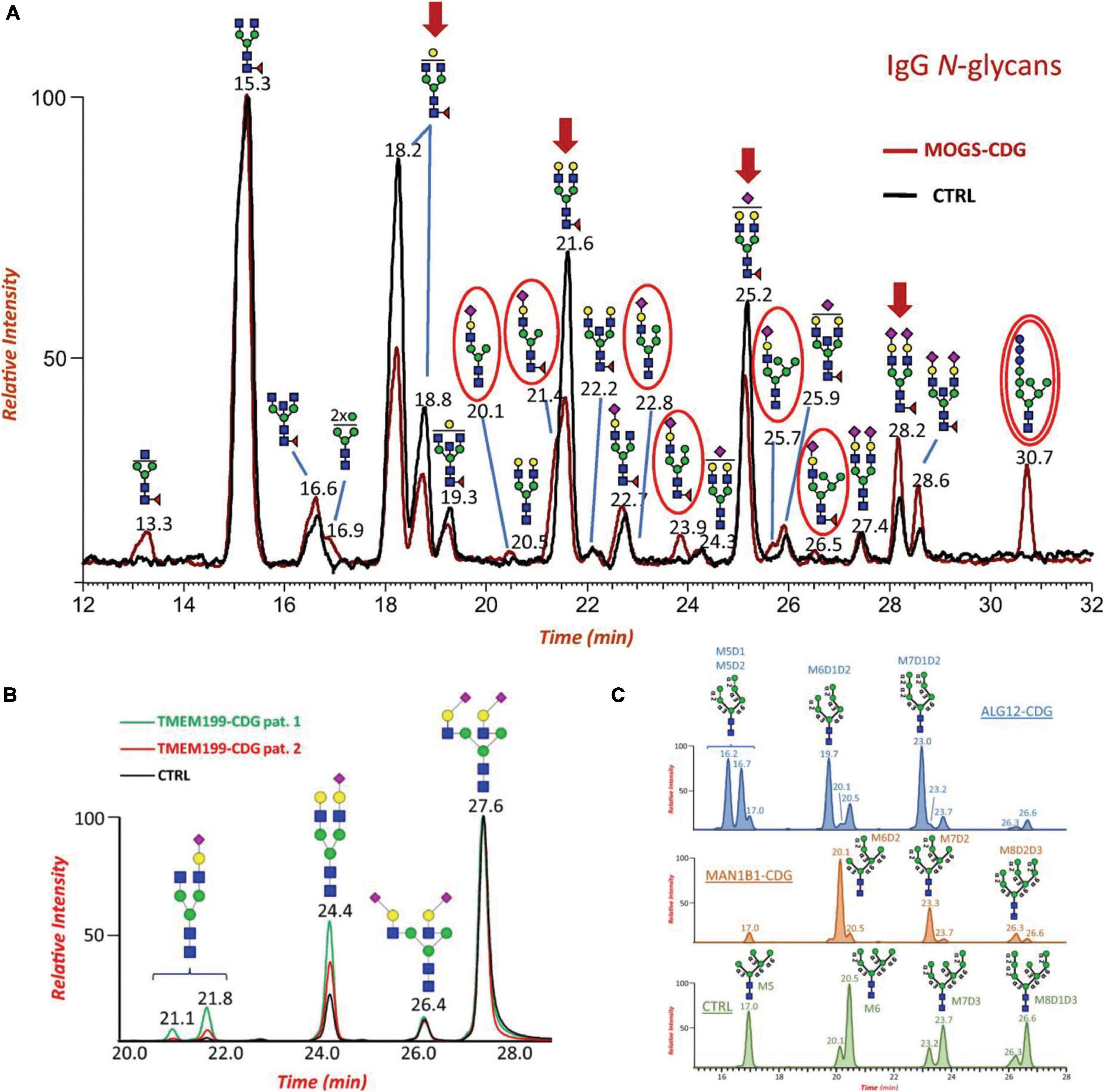
Figure 2. (A) Overlaid Total Ion Current Chromatograms (TICCs) of RapiFluor-MS labeled IgG N-glycans from a patient with MOGS-CDG (red line) and control (black line). Major changes of specific glycoforms are noted in patient profile by red arrows. The presence of disease-specific oligomannose N-glycan biomarkers (Glc3Man7GlcNAc2) is highlighted with a double red circle. (B) Extracted ion chromatograms (EICs) of major RapiFluor-MS labeled biantennary and sialylated N-glycans identified in serum of patient 1 with TMEM199-CDG (green line), patient 2 with TMEM199-CDG (red line) and control (black line). Disialo-isomers eluted at RT 27.6 and 26.4 min depending on NeuAc linkage positions, monosialo-biantennary species eluted at RT 24.4 min whereas monogalacto- monosialo- isomers, if present, eluted at two different retention times due to differential Gal-NeuAc elongation at the two branches, respectively. (C) Comparison of the extracted ion chromatograms (EICs) of the RapiFluor-MS labeled oligomannose serum N-glycans Man5 – 9GlcNAc3 from a patient with ALG12-CDG (blue chromatogram), a patient with MAN1B1-CDG (orange chromatogram), and a healthy control (green chromatogram). Dotted lines refer to linkages that should be missing in the final structure. Reproduced with the modification and permissions from Messina et al. (2021).
Not only N-glycan isomers, but also O-glycan isomers can be characterized by HILIC-MS. In a recent study of PD glycomics, Wilkinson et al. (2021) investigated the O-glycan expression of striatum and substantia nigra tissue in human PD. The O-glycans were labeled with 2-AB and analyzed by a FLR-HILIC-UPLC-MS (BEH glycan column, Waters; Waters Xevo G2 QTof MS). Baseline separations of both sialic linkage isomers and positional isomers were achieved. The isomeric structures were assigned by comparing retention time, MS and MS2 data between FLR-HILIC-UPLC-MS and PGC-LC-MS/MS with the assistance of exoglycosidase array digestion. This approach allowed the identification of 70 O-glycans overall, and revealed a significant increase of sialylation in PD. Although the aforementioned works have proved the potential of HILIC-MS in isomeric glycomic studies, there are fewer studies of neurological diseases relative to other diseases. In addition, larger biological cohorts of neurological diseases need to be further investigated using HILIC-MS.
Porous graphitic carbon in neurological glycomics
Besides HILIC, PGC is another separation technique that is widely applied in native and reducing end-labeled glycomic analysis. There are two major forces, hydrophobic interaction and a distinctive polar retention effect, that drive the separation of glycans on PGC, which makes it a dominant material for efficient isomeric separation and used in multiple diseases (Pereira, 2008; Smilowitz et al., 2013; Stavenhagen et al., 2015; Vitiazeva et al., 2015). PGC-LC-MS has been successfully used in glycomic analysis (Hua et al., 2011), and efforts to establish a PGC-MS glycan database have been recently conducted (Aldredge et al., 2012; Abrahams et al., 2018). Li et al. (2020) summarized the typical PGC-LC-MS protocols for native glycans derived from cells and tissues. Ashwood et al. (2019) introduced a glucose unit (GU) assisted standardization of PGC-LC-MS based glycomics. These data ensure the possibility of using PGC-LC-MS in neurological glycomic analysis.
In an early study, PGC (Hypercarb, Thermo Fisher Scientific) –LC-MS (LTQ Orbitrap XL, Thermo Fisher Scientific) was employed to analyze the native desialylated N-glycans derived from the immunoprecipitation pulled β-site amyloid precursor protein cleaving enzyme-1 (BACE1) (Kizuka et al., 2015). Figure 3 depicts the analysis of released N-glycans from BACE1. While the bisecting biosynthesis, immunoprecipitation, and PNGase F digestion of pulled down BACE1 are shown in Figures 3A–C, respectively, the separation of N-glycans on PGC column are exhibited in Figure 3D as a base peak chromatograph with the assignment of major glycan structures. The red asterisks denote the bisecting structures that were identified by the diagnostic ions in MS2. Efficient isomeric separation of both positional isomers and linkage isomers were achieved, demonstrating the promising application of PGC-LC-MS in native glycomic studies. An increased bisecting glycan level was observed in AD patients and could be the cause of the accumulation of β-amyloid in AD. Later, the same platform was used to further study the role of bisecting glycans on BACE1, and highlighted its positive effect for BACE1 cleavage (Kizuka et al., 2016).
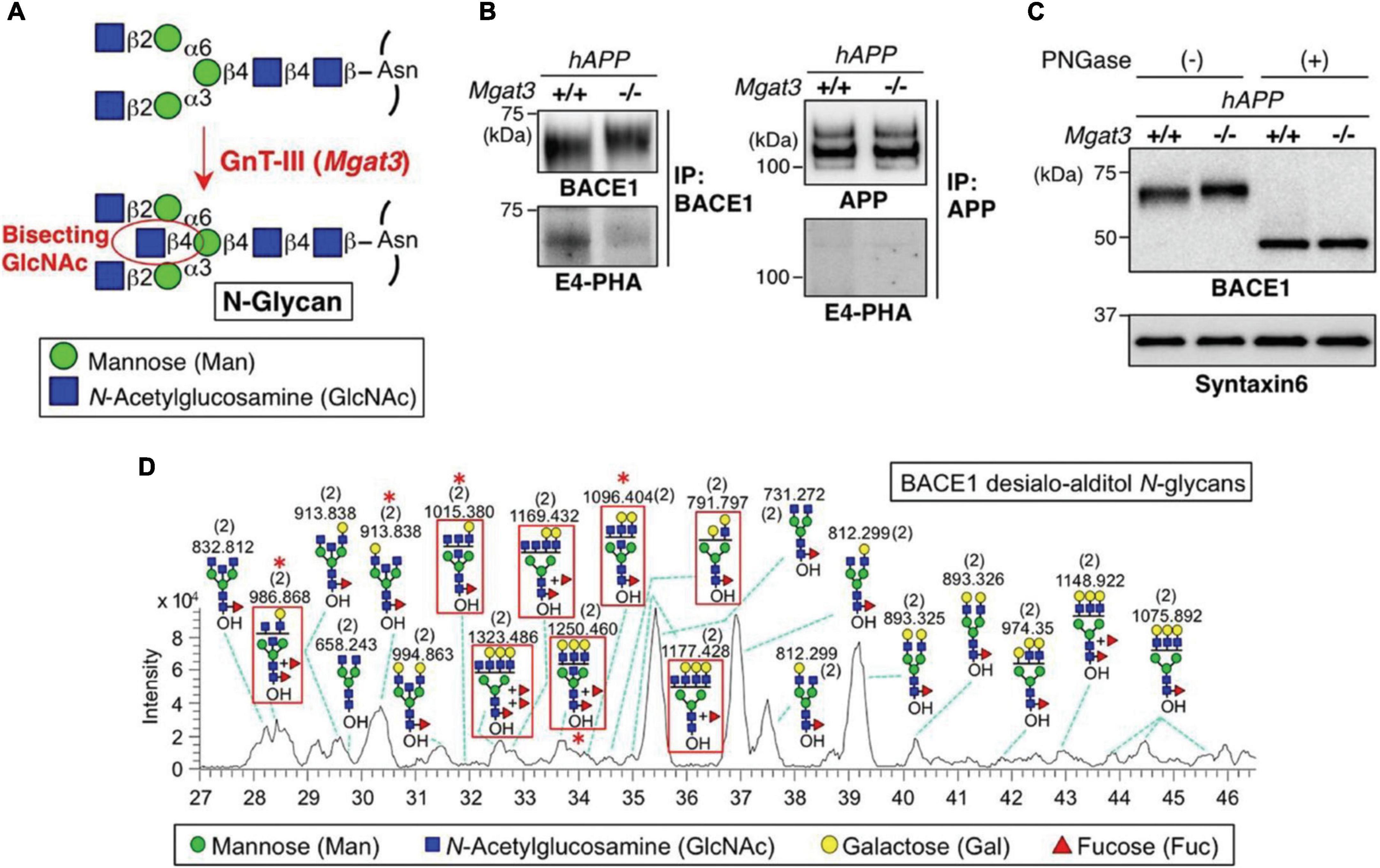
Figure 3. BACE1 is modified with bisecting GlcNAc in vivo. (A) Bisecting GlcNAc modification by GnT-III. (B) BACE1 or APP was immunoprecipitated from mouse brains and blotted with E4-PHA lectin (Lower) or anti-BACE1 or APP antibodies (Upper). hAPP indicates the APP23 transgenic mouse model for AD. (C) Proteins from mouse brain membrane fractions were treated with or without PNGase F and then immunoblotted for BACE1 or for syntaxin 6 (loading control). (D) LC–MS base peak chromatogram of desialo-alditol N-glycans derived from mouse brain BACE1. To simplify the results, N-glycans were chemically desialylated before LC–MS analysis. BACE1-specific glycans, judged by comparison with N-glycan structures from anti-BACE1 IgG, are highlighted by red squares. Asterisks indicate glycans demonstrated by MS/MS analysis to contain a bisecting GlcNAc structure. Numbers in parentheses indicate the charge state. Reprinted with the permissions from Kizuka et al. (2015).
Recently, Lee et al. (2020) investigated the spatial and temporal N-glycome expressions in human and mouse brains using a nano PGC-chip-LC (Agilent) coupled to an Agilent 6530 Q-TOF MS. The native glycomic analysis resulted in a total of 146 and 130 glycans identified in human and mouse brains, respectively. In addition, 37 isomers corresponding to 23 compositions were characterized by this approach. By comparing the glycan abundances among different brain regions, and between human and mouse, significant alterations of glycosylation were found during postnatal development, while changes of sialylation and fucosylation were observed during prefrontal cortex development. These studies highlighted the role of PGC-LC-MS for native glycan analysis in neurological glycomics.
The use of synthesized glycan isomers as internal standards is another efficient way to identify glycan isomers with high confidence. In an early study, Pabst et al. (2007) introduced the strategy of using biosynthesized glycan isomer standards to assist the accurate identification of PGC-LC-MS based fibrinogen and fibrin glycan isomers derived from different species. Recently, from the same group, Helm et al. (2021) performed PGC-LC-MS to analyze glycans derived from animal and human brains using a glycan retention Time Grid (glyco-TiGr) that contained biosynthesized glycan standards with characteristic mass labels to normalize retention time shift among different runs. This study confirmed that the majority of structures in human brain were the bisected type. Notably, for the first time, the authors reported the identification of hybrid-type glycans with galactosylated and even Lewis X containing bisected N-acetylglucosamine discovered in a natural source. Later, a similar strategy was performed with the use of 136 biosynthesized glycan isomer standards to unambiguously identify corresponding glycan isomers from human brain (Helm et al., 2022). A structure with a bisecting sialyl-lactose and structures with fucose and N-acetylgalactosamine on the same arm were identified in human brain.
Liquid chromatography-mass spectrometry analysis of permethylated glycans
Permethylation is one of the most common derivatization strategies in glycomic studies, as it can significantly enhance sensitivity by increasing ionization efficiency, stabilizing labile glycan structures such as sialic acid, and preventing fucose migration during MS/MS (Zhou et al., 2017c). In addition, permethylation provides more information for structural elucidation in MSn. After permethylation, glycans change from hydrophilic to hydrophobic, thus allowing separation on the most popular column in HPLC, the C18 column. Along with reverse phase chromatography such as C18, PGC is also a promising choice for separating permethylated glycans.
C18 in neurological glycomics
Reverse phase (RP) HPLC has been widely used in a variety of fields such as pharmaceutical, life science, industrial food, medical and biomedical, environmental, and more (Žuvela et al., 2019). Among RP materials, the C18 column is the most common and has been dominantly used in chemistry. C18-LC-MS has demonstrated efficient and reliable permethylated glycan profiling for years in many studies (Alley et al., 2012; Hu and Mechref, 2012; Mechref et al., 2012; Hu et al., 2013), with improved separation of permethylated glycans below 55°C (Zhou et al., 2016). Although C18-LC-MS is promising in glycan profiling, only partial isomeric separation was observed on a 15 cm C18 column (Thermo Fisher Scientific) (Hanisch and Muller, 2009). A glucose unit index (GUI) standardization was also recently estimated for permethylated glycan analysis using C18-LC-MS (Gautam et al., 2020).
In neurological glycomic studies, C18-LC-MS has been used to investigate glycan expressions in different CNS diseases. A nanoC18-LC-MS system equipped with an Acclaim® PepMap capillary column (Thermo Fisher Scientific) and a LTQ Orbitrap Velos MS (Thermo Fisher Scientific) was employed in a glioma study (Wildburger et al., 2015). Permethylated N-glycans released from brain tissue sections of attractor and non-attractor glioma stem cell xenograft (GSCXs) were analyzed, allowing the identification of 9 N-glycans whose expressions were altered between the two phenotypes. Although on-tissue N-glycan release in this study might lose analytical depth of the intratumoral microenvironment due to the thin slice of tissue, it demonstrated the success of C18-LC-MS in neurological glycomic analysis. In another study, the same C18-LC-MS system was used to investigate the permethylated N-glycan profiles in neuroblastoma cell lines (Hu et al., 2015).
Recently, Cho et al. (2019) applied this C18-LC-MS platform to investigate permethylated N-glycan expressions in AD cerebrospinal fluid (CSF). Over 88 glycans were identified and quantified in AD patient CSF and healthy controls. Several glycan extracted ion chromatograms (EIC) among different AD and control groups were demonstrated to show the separation of glycans and their differential abundances, as shown in Figure 4A. Further investigation revealed multiple abundant glycans that significantly altered between AD and controls either in female CSF (Figure 4Bi) or male CSF (Figure 4Bii). These glycans exhibited high AUC in the ROC analysis, as shown in Figures 4Biii,iv. The approach in this study also ensured the minimal sample volume (10 μL) of CSF relative to previous studies, making it a promising method of CSF glycomic neurological analysis. In addition to a conventional C18 column, most recently, a micro pillar array based C18 column (μPAC, PharmaFluidics) has been estimated to be reliable for glycomic analysis (Cho et al., 2021b). Although μPAC has not yet been used in CNS diseases, it has potential applications in this field.
