- Neurorestoration Group, Wolfson Centre for Age-Related Diseases, King’s College London, London, United Kingdom
The dorsal root ganglia (DRG) and trigeminal ganglia (TG) are clusters of cell bodies of highly specialized sensory neurons which are responsible for relaying information about our environment to the central nervous system. Despite previous efforts to characterize sensory neurons at the molecular level, it is still unknown whether those present in DRG and TG have distinct expression profiles and therefore a unique molecular fingerprint. To address this question, we isolated lumbar DRG and TG neurons using fluorescence-activated cell sorting from Advillin-GFP transgenic mice and performed RNA sequencing. Our transcriptome analyses showed that, despite being overwhelmingly similar, a number of genes are differentially expressed in DRG and TG neurons. Importantly, we identified 24 genes which were uniquely expressed in either ganglia, including an arginine vasopressin receptor and several homeobox genes, giving each population a distinct molecular fingerprint. We compared our findings with published studies to reveal that many genes previously reported to be present in neurons are in fact likely to originate from other cell types in the ganglia. Additionally, our neuron-specific results aligned well with a dataset examining whole human TG and DRG. We propose that the data can both improve our understanding of primary afferent biology and help contribute to the development of drug treatments and gene therapies which seek targets with unique or restricted expression patterns.
Introduction
Among the different types of cells present in the central (CNS) and peripheral (PNS) nervous systems, sensory neurons are of particular importance as they are continually relaying information about our environment. The trigeminal ganglion (TG) contains many of the sensory neurons innervating the head while the dorsal root ganglia (DRG) mostly innervate the rest of the body. Both groups are highly specialized pseudounipolar neurons that can detect and respond to a variety of chemical, mechanical, and thermal stimuli, serving as a warning system for mammals (Porter and Spencer, 1982; Luo et al., 1991; Capra and Dessem, 1992; Devor, 1999; Woolf and Ma, 2007; Dubin and Patapoutian, 2010). TG neurons, in addition, have some distinct chemosensitive properties relating to olfaction and the gustatory system (Gerhold and Bautista, 2009; Viana, 2011).
DRG and TG are formed by agglomerates of many thousands of primary afferent neurons. There is large heterogeneity, whereby different types of neurons have their own special perceptual modalities and therefore distinct cellular and molecular identities (Snider and McMahon, 1998; Devor, 1999; Marmigere and Ernfors, 2007; Dubin and Patapoutian, 2010; Reichling et al., 2013). Despite their functional similarities and capability to sense innocuous and noxious stimuli, DRG and TG neurons are very distinct in their location and connectivity. DRG afferents are found peripherally alongside the spinal cord and directly synapse onto spinal neurons, whereas TG neurons are localized at the base of the skull, projecting either directly to the brain stem or to the upper regions of the spinal cord (Capra and Dessem, 1992; Erzurumlu et al., 2010). Moreover, DRG neurons derive from the neural crest, whereas TG neurons have dual origin, containing cells originated both from cranial neural crest and trigeminal ectodermal placodes (Altman and Bayer, 1982; D’Amico-Martel and Noden, 1983; Durham and Garrett, 2010; Erzurumlu et al., 2010; Krispin et al., 2010). The distinction in cell fate at embryonic stages, together with some of the already mentioned exclusive functional characteristics and different connectivity patterns, suggests the existence of different molecular identities underlying either of these ganglia.
Studies have indeed started to characterize DRG and TG neurons at the molecular level using RNA sequencing (RNA-seq) (Manteniotis et al., 2013; Chiu et al., 2014; Perkins et al., 2014; Thakur et al., 2014; Flegel et al., 2015; Reynders et al., 2015; Hu et al., 2016; Sapio et al., 2016; Wu et al., 2016; Kogelman et al., 2017) enabling in-depth analysis and characterization of their genome-wide transcriptional profiles. To date a few studies have reported approximately 200 differentially expressed genes in both rodents and humans (Manteniotis et al., 2013; Flegel et al., 2015; Kogelman et al., 2017). Among them were many odorant-binding proteins, ion channels, and G protein-coupled receptors (Manteniotis et al., 2013; Flegel et al., 2015). However, non-neuronal cells account for a significant proportion of cells in these ganglia (Devor, 1999; Hanani, 2005; Durham and Garrett, 2010; Huang et al., 2013) and therefore influence the transcripts present in these datasets, with neuronal genes underrepresented. Thus, a comparison in the expression profiles between enriched neuronal cell populations remains to be performed.
In this study, we examined lumbar DRG and TG ganglia with a specific focus on differences in neuronal gene expression. Using a combination of fluorescence-activated cell sorting (FACS), a transgenic mouse model and RNA-seq analysis, we identified a number of genes exclusively expressed in either DRG or TG neurons. Furthermore, we correlated our data with published studies and reveal that many genes previously believed to be present in neurons, are in fact either expressed in glial cells or other cell types. These findings are not only important for the understanding of the biology of primary afferents as whole, but could also aid development of drug treatments and gene therapies which seek unequivocal targets.
Results
Isolation of Specific Populations of Neurons by FACS
We have previously demonstrated that conventional DRG dissociation results in a mixture of cells in which neurons only account for ∼10% of the population (Thakur et al., 2014). In this study we used a sorting strategy, employing a mouse line expressing GFP under the control of the advillin promotor (Avil-GFP), which labels sensory neurons (Gong et al., 2003) (Figure 1A and Supplementary Figure 1). Lumbar DRG and TG were dissociated, the cells were exposed to propidium iodide (PI) and isolated using FACS (Figure 1B; for gating strategies, please refer to section “Materials and Methods” and Supplementary Figure 2). From all GFP+/PI- singlets, 500 events were captured for each biological replicate consisting of n = 6 mice for DRG and n = 4 mice for TG. Culture and analysis of sorted cells revealed that despite limited satellite glial cells, neurons accounted for over 70% of our isolated population (data not shown), a percentage we estimate to be slightly undervalued, given the proliferating nature of non-neuronal cells.
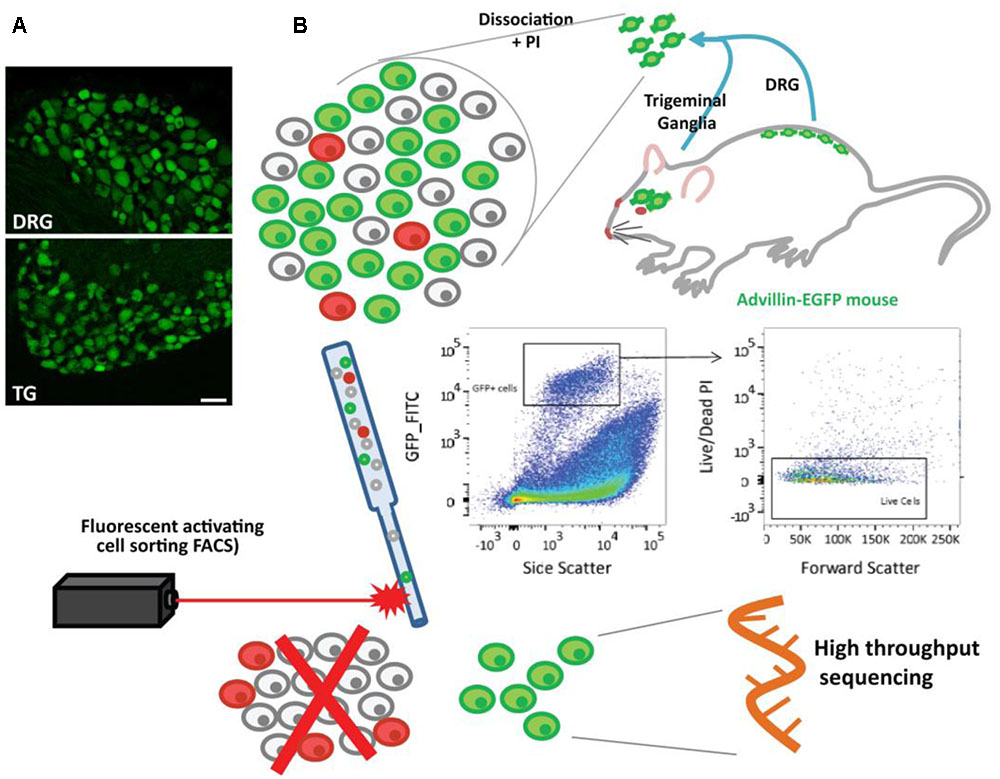
FIGURE 1. Sensory neurons in the Avil-GFP transgenic mouse and schematic representation of neuronal isolation using flow cytometry. (A) Representative picture of DRG and TG ganglion showing the expression of EGFP under the control of the advillin promoter (green cells) in sensory neurons. Scale bar: 50 μm. (B) Lumbar DRG and TG were extracted from Avil-EGFP mice. Neurons were dissociated and exposed to propidium iodide (PI), and EGFP+ cells (neurons) were sorted using the gating strategy above, where the non-neuronal cells and PI+ cells were excluded (represented in the cartoon as gray and red). RNA was then extracted and sequenced.
