- 1International Iberian Nanotechnology Laboratory, Braga, Portugal
- 2Medical School, Swansea University, Swansea, United Kingdom
- 3Neurology Department, Centro Hospitalar de São João, Porto, Portugal
- 4Department of Clinical Neurosciences and Mental Health, Faculdade de Medicina, Universidade do Porto, Porto, Portugal
- 5Department of Biomedicine, Faculdade de Medicina, Universidade do Porto, Porto, Portugal
- 6Graduate Programme in Areas of Basic and Applied Biology, Instituto de Ciências Biomédicas Abel Salazar, Universidade do Porto, Porto, Portugal
- 7Instituto de Investigação e Inovação em Saúde, Universidade do Porto, Porto, Portugal
- 8Center for Drug Discovery and Innovative Medicines (MedInUP), Universidade do Porto, Porto, Portugal
- 9Energy, Environment and Health Research Unit (FP-ENAS), University Fernando Pessoa, Porto, Portugal
- 10Faculty of Health Sciences, University Fernando Pessoa, Porto, Portugal
Neuroinflammation plays a critical role in the onset and progression of many neurological disorders, including Multiple Sclerosis, Alzheimer's and Parkinson's diseases. In these clinical conditions the underlying neuroinflammatory processes are significantly heterogeneous. Nevertheless, a common link is the chronic activation of innate immune responses and imbalanced secretion of pro and anti-inflammatory mediators. In light of this, the discovery of robust biomarkers is crucial for screening, early diagnosis, and monitoring of neurological diseases. However, the difficulty to investigate biochemical processes directly in the central nervous system (CNS) is challenging. In recent years, biomarkers of CNS inflammatory responses have been identified in different body fluids, such as blood, cerebrospinal fluid, and tears. In addition, progress in micro and nanotechnology has enabled the development of biosensing platforms capable of detecting in real-time, multiple biomarkers in clinically relevant samples. Biosensing technologies are approaching maturity where they will become deployed in community settings, at which point screening programs and personalized medicine will become a reality. In this multidisciplinary review, our goal is to highlight both clinical and recent technological advances toward the development of multiplex-based solutions for effective neuroinflammatory and neurodegenerative disease diagnostics and monitoring.
Neurodegeneration and Inflammation: A Clinical and Molecular Perspective
Neurological disorders account for an increasing number of disability-adjusted life-years worldwide, especially in high-income countries. Alzheimer's disease, Parkinson's disease and Multiple Sclerosis are the most prevalent causes of neurological disability (Hay et al., 2017). The three different conditions share features of neurodegeneration and neuroinflammation and their diagnosis rely mainly on clinical examination, complemented by imaging and biomarker analysis (Table 1) (Poewe et al., 2017; Lane et al., 2018; Reich et al., 2018).
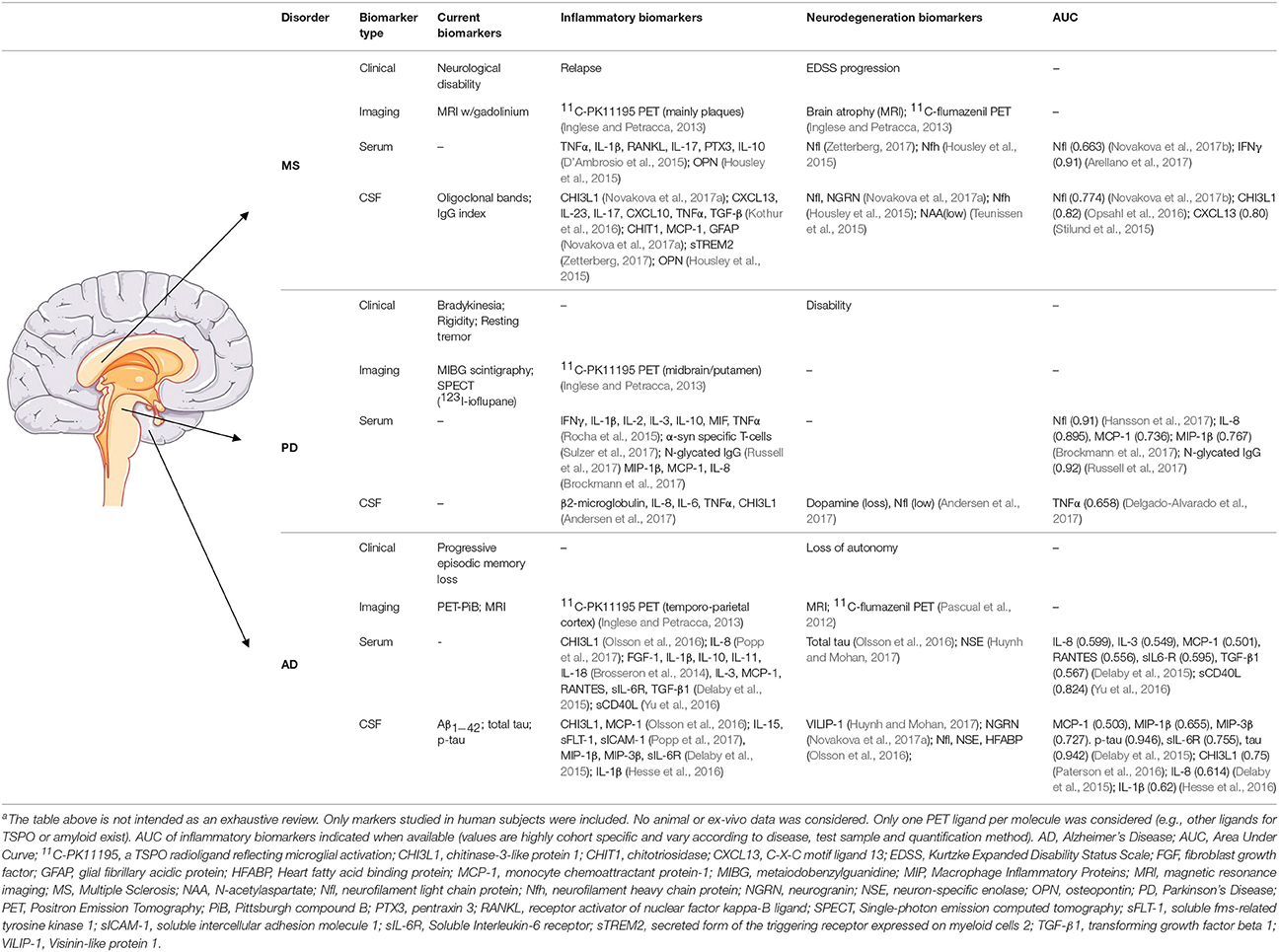
Table 1. Biomarkers of neurodegeneration and neuroinflammation in Multiple Sclerosis, Parkinson, and Alzheimer's diseasea.
Alzheimer's disease (AD) is a neurodegenerative disorder primarily affecting neocortical regions and characterized by progressive episodic memory loss leading to significant behavioral changes (McDonald et al., 2009; Lane et al., 2018). Definite AD diagnosis is histopathological, while diagnosis of probable/possible AD dementia is only made by clinical assessment. Diagnostic accuracy can be enhanced by further findings of low amyloid-beta (Aβ) levels and an increase in the total or phosphorylated tau protein in cerebrospinal fluid (CSF) (McKhann et al., 2011). Furthermore, positron emitting tomography (PET) showing increased amyloid deposition or decreased fluorodeoxyglucose uptake in the temporo-parietal cortex also represent acceptable evidence of the AD pathophysiological process (McKhann et al., 2011). Overall, AD pathology has been classically associated to the presence of amyloid plaques (neuritic plaques) and hyperphosphorylated tau aggregates (neurofibrillary tangles, NFTs) in the brain, which titrates the corresponding levels in the CSF. Amyloid plaques are believed to arise from an imbalance between Aβ1−42 production [via γ and β-secretase 1 (BACE1) cleavage of amyloid precursor protein] and its clearance, leading to the formation of toxic oligomers (AβO), subsequent synaptic dysfunction and neuronal cell death (Lane et al., 2018). In dominant inherited forms of AD (including mutations in γ-secretase subunits, PSEN1 and PSEN2) the formation of amyloid plaques is promoted by an increased production of Aβ1−42, while in sporadic AD it is mainly due to impaired Aβ clearance (Mawuenyega et al., 2010; Lane et al., 2018). Mutations in genes coding for proteins involved in Aβ clearance pathways represent risk factors for AD, among these are apolipoprotein E (APOE) and the immune receptors: triggering receptor expressed on myeloid cells 2 (TREM2), cluster of differentiation 33 (CD33), and complement region 1 (CR1). TREM2, CD33, and CR1 are expressed in microglia, the innate immune cells of the central nervous system (CNS) and have been found to be associated with a higher risk of AD (Polvikoski et al., 1995; Bradshaw et al., 2013; Crehan et al., 2013; Griciuc et al., 2013; Guerreiro et al., 2013; Farfel et al., 2016). Microglia activation can have a neurotoxic role in AD through activation of the complement system (e.g., C1q, C3) and the inflammasome, release of pro-inflammatory mediators [e.g., interleukin-1 (IL-1), IL-6 and tumor necrosis factor α (TNFα)] and leading to synaptic loss, mitogen-activated protein kinase (MAPK) activation and subsequent NFTs formation (Griffin et al., 2006; Heneka et al., 2013; Dursun et al., 2015; Wang et al., 2015; Hong et al., 2016; Fonseca et al., 2017; Liddelow et al., 2017). Despite the supporting evidence of the innate immunity pathways in AD pathogenesis, attempts to modulate the inflammatory response in patients with AD have mostly failed at improving cognition and halting disease progression (Bronzuoli et al., 2016; Dansokho and Heneka, 2017; Honig et al., 2018).