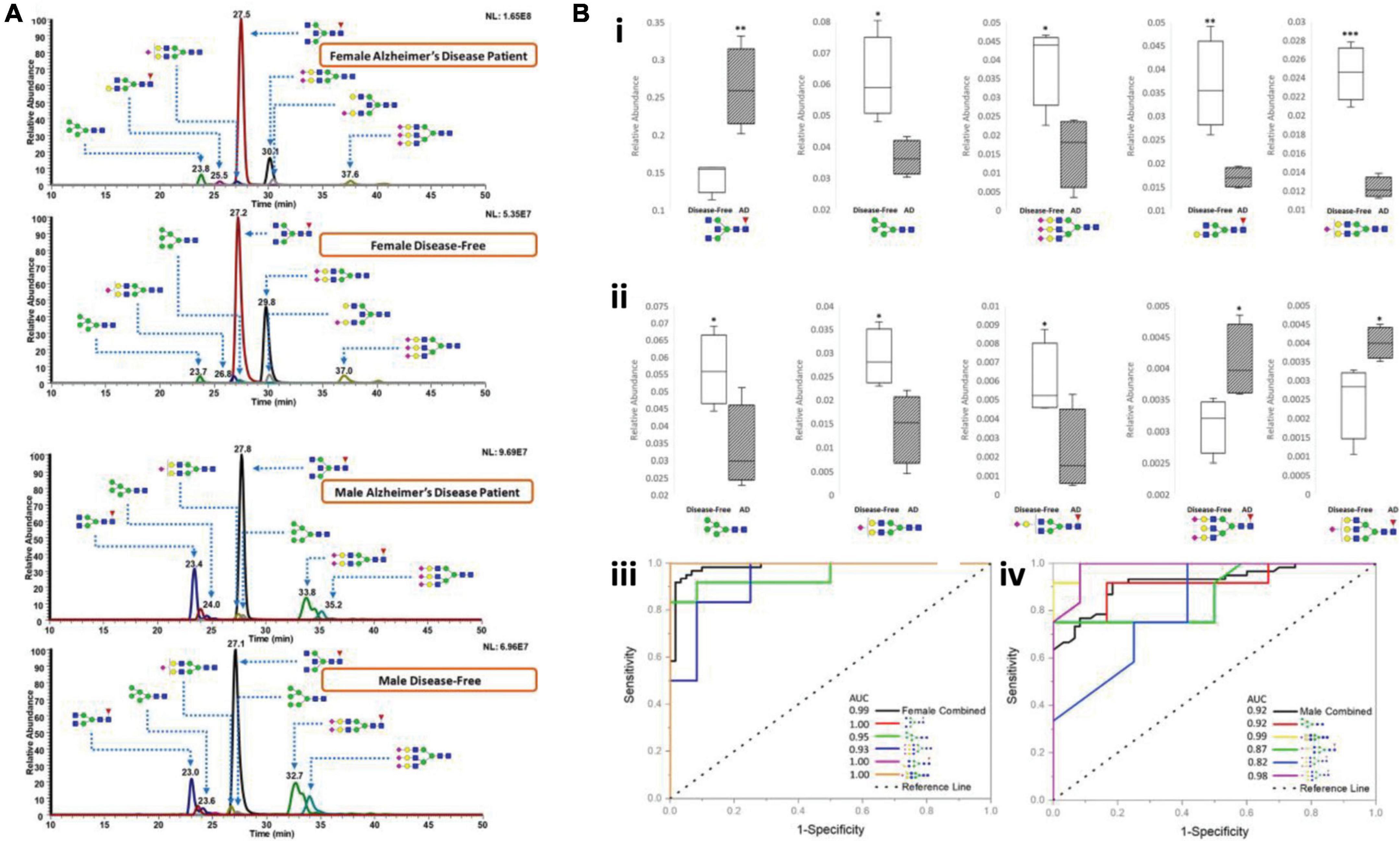
Figure 4. (A) Representative chromatograms of various N-glycans, comparing N-glycan expression in CSF between female AD patients and female disease-free, and between male AD patients and male disease-free. (B) Box and ROC plots depict the comparison between relative abundances of N-glycan structures in female CSF (i) and in male CSF (ii) that are statistically significant among the abundant structures. Solids, disease-free; stripes, Alzheimer’s disease. ROC curves for female (iii) and male (iv). Some of the plots are hidden due to overlapping. Blue square, N-acetylglucosamine (GluNAc); yellow square, N-acetylgalactosamine (GalNAc); green circle, mannose; yellow circle, galactose; red triangle, fucose; purple diamond, sialic acid. Reproduced with the modification and permissions from Cho et al. (2019). *p < 0.05; **p < 0.01; and ***p < 0.001.
Previously, C18 material was considered unsuitable for efficient separation of glycan isomers. However, a study has reported separation using C18-LC-MS for 2-AA labeled N-glycans and aminoxyTMT labeled N-glycans in AD (Gaunitz et al., 2020). Most recently, the isomeric separation ability of C18 was demonstrated using a 200 cm long μPAC column (PharmaFluidics) (Cho et al., 2021b) for the analysis of N-glycan isomers released from coronavirus (Cho et al., 2021a). Although this technique has not been used for neurological glycomic analysis, it can be an alternative to other isomeric glycomic approaches such as PGC- or HILIC-LC-MS.
Porous graphitic carbon in neurological glycomics
As the main interactions on PGC are hydrophobic and polar interactions, both of which fit for permethylated glycans, PGC-LC-MS is considered a method for the separation of permethylated glycans, especially their isomers. An early study showed only partial separation of permethylated Man7 and Man8 isomers derived from RNase B (Stavenhagen et al., 2015). Then, Zhou et al., 2017a,b introduced a high temperature PGC-LC-MS method that enables an efficient isomeric separation of both permethylated N-glycans and smaller glycans such as O-glycans, ganglioside glycans, and free oligosaccharide (Cho et al., 2020). With the development of these methods, PGC-LC-MS of isomeric permethylated glycomics has been applied to address many biological issues such as breast cancer (Peng et al., 2019), liver cancer (Huang et al., 2017b), colorectal cancer (Madunić et al., 2021), and more (Huang et al., 2017b; Xu et al., 2020; Zhang et al., 2020b; Flynn et al., 2021). In addition, the use of GUI has also been estimated for PGC-LC-MS analysis of permethylated glycan isomers (Gautam et al., 2020).
In the field of neurological glycomics, the established PGC-LC-MS platform has been applied to characterize glycan isomers in several studies. Dong et al. (2018) investigated the isomeric distribution of permethylated glycans in an idiopathic rapid eye movement sleep behavior disorder (IRBD, considered an early sign of neurodegeneration; Assogna et al., 2021) using PGC-LC-MS (Hypercarb column, Thermo Fisher Scientific). Linkage isomers as well as positional isomers were resolved, allowing 34 glycan isomers to be identified and quantified, of which 10 isomers were significantly changed in RBD patients. Recently, the same approach was used to investigate the isomeric glycan distribution alterations from patients with restless legs syndrome (RLS) (Dong et al., 2020). A total of 38 isomers were identified, revealing 13 isomers with expression alterations in RLS patients. Figure 5 shows two examples of isomeric separation of disialylated glycans. Baseline separations for all isomers were achieved, enabling the differentiation of significant expression alterations for each isomer between RLS and control samples (insets of Figure 5).
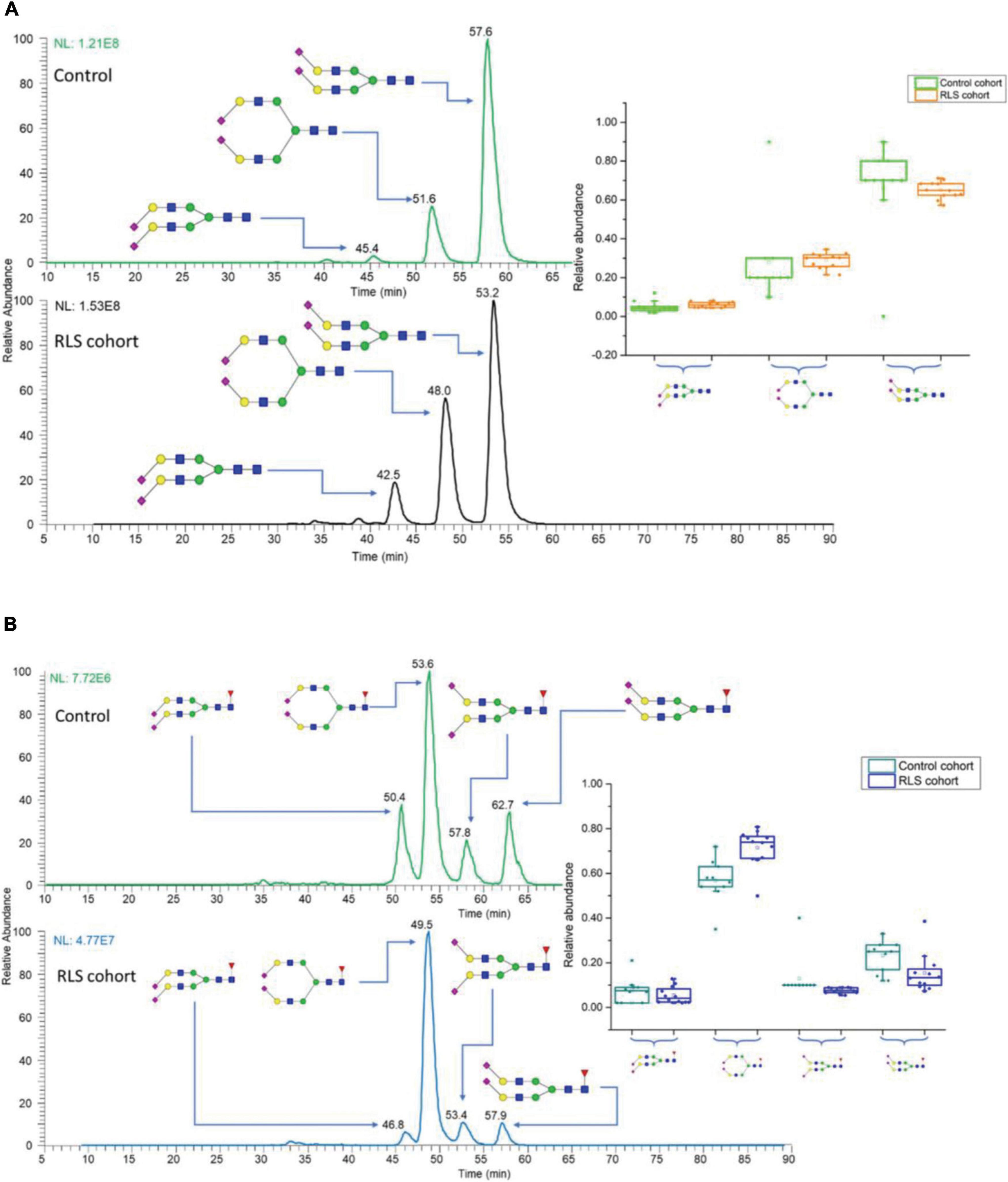
Figure 5. EICs of N-glycan isomers with different linkages in control and restless legs syndrome (RLS) cohorts, separated on PGC (A) disialylated linkage isomers, (B) disialylated linkage isomers with core fucose connections. Insets are the box plots showing the distributions of disialylated isomers among 10 studied subjects in the control and 12 subjects in the RLS cohorts. The box stands for the first quartile and third quartile; the line represents median; and the whiskers above and below the box set the limits for outliers. Reprinted with the permission from Dong et al. (2020).
Recently, a mesoporous graphitic carbon (MGC) column was reported to replace the PGC column (Gautam et al., 2021). The mechanism of MGC is considered similar to PGC, but the smaller particle size and pore size of MGC provides a larger surface area for a better interaction between stationary phase and glycans in LC-MS analysis. These features enabled efficient isomeric separation of permethylated glycans on a 1 cm short MGC column with higher efficiency and reproducibility than a Hypercarb nano PGC column (Gautam et al., 2021). Although this technique has not yet been employed with neurological samples, it could be a better alternative to PGC-LC-MS for isomeric glycomic analysis in CNS diseases.
Capillary electrophoresis-mass spectrometry in neurological glycomics
Capillary electrophoresis (CE) is another major separation technique in addition to LC, providing benefits like low sample and solvent consumption and high sensitivity. Different from LC, the mechanism of CE separation is based on charges and hydrodynamic radius (sizes) of molecules (Stolz et al., 2019). Usually, glycans are required to be derivatized prior to CE-MS analysis for a complete separation. APTS through reductive amination is the routine glycan labeling method in CE-MS (Guttman et al., 1996; Guttman, 1997; Chen and Evangelista, 1998; Szabo et al., 2010). Additionally, aminoxyTMT labeling was reported to be used in CE-MS analysis of glycans (Zhong et al., 2015; Khatri et al., 2017). To date, several of the most common CE techniques employed in glycomic analysis include capillary zone electrophoresis (CZE) (Huang et al., 2017a; Lageveen-Kammeijer et al., 2019), microfluidics capillary electrophoresis (MCE) (Khatri et al., 2017; Snyder et al., 2017; Song et al., 2019), capillary gel electrophoresis (CGE), or DNA analyzer (Reusch et al., 2014; Feng et al., 2017). Because of high separation resolution, most of these CE-MS works reported isomeric separation of glycans, including resolving sialic acid linkage isomers and positional isomers. The selection of CE types were based on different analytical purposes: CZE, the most popular type, provides ultrahigh resolution (a million plates) and very low sample injection volume (nanoliters); MCE, an alternative to CZE, provides faster speed, higher resolution, lower sample volumes, and multiple functions on a single microchip (Fu et al., 2007); DNA analyzer is versatile in addition to analyzing DNAs/RNAs. These features enables the application of CE-MS in neurological glycomic analysis.
Recently, Váradi et al. (2019) introduced a CZE-MS method to monitor 2-AA labeled N-glycans derived from PD patients. A dynamic capillary coating strategy was used to allow the characterization of N-glycans using an Agilent 7100 capillary electrophoresis system coupled to an Agilent 6520 Accurate-Mass Q-TOF MS (Agilent) with the assistance of multiple stage exoglycosidases digestion. The CE-MS approach revealed 15 N-glycans whose expressions were significantly altered in PD samples when compared to controls, demonstrating the successful use of CE-MS in neurological glycomic studies. In this study, only ∼10 nL sample was injected. The smaller sample amount required by CE-MS than LC-MS could be an advantage when analyzing samples with limited quantities. However, very few CE-MS works have been focused on glycomic analysis in CNS diseases. This may be because the ultralow flow rate and necessity of salts in the migration solution can reduce the ionization efficiency of glycans, thus decreasing the sensitivity. Nevertheless, CE-MS is still a promising alternative for neurological glycomics, and should be considered in future studies.
Ion mobility-mass spectrometry in neurological glycomics
In recent decades, a gas-phase based separation technique, ion mobility (IM), has attracted great interest from glyco-scientists. Unlike the previously described techniques, IM separation happens post-glycan ionization where ions are separated in a gas phase. In IM, ions travel in a drift cell driven by an electric field with different velocities according to their different collision cross-sections (CCS) (Baker et al., 2007). The specific CCS that is converted from drift time is related to the size and shape of an ion and is independent from the instrument parameters, where small ions travel faster than larger ions. There are several common types of IM, including drift tube ion mobility (DTIMS), traveling wave ion mobility (TWIMS), field asymmetry ion mobility or differential mobility spectrometry (FAIMS or DMS), and structures for lossless ion manipulation (SLIM) (Deng et al., 2016, 2017; Kalenius et al., 2019).
The first IM-MS study on carbohydrates, reported by Liu and Clemmer (1997), used a home-made DTIMS. Later, the same group investigated glycan profiles from esophageal adenocarcinoma serum using IM-MS, which permitted the identification of more than 20 glycans (Gaye et al., 2012). In addition to glycan profiling, IM-MS has been demonstrated to separate glycan isomers (Manz et al., 2019; Pathak et al., 2020). To enhance the separation of glycans and glycan isomers, many studies have been conducted including the use of TWIMS (Pagel and Harvey, 2013; Harvey et al., 2018), DMS (Lane et al., 2019), and SLIM (Nagy et al., 2018; Ben Faleh et al., 2019; Warnke et al., 2019; Bansal et al., 2020; Wei et al., 2020b; Yalovenko et al., 2020), the establishment of a CCS database for glycans (Struwe et al., 2016; Glaskin et al., 2017), and the assessment of glycan-metal ions (Huang and Dodds, 2013; Xie et al., 2020). Through these endeavors, IM-MS has proved to be able to perform glycan and isomer analysis. However, most of the existing IM-MS studies were only focused on glycan standards or glycans released from purified glycoproteins. There are only a few projects that involved complex biological samples (Gaye et al., 2012; Hofmann et al., 2017; Jin et al., 2019), with barely any studies related to CNS diseases. Although IM-MS provides the advantage of being much less time-consuming, it is hindered by low resolution as well as low sensitivity due to the competitive ionization. However, IM-MS provides a secondary separation dimension of glycans, making it an ideal complement to other separation techniques. The combination of prior-ionization separation and IM-MS has been demonstrated (Jooß et al., 2019) and can be a future direction for glycomic analysis of CNS diseases.
Integration of neurological glycomics and proteomics
As discussed above, different technologies are paving a better way to identify novel glycan markers. Meanwhile, efforts have been made to combine different omic levels, such as glycomics and proteomics, to acquire a deeper understanding of biomolecular mechanisms of CNS disease progress. Wildburger et al. (2015) performed transcriptomics and glycomics to study the in-depth relations of biosynthesis pathways and glycan expressions in glioma using a glioma stem cell xenograft (GSCX) model of glioblastoma, where enrichment of high-mannose glycan biosynthesis and high-mannose glycan levels were related. Recently, the Zaia group introduced a serial on-slide tissue enzyme digestion method to achieve glycomics and proteomics with higher depth and throughput (Raghunathan et al., 2019). This method was then applied to investigate glycomic and proteomic alteration in human brain glioblastoma tissues (Sethi et al., 2022). The alteration of glycans, peptides, and glycopeptides were observed in glioblastoma samples when compared to controls. These works are examples to demonstrate the potential of integrating multiple omics for a deeper insight of neurological diseases in future studies. However, most of multi-omics studies were significantly limited by the sample size. In addition, comprehensive correlation between different omic levels is still a challenge.
Mass spectrometry-based fragmentation techniques facilitate glycomics studies in neurological diseases
One of the main advantages offered by MS-based glycomic analysis is the acquisition of rich structural information of glycans and isomers for a better characterization. However, the structural identification remains a challenge due to the large microheterogeneity of glycosylation. The assignment of all glycans in a glycomic study has never been completely achieved. Although NMR would allow accurate structural elucidation, the fact that NMR requires lots of pure sample (usually mg scale), which is not applicable for most biological samples, has hindered its use in glycomic analysis. In addition, the lack of glycan and glycan isomer standards necessitates the development of standard independent structural identification techniques from MS.
Characterize neurological glycans by different dissociation techniques
A variety of MS dissociation techniques have been developed to improve the identification of complex glycan structures. The deciphering of glycan structures is complicated by their microheterogeneities, particularly in the cases of glycan isomers that have lots of possible combinations of different monosaccharide linkages and positions. Therefore, different dissociation techniques are considered powerful tools to characterize the complex glycan structures and can provide compositional information through multiple glycan fragments or linkage information through cross-ring fragmentation.
Collision-induced dissociation/higher energy collision dissociation
The most common dissociation methods employed in glycomic studies are collision-induced dissociation (CID), collisional activation dissociation (CAD), and higher energy collision dissociation (HCD). CID happens in an ion trap, where the trapped ions are fragmented by applying an extra activation voltage, producing mostly B and Y ions (Han and Costello, 2013). CID is considered a soft fragmentation technique that mainly forms the rupture on a glycosidic bond but barely forms a cross-ring fragment. However, some derivatizations such as permethylation have provided more isomeric information in CAD (Shajahan et al., 2017). CID has been widely used in many glycomic analyses to acquire structural information for glycan compositions (Bruggink et al., 2010; Zhu et al., 2014; Everest-Dass et al., 2016; Ju et al., 2016; Gautam et al., 2020). As a common dissociation technique, CID was also applied in multiple glycomic studies for CNS diseases (Kizuka et al., 2015; Cho et al., 2019; Lee et al., 2020). In addition to CID, HCD is another common dissociation technique used in glycomic analysis. HCD happens in a HCD cell, where ions are fragmented and sent back to Orbitrap for a high-resolution analysis. Thus, compared to CID, HCD has the advantage of higher resolution and no limit of low-mass cutoff. Similar to CID, HCD has also been used for glycomic analysis of biological samples (Zhou et al., 2017b), as well as with CNS diseases (Hu et al., 2015; Wildburger et al., 2015; Kizuka et al., 2016). However, a comparison between CID and HCD indicates that CID takes advantages over HCD in studies that need a faster scan speed (Jedrychowski et al., 2011) such as proteomics for complex biological samples. While HCD commonly provided higher software identification scores and numbers, in some cases (e.g., a phosphoproteome study), CID offered larger data sets. Moreover, the stepped-collision option of HCD in modern MS instruments (e.g., Q-Exactive HF) enables a better fragmentation strategy for omics studies. In addition, the combination of HCD and IT detection can have an even faster scan speed with advantages of both methods, as was recently proved by Tu et al. (2016). However, most of the N- and O-glycomics in CNS disease studies use routine CID or HCD as their fragmentation method.