RNA-Sequencing of DRG and TG Neurons
Following the isolation of neurons by FACS, high-quality RNA was extracted (average RIN 8.75, min 7.6, max 9.9) and RNA-seq was performed on polyA+ mRNA using six DRG and four TG replicates. Between 20.7 and 30.3 million reads were uniquely aligned to the mm10 genome using STAR (Dobin et al., 2013), corresponding to a median mapping percentage of 85.8%. Normalized gene expression values and differential gene expression were generated using the cufflinks and DESeq2 algorithms (Trapnell et al., 2010; Love et al., 2014), respectively. Power calculations (using RNAPower; Hart et al., 2013) indicated that we were well-powered to detect twofold differences in gene expression (71% chance for all genes and 81–87% chance for the top 50% of all expressed genes).
A total of 12,638 genes were detected in either DRG or TG neurons (FPKM > 1, in at least two of the replicates for each condition, Supplementary Table 1). Our DRG and TG data were strongly correlated with the ranked expression profiles of pure DRG nociceptors (Thakur et al., 2014): Spearman’s rho rs = 0.65, Figure 2 and Supplementary Table 2. Correlations are very sensitive to library batch effects and further depend on the number and expression levels of the gene sets included in the analysis. We therefore also conducted presence/absence calls, which again showed good overlap: 80% of the top 8000 genes were identified as present in both our current RNA-seq study and the DRG nociceptors dataset (Supplementary Table 2).
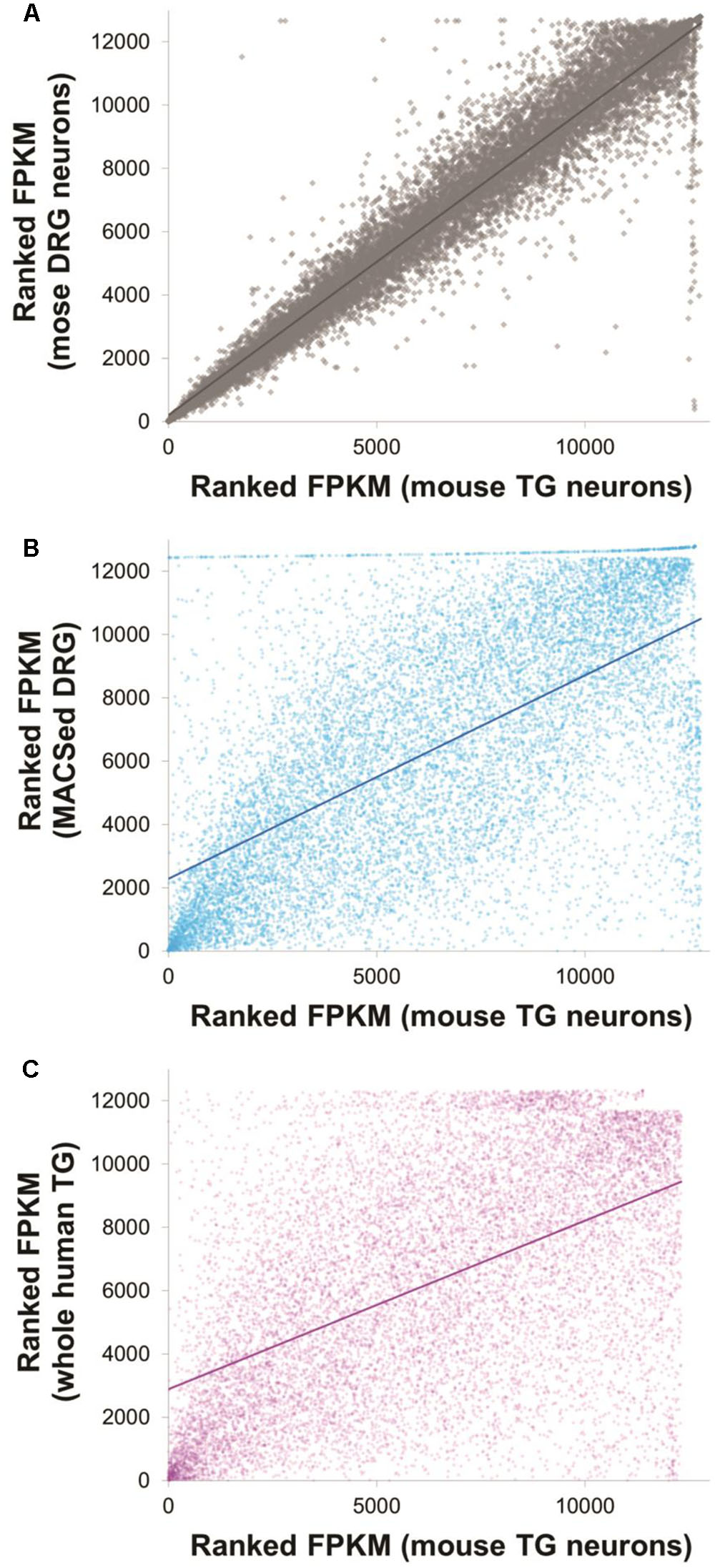
FIGURE 2. Expression profiles of mouse trigeminal neurons were well correlated with other, previously published datasets. Ranked FPKM values for mouse TG neurons obtained in this dataset are plotted against ranked FPKM values of three different comparison datasets: mouse DRG neurons obtained as part of this particular study (A, Spearman’s rs = 0.97), magnetically sorted DRG neurons obtained by Thakur et al. (2014) (B, Spearman’s rs = 0.64) and whole human TG data obtained by Flegel et al. (2015) (C, Spearman’s rs = 0.53). Solid lines indicate linear correlations. Note that within study correlations are necessarily higher than between study correlations due to the absence of technical batch effects stemming, e.g., from separate library preparation. See Supplementary Table 2 for the underlying data and for additional graphs of presence/absence calls.
Next, we looked for differences between our DRG and TG samples. DESeq2 analysis revealed 97 genes that were differentially expressed at adjusted p-value (adj. p) < 0.05 (Figures 3A,B), which means that the great majority of genes (more than 99%) were commonly expressed. Of the genes which were different, 44 were upregulated in DRG neurons and 19 were upregulated in TG neurons, with 24 of the genes being present exclusively in either ganglia (Table 1). Surprisingly, the vast majority of the latter were homeobox genes (Hox), accounting for ∼50% of the upregulated genes in the DRG (Figure 3B and Table 1). Further to these neuronal genes, an additional 34 genes emerging from DESeq2 analysis were either not deemed to be expressed according to our FPKM expression cut-off or were not of unambiguously neuronal origin (Supplementary Table 1). The latter was determined by using a previously published RNA-seq dataset (Thakur et al., 2014) in which DRG neurons were magnetically sorted, which prevents any contamination with satellite glial cells (see instruction sheet/tab of Supplementary Table 1 for further information).