Parkinson's disease (PD), the second most common neurodegenerative disorder, is characterized by the early and progressive loss of dopaminergic neurons in the substantia nigra pars compacta associated with abnormal α-synuclein (α-syn) deposition (Kalia and Lang, 2015). The resulting striatal dopamine deficiency leads to a movement disorder with a clinically recognizable triad of motor symptoms: bradykinesia (“slow movement”) together with resting tremor and/or rigidity initially restricted to one limb or hemibody, slowly progressing to affect the rest of the body. However, PD is also associated with pathological changes in other brain regions causing non-motor symptoms (e.g., hyposmia, dysautonomia, sleep, and psychiatric/cognitive disorders) that add to overall disability and can precede motor dysfunction (Kalia and Lang, 2015). These likely reflects the distribution of α-syn aggregates to other regions of the nervous system (Postuma et al., 2015; Poewe et al., 2017). PD diagnosis is exclusively clinical. However, ancillary tests include metaiodobenzylguanidine (MIBG) scintigraphy demonstrating cardiac sympathetic denervation, olfactory function testing and pre-synaptic dopamine (DA) receptor 123I-ioflupane single-photon emission computed tomography (SPECT) imaging. Biomarker analysis, including α-syn, in serum or CSF, is not performed in standard clinical practice (Postuma et al., 2015). Nevertheless, α-syn aggregates in specific brain regions are recognized neuropathological hallmarks of PD. In fact, α-syn mutation is responsible for heritable forms of PD (Poewe et al., 2017). Other genes identified in inherited PD and corresponding proteins, include PARK7 (deglycase DJ-1), GBA (glucocerebrosidase), PRKN (parkin), and LRRK2 (leucine-rich repeat kinase 2) which are expressed in microglia (Lee et al., 2017). At large, the physiological functions of PD-associated genes in immune cells remain elusive. Nevertheless, it is possible that mutations in those genes can alter their normal microglia functions worsening the progression of inflammation-mediated PD neurodegeneration (Lee et al., 2017). Studies found signs of microglia activation and chronic inflammation in the brains of PD patients (McGeer et al., 1988; Gerhard et al., 2006) and α-syn aggregates are capable of activating microglia in vitro and in mouse models (Brochard et al., 2009). Pro-inflammatory cytokines, such as TNFα, IL-1β, IL-6, IL-2, and IL-10 are increased in postmortem brain (Mogi et al., 1994b), CSF (Mogi et al., 1994a), and serum (Dufek et al., 2009; Williams-Gray et al., 2016) of PD patients and may be predictive of disease progression.
Multiple Sclerosis (MS) is a chronic inflammatory demyelinating disorder of the CNS of unknown etiology but with a genetic predisposition and environmental influence (Dendrou et al., 2015; Reich et al., 2018). Initial symptoms are variable and related with the affected area of the CNS (Mowry et al., 2009; Pires et al., 2016). Diagnosis of MS requires clinical or radiological evidence of lesion dissemination in time and/or space. Magnetic resonance imaging (MRI) is the conventional diagnostic tool, while serum and CSF testing are useful in excluding other pathologies. The presence of CSF-restricted oligoclonal bands (OCBs) supports MS diagnosis, however it is not MS-specific (Thompson et al., 2017). CSF-restricted OCBs can be found in other diseases whose clinical and imaging characteristics differ from MS, such as systemic inflammatory disorders with CNS expression (e.g., Systemic Lupus Erythematous, Sarcoidosis, and Behçet's disease), CNS infections (e.g., Neurosyphilis, HIV, Neuroborreliosis, Subacute Sclerosing Panencephalitis) and in some hereditary disorders (e.g., Ataxia-telangiectasia and Adrenoleukodystrophy) (Giovannoni, 2014).
In MS lesions, histopathology reveals profound myelin loss, increased inflammatory response, and secondary axonal degeneration. Microglia activation perpetuates the underlying inflammatory response at the demyelinated plaque and at sites remote from the lesion (Dendrou et al., 2015). Microglia-driven production of reactive oxygen and nitrogen species, which stress the neuronal and mitochondrial metabolism, promotes neuronal death (Schuh et al., 2014; Choi et al., 2017; Luo et al., 2017) which leads to the release of cytoskeletal elements into the CSF, such as neurofilaments (NFs). NFs are promising biomarkers for predicting lesion burden, therapeutic response, and disease progression (Zetterberg, 2017).
CNS tissue damage in MS results from an intricate interplay between the immune system, glial cells, and neurons. Although there is ongoing debate regarding MS origin, i.e., the “outside-in” (peripheral immune cell invasion of the CNS) or “inside-out” (CNS-intrinsic initiation of the inflammatory cascade) models (Reich et al., 2018), studies in animal models, and in patient CSF and blood samples have disclosed a critical role for adaptive immunity (auto-reactive T and B cells and autoantibodies) (Reich et al., 2018). Despite the knowledge gap regarding MS initial immunopathogenesis, therapies directed both at T cells and B cells have been effective in reducing relapse rate and disease progression (Pires et al., 2016; Reich et al., 2018).
Although the trigger for inflammation might be specific for each of the diseases mentioned above, evidence suggests that AD, PD, and MS share common cellular and molecular mechanisms for sensing, transducing and amplifying inflammation that results in the production of mediators of inflammation, neurotoxicity and, ultimately, neuronal cell death (Yadav et al., 2015; Guillot-Sestier and Town, 2017). Activation of microglial cells is a key event in such neuroinflammatory processes (Ginhoux and Guilliams, 2016). Under physiological conditions microglia assume immune surveillance functions but upon tissue damage or infection they change their morphology and transcriptomic profile enabling them to restore tissue homeostasis (Crotti and Ransohoff, 2016). Through pattern recognition receptors, including TREM2, microglial cells recognize environmental cues that instruct them to initiate inflammatory responses by triggering downstream signaling pathways regulating the activity of the transcription factors AP-1 and NF-κB, which in turn control the production and release of inflammatory mediators, such as the cytokines TNFα, IL-1β, IL-6, and IL-8, reactive oxygen and nitrogen species (Ortiz et al., 2013; Leszek et al., 2016; Labzin et al., 2018). The analysis of inflammatory profile, in association with classical disease-specific biomarkers could potentially increase diagnostic and prognostic accuracy (Table 1).
Sensing Circulating Biomarkers of Neurodegeneration and Neuroinflammation
Over the past years, great efforts have been made to identify biomarkers associated with CNS diseases in clinically relevant samples. A biomarker is defined as a measurable biologically plausible parameter, usually being an indicator of an underlying disease mechanism (Atkinson et al., 2001). In addition, an ideal biomarker should also be readily accessible, highly sensitive, and specific and its levels should correlate with disease progression and/or treatment response, allowing disease risk stratification (Bennett and Devarajan, 2016). Biomarker cut-off values determine the clinical sensitivity (ratio of true positives over all individuals with disease) and specificity (ratio of true negatives over all individuals without disease). The Receiver Operating Characteristic (ROC) curve is a graphic display of sensitivity versus (1-specificity), and its Area Under the Curve (AUC) provides a useful measure for optimal cut-off value selection (Parikh and Thiessen-Philbrook, 2014). AUC values for single biomarkers are shown in Table 1. Recent studies suggest that the combination of multiple biomarkers increases the AUC value, therefore increasing the accuracy of the disease diagnostic tests (Spellman et al., 2015; Lue et al., 2016).
Although many neurological studies have relied on the biochemical analysis of CSF, the physiological sample of reference for CNS disorders, these biomarkers are also present in more accessible biological fluids, making sample acquisition less invasive, as exemplified for TNFα and OCBs that are present in higher amounts in tears of PD and MS patients, respectively (Devos et al., 2001; Çomoglu et al., 2013). Nevertheless, this biochemical profiling has mostly relied on microarray technologies (Choi et al., 2008; Craig-Schapiro et al., 2011; Martins et al., 2011; Koziorowski et al., 2012; Edwards et al., 2013; Laske et al., 2013; Burman et al., 2014; Delaby et al., 2015; Cala et al., 2016; Hegen et al., 2016; Lue et al., 2016) and liquid chromatography-mass spectroscopy (Musunuri et al., 2014; Hölttä et al., 2015; Spellman et al., 2015; Paterson et al., 2016) which, although effective for large biomarker panel assessment, are not suitable for point-of-care testing. On the other hand, identification and validation of potential biomarkers is often hindered by their low concentrations in the test fluid and inherent variability across control and patient samples. As such, there is a need for new technologies with lower limit of detection (LOD) and higher sensitivity.
Biosensors are analytical devices capable of converting specific biorecognition events into a measurable signal. Conventional biosensors are composed of a receptor (e.g., antibody, enzyme, and DNA) which specifically recognizes the biomarker (e.g., antigen, enzyme substrate, and DNA) of interest and a transducer which converts biochemical interactions into a quantifiable electrical signal proportional to biomarker concentrations. Biosensors are commonly classified in electrochemical, optical, piezoelectric, or magnetic, based on the signal transduction mechanism. These technologies have broad applications in health (Zhang et al., 2017; El Harrad et al., 2018), food (Law et al., 2014; Vasilescu and Marty, 2016), and environmental sciences (Rapini and Marrazza, 2017; Kumar et al., 2018). Over the past years, the critical role of inflammation in disease has led researchers to develop biosensors for the specific detection of inflammatory mediators in clinically relevant body fluids. Although most inflammation-targeted biosensors have not been tested in the context of neuroinflammatory diseases, the clinical potential of these technologies is undeniable (Table 2). Recently, Baraket et al. developed an electrochemical biosensor to monitor IL-1β and IL-10 cytokine levels after the implantation of left ventricular assist devices (LVADs) in patients with heart failure while waiting for compatible donors (Baraket et al., 2017). Given the non-biocompatible nature of the LVAD, many patients suffer from acute inflammation in which several pro and anti-inflammatory cytokines are secreted, such as IL-1β and IL-10, respectively. The proposed biosensor was capable of detecting both cytokines within the range of 1–15 pg/mL, relevant to predict the first signs of inflammation (Stumpf et al., 2003).