Examples of common dissociation methods used in CNS diseases are shown in Figure 6. The bi-antennary sialylated N-glycan structure derived from IgG of PD patient (Figure 6A) and sulfated O-glycan structure derived from nigrostriatal tissue of PD patient (Figure 6B) are confirmed by corresponding CID MS2 spectra with high abundant fragment peaks assigned. Comparably, a tri-antennary tri-sialylated glycan structure is also efficiently identified by HCD MS2 spectrum (Figure 6C). It is easier with HCD to acquire oxonium ions for large structures than CID due to the one third m/z cutoff in CID. Noticeably, in all these MS2 spectra, there are no cross-ring fragmentations assigned, indicating that CID/HCD are not ideal dissociating techniques for glycan isomer identification. Therefore, there is a need for the development of novel dissociation methods to obtain adequate glycosidic bond fragmentation as well as enough cross-ring fragmentation.
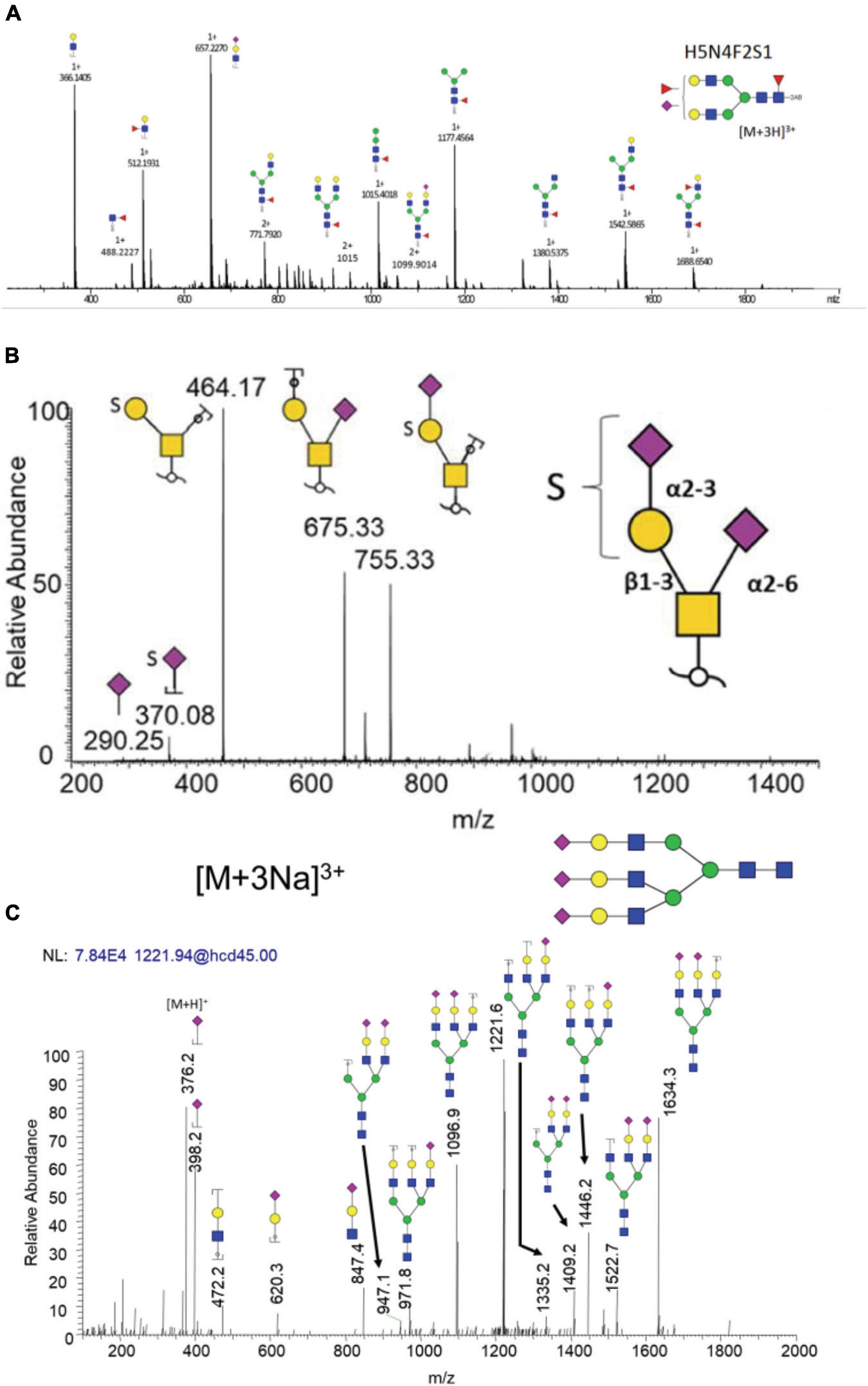
Figure 6. Examples of common dissociation methods used in CNS diseases. (A) CID of bi-antennary sialylated and fucosylated N-glycan derived from IgG of PD patients. (B) CID of sulfated O-glycan derived from nigrostriatal tissue of PD patients. (C) HCD of tri-antennary tri-sialylated N-glycan derived from neuroblastoma cell line. Reproduced with the modification and permissions of panel (A) from Russell et al. (2017), panel (B) from Wilkinson et al. (2021), and panel (C) from Hu et al. (2015).
Dissociation techniques that enhance isomeric identification
Several advanced dissociation techniques have been developed recently. Electronic excitation dissociation (EED), a high energy dissociation method, was first introduced by Yu et al. (2013). In EED, the detachment and recapture of an electron forms a di-radical that generates extensive fragments including cross-ring fragments. The optimized EED has been used to identify glycan isomers (Tang et al., 2018a,b; Wei et al., 2020a,b). An alternative to EED is ultraviolet photodissociation (UVPD), which provides selective cross-ring fragmentation (Devakumar et al., 2007). UVPD happens in an ion trap or HCD cell, where a laser beam triggers the fragmentation, providing a variety of cross-ring fragmentations. It can be combined with HCD or CID to obtain more structural information of glycans (Klein et al., 2019b). UVPD for glycomic analysis has been reported in several studies (Madsen et al., 2013; Ropartz et al., 2014, 2015, 2016; Klein et al., 2019a). Recently, a 266 nm UVPD initiated radical-directed dissociation (RDD) was introduced to enhance the glycan isomeric identification (Riggs et al., 2018) while a hybrid UVPD-CID approach, activated-electron photodetachment (a-EPD), was developed to acquire more linkage information with a less-congested spectrum (Crittenden et al., 2019).
Besides UVPD, infrared multiphoton dissociation (IRMPD) is another laser-induced fragmentation method which uses an infrared laser instead of a UV laser. Unlike EED and UVPD, IRMPD is a low energy dissociation method (Brodbelt, 2014). Therefore, its fragmentation pattern is similar to CID, but faster in analysis with less ion loss (An and Lebrilla, 2011). IRMPD has been successfully applied in glycan profiling and isomeric studies (Zhou and Håkansson, 2013; Jang et al., 2015; Tan and Polfer, 2015; Renois-Predelus et al., 2018; Schindler et al., 2018). Although these new dissociation methods have demonstrated their potential in glycomic studies, most them were insufficient in biological sample analysis, and have not been applied to address real biomedical issues. Nevertheless, the techniques offer great potential for neurological glycomics in future studies.
Other dissociation-based techniques
Characterize neurological glycans by MSn
The MSn technique is considered a powerful tool for isomeric identification of glycans. Theoretically, with consequential fragmentation it can decipher all linkage and positional information of glycan structures. However, the fact that each fragmentation round induces a dramatic decrease of signal intensity limits its application in biological samples, which rarely have enough glycan amount to go deeper than MS3. However, MS3to 5 has been employed in research to distinguish glycan isomers (Ashline et al., 2014, 2017; Kurz et al., 2021). Unfortunately, MSn has not been used to address glycan alterations in CNS diseases, probably due to the difficulty of obtaining large quantities of clinical samples.
Targeted neurological glycan analysis by multiple reaction monitoring and parallel reaction monitoring
Besides identification techniques, targeted analysis such as multiple reaction monitoring/selected reaction monitoring (MRM/SRM) and parallel reaction monitoring (PRM) have been introduced to improve the glycan quantitation. MRM/SRM happens in a triple quadrupole MS instrument, which allows the precursor ion selection in the first quad, CID fragmentation in the second quad, and fragment ion selections in the third quad prior to the detector. Through these steps, most contaminants that have different m/z of either precursor or fragment ion would be removed, thus increasing the quantitation sensitivity and accuracy. An alternative to MRM, PRM is coupled to an Orbitrap instrument which provides high resolution to fragment ions. Another advantage of PRM is that it enables the monitoring of all fragment ions simultaneously, thus allowing the selection of any fragments for quantitation. The MRM for glycomic analysis has been demonstrated (Zhou et al., 2015), while PRM has been proved in a glycoproteomic study (Gutierrez Reyes et al., 2021). The pitfall of both MRM and PRM is that they cannot be utilized in untargeted glycomic analysis, thus limiting their use in initial glycomic studies of CNS diseases. However, with ever increasing glycomic studies being performed, more glycan targets in CNS diseases will be targeted, which could eventually provide a large stage for MRM and PRM in this field.
Analysis of neurological glycans by negative mode mass spectrometry
Most of MS-based glycomic studies for neurological glycan analysis are based on positive mode. However, several studies have demonstrated that negative ionization mode and subsequent CID or HCD fragmentation can be used to analyze glycans (Chai et al., 2001; Pfenninger et al., 2002), and provide benefit in some glycomic areas such as sulfation and sialylation which introduce negative charges to glycan structures (Kuo and Khoo, 2020). In addition, compared to positive mode (B- and Y-ions), negative mode with CID can generate informative A-type cross ring fragments in addition to C-ions, which could provide more structural information than the corresponding positive mode (Harvey, 2005a,b,c). Negative mode with HCD generates C- and Z-ions and cross-ring fragments (Szabo et al., 2017). These features of negative mode glycomics helped to identify N- and O-glycan isomers using a PGC-LC coupled to a negative-ESI-CID-MS/MS (Ashwood et al., 2018). Negative mode glycomics have also been applied to investigate bisecting glycan modifications of the β-site amyloid precursor protein cleaving enzyme-1 in Alzheimer’s disease (Kizuka et al., 2015).
Glycan markers in nervous system diseases
Glycan markers in Alzheimer’s disease and other dementias
Considering that most of the main proteins that have been linked to Alzheimer’s disease are glycosylated and/or are responsible for the post-translational glycosylation of other downstream proteins, the premise of a link between glycans and this burdening neurodegenerative disease is to be supported (Haukedal and Freude, 2020). Several studies have investigated the glycosylation profile of such key proteins in AD patients and discovered an abnormality in the glycan profile of AD patients: the highly expressed special N-glycans, bisecting GlcNAc, were found to be more abundant in AD patients with additional reports revealing that such an increase delays β-site amyloid precursor protein-cleaving enzyme-1 (BACE1) degradation (Hwang et al., 2010) and hence promotes AD pathogenesis (Kizuka et al., 2017). Also, the glycosyltransferase N-acetylglucosaminyltransferase III (GnT-III) which is essential for bisecting GlcNAc residue synthesis is upregulated in Aβ-treated neuroblastoma cells (Akasaka-Manya et al., 2010). In the following section, we will briefly discuss the glycosylation patterns of some that were studied over the years in relation to AD pathogenesis. In the last decades, many studies have revealed the correlation between differential glycosylation and the accumulation of the β-amyloid peptide (Aβ) within the brain (Yu et al., 2000; Kitazume et al., 2001; Yang et al., 2002; Vanoni et al., 2008; Jacobsen and Iverfeldt, 2011; Zhang et al., 2011; Kizuka et al., 2015, 2017; Yang and Qian, 2017). Although the hypothesis of Aβ plays a role in AD progress is recently no longer as attractive as before, we are summarizing some of the reported studies on Aβ.
Other studies have investigated amyloid precursor protein (APP) and its glycosylation pattern and specificity in AD considering that one of the big hallmarks of this multifactorial disease is the accumulation of the β-amyloid peptide (Aβ) within the brain (Zhang et al., 2011), Aβ being the end product of sequential cleavages of APP that occur in the amyloidogenic pathway. APP is characterized by the presence of both N-glycosylation and O-glycosylation sites which encouraged researchers to investigate the possible link between APP N-glycans and O-glycans and the production of Aβ. When Kizuka et al. (2017) manipulated glycan structures, APP transport and trafficking were altered; also, a decrease in N-glycans resulting from a disruption of their formation or maturation seems to cause a decrease in APP levels. Moreover, in vitro studies have revealed the role that O-GlcNAcylation plays in APP processing and hence Aβ secretion by modifying α-secretase activity (Jacobsen and Iverfeldt, 2011). Convergent results were also found in vivo: an increase in O-GlcNAcylation as a result of O-GlcNAcase inhibition decreases the activity of γ-secretase in 5xFAD mice (Yang and Qian, 2017). The later cleaving enzyme is composed of four subunits with only one of them known to be N-glycosylated: nicastrin. This subunit has in fact around 15 identified glycosylation sites and can be found either in its mature form when conjugated with complex N-glycans, or its immature form when complex glycosylation is absent (Yu et al., 2000). It is important to note that the glycosylation of nicastrin is reliant on the two presenilin catalytic subunits of γ-secretase (PSEN1 and PSEN2) and, considering its involvement in the substrate interactions of the cleaving enzyme (Yang et al., 2002), analyzing glycan structures in nicastrin and their possible impact on AD pathogenesis seems to be a promising objective to be worked on.
On another hand, when investigating the impact that glycosylation has on APP with the amyloidogenic pathway, studies have shown that BACE1 (or β-secretase) has four potential N-glycosylation sites which alter the folding and maturity of BACE1 hence affecting its activity and secretion rates (Vanoni et al., 2008). As previously mentioned, BACE1 in AD is characterized by the increased existence of bisecting GlcNAc on its glycosylation sites which seems to cause an increase in Aβ formation because such post-transcriptional modification causes a decrease in lysosomal degradation of BACE1 (Kizuka et al., 2015). Moreover, BACE1 activity influences post-translational modifications of other proteins such as the sialylation of its substrate β-galactoside α2,6-sialyltransferase-1 (ST6GaI1) which has been shown to increase APP and Aβ levels (Kitazume et al., 2001).
Another protein that has been of interest to Alzheimer’s disease in the field of glycosylation is the tau protein. This cytosolic enzyme, which has three N-glycosylation sites at N167, N359, and N410, has been shown to be glycosylated in AD brain, which is unexpected considering that the endoplasmic reticulum (ER) and Golgi are the sites of N-glycosylation (Sato et al., 2001). Studies have revealed the existence of three states of tau in AD brain, each representing a different stage of tau pathology of AD: AD-tau, hyperphosphorylated-tau (AD P-tau), and Paired Helical Filaments-tau (PHF-tau). Liu et al. (2002) discovered that the non-hyperphosphorylated tau was glycosylated in AD brain while it is non-glycosylated in normal brains, and that this glycosylation happens through N-linkage mainly; this makes tau more prone to become hyperphosphorylated without affecting its biological activity directly but by facilitating its substrate-interaction with cAMP-dependent protein kinase. Converging results in mice support the in vitro findings: the molecular weight smears of tau in AD brains were higher and reveal hyper phosphorylation of tau at multiple sites accompanied with a loss of the N-terminal portion (Li et al., 2019). Additional research has revealed that N-glycosylation at the position N359 and the absence of N-glycans at the position N410 seems to aggravate AD symptoms both in vitro and in vivo by intensifying the aggregation of tau (Losev et al., 2021).
Another protein that has appealed to researchers investigating the role of N-glycans in neurodegenerative diseases is the enzyme responsible for the degradation of the neurotransmitter acetylcholine, acetylcholinesterase (AChE). Studies revealed that the level and distribution of AChE isoforms are different in AD: in the frontal cortex of AD patients where amyloid aggregates are usually found, an elevated level of abnormally glycosylated AChE isoforms was found, which was not the case in the cerebellum where amyloid accumulation is absent (Appleyard and McDonald, 1992; Saez-Valero et al., 1999).
The brain chondroitin sulfate-glycosaminoglycan (CS-GAG) sulfation in perineuronal nets (PNNs) has also been related to AD (Miyata et al., 2012). The PNN sulfation change is more likely to promote AD pathology rather than PNN abundance (Huynh et al., 2019; Logsdon et al., 2022). Recently, Logsdon et al. (2022) discovered that CS-GAG were hyper sulfated in AD brain while the total amount of CS-GAG did not change when compared to controls using LC-MS/MS + MRM. These changes were also found to be positively related to P-tau accumulation, and further correlated with impaired cognitive function. This work highlighted the importance of CS-GAG investigation in CNS diseases and provided more clues for future studies. However, these findings might be affected by pre-mortem drug treatment, and could not explain the changes of brain matrix glycans that occurred between Mini-Mental State Examination testing and time of death.
Another indicator that commonly occurs in CNS diseases and injuries is the glial scar (Nicaise et al., 2022). Recent studies have related glial scars to neurodegenerative disease including AD (D’Ambrosi and Apolloni, 2020). Glycosylation changes have been observed and linked to spinal cord regeneration with a decrease of complex glycans in spinal cord injury models (Ronan et al., 2022). In addition, the sulfation pattern of glycosaminoglycans was also observed to be altered in active gliosis in animal models after brain injuries (Alonge et al., 2021). These investigations indicate that glycosylation and glycosaminoglycan sulfation patterns in glial scarring might be explored as potential markers to indicate CNS diseases. However, the above-mentioned studies were not able to analyze enough human cohorts to make a solid conclusion. More research in this area related to large quantities of clinical brain samples should be considered in the future.
Glycan markers in Parkinson’s disease and parkinsonian syndromes
Even though scientists have not been able to elucidate the mechanisms by which α-synuclein aggregates and induces toxicity, it is well established that the aggregation of this protein which causes the formation of Lewy bodies is linked with the pathogenesis of Parkinson’s disease (Conway et al., 2000). And α-synuclein, like most of the human system’s proteins, can be modified post-translation through glycation and glycosylation which will alter its activity and interaction with other macromolecules such as proteins, hormones, and neurotransmitters, hence affecting its tendency to form aggregates (Vicente Miranda et al., 2017). Padmaraju et al. (2011) demonstrated that the glycation of this neuronal protein in one of its 15 lysine residues stabilizes the formed oligomers (Krumova et al., 2011) and, hence, accelerates the aggregation of α-synuclein into oligomeric forms. Accordingly, the accumulation of α-synuclein into molten globule-like structures result from the glycation of this protein with a ribose (Chen et al., 2010b). Hence, knowing that α-synuclein aggregation, and especially its aggregation in its oligomeric forms, cause increased toxicity, studying the role of AGE-α-synuclein in relation to PD pathogenesis has become important.
Many studies using mass spectrometry and western blot analysis revealed that AGEs that are present in the brains of PD patients at the periphery of Lewy bodies, in the substantia nigra, amygdala, and cerebral cortex are intensely modified (Castellani et al., 1996; Dalfo et al., 2005; Bayarsaikhan et al., 2015). Also, AGE receptors (RAGE) expression was increased in the substantia nigra and the frontal cortex in brains characterized by early stages of Parkinsonian neuropathology. Moreover, AGEs and α-synuclein are similarly distributed in Lewy bodies when double immunofluorescence was applied on pre-parkinsonian brains. Choi and Lim, who investigated the link between AGEs and α-synuclein accumulation in a mice model of parkinsonism (MPTP-induced mice), reached similar conclusions: there was co-localization of the well-known AGEs N(ε)-(carboxymethyl)lysine (CML) and N(ε)-(carboxyethyl)lysine (CEL) and the parkinsonian protein α-synuclein. They also detected greater amounts of the oligomeric form of α-synuclein in the substantial nigra region of MPTP-brains compared to the control, non-parkinsonian brains (Choi and Lim, 2010). Moreover, α-synuclein can undergo O-GlcNAcetylation at nine different sites; depending on the site(s) where the modification(s) is/are occurring, the tendency of the acetylated protein to aggregate is altered, usually leading to a decrease in α-synuclein aggregation (Levine et al., 2019).