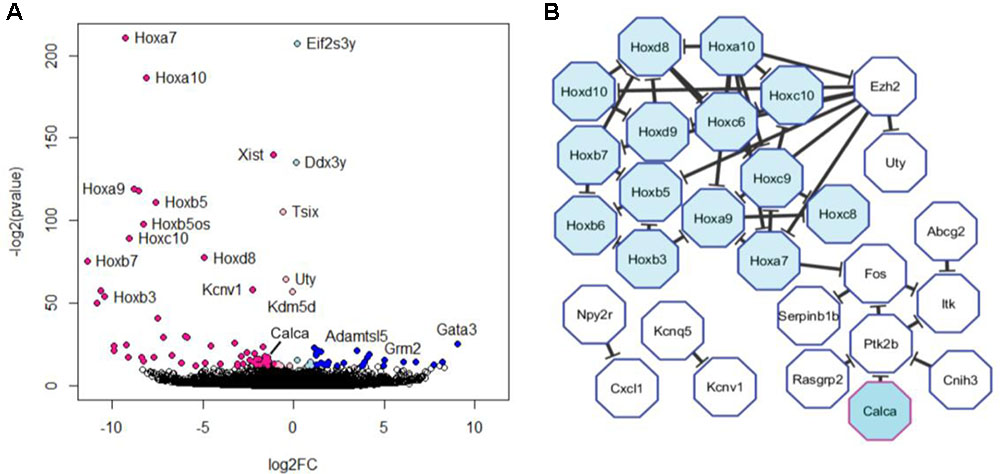
FIGURE 3. Differential expression of trigeminal versus dorsal root ganglion transcripts. (A) Volcano plot of differentially expressed genes obtained using Deseq2. Colored dots represent genes that were significantly dysregulated at FDR < 0.05. Genes upregulated in trigeminal ganglion are depicted in light (log2FC > 0) and dark blue (log2FC > 1). Genes upregulated in dorsal root ganglion are depicted in light (log2FC < 0) and dark pink (log2FC < –1). Note that this graph includes genes which are not expressed according to our cut-off as well as those which are enriched in satellite glial cells (see Supplementary Table 1). (B) The 44 unambiguously neuronal genes that were found to be upregulated in dorsal root ganglion at FDR < 0.05 were fed into a protein–protein interaction analysis. The resulting network is depicted here and includes 28 genes, 50% of which were Hox genes.
Validation of Differentially Expressed Genes
Given the identification of several differentially expressed genes between DRG and TG neurons in our dataset, we next went on to validate genes with potential functional relevance to pain states. Calcitonin gene-related peptide—CGRP (encoded by the gene CALCA)—is primarily expressed by sensory neurons and known to play a key role in inflammation and neuronal sensitization, particularly during nerve damage (Donnerer and Stein, 1992; Benemei et al., 2009; Yu et al., 2009; Russell et al., 2014) as well as in migraine (Diener et al., 2015; Karsan and Goadsby, 2015; Edvinsson, 2017; Iyengar et al., 2017). Our transcriptome analysis revealed a large difference of approximately 2.5-fold increased expression of the CGRP gene in DRG in relation to TG neurons (AVRG FPKM DRG: 8216 and TG: 3430; adj. p < 0.05; Figure 4A). To validate this finding we performed a CGRP ELISA from samples extracted from DRG and TG neurons. Results showed that whole DRG has significantly higher level of CGRP protein (Figure 4B); indeed, total CGRP levels were over threefold higher when compared to TG (n = 4/group; p < 0.01; Figure 4B). We also carried out qPCR, where we showed a higher amount of CGRP mRNA in the DRG in an independent cohort of animals (n = 3 DRG; n = 4 TG; p = 0.056; Figure 5A).
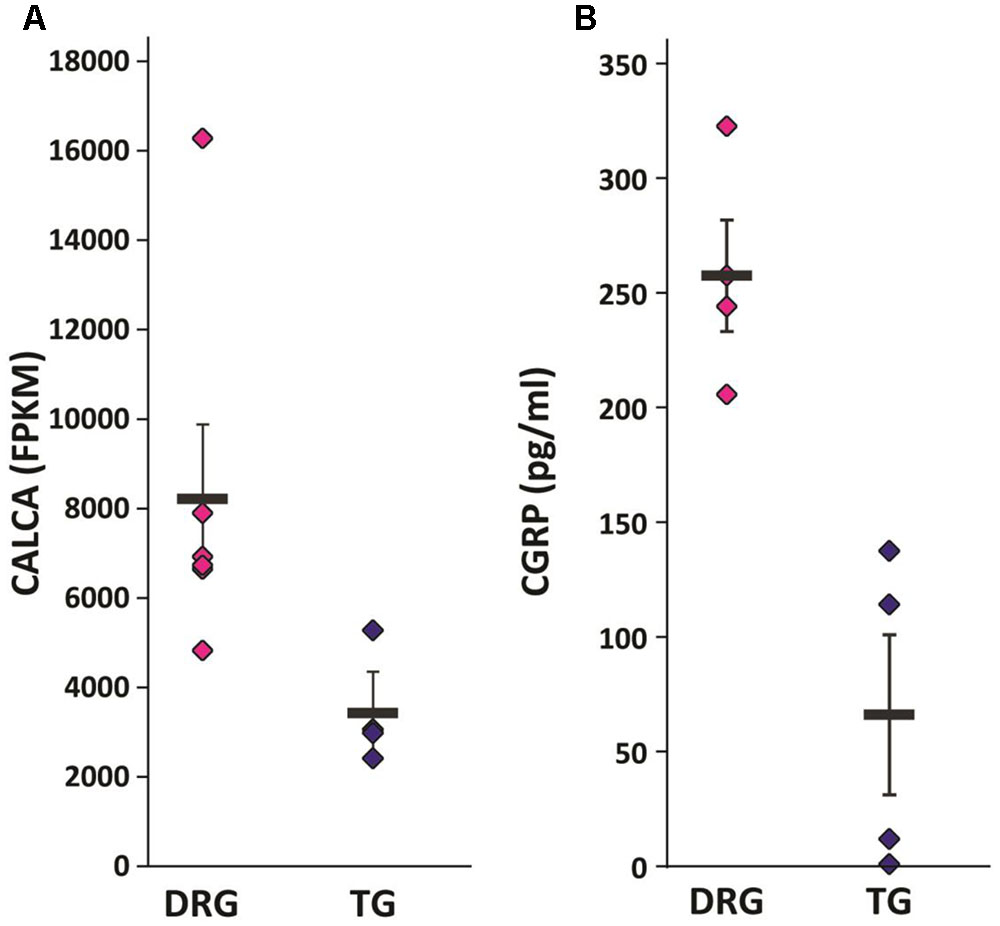
FIGURE 4. Validation of selected targets. (A) CGRP mRNA (Calca) was found to be significantly upregulated in our RNA-seq dataset. Plotted here are individual FPKM values, including means and standard errors of the means. (B) CGRP ELISA showed significantly increased protein levels in the DRG versus the TG of a separate cohort of mice: n = 4, independent samples t-test (two-tailed, heteroscedastic), p = 0.005.
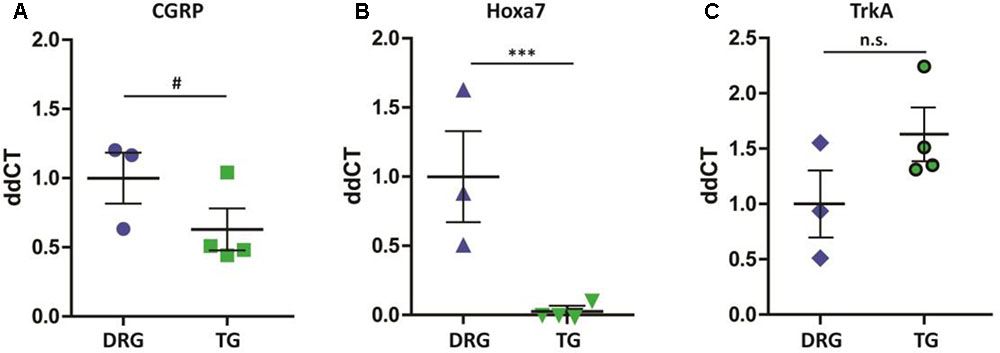
FIGURE 5. qPCR validation of differentially expressed genes. (A) Similarly to the RNA-seq dataset, CGRP mRNA (Calca) was higher in the DRG when compared to TG (#p = 0.056). (B) Hoxa7 gene is exclusively expressed in DRG neurons (∗∗∗p < 0.001), (C) whereas TrkA (Ntrk1) is equally expressed across the two groups of neurons (p > 0.05). Results shown are from samples extracted from a separate cohort of mice: n = 3 DRG and n = 4 TG. Plotted here are individual ddCT values normalized to GAPDH, including means and standard errors of the means; p-values were obtained using a Mann–Whitney test.