Increased levels of pro-inflammatory cytokines in the CSF and serum of MS patients can alter the permeability of the blood-brain-barrier and promote T-lymphocyte migration into the brain and disease progression (Khaibullin et al., 2017). Therefore, cytokine detection in minimally invasive body fluids represents an attractive alternative for timely diagnosis of MS patients. Moreover, it allows early identification of relapsing patients and prediction of anti-inflammatory therapy failure, of outmost interest for effective clinical intervention. Elevated serum levels of matrix metalloproteinase-9 (MMP-9) have been associated with ongoing neuroinflammation processes and are indicative of MS relapse (Fainardi et al., 2006). Biela et al. developed an electrochemical biosensor for the sensitive and rapid detection of MMP-9 in clinically relevant ranges (50–400 ng/mL) (Biela et al., 2015). The biosensor was coated with a hydrogel and cross-linked peptides with specific MMP-9 cleavage sites. Exposure to MMP-9 resulted in the degradation of the hydrogel-peptide film and, consequently, produced an electrochemical signal. Importantly, the authors confirmed the specificity of the biosensor for MMP-9 detection against MMP-2, also present in the blood. Additionally, an electrochemical biosensor for IL-12 detection was developed by Bhavsar et al. for automated real-time biomarker analysis (Bhavsar et al., 2009). Although the biosensor was not validated with patient samples, the authors confirmed IL-12 detection in spiked samples of fetal bovine serum, showing a LOD of 3.5 pg/mL, lower than reported values for IL-12 expression in MS patients (Drulović et al., 1998).
In 2015, Chen and co-workers introduced for the first time a biosensor for simultaneous detection of multiple cytokines (Chen et al., 2015) and real-time monitoring of the inflammatory response of two neonates after a cardiopulmonary bypass surgery. This technology is based on a microfluidic surface plasmon resonance (LSPR) sensor capable of detecting multiple analytes through refractometric measurements. The authors demonstrated parallel multiplex analysis of six cytokines (IL-2, IL-4, IL-6, IL-10, TNFα, interferon γ (IFNγ) with a linear range of detection between 5 and 20 pg/mL, only requiring 1 μL of serum sample. Conventionally, nanoplasmonic biosensors are not suitable for point-of-care medical applications due to their limited sensitivity and optical microscope requirements. Nevertheless, the authors employed dark-field imaging with nanorods conjugated with antibodies to improve the sensitivity 10 times more than conventional LSPR chips.
The quantification of inflammatory mediators in minimally invasive samples of patients with neurodegenerative diseases provides valuable clinical information regarding their immune status. Nevertheless, it is insufficient to provide an accurate diagnosis. A comprehensive analysis and quantification of disease-specific biomarkers allied with immune system surveillance may improve patient prognosis by allowing timely and accurate diagnosis while enabling patient stratification for personalized treatment (Table 2).
AD has been by far the most intensely studied neurodegenerative pathology toward the development of effective and sensitive diagnostic platforms with sensors targeting Aβ peptides and oligomers in blood and CSF (Oh et al., 2013; Kim et al., 2016a; Li et al., 2016; Carneiro et al., 2017). Of these, Carneiro et al. recently reported an electrochemical biosensor for the detection of Aβ1−42 with a LOD of 5.2 pg/mL and wide dynamic range (10–1,000 pg/mL) provided by the use of gold nanoparticles (NPs) (Carneiro et al., 2017). This is particularly significant for the assessment of Aβ1−42 levels which are below 500 pg/mL in CSF of AD patients (Gagni et al., 2013). Also, Rushworth et al. developed a novel, label-free impedimetric biosensor for the specific detection of AβO. A fragment of the cellular prion protein (PrPC residues 95–110), which mediates the neuronal binding and toxicity of AβO, was used as a recognition element for the specific detection of the oligomers. The biosensor presented a LOD of 0.5 pM and successfully detected cell-derived AβO from conditioned media of 7PA2 Chinese Hamster Ovary (CHO) cells that naturally secrete AβO (Rushworth et al., 2014). Interestingly, to validate the detection of AβO in conditioned media, the authors cultured the cells in the presence of βIV (BACE1 inhibitor), which prevents the generation of AβO by inactivation of BACE1. This experiment clearly demonstrated the biosensor's capability of functioning as a reliable source of AβO detection for AD diagnosis while also validating its use as a drug screening platform for BACE1. In 2011, Christopeit and colleagues developed a sophisticated drug screening platform with immobilized BACE1 on a plasma membrane-mimicking lipid layer (Christopeit et al., 2011). Vilela et al. reported an optical biosensor based on graphene oxide and upconversion NPs for the specific detection of BACE1 mRNA with a LOD of 500 fM (Vilela et al., 2017). The biosensor showed high specificity for BACE1 detection in spiked samples of healthy patient's plasma and cell lysates as well as long-term storage stability, demonstrating the clinical potential of the sensor.
Although Aβ1−42 and tau protein are well-established as AD diagnostic markers, they fail to provide the necessary specificity for effective diagnosis and disease progression assessment. Recent evidence suggests that the combination of multiple biomarkers may provide a more reliable and accurate diagnosis. For instance, Lewczuk et al. verified that Aβ1−42/Aβ1−40 concentration ratio is a better predictor of AD than Aβ1−42 alone (Lewczuk et al., 2015). Given the preponderant role of neuroinflammation in AD, monitoring circulating inflammatory mediators, such as cytokines, chemokines, and growth factors could provide valuable insights for early screening and treatment response evaluation (Laske et al., 2013; Delaby et al., 2015). Nevertheless, multiplex biosensor development for AD is still scarce, with only a few studies focused on Aβ detection (Xia et al., 2010; Liu et al., 2014).
Currently available biosensors are targeting markers such as the acetylcholine neurotransmitter (Chauhan and Pundir, 2014; Chauhan et al., 2017; Moreira et al., 2017), which is essential for memory processing, fibrinogen (Kim et al., 2016b), a clotting protein associated with Aβ aggregation (Cortes-Canteli et al., 2012) and APOE (Sciacca et al., 2013; Cheng et al., 2014). Nevertheless, single biomarker detection has fallen short for reliable AD diagnosis. Recently, Yang et al. devised an electrochemical biosensor for small-molecule O-GlcNAc transferase (OGT) inhibitor screening as an alternative to the conventional approaches (Yang et al., 2017). As it is known that aberrant activity of OGT may be involved in neurodegeneration and AD (Yuzwa and Vocadlo, 2014), the screening of OGT inhibitors could potentially lead to the development of targeted therapeutics and protein glycosylation pathway research. In this work, the authors studied the impact of concentration and incubation time of benzoxazolinone (BZX) and alloxan, which are known OGT inhibitors. This proof-of-concept study paves the way for the optimization of a label-free integrated platform for high-throughput drug screening of OGT inhibitors, specifically if multiple analytes or enzymes for O-linked glycosylation are analyzed simultaneously.
For PD, Yildirim et al. reported an optical technique for the detection of dopamine (DA) based on its oxidation and subsequent aggregation into NPs (polydopamine) (Yildirim and Bayindir, 2014). Interestingly, these NPs hold fluorescent properties, which allow the determination of DA concentrations with a detection limit of 40 nM. Additionally, Yue et al. reported the development of an electrochemical biosensor of vertically aligned ZnO nanowires on a 3D graphene foam for the detection of DA, uric acid (UA), and ascorbic acid (AA) (Yue et al., 2014). The use of 3D graphene foam enhanced electron transport due to its high conductivity and the vertical ZnO nanowires provided higher surface area. Importantly, the authors demonstrated the selectivity of the assay for DA, UA, and AA detection. The development of electrochemical biosensors for the specific detection of these molecules is particularly challenging, as they co-exist in serum with similar redox potential, thus limiting their oxidative peak discrimination. Of note, they verified that the UA serum levels for healthy individuals ranged from 325 to 385 μM, while PD patients presented values between 245 and 285 μM, suggesting that UA could be a potential marker for PD. Sensitive detection of DA has also been performed using electrolyte-gated field-effect transistors (EGFETs) with nanovesicles in a conducting polymer with immobilized human DA receptor D1 (Park et al., 2014). The authors reported a minimum detectable level of 1 nM for spiked DA in human serum, suggesting that this biosensor is suitable for PD diagnosis as DA reported values for PD are within the nM range. In a similar approach, Lee et al. developed a sensitive and reusable EGFET for DA detection using conductive polymer NPs coated with Pt particles (Lee et al., 2015), which act as catalysts for DA oxidation, enhancing signal detection, response time, and sensitivity. This sensor was able to detect DA in the fM concentration with minimal interference of AA or UA.
Although α-syn has been the most intensely studied and recognized biomarker for PD, its application in biosensing is very limited. A photoelectrochemical biosensor was developed by An et al. based on Au-doped TiO2 nanotube arrays for sensitive α-syn quantification with a detection limit of 34 pg/mL (An et al., 2010). Thrombin was been reported to induce apoptosis of dopaminergic neurons in rat substancia nigra (Choi et al., 2003) and microglia activation by inducing the expression of pro-inflammatory mediators TNFα, IL-1β, IL-6, and nitric oxide (Lee et al., 2005). Therefore, the detection and quantification of thrombin in the blood or CSF samples of PD patients could predict ongoing neuroinflammation while enabling disease diagnosis. An electrochemical biosensor for thrombin detection was developed by Bafrooei et al. using aptamers functionalized on a nanocomposite of multiwalled carbon nanotubes and TiO2 NPs (Heydari-Bafrooei et al., 2016). The aptasensor showed high specificity, sensitivity (in fM range) in blood, or CSF of PD patients.
The heterogeneous nature of MS, characterized by distinct patterns associated with the demyelination process, makes it highly improbable that a single diagnostic marker is capable of covering the full spectrum of MS subtypes (Lucchinetti et al., 2000). Lolli et al. developed a synthetic glycoprotein antigen probe, CSF114(Glc), for the specific recognition of autoantibodies present in the serum of MS patients (Lolli et al., 2005). The authors proved that the antibodies specific for CSF114(Glc) recognized myelin and oligodendrocyte autoantigens in human brain tissue. This knowledge enabled the development of a specific method for the identification of MS patients with antibody-mediated demyelination, a specific subset of MS patients. The same group later reported the development of a gold surface plasmon resonance (SPR) biosensor with covalently immobilized CSF114(Glc) for real-time MS diagnosis from serum (Real-Fernández et al., 2012). This SPR biosensor presented a mild sensitivity (36%) and elevated specificity (95%) relative to the identification of MS patients vs. healthy blood donors. Other than MS diagnosis, multiple autoantibody identification, and further clinical correlation could potentially be used to direct therapy and monitor its response.