The triggering receptor expressed on myeloid cells 2 (TREM2) surface receptor is another PD-associated protein that has been investigated in relation to its glycosylation patterns being characterized by two N-glycosylation sites on its immunoglobulin domain. This type I membrane protein, which is even expressed on microglia, seems to have anti-inflammatory properties in various diseases (Rayaprolu et al., 2013). Treatment of TREM2 with different glycosidases revealed the presence of N-linked glycosylation with high mannose and uncovered the modification of TREM2 in the Golgi by the addition of complex oligosaccharide chains (Park et al., 2015). Also, changes in the complex N-glycans that decorate TREM2 alter the conformation and hence the trafficking of this protein, disrupting its antioxidant and protective properties in some pathogenic states.
Another protein that has been inspected in relation to PD pathogenesis the DJ-1 protein, an antioxidant protein that is coded by the PARK7 gene, the latter known to be linked to familial PD. This Parkinsonism-associated protein repairs glyoxal- and methylglyoxal-glycated proteins through its deglycase activity and hence decreases AGEs by degrading the early glycation adducts (Advedissian et al., 2016). In fact, when Advedissian et al. (2016) used small interfering RNAs to deplete DJ-1 in cell culture, an important increase in protein glycation levels was observed, demonstrating the essential role that DJ-1 deglycase plays in averting protein glycation. And because α-synuclein has been demonstrated to be a substrate for DJ-1’s deglycase activity, DJ-1 thus influences α-synuclein aggregation and affects Parkinson’s pathogenesis (Sharma et al., 2019).
Parkin, which is a component of the E3 ubiquitin ligase complex encoded by the gene PARK2, is implicated in Parkinson’s pathogenesis (Imai et al., 2000). This enzyme composed of 426 amino acids not only preserves the activity and integrity of the mitochondria through its capacity to degrade many mitophagia-related membrane proteins, but has been shown to play a role in glutamatergic transmission. One important aspect of parkin is that this protein does not interact with non-glycosylated α-synuclein but does affect α-synuclein aggregation via its interaction with synphilin-1; this interaction has been established both in vivo and in vitro. Such findings come to support the discovery that mutations in parkin are associated with PD symptoms and progression (Chung et al., 2001; Shimura et al., 2001).
One last protein that might be of interest in relation to PD is the Narp protein. In fact, the neuronal pentraxin II (NPTXII) gene upregulated in the substantia nigra of parkinsonian brains has been shown to be in tight association with the aggregation of α-synuclein in both the substantia nigra and cerebral cortex of PD brains (Moran et al., 2008). However, even though this protein is known to have glycosylation sites, little research has focused on its glycosylation and the effect it has on its functions. Hence, more studies are to be conducted to have a better understanding of its role in PD pathogenesis.
Glycan markers in traumatic brain injury
TBI is a critical and increasing global and socio-economic problem (Maas et al., 2017; GBD 2016 Traumatic Brain Injury and Spinal Cord Injury Collaborators, 2019). Despite its high burden, to date, patient characterization and therapeutic decision-making remain a major challenge, and novel markers capable of providing insights into the underlying pathophysiological pathways and improving clinical phenotyping are urgently needed to positively impact patient outcomes (Mondello et al., 2020). Deciphering the astonishing diversity of glycans and their pathobiological roles can enhance our understanding of the heterogeneity and complexity of traumatic brain injury (TBI) while providing opportunities to identify novel therapeutic targets (Kobeissy et al., 2022).
Previous experimental studies have indicated the potential relevance of altered glycosylation in TBI. A comparative analysis of aspirin and clopidogrel treatment in a controlled cortical impact (CCI) model has shown that glycomic changes are indicative of response to the therapeutic interventions (predictive markers) and associated with injury (neuroinflammation) and recovery patterns (Abou-Abbass et al., 2016). More recently, Mondello et al. (2022) performed the first comprehensive profiling of the N-glycome in serial serum samples from patients with moderate to severe TBI. Using cutting-edge highly sensitive liquid chromatography–tandem mass spectroscopy (LC-MS/MS) technologies, serum glycans were analyzed and characterized, identifying a detectable glycofingerprint of TBI and two brain-specific prognostic glycomarker candidates (i.e., HexNAc5Hex3 and HexNAc5Hex4NeuAc1). Taken together, these findings emphasize the need for biomarker research in TBI to move into the field of glycomics, which may also be a powerful tool to unveil possible pathogenetic and pathophysiological mechanism(s) and links between TBI and neurodegenerative diseases.
Glycan markers in other CNS diseases
Glycosylation changes have also been linked to other CNS diseases. The glycomic patterns were found to be altered in Huntington’s disease (HD) mice (Gizaw et al., 2015). The increased levels of core-fucosylated and bisection N-glycans were observed in HD brain tissues when compared to the controls. In recent studies of amyotrophic lateral sclerosis (ALS) (Edri-Brami et al., 2012; Costa et al., 2019), the increase of glycan sialylation and decrease of fucosylation were found in ALS patient sera. Subsequent glycomic analysis revealed the relation of serum IgG glycans with ALS (Edri-Brami et al., 2012). In addition, galactosylation of CSF IgG could be a potential biomarker for ALS (Costa et al., 2019). Multiple sclerosis (MS) disease is another CNS disease, and studies have provided evidence that myelin oligodendrocyte glycoprotein (MOG) specific antibodies and T-cells might contribute to MS (Khare et al., 2018; Bronge et al., 2019). In addition to these neurodegenerative diseases, glycans are also associated with several neurological disorders. Highly branched plasma N-glycan structures were reported to be positively related to major depressive disorder (MDD) (Park et al., 2018), while decreased levels of agalactosylated N-glycans and increased levels of triantennary N-glycans were found in MDD serum (Boeck et al., 2018). Moreover, increased branch-fucosylation of biantennary glycans and decreased levels of some tri- and tetra-antennary glycans were identified in attention-deficit hyperactivity disorder (ADHD) children patient plasma (Pivac et al., 2011).
These studies demonstrate the importance of glycosylation in many CNS diseases which could be potential biomarkers in the future. Glycan changes have also been observed in many other diseases, including but not limited to idiopathic REM sleep behavior disorder (iRBD) (Dong et al., 2018), restless legs syndrome (RLS) (Dong et al., 2020), schizophrenia (Stanta et al., 2010; Tucholski et al., 2013), and post-traumatic stress disorder (PTSD) (Tudor et al., 2019). Although differential glycan abundances were found in different CNS diseases, the limited sample sizes in most of the studies hindered the confident discovery of glycol-markers for these diseases. More clinical cohorts need to be investigated in future studies.
Concluding remarks
We have discussed a variety of advanced MS-based strategies and approaches for in-depth glycomic analysis. These techniques have been successfully applied toward glycan biomarker discovery in many nervous system diseases. Among them, LC-MS with various derivatization strategies is the most common approach due to its high sensitivity, resolution, reproducibility, and robustness. CE and IM could be promising complements for LC as they provide secondary separation dimensions. In addition to separation methods, multiple dissociation and acquisition strategies have also been developed to enhance the identification of glycans, especially for glycan isomers. Together, these MS-based glycomic tools have ensured reliable and efficient analysis to discover novel glycan markers in neurobiological diseases such as Alzheimer’s disease, Parkinson’s disease, traumatic brain injury, and more. However, huge challenges remain for comprehensive characterization of glycan isomers using current technologies. More efficient derivatization and separation approaches need to be developed to achieve a complete separation of all glycan isomers. Meanwhile, effective dissociation methods should also be studied where the combination of different dissociation techniques could be one resolution. In addition to technique development, the biological applications for clinical samples derived from neurological diseases should be addressed. More biomedical and clinical cohorts need to be analyzed to discover and verify potential glycan markers for better prognosis and diagnosis of these diseases.
Author contributions
All authors listed have made a substantial, direct, and intellectual contribution to the work, and approved it for publication.
Funding
This work was supported by the grant from National Institutes of Health (NIH, 1R01GM112490-08). This work was also supported by the Robert A. Welch Foundation under (Grant No. D-0005).
Conflict of interest
The authors declare that the research was conducted in the absence of any commercial or financial relationships that could be construed as a potential conflict of interest.
Publisher’s note
All claims expressed in this article are solely those of the authors and do not necessarily represent those of their affiliated organizations, or those of the publisher, the editors and the reviewers. Any product that may be evaluated in this article, or claim that may be made by its manufacturer, is not guaranteed or endorsed by the publisher.
References
Abd Hamid, U. M., Royle, L., Saldova, R., Radcliffe, C. M., Harvey, D. J., Storr, S. J., et al. (2008). A strategy to reveal potential glycan markers from serum glycoproteins associated with breast cancer progression. Glycobiology 18, 1105–1118. doi: 10.1093/glycob/cwn095
Abou-Abbass, H., Bahmad, H., Abou-El-Hassan, H., Zhu, R., Zhou, S., Dong, X., et al. (2016). Deciphering glycomics and neuroproteomic alterations in experimental traumatic brain injury: Comparative analysis of aspirin and clopidogrel treatment. Electrophoresis 37, 1562–1576. doi: 10.1002/elps.201500583
Abrahams, J. L., Campbell, M. P., and Packer, N. H. (2018). Building a PGC-LC-MS N-glycan retention library and elution mapping resource. Glycoconj. J. 35, 15–29. doi: 10.1007/s10719-017-9793-4
Advedissian, T., Deshayes, F., Poirier, F., Viguier, M., and Richarme, G. (2016). The Parkinsonism-associated protein DJ-1/Park7 prevents glycation damage in human keratinocyte. Biochem. Biophys. Res. Commun. 473, 87–91. doi: 10.1016/j.bbrc.2016.03.056
Ahmed, R. M., Paterson, R. W., Warren, J. D., Zetterberg, H., O’Brien, J. T., Fox, N. C., et al. (2014). Biomarkers in dementia: Clinical utility and new directions. J. Neurol. Neurosurg. Psychiatry. 85, 1426–1434.
Ahn, J., Bones, J., Yu, Y. Q., Rudd, P. M., and Gilar, M. (2010). Separation of 2-aminobenzamide labeled glycans using hydrophilic interaction chromatography columns packed with 1.7 microm sorbent. J. Chromatogr. B Analyt. Technol. Biomed. Life Sci. 878, 403–408. doi: 10.1016/j.jchromb.2009.12.013
Aichler, M., and Walch, A. (2015). MALDI imaging mass spectrometry: Current frontiers and perspectives in pathology research and practice. Lab. Invest. 95, 422–431. doi: 10.1038/labinvest.2014.156
Akasaka-Manya, K., Manya, H., Sakurai, Y., Wojczyk, B. S., Kozutsumi, Y., Saito, Y., et al. (2010). Protective effect of N-glycan bisecting GlcNAc residues on beta-amyloid production in Alzheimer’s disease. Glycobiology 20, 99–106. doi: 10.1093/glycob/cwp152
Aldredge, D., An, H. J., Tang, N., Waddell, K., and Lebrilla, C. B. (2012). Annotation of a serum N-glycan library for rapid identification of structures. J. Proteome Res. 11, 1958–1968. doi: 10.1021/pr2011439
Alley, W. R. J., Vasseur, J. A., Goetz, J. A., Svoboda, M., Mann, B. F., Matei, D. E., et al. (2012). N-linked glycan structures and their expressions change in the blood sera of ovarian cancer patients. J. Proteome Res. 11, 2282–2300.
Alonge, K. M., Herbert, M. J., Yagi, M., Cook, D. G., Banks, W. A., and Logsdon, A. F. (2021). Changes in brain matrix glycan sulfation associate with reactive gliosis and motor coordination in mice with head trauma. Front. Behav. Neurosci. 15:745288. doi: 10.3389/fnbeh.2021.745288
Alzheimers Association, (2020). 2020 Alzheimer’s disease facts and figures. Alzheimers Dement. 16:391–460. doi: 10.1002/alz.12068
An, H. J., and Lebrilla, C. B. (2011). Structure elucidation of native N- and O-linked glycans by tandem mass spectrometry (tutorial). Mass Spectrom. Rev. 30, 560–578. doi: 10.1002/mas.20283
Angel, P. M., Spraggins, J. M., Baldwin, H. S., and Caprioli, R. (2012). Enhanced sensitivity for high spatial resolution lipid analysis by negative ion mode matrix assisted laser desorption ionization imaging mass spectrometry. Anal. Chem. 84:1557. doi: 10.1021/ac202383m
Appleyard, M. E., and McDonald, B. (1992). Acetylcholinesterase and butyrylcholinesterase activities in cerebrospinal fluid from different levels of the neuraxis of patients with dementia of the Alzheimer type. J. Neurol. Neurosurg. Psychiatry. 55, 1074–1078.
Arnold, J. N., Saldova, R., Galligan, M. C., Murphy, T. B., Mimura-Kimura, Y., Telford, J. E., et al. (2011). Novel glycan biomarkers for the detection of lung cancer. J. Proteome Res. 10, 1755–1764. doi: 10.1021/pr101034t
Ashline, D. J., Yu, Y., Lasanajak, Y., Song, X., Hu, L., Ramani, S., et al. (2014). Structural characterization by multistage mass spectrometry (MSn) of human milk glycans recognized by human rotaviruses. Mol. Cell. Proteomics 13, 2961–2974. doi: 10.1074/mcp.M114.039925
Ashline, D. J., Zhang, H., and Reinhold, V. N. (2017). Isomeric complexity of glycosylation documented by MSn. Anal. Bioanal. Chem. 409, 439–451. doi: 10.1007/s00216-016-0018-7
Ashwood, C., Lin, C. H., Thaysen-Andersen, M., and Packer, N. H. (2018). Discrimination of isomers of released N- and O-glycans using diagnostic product ions in negative ion PGC-LC-ESI-MS/MS. J. Am. Soc. Mass Spectrom. 29, 1194–1209. doi: 10.1007/s13361-018-1932-z
Ashwood, C., Pratt, B., MacLean, B. X., Gundry, R. L., and Packer, N. H. (2019). Standardization of PGC-LC-MS-based glycomics for sample specific glycotyping. Analyst 144, 3601–3612. doi: 10.1039/c9an00486f
Assogna, F., Liguori, C., Cravello, L., Macchiusi, L., Belli, C., Placidi, F., et al. (2021). Cognitive and neuropsychiatric profiles in idiopathic rapid eye movement sleep behavior disorder and Parkinson’s disease. J. Pers. Med. 11:51. doi: 10.3390/jpm11010051
Baker, E. S., Clowers, B. H., Li, F., Tang, K., Tolmachev, A. V., Prior, D. C., et al. (2007). Ion mobility spectrometry-mass spectrometry performance using electrodynamic ion funnels and elevated drift gas pressures. J. Am. Soc. Mass Spectrom. 18, 1176–1187. doi: 10.1016/j.jasms.2007.03.031
Banazadeh, A., Peng, W., Veillon, L., and Mechref, Y. (2018). Carbon nanoparticles and graphene nanosheets as maldi matrices in glycomics: A new approach to improve glycan profiling in biological samples. J. Am. Soc. Mass Spectrom. 29, 1892–1900. doi: 10.1007/s13361-018-1985-z
Bansal, P., Yatsyna, V., AbiKhodr, A. H., Warnke, S., Ben Faleh, A., Yalovenko, N., et al. (2020). Using SLIM-based IMS-IMS together with cryogenic infrared spectroscopy for glycan analysis. Anal. Chem. 92, 9079–9085. doi: 10.1021/acs.analchem.0c01265
Barresi, R., Michele, D. E., Kanagawa, M., Harper, H. A., Dovico, S. A., Satz, J. S., et al. (2004). LARGE can functionally bypass alpha-dystroglycan glycosylation defects in distinct congenital muscular dystrophies. Nat. Med. 10, 696–703.
Barry, J. A., Ait-Belkacem, R., Hardesty, W. M., Benakli, L., Andonian, C., Licea-Perez, H., et al. (2019). Multicenter validation study of quantitative imaging mass spectrometry. Anal Chem. 91:6266. doi: 10.1021/acs.analchem.9b01016
Bayarsaikhan, E., Bayarsaikhan, D., Lee, J., Son, M., Oh, S., Moon, J., et al. (2015). Microglial AGE-albumin is critical for neuronal death in Parkinson’s disease: A possible implication for theranostics. Int. J. Nanomed. 10, 281–292. doi: 10.2147/IJN.S95077
Ben Faleh, A., Warnke, S., and Rizzo, T. R. (2019). Combining ultrahigh-resolution ion-mobility spectrometry with cryogenic infrared spectroscopy for the analysis of glycan mixtures. Anal. Chem. 91, 4876–4882. doi: 10.1021/acs.analchem.9b00659
Biskup, K., Braicu, E. I., Sehouli, J., Fotopoulou, C., Tauber, R., Berger, M., et al. (2013). Serum glycome profiling: A biomarker for diagnosis of ovarian cancer. J. Proteome Res. 12, 4056–4063.
Biskup, K., Braicu, E. I., Sehouli, J., Tauber, R., and Blanchard, V. (2017). The ascites N-glycome of epithelial ovarian cancer patients. J. Proteomics 157, 33–39. doi: 10.1016/j.jprot.2017.02.001
Blaschke, C. R. K., Black, A. P., Mehta, A. S., Angel, P. M., and Drake, R. R. (2020). Rapid N-glycan profiling of serum and plasma by a novel slide-based imaging mass spectrometry workflow. J. Am. Soc. Mass Spectrom. 31, 2511–2520. doi: 10.1021/jasms.0c00213
Boeck, C., Pfister, S., Bürkle, A., Vanhooren, V., Libert, C., Salinas-Manrique, J., et al. (2018). Alterations of the serum N-glycan profile in female patients with Major Depressive Disorder. J. Affect. Disord. 234, 139–147.
Brodbelt, J. S. (2014). Photodissociation mass spectrometry: New tools for characterization of biological molecules. Chem. Soc. Rev. 43, 2757–2783.
Bronge, M., Ruhrmann, S., Carvalho-Queiroz, C., Nilsson, O. B., Kaiser, A., Holmgren, E., et al. (2019). Myelin oligodendrocyte glycoprotein revisited-sensitive detection of MOG-specific T-cells in multiple sclerosis. J. Autoimmun. 102, 38–49. doi: 10.1016/j.jaut.2019.04.013
Bruggink, C., Poorthuis, B. J., Piraud, M., Froissart, R., Deelder, A. M., and Wuhrer, M. (2010). Glycan profiling of urine, amniotic fluid and ascitic fluid from galactosialidosis patients reveals novel oligosaccharides with reducing end hexose and aldohexonic acid residues. FEBS J. 277, 2970–2986. doi: 10.1111/j.1742-4658.2010.07707.x
Carraway, K. L., and Hull, S. R. (1991). Cell surface mucin-type glycoproteins and mucin-like domains. Glycobiology 1, 131–138.
Castellani, R., Smith, M. A., Richey, G. L., and Perry, G. (1996). Glycoxidation and oxidative stress in Parkinson disease and diffuse Lewy body disease. Brain Res. 737, 195–200.
Chai, W., Piskarev, V., and Lawson, A. M. (2001). Negative-ion electrospray mass spectrometry of neutral underivatized oligosaccharides. Anal. Chem. 73, 651–657.
Chen, C. C., Engelborghs, S., Dewaele, S., Le Bastard, N., Martin, J. J., Vanhooren, V., et al. (2010a). Altered serum glycomics in Alzheimer disease: A potential blood biomarker? Rejuvenation Res. 13, 439–444.