In addition to CGRP, our RNA-seq data also show a differential expression of 15 Hox genes, all of which were exclusively expressed in DRG extracted from the lumbar region. To verify these findings, we performed qPCR in DRG and TG ganglia from a separate cohort of animals. Our results revealed Hoxa7 mRNA to be present only in lumbar DRGs but not in TG neurons (Figure 5B, n = 3 DRG; n = 4 TG; p < 0.01). As a positive internal control, we probed for the Ntrk1 gene (TrkA) and, confirming our RNA-seq analysis, found no difference between the groups (Figure 5C).
Comparison between Mouse and Human Datasets
Next, to characterize the similarity between the molecular profiles generated from mouse and human TG, we compared our data to the work of Flegel and collaborators (Flegel et al., 2015). 12,317 genes were found to be expressed in either dataset, 90% of which were identified as equally present or absent in mouse and human (Supplementary Table 2). Rank correlations were moderate (Spearman’s rho rs = 0.53, Figure 2 and Supplementary Table 2). Of the 63 differentially expressed neuronal genes identified in our rodent DRG–TG data, almost 70% followed the same trend toward up- or downregulation in the human data set (Figure 6 and Supplementary Table 3). Of the genes consistently regulated across species, 21 showed at least a twofold higher FPKM in human DRG compared to TG, while five showed a twofold increase in TG. Together, these analyses demonstrate the validity of our newly generated data and confirm the high molecular similarities between mouse and human sensory ganglia.
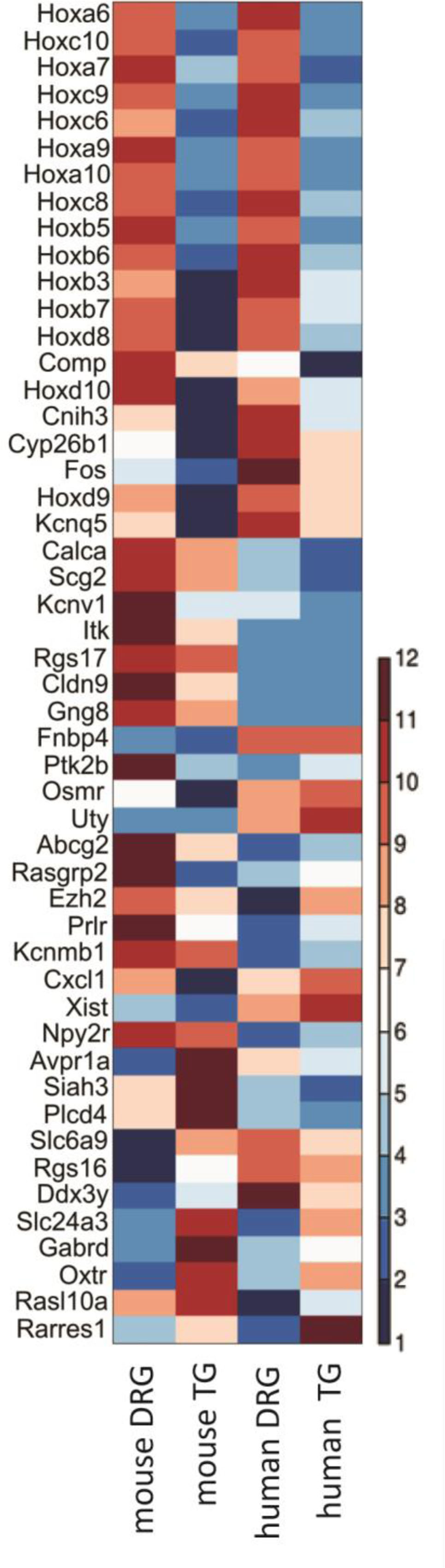
FIGURE 6. Interspecies comparison of differentially expressed neuronal transcripts in mouse and whole ganglion transcripts in human. Of the 63 genes found to be differentially expressed in DRG and TG, 50 could also be easily identified in a dataset examining expression in human (Flegel et al., 2015). Depicted here is a standardized heatmap of the average log2 (FPKM) values from the two different datasets. Blue indicates negative values, i.e., FPKM < 1, with the genes likely lowly expressed or absent. Increasing levels of expression are represented by increasing degrees of red. See Supplementary Table 3 for the underlying raw FPKM values.
Discussion
Our study is the first to provide a molecular profile of enriched TG and DRG neurons. Isolation of cells by FACS, followed by high throughput sequencing demonstrated key differences in expression level between neuron-specific genes in TG and DRG, among them ion channels as well as genes reportedly involved in pain processing. We validated our results in independent cohorts at message and protein level. Finally, we could demonstrate consistency between our newly generated RNA-seq and a previously published dataset examining whole human TG and DRG.
Studies to date have yielded significant insight into the cell biology of both TG and DRG, giving a clear idea of fiber demography, function, ratio, and distribution of myelinated and non-myelinated fibers in each of these ganglia, as well as how they contribute to nociception, pain, and migraine (Snider and McMahon, 1998; Woolf and Ma, 2007; Dubin and Patapoutian, 2010; DaSilva and DosSantos, 2012; Akerman et al., 2017; Goadsby et al., 2017). However, relatively little is known about the molecular characteristics of these distinct neuronal populations, and how they compare at the transcriptional level. Our data revealed that, despite distinct embryogenic origin and processing capabilities, DRG and TG are surprisingly similar, with an almost identical molecular fingerprint. Nevertheless, we could identify 63 genes which were differentially expressed in these two primary neuron populations, including 24 genes that were found exclusively in either of the ganglia (Table 1).
Our results are somewhat unexpected, especially when considering the highly specialized olfactory properties unique to the TG ganglia. Indeed, in a recent study, Manteniotis and collaborators identified almost 200 genes as differentially expressed between whole DRG and TG (Manteniotis et al., 2013). The group described the presence of a number of TG-specific genes and hypothesized their involvement in olfactory processing (Manteniotis et al., 2013). Our results, however, show that none of the genes listed by the authors—including Olfr420, Gm14744, and Lcn3—are expressed by isolated neurons. We believe the contrasting results might be due to tissue composition, given that the majority of cells after DRG dissociation are non-neuronal (Thakur et al., 2014). This can lead to misinterpretation of RNA-seq data: neuron-specific genes could appear falsely downregulated if one type of ganglion were to contain a higher proportion of non-neuronal cells.
Similarly, differences in myelin composition or resident macrophage populations are visible and likely prominent in transcriptional datasets comparing whole DRG and TG. A good example is Kirrel2, which was initially described to be exclusively expressed in TG ganglia (Manteniotis et al., 2013), but is in fact not present in pure TG neurons. Rather, it is highly expressed by astrocytes and oligodendrocytes (Zhang et al., 2014), suggesting differential expression in the satellite glia or myelin of TG and DRG. Similarly, the calcium channels subunits Cacna1g and Cacna1s, which were proposed to be exclusively present in DRG (Manteniotis et al., 2013), are in fact absent from DRG neurons, as shown by our data and previously published work (Thakur et al., 2014; Usoskin et al., 2015). Instead, these genes are found to be expressed in other cells such as microglia and macrophages, both in the CNS and PNS (Gosselin et al., 2014; Lavin et al., 2014; Zhang et al., 2014; Denk et al., 2016), suggesting the non-neuronal origin of these transcripts. Notably, our results and analyses are not disputing the identity of genes previously reported as exclusive to the TG or DRG. We are only providing novel insight into which gene differences are likely to be of neuronal origin. In this context, it is also important to bear in mind that our dissociation method will favor medium and small diameter neurons over large diameter neurons (Supplementary Figure 3). Indeed, genes like Hcn2 and Cacna2d4, which according to single cell data are likely to be exclusively expressed in large diameter DRG neurons (Usoskin et al., 2015; Li et al., 2016), do not pass our FPKM expression cut-off.