Conclusion
An increasing number of studies are uncovering the beneficial and detrimental roles of microglia in neurodegenerative disease onset and progression. Pro-inflammatory cytokines can be used in combination with classical biomarkers for neurodegenerative and neuroinflammatory disease diagnostics and monitoring of disease progression. Technologies for simultaneous detection and quantification of different biomarkers are rapidly developing and future devices are aimed at bringing valuable advantages, specifically related to lower sample volumes, detection time and limits, higher specificity and sensitivity. Decreasing the need for biological samples processing, while integrating biosensing platforms in portable lab-on-a-chip systems would, in turn, allow point-of-care use by semi-skilled operators toward real-time and in situ early diagnostics of neuroinflammatory and neurodegenerative diseases. Altogether, these advantages will surely bring great benefits for both academic and medical fields.
Author Contributions
All authors listed have made a substantial, direct and intellectual contribution to the work, and approved it for publication.
Funding
IM and AC acknowledge the financial support from the Marie Curie COFUND Programme NanoTRAINforGrowth from the European Union's Seventh Framework Programme for research, technological development and demonstration under grant agreement no 600375. This article is a result of the project Nanotechnology based functional solutions (NORTE-01-0145-FEDER-000019), co-financed by Norte Portugal Regional Operational Programme (NORTE 2020), under the PORTUGAL 2020 Partnership Agreement, through the European Regional Development Fund (ERDF). PM acknowledges the Ph.D. fellowship from Fundação para a Ciência e Tecnologia, Portugal (PD/BD/105751/2014).
Conflict of Interest Statement
The authors declare that the research was conducted in the absence of any commercial or financial relationships that could be construed as a potential conflict of interest.
Acknowledgments
The authors acknowledge Helena Sofia Domingues for critical reading of the manuscript.
References
An, Y., Tang, L., Jiang, X., Chen, H., Yang, M., Jin, L., et al. (2010). A photoelectrochemical immunosensor based on au-doped TiO2 nanotube arrays for the detection of alpha-synuclein. Chemistry 16, 14439–14446. doi: 10.1002/chem.201001654
Andersen, A. D., Binzer, M., Stenager, E., and Gramsbergen, J. B. (2017). Cerebrospinal fluid biomarkers for Parkinson's disease - a systematic review. Acta Neurol. Scand. 135, 34–56. doi: 10.1111/ane.12590
Arellano, G., Acuña, E., Reyes, L. I., Ottum, P. A., Sarno, P., De Villarroel, L., et al. (2017). Th1 and Th17 cells and associated cytokines discriminate among clinically isolated syndrome and multiple sclerosis phenotypes. Front. Immunol. 8:753. doi: 10.3389/fimmu.2017.00753
Arya, S. K., and Estrela, P. (2017). Electrochemical immunosensor for tumor necrosis factor-alpha detection in undiluted serum. Methods 116, 125–131. doi: 10.1016/j.ymeth.2016.12.001
Atkinson, A. J., Colburn, W. A., DeGruttola, V. G., DeMets, D. L., Downing, G. J., Hoth, D. F., et al. (2001). Biomarkers and surrogate endpoints: preferred definitions and conceptual framework. Clin. Pharmacol. Ther. 69, 89–95. doi: 10.1067/mcp.2001.113989
Aydin, E. B., Aydin, M., and Sezgintürk, M. K. (2017). A highly sensitive immunosensor based on ITO thin films covered by a new semi-conductive conjugated polymer for the determination of TNFα in human saliva and serum samples. Biosens. Bioelectron. 97, 169–176. doi: 10.1016/j.bios.2017.05.056
Baraket, A., Lee, M., Zine, N., Sigaud, M., Bausells, J., and Errachid, A. (2017). A fully integrated electrochemical biosensor platform fabrication process for cytokines detection. Biosens. Bioelectron. 93, 170–175. doi: 10.1016/j.bios.2016.09.023
Baraket, A., Lee, M., Zine, N., Yaakoubi, N., Bausells, J., and Errachid, A. (2016). A flexible electrochemical micro lab-on-chip: application to the detection of interleukin-10. Microchim. Acta 183, 2155–2162. doi: 10.1007/s00604-016-1847-y
Bennett, M. R., and Devarajan, P. (2016). “Characteristics of an ideal biomarker of kidney diseases,” in Biomarkers of Kidney Disease, ed C. L. Edelstein (San Diego, CA: Elsevier), 1–20. doi: 10.1016/B978-0-12-803014-1.00001-7
Bhavsar, K., Fairchild, A., Alonas, E., Bishop, D. K., La Belle, J. T., Sweeney, J., et al. (2009). A cytokine immunosensor for Multiple Sclerosis detection based upon label-free electrochemical impedance spectroscopy using electroplated printed circuit board electrodes. Biosens. Bioelectron. 25, 506–509. doi: 10.1016/j.bios.2009.07.017
Biela, A., Watkinson, M., Meier, U. C., Baker, D., Giovannoni, G., Becer, C. R., et al. (2015). Disposable MMP-9 sensor based on the degradation of peptide cross-linked hydrogel films using electrochemical impedance spectroscopy. Biosens. Bioelectron. 68, 660–667. doi: 10.1016/j.bios.2015.01.060
Bradshaw, E. M., Chibnik, L. B., Keenan, B. T., Ottoboni, L., Raj, T., Tang, A., et al. (2013). CD33 Alzheimer's disease locus: Altered monocyte function and amyloid biology. Nat. Neurosci. 16, 848–850. doi: 10.1038/nn.3435
Brochard, V., Combadière, B., Prigent, A., Laouar, Y., Perrin, A., Beray-Berthat, V., et al. (2009). Infiltration of CD4+ lymphocytes into the brain contributes to neurodegeneration in a mouse model of Parkinson disease. J. Clin. Invest. 119, 182–192. doi: 10.1172/JCI36470
Brockmann, K., Schulte, C., Schneiderhan-Marra, N., Apel, A., Pont-Sunyer, C., Vilas, D., et al. (2017). Inflammatory profile discriminates clinical subtypes in LRRK2-associated Parkinson's disease. Eur. J. Neurol. 24, 427-e6. doi: 10.1111/ene.13223
Bronzuoli, M. R., Iacomino, A., Steardo, L., and Scuderi, C. (2016). Targeting neuroinflammation in Alzheimer's disease. J. Inflamm. Res. 9, 199–208. doi: 10.2147/JIR.S86958
Brosseron, F., Krauthausen, M., Kummer, M., and Heneka, M. T. (2014). Body fluid cytokine levels in mild cognitive impairment and Alzheimer's disease: a comparative overview. Mol. Neurobiol. 50, 534–544. doi: 10.1007/s12035-014-8657-1
Burman, J., Svensson, E., Fransson, M., Loskog, A. S. I., Zetterberg, H., Raininko, R., et al. (2014). The cerebrospinal fluid cytokine signature of multiple sclerosis: A homogenous response that does not conform to the Th1/Th2/Th17 convention. J. Neuroimmunol. 277, 153–159. doi: 10.1016/j.jneuroim.2014.10.005
Cala, C. M., Moseley, C. E., Steele, C., Dowdy, S. M., Cutter, G. R., Ness, J. M., et al. (2016). T cell cytokine signatures: biomarkers in pediatric multiple sclerosis. J. Neuroimmunol. 297, 1–8. doi: 10.1016/j.jneuroim.2016.04.015
Carneiro, P., Loureiro, J., Delerue-Matos, C., Morais, S., and do Carmo Pereira, M. (2017). Alzheimer's disease: Development of a sensitive label-free electrochemical immunosensor for detection of amyloid beta peptide. Sensors Actu. B Chem. 239, 157–165. doi: 10.1016/j.snb.2016.07.181
Chauhan, N., Chawla, S., Pundir, C. S., and Jain, U. (2017). An electrochemical sensor for detection of neurotransmitter-acetylcholine using metal nanoparticles, 2D material and conducting polymer modified electrode. Biosens. Bioelectron. 89, 377–383. doi: 10.1016/j.bios.2016.06.047
Chauhan, N., and Pundir, C. S. (2014). Amperometric determination of acetylcholine—A neurotransmitter, by chitosan/gold-coated ferric oxide nanoparticles modified gold electrode. Biosens. Bioelectron. 61, 1–8. doi: 10.1016/j.bios.2014.04.048
Chen, P., Chung, M. T., McHugh, W., Nidetz, R., Li, Y., Fu, J., et al. (2015). Multiplex serum cytokine immunoassay using nanoplasmonic biosensor microarrays. ACS Nano 9, 4173–4181. doi: 10.1021/acsnano.5b00396
Cheng, X. R., Hau, B. Y., Endo, T., and Kerman, K. (2014). Au nanoparticle-modified DNA sensor based on simultaneous electrochemical impedance spectroscopy and localized surface plasmon resonance. Biosens. Bioelectron. 53, 513–518. doi: 10.1016/j.bios.2013.10.003
Choi, C., Jeong, J. H., Jang, J. S., Choi, K., Kwon, J. L., Kwon, J., et al. (2008). Multiplex analysis of cytokines in the serum and cerebrospinal fluid of patients with Alzheimer's disease by color-coded bead technology. J. Clin. Neurol. 4, 84–88. doi: 10.3988/jcn.2008.4.2.84
Choi, I.-Y., Lee, P., Adany, P., Hughes, A. J., Belliston, S., Denney, D. R., et al. (2017). In vivo evidence of oxidative stress in brains of patients with progressive multiple sclerosis. Ther. Adv. Hematol. 8, 153 −156. doi: 10.1177/1352458517711568
Choi, S.-H., Le, D. Y., Ryu, J. K., Kim, J., Joe, E. H., and Jin, B. K. (2003). Thrombin induces nigral dopaminergic neurodegeneration in vivo by altering expression of death-related proteins. Neurobiol. Dis. 14, 181–193. doi: 10.1016/S0969-9961(03)00085-8
Christopeit, T., Stenberg, G., Gossas, T., Nyström, S., Baraznenok, V., Lindström, E., et al. (2011). A surface plasmon resonance-based biosensor with full-length BACE1 in a reconstituted membrane. Anal. Biochem. 414, 14–22. doi: 10.1016/j.ab.2011.02.041
Çomoglu, S. S., Güven, H., Acar, M., Öztürk, G., and Koçer, B. (2013). Tear levels of tumor necrosis factor-alpha in patients with Parkinson's disease. Neurosci. Lett. 553, 63–67. doi: 10.1016/j.neulet.2013.08.019
Cortes-Canteli, M., Zamolodchikov, D., Ahn, H. J., Strickland, S., and Norris, E. H. (2012). Fibrinogen and altered hemostasis in Alzheimer's disease. J. Alzheimer's Dis. 32, 599–608. doi: 10.3233/JAD-2012-120820
Craig-Schapiro, R., Kuhn, M., Xiong, C., Pickering, E. H., Liu, J., Misko, T. P., et al. (2011). Multiplexed immunoassay panel identifies novel CSF biomarkers for alzheimer's disease diagnosis and prognosis. PLoS ONE 6:e18850. doi: 10.1371/journal.pone.0018850
Crehan, H., Hardy, J., and Pocock, J. (2013). Blockage of CR1 prevents activation of rodent microglia. Neurobiol. Dis. 54, 139–149. doi: 10.1016/j.nbd.2013.02.003
Crotti, A., and Ransohoff, R. M. (2016). Microglial physiology and pathophysiology: insights from genome-wide transcriptional profiling. Immunity 44, 505–515. doi: 10.1016/j.immuni.2016.02.013
D'Ambrosio, A., Pontecorvo, S., Colasanti, T., Zamboni, S., Francia, A., and Margutti, P. (2015). Peripheral blood biomarkers in multiple sclerosis. Autoimmun. Rev. 14, 1097–1110. doi: 10.1016/j.autrev.2015.07.014
Dai, Y., Molazemhosseini, A., and Liu, C. C. (2017). A single-use, in vitro biosensor for the detection of t-tau protein, a biomarker of neuro-degenerative disorders, in pbs and human serum using differential pulse voltammetry (DPV). Biosensors 7, 1–11. doi: 10.3390/bios7010010
Dansokho, C., and Heneka, M. T. (2017). Neuroinflammatory responses in Alzheimer's disease. J. Neural Transm. 125, 771–779. doi: 10.1007/s00702-017-1831-7.