Chen, F.-T., and Evangelista, R. A. (1998). Profiling glycoprotein n-linked oligosaccharide by capillary electrophoresis. Electrophoresis 19, 2639–2644. doi: 10.1002/elps.1150191512
Chen, L., Wei, Y., Wang, X., and He, R. (2010b). Ribosylation rapidly induces alpha-synuclein to form highly cytotoxic molten globules of advanced glycation end products. PLoS One 5:e9052. doi: 10.1371/journal.pone.0009052
Chen, S. Y., Dong, M., Yang, G., Zhou, Y., Clark, D. J., Lih, T. M., et al. (2020). Glycans, glycosite, and intact glycopeptide analysis of n-linked glycoproteins using liquid handling systems. Anal. Chem. 92, 1680–1686. doi: 10.1021/acs.analchem.9b03761
Cho, B. G., Gautam, S., Peng, W., Huang, Y., Goli, M., and Mechref, Y. (2021a). Direct comparison of N-glycans and their isomers derived from spike glycoprotein 1 of MERS-CoV, SARS-CoV-1, and SARS-CoV-2. J. Proteome Res. 20, 4357–4365. doi: 10.1021/acs.jproteome.1c00323
Cho, B. G., Jiang, P., Goli, M., Gautam, S., and Mechref, Y. (2021b). Using micro pillar array columns (μPAC) for the analysis of permethylated glycans. Analyst 146, 4374–4383. doi: 10.1039/d1an00643f
Cho, B. G., Peng, W., and Mechref, Y. (2020). Separation of permethylated O-glycans, free oligosaccharides, and glycosphingolipid-glycans using porous graphitized carbon (PGC) column. Metabolites 10:433. doi: 10.3390/metabo10110433
Cho, B. G., Veillon, L., and Mechref, Y. N. – (2019). Glycan profile of cerebrospinal fluids from Alzheimer’s disease patients using liquid chromatography with mass spectrometry. J. Proteome Res. 18, 3770–3779.
Choi, Y. G., and Lim, S. N. (2010). (varepsilon)-(carboxymethyl)lysine linkage to alpha-synuclein and involvement of advanced glycation end products in alpha-synuclein deposits in an MPTP-intoxicated mouse model. Biochimie 92, 1379–1386. doi: 10.1016/j.biochi.2010.06.025
Chung, K. K., Zhang, Y., Lim, K. L., Tanaka, Y., Huang, H., Gao, J., et al. (2001). Parkin ubiquitinates the alpha-synuclein-interacting protein, synphilin-1: Implications for Lewy-body formation in Parkinson disease. Nat. Med. 7, 1144–1150. doi: 10.1038/nm1001-1144
Clarke, A., Harmon, B., and DeFelippis, M. R. (2009). Analysis of 3-(acetylamino)-6-aminoacridine-derivatized oligosaccharides from recombinant monoclonal antibodies by liquid chromatography-mass spectrometry. Anal. Biochem. 390, 209–211. doi: 10.1016/j.ab.2009.04.022
Condina, M. R., Mittal, P., Briggs, M. T., Oehler, M. K., Klingler-Hoffmann, M., and Hoffmann, P. (2019). Egg white as a quality control in matrix-assisted laser desorption/ionization mass spectrometry imaging (MALDI-MSI). Anal. Chem. 91:14846.
Conway, K. A., Lee, S. J., Rochet, J. C., Ding, T. T., Williamson, R. E., and Lansbury, P. T. Jr. (2000). Acceleration of oligomerization, not fibrillization, is a shared property of both alpha-synuclein mutations linked to early-onset Parkinson’s disease: Implications for pathogenesis and therapy. Proc. Natl. Acad. Sci. U.S.A. 97, 571–576.
Costa, J., Streich, L., Pinto, S., Pronto-Laborinho, A., Nimtz, M., Conradt, H. S., et al. (2019). Exploring cerebrospinal fluid IgG N-glycosylation as potential biomarker for amyotrophic lateral sclerosis. Mol. Neurobiol. 56, 5729–5739. doi: 10.1007/s12035-019-1482-9
Crittenden, C. M., Escobar, E. E., Williams, P. E., Sanders, J. D., and Brodbelt, J. S. (2019). Characterization of antigenic oligosaccharides from gram-negative bacteria via activated electron photodetachment mass spectrometry. Anal. Chem. 91, 4672–4679. doi: 10.1021/acs.analchem.9b00048
Dalfo, E., Portero-Otin, M., Ayala, V., Martinez, A., Pamplona, R., and Ferrer, I. (2005). Evidence of oxidative stress in the neocortex in incidental Lewy body disease. J. Neuropathol. Exp. Neurol. 64, 816–830. doi: 10.1097/01.jnen.0000179050.54522.5a
D’Ambrosi, N., and Apolloni, S. (2020). Fibrotic scar in neurodegenerative diseases. Front. Immunol. 11:1394. doi: 10.3389/fimmu.2020.01394
de Haan, N., Reiding, K. R., Haberger, M., Reusch, D., Falck, D., and Wuhrer, M. (2015). Linkage-specific sialic acid derivatization for MALDI-TOF-MS profiling of IgG glycopeptides. Anal. Chem. 87, 8284–8291. doi: 10.1021/acs.analchem.5b02426
de Leoz, M. L., Young, L. J., An, H. J., Kronewitter, S. R., Kim, J., Miyamoto, S., et al. (2011). High-mannose glycans are elevated during breast cancer progression. Mol. Cell. Proteomics 10:M110.002717. doi: 10.1074/mcp.M110
Deguchi, K., Keira, T., Yamada, K., Ito, H., Takegawa, Y., Nakagawa, H., et al. (2008). Two-dimensional hydrophilic interaction chromatography coupling anion-exchange and hydrophilic interaction columns for separation of 2-pyridylamino derivatives of neutral and sialylated N-glycans. J. Chromatogr. A 1189, 169–174. doi: 10.1016/j.chroma.2007.09.028
Deng, L., Ibrahim, Y. M., Hamid, A. M., Garimella, S. V. B., Webb, I. K., Zheng, X., et al. (2016). Ultra-high resolution ion mobility separations utilizing traveling waves in a 13 m serpentine path length structures for lossless ion manipulations module. Anal. Chem. 88, 8957–8964. doi: 10.1021/acs.analchem.6b01915
Deng, L., Webb, I. K., Garimella, S. V. B., Hamid, A. M., Zheng, X., Norheim, R. V., et al. (2017). Serpentine ultralong path with extended routing (SUPER) high resolution traveling wave ion mobility-MS using structures for lossless ion manipulations. Anal. Chem. 89, 4628–4634. doi: 10.1021/acs.analchem.7b00185
Devakumar, A., Mechref, Y., Kang, P., Novotny, M. V., and Reilly, J. P. (2007). Laser-induced photofragmentation of neutral and acidic glycans inside an ion-trap mass spectrometer. Rapid Commun. Mass Spectrom. 21, 1452–1460. doi: 10.1002/rcm.2981
Doherty, M., Theodoratou, E., Walsh, I., Adamczyk, B., Stockmann, H., Agakov, F., et al. (2018). Plasma N-glycans in colorectal cancer risk. Sci. Rep. 8:8655. doi: 10.1038/s41598-018-26805-7
Dong, X., Mondello, S., Kobeissy, F., Ferri, R., and Mechref, Y. (2020). Serum glycomics profiling of patients with primary restless legs syndrome using LC-MS/MS. J. Proteome Res. 19, 2933–2941. doi: 10.1021/acs.jproteome.9b00549
Dong, X., Mondello, S., Kobeissy, F., Talih, F., Ferri, R., and Mechref, Y. L. C.-M. S. (2018). /MS glycomics of idiopathic rapid eye movement sleep behavior disorder. Electrophoresis 39, 3096–3103. doi: 10.1002/elps.201800316
Drake, R. R., Powers, T. W., Norris-Caneda, K., Mehta, A. S., and Angel, P. M. (2018). In Situ imaging of N-glycans by MALDI imaging mass spectrometry of fresh or formalin-fixed paraffin-embedded tissue. Curr. Protoc. Protein Sci. 94:e68. doi: 10.1002/cpps.68
Duvet, S., Mouajjah, D., Péanne, R., Matthijs, G., Raymond, K., Jaeken, J., et al. (2018). Use of endoglycosidase H as a diagnostic tool for MAN1B1-CDG patients. Electrophoresis 39, 3133–3141. doi: 10.1002/elps.201800020
Edri-Brami, M., Rosental, B., Hayoun, D., Welt, M., Rosen, H., Wirguin, I., et al. (2012). Glycans in sera of amyotrophic lateral sclerosis patients and their role in killing neuronal cells. PLoS One 7:e35772. doi: 10.1371/journal.pone.0035772
Ellis, S. R., Bruinen, A. L., and Heeren, R. M. A. (2014). A critical evaluation of the current state-of-the-art in quantitative imaging mass spectrometry. Anal. Bioanal. Chem. 406:1275. doi: 10.1007/s00216-013-7478-9
Etters, L., Goodall, D., and Harrison, B. E. (2008). Caregiver burden among dementia patient caregivers: A review of the literature. J. Am. Acad. Nurse Pract. 20, 423–428.
Everest-Dass, A. V., Briggs, M. T., Kaur, G., Oehler, M. K., Hoffmann, P., and Packer, N. H. (2016). N-glycan MALDI imaging mass spectrometry on formalin-fixed paraffin-embedded tissue enables the delineation of ovarian cancer tissues. Mol. Cell. Proteomics 15, 3003–3016. doi: 10.1074/mcp.M116.059816
Everest-Dass, A. V., Moh, E. S. X., Ashwood, C., Shathili, A. M. M., and Packer, N. H. (2018). Human disease glycomics: Technology advances enabling protein glycosylation analysis – part 1. Expert. Rev. Proteomics 15, 165–182.
Feng, H. T., Lim, S., Laserna, A. K. C., Li, P., Yin, X., Simsek, E., et al. (2017). High throughput human plasma N-glycan analysis using DNA analyzer and multivariate analysis for biomarker discovery. Anal. Chim. Acta 995, 106–113. doi: 10.1016/j.aca.2017.09.003
Ferguson, M. A. (1992). Glycosyl-phosphatidylinositol membrane anchors: The tale of a tail. Biochem. Soc. Trans. 20, 243–256. doi: 10.1042/bst0200243
Ferguson, M. A. J. (1991). Lipid anchors on membrane proteins. Curr. Opin. Struct. Biol. 1, 522–529.
Flynn, R. A., Pedram, K., Malaker, S. A., Batista, P. J., Smith, B. A. H., Johnson, A. G., et al. (2021). Small RNAs are modified with N-glycans and displayed on the surface of living cells. Cell 184, 3109–3124. doi: 10.1016/j.cell.2021.04.023
Fu, L. M., Leong, J. C., Lin, C. F., Tai, C. H., and Tsai, C. H. (2007). High performance microfluidic capillary electrophoresis devices. Biomed. Microdevices 9, 405–412.
Gaunitz, S., Tjernberg, L. O., and Schedin-Weiss, S. (2020). The N-glycan profile in cortex and hippocampus is altered in Alzheimer disease. J. Neurochem. 159, 292–304. doi: 10.1111/jnc.15202
Gautam, S., Banazadeh, A., Cho, B. G., Goli, M., Zhong, J., and Mechref, Y. (2021). Mesoporous graphitized carbon column for efficient isomeric separation of permethylated glycans. Anal. Chem. 93, 5061–5070. doi: 10.1021/acs.analchem.0c04395
Gautam, S., Peng, W., Cho, B. G., Huang, Y., Banazadeh, A., Yu, A., et al. (2020). Glucose unit index (GUI) of permethylated glycans for effective identification of glycans and glycan isomers. Analyst 145, 6656–6667. doi: 10.1039/d0an00314j
Gaye, M. M., Valentine, S. J., Hu, Y., Mirjankar, N., Hammoud, Z. T., Mechref, Y., et al. (2012). Ion mobility-mass spectrometry analysis of serum N-linked glycans from esophageal adenocarcinoma phenotypes. J. Proteome Res. 11, 6102–6110. doi: 10.1021/pr300756e
GBD 2016 Traumatic Brain Injury and Spinal Cord Injury Collaborators (2019). Global, regional, and national burden of traumatic brain injury and spinal cord injury, 1990-2016: A systematic analysis for the global burden of disease study 2016. Lancet Neurol. 18, 56–87.
Gil, G. C., Kim, Y. G., and Kim, B. G. (2008). A relative and absolute quantification of neutral N-linked oligosaccharides using modification with carboxymethyl trimethylammonium hydrazide and matrix-assisted laser desorption/ionization time-of-flight mass spectrometry. Anal. Biochem. 379, 45–59. doi: 10.1016/j.ab.2008.04.039
Gizaw, S. T., Koda, T., Amano, M., Kamimura, K., Ohashi, T., Hinou, H., et al. (2015). A comprehensive glycome profiling of Huntington’s disease transgenic mice. Biochim. Biophys. Acta 1850, 1704–1718. doi: 10.1016/j.bbagen.2015.04.006
Gizaw, S. T., Ohashi, T., Tanaka, M., Hinou, H., and Nishimura, S. (2016). Glycoblotting method allows for rapid and efficient glycome profiling of human Alzheimer’s disease brain, serum and cerebrospinal fluid towards potential biomarker discovery. Biochim. Biophys. Acta 1860, 1716–1727. doi: 10.1016/j.bbagen.2016.03.009
Glaskin, R. S., Khatri, K., Wang, Q., Zaia, J., and Costello, C. E. (2017). Construction of a database of collision cross section values for glycopeptides, glycans, and peptides determined by IM-MS. Anal. Chem. 89, 4452–4460. doi: 10.1021/acs.analchem.6b04146
Gomes de Oliveira, A. G., Roy, R., Raymond, C., Bodnar, E. D., Tayi, V. S., Butler, M., et al. (2015). A systematic study of glycopeptide esterification for the semi-quantitative determination of sialylation in antibodies. Rapid Commun. Mass Spectrom. 29, 1817–1826. doi: 10.1002/rcm.7287
Groseclose, M. R., Andersson, M., Hardesty, W. M., and Caprioli, R. M. (2007). Identification of proteins directly from tissue: In situ tryptic digestions coupled with imaging mass spectrometry. J. Mass Spectrom. 42:254. doi: 10.1002/jms.1177
Gutierrez Reyes, C. D., Huang, Y., Atashi, M., Zhang, J., Zhu, J., Liu, S., et al. (2021). PRM-MS quantitative analysis of isomeric N-glycopeptides derived from human serum haptoglobin of patients with cirrhosis and hepatocellular carcinoma. Metabolites 11:563. doi: 10.3390/metabo11080563
Guttman, A. (1997). Analysis of monosaccharide composition by capillary electrophoresis. J. Chromatogr. A 763, 271–277.
Guttman, A., Chen, F.-T. A., Evangelista, R. A., and Cooke, N. (1996). High-resolution capillary gel electrophoresis of reducing oligosaccharides labeled with 1-aminopyrene-3,6,8-trisulfonate. Anal. Biochem. 233, 234–242. doi: 10.1006/abio.1996.0034
Haeuptle, M. A., and Hennet, T. (2009). Congenital disorders of glycosylation: An update on defects affecting the biosynthesis of dolichol-linked oligosaccharides. Hum. Mutat. 30, 1628–1641. doi: 10.1002/humu.21126
Hanamatsu, H., Nishikaze, T., Miura, N., Piao, J., Okada, K., Sekiya, S., et al. (2018). Sialic acid linkage specific derivatization of glycosphingolipid glycans by ring-opening aminolysis of lactones. Anal. Chem. 90, 13193–13199. doi: 10.1021/acs.analchem.8b02775
Hanamatsu, H., Nishikaze, T., Tsumoto, H., Ogawa, K., Kobayashi, T., Yokota, I., et al. (2019). Comparative glycomic analysis of sialyl linkage isomers by sialic acid linkage-specific alkylamidation in combination with stable isotope labeling of α2,3-linked sialic acid residues. Anal. Chem. 91, 13343–13348. doi: 10.1021/acs.analchem.9b03617
Hanisch, F. G., and Muller, S. (2009). Analysis of methylated O-glycan alditols by reversed-phase NanoLC coupled CAD-ESI mass spectrometry. Methods Mol. Biol. 534, 107–115. doi: 10.1007/978-1-59745-022-5_8
Harris, R. J., Leonard, C. K., Guzzetta, A. W., and Spellman, M. W. (1991). Tissue plasminogen activator has an O-linked fucose attached to threonine-61 in the epidermal growth factor domain. Biochemistry. 30, 2311–2314. doi: 10.1021/bi00223a004
Harvey, D. J. (1999). Matrix-assisted laser desorption/ionization mass spectrometry of carbohydrates. Mass Spectrom. Rev. 18, 349–450.
Harvey, D. J. (2005b). Fragmentation of negative ions from carbohydrates: Part 1. Use of nitrate and other anionic adducts for the production of negative ion electrospray spectra from N-linked carbohydrates. J. Am. Soc. Mass Spectrom. 16, 622–630. doi: 10.1016/j.jasms.2005.01.004
Harvey, D. J. (2005c). Fragmentation of negative ions from carbohydrates: part 2. Fragmentation of high-mannose N-linked glycans. J. Am. Soc. Mass Spectrom. 16, 631–646. doi: 10.1016/j.jasms.2005.01.005
Harvey, D. J. (2005a). Fragmentation of negative ions from carbohydrates: Part 3. Fragmentation of hybrid and complex N-linked glycans. J. Am. Soc. Mass Spectrom. 16, 647–659. doi: 10.1016/j.jasms.2005.01.006
Harvey, D. J., Seabright, G. E., Vasiljevic, S., Crispin, M., and Struwe, W. B. (2018). Isomer information from ion mobility separation of high-mannose glycan fragments. J. Am. Soc. Mass Spectrom. 29, 972–988.
Hasan, M. M., Mimi, M. A., Mamun, M. A., Islam, A., Waliullah, A. S. M., Nabi, M. M., et al. (2021). Mass spectrometry imaging for glycome in the brain. Front. Neuroanat. 15:711955. doi: 10.3389/fnana.2021
Haukedal, H., and Freude, K. K. (2020). Implications of glycosylation in Alzheimer’s disease. Front. Neurosci. 14:625348. doi: 10.3389/fnins.2020
Hawkinson, T. R., Clarke, H. A., Young, L. E. A., Conroy, L. R., Markussen, K. H., Kerch, K. M., et al. (2022). In situ spatial glycomic imaging of mouse and human Alzheimer’s disease brains. Alzheimers Dement 18:1721–1735. doi: 10.1002/alz.12523
Heijs, B., Holst, S., Briaire-de Bruijn, I. H., van Pelt, G. W., de Ru, A. H., van Veelen, P. A., et al. (2016). Multimodal mass spectrometry imaging of N-glycans and proteins from the same tissue section. Anal. Chem. 88:7745. doi: 10.1021/acs.analchem.6b01739
Heijs, B., Potthoff, A., Soltwisch, J., and Dreisewerd, K. (2020). MALDI-2 for the enhanced analysis of N-linked glycans by mass spectrometry imaging. Anal. Chem. 92, 13904–13911. doi: 10.1021/acs.analchem.0c02732
Helm, J., Grünwald-Gruber, C., Thader, A., Urteil, J., Führer, J., Stenitzer, D., et al. (2021). Bisecting Lewis X in hybrid-Type N-glycans of human brain revealed by deep structural glycomics. Anal. Chem. 93, 15175–15182. doi: 10.1021/acs.analchem.1c03793
Helm, J., Hirtler, L., and Altmann, F. (2022). Towards mapping of the human brain N-glycome with standardized graphitic carbon chromatography. Biomolecules 12:85. doi: 10.3390/biom12010085
Herrera, H., Dilday, T., Uber, A., Scott, D., Zambrano, J. N., Wang, M., et al. (2019). Core-fucosylated tetra-antennary N-glycan containing a single N-acetyllactosamine branch is associated with poor survival outcome in breast cancer. Int. J. Mol. Sci. 20:2528. doi: 10.3390/ijms20102528
Hofmann, J., Stuckmann, A., Crispin, M., Harvey, D. J., Pagel, K., and Struwe, W. B. (2017). Identification of lewis and blood group carbohydrate epitopes by ion mobility-tandem-mass spectrometry fingerprinting. Anal. Chem. 89, 2318–2325. doi: 10.1021/acs.analchem.6b03853
Holst, S., Heijs, B., de Haan, N., van Zeijl, R. J., Briaire-de Bruijn, I. H., van Pelt, G. W., et al. (2016). Linkage-specific in situ sialic acid derivatization for N-glycan mass spectrometry imaging of formalin-fixed paraffin-embedded tissues. Anal. Chem. 88, 5904–5913. doi: 10.1021/acs.analchem.6b00819
Hu, Y., and Mechref, Y. (2012). Comparing MALDI-MS, RP-LC-MALDI-MS and RP-LC-ESI-MS glycomic profiles of permethylated N-glycans derived from model glycoproteins and human blood serum. Electrophsresis 33, 1768–1777. doi: 10.1002/elps.201100703
Hu, Y., Mayampurath, A., Khan, S., Cohen, J. K., Mechref, Y., and Volchenboum, S. L. (2015). N-linked glycan profiling in neuroblastoma cell lines. J. Proteome Res. 14, 2074–2081.