The identification of differentially expressed genes allows for the manipulation and targeting of neuronal subpopulations. Here we show a remarkable difference in the expression of the CGRP gene (CALCA) in the two types of ganglia. CGRP is well known for its role as vasodilator and plays a central role in peripheral sensitization, hyperalgesia, and migraine (Benemei et al., 2009; Russell et al., 2014; Iyengar et al., 2017; Schou et al., 2017). Our dataset confirms the presence of CGRP in both TG and DRG neurons, with the latter having over twofold higher expression of this gene—a result which is in line with RNA-seq data derived from human samples (Flegel et al., 2015). These molecular data may seem counterintuitive at first glance. Migraine patients have elevated levels of CGRP (Karsan and Goadsby, 2015; Edvinsson, 2017; Iyengar et al., 2017), the reduction of which has repeatedly been shown to constitute a promising treatment avenue (Olesen et al., 2004; Diener et al., 2015; Karsan and Goadsby, 2015; Mitsikostas and Rapoport, 2015). In contrast, chronic pain conditions involving other areas of the body do not appear to be alleviated by modulation of CGRP (Schou et al., 2017). Yet, DRG appear to express much higher RNA message levels. The discrepancy becomes less jarring when one considers that nerve injury models which cause neuropathic pain conditions are commonly reported to cause downregulation of CGRP (LaCroix-Fralish et al., 2011; Reinhold et al., 2015). This suggests that the gene may be implicated in divergent mechanistic processes in cranial versus peripheral pain conditions.
Another finding from our dataset is the differential regulation of the acid-sensing ion channel subtype-1 (ASIC1), which we identified to have higher expression in TG versus DRG neurons. In addition to having been implicated in neurite outgrowth, neuronal differentiation, synaptic plasticity, learning, memory as well as neuronal injury (Huang et al., 2013; Deval and Lingueglia, 2015; Liu et al., 2017), ASIC1 has a well-documented link to mechanoreception, nociception, and pain (Deval and Lingueglia, 2015; Omerbasic et al., 2015). Previous studies also indicated that ASIC1 could be an analgesic target during pain states (Mazzuca et al., 2007; Diochot et al., 2012). Most notably, ASIC1 has recently been shown to be a new potential drug target for migraine (Holland et al., 2012; Dussor, 2015). Our results are directly relevant to these findings, as we show that expression of the ASIC1 gene is notably higher in TG neurons when compared to DRG. Moreover, we newly identified ASIC1 as differentially expressed compared to previous datasets (Manteniotis et al., 2013; Flegel et al., 2015), likely because its presence in oligodendrocytes (Zhang et al., 2014) would have obscured the neuron-specific difference in mixed tissues. This again highlights the importance of studying cell populations in isolation, as dilution effects can obscure crucial targets that might be used in clinic.
Besides genes linked to pain processing, our study also identified 15 Hox genes which are exclusively expressed in lumbar DRG neurons compared to TG. Hox genes are recognized for their key role as regulators of system development and cell fate specification during embryogenesis (Wellik, 2007; Mallo and Alonso, 2013; Philippidou and Dasen, 2013). Their continued presence in adult DRG is the most striking difference between the two types of ganglia: it can be detected at the level of whole TG and DRG (Manteniotis et al., 2013; Flegel et al., 2015; Kogelman et al., 2017), and similar anterior–posterior patterns are still discernible in adult CNS (Hutlet et al., 2016). In the brain, the expression of Hox genes after development has been linked to the regulation of key proteins that are involved in synapse formation and plasticity (Hutlet et al., 2016). Evidence is still sparse, but if it were to consolidate, Hox genes could represent an interesting exploratory drug target for some forms of chronic pain where changes in synaptic plasticity are well-recognized (Luo et al., 2014; Kuner and Flor, 2016). The absence of Hox genes from the TG is likely due to the distinctive pattern of homeobox gene expression during development (Hodge et al., 2007). We propose that, in the near future, these unique genes could also be used as “guides” in gene therapy, to either knockdown or overexpress genes in specific neurons during chronic pain states, as it has been proposed and already achieved in some specific cell types (Jang et al., 2007; Waehler et al., 2007; Clarke and McMillan, 2014).
Unveiling the specific molecular identities of sensory neurons is crucial for understanding the biology of these highly specialized cells under healthy conditions as well as for developing effective therapies in disease. This is the first report of a molecular comparison between enriched DRG and TG neurons. Our RNA-seq analyses show that despite unexpected similarity, these two populations of neurons have some unique molecular characteristics, as evinced by 63 differentially expressed genes. We are confident that our results will benefit the scientific community by providing easily searchable, neuron-specific information on DRG and TG expression patterns. Furthermore, good agreement between mouse neurons and human tissue suggests that our analyses may aid in the continued quest for better drugs against chronic pain conditions, including migraine.
Materials and Methods
Transgenic Mice
Avil-GFP mice [see gensat.org: STOCK Tg (Avil-EGFP) QD84Gsat/Mmucd for BAC expression levels] were bred in-house for several generations on a CD1 background. In all experiments, adult, age-matched (3–6 months) littermate controls from both genders were used. Mice were kept in a 12-h light–dark cycle, with food and water ad libitum. All experiments were performed in accordance with the UK Animals (Scientific Procedures) Act 1986 and Local Ethical Committee approval.
Fluorescence-Activated Cell Sorting
Avil-GFP mice were deeply anesthetized with pentobarbital and transcardially perfused with 10 ml of PBS. TG (two ganglia/animal) or lumbar DRG (L1–L5, n = 10 lumbar ganglia/animal) were dissected out. Samples were then incubated in 3 mg/ml dispase, 0.1% collagenase and 200 U/ml DNase for 60 min at 37°C in a CO2 incubator, followed by gentle trituration in 1 ml of FACS buffer (5% trehalose, 0.05 mM APV, 15 mM HEPES in DPBS). PI (2.5 μg/ml) was added to the cell suspension so dead cells could be identified during sorting.
Following dissociation, neuronal cell isolation was performed using a BD FACS Aria II Cell Sorter at the NIHR BRC flow core facility at King’s College London (nozzle size 100 μM). Cell populations were gated on GFP+, PI- signal with the help of unstained and single-staining controls. For every experiment, cells from a non-transgenic control animal were used for compensation controls. Positive events (500/animal) were sorted directly into Qiagen RLT buffer and beta-mercaptoethanol and stored at -80°C until the entirety of the biological samples were collected and processed for RNA extraction using a RNeasy Micro kit (Qiagen) with minor modifications of the manufacturer’s protocol. Sample quality was confirmed on an Agilent Bioanalyzer (average RIN value of 8.75, min 7.6, max 9.9).
Sequencing
RNA-seq was performed as previously described (Thakur et al., 2014). In brief, samples were sent for batch-controlled library preparation (SMARTer Ultra Low Input HV kit, 634820, Clontech) and sequencing at the High-Throughput Genomics Group at the Wellcome Trust Centre for Human Genetics at Oxford University. Following amplification, samples were multiplexed in replicate flow cells on an Illumina HiSeq 4000 platform. Reads were uniquely aligned to the mm10 genome using STAR (Dobin et al., 2013), yielding a median mapping percentage of 85.8% (min 80.7%, max 87.6%, standard mapping parameters, bar—outFilterMultimapNmax 1). Normalized gene expression values and differential gene expression data were generated using the cufflinks and DESeq2 algorithms (Trapnell et al., 2010; Love et al., 2014), respectively. We applied an FPKM expression cut-off, based on the underlying distribution of our cufflinks data: FPKM ≥ 1 in at least two of the replicates for each condition. Please note that expression cut-offs will be specific to each individual RNA-seq dataset (as FPKM values are modeled on a curve) and are necessarily somewhat arbitrary. Ours is quite liberal and will likely overestimate the presence of particular gene transcripts. Raw and processed data are available under GEO accession GSE100175.