Delaby, C., Gabelle, A., Blum, D., Schraen-Maschke, S., Moulinier, A., Boulanghien, J., et al. (2015). Central nervous system and peripheral inflammatory processes in Alzheimer's disease: biomarker profiling approach. Front. Neurol. 6:181. doi: 10.3389/fneur.2015.00181
Delgado-Alvarado, M., Gago, B., Gorostidi, A., Jiménez-Urbieta, H., Dacosta-Aguayo, R., Navalpotro-Gómez, I., et al. (2017). Tau/α-synuclein ratio and inflammatory proteins in Parkinson's disease: an exploratory study. Mov. Disord. 32, 1066–1073. doi: 10.1002/mds.27001
Dendrou, C. A., Fugger, L., and Friese, M. A. (2015). Immunopathology of multiple sclerosis. Nat. Rev. Immunol. 15, 545–558. doi: 10.1038/nri3871
Derkus, B., Acar Bozkurt, P., Tulu, M., Emregul, K. C., Yucesan, C., and Emregul, E. (2017). Simultaneous quantification of myelin basic protein and tau proteins in cerebrospinal fluid and serum of multiple Sclerosis patients using nanoimmunosensor. Biosens. Bioelectron. 89, 781–788. doi: 10.1016/j.bios.2016.10.019
Derkus, B., Emregul, E., Yucesan, C., and Cebesoy Emregul, K. (2013). Myelin basic protein immunosensor for multiple sclerosis detection based upon label-free electrochemical impedance spectroscopy. Biosens. Bioelectron. 46, 53–60. doi: 10.1016/j.bios.2013.01.060
Devos, D., Forzy, G., de Seze, J., Caillez, S., Louchart, P., Gallois, P., et al. (2001). Silver stained isoelectrophoresis of tears and cerebrospinal fluid in multiple sclerosis. J. Neurol. 248, 672–675. doi: 10.1007/PL00007833
Drulović, J., Mostarica-Stojkovi,ć, M., Levi,ć, Z., Mesaros, S., Stojsavljevi,ć, N., Popadi,ć, D., et al. (1998). Serum interleukin-12 levels in patients with multiple sclerosis. Neurosci. Lett. 251, 129–132. doi: 10.1016/S0304-3940(98)00520-5
Dufek, M., Hamanov,á, M., Lokaj, J., Goldemund, D., Rektorov,á, I., Michálková, Z., et al. (2009). Serum inflammatory biomarkers in Parkinson's disease. Park. Relat. Disord. 15, 318–320. doi: 10.1016/j.parkreldis.2008.05.014
Dursun, E., Gezen-Ak, D., Hanagasi, H., Bilgi,ç, B., Lohmann, E., Ertan, S., et al. (2015). The interleukin 1 alpha, interleukin 1 beta, interleukin 6 and alpha-2-macroglobulin serum levels in patients with early or late onset Alzheimer's disease, mild cognitive impairment or Parkinson's disease. J. Neuroimmunol. 283, 50–57. doi: 10.1016/j.jneuroim.2015.04.014.
Edwards, K. R., Goya, J., Plavina, T., Czerkowicz, J., Goelz, S., Ranger, A., et al. (2013). Feasibility of the use of combinatorial chemokine arrays to study blood and CSF in multiple sclerosis. PLoS ONE 8:e81007. doi: 10.1371/journal.pone.0081007
El Harrad, L., Bourais, I., Mohammadi, H., and Amine, A. (2018). Recent advances in electrochemical biosensors based on enzyme inhibition for clinical and pharmaceutical applications. Sensors 18:164. doi: 10.3390/s18010164
Fainardi, E., Castellazzi, M., Bellini, T., Manfrinato, M. C., Baldi, E., Casetta, I., et al. (2006). Cerebrospinal fluid and serum levels and intrathecal production of active matrix metalloproteinase-9 (MMP-9) as markers of disease activity in patients with multiple sclerosis. Mult. Scler. 12, 294–301. doi: 10.1191/135248506ms1274oa
Farfel, J. M., Yu, L., Buchman, A. S., Schneider, J. A., De Jager, P. L., and Bennett, D. A. (2016). Relation of genomic variants for Alzheimer disease dementia to common neuropathologies. Neurology 87, 489–496. doi: 10.1212/WNL.0000000000002909
Fonseca, M. I., Chu, S.-H., Hernandez, M. X., Fang, M. J., Modarresi, L., Selvan, P., et al. (2017). Cell-specific deletion of C1qa identifies microglia as the dominant source of C1q in mouse brain. J. Neuroinflammation 14:48. doi: 10.1186/s12974-017-0814-9
Gagni, P., Sola, L., Cretich, M., and Chiari, M. (2013). Development of a high-sensitivity immunoassay for amyloid-beta 1-42 using a silicon microarray platform. Biosens. Bioelectron. 47, 490–495. doi: 10.1016/j.bios.2013.03.077
Gerhard, A., Pavese, N., Hotton, G., Turkheimer, F., Es, M., Hammers, A., et al. (2006). In vivo imaging of microglial activation with [11C](R)-PK11195 PET in idiopathic Parkinson's disease. Neurobiol. Dis. 21, 404–412. doi: 10.1016/j.nbd.2005.08.002
Ginhoux, F., and Guilliams, M. (2016). Tissue-Resident Macrophage ontogeny and homeostasis. Immunity 44, 439–449. doi: 10.1016/j.immuni.2016.02.024
Giovannoni, G. (2014). “Chapter 30: Cerebrospinal fluid analysis,” in Multiple Sclerosis and Related Disorders, ed D. S. Goodin (San Diego, CA: Elsevier), 681–702. doi: 10.1016/B978-0-444-52001-2.00029-7
Govindaraju, S., Ankireddy, S. R., Viswanath, B., Kim, J., Yun, K., Thirumalraj, B., et al. (2017). Fluorescent gold nanoclusters for selective detection of dopamine in cerebrospinal fluid. Sci. Rep. 7, 1–12. doi: 10.1038/srep40298
Griciuc, A., Serrano-Pozo, A., Parrado, A. R., Lesinski, A. N., Asselin, C. N., Mullin, K., et al. (2013). Alzheimer's disease risk gene CD33 inhibits microglial uptake of amyloid beta. Neuron 78, 631–643. doi: 10.1016/j.neuron.2013.04.014
Griffin, W. S. T., Liu, L., Li, Y., Mrak, R. E., and Barger, S. W. (2006). Interleukin-1 mediates Alzheimer and Lewy body pathologies. J. Neuroinflammation 3:5. doi: 10.1186/1742-2094-3-5
Guerreiro, R., Wojtas, A., Bras, J., Carrasquillo, M., Rogaeva, E., Majounie, E., et al. (2013). TREM2 variants in Alzheimer's disease. N. Engl. J. Med. 368, 117–127. doi: 10.1056/NEJMoa1211851.