Hu, Y., Zhou, S., Khalil, S. I., Renteria, C. L., and Mechref, Y. (2013). Glycomic profiling of tissue sections by LC-MS. Anal. Chem. 85, 4074–4079.
Hua, S., An, H. J., Ozcan, S., Ro, G. S., Soares, S., DeVere-White, R., et al. (2011). Comprehensive native glycan profiling with isomer separation and quantitation for the discovery of cancer biomarkers. Analyst 136, 3663–3671. doi: 10.1039/c1an15093f
Huang, C., Liu, Y., Wu, H., Sun, D., and Li, Y. (2017a). Characterization of IgG glycosylation in rheumatoid arthritis patients by MALDI-TOF-MS(n) and capillary electrophoresis. Anal. Bioanal. Chem. 409, 3731–3739. doi: 10.1007/s00216-017-0302-1
Huang, Y., and Dodds, E. D. (2013). Ion mobility studies of carbohydrates as group I adducts: Isomer specific collisional cross section dependence on metal ion radius. Anal. Chem. 85, 9728–9735. doi: 10.1021/ac402133f
Huang, Y., Zhou, S., Zhu, J., Lubman, D. M., and Mechref, Y. (2017b). LC-MS/MS isomeric profiling of permethylated N-glycans derived from serum haptoglobin of hepatocellular carcinoma (HCC) and cirrhotic patients. Electrophoresis 38, 2160–2167. doi: 10.1002/elps.201700025
Huffman, J. E., Pučić-Baković, M., Klarić, L., Hennig, R., Selman, M. H., Vučković, F., et al. (2014). Comparative performance of four methods for high-throughput glycosylation analysis of immunoglobulin G in genetic and epidemiological research. Mol. Cell. Proteomics 13, 1598–1610. doi: 10.1074/mcp.M113.037465
Huynh, M. B., Ouidja, M. O., Chantepie, S., Carpentier, G., Maïza, A., Zhang, G., et al. (2019). Glycosaminoglycans from Alzheimer’s disease hippocampus have altered capacities to bind and regulate growth factors activities and to bind tau. PLoS One 14:e0209573. doi: 10.1371/journal.pone.0209573
Hwang, H., Zhang, J., Chung, K. A., Leverenz, J. B., Zabetian, C. P., Peskind, E. R., et al. (2010). Glycoproteomics in neurodegenerative diseases. Mass Spectrom. Rev. 29, 79–125.
Imai, Y., Soda, M., and Takahashi, R. (2000). Parkin suppresses unfolded protein stress-induced cell death through its E3 ubiquitin-protein ligase activity. J. Biol. Chem. 275, 35661–35664.
Inyoung, K., Songhui, K., Dongwon, S., and Jeongkwon, K. (2016). Matrix additives in MALDI-TOF MS analysis of glycans. Bull. Kor. Chem. Soc. 37, 105–107.
Jacobsen, K. T., and Iverfeldt, K. O. (2011). GlcNAcylation increases non-amyloidogenic processing of the amyloid-beta precursor protein (APP). Biochem. Biophys. Res. Commun. 404, 882–886. doi: 10.1016/j.bbrc.2010.12.080
Jang, K. S., Nani, R. R., Kalli, A., Levin, S., Muller, A., Hess, S., et al. (2015). A cationic cysteine-hydrazide as an enrichment tool for the mass spectrometric characterization of bacterial free oligosaccharides. Anal. Bioanal. Chem. 407, 6181–6190. doi: 10.1007/s00216-015-8798-8
Jedrychowski, M. P., Huttlin, E. L., Haas, W., Sowa, M. E., Rad, R., and Gygi, S. P. (2011). Evaluation of HCD- and CID-type fragmentation within their respective detection platforms for murine phosphoproteomics. Mol. Cell. Proteomics 10:M111.009910. doi: 10.1074/mcp.M111
Jensen, P. H., Mysling, S., Højrup, P., and Jensen, O. N. (2013). Glycopeptide enrichment for MALDI-TOF mass spectrometry analysis by hydrophilic interaction liquid chromatography solid phase extraction (HILIC SPE). Methods Mol. Biol. 951, 131–144.
Jiao, J., Zhang, Y., Yang, P., and Lu, H. (2015). Hydrazinonicotinic acid as a novel matrix for highly sensitive and selective MALDI-MS analysis of oligosaccharides. Analyst 140, 156–161. doi: 10.1039/c4an01659a
Jin, C., Harvey, D. J., Struwe, W. B., and Karlsson, N. G. (2019). Separation of isomeric O-glycans by ion mobility and liquid chromatography–mass spectrometry. Anal. Chem. 91, 10604–10613. doi: 10.1021/acs.analchem.9b01772
Jooß, K., Meckelmann, S. W., Klein, J., Schmitz, O. J., and Neusüß, C. (2019). Capillary zone electrophoresis coupled to drift tube ion mobility-mass spectrometry for the analysis of native and APTS-labeled N-glycans. Anal. Bioanal. Chem. 411, 6255–6264. doi: 10.1007/s00216-018-1515-7
Ju, L., Wang, Y., Xie, Q., Xu, X., Li, Y., Chen, Z., et al. (2016). Elevated level of serum glycoprotein bifucosylation and prognostic value in Chinese breast cancer. Glycobiology 26, 460–471. doi: 10.1093/glycob/cwv117
Kalenius, E., Groessl, M., and Rissanen, K. (2019). Ion mobility–mass spectrometry of supramolecular complexes and assemblies. Nat. Rev. Chem. 3, 4–14.
Kamiyama, T., Yokoo, H., Furukawa, J., Kurogochi, M., Togashi, T., Miura, N., et al. (2013). Identification of novel serum biomarkers of hepatocellular carcinoma using glycomic analysis. Hepatology 57, 2314–2325.
Kang, P., Mechref, Y., Klouckova, I., and Novotny, M. V. (2005). Solid-phase permethylation of glycans for mass spectrometric analysis. Rapid Commun. Mass Spectrom. 19, 3421–3428.
Kaprio, T., Satomaa, T., Heiskanen, A., Hokke, C. H., Deelder, A. M., Mustonen, H., et al. (2015). N-glycomic profiling as a tool to separate rectal adenomas from carcinomas. Mol. Cell. Proteomics 14, 277–288. doi: 10.1074/mcp.M114.041632
Karas, M., Ehring, H., Nordhoff, E., Stahl, B., Strupat, K., Hillenkamp, F., et al. (1993). Matrix-assisted laser-desorption ionization mass-spectrometry with additives to 2,5-dihydroxybenzoic acid. Org. Mass Spectrom. 28, 1476–1481.
Khare, P., Challa, D. K., Devanaboyina, S. C., Velmurugan, R., Hughes, S., Greenberg, B. M., et al. (2018). Myelin oligodendrocyte glycoprotein-specific antibodies from multiple sclerosis patients exacerbate disease in a humanized mouse model. J. Autoimmun. 86, 104–115. doi: 10.1016/j.jaut.2017.09.002
Khatri, K., Klein, J. A., Haserick, J. R., Leon, D. R., Costello, C. E., McComb, M. E., et al. (2017). Microfluidic capillary electrophoresis-mass spectrometry for analysis of monosaccharides, oligosaccharides, and glycopeptides. Anal. Chem. 89, 6645–6655. doi: 10.1021/acs.analchem.7b00875
Kim, K. J., Kim, Y. W., Hwang, C. H., Park, H. G., Yang, Y. H., Koo, M., et al. (2015). A MALDI-MS-based quantitative targeted glycomics (MALDI-QTaG) for total N-glycan analysis. Biotechnol. Lett. 37, 2019–2025. doi: 10.1007/s10529-015-1881-6
Kitazume, S., Tachida, Y., Oka, R., Shirotani, K., Saido, T. C., and Hashimoto, Y. (2001). Alzheimer’s beta-secretase, beta-site amyloid precursor protein-cleaving enzyme, is responsible for cleavage secretion of a Golgi-resident sialyltransferase. Proc. Natl. Acad. Sci. U.S.A. 98, 13554–13559. doi: 10.1073/pnas.241509198
Kizuka, Y., Kitazume, S., and Taniguchi, N. (2017). N-glycan and Alzheimer’s disease. Biochim. Biophys. Acta Gen. Subj. 1861, 2447–2454.
Kizuka, Y., Kitazume, S., Fujinawa, R., Saito, T., Iwata, N., Saido, T. C., et al. (2015). An aberrant sugar modification of BACE1 blocks its lysosomal targeting in Alzheimer’s disease. EMBO Mol. Med. 7, 175–189. doi: 10.15252/emmm.201404438
Kizuka, Y., Nakano, M., Kitazume, S., Saito, T., Saido, T. C., and Taniguchi, N. (2016). Bisecting GlcNAc modification stabilizes BACE1 protein under oxidative stress conditions. Biochem. J. 473, 21–30. doi: 10.1042/BJ20150607
Klapoetke, S., Zhang, J., Becht, S., Gu, X., and Ding, X. (2010). The evaluation of a novel approach for the profiling and identification of N-linked glycan with a procainamide tag by HPLC with fluorescent and mass spectrometric detection. J. Pharm. Biomed. Anal. 53, 315–324. doi: 10.1016/j.jpba.2010.03.045
Klein, D. R., Leach, F. E. III, Amster, I. J., and Brodbelt, J. S. (2019a). Structural characterization of glycosaminoglycan carbohydrates using ultraviolet photodissociation. Anal. Chem. 91, 6019–6026.
Klein, D. R., Powers, M. J., Trent, M. S., and Brodbelt, J. S. (2019b). Top-down characterization of lipooligosaccharides from antibiotic-resistant bacteria. Anal. Chem. 91, 9608–9615. doi: 10.1021/acs.analchem.9b00940
Kobeissy, F., Kobaisi, A., Peng, W., Barsa, C., Goli, M., Sibahi, A., et al. (2022). Glycomic and glycoproteomic techniques in neurodegenerative disorders and neurotrauma: Towards personalized markers. Cells 11:581. doi: 10.3390/cells11030581
Kozak, R. P., Tortosa, C. B., Fernandes, D. L., and Spencer, D. I. (2015). Comparison of procainamide and 2-aminobenzamide labeling for profiling and identification of glycans by liquid chromatography with fluorescence detection coupled to electrospray ionization-mass spectrometry. Anal. Biochem. 486, 38–40. doi: 10.1016/j.ab.2015.06.006
Kristic, J., and Lauc, G. (2017). Ubiquitous importance of protein glycosylation. Methods Mol. Biol. 1503, 1–12.
Krumova, P., Meulmeester, E., Garrido, M., Tirard, M., Hsiao, H. H., Bossis, G., et al. (2011). Sumoylation inhibits alpha-synuclein aggregation and toxicity. J. Cell Biol. 194, 49–60.
Kuo, C. W., and Khoo, K. H. (2020). Strategic applications of negative-mode LC-MS/MS analyses to expedite confident mass spectrometry-based identification of multiple glycosylated peptides. Anal. Chem. 92, 7612–7620. doi: 10.1021/acs.analchem.0c00236
Kurz, S., Sheikh, M. O., Lu, S., Wells, L., and Tiemeyer, M. (2021). Separation and identification of permethylated glycan isomers by reversed phase NanoLC-NSI-MS(n). Mol. Cell Proteomics 20:100045. doi: 10.1074/mcp.RA120.002266
Kyselova, Z., Mechref, Y., Kang, P., Goetz, J. A., Dobrolecki, L. E., Sledge, G. W., et al. (2008). Breast cancer diagnosis and prognosis through quantitative measurements of serum glycan profiles. Clin. Chem. 54, 1166–1175. doi: 10.1373/clinchem.2007.087148
Lageveen-Kammeijer, G. S. M., de Haan, N., Mohaupt, P., Wagt, S., Filius, M., Nouta, J., et al. (2019). Highly sensitive CE-ESI-MS analysis of N-glycans from complex biological samples. Nat. Commun. 10:2137. doi: 10.1038/s41467-019-09910-7
Lane, C. S., McManus, K., Widdowson, P., Flowers, S. A., Powell, G., Anderson, I., et al. (2019). Separation of sialylated glycan isomers by differential mobility spectrometry. Anal. Chem. 91, 9916–9924.
Laroy, W., Contreras, R., and Callewaert, N. (2006). Glycome mapping on DNA sequencing equipment. Nat. Protoc. 1, 397–405.
Lattova, E., and Perreault, H. (2003). Labelling saccharides with phenylhydrazine for electrospray and matrix-assisted laser desorption–ionization mass spectrometry. J. Chromatogr. B 793, 167–179.
Lauber, M. A., Yu, Y. Q., Brousmiche, D. W., Hua, Z., Koza, S. M., Magnelli, P., et al. (2015). Rapid preparation of released N-glycans for HILIC analysis using a labeling reagent that facilitates sensitive fluorescence and ESI-MS detection. Anal. Chem. 87, 5401–5409. doi: 10.1021/acs.analchem.5b00758
Lee, J., Ha, S., Kim, M., Kim, S. W., Yun, J., Ozcan, S., et al. (2020). Spatial and temporal diversity of glycome expression in mammalian brain. Proc. Natl. Acad. Sci. U.S.A. 117, 28743–28753. doi: 10.1073/pnas.2014207117
Lemoine, J., Cabanes-Macheteau, M., Bardor, M., Michalski, J. C., Faye, L., and Lerouge, P. (2000). Analysis of 8-aminonaphthalene-1,3,6-trisulfonic acid labelled N-glycans by matrix-assisted laser desorption/ionisation time-of-flight mass spectrometry. Rapid Commun. Mass Spectrom. 14, 100–104. doi: 10.1002/(SICI)1097-0231(20000130)14:2<100::AID-RCM845>3.0.CO;2-W
Levine, P. M., Galesic, A., Balana, A. T., Mahul-Mellier, A. L., Navarro, M. X., De Leon, C. A., et al. (2019). alpha-synuclein O-GlcNAcylation alters aggregation and toxicity, revealing certain residues as potential inhibitors of Parkinson’s disease. Proc. Natl. Acad. Sci. U.S.A. 116, 1511–1519. doi: 10.1073/pnas.1808845116
Li, L., Jiang, Y., Hu, W., Tung, Y. C., Dai, C., Chu, D., et al. (2019). Pathological alterations of Tau in Alzheimer’s disease and 3xTg-AD mouse brains. Mol. Neurobiol. 56, 6168–6183.
Li, Q., Xie, Y., Wong, M., Barboza, M., and Lebrilla, C. B. (2020). Comprehensive structural glycomic characterization of the glycocalyxes of cells and tissues. Nat. Protoc. 15, 2668–2704. doi: 10.1038/s41596-020-0350-4
Liang, Q., Macher, T., Xu, Y., Bao, Y., and Cassady, C. J. (2014). MALDI MS in-source decay of glycans using a glutathione-capped iron oxide nanoparticle matrix. Anal. Chem. 86, 8496–8503. doi: 10.1021/ac502422a
Lis, H., and Sharon, N. (1993). Protein glycosylation. Structural and functional aspects. Eur. J. Biochem. 218, 1–27.
Liu, F., Zaidi, T., Iqbal, K., Grundke-Iqbal, I., Merkle, R. K., and Gong, C.-X. (2002). Role of glycosylation in hyperphosphorylation of tau in Alzheimer’s disease. FEBS Lett. 512, 101–106.
Liu, K., Paterson, A. J., Chin, E., and Kudlow, J. E. (2000). Glucose stimulates protein modification by O-linked GlcNAc in pancreatic beta cells: Linkage of O-linked GlcNAc to beta cell death. Proc. Natl. Acad. Sci. U.S.A. 97, 2820–2825. doi: 10.1073/pnas.97.6.2820
Liu, S., Cheng, L., Fu, Y., Liu, B. F., and Liu, X. (2018). Characterization of IgG N-glycome profile in colorectal cancer progression by MALDI-TOF-MS. J. Proteomics 181, 225–237. doi: 10.1016/j.jprot.2018.04.026
Liu, Y., and Clemmer, D. E. (1997). Characterizing oligosaccharides using injected-ion mobility/mass spectrometry. Anal. Chem. 69, 2504–2509. doi: 10.1021/ac9701344
Logsdon, A. F., Francis, K. L., Richardson, N. E., Hu, S. J., Faber, C. L., Phan, B. A., et al. (2022). Decoding perineuronal net glycan sulfation patterns in the Alzheimer’s disease brain. Alzheimers Dement 18, 942–954. doi: 10.1002/alz.12451
Losev, Y., Frenkel-Pinter, M., Abu-Hussien, M., Viswanathan, G. K., Elyashiv-Revivo, D., Geries, R., et al. (2021). Differential effects of putative N-glycosylation sites in human Tau on Alzheimer’s disease-related neurodegeneration. Cell. Mol. Life Sci. 78, 2231–2245. doi: 10.1007/s00018-020-03643-3
Maas, A. I. R., Menon, D. K., Adelson, P. D., Andelic, N., Bell, M. J., Belli, A., et al. (2017). Traumatic brain injury: Integrated approaches to improve prevention, clinical care, and research. Lancet Neurol. 16, 987–1048.
Madsen, J. A., Ko, B. J., Xu, H., Iwashkiw, J. A., Robotham, S. A., Shaw, J. B., et al. (2013). Concurrent automated sequencing of the glycan and peptide portions of O-linked glycopeptide anions by ultraviolet photodissociation mass spectrometry. Anal. Chem. 85, 9253–9261. doi: 10.1021/ac4021177
Madunić, K., Zhang, T., Mayboroda, O. A., Holst, S., Stavenhagen, K., Jin, C., et al. (2021). Colorectal cancer cell lines show striking diversity of their O-glycome reflecting the cellular differentiation phenotype. Cell. Mol. Life Sci. 78, 337–350. doi: 10.1007/s00018-020-03504-z
Malaker, S. A., Quanico, J., Raffo-Romero, A., Kobeissy, F., Aboulouard, S., Tierny, D., et al. (2022). On-tissue spatially resolved glycoproteomics guided by N-glycan imaging reveal global dysregulation of canine glioma glycoproteomic landscape. Cell Chem. Biol. 29, 30–42. doi: 10.1016/j.chembiol.2021.05.007
Manz, C., Grabarics, M., Hoberg, F., Pugini, M., Stuckmann, A., Struwe, W. B., et al. (2019). Separation of isomeric glycans by ion mobility spectrometry – the impact of fluorescent labelling. Analyst 144, 5292–5298. doi: 10.1039/c9an00937j
Martin, P. T. (2007). Congenital muscular dystrophies involving the O-mannose pathway. Curr. Mol. Med. 7, 417–425. doi: 10.2174/156652407780831601
Mechref, Y., and Novotny, M. V. (1998). Matrix-assisted laser desorption ionization mass spectrometry of acidic glycoconjugates facilitated by the use of spermine as a co-matrix. J. Am. Soc. Mass Spectrom. 9, 1293–1302. doi: 10.1016/S1044-0305(98)00106-8
Mechref, Y., Hu, Y., Garcia, A., Zhou, S., Desantos-Garcia, J. L., and Hussein, A. (2012). Defining putative glycan cancer biomarkers by MS. Bioanalysis 4, 2457–2469.