ELISA
CGRP (Calca) protein levels were measured from freshly extracted DRG and TG samples using a CGRP ELISA kit according to manufacturer’s instructions (Bertin Pharma, 589001). Briefly, the tissue was macerated with a hand-held homogenizer in 200 μl EIA buffer, and the resultant protein was bound by anti-CGRP antibody for 5 min at room temperature. Samples were washed five times and the signal developed with Ellman’s reagent and read on an ELISA plate reader (Molecular Devices, 410 nM). All samples, standards and negative controls were processed in duplicate. The resulting standard curve was linear as required (y = 0.004x + 0.0973, R2 = 0.98), and all samples fell within the limits of the standard samples.
qRT-PCR
Animals were deeply anesthetized with pentobarbital and transcardially perfused with PBS. DRG (lumbar, L3–L6) and TG were collected and RNA was prepared with the RNeasy Micro kit (Qiagen). Total RNA (500 μg) was converted to cDNA using the SuperScript® III Reverse Transcriptase kit (Thermo Fisher Scientific). Quantitative real-time PCR (qPCR) was performed in duplicate with a SYBR green master mix (Roche Diagnostics Limited) with the addition of the selected gene primer sequences (Sigma)—see below. ΔCts were calculated in relation to a house keeping gene (GAPDH). In all experiments, qPCR reactions were run in a Roche Lightcycler 480 PCR machine. Results were analyzed by the standard ΔΔCt method. All primers were checked for their efficiency and specificity beforehand.
Cgrp_F: CTGAGGGCTCTAGCTTGGAC;
Cgrp_R: TGCCAAAATGGGATTGGTGG
Hoxa7_F: CAGTTTCCGCATCTACCCCT;
Hoxa7_R: CGTCTGGTAGCGCGTGT
TrkA_F: GAAGAATGTGACGTGCTGGG;
TrkA_R: GAAGGAGACGCTGACTTGGA
Gapdh_F: GGTCCCAGCTTAGGTTCATCA;
Gapdh_R: CCAATACGGCCAAATCCGTTC
Imaging
Avil-GFP mice were deeply anesthetized with pentobarbital and transcardially perfused with PBS followed by a 4% PFA solution. Tissue was dissected out (DRG and TG), post fixed for 2 h and placed in 30% sucrose solution and left overnight at 4°C. Samples were then embedded in OCT and cut on a cryostat (10 μm sections). Slides were incubated with blocking buffer (10% donkey serum, 0.02% Triton X-100 in PBS), for ∼6 h at room temperature. Sections were then incubated with anti-NeuN antibody (1:1000; Rabbit—Millipore) overnight at room temperature. Primary antibody was removed by washing with PBS, three times. Secondary antibody conjugated with Alexa 594 (Invitrogen) was diluted in the blocking buffer (1:1000), and slides were incubated for at least 2 h at room temperature. Slides were then mounted using Fluoromount-G® medium (Southern Biotechnology) and allowed to dry for 48 h. Endogenous EGFP alongside with Alexa 594 from the sections were imaged using the tile imaging mode (mosaic function) in a Zeiss 710 LSM Axio Imager.Z2 Microscope, at 20×/0.8 DICII magnification. Images were processed (stitched and scale bars placed) using the Zen 2012—Blue Edition software (Zeiss).
Statistics
For all the validation experiments, normality was confirmed by Shapiro–Wilk test. If samples showed a normal distribution, parametric tests were used, otherwise, samples were analyzed by a non-parametric test.
For the CGRP ELISA assay experiments, an independent samples t-test (two-tailed, heteroscedastic) was used. For the qPCR experiments, a Mann–Whitney non-parametric test was performed.
Statistical analyses were performed using Prism 5 software or SPSS.
Author Contributions
DL, FD, and SM contributed to the design of the experiments and interpretation of the data. DL and FD performed the flow cytometry, molecular and cell biology experiments. FD performed the bioinformatics analyses. SM conceived the project. DL wrote the manuscript.
Funding
This research was funded by the Wellcome Trust (102645/Z/13/Z) and by an MRC ERA-NET Neuron grant (MR/M501785/1).
Conflict of Interest Statement
The authors declare that the research was conducted in the absence of any commercial or financial relationships that could be construed as a potential conflict of interest.
Acknowledgments
We would like to thank the High-Throughput Genomics Group at the Wellcome Trust Centre for Human Genetics (funded by Wellcome Trust grant 090532/Z/09/Z) for the generation of the sequencing data. The work was further supported by the National Institute for Health Research (NIHR) Biomedical Research Centre based at Guy’s and St. Thomas’ NHS Foundation Trust and King’s College London. We would like to thank Dr. Philip Holland for useful discussion and comments on the manuscript. The views expressed are those of the author(s) and not necessarily those of the NHS, the NIHR, or the Department of Health.
Supplementary Material
The Supplementary Material for this article can be found online at: http://journal.frontiersin.org/article/10.3389/fnmol.2017.00304/full#supplementary-material
Abbreviations
Avil, advillin; AVRG, average; CGRP, calcitonin gene-related peptide; DRG, dorsal root ganglia; FACS, fluorescence-activated cell sorting; FPKM, Fragments Per Kilobase of transcript per Million mapped reads; GFP or EGFP, (enhanced) green fluorescent protein; Hox, homeobox; mRNA, messenger RNA; PI, propidium iodide; qPCR, quantitative polymerase chain reaction; RIN, RNA integrity number; RNA, ribonucleic acid; RNA-seq, RNA sequencing; TG, trigeminal ganglia.
References
Akerman, S., Romero-Reyes, M., and Holland, P. R. (2017). Current and novel insights into the neurophysiology of migraine and its implications for therapeutics. Pharmacol. Ther. 172, 151–170. doi: 10.1016/j.pharmthera.2016.12.005
Altman, J., and Bayer, S. A. (1982). Development of the cranial nerve ganglia and related nuclei in the rat. Adv. Anat. Embryol. Cell Biol. 74, 1–90. doi: 10.1007/978-3-642-68479-1_1
Benemei, S., Nicoletti, P., Capone, J. G., and Geppetti, P. (2009). CGRP receptors in the control of pain and inflammation. Curr. Opin. Pharmacol. 9, 9–14. doi: 10.1016/j.coph.2008.12.007
Capra, N. F., and Dessem, D. (1992). Central connections of trigeminal primary afferent neurons: topographical and functional considerations. Crit. Rev. Oral Biol. Med. 4, 1–52. doi: 10.1177/10454411920040010101
Chiu, I. M., Barrett, L. B., Williams, E. K., Strochlic, D. E., Lee, S., Weyer, A. D., et al. (2014). Transcriptional profiling at whole population and single cell levels reveals somatosensory neuron molecular diversity. Elife 3:e06720. doi: 10.7554/eLife.04660
Clarke, D. T., and McMillan, N. A. (2014). Gene delivery: cell-specific therapy on target. Nat. Nanotechnol. 9, 568–569. doi: 10.1038/nnano.2014.155
D’Amico-Martel, A., and Noden, D. M. (1983). Contributions of placodal and neural crest cells to avian cranial peripheral ganglia. Am. J. Anat. 166, 445–468. doi: 10.1002/aja.1001660406
DaSilva, A. F., and DosSantos, M. F. (2012). The role of sensory fiber demography in trigeminal and postherpetic neuralgias. J. Dent. Res. 91, 17–24. doi: 10.1177/0022034511411300