Guillot-Sestier, M. V., and Town, T. (2017). Let's make microglia great again in neurodegenerative disorders. J. Neural. Transm. 125, 751–770. doi: 10.1007/s00702-017-1792-x
Hansson, O., Janelidze, S., Hall, S., Magdalinou, N., Lees, A. J., Andreasson, U., et al. (2017). Blood-based NfL. Neurology 88, 930–937. doi: 10.1212/WNL.0000000000003680
Hay, S. I., Abajobir, A. A., Abate, K. H., Abbafati, C., Abbas, K. M., Abd-Allah, F., et al. (2017). Global, regional, and national disability-adjusted life-years (DALYs) for 333 diseases and injuries and healthy life expectancy (HALE) for 195 countries and territories, 1990-2016: a systematic analysis for the Global Burden of Disease Study 2016. Lancet 390, 1260–1344. doi: 10.1016/S0140-6736(17)32130-X
Hegen, H., Adrianto, I., Lessard, C. J., Millonig, A., Bertolotto, A., Comabella, M., et al. (2016). Cytokine profiles show heterogeneity of interferon-beta response in multiple sclerosis patients. Neurol. Neuroimmunol. Neuroinflammation 3:e202. doi: 10.1212/NXI.0000000000000202
Heneka, M. T., Kummer, M. P., Stutz, A., Delekate, A., Schwartz, S., Vieira-Saecker, A., et al. (2013). NLRP3 is activated in Alzheimer's disease and contributes to pathology in APP/PS1 mice. Nature 493, 674–678. doi: 10.1038/nature11729
Hesse, R., Wahler, A., Gummert, P., Kirschmer, S., Otto, M., Tumani, H., et al. (2016). Decreased IL-8 levels in CSF and serum of AD patients and negative correlation of MMSE and IL-1β. BMC Neurol. 16:185. doi: 10.1186/s12883-016-0707-z
Heydari-Bafrooei, E., Amini, M., and Ardakani, M. H. (2016). An electrochemical aptasensor based on TiO2/MWCNT and a novel synthesized Schiff base nanocomposite for the ultrasensitive detection of thrombin. Biosens. Bioelectron. 85, 828–836. doi: 10.1016/j.bios.2016.06.012
Hölttä, M., Minthon, L., Hansson, O., Holmén-Larsson, J., Pike, I., Ward, M., et al. (2015). An integrated workflow for multiplex CSF proteomics and peptidomics-identification of candidate cerebrospinal fluid biomarkers of alzheimer's disease. J. Proteome Res. 14, 654–663. doi: 10.1021/pr501076j
Hong, S., Beja-Glasser, V. F., Nfonoyim, B. M., Frouin, A., Li, S., Ramakrishnan, S., et al. (2016). Complement and microglia mediate early synapse loss in Alzheimer mouse models. Science 352, 712–716. doi: 10.1126/science.aad8373
Honig, L. S., Vellas, B., Woodward, M., Boada, M., Bullock, R., Borrie, M., et al. (2018). Trial of solanezumab for mild dementia due to Alzheimer's Disease. N. Engl. J. Med. 378, 321–330. doi: 10.1056/NEJMoa1705971
Housley, W. J., Pitt, D., and Hafler, D. A. (2015). Biomarkers in multiple sclerosis. Clin. Immunol. 161, 51–58. doi: 10.1016/j.clim.2015.06.015
Huang, J., Chen, H., Niu, W., Fam, D. W. H., Palaniappan, A., Larisika, M., et al. (2015). Highly manufacturable graphene oxide biosensor for sensitive Interleukin-6 detection. RSC Adv. 5, 39245–39251. doi: 10.1039/C5RA05854F
Huang, Q., Chen, H., Xu, L., Lu, D., Tang, L., Jin, L., et al. (2013). Visible-light-activated photoelectrochemical biosensor for the study of acetylcholinesterase inhibition induced by endogenous neurotoxins. Biosens. Bioelectron. 45, 292–299. doi: 10.1016/j.bios.2013.01.075
Huynh, R. A., and Mohan, C. (2017). Alzheimer's disease: biomarkers in the genome, blood, and cerebrospinal fluid. Front. Neurol. 8:102. doi: 10.3389/fneur.2017.00102
Inglese, M., and Petracca, M. (2013). Imaging multiple sclerosis and other neurodegenerative diseases. Prion 7, 47–54. doi: 10.4161/pri.22650
Kalia, L. V., and Lang, A. E. (2015). Parkinson's disease. Lancet 386, 896–912. doi: 10.1016/S0140-6736(14)61393-
Khaibullin, T., Ivanova, V., Martynova, E., Cherepnev, G., Khabirov, F., Granatov, E., et al. (2017). Elevated levels of proinflammatory cytokines in cerebrospinal fluid of multiple sclerosis patients. Front. Immunol. 8:531. doi: 10.3389/fimmu.2017.00531
Kim, H., Lee, J. U., Song, S., Kim, S., and Sim, S. J. (2018). A shape-code nanoplasmonic biosensor for multiplex detection of Alzheimer's disease biomarkers. Biosens. Bioelectron. 101, 96–102. doi: 10.1016/j.bios.2017.10.018
Kim, J., Chae, M.-S., Lee, S. M., Jeong, D., Lee, B. C., Lee, J. H., et al. (2016a). Wafer-scale high-resolution patterning of reduced graphene oxide films for detection of low concentration biomarkers in plasma. Sci. Rep. 6:31276. doi: 10.1038/srep31276
Kim, J., Kim, S., Nguyen, T. T., Lee, R., Li, T., Yun, C., et al. (2016b). Label-free quantitative immunoassay of fibrinogen in alzheimer disease patient plasma using fiber optical surface plasmon resonance. J. Electron. Mater. 45, 2354–2360. doi: 10.1007/s11664-015-4292-5
Kothur, K., Wienholt, L., Brilot, F., and Dale, R. C. (2016). CSF cytokines/chemokines as biomarkers in neuroinflammatory CNS disorders: a systematic review. Cytokine 77, 227–237. doi: 10.1016/j.cyto.2015.10.001
Koziorowski, D., Tomasiuk, R., Szlufik, S., and Friedman, A. (2012). Inflammatory cytokines and NT-proCNP in Parkinson's disease patients. Cytokine 60, 762–766. doi: 10.1016/j.cyto.2012.07.030
Kumar, N., Hu, Y., Singh, S., and Mizaikoff, B. (2018). Emerging biosensor platforms for the assessment of water-borne pathogens. Analyst 143, 359–373. doi: 10.1039/C7AN00983F
Labzin, L. I., Heneka, M. T., and Latz, E. (2018). Innate immunity and neurodegeneration. Annu. Rev. Med. 69, 437–449. doi: 10.1146/annurev-med-050715-104343
Lane, C. A., Hardy, J., and Schott, J. M. (2018). Alzheimer's disease. Eur. J. Neurol. 25, 59–70. doi: 10.1111/ene.13439
Laske, C., Schmohl, M., Leyhe, T., Stransky, E., Maetzler, W., Berg, D., et al. (2013). Immune profiling in blood identifies sTNF-R1 performing comparably well as biomarker panels for classification of alzheimer's disease patients. J. Alzheimer's Dis. 34, 367–375. doi: 10.3233/JAD-121558
Law, J. W. F., Mutalib, N. S. A., Chan, K. G., and Lee, L. H. (2014). Rapid methods for the detection of foodborne bacterial pathogens: principles, applications, advantages and limitations. Front. Microbiol. 5:770. doi: 10.3389/fmicb.2014.00770
Lee, D. Y., Oh, Y. J., and Jin, B. K. (2005). Thrombin-activated microglia contribute to death of dopaminergic neurons in rat mesencephalic cultures: dual roles of mitogen-activated protein kinase signaling pathways. Glia 51, 98–110. doi: 10.1002/glia.20190
Lee, H., James, W. S., and Cowley, S. A. (2017). LRRK2 in peripheral and central nervous system innate immunity: its link to Parkinson's disease. Biochem. Soc. Trans. 45, 131–139. doi: 10.1042/BST20160262
Lee, J. S., Oh, J., Kim, S. G., and Jang, J. (2015). Highly sensitive and selective field-effect-transistor nonenzyme dopamine sensors based on Pt/conducting polymer hybrid nanoparticles. Small 11, 2399–2406. doi: 10.1002/smll.201403263
Lei, K. F., Tseng, H. P., Lee, C. Y., and Tsang, N. M. (2016). Quantitative study of cell invasion process under extracellular stimulation of cytokine in a microfluidic device. Sci. Rep. 6, 6–13. doi: 10.1038/srep25557
Leszek, J. E., Barreto, G., Gsiorowski, K., Koutsouraki, E., Ávila-Rodrigues, M., and Aliev, G. (2016). Inflammatory mechanisms and oxidative stress as key factors responsible for progression of neurodegeneration: role of brain innate immune system. CNS Neurol. Disord. Drug Targets 15, 329–336. doi: 10.2174/1871527315666160202125914
Lewczuk, P., Lelental, N., Spitzer, P., Maler, J. M., and Kornhuber, J. (2015). Amyloid-β 42/40 cerebrospinal fluid concentration ratio in the diagnostics of Alzheimer's disease: validation of two novel assays. J. Alzheimers. Dis. 43, 183–191. doi: 10.3233/JAD-140771
Li, S.-S., Lin, C.-W., Wei, K.-C., Huang, C.-Y., Hsu, P.-H., Liu, H.-L., et al. (2016). Non-invasive screening for early Alzheimer's disease diagnosis by a sensitively immunomagnetic biosensor. Sci. Rep. 6:25155. doi: 10.1038/srep25155
Liddelow, S. A., Guttenplan, K. A., Clarke, L. E., Bennett, F. C., Bohlen, C. J., Schirmer, L., et al. (2017). Neurotoxic reactive astrocytes are induced by activated microglia. Nature 541, 481–487. doi: 10.1038/nature21029
Liu, L., He, Q., Zhao, F., Xia, N., Liu, H., Li, S., et al. (2014). Competitive electrochemical immunoassay for detection of beta-amyloid (1-42) and total beta-amyloid peptides using p-aminophenol redox cycling. Biosens. Bioelectron. 51, 208–212. doi: 10.1016/j.bios.2013.07.047
Lolli, F., Mulinacci, B., Carotenuto, A., Bonetti, B., Sabatino, G., Mazzanti, B., et al. (2005). An N-glucosylated peptide detecting disease-specific autoantibodies, biomarkers of multiple sclerosis. Proc. Natl. Acad. Sci. U.S.A. 102, 10273–10278. doi: 10.1073/pnas.0503178102
Lucchinetti, C., Brück, W., Parisi, J., Scheithauer, B., Rodriguez, M., and Lassmann, H. (2000). Heterogeneity of multiple sclerosis lesions: implications for the pathogenesis of demyelination. Ann. Neurol. 47, 707–17. doi: 10.1002/1531-8249(200006)47:6<707::AID-ANA3>3.0.CO;2-Q
Lue, L.-F., Schmitz, C. T., Snyder, N. L., Chen, K., Walker, D. G., Davis, K. J., et al. (2016). Converging mediators from immune and trophic pathways to identify Parkinson disease dementia. Neurol. Neuroimmunol. Neuroinflammation 3:e193. doi: 10.1212/NXI.0000000000000193
Luo, C., Jian, C., Liao, Y., Huang, Q., Wu, Y., Liu, X., et al. (2017). The role of microglia in multiple sclerosis. Neuropsychiatr. Dis. Treat. 13, 1661–1667. doi: 10.2147/NDT.S140634
Martins, T. B., Rose, J. W., Jaskowski, T. D., Wilson, A. R., Husebye, D., Seraj, H. S., et al. (2011). Analysis of proinflammatory and anti-inflammatory cytokine serum concentrations in patients with multiple sclerosis by using a multiplexed immunoassay. Am. J. Clin. Pathol. 136, 696–704. doi: 10.1309/AJCP7UBK8IBVMVNR
Mawuenyega, K. G., Sigurdson, W., Ovod, V., Munsell, L., Kasten, T., Morris, J. C., et al. (2010). Decreased clearance of CNS beta-amyloid in Alzheimer's disease. Science 330:1774. doi: 10.1126/science.1197623
McDonald, C. R., McEvoy, L. K., Gharapetian, L., Fennema-Notestine, C., Hagler, D. J., Holland, D., et al. (2009). Regional rates of neocortical atrophy from normal aging to early Alzheimer disease. Neurology 73, 457–465. doi: 10.1212/WNL.0b013e3181b16431
McGeer, P. L., Itagaki, S., Boyes, B. E., and McGeer, E. G. (1988). Reactive microglia are positive for HLA-DR in the substantia nigra of Parkinson's and Alzheimer's disease brains. Neurology 38, 1285–1285.