Messina, A., Palmigiano, A., Esposito, F., Fiumara, A., Bordugo, A., Barone, R., et al. (2021). HILIC-UPLC-MS for high throughput and isomeric N-glycan separation and characterization in congenital disorders glycosylation and human diseases. Glycoconj. J. 38, 201–211. doi: 10.1007/s10719-020-09947-7
Metzger, J. O., Woisch, R., Tuszynski, W., and Angermann, R. (1994). New-type of matrix for matrix-assisted laser-desorption mass-spectrometry of polysaccharides and proteins. Fresenius J. Anal. Chem. 349, 473–474.
Miyata, S., Komatsu, Y., Yoshimura, Y., Taya, C., and Kitagawa, H. (2012). Persistent cortical plasticity by upregulation of chondroitin 6-sulfation. Nat. Neurosci. 15, s1–s2. doi: 10.1038/nn.3023
Mohr, M. D., Bornsen, K. O., and Widmer, H. M. (1995). Matrix-assisted laser-desorption ionization mass-spectrometry – improved matrix for oligosaccharides. Rapid Commun. Mass Spectrom. 9, 809–814.
Mondello, S., Guedes, V. A., Lai, C., Czeiter, E., Amrein, K., Kobeissy, F., et al. (2020). Circulating brain injury exosomal proteins following moderate-to-severe traumatic brain injury: Temporal profile, outcome prediction and therapy implications. Cells 9:977. doi: 10.3390/cells9040977
Mondello, S., Sandner, V., Goli, M., Czeiter, E., Amrein, K., Kochanek, P. M., et al. (2022). Exploring serum glycome patterns to inform novel biomarker discovery, endophenotyping, and novel therapeutic targets after moderate to severe traumatic brain injury. EClinialMedicine 50:101494. doi: 10.1016/j.eclinm.2022.101494
Moran, L. B., Hickey, L., Michael, G. J., Derkacs, M., Christian, L. M., Kalaitzakis, M. E., et al. (2008). Neuronal pentraxin II is highly upregulated in Parkinson’s disease and a novel component of Lewy bodies. Acta Neuropathol. 115, 471–478. doi: 10.1007/s00401-007-0309-3
Moravcová, D., Čmelík, R., and Křenková, J. (2021). Separation of labeled isomeric oligosaccharides by hydrophilic interaction liquid chromatography – the role of organic solvent in manipulating separation selectivity of the amide stationary phase. J. Chromatogr. A 1651, 462303. doi: 10.1016/j.chroma.2021.462303
Nagy, G., Attah, I. K., Garimella, S. V. B., Tang, K., Ibrahim, Y. M., Baker, E. S., et al. (2018). Unraveling the isomeric heterogeneity of glycans: Ion mobility separations in structures for lossless ion manipulations. Chem. Commun. 54, 11701–11704. doi: 10.1039/c8cc06966b
Nicaise, A. M., D’Angelo, A., Ionescu, R. B., Krzak, G., Willis, C. M., and Pluchino, S. (2022). The role of neural stem cells in regulating glial scar formation and repair. Cell Tissue Res. 387, 399–414.
Nikzad-Terhune, K. A., Anderson, K. A., Newcomer, R., and Gaugler, J. E. (2010). Do trajectories of at-home dementia caregiving account for burden after nursing home placement? A growth curve analysis. Soc. Work Health Care 49, 734–752. doi: 10.1080/00981381003635296
Nishikaze, T., Tsumoto, H., Sekiya, S., Iwamoto, S., Miura, Y., and Tanaka, K. (2017). Differentiation of sialyl linkage isomers by one-pot sialic acid derivatization for mass spectrometry-based glycan profiling. Anal. Chem. 89, 2353–2360. doi: 10.1021/acs.analchem.6b04150
Pabst, M., Bondili, J. S., Stadlmann, J., Mach, L., and Altmann, F. (2007). Mass + retention time = structure: A strategy for the analysis of N-glycans by carbon LC-ESI-MS and its application to fibrin N-glycans. Anal. Chem. 79, 5051–5057. doi: 10.1021/ac070363i
Padmaraju, V., Bhaskar, J. J., Prasada Rao, U. J., Salimath, P. V., and Rao, K. S. (2011). Role of advanced glycation on aggregation and DNA binding properties of alpha-synuclein. J. Alzheimers Dis. 24(Suppl. 2) 211–221.
Pagel, K., and Harvey, D. J. (2013). Ion mobility-mass spectrometry of complex carbohydrates: Collision cross sections of sodiated N-linked glycans. Anal. Chem. 85, 5138–5145. doi: 10.1021/ac400403d
Palmigiano, A., Messina, A., Bua, R. O., Barone, R., Sturiale, L., Zappia, M., et al. (2018). CSF N-glycomics using MALDI MS techniques in Alzheimer’s disease. Methods Mol. Biol. 1750, 75–91. doi: 10.1007/978-1-4939-7704-8_5
Park, D. I., Štambuk, J., Razdorov, G., Pučić-Baković, M., Martins-de-Souza, D., Lauc, G., et al. (2018). Blood plasma/IgG N-glycome biosignatures associated with major depressive disorder symptom severity and the antidepressant response. Sci. Rep. 8:179. doi: 10.1038/s41598-017-17500-0
Park, J. S., Ji, I. J., An, H. J., Kang, M. J., Kang, S. W., Kim, D. H., et al. (2015). Disease-associated mutations of TREM2 alter the processing of N-linked oligosaccharides in the Golgi apparatus. Traffic 16, 510–518. doi: 10.1111/tra.12264
Parodi, A. J. (2000). Role of N-oligosaccharide endoplasmic reticulum processing reactions in glycoprotein folding and degradation. Biochem. J. 348, 1–13.
Pathak, P., Baird, M. A., and Shvartsburg, A. A. (2020). High-resolution ion mobility separations of isomeric glycoforms with variations on the peptide and glycan levels. J. Am. Soc. Mass Spectrom. 31, 1603–1609. doi: 10.1021/jasms.0c00183
Peng, W., Goli, M., Mirzaei, P., and Mechref, Y. (2019). Revealing the biological attributes of N-glycan isomers in breast cancer brain metastasis using porous graphitic carbon (PGC) Liquid chromatography-tandem mass spectrometry (LC-MS/MS). J. Proteome Res. 18, 3731–3740. doi: 10.1021/acs.jproteome.9b00429
Pereira, L. (2008). Porous graphitic carbon as a stationary phase in HPLC: Theory and applications. J. Liq. Chromatogr. R T 31, 1687–1731.
Pfenninger, A., Karas, M., Finke, B., and Stahl, B. (2002). Structural analysis of underivatized neutral human milk oligosaccharides in the negative ion mode by nano-electrospray MS(n) (part 1: methodology). J. Am. Soc. Mass Spectrom. 13, 1331–1340.
Pinho, S. S., and Reis, C. A. (2015). Glycosylation in cancer: Mechanisms and clinical implications. Nat. Rev. Cancer 15, 540–555.
Pivac, N., Knezević, A., Gornik, O., Pucić, M., Igl, W., Peeters, H., et al. (2011). Human plasma glycome in attention-deficit hyperactivity disorder and autism spectrum disorders. Mol. Cell. Proteomics 10:M110.004200. doi: 10.1074/mcp.M110.004200
Powers, T. W., Holst, S., Wuhrer, M., Mehta, A. S., and Drake, R. R. (2015). Two-dimensional N-glycan distribution mapping of hepatocellular carcinoma tissues by MALDI-imaging mass spectrometry. Biomolecules 5, 2554–2572. doi: 10.3390/biom5042554
Powers, T. W., Jones, E. E., Betesh, L. R., Romano, P. R., Gao, P., Copland, J. A., et al. (2013). Matrix assisted laser desorption ionization imaging mass spectrometry workflow for spatial profiling analysis of N-linked glycan expression in tissues. Anal. Chem. 85, 9799–9806. doi: 10.1021/ac402108x
Qin, X., Guo, Y., Du, H., Zhong, Y., Zhang, J., Li, X., et al. (2017). Comparative analysis for glycopatterns and complex-type N-glycans of glycoprotein in sera from chronic hepatitis B- and C-infected patients. Front. Physiol. 8:596. doi: 10.3389/fphys.2017.00596
Raghunathan, R., Hogan, J. D., Labadorf, A., Myers, R. H., and Zaia, J. (2020). A glycomics and proteomics study of aging and Parkinson’s disease in human brain. Sci. Rep. 10:12804. doi: 10.1038/s41598-020-69480-3
Raghunathan, R., Polinski, N. K., Klein, J. A., Hogan, J. D., Shao, C., Khatri, K., et al. (2018). Glycomic and proteomic changes in aging brain nigrostriatal pathway. Mol. Cell. Proteomics 17, 1778–1787. doi: 10.1074/mcp.RA118.000680
Raghunathan, R., Sethi, M. K., and Zaia, J. (2019). On-slide tissue digestion for mass spectrometry based glycomic and proteomic profiling. MethodsX 6, 2329–2347. doi: 10.1016/j.mex.2019.09.029
Rayaprolu, S., Mullen, B., Baker, M., Lynch, T., Finger, E., Seeley, W. W., et al. (2013). TREM2 in neurodegeneration: Evidence for association of the p.R47H variant with Frontotemporal dementia and Parkinson’s disease. Mol. Neurodegener. 8:19. doi: 10.1186/1750-1326-8-19
Rebelo, A. L., Gubinelli, F., Roost, P., Jan, C., Brouillet, E., Van Camp, N., et al. (2021). Complete spatial characterisation of N-glycosylation upon striatal neuroinflammation in the rodent brain. J. Neuroinflammation 18:116. doi: 10.1186/s12974-021-02163-6
Reiding, K. R., Blank, D., Kuijper, D. M., Deelder, A. M., and Wuhrer, M. (2014). High-throughput profiling of protein N-glycosylation by MALDI-TOF-MS employing linkage-specific sialic acid esterification. Anal. Chem. 86, 5784–5793. doi: 10.1021/ac500335t
Renois-Predelus, G., Schindler, B., and Compagnon, I. (2018). Analysis of sulfate patterns in glycosaminoglycan oligosaccharides by MS(n) coupled to infrared ion spectroscopy: The case of GalNAc4S and GalNAc6S. J. Am. Soc. Mass Spectrom. 29, 1242–1249. doi: 10.1007/s13361-018-1955-5
Reusch, D., Haberger, M., Kailich, T., Heidenreich, A. K., Kampe, M., Bulau, P., et al. (2014). High-throughput glycosylation analysis of therapeutic immunoglobulin G by capillary gel electrophoresis using a DNA analyzer. MAbs 6, 185–196. doi: 10.4161/mabs.26712
Richard, D., Cummings, S. K., Schneider, W. J., Hobgood, K. K., Tolleshaug, H., Brown, M. S., et al. (1983). Biosynthesis of N- and O-linked oligosacchardies of the low density lipoprotein receptor. J. Biol. Chem. 258, 15261–15273.
Richardson, T. J., Lee, S. J., Berg-Weger, M., and Grossberg, G. T. (2013). Caregiver health: Health of caregivers of Alzheimer’s and other dementia patients. Curr. Psychiatry Rep. 15:367. doi: 10.1007/s11920-013-0367-2
Riggs, D. L., Hofmann, J., Hahm, H. S., Seeberger, P. H., Pagel, K., and Julian, R. R. (2018). Glycan isomer identification using ultraviolet photodissociation initiated radical chemistry. Anal. Chem. 90, 11581–11588. doi: 10.1021/acs.analchem.8b02958
Ronan, R., Kshirsagar, A., Rebelo, A. L., Sunny, A., Kilcoyne, M., Flaherty, R. O., et al. (2022). Distinct glycosylation responses to spinal cord injury in regenerative and nonregenerative models. J. Proteome Res. 21, 1449–1466. doi: 10.1021/acs.jproteome.2c00043
Ropartz, D., Giuliani, A., Fanuel, M., Herve, C., Czjzek, M., and Rogniaux, H. (2016). Online coupling of high-resolution chromatography with extreme UV photon activation tandem mass spectrometry: Application to the structural investigation of complex glycans by dissociative photoionization. Anal. Chim. Acta 933, 1–9. doi: 10.1016/j.aca.2016.05.036
Ropartz, D., Giuliani, A., Herve, C., Geairon, A., Jam, M., Czjzek, M., et al. (2015). High-energy photon activation tandem mass spectrometry provides unprecedented insights into the structure of highly sulfated oligosaccharides extracted from macroalgal cell walls. Anal. Chem. 87, 1042–1049. doi: 10.1021/ac5036007
Ropartz, D., Lemoine, J., Giuliani, A., Bittebiere, Y., Enjalbert, Q., Antoine, R., et al. (2014). Deciphering the structure of isomeric oligosaccharides in a complex mixture by tandem mass spectrometry: Photon activation with vacuum ultra-violet brings unique information and enables definitive structure assignment. Anal. Chim. Acta 807, 84–95. doi: 10.1016/j.aca.2013.11.018
Royle, L., Campbell, M. P., Radcliffe, C. M., White, D. M., Harvey, D. J., Abrahams, J. L., et al. (2008). HPLC-based analysis of serum N-glycans on a 96-well plate platform with dedicated database software. Anal. Biochem. 376, 1–12. doi: 10.1016/j.ab.2007.12.012
Ruhaak, L. R., Huhn, C., Waterreus, W. J., de Boer, A. R., Neusüss, C., Hokke, C. H., et al. (2008). Hydrophilic interaction chromatography-based high-throughput sample preparation method for N-glycan analysis from total human plasma glycoproteins. Anal. Chem. 80, 6119–6126. doi: 10.1021/ac800630x
Russell, A. C., Simurina, M., Garcia, M. T., Novokmet, M., Wang, Y., Rudan, I., et al. (2017). The N-glycosylation of immunoglobulin G as a novel biomarker of Parkinson’s disease. Glycobiology 27, 501–510. doi: 10.1093/glycob/cwx022
Rzagalinski, I., and Volmer, D. A. (2017). Quantification of low molecular weight compounds by MALDI imaging mass spectrometry – a tutorial review. Biochim. Biophys. Acta Proteins Proteomics. 1865:726. doi: 10.1016/j.bbapap.2016.12.011
Saez-Valero, J., Sberna, G., McLean, C. A., and Small, D. H. (1999). Molecular isoform distribution and glycosylation of acetylcholinesterase are altered in brain and cerebrospinal fluid of patients with Alzheimer’s disease. J. Neurochem. 72, 1600–1608. doi: 10.1046/j.1471-4159.1999.721600.x
Samal, J., Saldova, R., Rudd, P. M., Pandit, A., and O’Flaherty, R. (2020). Region-specific characterization of N-glycans in the striatum and Substantia nigra of an adult rodent brain. Anal. Chem. 92, 12842–12851. doi: 10.1021/acs.analchem.0c01206
Sato, Y., Naito, Y., Grundke-Iqbal, I., Iqbal, K., and Endo, T. (2001). Analysis ofN-glycans of pathological tau: Possible occurrence of aberrant processing of tau in Alzheimer’s disease. FEBS Lett. 496, 152–160. doi: 10.1016/s0014-5793(01)02421-8
Schedin-Weiss, S., Gaunitz, S., Sui, P., Chen, Q., Haslam, S. M., Blennow, K., et al. (2020). Glycan biomarkers for Alzheimer disease correlate with T-tau and P-tau in cerebrospinal fluid in subjective cognitive impairment. FEBS J. 287, 3221–3234. doi: 10.1111/febs.15197
Schindler, B., Laloy-Borgna, G., Barnes, L., Allouche, A. R., Bouju, E., Dugas, V., et al. (2018). Online separation and identification of isomers using infrared multiple photon dissociation ion spectroscopy coupled to liquid chromatography: Application to the analysis of disaccharides regio-isomers and monosaccharide anomers. Anal. Chem. 90, 11741–11745. doi: 10.1021/acs.analchem.8b02801
Seeley, E. H., and Caprioli, R. M. (2008). Molecular imaging of proteins in tissues by mass spectrometry. Proc. Natl. Acad. Sci. U.S.A. 105:18126.
Sethi, M. K., Downs, M., Shao, C., Hackett, W. E., Phillips, J. J., and Zaia, J. (2022). In-depth matrisome and glycoproteomic analysis of human brain glioblastoma versus control tissue. Mol. Cell. Proteomics 21:100216. doi: 10.1016/j.mcpro.2022
Shajahan, A., Heiss, C., Ishihara, M., and Azadi, P. (2017). Glycomic and glycoproteomic analysis of glycoproteins-a tutorial. Anal. Bioanal. Chem. 409, 4483–4505. doi: 10.1007/s00216-017-0406-7
Sharma, N., Rao, S. P., and Kalivendi, S. V. (2019). The deglycase activity of DJ-1 mitigates alpha-synuclein glycation and aggregation in dopaminergic cells: Role of oxidative stress mediated downregulation of DJ-1 in Parkinson’s disease. Free Radic. Biol. Med. 135, 28–37. doi: 10.1016/j.freeradbiomed.2019.02.014
Shi, M., Caudle, W. M., and Zhang, J. (2009). Biomarker discovery in neurodegenerative diseases: A proteomic approach. Neurobiol. Dis. 35, 157–164.
Shi, M., Movius, J., Dator, R., Aro, P., Zhao, Y., Pan, C., et al. (2015). Cerebrospinal fluid peptides as potential Parkinson disease biomarkers: A staged pipeline for discovery and validation. Mol. Cell. Proteomics 14, 544–555. doi: 10.1074/mcp.M114.040576
Shi, Y., Li, Z., Felder, M. A., Yu, Q., Shi, X., Peng, Y., et al. (2019). Mass spectrometry imaging of N-glycans from formalin-fixed paraffin-embedded tissue sections using a novel subatmospheric pressure ionization source. Anal. Chem. 91:12942. doi: 10.1021/acs.analchem.9b02995
Shimura, H., Schlossmacher, M. G., Hattori, N., Frosch, M. P., Trockenbacher, A., Schneider, R., et al. (2001). Ubiquitination of a new form of alpha-synuclein by parkin from human brain: Implications for Parkinson’s disease. Science. 293, 263–269.
Shubhakar, A., Kozak, R. P., Reiding, K. R., Royle, L., Spencer, D. I., Fernandes, D. L., et al. (2016). Automated high-throughput permethylation for glycosylation analysis of biologics using MALDI-TOF-MS. Anal. Chem. 88, 8562–8569.
Smilowitz, J. T., Totten, S. M., Huang, J., Grapov, D., Durham, H. A., Lammi-Keefe, C. J., et al. (2013). Human milk secretory immunoglobulin a and lactoferrin N-glycans are altered in women with gestational diabetes mellitus. J. Nutr. 143, 1906–1912. doi: 10.3945/jn.113.180695
Snyder, C. M., Zhou, X., Karty, J. A., Fonslow, B. R., Novotny, M. V., and Jacobson, S. C. (2017). Capillary electrophoresis-mass spectrometry for direct structural identification of serum N-glycans. J. Chromatogr. A 1523, 127–139. doi: 10.1016/j.chroma.2017.09.009
Soltwisch, J., Kettling, H., Vens-Cappell, S., Wiegelmann, M., Müthing, J., and Dreisewerd, K. (2015). Mass spectrometry imaging with laser-induced postionization. Science 348, 211–215.