Denk, F., Crow, M., Didangelos, A., Lopes, D. M., and Mcmahon, S. B. (2016). Persistent alterations in microglial enhancers in a model of chronic pain. Cell Rep. 15, 1771–1781. doi: 10.1016/j.celrep.2016.04.063
Deval, E., and Lingueglia, E. (2015). Acid-Sensing Ion Channels and nociception in the peripheral and central nervous systems. Neuropharmacology 94, 49–57. doi: 10.1016/j.neuropharm.2015.02.009
Devor, M. (1999). Unexplained peculiarities of the dorsal root ganglion. Pain Suppl. 6, S27–S35. doi: 10.1016/S0304-3959(99)00135-9
Diener, H. C., Charles, A., Goadsby, P. J., and Holle, D. (2015). New therapeutic approaches for the prevention and treatment of migraine. Lancet Neurol. 14, 1010–1022. doi: 10.1016/S1474-4422(15)00198-2
Diochot, S., Baron, A., Salinas, M., Douguet, D., Scarzello, S., Dabert-Gay, A. S., et al. (2012). Black mamba venom peptides target acid-sensing ion channels to abolish pain. Nature 490, 552–555. doi: 10.1038/nature11494
Dobin, A., Davis, C. A., Schlesinger, F., Drenkow, J., Zaleski, C., Jha, S., et al. (2013). STAR: ultrafast universal RNA-seq aligner. Bioinformatics 29, 15–21. doi: 10.1093/bioinformatics/bts635
Donnerer, J., and Stein, C. (1992). Evidence for an increase in the release of CGRP from sensory nerves during inflammation. Ann. N. Y. Acad. Sci. 657, 505–506. doi: 10.1111/j.1749-6632.1992.tb22814.x
Dubin, A. E., and Patapoutian, A. (2010). Nociceptors: the sensors of the pain pathway. J. Clin. Invest. 120, 3760–3772. doi: 10.1172/JCI42843
Durham, P. L., and Garrett, F. G. (2010). Development of functional units within trigeminal ganglia correlates with increased expression of proteins involved in neuron-glia interactions. Neuron Glia Biol. 6, 171–181. doi: 10.1017/S1740925X10000232
Dussor, G. (2015). ASICs as therapeutic targets for migraine. Neuropharmacology 94, 64–71. doi: 10.1016/j.neuropharm.2014.12.015
Edvinsson, L. (2017). The trigeminovascular pathway: role of CGRP and CGRP receptors in migraine. Headache 57(Suppl. 2), 47–55. doi: 10.1111/head.13081
Erzurumlu, R. S., Murakami, Y., and Rijli, F. M. (2010). Mapping the face in the somatosensory brainstem. Nat. Rev. Neurosci. 11, 252–263. doi: 10.1038/nrn2804
Flegel, C., Schobel, N., Altmuller, J., Becker, C., Tannapfel, A., Hatt, H., et al. (2015). RNA-seq analysis of human trigeminal and dorsal root ganglia with a focus on chemoreceptors. PLOS ONE 10:e0128951. doi: 10.1371/journal.pone.0128951
Gerhold, K. A., and Bautista, D. M. (2009). Molecular and cellular mechanisms of trigeminal chemosensation. Ann. N. Y. Acad. Sci. 1170, 184–189. doi: 10.1111/j.1749-6632.2009.03895.x
Goadsby, P. J., Holland, P. R., Martins-Oliveira, M., Hoffmann, J., Schankin, C., and Akerman, S. (2017). Pathophysiology of migraine: a disorder of sensory processing. Physiol. Rev. 97, 553–622. doi: 10.1152/physrev.00034.2015
Gong, S., Zheng, C., Doughty, M. L., Losos, K., Didkovsky, N., Schambra, U. B., et al. (2003). A gene expression atlas of the central nervous system based on bacterial artificial chromosomes. Nature 425, 917–925. doi: 10.1038/nature02033
Gosselin, D., Link, V. M., Romanoski, C. E., Fonseca, G. J., Eichenfield, D. Z., Spann, N. J., et al. (2014). Environment drives selection and function of enhancers controlling tissue-specific macrophage identities. Cell 159,1327–1340. doi: 10.1016/j.cell.2014.11.023
Hanani, M. (2005). Satellite glial cells in sensory ganglia: from form to function. Brain Res. Brain Res. Rev. 48, 457–476. doi: 10.1016/j.brainresrev.2004.09.001
Hart, S. N., Therneau, T. M., Zhang, Y., Poland, G. A., and Kocher, J. P. (2013). Calculating sample size estimates for RNA sequencing data. J. Comput. Biol. 20, 970–978. doi: 10.1089/cmb.2012.0283
Hodge, L. K., Klassen, M. P., Han, B. X., Yiu, G., Hurrell, J., Howell, A., et al. (2007). Retrograde BMP signaling regulates trigeminal sensory neuron identities and the formation of precise face maps. Neuron 55, 572–586. doi: 10.1016/j.neuron.2007.07.010
Holland, P. R., Akerman, S., Andreou, A. P., Karsan, N., Wemmie, J. A., and Goadsby, P. J. (2012). Acid-sensing ion channel 1: a novel therapeutic target for migraine with aura. Ann. Neurol. 72, 559–563. doi: 10.1002/ana.23653
Hu, G., Huang, K., Hu, Y., Du, G., Xue, Z., Zhu, X., et al. (2016). Single-cell RNA-seq reveals distinct injury responses in different types of DRG sensory neurons. Sci. Rep. 6:31851. doi: 10.1038/srep31851
Huang, L. Y., Gu, Y., and Chen, Y. (2013). Communication between neuronal somata and satellite glial cells in sensory ganglia. Glia 61, 1571–1581. doi: 10.1002/glia.22541
Hutlet, B., Theys, N., Coste, C., Ahn, M. T., Doshishti-Agolli, K., Lizen, B., et al. (2016). Systematic expression analysis of Hox genes at adulthood reveals novel patterns in the central nervous system. Brain Struct. Funct. 221, 1223–1243. doi: 10.1007/s00429-014-0965-8
Iyengar, S., Ossipov, M. H., and Johnson, K. W. (2017). The role of calcitonin gene-related peptide in peripheral and central pain mechanisms including migraine. Pain 158, 543–559. doi: 10.1097/j.pain.0000000000000831
Jang, J. H., Lim, K. I., and Schaffer, D. V. (2007). Library selection and directed evolution approaches to engineering targeted viral vectors. Biotechnol. Bioeng. 98, 515–524. doi: 10.1002/bit.21541
Karsan, N., and Goadsby, P. J. (2015). Calcitonin gene-related peptide and migraine. Curr. Opin. Neurol. 28, 250–254. doi: 10.1097/WCO.0000000000000191
Kogelman, L. J. A., Christensen, R. E., Pedersen, S. H., Bertalan, M., Hansen, T. F., Jansen-Olesen, I., et al. (2017). Whole transcriptome expression of trigeminal ganglia compared to dorsal root ganglia in Rattus Norvegicus. Neuroscience 350, 169–179. doi: 10.1016/j.neuroscience.2017.03.027
Krispin, S., Nitzan, E., and Kalcheim, C. (2010). The dorsal neural tube: a dynamic setting for cell fate decisions. Dev. Neurobiol. 70, 796–812. doi: 10.1002/dneu.20826
Kuner, R., and Flor, H. (2016). Structural plasticity and reorganisation in chronic pain. Nat. Rev. Neurosci. 18, 20–30. doi: 10.1038/nrn.2016.162
LaCroix-Fralish, M. L., Austin, J. S., Zheng, F. Y., Levitin, D. J., and Mogil, J. S. (2011). Patterns of pain: meta-analysis of microarray studies of pain. Pain 152, 1888–1898. doi: 10.1016/j.pain.2011.04.014
Lavin, Y., Winter, D., Blecher-Gonen, R., David, E., Keren-Shaul, H., Merad, M., et al. (2014). Tissue-resident macrophage enhancer landscapes are shaped by the local microenvironment. Cell 159, 1312–1326. doi: 10.1016/j.cell.2014.11.018
Li, C. L., Li, K. C., Wu, D., Chen, Y., Luo, H., Zhao, J. R., et al. (2016). Somatosensory neuron types identified by high-coverage single-cell RNA-sequencing and functional heterogeneity. Cell Res. 26, 83–102. doi: 10.1038/cr.2015.149
Liu, M., Inoue, K., Leng, T., Zhou, A., Guo, S., and Xiong, Z. G. (2017). ASIC1 promotes differentiation of neuroblastoma by negatively regulating Notch signaling pathway. Oncotarget 8, 8283–8293. doi: 10.18632/oncotarget.14164
Love, M. I., Huber, W., and Anders, S. (2014). Moderated estimation of fold change and dispersion for RNA-seq data with DESeq2. Genome Biol. 15, 550. doi: 10.1186/s13059-014-0550-8