McKhann, G. M., Knopman, D. S., Chertkow, H., Hyman, B. T., Jack, C. R., Kawas, C. H., et al. (2011). The diagnosis of dementia due to Alzheimer's disease: recommendations from the National Institute on Aging-Alzheimer's Association workgroups on diagnostic guidelines for Alzheimer's disease. Alzheimer's Dement. 7, 263–269. doi: 10.1016/j.jalz.2011.03.005
Mogi, M., Harada, M., Kondo, T., Riederer, P., Inagaki, H., Minami, M., et al. (1994a). Interleukin-1β, interleukin-6, epidermal growth factor and transforming growth factor-α are elevated in the brain from parkinsonian patients. Neurosci. Lett. 180, 147–150. doi: 10.1016/0304-3940(94)90508-8
Mogi, M., Harada, M., Riederer, P., Narabayashi, H., Fujita, K., and Nagatsu, T. (1994b). Tumor necrosis factor-α (TNF-α) increases both in the brain and in the cerebrospinal fluid from parkinsonian patients. Neurosci. Lett. 165, 208–210. doi: 10.1016/0304-3940(94)90746-3
Moreira, F. T. C., Sale, M. G. F., and Di Lorenzo, M. (2017). Towards timely Alzheimer diagnosis: a self-powered amperometric biosensor for the neurotransmitter acetylcholine. Biosens. Bioelectron. 87, 607–614. doi: 10.1016/j.bios.2016.08.104
Mowry, E. M., Deen, S., Malikova, I., Pelletier, J., Bacchetti, P., and Waubant, E. (2009). The onset location of multiple sclerosis predicts the location of subsequent relapses. J. Neurol. Neurosurg. Psychiatr. 80, 400–403. doi: 10.1136/jnnp.2008.157305
Musunuri, S., Wetterhall, M., Ingelsson, M., Lannfelt, L., Artemenko, K., Bergquist, J., et al. (2014). Quantification of the brain proteome in Alzheimer's disease using multiplexed mass spectrometry. J. Proteome Res. 13, 2056–2068. doi: 10.1021/pr401202d
Novakova, L., Axelsson, M., Khademi, M., Zetterberg, H., Blennow, K., Malmeström, C., et al. (2017a). Cerebrospinal fluid biomarkers as a measure of disease activity and treatment efficacy in relapsing-remitting multiple sclerosis. J. Neurochem. 141, 296–304. doi: 10.1111/jnc.13881
Novakova, L., Zetterberg, H., Sundström, P., Axelsson, M., Khademi, M., Gunnarsson, M., et al. (2017b). Monitoring disease activity in multiple sclerosis using serum neurofilament light protein. Neurology 89, 2230–2237. doi: 10.1212/WNL.0000000000004683
Oh, J., Yoo, G., Chang, Y. W., Kim, H. J., Jose, J., Kim, E., et al. (2013). A carbon nanotube metal semiconductor field effect transistor-based biosensor for detection of amyloid-beta in human serum. Biosens. Bioelectron. 50, 345–350. doi: 10.1016/j.bios.2013.07.004
Olsson, B., Lautner, R., Andreasson, U., Öhrfelt, A., Portelius, E., Bjerke, M., et al. (2016). CSF and blood biomarkers for the diagnosis of Alzheimer's disease: a systematic review and meta-analysis. Lancet Neurol. 15, 673–684. doi: 10.1016/S1474-4422(16)00070-3
Opsahl, J. A., Vaudel, M., Guldbrandsen, A., Aasebø, E., Van Pesch, V., Franciotta, D., et al. (2016). Label-free analysis of human cerebrospinal fluid addressing various normalization strategies and revealing protein groups affected by multiple sclerosis. Proteomics 16, 1154–1165. doi: 10.1002/pmic.201500284
Ortiz, G. G., Pacheco-Moisés, F. P., Bitzer-Quintero, O. K., Ramírez-Anguiano, A. C., Flores-Alvarado, L. J., Ramírez-Ramírez, V., et al. (2013). Immunology and oxidative stress in multiple sclerosis: clinical and basic approach. Clin. Dev. Immunol. 2013:708659. doi: 10.1155/2013/708659
Parikh, C. R., and Thiessen-Philbrook, H. (2014). Key concepts and limitations of statistical methods for evaluating biomarkers of kidney disease. J. Am. Soc. Nephrol. 25, 1621–1629. doi: 10.1681/ASN.2013121300
Park, S. J., Song, H. S., Kwon, O. S., Chung, J. H., Lee, S. H., An, J. H., et al. (2014). Human dopamine receptor nanovesicles for gate-potential modulators in high-performance field-effect transistor biosensors. Sci. Rep. 4:4342. doi: 10.1038/srep04342
Pascual, B., Prieto, E., Arbizu, J., Marti-Climent, J. M., Peñuelas, I., Quincoces, G., et al. (2012). Decreased carbon-11-flumazenil binding in early Alzheimer's disease. Brain 135, 2817–2825. doi: 10.1093/brain/aws210
Paterson, R. W., Heywood, W. E., Heslegrave, A. J., Magdalinou, N. K., Andreasson, U., Sirka, E., et al. (2016). A targeted proteomic multiplex CSF assay identifies increased malate dehydrogenase and other neurodegenerative biomarkers in individuals with Alzheimer's disease pathology. Transl. Psychiatry 6:e952. doi: 10.1038/tp.2016.194
Pires, L. R., Marques, F., Sousa, J. C., Cerqueira, J., and Pinto, I. M. (2016). Nano- and micro-based systems for immunotolerance induction in multiple sclerosis. Hum. Vaccines Immunother. 12, 1886–1890. doi: 10.1080/21645515.2016.1138190
Poewe, W., Seppi, K., Tanner, C. M., Halliday, G. M., Brundin, P., Volkmann, J., et al. (2017). Parkinson disease. Nat. Rev. Dis. Prim. 3, 1–21. doi: 10.1038/nrdp.2017.13
Polvikoski, T., Sulkava, R., Haltia, M., Kainulainen, K., Vuorio, A., Verkkoniemi, A., et al. (1995). Apolipoprotein E, dementia, and cortical deposition of beta-amyloid protein. N. Engl. J. Med. 333, 1242–1247. doi: 10.1056/NEJM199511093331902
Popp, J., Oikonomidi, A., Tautvydait,e, D., Dayon, L., Bacher, M., Migliavacca, E., et al. (2017). Markers of neuroinflammation associated with Alzheimer's disease pathology in older adults. Brain. Behav. Immun. 62, 203–211. doi: 10.1016/j.bbi.2017.01.020
Postuma, R. B., Berg, D., Stern, M., Poewe, W., Olanow, C. W., Oertel, W., et al. (2015). MDS clinical diagnostic criteria for Parkinson's disease. Mov. Disord. 30, 1591–1601. doi: 10.1002/mds.26424
Prabhulkar, S., Piatyszek, R., Cirrito, J. R., Wu, Z. Z., and Li, C. Z. (2012). Microbiosensor for Alzheimer's disease diagnostics: detection of amyloid beta biomarkers. J. Neurochem. 122, 374–381. doi: 10.1111/j.1471-4159.2012.07709.x
Rapini, R., and Marrazza, G. (2017). Electrochemical aptasensors for contaminants detection in food and environment: recent advances. Bioelectrochemistry 118, 47–61. doi: 10.1016/j.bioelechem.2017.07.004
Real-Fernández, F., Passalacqua, I., Peroni, E., Chelli, M., Lolli, F., Papini, A. M., et al. (2012). Glycopeptide-based antibody detection in multiple sclerosis by surface plasmon resonance. Sensors 12, 5596–5607. doi: 10.3390/s120505596
Reich, D. S., Lucchinetti, C. F., and Calabresi, P. A. (2018). Multiple sclerosis. N. Engl. J. Med. 378, 169–180. doi: 10.1056/NEJMra1401483
Rocha, N. P., De Miranda, A. S., and Teixeira, A. L. (2015). Insights into neuroinflammation in Parkinson's disease: from biomarkers to anti-inflammatory based therapies. Biomed. Res. Int. 2015:628192. doi: 10.1155/2015/628192
Rushworth, J. V., Ahmed, A., Griffiths, H. H., Pollock, N. M., Hooper, N. M., and Millner, P. A. (2014). A label-free electrical impedimetric biosensor for the specific detection of Alzheimer's amyloid-beta oligomers. Biosens. Bioelectron. 56, 83–90. doi: 10.1016/j.bios.2013.12.036
Russell, A. C., Šimurina, M., Garcia, M. T., Novokmet, M., Wang, Y., Rudan, I., et al. (2017). The N-glycosylation of immunoglobulin G as a novel biomarker of Parkinson's disease. Glycobiology 27, 501–510. doi: 10.1093/glycob/cwx022
Schuh, C., Wimmer, I., Hametner, S., Haider, L., Van Dam, A. M., Liblau, R. S., et al. (2014). Oxidative tissue injury in multiple sclerosis is only partly reflected in experimental disease models. Acta Neuropathol. 128, 247–266. doi: 10.1007/s00401-014-1263-5
Sciacca, B., François, A., Klingler-Hoffmann, M., Brazzatti, J., Penno, M., Hoffmann, P., et al. (2013). Radiative-surface plasmon resonance for the detection of apolipoprotein E in medical diagnostics applications. Nanomedicine 9, 550–557. doi: 10.1016/j.nano.2012.10.007