Song, W., Zhou, X., Benktander, J. D., Gaunitz, S., Zou, G., Wang, Z., et al. (2019). In-depth compositional and structural characterization of N-GLYCANS DERIVED FROM HUMAN URINARY EXosomes. Anal. Chem. 91, 13528–13537. doi: 10.1021/acs.analchem.9b02620
Stahl, B., Steup, M., Karas, M., and Hillenkamp, F. (1991). Analysis of neutral oligosaccharides by matrix-assisted laser desorption – ionization mass-spectrometry. Anal. Chem. 63, 1463–1466.
Stahl, B., Thurl, S., Zeng, J. R., Karas, M., Hillenkamp, F., Steup, M., et al. (1994). Oligosaccharides from human-milk as revealed by matrix-assisted laser-desorption ionization mass-spectrometry. Anal. Biochem. 223, 218–226.
Stanback, A. E., Conroy, L. R., Young, L. E. A., Hawkinson, T. R., Markussen, K. H., Clarke, H. A., et al. (2021). Regional N-glycan and lipid analysis from tissues using MALDI-mass spectrometry imaging. STAR Protoc. 2:100304. doi: 10.1016/j.xpro.2021
Stanta, J. L., Saldova, R., Struwe, W. B., Byrne, J. C., Leweke, F. M., Rothermund, M., et al. (2010). Identification of N-glycosylation changes in the CSF and serum in patients with schizophrenia. J. Proteome Res. 9, 4476–4489.
Stavenhagen, K., Kolarich, D., and Wuhrer, M. (2015). Clinical glycomics employing graphitized carbon liquid chromatography-mass spectrometry. Chromatographia 78, 307–320. doi: 10.1007/s10337-014-2813-7
Stolz, A., Jooß, K., Höcker, O., Römer, J., Schlecht, J., and Neusüß, C. (2019). Recent advances in capillary electrophoresis-mass spectrometry: Instrumentation, methodology and applications. Electrophoresis 40, 79–112. doi: 10.1002/elps.201800331
Struwe, W. B., Pagel, K., Benesch, J. L. P., Harvey, D. J., and Campbell, M. P. (2016). GlycoMob: an ion mobility-mass spectrometry collision cross section database for glycomics. Glycoconj. J. 33, 399–404. doi: 10.1007/s10719-015-9613-7
Super, N., Ahuja, R., and Proff, K. (2019). Reducing the cost and risk of dementia recommendations to improve brain health and decrease disparities. Santa Monica, CA: Milken Institute.
Suzuki, N., Abe, T., and Natsuka, S. (2019). Quantitative LC-MS and MS/MS analysis of sialylated glycans modified by linkage-specific alkylamidation. Anal. Biochem. 567, 117–127. doi: 10.1016/j.ab.2018.11.014
Szabo, Z., Guttman, A., Rejtar, T., and Karger, B. L. (2010). Improved sample preparation method for glycan analysis of glycoproteins by CE-LIF and CE-MS. Electrophoresis 31, 1389–1395. doi: 10.1002/elps.201000037
Szabo, Z., Thayer, J. R., Agroskin, Y., Lin, S., Liu, Y., Srinivasan, K., et al. (2017). In-depth analyses of native N-linked glycans facilitated by high-performance anion exchange chromatography-pulsed amperometric detection coupled to mass spectrometry. Anal. Bioanal. Chem. 409, 3089–3101. doi: 10.1007/s00216-017-0248-3
Tan, Y., and Polfer, N. C. (2015). Linkage and anomeric differentiation in trisaccharides by sequential fragmentation and variable-wavelength infrared photodissociation. J. Am. Soc. Mass Spectrom. 26, 359–368. doi: 10.1007/s13361-014-1025-6
Tang, Y., Pu, Y., Gao, J., Hong, P., Costello, C. E., and Lin, C. (2018a). De novo glycan sequencing by electronic excitation dissociation and fixed-charge derivatization. Anal. Chem. 90, 3793–3801. doi: 10.1021/acs.analchem.7b04077
Tang, Y., Wei, J., Costello, C. E., and Lin, C. (2018b). Characterization of isomeric glycans by reversed phase liquid chromatography-electronic excitation dissociation tandem mass spectrometry. J. Am. Soc. Mass Spectrom. 29, 1295–1307. doi: 10.1007/s13361-018-1943-9
Ten Hagen, K. G., Bedi, G. S., Tetaert, D., Kingsley, P. D., Hagen, F. K., Balys, M. M., et al. (2001). Cloning and characterization of a ninth member of the UDP-GalNAc: polypeptide N-acetylgalactosaminyltransferase family, ppGaNTase-T9. J. Biol. Chem. 276, 17395–17404. doi: 10.1074/jbc.M009638200
Terkelsen, T., Haakensen, V. D., Saldova, R., Gromov, P., Hansen, M. K., Stockmann, H., et al. (2018). N-glycan signatures identified in tumor interstitial fluid and serum of breast cancer patients: Association with tumor biology and clinical outcome. Mol. Oncol. 12, 972–990. doi: 10.1002/1878-0261.12312
Tobias, F., and Hummon, A. B. (2020). Considerations for MALDI-based quantitative mass spectrometry imaging studies. J. Proteome Res. 19, 3620–3630. doi: 10.1021/acs.jproteome.0c00443
Toghi Eshghi, S., Yang, S., Wang, X., Shah, P., Li, X., and Zhang, H. (2014). Imaging of N-linked glycans from formalin-fixed paraffin-embedded tissue sections using MALDI mass spectrometry. ACS Chem. Biol. 9, 2149–2156. doi: 10.1021/cb500405h
Tu, C., Li, J., Shen, S., Sheng, Q., Shyr, Y., and Qu, J. (2016). Performance investigation of proteomic identification by HCD/CID fragmentations in combination with high/low-resolution detectors on a tribrid, high-field orbitrap instrument. PLoS One 11:e0160160. doi: 10.1371/journal.pone
Tucholski, J., Simmons, M. S., Pinner, A. L., Haroutunian, V., McCullumsmith, R. E., and Meador-Woodruff, J. H. (2013). Abnormal N-linked glycosylation of cortical AMPA receptor subunits in schizophrenia. Schizophr. Res. 146, 177–183. doi: 10.1016/j.schres.2013.01.031
Tudor, L., Nedic Erjavec, G., Nikolac Perkovic, M., Konjevod, M., Svob Strac, D., Uzun, S., et al. (2019). N-glycomic profile in combat related post-traumatic stress disorder. Biomolecules 9:834. doi: 10.3390/biom9120834
Unterieser, I., and Mischnick, P. (2011). Labeling of oligosaccharides for quantitative mass spectrometry. Carbohydr. Res. 346, 68–75.
Vanguilder, H. D., and Freeman, W. M. (2011). The hippocampal neuroproteome with aging and cognitive decline: Past progress and future directions. Front. Aging Neurosci. 3:8. doi: 10.3389/fnagi.2011.00008
Vanoni, O., Paganetti, P., and Molinari, M. (2008). Consequences of individual N-glycan deletions and of proteasomal inhibition on secretion of active BACE. Mol. Biol. Cell 19, 4086–4098. doi: 10.1091/mbc.e08-05-0459
Váradi, C., Nehéz, K., Hornyák, O., Viskolcz, B., and Bones, J. (2019). Serum N-glycosylation in Parkinson’s disease: A novel approach for potential alterations. Molecules 24:2220. doi: 10.3390/molecules24122220
Vicente Miranda, H., Szego, E. M., Oliveira, L. M. A., Breda, C., Darendelioglu, E., de Oliveira, R. M., et al. (2017). Glycation potentiates alpha-synuclein-associated neurodegeneration in synucleinopathies. Brain 140, 1399–1419.
Vitaliano, P. P., Zhang, J., and Scanlan, J. M. (2003). Is caregiving hazardous to one’s physical health? A meta-analysis. Psychol. Bull. 129, 946–972. doi: 10.1037/0033-2909.129.6.946
Vitiazeva, V., Kattla, J. J., Flowers, S. A., Lindén, S. K., Premaratne, P., Weijdegård, B., et al. (2015). The O-linked glycome and blood group antigens ABO on mucin-type glycoproteins in mucinous and serous epithelial ovarian tumors. PLoS One 10:e0130197. doi: 10.1371/journal.pone.0130197
Walker, S. H., Lilley, L. M., Enamorado, M. F., Comins, D. L., and Muddiman, D. C. (2011). Hydrophobic derivatization of N-linked glycans for increased ion abundance in electrospray ionization mass spectrometry. J. Am. Soc. Mass Spectrom. 22, 1309–1317. doi: 10.1007/s13361-011-0140-x
Wang, X., Deng, Z., Huang, C., Zhu, T., Lou, J., Wang, L., et al. (2018). Differential N-glycan patterns identified in lung adenocarcinoma by N-glycan profiling of formalin-fixed paraffin-embedded (FFPE) tissue sections. J. Proteomics 172, 1–10. doi: 10.1016/j.jprot.2017.11.010
Warnke, S., Ben Faleh, A., Scutelnic, V., and Rizzo, T. R. (2019). Separation and identification of glycan anomers using ultrahigh-resolution ion-mobility spectrometry and cryogenic ion spectroscopy. J. Am. Soc. Mass Spectrom. 30, 2204–2211. doi: 10.1007/s13361-019-02333-0
Wei, J., Tang, Y., Bai, Y., Zaia, J., Costello, C. E., Hong, P., et al. (2020a). Toward automatic and comprehensive glycan characterization by online PGC-LC-EED MS/MS. Anal. Chem. 92, 782–791. doi: 10.1021/acs.analchem.9b03183
Wei, J., Tang, Y., Ridgeway, M. E., Park, M. A., Costello, C. E., and Lin, C. (2020b). Accurate identification of isomeric glycans by trapped ion mobility spectrometry-electronic excitation dissociation tandem mass spectrometry. Anal. Chem. 92, 13211–13220. doi: 10.1021/acs.analchem.0c02374
West, C. A., Liang, H., Drake, R. R., and Mehta, A. S. (2020). New enzymatic approach to distinguish fucosylation isomers of N-linked glycans in tissues using MALDI imaging mass spectrometry. J. Proteome Res. 19, 2989–2996. doi: 10.1021/acs.jproteome.0c00024
Wheeler, S. F., Domann, P., and Harvey, D. J. (2009). Derivatization of sialic acids for stabilization in matrix-assisted laser desorption/ionization mass spectrometry and concomitant differentiation of alpha(2 –> 3)- and alpha(2 –> 6)-isomers. Rapid Commun. Mass Spectrom. 23, 303–312. doi: 10.1002/rcm.3867
Wildburger, N. C., Zhou, S., Zacharias, L. G., Kroes, R. A., Moskal, J. R., Schmidt, M., et al. (2015). Integrated transcriptomic and glycomic profiling of glioma stem cell xenografts. J. Proteome Res. 14, 3932–3939. doi: 10.1021/acs.jproteome.5b00549
Wilkinson, H., Thomsson, K. A., Rebelo, A. L., Hilliard, M., Pandit, A., Rudd, P. M., et al. (2021). The O-glycome of human nigrostriatal tissue and its alteration in Parkinson’s disease. J. Proteome Res. 20, 3913–3924. doi: 10.1021/acs.jproteome.1c00219
Wilson, D. F., and Massey, W. (1987). Scanning electron microscopy of incinerated teeth. Am. J. Forensic Med. Pathol. 8, 32–38.
Witters, P., Honzik, T., Bauchart, E., Altassan, R., Pascreau, T., Bruneel, A., et al. (2019). Long-term follow-up in PMM2-CDG: Are we ready to start treatment trials? Genet. Med. 21, 1181–1188. doi: 10.1038/s41436-018-0301-4
Wuhrer, M., Koeleman, C. A., Hokke, C. H., and Deelder, A. M. (2004). Nano-scale liquid chromatography-mass spectrometry of 2-aminobenzamide-labeled oligosaccharides at low femtomole sensitivity. Int. J. Mass Spectrom. 232, 51–57.
Xia, B., Zhang, W., Li, X., Jiang, R., Harper, T., Liu, R., et al. (2013). Serum N-glycan and O-glycan analysis by mass spectrometry for diagnosis of congenital disorders of glycosylation. Anal. Biochem. 442, 178–185. doi: 10.1016/j.ab.2013.07.037
Xie, C., Wu, Q., Zhang, S., Wang, C., Gao, W., Yu, J., et al. (2020). Improving glycan isomeric separation via metal ion incorporation for drift tube ion mobility-mass spectrometry. Talanta 211:120719. doi: 10.1016/j.talanta.2020
Xie, Y., and Butler, M. (2022). Construction of an instant PC-derivatized glycan glucose unit database: A foundation work for high-throughput and high-sensitivity glycomic analysis. Glycobiology 32, 289–303. doi: 10.1093/glycob/cwab128
Xu, G., Goonatilleke, E., Wongkham, S., and Lebrilla, C. B. (2020). Deep structural analysis and quantitation of O-linked glycans on cell membrane reveal high abundances and distinct glycomic profiles associated with cell type and stages of differentiation. Anal. Chem. 92, 3758–3768. doi: 10.1021/acs.analchem.9b05103
Yalovenko, N., Yatsyna, V., Bansal, P., AbiKhodr, A. H., and Rizzo, T. R. (2020). Analyzing glycans cleaved from a biotherapeutic protein using ultrahigh-resolution ion mobility spectrometry together with cryogenic ion spectroscopy. Analyst 145, 6493–6499. doi: 10.1039/d0an01206h
Yang, D. S., Tandon, A., Chen, F., Yu, G., Yu, H., Arawaka, S., et al. (2002). Mature glycosylation and trafficking of nicastrin modulate its binding to presenilins. J. Biol. Chem. 277, 28135–28142.
Yang, E., Dufresne, M., and Chaurand, P. (2019). Enhancing ganglioside species detection for MALDI-TOF imaging mass spectrometry in negative reflectron mode. Int. J. Mass Spectrom. 437:3.
Yang, X., and Qian, K. (2017). Protein O-GlcNAcylation: Emerging mechanisms and functions. Nat. Rev. Mol. Cell Biol. 18, 452–465. doi: 10.1038/nrm.2017.22
Youings, A., Chang, S. C., Dwek, R. A., and Scragg, I. G. (1996). Site-specific glycosylation of human immunoglobulin G is altered in four rheumatoid arthritis patients. Biochem. J. 314(Pt 2), 621–630. doi: 10.1042/bj3140621
Yu, G., Nishimura, M., Arawaka, S., Levitan, D., Zhang, L., Tandon, A., et al. (2000). Nicastrin modulates presenilin-mediated notch/glp-1 signal transduction and betaAPP processing. Nature 407, 48–54. doi: 10.1038/35024009
Yu, X., Jiang, Y., Chen, Y., Huang, Y., Costello, C. E., and Lin, C. (2013). Detailed glycan structural characterization by electronic excitation dissociation. Anal. Chem. 85, 10017–10021.
Zhang, H., Shi, X., Vu, N. Q., Li, G., Li, Z., Shi, Y., et al. (2020a). On-Tissue Derivatization with Girard’s reagent P enhances N-glycan signals for formalin-fixed paraffin-embedded tissue sections in MALDI mass spectrometry imaging. Anal. Chem. 92, 13361–13368. doi: 10.1021/acs.analchem.0c02704
Zhang, J., Goodlett, D. R., and Montine, T. J. (2005). Proteomic biomarker discovery in cerebrospinal fluid for neurodegenerative diseases. J. Alzheimers Dis. 8, 377–386.
Zhang, T., Madunić, K., Holst, S., Zhang, J., Jin, C., Ten Dijke, P., et al. (2020b). Development of a 96-well plate sample preparation method for integrated N- and O-glycomics using porous graphitized carbon liquid chromatography-mass spectrometry. Mol. Omics. 16, 355–363. doi: 10.1039/c9mo00180h
Zhang, Y. W., Thompson, R., Zhang, H., and Xu, H. (2011). APP processing in Alzheimer’s disease. Mol. Brain 4:3.
Zhang, Y., Wang, B., Jin, W., Wen, Y., Nan, L., Yang, M., et al. (2019). Sensitive and robust MALDI-TOF-MS glycomics analysis enabled by Girard’s reagent T on-target derivatization (GTOD) of reducing glycans. Anal. Chim. Acta 1048, 105–114. doi: 10.1016/j.aca.2018.10.015
Zhong, X., Chen, Z., Snovida, S., Liu, Y., Rogers, J. C., and Li, L. (2015). Capillary electrophoresis-electrospray ionization-mass spectrometry for quantitative analysis of glycans labeled with multiplex carbonyl-reactive tandem mass tags. Anal. Chem. 87, 6527–6534. doi: 10.1021/acs.analchem.5b01835
Zhou, S., Dong, X., Veillon, L., Huang, Y., and Mechref, Y. (2017a). LC-MS/MS analysis of permethylated N-glycans facilitating isomeric characterization. Anal. Bioanal. Chem. 409, 453–466. doi: 10.1007/s00216-016-9996-8
Zhou, S., Hu, Y., and Mechref, Y. (2016). High-temperature LC-MS/MS of permethylated glycans derived from glycoproteins. Electrophoresis 37, 1506–1513.
Zhou, S., Hu, Y., DeSantos-Garcia, J. L., and Mechref, Y. (2015). Quantitation of permethylated N-glycans through multiple-reaction monitoring (MRM) LC-MS/MS. J. Am. Soc. Mass Spectrom. 26, 596–603. doi: 10.1007/s13361-014-1054-1
Zhou, S., Huang, Y., Dong, X., Peng, W., Veillon, L., Kitagawa, D. A. S., et al. (2017b). Isomeric separation of permethylated glycans by porous graphitic carbon (PGC)-LC-MS/MS at high temperatures. Anal. Chem. 89, 6590–6597.
Zhou, S., Veillon, L., Dong, X., Huang, Y., and Mechref, Y. (2017c). Direct comparison of derivatization strategies for LC-MS/MS analysis of N-glycans. Analyst 142, 4446–4455. doi: 10.1039/c7an01262d
Zhou, W., and Håkansson, K. (2013). Electron capture dissociation of divalent metal-adducted sulfated N-glycans released from bovine thyroid stimulating hormone. J. Am. Soc. Mass Spectrom. 24, 1798–1806. doi: 10.1007/s13361-013-0700-3
Zhu, J., Lin, Z., Wu, J., Yin, H., Dai, J., Feng, Z., et al. (2014). Analysis of serum haptoglobin fucosylation in hepatocellular carcinoma and liver cirrhosis of different etiologies. J. Proteome Res. 13, 2986–2997. doi: 10.1021/pr500128t
Zubair, F., Laibinis, P. E., Swisher, W. G., Yang, J., Spraggins, J. M., Norris, J. L., et al. (2016). Trypsin and MALDI matrix pre-coated targets simplify sample preparation for mapping proteomic distributions within biological tissues by imaging mass spectrometry. J. Mass Spectrom. 51:1168. doi: 10.1002/jms.3888
Keywords: mass spectrometry (MS), glycomics, nervous system diseases, glycan markers, LC-MS/MS
Citation: Peng W, Kobeissy F, Mondello S, Barsa C and Mechref Y (2022) MS-based glycomics: An analytical tool to assess nervous system diseases. Front. Neurosci. 16:1000179. doi: 10.3389/fnins.2022.1000179
Received: 21 July 2022; Accepted: 05 October 2022;
Published: 03 November 2022.
Edited by:
Harry Pantazopoulos, University of Mississippi Medical Center, United StatesReviewed by:
Kimberly Michele Alonge, University of Washington, United StatesFriedrich Altmann, University of Natural Resources and Life Sciences, Austria
Copyright © 2022 Peng, Kobeissy, Mondello, Barsa and Mechref. This is an open-access article distributed under the terms of the Creative Commons Attribution License (CC BY). The use, distribution or reproduction in other forums is permitted, provided the original author(s) and the copyright owner(s) are credited and that the original publication in this journal is cited, in accordance with accepted academic practice. No use, distribution or reproduction is permitted which does not comply with these terms.
*Correspondence: Yehia Mechref, yehia.mechref@ttu.edu