Luo, C., Kuner, T., and Kuner, R. (2014). Synaptic plasticity in pathological pain. Trends Neurosci. 37, 343–355. doi: 10.1016/j.tins.2014.04.002
Luo, P. F., Wang, B. R., Peng, Z. Z., and Li, J. S. (1991). Morphological characteristics and terminating patterns of masseteric neurons of the mesencephalic trigeminal nucleus in the rat: an intracellular horseradish peroxidase labeling study. J. Comp. Neurol. 303, 286–299. doi: 10.1002/cne.903030210
Mallo, M., and Alonso, C. R. (2013). The regulation of Hox gene expression during animal development. Development 140, 3951–3963. doi: 10.1242/dev.068346
Manteniotis, S., Lehmann, R., Flegel, C., Vogel, F., Hofreuter, A., Schreiner, B. S., et al. (2013). Comprehensive RNA-Seq expression analysis of sensory ganglia with a focus on ion channels and GPCRs in Trigeminal ganglia. PLOS ONE 8:e79523. doi: 10.1371/journal.pone.0079523
Marmigere, F., and Ernfors, P. (2007). Specification and connectivity of neuronal subtypes in the sensory lineage. Nat. Rev. Neurosci. 8, 114–127. doi: 10.1038/nrn2057
Mazzuca, M., Heurteaux, C., Alloui, A., Diochot, S., Baron, A., Voilley, N., et al. (2007). A tarantula peptide against pain via ASIC1a channels and opioid mechanisms. Nat. Neurosci. 10, 943–945. doi: 10.1038/nn1940
Mitsikostas, D. D., and Rapoport, A. M. (2015). New players in the preventive treatment of migraine. BMC Med. 13:279. doi: 10.1186/s12916-015-0522-1
Olesen, J., Diener, H. C., Husstedt, I. W., Goadsby, P. J., Hall, D., Meier, U., et al. (2004). Calcitonin gene-related peptide receptor antagonist BIBN 4096 BS for the acute treatment of migraine. N. Engl. J. Med. 350, 1104–1110. doi: 10.1056/NEJMoa030505
Omerbasic, D., Schuhmacher, L. N., Bernal Sierra, Y. A., Smith, E. S., and Lewin, G. R. (2015). ASICs and mammalian mechanoreceptor function. Neuropharmacology 94, 80–86. doi: 10.1016/j.neuropharm.2014.12.007
Perkins, J. R., Antunes-Martins, A., Calvo, M., Grist, J., Rust, W., Schmid, R., et al. (2014). A comparison of RNA-seq and exon arrays for whole genome transcription profiling of the L5 spinal nerve transection model of neuropathic pain in the rat. Mol Pain 10:7. doi: 10.1186/1744-8069-10-7
Philippidou, P., and Dasen, J. S. (2013). Hox genes: choreographers in neural development, architects of circuit organization. Neuron 80, 12–34. doi: 10.1016/j.neuron.2013.09.020
Porter, J. D., and Spencer, R. F. (1982). Localization of morphology of cat extraocular muscle afferent neurones identified by retrograde transport of horseradish peroxidase. J. Comp. Neurol. 204, 56–64. doi: 10.1002/cne.902040107
Reichling, D. B., Green, P. G., and Levine, J. D. (2013). The fundamental unit of pain is the cell. Pain 154(Suppl. 1), S2–S9. doi: 10.1016/j.pain.2013.05.037
Reinhold, A. K., Batti, L., Bilbao, D., Buness, A., Rittner, H. L., and Heppenstall, P. A. (2015). Differential transcriptional profiling of damaged and intact adjacent dorsal root ganglia neurons in neuropathic pain. PLOS ONE 10:e0123342. doi: 10.1371/journal.pone.0123342
Reynders, A., Mantilleri, A., Malapert, P., Rialle, S., Nidelet, S., Laffray, S., et al. (2015). Transcriptional profiling of cutaneous MRGPRD free nerve endings and C-LTMRs. Cell Rep. doi: 10.1016/j.celrep.2015.01.022 [Epub ahead of print].
Russell, F. A., King, R., Smillie, S. J., Kodji, X., and Brain, S. D. (2014). Calcitonin gene-related peptide: physiology and pathophysiology. Physiol. Rev. 94, 1099–1142. doi: 10.1152/physrev.00034.2013
Sapio, M. R., Goswami, S. C., Gross, J. R., Mannes, A. J., and Iadarola, M. J. (2016). Transcriptomic analyses of genes and tissues in inherited sensory neuropathies. Exp. Neurol. 283, 375–395. doi: 10.1016/j.expneurol.2016.06.023
Schou, W. S., Ashina, S., Amin, F. M., Goadsby, P. J., and Ashina, M. (2017). Calcitonin gene-related peptide and pain: a systematic review. J. Headache Pain 18, 34. doi: 10.1186/s10194-017-0741-2
Snider, W. D., and McMahon, S. B. (1998). Tackling pain at the source: new ideas about nociceptors. Neuron 20, 629–632. doi: 10.1016/S0896-6273(00)81003-X
Thakur, M., Crow, M., Richards, N., Davey, G. I., Levine, E., Kelleher, J. H., et al. (2014). Defining the nociceptor transcriptome. Front. Mol. Neurosci. 7:87. doi: 10.3389/fnmol.2014.00087
Trapnell, C., Williams, B. A., Pertea, G., Mortazavi, A., Kwan, G., Van Baren, M. J., et al. (2010). Transcript assembly and quantification by RNA-Seq reveals unannotated transcripts and isoform switching during cell differentiation. Nat. Biotechnol. 28, 511–515. doi: 10.1038/nbt.1621
Usoskin, D., Furlan, A., Islam, S., Abdo, H., Lonnerberg, P., Lou, D., et al. (2015). Unbiased classification of sensory neuron types by large-scale single-cell RNA sequencing. Nat. Neurosci. 18, 145–153. doi: 10.1038/nn.3881
Viana, F. (2011). Chemosensory properties of the trigeminal system. ACS Chem. Neurosci. 2, 38–50. doi: 10.1021/cn100102c
Waehler, R., Russell, S. J., and Curiel, D. T. (2007). Engineering targeted viral vectors for gene therapy. Nat. Rev. Genet. 8, 573–587. doi: 10.1038/nrg2141
Wellik, D. M. (2007). Hox patterning of the vertebrate axial skeleton. Dev. Dyn. 236, 2454–2463. doi: 10.1002/dvdy.21286
Woolf, C. J., and Ma, Q. (2007). Nociceptors–noxious stimulus detectors. Neuron 55, 353–364. doi: 10.1016/j.neuron.2007.07.016
Wu, S., Marie Lutz, B., Miao, X., Liang, L., Mo, K., Chang, Y. J., et al. (2016). Dorsal root ganglion transcriptome analysis following peripheral nerve injury in mice. Mol. Pain 12:1744806916629048. doi: 10.1177/1744806916629048
Yu, L. C., Hou, J. F., Fu, F. H., and Zhang, Y. X. (2009). Roles of calcitonin gene-related peptide and its receptors in pain-related behavioral responses in the central nervous system. Neurosci. Biobehav. Rev. 33, 1185–1191. doi: 10.1016/j.neubiorev.2009.03.009
Keywords: DRG, trigeminal ganglia, RNA sequencing, transcriptome, pain, migraine
Citation: Lopes DM, Denk F and McMahon SB (2017) The Molecular Fingerprint of Dorsal Root and Trigeminal Ganglion Neurons. Front. Mol. Neurosci. 10:304. doi: 10.3389/fnmol.2017.00304
Received: 10 July 2017; Accepted: 11 September 2017;
Published: 26 September 2017.
Edited by:
Guilherme Lucas, University of São Paulo, BrazilReviewed by:
Patrick M. Dougherty, University of Texas MD Anderson Cancer Center, United StatesFelix Viana, Spanish Council of Scientific Research, Spain
Peter John Shortland, Western Sydney University, Australia
Copyright © 2017 Lopes, Denk and McMahon. This is an open-access article distributed under the terms of the Creative Commons Attribution License (CC BY). The use, distribution or reproduction in other forums is permitted, provided the original author(s) or licensor are credited and that the original publication in this journal is cited, in accordance with accepted academic practice. No use, distribution or reproduction is permitted which does not comply with these terms.
*Correspondence: Douglas M. Lopes, ZG91Z2xhcy5sb3Blc0BrY2wuYWMudWs=
†These authors are joint first authors.