Song, Y., Chen, P., Chung, M. T., Nidetz, R., Park, Y., Liu, Z., et al. (2017). AC electroosmosis-enhanced nanoplasmofluidic detection of ultralow-concentration cytokine. Nano Lett. 17, 2374–2380. doi: 10.1021/acs.nanolett.6b05313
Spellman, D. S., Wildsmith, K. R., Honigberg, L. A., Tuefferd, M., Baker, D., Raghavan, N., et al. (2015). Development and evaluation of a multiplexed mass spectrometry based assay for measuring candidate peptide biomarkers in Alzheimer' s Disease Neuroimaging Initiative (ADNI) CSF. Proteomics Clin. Appl. 9, 715–731. doi: 10.1002/prca.201400178
Stilund, M., Gjelstrup, M. C., Petersen, T., Møller, H. J., Rasmussen, P. V., and Christensen, T. (2015). Biomarkers of inflammation and axonal degeneration/damage in patients with newly diagnosed multiple sclerosis: contributions of the soluble CD163 CSF/serum ratio to a biomarker panel. PLoS ONE 10:e119681. doi: 10.1371/journal.pone.0119681
Stumpf, C., Lehner, C., Yilmaz, A., Daniel, W. G., and Garlichs, C. D. (2003). Decrease of serum levels of the anti-inflammatory cytokine interleukin-10 in patients with advanced chronic heart failure. Clin. Sci. 105, 45–50. doi: 10.1042/CS20020359
Sulzer, D., Alcalay, R. N., Garretti, F., Cote, L., Kanter, E., Agin-Liebes, J., et al. (2017). T cells from patients with Parkinson's disease recognize α-synuclein peptides. Nature 546, 656–661. doi: 10.1038/nature22815
Teunissen, C. E., Malekzadeh, A., Leurs, C., Bridel, C., and Killestein, J. (2015). Body fluid biomarkers for multiple sclerosis—the long road to clinical application. Nat. Rev. Neurol. 11, 585–596. doi: 10.1038/nrneurol.2015.173
Thompson, A. J., Banwell, B. L., Barkhof, F., Carroll, W. M., Coetzee, T., Comi, G., et al. (2017). Diagnosis of multiple sclerosis: 2017 revisions of the McDonald criteria. Lancet Neurol. 17, 162–173. doi: 10.1016/S1474-4422(17)30470-2
Vasilescu, A., and Marty, J. L. (2016). Electrochemical aptasensors for the assessment of food quality and safety. Trends Anal. Chem. 79, 60–70. doi: 10.1016/j.trac.2015.11.024
Vilela, P., El-Sagheer, A., Millar, T. M., Brown, T., Muskens, O. L., and Kanaras, A. G. (2017). Graphene oxide-upconversion nanoparticle based optical sensors for targeted detection of mRNA biomarkers present in Alzheimer's disease and prostate cancer. ACS Sens. 2, 52–56. doi: 10.1021/acssensors.6b00651
Wang, S. X., Acha, D., Shah, A. J., Hills, F., Roitt, I., Demosthenous, A., et al. (2017). Detection of the tau protein in human serum by a sensitive four-electrode electrochemical biosensor. Biosens. Bioelectron. 92, 482–488. doi: 10.1016/j.bios.2016.10.077
Wang, W.-Y., Tan, M., Yu, J.-T., and Tan, L. (2015). Role of pro-inflammatory cytokines released from microglia in Alzheimer' s disease. Ann. Transl. Med. 3, 1–15. doi: 10.3978/j.issn.2305-5839.2015.03.49
Williams-Gray, C. H., Wijeyekoon, R., Yarnall, A. J., Lawson, R. A., Breen, D. P., Evans, J. R., et al. (2016). Serum immune markers and disease progression in an incident Parkinson's disease cohort (ICICLE-PD). Mov. Disord. 31, 995–1003. doi: 10.1002/mds.26563
Xia, N., Liu, L., Harrington, M. G., Wang, J., and Zhou, F. (2010). Regenerable and simultaneous surface plasmon resonance detection of A beta(1-40) and A beta(1-42) peptides in cerebrospinal fluids with signal amplification by streptavidin conjugated to an n-terminus-specific antibody. Anal. Chem. 82, 10151–10157. doi: 10.1021/ac102257m
Xia, N., Zhou, B., Huang, N., Jiang, M., Zhang, J., and Liu, L. (2016). Visual and fluorescent assays for selective detection of beta-amyloid oligomers based on the inner filter effect of gold nanoparticles on the fluorescence of CdTe quantum dots. Biosens. Bioelectron. 85, 625–632. doi: 10.1016/j.bios.2016.05.066
Xing, Y., Feng, X. Z., Zhang, L., Hou, J., Han, G. C., and Chen, Z. (2017). A sensitive and selective electrochemical biosensor for the determination of beta-amyloid oligomer by inhibiting the peptide-triggered in situ assembly of silver nanoparticles. Int. J. Nanomedicine 12, 3171–3179. doi: 10.2147/IJN.S132776
Yadav, S. K., Mindur, J. E., Ito, K., and Dhib-Jalbut, S. (2015). Advances in the immunopathogenesis of multiple sclerosis. Curr. Opin. Neurol. 28, 206–219. doi: 10.1097/WCO.0000000000000205
Yang, Y., Gu, Y., Wan, B., Ren, X., and Guo, L. H. (2017). Label-free electrochemical biosensing of small-molecule inhibition on O-GlcNAc glycosylation. Biosens. Bioelectron. 95, 94–99. doi: 10.1016/j.bios.2017.04.009
Yildirim, A., and Bayindir, M. (2014). Turn-on fluorescent dopamine sensing based on in situ formation of visible light emitting polydopamine nanoparticles. Anal. Chem. 86, 5508–5512. doi: 10.1021/ac500771q
Yu, S., Liu, Y. P., Liu, Y. H., Jiao, S. S., Liu, L., Wang, Y. J., et al. (2016). Diagnostic utility of VEGF and soluble CD40L levels in serum of Alzheimer's patients. Clin. Chim. Acta 453, 154–159. doi: 10.1016/j.cca.2015.12.018
Yue, H. Y., Huang, S., Chang, J., Heo, C., Yao, F., Adhikari, S., et al. (2014). ZnO nanowire arrays on 3D hierachical graphene foam: Biomarker detection of parkinson's disease. ACS Nano 8, 1639–1646. doi: 10.1021/nn405961p
Yuzwa, S. A., and Vocadlo, D. J. (2014). O-GlcNAc and neurodegeneration: biochemical mechanisms and potential roles in Alzheimer's disease and beyond. Chem. Soc. Rev. 43, 6839–6858. doi: 10.1039/c4cs00038b
Zetterberg, H. (2017). Fluid biomarkers for microglial activation and axonal injury in multiple sclerosis. Acta Neurol. Scand. 136, 15–17. doi: 10.1111/ane.12845
Zhang, X., Zambrano, A., Lin, Z. T., Xing, Y., Rippy, J., and Wu, T. (2017). Immunosensors for biomarker detection in autoimmune diseases. Arch. Immunol. Ther. Exp. 65, 111–121. doi: 10.1007/s00005-016-0419-5
Zhang, Y., Zhang, B., Ye, X., Yan, Y., Huang, L., Jiang, Z., et al. (2016). Electrochemical immunosensor for interferon-γ based on disposable ITO detector and HRP-antibody-conjugated nano gold as signal tag. Mater. Sci. Eng. C 59, 577–584. doi: 10.1016/j.msec.2015.10.066
Keywords: neuroinflammation, biomarkers, Alzheimer's disease, Parkinson's disease, Multiple Sclerosis, biosensors, multiplex
Citation: Abreu CM, Soares-dos-Reis R, Melo PN, Relvas JB, Guimarães J, Sá MJ, Cruz AP and Mendes Pinto I (2018) Emerging Biosensing Technologies for Neuroinflammatory and Neurodegenerative Disease Diagnostics. Front. Mol. Neurosci. 11:164. doi: 10.3389/fnmol.2018.00164
Received: 22 February 2018; Accepted: 30 April 2018;
Published: 16 May 2018.
Edited by:
Nashat Abumaria, Fudan University, ChinaReviewed by:
Juan Pablo De Rivero Vaccari, University of Miami, United StatesMaciej Maurycy Lalowski, University of Helsinki, Finland
Copyright © 2018 Abreu, Soares-dos-Reis, Melo, Relvas, Guimarães, Sá, Cruz and Mendes Pinto. This is an open-access article distributed under the terms of the Creative Commons Attribution License (CC BY). The use, distribution or reproduction in other forums is permitted, provided the original author(s) and the copyright owner are credited and that the original publication in this journal is cited, in accordance with accepted academic practice. No use, distribution or reproduction is permitted which does not comply with these terms.
*Correspondence: Andrea P. Cruz, YW5kcmVhLmNydXpAaW5sLmludA==
Inês Mendes Pinto, aW5lcy5tLnBpbnRvQGlubC5pbnQ=
†These authors have contributed equally to this work.